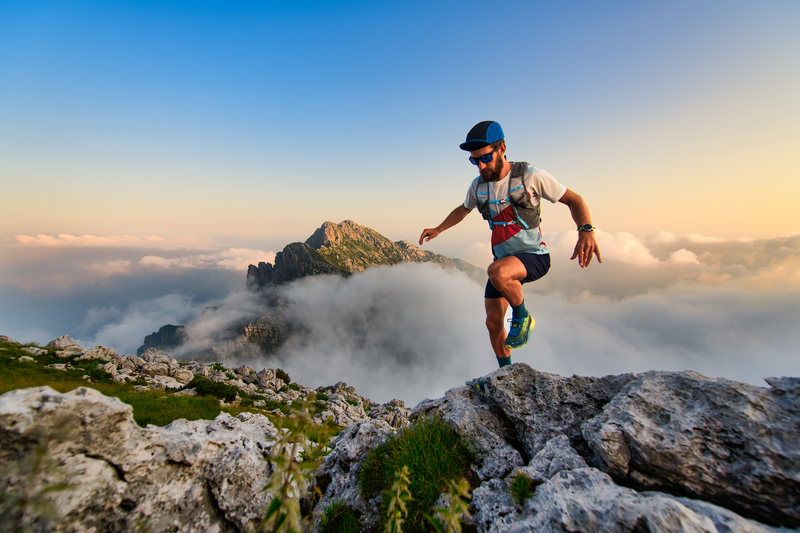
94% of researchers rate our articles as excellent or good
Learn more about the work of our research integrity team to safeguard the quality of each article we publish.
Find out more
ORIGINAL RESEARCH article
Front. Environ. Sci. , 17 June 2021
Sec. Environmental Informatics and Remote Sensing
Volume 9 - 2021 | https://doi.org/10.3389/fenvs.2021.647966
This article is part of the Research Topic Emerging Technologies and Techniques for Remote Sensing of Coastal and Inland Waters View all 14 articles
Ultraviolet (UV)-visible imaging spectroscopy is an emerging and highly anticipated technology, expected to improve the remote sensing of coastal waters and expand its range of applications. Upcoming NASA satellite missions including PACE and GLIMR will feature imaging spectrometers capable of measuring hyperspectral remote-sensing reflectance (Rrs) across the visible range and well into the near-infrared and ultraviolet domains. The availability of UV reflectance is expected to facilitate the remote sensing of chromophoric dissolved organic matter (CDOM) in optically complex waters, thereby improving coastal water-quality monitoring. Although this argument is well supported by the dominance of CDOM absorption in the UV domain, few studies have directly evaluated the potential advantages conferred by UV reflectance for monitoring CDOM-related coastal water quality. Here, we took advantage of a 6-week wastewater diversion event in Santa Monica Bay, California in 2015 and the availability of Portable Remote Imaging SpectroMeter (PRISM) imagery acquired during the diversion to assess if UV-visible imaging spectroscopy could facilitate the detection of CDOM and help differentiate wastewater effluent-derived CDOM from other sources. A comparison of local empirical algorithms with varying amounts of spectral information implemented on PRISM data showed that incorporating UV Rrs as a predictor significantly improved retrieval of CDOM absorption coefficients (ag). Optimal performance was reached when combining Rrs(365), Rrs(400), and Rrs(700) as predictors of ag in a multiple linear regression. The use of the entire UV-visible spectrum (365–700 nm) in a partial-least-squares regression (PLSR) did not improve retrievals, indicating that a few carefully chosen predictors in the UV-visible domain were sufficient to empirically differentiate CDOM from phytoplankton in coastal waters minimally influenced by sediments or bottom reflectance. Finally, the development of a new fluorescence-based indicator of effluent-derived CDOM (effluent fluorescence ratio, EFR) helped demonstrate the feasibility of remotely detecting CDOM from wastewater. A PLSR-based algorithm using Rrs
Urban coastal waters are productive environments that provide important ecosystem services to humans, including the dilution of terrestrial inputs (IOCCG, 2008; Rabalais et al., 2009; McLaughlin et al., 2017), fisheries and aquaculture, and various recreational and transportation services (Halpern et al., 2012; Caron et al., 2017; Gierach et al., 2017). The rapid expansion of urban centers around the world has dramatically increased the impact of human activities on land use, runoff, hydrodynamics, atmospheric deposition, and local climate at the land-ocean interface, and these can influence the water quality of the adjacent coastal waters (McKinney, 2002; Halpern et al., 2008; Halpern et al., 2012). Increased runoff from impervious surfaces (Ackerman and Weisberg, 2003; Bay et al., 2003; Dojiri et al., 2003) and pollution point sources (e.g., wastewater effluent) can lead to elevated concentrations of nutrients, organic matter, and contaminants in urban coastal waters and negatively impact water quality in this environment. These water quality impacts have serious consequences for the services these ecosystems provide and for human health in these densely populated areas.
Chromophoric dissolved organic matter (CDOM) is a major optical water-quality indicator that can be diagnostic of runoff and point sources of pollution in urban coastal waters (IOCCG, 2015; Fichot et al., 2016; Cao et al., 2018). CDOM is ubiquitous and naturally present in coastal waters, where it is not only produced in situ by biological processes, but is also strongly influenced by terrestrial sources (e.g., soils) through runoff (Hansell and Carlson, 2014). The spectral optical properties of CDOM (absorption and fluorescence) have therefore been used in various proxies of terrestrial runoff and/or as indicators of dissolved organic matter (DOM) source and degradation state in coastal waters (Vodacek et al., 1997; Hernes and Benner, 2003; Stedmon and Markager, 2003; Boyd and Osburn, 2004; Chen et al., 2004; Helms et al., 2008; Tzortziou et al., 2008; Fichot and Benner, 2012; Murphy et al., 2013; Yamashita et al., 2013). In urban waters, wastewater effluent represents another potentially significant source of CDOM with characteristic optical properties (Goldman et al., 2012; Devlin et al., 2015), which can be leveraged and used in optical proxies indicative of CDOM-related pollution.
CDOM is optically active and therefore has the advantage of being amenable to ocean-color remote sensing (Siegel et al., 2002; Mannino et al., 2008; Swan et al., 2013; Cao et al., 2018; Werdell et al., 2018). Ocean-color remote sensing can facilitate the monitoring of several optical water quality indicators (e.g., phytoplankton, turbidity, CDOM) over large areas and could enable the detection of CDOM-related pollution in urban coastal waters (IOCCG, 2015). However, it faces major challenges in these types of waters, where a combination of difficult atmospheric corrections and optical complexity of the waters can lead to large uncertainties and errors in the derived water-quality products (Aurin and Dierssen, 2012; Werdell et al., 2018). Coastal waters are generally optically complex, (IOCCG, 2000) because they are influenced by a combination of riverine and coastal-wetland inputs, upwelling of deep water, phytoplankton blooms, and in some cases urban wastewater effluent. This optical complexity often cannot be accurately resolved using existing multispectral sensors, which have constrained spectral ranges and resolutions (Aurin and Dierssen, 2012; Dekker et al., 2018). Considering that CDOM optical properties are most prominent in the ultraviolet (UV) and blue regions, these spectral limitations are particularly restrictive for detecting CDOM and distinguishing among different CDOM sources. UV observations are beyond the spectral ranges of many current ocean color sensors, and phytoplankton can interfere with or even dominate optical variability at blue wavelengths in more phytoplankton-dominated waters (Mobley et al., 2005; Zhu et al., 2011; Fichot et al., 2016; Werdell et al., 2018).
