- 1School of Resource and Environmental Management, Simon Fraser University, Burnaby, BC, Canada
- 2Biological Sciences, Simon Fraser University, Burnaby, BC, Canada
- 3Insectarium de Montréal, Montréal, QC, Canada
- 4Great Lakes Institute for Environmental Research, University of Windsor, Windsor, ON, Canada
The Monarch butterfly eastern population (Danaus plexippus) is in decline primarily due to habitat loss. Current habitat restoration programs focus on re-establishing milkweed, the primary food resource for Monarch caterpillars, in the central United States of America. However, individual components of the Monarch life cycle function as part of an integrated whole. Here we develop the MOBU-SDyM, a migration-wide systems dynamics model of the Monarch butterfly migratory cycle to explore alternative management strategies’ impacts. Our model offers several advances over previous efforts, considering complex variables such as dynamic temperature-dependent developmental times, dynamic habitat availability, and weather-related mortality across the entire range. We first explored whether the predominant focus of milkweed restoration in the mid-range of the Monarch’s migration could be overestimating the Monarch’s actual habitat requirements. Second, we examined the robustness of using the recommended 1.2–1.6 billion milkweed stems as a policy objective when accounting for factors such as droughts, changes in temperature, and the stems’ effective usability by the Monarchs. Third, we used the model to estimate the number and distribution of stems across the northern, central, and southern regions of the breeding range needed to reach a self-sustainable long-term Monarch population of six overwintering hectares. Our analysis revealed that concentrating milkweed growth in the central region increases the size of the overwintering colonies more so than equivalent growth in the south region, with growth in the northern region having a negligible effect. However, even though simulating an increase in milkweed stems in the south did not play a key role in increasing the size of the overwintering colonies, it plays a paramount role in keeping the population above a critically small size. Abiotic factors considerably influenced the actual number of stems needed, but, in general, our estimates of required stems were 43–91% larger than the number of stems currently set as a restoration target: our optimal allocation efforts were 7.35, 92, and 0.15% to the south, central, and northern regions, respectively. Systems dynamics’ analytical and computational strengths provided us with new avenues to investigate the Monarch’s migration as a complex biological system and to contribute to more robust restoration policies for this unique species.
1. Introduction
There is a broad agreement that habitat loss is one of the most critical causes of species decline worldwide (Andrén, 1997), particularly with habitat species where life history is tightly bound to a limited number of habitat elements, such as specific host plants for breeding or feeding (Matthews et al., 2014). However, in many such species, knowledge of host-plant phenology is still not at the level needed for effective management. As a result, it is common practice to simplify the underlying dynamics driving host-plant phenology and assume that host availability is relatively constant. This assumption is potentially risk-prone, given the effects of climate change. This paper explores the implications of such assumptions for the eastern North American Monarch Butterfly, Danaus plexippus (referred from here on as “Monarch”).
The eastern North American population of the Monarch Butterfly has the longest migration of any insect (Carlos Galindo-Leal, 2005). Every winter, individuals across the Eastern United States and Canada travel up to 4,000 km south to establish highly concentrated overwintering colonies within a few forest patches in Mexico, concentrated in the Monarch Butterfly Biosphere Reserve (Urquhart and Urquhart, 1976; Santiago et al., 2001). The high concentration of overwintering individuals allows the estimation of their population size by measuring the total area occupied by the overwintering colonies (Oberhauser et al., 2008). Although this estimate is considered inaccurate (Hristov et al., 2019), it has been the primary tool for designing Monarch’s conservation strategies. Critically, the total area of the Monarch overwintering colonies in Mexico has steeply decreased over the past two decades (Rendón-Salinas et al., 2019) with a noticeable downward trend that reached an all-time low during the 2013/14 overwintering season of 0.69 ha versus the long-time average of approximately 6 ha (Rendón-Salinas et al., 2019).
The Monarch’s primary conservation challenge is habitat loss due to its specialized habitat needs, both during the breeding and the overwintering stages (Flockhart et al., 2015). On the overwintering grounds, canopy integrity is critical, and its loss, due primarily to illegal logging (Oberhauser et al., 2008; Vidal et al., 2013), exposes the Monarchs to sub-freezing temperatures (Oberhauser et al., 2008) that reduce survival (Anderson and Brower, 1996). However, since the Monarch Biosphere Reserve’s core zone has been virtually free from illegal logging for the last several years (WWF-Mexico, 2019), the breeding range currently receives the most attention and conservation efforts (Thogmartin et al., 2017a). While breeding and feeding in the United States and Canada, the Monarch requires patches of land with plants from the Asclepias genus, mainly Asclepias syriaca, commonly known as milkweed (Malcolm and Brower, 1986; Batalden and Oberhauser, 2015), linking their dynamics to milkweed habitat availability during this stage.
The leading hypothesis explaining the Monarch’s decline is the loss of milkweed, driven by changing farming practices across the United States and Canada breeding areas. Milkweed grows naturally in disturbed areas such as ditches, abandoned land, side roads, and farm outskirts (Jeffery and Robison, 1971; Oberhauser et al., 2008). Evidence shows that milkweed abundance has decreased considerably over the last two decades due to changes in agricultural methods, including the introduction of genetically modified crops coupled with specialized herbicides such as glyphosate (Pleasants and Oberhauser, 2012). Researchers have cited this decrease as the primary cause of the Monarch’s decline (Hartzler and Buhler, 2000; Hartzler, 2010; L. Brower