Recent advances in imaging spectroscopy (hyperspectral imagery) with broader and finer spectral capabilities than multispectral ocean-color sensors are expected to improve retrieval accuracy for in-water constituents in complex coastal waters from remote-sensing reflectance,
In this study, we evaluated the utility of UV-visible imaging spectroscopy for detecting CDOM accurately in urban coastal waters. We also tested the feasibility of remotely differentiating effluent-derived CDOM from other sources (e.g. terrestrial runoff). We specifically assessed the value of UV reflectance and of enhanced spectral resolution. This study took advantage of a 6-week wastewater diversion event and a related water-quality monitoring effort that took place in the urban waters of Santa Monica Bay (Southern California) in fall 2015 (City of Los Angeles, Environmental Monitoring Division, 2017; Trinh et al., 2017), and leveraged imagery from the NASA/JPL Portable Remote Imaging SpectroMeter (PRISM) airborne instrument (Mouroulis et al., 2014; Thompson et al., 2019). We used the PRISM data, as well as in-situ hyperspectral measurements of
Santa Monica Bay is a semi-enclosed coastal bay in the Southern California Bight that ranges from Malibu in the north to the Palos Verdes peninsula in the south (Figure 1). Santa Monica Bay is directly adjacent to the heavily urbanized greater Los Angeles area, which includes many densely populated coastal communities such as Santa Monica and Venice as well as the heavily trafficked Marina del Rey and Los Angeles International Airport. The bay provides valuable resources in the form of recreation, fisheries, transportation, wastewater disposal, water for industrial processes, and various other ecosystem services (Ackerman and Weisberg, 2003; Bay et al., 2003; Dojiri et al., 2003). The northern margin of Santa Monica Bay is dominated by the Santa Monica Mountains National Recreation Area, and has higher topographic relief and a sparser population (Bay et al., 2003) than the rest of the bay. Across much of the bay, water depth is less than 50 m but can reach depths greater than 500 m in submarine canyons that chisel through the bay (Figure 1).
FIGURE 1. Map of Santa Monica Bay with the sampling stations including the 1-mile outfall station (D9W, red triangle), the stepout stations surrounding the outfall (orange diamonds), and the other stations (yellow circles). The 1-mile and 5-mile outfall pipes extending from the Hyperion Wastewater Treatment Plant are represented by black lines.
This urban coastal-water system consists of heterogeneous, dynamic and optically complex waters influenced by marine currents, precipitation, and point sources of nutrients (Howard et al., 2014; Howard et al., 2017) or pollution (Trinh et al., 2017). Relative to the nearby open ocean, productivity in Santa Monica Bay is high, stimulated by nutrient inputs from spring upwelling events, as well as point sources of nutrients within the bay (Bray et al., 1999). Nutrient availability and currents in the bay are also influenced by the introduction of cold waters from the California Current System, intensified by seasonal coastal upwelling (Caron et al., 2017; Trinh et al., 2017). Local currents within Santa Monica Bay are highly variable, influenced by interactions of wind and strong temperature gradients with regional currents in the Southern California Bight (Washburn et al., 2003; Caron et al., 2017; City of Los Angeles, Environmental Monitoring Division, 2017).
Santa Monica Bay receives less runoff than most river-influenced coastal margins due to the dry local climate of Southern California, with salinity in the bay typically varying over a relatively narrow range (33 ± 2 PSU) outside of terrigenous freshwater plumes (Tiefenthaler et al., 2000). Santa Monica Bay receives low riverine inputs relative to other coastal areas. Low precipitation conditions are punctuated by intermittent, although often intense, rain events (Bay et al., 2003; Dojiri et al., 2003). Runoff reaches the bay through several highly engineered, concrete-hardened urban creeks in greater Los Angeles (Ballona Creek and Santa Monica Creek) and through the more natural creek and river systems that drain the Santa Monica Mountains to the north of the bay (including Malibu Creek and Topanga Creek (Ackerman and Weisberg, 2003; Bay et al., 2003; Dojiri et al., 2003). These intermittent runoff events have been shown to substantially influence coastal water quality and biogeochemistry in the bay (City of Los Angeles, Environmental Monitoring Division, 2017).
The Hyperion Wastewater Reclamation Plant is the largest wastewater treatment facility in Los Angeles. It released approximately 230 million gallons of secondary-treated wastewater effluent into Santa Monica Bay daily in 2015 (City of Los Angeles, Environmental Monitoring Division, 2017). This effluent is treated with physically, chemically, and bacterially mediated processes to remove sediments and organo-solids. During normal operations, effluent is released through a 5-mile offshore outfall in waters that are more than 60 m deep (Lyon and Sutula, 2011; City of Los Angeles, Environmental Monitoring Division, 2017; Gierach et al., 2017; Trinh et al., 2017). In fall 2015, during scheduled maintenance lasting 6 weeks (September 21, 2015-November 2, 2015), secondary-treated effluent from Hyperion was released at an older 1-mile offshore outfall in waters less than 20 m deep (City of Los Angeles, Environmental Monitoring Division, 2017). High surface concentrations of organic matter, coliform bacteria, and algal blooms were associated with the effluent plume and impacted surface water quality. Preemptive closure of nearby beaches was therefore necessary at times during the diversion (City of Los Angeles, Environmental Monitoring Division, 2017). The water quality monitoring efforts described in this paper, including in-situ sampling, were led by the City of Los Angeles Environmental Monitoring Division (CLAEMD). Field measurements during the water quality monitoring efforts were aided by the collection of PRISM airborne imagery.
Eighty-three surface water samples were collected before, during, and after the wastewater diversion (sampling from September 16-November 11, 2015) aboard the R/V La Mer and R/V Marine Surveyor along a zig-zagging pattern of stations oriented northwest-southeast in Santa Monica Bay (Figure 1 and Table 1). One station was located directly above the 1-mile Hyperion effluent outfall (Station D9W), and four more stations were positioned at “stepout” locations approximately 750 m north, south, east, and west of the outfall (Figure 1). The dispersion of the effluent from the outfall during the diversion was driven by complex and variable current patterns in Santa Monica Bay during the diversion (City of Los Angeles, Environmental Monitoring Division, 2017). As a result, some stepout stations were heavily influenced by effluent whereas others were minimally impacted. Salinity was measured at 1-m depth using an SBE 19-plus Conductivity-Temperature-Depth (CTD) rosette (Seabird Scientific®) equipped with 1.7 L Niskin bottles. Two WETLabs® WETStar single-channel fluorescence sensors were also mounted in the CTD rosette and provided simultaneous measurements of chlorophyll-a fluorescence (460 nm excitation and 695 nm emission) and DOM fluorescence (370 nm excitation and 460 nm emission). Surface water samples were collected at 1 m depth using the Niskin bottles of the CTD rosette. Samples were gravity filtered through 0.7 µm filters (GF/F glass-fiber filters) directly from the Niskin bottles into clean borosilicate EPA clear glass vials (acid washed with 10%-HCl and furnaced at 450°C for 4 h) and placed immediately at 4°C in the dark until analysis in the laboratory. Glass-fiber filters with a 0.7 µm effective pore size were used in this study as a clean filtration method that allowed samples to be rapidly filtered between sample collections. Differences between filtration through 0.7 and 0.2 µm filters are likely to be small for the relatively low particle concentrations measured in Santa Monica Bay (Laanen et al., 2011). Scattering effects from any remaining sub-micron-scale particles after 0.7 µm filtration are expected to be further mitigated by the spectral fitting routine applied during laboratory analysis for CDOM absorption (Zhu et al., 2020; described below).
TABLE 1. Summary of in-situ variables. Ranges of observed environmental parameters are reported for each sampling day. Gray rows denote sampling conducted during the wastewater effluent diversion.
In-situ radiometric measurements were collected nearly simultaneously with sample collection at 45 stations using a Satlantic Inc. HyperPro free-falling optical profiling system (Seabird Scientific). The HyperPro system is composed of three, hyperspectral Satlantic Hyper Ocean Color Radiometers (HyperOCR): two HyperOCR mounted on the profiler to measure underwater downwelling irradiance
Absorption-coefficient spectra of CDOM,
FIGURE 2. Map of in-situ ag(365) measured during the field campaign in the fall 2015. Pre-diversion samples were measured on September 16 after a heavy precipitation event on September 15 (>6 cm of rain) and exhibited higher CDOM content at the north end of the bay. During the wastewater diversion, the highest ag(365) were observed near the 1-mile outfall. After the end of the wastewater diversion, there was little precipitation, and ag(365) was low at all sampling stations across Santa Monica Bay.
An exponential fit of the absorbance spectrum from 500 to 700-nm was used to compute an offset value that was subtracted from the entire absorbance spectrum (Fichot and Benner, 2011; Zhu et al., 2020). Offset-corrected spectral absorbances were converted to Napierian CDOM absorption coefficients, [m−1]. This offset correction is well suited to coastal waters, because it does not assume that absorption coefficients in the 680–700 nm are negligible as is often done in procedures used to correct CDOM absorbance for open ocean waters (Johannessen et al., 2003).
Seventy-six filtered (0.7 µm) surface water samples and the five effluent-dilution samples were analyzed for excitation and emission matrix (EEM) fluorescence using a Photon Technology International PTI 814 spectrofluorometer with a 1 cm quartz cuvette (Walker et al., 2009). Excitation was performed in 5 nm increments from 240 to 450 nm, and emission was measured in 2 nm increments from 300 to 600 nm. During the measurement, the emission signal was normalized to a reference detector to remove fluctuations of the light source. The raw EEM spectra were exported to MATLAB and processed with the drEEM toolbox (Murphy et al., 2013) to correct for the inner filter effect and to apply a Raman calibration. Finally, a Raman-normalized excitation and emission spectrum of Milli-Q water (18.2 MΩ cm) was subtracted from sample EEM spectra to remove the Raman signal. EEM fluorescence was not measured on September 24 and at two stations on October 21 because of low sample volume.
Dissolved organic carbon (DOC) was measured by high-temperature combustion using a Shimadzu TOC-V analyzer equipped with an autosampler (Fichot and Benner, 2011). Samples were gravity filtered using pre-combusted glass fiber filters (0.7 µm pore size), acidified with 2 mol L−1 HCl, and stored at 4°C in borosilicate glass bottles until analysis within a few days of sampling. DOC concentration in the blanks were negligible, and accuracy and consistency of measured DOC concentrations was checked by measuring a deep seawater reference standard (University of Miami) every sixth sample.
A sample of undiluted wastewater effluent was provided by the Hyperion Wastewater Reclamation Plant for our use in a dilution experiment. In order to simulate the effects of effluent-derived DOM on the absorption and fluorescence characteristics of the water, the effluent sample was diluted in a surface seawater sample obtained in offshore Santa Monica Bay after the diversion. Specifically, the pure effluent sample (100%) was diluted to generate seawater solutions containing 5, 1, 0.2, and 0% (no addition) of effluent by volume. Each sample was then analyzed for CDOM absorption spectra and EEM fluorescence as described above.
We developed local empirical algorithms for the remote retrieval of CDOM absorption coefficient from UV-visible
UV-red and blue-red band-ratio algorithms were compared to assess the utility of including UV reflectance in simple empirical algorithms to facilitate the retrieval of accurate
1) univariate regression on a blue-red reflectance band ratio
2) univariate regression on a UV-red reflectance band ratio
3) visible multiple linear regression on
4) UV-visible multiple linear regression on
5) UV-visible partial least squares regression on full-spectrum
Empirical algorithms for deriving bio-optical properties from
We also tested empirical algorithms for inferring CDOM using partial least squares regression. PLSR is a statistical technique for collapsing many highly correlated explanatory variables into a smaller number of uncorrelated predictors ranked in order of explanatory power (Mevik and Wehrens, 2007). Linear combinations of predictors are used to create components that have maximized correlation with the response variable. To predict bio-optical parameters, PLSR was implemented using the MATLAB plsregress function from the Statistics and Machine Learning Toolbox™. PLSR models were tested for both log-transformed and linear Rrs and log-transformed and linear bio-optical properties.
PLSR was tested for overfitting using a “leave-p-out” cross-validation routine with p = 4. Four data points, representing approximately 10% of the available data, was selected as an appropriate subset for validation in order to balance bias and variance in cross-validation (Arlot and Celisse, 2010). During “leave-four-out” cross-validation, PLSR coefficients were calibrated using the remaining n−4 data points, and the resulting empirical algorithm was validated using the four points that had been held out. Error statistics (root mean squared error (RMSE), mean absolute error (MAE), mean absolute percent error (MAPE), and R2) were calculated between the fitted and measured parameter of interest on the four points that were held out. This process was repeated for all possible subsets of four stations (40 choose four combinations for predicting EEM fluorescence peak ratio and 45 choose four combinations for fits on
Full error statistics for empirical algorithm calibration are presented in Table 2. The performance of these empirical algorithms was also compared to QAA retrievals to assess the performance of locally calibrated empirical algorithms against a well-established semi-analytical approach. The same procedure was used to calibrate empirical algorithms for
TABLE 2. Summary statistics associated with the performance comparison of the six ag(443) algorithms (see also Figure 8). Statistics include root mean squared error (RMSE), mean average percent error (MAPE), mean absolute error (MAE), and R-squared for each algorithm.
We also assessed the feasibility of remotely detecting CDOM source information, specifically inferring a fluorescence proxy for the degree of effluent impact. From in-situ stations where both
PRISM imagery was collected during a flyover on October 26, 2015. The instrument, mounted on an ER-2 aircraft flying at an approximate altitude of 20 km, captured a swath from northwest to southeast along the coastline of Santa Monica Bay. Atmospheric correction was conducted using an Optimal Estimation formulation that simultaneously models surface and atmospheric reflectance contributions from statistical priors (Thompson et al., 2018). The atmospheric correction approach, including the calibration and orthorectification of the PRISM imagery were described at length in a recent manuscript (Thompson et al., 2019). The spatial resolution of the image is approximately 20 m, and includes spectral measurements made approximately every 3 nm from 350 to 1,050 nm. This high spatial resolution provides opportunities for detecting patterns in water quality at small scales, but also introduces additional challenges for interpreting data in some areas. For example, several boats are visible in maps of
Statistical analyses were conducted in R (R Core Team, 2018) and MATLAB version R2018b. Figures were produced in R using the fields package, and in MATLAB. Multiple linear regression and partial least squares regression were implemented using MATLAB version R2018b.
The spatial distribution of CDOM absorption in Santa Monica Bay was very variable over the course of the fall sampling, as local inputs from riverine and wastewater effluent sources were added to the bay (Figure 2). On September 16, following a major rain event before the diversion (>6 cm of rain fell on September 15 in the wettest September storm in more than 100 years), higher
The relationships between CDOM, chlorophyll-a, and salinity in this data set were complex and variable, and were consistent with variable sourcing of in-water constituents (Figure 3). Overall, these urban coastal waters exhibited general correlations between chlorophyll-a,
FIGURE 3. Comparison of in-situ environmental parameters measured during the field campaign: (A)ag(365) versus chlorophyll-a fluorescence with color scale indicative of salinity, (B) salinity versus ag(365) with color scale indicative of chlorophyll-a fluorescence, and (C) salinity versus chlorophyll-a fluorescence with color scale indicative of ag(365). In situ chlorophyll-a fluorescence was not measured at all stations (black symbols in panel B do not have chlorophyll-a data).
Overall, the variability of the CDOM absorption coefficient spectra adhered to expected relationships, but did not enable the identification of different CDOM sources. The absorption spectra followed the typical exponential spectral shape for CDOM (Supplementary Figure S3), and
FIGURE 4. Plots of CDOM absorption characteristics: (A) relationship between absorption coefficients of CDOM at 365 and 443 nm, (B) relationship between ag(365) and [DOC], (C) spectral slope in the UV-B (S275–295) versus UV-A to visible (S365–443) domain, (D) relationship between ag(365) and S275–295, (E) relationship between ag(365) and S365–443, and (F) relationship between S275–295 and [DOC]-specific absorption by CDOM (
Spectral slopes of CDOM absorption coefficient also did not offer opportunities to differentiate effluent-derived CDOM from other sources. Absorption spectral slopes between 275 and 295 nm (
Samples collected near the effluent outfall during the diversion exhibited high
In contrast to CDOM absorption, EEM fluorescence proved useful for separating effluent-derived CDOM from other sources. Samples collected around the 1-mile outfall during the diversion exhibited a distinct fluorescence peak (
FIGURE 5. CDOM fluorescence excitation emission matrices (EEMs): (A) EEM of a pre-diversion sample with high ag(365) associated with terrigenous runoff that fluoresces strongly at the runoff-associated peak
Samples collected at or near the wastewater effluent outfall during the diversion fluoresced more intensely at the effluent peak (higher FE values) than other samples with similar
FIGURE 6. Relationships between: (A) intensity of the effluent-associated fluorescence peak
The dependence of FE and FR on CDOM source provided an opportunity to distinguish CDOM from effluent-influenced samples. Here, we propose EFR,
as an optical indicator of effluent-impacted waters, where
The effluent dilution experiment clearly revealed the dependence of the EFR on the fraction of wastewater effluent diluted in a local seawater sample (Figure 6C). EFR values increased asymptotically with the fraction of effluent from a value of approximately 0.75 for 0% effluent to almost 2 for 5% effluent. The increased utility of EFR over
The spatial distribution of EFR values in Santa Monica Bay during the sampling window (Figure 7) was very consistent with the modeled dispersion of the effluent (Supplementary Figure S4). No EFR higher than 0.8 were detected before or after the diversion, and the highest EFR values detected during the diversion (>1.5) were at the wastewater outfall. Higher EFR values (>1.0) also occurred in the area surrounding the outfall (stepout stations). Samples with increased EFR generally occurred near the path of effluent plume, as inferred in particle trajectory modeling (Supplementary Figure S4) conducted by the Southern California Coastal Observatory System based high frequency radar observations of local currents in Santa Monica Bay (City of Los Angeles, Environmental Monitoring Division, 2017).
FIGURE 7. Distribution of in-situ EFR in Santa Monica Bay during the sampling window. No EEM analysis was conducted for September 24. EFR values >1.0 were only detected near the outfall during the diversion. Data from September 16 (after major rain event) show that the EFR is largely insensitive to runoff.
The effluent fluorescent peak FE is a well-positioned fluorescence feature in CDOM because it has the potential to influence remote-sensing reflectance. This fluorescence is particularly intense, meaning it can produce a significant radiance. Furthermore, unlike most other CDOM fluorescence peaks which require excitation at wavelengths shorter than 300 nm, the FE peak is excited by UV radiation (∼350 nm) that is naturally present in underwater solar irradiance (Fichot and Miller, 2010). It also emits broadly in the blue part of the spectrum (∼440 nm). Phytoplankton also absorb strongly in this wavelength range creating potential for fluorescence reabsorption and possibly introducing further challenges for remote detection. However, past studies (Hoge et al., 1993; Green and Blough, 1994; Lee et al., 1994), have included remote detection of CDOM fluorescence features despite interference from phytoplankton absorption, indicating that some changes in CDOM fluorescence are remotely detectable. Therefore, we expect that the sun-induced fluorescence of effluent-derived CDOM could drive detectable changes in remote-sensing reflectance, allowing for the fully remote detection of wastewater effluent based on its optical characteristics. As an optical proxy based in part on
We compared the performance of empirical algorithms developed for
FIGURE 8. Performance comparison of six different ag(443) algorithms. In-situ data used for calibration (n = 41) are displayed as blue circles, and end-to-end validation points comparing measured ag(443) to inferred ag(443) from nearest neighbor PRISM pixels (n = 4) are displayed as orange diamonds. Most of the ag(443) algorithms presented here, with the exception of the QAA, are empirical and were developed using coincident in-situ measurements of Rrs and ag(443): (A) log-log fit on blue-red band ratio Rrs(443)/Rrs(665), (B) log-log fit on UV-red band ratio Rrs(365)/Rrs(665), (C) the QAA algorithm v.6, which exhibited one major outlier and is plotted without it to facilitate comparison (inset plot includes outlier), (D) log-log visible-only multiple linear regression (MLR) on Rrs(412), Rrs(443), Rrs(700); (E) log-log UV-visible MLR on Rrs(365), Rrs(400), Rrs(700), and (F) a log-log partial-least-square regression (PLSR) on the full spectrum Rrs(365–700), using the first five components. The sample collected at station D9W on October 14 was an outlier, with low inferred ag(443) relative to measured ag(443) for all algorithms. This sample is indicated with a dashed circle.
To compare empirical CDOM absorption retrieval to an established semi-analytical method, we included
Across all of the algorithms tested, the sample collected at D9W (wastewater outfall) on October 14 was an outlier, with inferred
The CDOM algorithms were implemented on the PRISM remote-sensing reflectance (Figure 9) to produce maps of CDOM at 20 m resolution in Santa Monica Bay for October 26. Mapped
FIGURE 9. Remote-sensing reflectance spectra,
The spatial distributions and inferred ranges of
FIGURE 10. Maps of
We also conducted an end-to-end validation (Figure 8 and Supplementary Figure S8) for which
Algorithms for retrieving EFR were developed using a five-component PLSR on
FIGURE 11. Performance of an empirical PLSR-based algorithm for Effluent Fluorescence Ratio (EFR) and its implementation on the PRISM
TABLE 3. Summary statistics associated with the performance comparison of the two EFR algorithms (see also Figure 11 and Supplementary Figure S10). Statistics include root mean squared error (RMSE), mean average percent error (MAPE), mean absolute error (MAE), and R-squared for each algorithm.
The EFR algorithm was applied to the PRISM imagery and generated maps of EFR at 20 m resolution across Santa Monica Bay. The range of remotely sensed EFR values matched that of the in-situ data. The map of EFR derived from the PLSR algorithm correctly detected the highest EFR at the wastewater effluent outfall (EFR between 0.85 and 1.25) and low EFR (<0.85) across the rest of Santa Monica Bay. In this respect, the PLSR algorithm appeared to successfully infer the wastewater effluent-influenced plume of DOM at the outfall, while minimizing sensitivity to CDOM from runoff and other sources. The MLR algorithm did not detect a similar maximum at the outfall (Supplementary Figure S10), and retrieved high EFR at other locations with high CDOM. Fitting and mapping of the MLR algorithm suggested that the small number of spectral
Incorporating UV reflectance in empirical band-ratio algorithms offered only minor improvements to the accuracy of CDOM retrievals in Santa Monica Bay. A single blue-red band-ratio algorithm did not perform well in these waters and produced a spatial distribution of CDOM that closely resembled the distribution of chlorophyll-a (Trinh et al., 2017). Surprisingly, replacing the blue band (443 nm) with a UV band (365 nm), a domain where CDOM has a stronger influence, only slightly improved the accuracy of CDOM retrievals. The average percent error of
However, the inclusion of both UV and blue reflectance in a simple multi-band regression algorithm (MLR-based) significantly improved
The inclusion of additional spectral bands did not improve the performance of the empirical
Although the three-band approach optimally retrieved
The EFR, a new fluorescence-based water quality indicator that we introduced in this study, facilitated the differentiation of effluent-derived CDOM from other sources of CDOM in coastal waters. The fact that all effluent-impacted waters in Santa Monica Bay had high CDOM, but not all high-CDOM waters were effluent-impacted presented challenges for prescriptively identifying effluent. Here, the EFR leveraged the enhanced fluorescence of effluent-impacted samples at the
Here, we tested the feasibility of retrieving EFR empirically from remote sensing. In contrast to other CDOM fluorescence peaks in EEM spectra, the
The presence of effluent in surface waters was only detected at relatively high concentrations of effluent. CDOM fluorescence is a relatively weak IOP, and its small influence relative to backscattering or absorption is likely responsible for the challenge of detecting effluent at low concentrations. The re-absorption of emitted fluorescence by effluent-CDOM and other absorbing constituents further decreased the influence of fluorescence signatures on ocean color. Due to the resulting small impact of fluorescence on
This feasibility study identified purely empirical relationships and did not explore the mechanistic relationship between effluent-impacted CDOM fluorescence and
This study revealed that some improvements to CDOM-related coastal water quality monitoring can be made possible through the use of UV-visible imaging spectroscopy, an area where visible multispectral approaches have had limited success (IOCCG, 2000; Aurin and Dierssen, 2012; IOCCG, 2015; Dekker et al., 2018). The upcoming PACE and GLIMR missions will include UV-visible imaging spectrometers with comparable specifications as PRISM and are therefore expected to provide such improvements in coastal water quality monitoring. Here, the inclusion of UV reflectance (using simple-to-implement empirical algorithms) not only facilitated the accurate detection of CDOM in urban coastal waters, but also enabled its sourcing. This study also showed that high spectral resolution was essential to remotely differentiating effluent-derived CDOM. Stepping beyond the usual retrieval of
The extent to which water-quality monitoring capabilities will be enhanced by UV-visible imaging spectroscopy will be contingent on our ability to apply accurate atmospheric corrections and on the availability of high-quality in‐situ validation data (IOCCG, 2012). The extended spectral range, enhanced spectral resolution, and improved signal-to-noise ratio of imaging spectrometers in upcoming missions (e.g., PACE and GLIMR) should facilitate the detection of CDOM and its subtler IOPs like effluent-CDOM fluorescence, but can only do so if accurate
The raw data supporting the conclusion of this article will be made available by the authors, without undue reservation.
JH and CF directed the study. CF led the project planning, conducted field data collection, processed in-situ radiometry, and contributed to data analysis and writing. JH led the data analysis and writing of the manuscript. KK conducted the CDOM, EEM, and DOC analyses on the samples. MG provided support for PRISM imagery collection and participated in the field data acquisition. DT conducted the atmospheric correction of the PRISM imagery. CC provided field campaign leadership and support. All authors commented and edited the manuscript.
This research was supported by a NASA Water Quality grant 80NSSC18K0344 to CF, and NASA FINESST grant 80NSSC20K1648 to graduate student JH (PI CF). Part of this research was performed at the Jet Propulsion Laboratory, California Institute of Technology, under a contract with the National Aeronautics and Space Administration (80NM0018D0004). Copyright 2020, All Rights Reserved.
The authors declare that the research was conducted in the absence of any commercial or financial relationships that could be construed as a potential conflict of interest.
We thank the crew of the R/V La Mer and R/V Marine Surveyor, as well as the employees of the City of Los Angeles who participated in the sampling. We also thank Rebecca Trinh for her assistance in collecting radiometry data, and Jesus Mariano Duran and Frazier Peluso who helped with the analysis of CDOM samples.
The Supplementary Material for this article can be found online at: https://www.frontiersin.org/articles/10.3389/fenvs.2021.647966/full#supplementary-material
Ackerman, D., and Weisberg, S. B. (2003). Relationship Between Rainfall and Beach Bacterial Concentrations on Santa Monica Bay Beaches. J. Water Health 1, 85–89. doi:10.2166/wh.2003.0010
Arlot, S., and Celisse, A. (2010). A Survey of Cross-Validation Procedures for Model Selection. Statist. Surv. 4, 40–79. doi:10.1214/09-SS054
Aurin, D. A., and Dierssen, H. M. (2012). Advantages and Limitations of Ocean Color Remote Sensing in CDOM-Dominated, Mineral-Rich Coastal and Estuarine Waters. Remote Sens. Environ. 125, 181–197. doi:10.1016/j.rse.2012.07.001
Bauer, J. E., Cai, W.-J., Raymond, P. A., Bianchi, T. S., Hopkinson, C. S., and Regnier, P. A. G. (2013). The Changing Carbon Cycle of the Coastal Ocean. Nature 504, 61–70. doi:10.1038/nature12857
Bay, S., Jones, B. H., Schiff, K., and Washburn, L. (2003). Water Quality Impacts of Stormwater Discharges to Santa Monica Bay. Mar. Environ. Res. 56, 205–223. doi:10.1016/S0141-1136(02)00331-8
Bowers, D. G., and Brett, H. L. (2008). The Relationship Between CDOM and Salinity in Estuaries: An Analytical and Graphical Solution. J. Mar. Syst. 73, 1–7. doi:10.1016/j.jmarsys.2007.07.001
Bray, N. A., Keyes, A., and Morawitz, W. M. L. (1999). The California Current System in the Southern California Bight and the Santa Barbara Channel. J. Geophys. Res. 104, 7695–7714. doi:10.1029/1998jc900038
Brezonik, P. L., Olmanson, L. G., Finlay, J. C., and Bauer, M. E. (2015). Factors Affecting the Measurement of CDOM by Remote Sensing of Optically Complex Inland Waters. Remote Sens. Environ. 157, 199–215. doi:10.1016/j.rse.2014.04.033
Bricaud, A., Babin, M., Morel, A., and Claustre, H. (1995). Variability in the Chlorophyll-specific Absorption Coefficients of Natural Phytoplankton: Analysis and Parameterization. J. Geophys. Res. 100, 13321–13332. doi:10.1029/95jc00463
Boyd, T. J., and Osburn, C. L. (2004). Changes in CDOM Fluorescence From Allochthonous and Autochthonous Sources During Tidal Mixing and Bacterial Degradation in Two Coastal Estuaries. Mar. Chem. 89, 189–210. doi:10.1016/j.marchem.2004.02.012
Cai, W.-J. (2011). Estuarine and Coastal Ocean Carbon Paradox: CO2 Sinks or Sites of Terrestrial Carbon Incineration?. Annu. Rev. Mar. Sci. 3, 123–145. doi:10.1146/annurev-marine-120709-142723
Cao, F., Tzortziou, M., Hu, C., Mannino, A., Fichot, C. G., del Vecchio, R., et al. (2018). Remote Sensing Retrievals of Colored Dissolved Organic Matter and Dissolved Organic Carbon Dynamics in North American Estuaries and Their Margins. Remote Sens. Environ. 205, 151–165. doi:10.1016/j.rse.2017.11.014
Caron, D. A., Gellene, A. G., Smith, J., Seubert, E. L., Campbell, V., Sukhatme, G. S., et al. (2017). Response of Phytoplankton and Bacterial Biomass During a Wastewater Effluent Diversion Into Nearshore Coastal Waters. Estuar. Coast. Shelf Sci. 186, 223–236. doi:10.1016/j.ecss.2015.09.013
Carstea, E. M., Bridgeman, J., Baker, A., and Reynolds, D. M. (2016). Fluorescence Spectroscopy for Wastewater Monitoring: A Review. Water Res. 95, 205–219. doi:10.1016/j.watres.2016.03.021
Chen, R. F., Bissett, P., Coble, P., Conmy, R., Gardner, G. B., Moran, M. A., et al. (2004). Chromophoric Dissolved Organic Matter (CDOM) Source Characterization in the Louisiana Bight. Mar. Chem. 89, 257–272. doi:10.1016/j.marchem.2004.03.017
City of Los Angeles, Environmental Monitoring Division (2017). Fall 2015 Hyperion Treatment Plant Effluent Diversion to the 1-Mile Outfall Comprehensive Monitoring Program Final Report. Los Angeles: Environmental Monitoring Division, Bureau of Sanitation, Department of Public Works, City of Los Angeles.
Dekker, A. G., Phinn, S. R., Anstee, J., Bissett, P., Brando, V. E., Casey, B., et al. (2011). Intercomparison of Shallow Water Bathymetry, Hydro-Optics, and Benthos Mapping Techniques in Australian and Caribbean Coastal Environments. Limnol. Oceanogr. Methods 9, 396–425. doi:10.4319/lom.2011.9.396
Dekker, A. G., Pinnel, N., Gege, P., Briottet, X., Court, A., Peters, S., et al. (2018). Feasibility Study for an Aquatic Ecosystem Earth Observing System. Committee on Earth Observation Satellites. Available online: hal-02172188. (Accessed December 14, 2020).
del Vecchio, R., and Blough, N. v. (2004). Spatial and Seasonal Distribution of Chromophoric Dissolved Organic Matter and Dissolved Organic Carbon in the Middle Atlantic Bight. Mar. Chem. 89, 169–187. doi:10.1016/j.marchem.2004.02.027
Devlin, M., Petus, C., da Silva, E., Tracey, D., Wolff, N., Waterhouse, J., et al. (2015). Water Quality and River Plume Monitoring in the Great Barrier Reef: An Overview of Methods Based on Ocean Colour Satellite Data. Remote Sens. 7, 12909–12941. doi:10.3390/rs71012909
Dojiri, M., Yamaguchi, M., Weisberg, S. B., and Lee, H. J. (2003). Changing Anthropogenic Influence on the Santa Monica Bay Watershed. Mar. Environ. Res. 56, 1–14. doi:10.1016/S0141-1136(03)00003-5
Ferrari, G. M., Dowell, M. D., Grossi, S., and Targa, C. (1996). Relationship Between the Optical Properties of Chromophoric Dissolved Organic Matter and Total Concentration of Dissolved Organic Carbon in the Southern Baltic Sea Region. Mar. Chem. 55, 299–316. doi:10.1016/S0304-4203(96)00061-8
Fichot, C. G., and Benner, R. (2011). A Novel Method to Estimate DOC Concentrations from CDOM Absorption Coefficients in Coastal Waters. Geophys. Res. Lett. 38, L03610. doi:10.1029/2010GL046152
Fichot, C. G., and Benner, R. (2014). The Fate of Terrigenous Dissolved Organic Carbon in a River-Influenced Ocean Margin. Glob. Biogeochem. Cycles 28, 300–318. doi:10.1002/2013GB004670
Fichot, C. G., and Benner, R. (2012). The Spectral Slope Coefficient of Chromophoric Dissolved Organic Matter (S 275-295 ) as a Tracer of Terrigenous Dissolved Organic Carbon in River-Influenced Ocean Margins. Limnol. Oceanogr. 57, 1453–1466. doi:10.4319/lo.2012.57.5.1453
Fichot, C. G., Downing, B. D., Bergamaschi, B. A., Windham-Myers, L., Marvin-Dipasquale, M., Thompson, D. R., et al. (2016). High-Resolution Remote Sensing of Water Quality in the San Francisco Bay-Delta Estuary. Environ. Sci. Technol. 50, 573–583. doi:10.1021/acs.est.5b03518
Fichot, C. G., and Miller, W. L. (2010). An Approach to Quantify Depth-Resolved marine Photochemical Fluxes Using Remote Sensing: Application to Carbon Monoxide (CO) Photoproduction. Remote Sens. Environ. 114, 1363–1377. doi:10.1016/j.rse.2010.01.019
Gierach, M. M., Holt, B., Trinh, R., Jack Pan, B., and Rains, C. (2017). Satellite Detection of Wastewater Diversion Plumes in Southern California. Estuar. Coast. Shelf Sci. 186, 171–182. doi:10.1016/j.ecss.2016.10.012
Goldman, J. H., Rounds, S. A., and Needoba, J. A. (2012). Applications of Fluorescence Spectroscopy for Predicting Percent Wastewater in an Urban Stream. Environ. Sci. Technol. 46, 4374–4381. doi:10.1021/es2041114
Green, S. A., and Blough, N. V. (1994). Optical Absorption and Fluorescence Properties of Chromophoric Dissolved Organic Matter in Natural Waters. Limnol. Oceanogr. 39, 1903–1916. doi:10.4319/lo.1994.39.8.1903
Halpern, B. S., Longo, C., Hardy, D., McLeod, K. L., Samhouri, J. F., Katona, S. K., et al. (2012). An index to Assess the Health and Benefits of the Global Ocean. Nature 488, 615–620. doi:10.1038/nature11397
Halpern, B. S., Walbridge, S., Selkoe, K. A., Kappel, C. v., Micheli, F., D’Agrosa, C., et al. (2008). A Global Map of Human Impact on marine Ecosystems. Science 319, 948–952. doi:10.1126/science.1149345
Hansell, D. A., and Carlson, C. A. (2014). Biogeochemistry of Marine Dissolved Organic Matter. 2nd Edn. San Diego, CA: Elsevier.
Helms, J. R., Stubbins, A., Ritchie, J. D., Minor, E. C., Kieber, D. J., and Mopper, K. (2008). Absorption Spectral Slopes and Slope Ratios as Indicators of Molecular Weight, Source, and Photobleaching of Chromophoric Dissolved Organic Matter. Limnol. Oceanogr. 53, 955–969. doi:10.4319/lo.2008.53.3.0955
Hernes, P. J., and Benner, R. (2003). Photochemical and Microbial Degradation of Dissolved Lignin Phenols: Implications for the Fate of Terrigenous Dissolved Organic Matter in marine Environments. J. Geophys. Res. 108, 7-1–7-9. doi:10.1029/2002jc001421
Hoge, F. E., Vodacek, A., and Blough, N. v. (1993). Inherent Optical Properties of the Ocean: Retrieval of the Absorption Coefficient of Chromophoric Dissolved Organic Matter From Fluorescence Measurements. Limnol. Oceanogr. 38, 1394–1402. doi:10.4319/lo.1993.38.7.1394
Howard, M. D. A., Kudela, R. M., and McLaughlin, K. (2017). New Insights Into Impacts of Anthropogenic Nutrients on Urban Ecosystem Processes on the Southern California Coastal Shelf: Introduction and Synthesis. Estuar. Coast. Shelf Sci. 186, 163–170. doi:10.1016/j.ecss.2016.06.028
Howard, M. D. A., Sutula, M., Caron, D. A., Chao, Y., Farrara, J. D., Frenzel, H., et al. (2014). Anthropogenic Nutrient Sources Rival Natural Sources on Small Scales in the Coastal Waters of the Southern California Bight. Limnol. Oceanogr. 59, 285–297. doi:10.4319/lo.2014.59.1.0285
IOCCG (2000). “Remote Sensing of Ocean Colour in Coastal and Other Optically-Complex Waters,” in Reports of the International Ocean-Colour Coordinating Group, No. 3. Editor S. Sathyendranath (Dartmouth, Canada: IOCCG). Available at: https://ioccg.org/wp-content/uploads/2015/10/ioccg-report-03.pdf (Accessed December 14, 2020).
IOCCG (2008). “Why Ocean Colour? the Societal Benefits of Ocean-Colour Technology,” in Reports of the International Ocean-Colour Coordinating Group, No. 7. Editors T. Platt, N. Hoepffner, V. Stuart, and C. Brown (Dartmouth, Canada: IOCCG). Available at: https://ioccg.org/wp-content/uploads/2015/10/ioccg-report-07.pdf (Accessed December 14, 2020).
IOCCG (2012). “Mission Requirements for Future Ocean-Colour Sensors,” in Reports of the International Ocean-Colour Coordinating Group, No. 13. Editors C. R. McClain, and G. Meister (Dartmouth, Canada: IOCCG). Available at: http://www.ioccg.org/reports/IOCCG_Report13.pdf (Accessed December 14, 2020).
IOCCG (2015). “Earth Observations in Support of Global Water Quality Monitoring,” in Reports of the International Ocean-Colour Coordinating Group, No. 17. Editors A. Dekker, and C. Binding (Dartmouth, Canada: International Ocean Colour Coordinating Group). Available at: https://ioccg.org/wp-content/uploads/2018/09/ioccg_report_17-wq-rr.pdf (Accessed December 14, 2020).
Johannessen, S. C., Miller, W. L., and Cullen, J. J. (2003). Calculation of UV Attenuation and Colored Dissolved Organic Matter Absorption Spectra From Measurements of Ocean Color. J. Geophys. Res. 108, 17-1–17-13. doi:10.1029/2000jc000514
Kahru, M., di Lorenzo, E., Manzano-Sarabia, M., and Mitchell, B. G. (2012). Spatial and Temporal Statistics of Sea Surface Temperature and Chlorophyll Fronts in the California Current. J. Plankton Res. 34, 749–760. doi:10.1093/plankt/fbs010
Kutser, T., Metsamaa, L., Strömbeck, N., and Vahtmäe, E. (2006). Monitoring Cyanobacterial Blooms by Satellite Remote Sensing. Estuar. Coast. Shelf Sci. 67, 303–312. doi:10.1016/j.ecss.2005.11.024
Laanen, M. L., Peters, S. W. M., Dekker, A. G., and Woerd, H. J. v. d. (2011). Assessment of the Scattering by Sub-Micron Particles in Inland Waters. J. Eur. Opt. Soc. Rapid. Publ. 6, 15. doi:10.2971/jeos.2011.11046
Lee, Z., Carder, K. L., and Arnone, R. A. (2002). Deriving Inherent Optical Properties From Water Color: A Multiband Quasi-Analytical Algorithm for Optically Deep Waters. Appl. Opt. 41, 5755. doi:10.1364/ao.41.005755
Lee, Z., Carder, K. L., Hawes, S. K., Steward, R. G., Peacock, T. G., and Davis, C. O. (1994). Model for the Interpretation of Hyperspectral Remote-Sensing Reflectance. Appl. Opt. 33, 5721. doi:10.1364/ao.33.005721
Lee, Z., Lubac, B., Werdell, J., and Arnone, R. (2009). An Update of the Quasi-Analytical Algorithm (QAA_v5). Available at: https://www.ioccg.org/groups/Software_OCA/QAA_v5.pdf (Accessed December 6, 2020).
Lee, Z., Lubac, B., and Werdell, J. (2014). Update of the Quasi-Analytical Algorithm (QAA_v6). Available at: http://www.ioccg.org/groups/Software_OCA/QAA_v6_2014209.pdf (Accessed December 6, 2020).
Lyon, G. S., and Sutula, M. A. (2011). “Effluent Discharges to the Southern California Bight From Large Municipal Wastewater Treatment Facilities From 2005 to 2009,” in South California Coastal Watershed Research Project Annual Report 2011, Editors Weisberg, S. B., and Miller, K.. Costa Mesa, CA, 223–236.
Mannino, A., Russ, M. E., and Hooker, S. B. (2008). Algorithm Development and Validation for Satellite-Derived Distributions of DOC and CDOM in the U.S. Middle Atlantic Bight. J. Geophys. Res. 113, 1–19. doi:10.1029/2007JC004493
Mannino, A., Signorini, S. R., Novak, M. G., Wilkin, J., Friedrichs, M. A. M., and Najjar, R. G. (2016). Dissolved Organic Carbon Fluxes in the Middle Atlantic Bight: An Integrated Approach Based on Satellite Data and Ocean Model Products. J. Geophys. Res. Biogeosci. 121, 312–336. doi:10.1002/2015JG003031
McKinney, M. L. (2002). Urbanization, Biodiversity, and Conservation. Bioscience 52, 583–590. doi:10.1641/0006-3568(2002)052
McLaughlin, K., Nezlin, N. P., Howard, M. D. A., Beck, C. D. A., Kudela, R. M., Mengel, M. J., et al. (2017). Rapid Nitrification of Wastewater Ammonium Near Coastal Ocean Outfalls, Southern California, USA. Estuar. Coast. Shelf Sci. 186, 263–275. doi:10.1016/j.ecss.2016.05.013
Mevik, B.-H., and Wehrens, R. (2007). TheplsPackage: Principal Component and Partial Least Squares Regression in R. J. Stat. Soft. 18, 1–23. doi:10.18637/jss.v018.i02
Mobley, C. D. (1989). A Numerical Model for the Computation of Radiance Distributions in Natural Waters With Wind-Roughened Surfaces. Limnol. Oceanogr. 34, 1473–1483. doi:10.4319/lo.1989.34.8.1473
Mobley, C. D., Boss, E., and Roesler, C. (2020). A Collaborative Web-Based Book on Optical Oceanography. Available at: https://www.oceanopticsbook.info/view/radiative-transfer-theory/level-2/hydrolight (Accessed December 21, 2020).
Mobley, C. D., Gentili, B., Gordon, H. R., Jin, Z., Kattawar, G. W., Morel, A., et al. (1993). Comparison of Numerical Models for Computing Underwater Light fields. Appl. Opt. 32, 7484–7504. doi:10.1364/AO.32.007484
Mobley, C. D., Sundman, L. K., Davis, C. O., Bowles, J. H., Downes, T. V., Leathers, R. A., et al. (2005). Interpretation of Hyperspectral Remote-Sensing Imagery by Spectrum Matching and Look-Up Tables. Appl. Opt. 44, 3576. doi:10.1364/AO.44.003576
Morrison, J. R., and Nelson, N. B. (2004). Seasonal Cycle of Phytoplankton UV Absorption at the Bermuda Atlantic Time-Series Study (BATS) Site. Limnol. Oceanogr. 49, 215–224. doi:10.4319/lo.2004.49.1.0215
Mouroulis, P., van Gorp, B., Green, R. O., Dierssen, H., Wilson, D. W., Eastwood, M., et al. (2014). Portable Remote Imaging Spectrometer Coastal Ocean Sensor: Design, Characteristics, and First Flight Results. Appl. Opt. 53, 1363. doi:10.1364/ao.53.001363
Mouw, C. B., Greb, S., Aurin, D., DiGiacomo, P. M., Lee, Z., Twardowski, M., et al. (2015). Aquatic Color Radiometry Remote Sensing of Coastal and Inland Waters: Challenges and Recommendations for Future Satellite Missions. Remote Sens. Environ. 160, 15–30. doi:10.1016/j.rse.2015.02.001
Murphy, K. R., Stedmon, C. A., Graeber, D., and Bro, R. (2013). Fluorescence Spectroscopy and Multi-Way Techniques. PARAFAC. Anal. Methods 5, 6557–6566. doi:10.1039/c3ay41160e
Najjar, R. G., Herrmann, M., Alexander, R., Boyer, E. W., Burdige, D. J., Butman, D., et al. (2018). Carbon Budget of Tidal Wetlands, Estuaries, and Shelf Waters of Eastern North America. Glob. Biogeochem. Cycles 32, 389–416. doi:10.1002/2017GB005790
R Core Team (2018). R: A Language and Environment for Statistical Computing. Vienna, Austria: R Foundation for Statistical Computing.
Rabalais, N. N., Turner, R. E., Díaz, R. J., and Justić, D. (2009). Global Change and Eutrophication of Coastal Waters. ICES J. Mar. Sci. 66, 1528–1537. doi:10.1093/icesjms/fsp047
Savitzky, A., and Golay, M. J. E. (1964). Smoothing and Differentiation of Data by Simplified Least Squares Procedures. Anal. Chem. 36, 1627–1639. doi:10.1021/ac60214a047
Siegel, D. A., Maritorena, S., Nelson, N. B., Hansell, D. A., and Lorenzi-Kayser, M. (2002). Global Distribution and Dynamics of Colored Dissolved and Detrital Organic Materials. J. Geophys. Res. 107, 21–1. doi:10.1029/2001jc000965
Stedmon, C. A., and Markager, S. (2003). Behaviour of the Optical Properties of Coloured Dissolved Organic Matter Under Conservative Mixing. Estuar. Coast. Shelf Sci. 57, 973–979. doi:10.1016/S0272-7714(03)00003-9
Sullivan, J. M., Twardowski, M. S., Zaneveld, J. R. v., Moore, C. M., Barnard, A. H., Donaghay, P. L., et al. (2006). Hyperspectral Temperature and Salt Dependencies of Absorption by Water and Heavy Water in the 400-750 nm Spectral Range. Appl. Opt. 45, 5294. doi:10.1364/AO.45.005294
Swan, C. M., Nelson, N. B., Siegel, D. A., and Fields, E. A. (2013). A Model for Remote Estimation of Ultraviolet Absorption by Chromophoric Dissolved Organic Matter Based on the Global Distribution of Spectral Slope. Remote Sens. Environ. 136, 277–285. doi:10.1016/j.rse.2013.05.009
Thompson, D. R., Cawse-Nicholson, K., Erickson, Z., Fichot, C. G., Frankenberg, C., Gao, B.-C., et al. (2019). A Unified Approach to Estimate Land and Water Reflectances with Uncertainties for Coastal Imaging Spectroscopy. Remote Sens. Environ. 231, 111198. doi:10.1016/j.rse.2019.05.017
Thompson, D. R., Natraj, V., Green, R. O., Helmlinger, M. C., Gao, B.-C., and Eastwood, M. L. (2018). Optimal Estimation for Imaging Spectrometer Atmospheric Correction. Remote Sens. Environ. 216, 355–373. doi:10.1016/j.rse.2018.07.003
Tiefenthaler, L. L., Schiff, K. C., and Leecaster, M. K. (2000). Temporal Variability Patterns of Stormwater Concentrations in Urban Stormwater Runoff,” Southern California Coastal Water Research Project Annual Report. Westminster, CA, 52–62.
Tilstone, G. H., Airs, R. L., Vicente, V. M., Widdicombe, C., Llewellyn, C., and Stramski, D. (2010). High Concentrations of Mycosporine-Like Amino Acids and Colored Dissolved Organic Matter in the Sea Surface Microlayer off the Iberian Peninsula. Limnol. Oceanogr. 55, 1835–1850. doi:10.4319/lo.2010.55.5.1835
Trinh, R. C., Fichot, C. G., Gierach, M. M., Holt, B., Malakar, N. K., Hulley, G., et al. (2017). Application of Landsat 8 for Monitoring Impacts of Wastewater Discharge on Coastal Water Quality. Front. Mar. Sci. 4, 329. doi:10.3389/fmars.2017.00329
Tzortziou, M., Neale, P. J., Osburn, C. L., Megonigal, J. P., Maie, N., and JaffÉ, R. (2008). Tidal Marshes as a Source of Optically and Chemically Distinctive Colored Dissolved Organic Matter in the Chesapeake Bay. Limnol. Oceanogr. 53, 148–159. doi:10.4319/lo.2008.53.1.0148
Vandermeulen, R. A., Mannino, A., Neeley, A., Werdell, J., and Arnone, R. (2017). Determining the Optimal Spectral Sampling Frequency and Uncertainty Thresholds for Hyperspectral Remote Sensing of Ocean Color. Opt. Express 25, A785. doi:10.1364/oe.25.00a785
Vodacek, A., Blough, N. v., DeGrandpre, M. D., DeGrandpre, M. D., and Nelson, R. K. (1997). Seasonal Variation of CDOM and DOC in the Middle Atlantic Bight: Terrestrial Inputs and Photooxidation. Limnol. Oceanogr. 42, 674–686. doi:10.4319/lo.1997.42.4.0674
Vodacek, A., Hogel, F. E., Swift, R. N., Yungel, J. K., Peltzer, E. T., and Blough, N. V. (1995). The Use of In Situ and Airborne Fluorescence Measurements to Determine UV Absorption Coefficients and DOC Concentrations in Surface Waters. Limnol. Oceanogr. 40, 411–415. doi:10.4319/lo.1995.40.2.0411
Walker, S. A., Amon, R. M. W., Stedmon, C., Duan, S., and Louchouarn, P. (2009). The Use of PARAFAC Modeling to Trace Terrestrial Dissolved Organic Matter and Fingerprint Water Masses in Coastal Canadian Arctic Surface Waters. J. Geophys. Res. 114, 1–12. doi:10.1029/2009JG000990
Washburn, L., McClure, K. A., Jones, B. H., and Bay, S. M. (2003). Spatial Scales and Evolution of Stormwater Plumes in Santa Monica Bay. Mar. Environ. Res. 56, 103–125. doi:10.1016/S0141-1136(02)00327-6
Werdell, P. J., McKinna, L. I. W., Boss, E., Ackleson, S. G., Craig, S. E., Gregg, W. W., et al. (2018). An Overview of Approaches and Challenges for Retrieving marine Inherent Optical Properties from Ocean Color Remote Sensing. Prog. Oceanogr. 160, 186–212. doi:10.1016/j.pocean.2018.01.001
Yamashita, Y., Boyer, J. N., and Jaffé, R. (2013). Evaluating the Distribution of Terrestrial Dissolved Organic Matter in a Complex Coastal Ecosystem Using Fluorescence Spectroscopy. Cont. Shelf Res. 66, 136–144. doi:10.1016/j.csr.2013.06.010
Zheng, G., Stramski, D., and Digiacomo, P. M. (2015). A Model for Partitioning the Light Absorption Coefficient of Natural Waters Into Phytoplankton, Nonalgal Particulate, and Colored Dissolved Organic Components: A Case Study for the Chesapeake Bay. J. Geophys. Res. Oceans 120, 2601–2621. doi:10.1002/2014JC010604
Zhu, W., Yu, Q., Tian, Y. Q., Chen, R. F., and Gardner, G. B. (2011). Estimation of Chromophoric Dissolved Organic Matter in the Mississippi and Atchafalaya River Plume Regions Using Above-Surface Hyperspectral Remote Sensing. J. Geophys. Res. 116, 1–22. doi:10.1029/2010JC006523
Keywords: imaging spectroscopy, hyperspectral, UV reflectance, water quality, CDOM, fluorescence, wastewater, coastal
Citation: Harringmeyer JP, Kaiser K, Thompson DR, Gierach MM, Cash CL and Fichot CG (2021) Detection and Sourcing of CDOM in Urban Coastal Waters With UV-Visible Imaging Spectroscopy. Front. Environ. Sci. 9:647966. doi: 10.3389/fenvs.2021.647966
Received: 30 December 2020; Accepted: 25 May 2021;
Published: 17 June 2021.
Edited by:
Sherry L. Palacios, California State University, Monterey Bay, United StatesReviewed by:
Ana B. Ruescas, University of Valencia, SpainCopyright © 2021 Harringmeyer, Kaiser, Thompson, Gierach, Cash and Fichot. This is an open-access article distributed under the terms of the Creative Commons Attribution License (CC BY). The use, distribution or reproduction in other forums is permitted, provided the original author(s) and the copyright owner(s) are credited and that the original publication in this journal is cited, in accordance with accepted academic practice. No use, distribution or reproduction is permitted which does not comply with these terms.
*Correspondence: Joshua P. Harringmeyer, am9zaHVhcGhAYnUuZWR1; Cédric G. Fichot, Y2dmaWNob3RAYnUuZWR1
†ORCID: Karl Kaiser, orcid.org/0000-0002-4951-4665
Disclaimer: All claims expressed in this article are solely those of the authors and do not necessarily represent those of their affiliated organizations, or those of the publisher, the editors and the reviewers. Any product that may be evaluated in this article or claim that may be made by its manufacturer is not guaranteed or endorsed by the publisher.
Research integrity at Frontiers
Learn more about the work of our research integrity team to safeguard the quality of each article we publish.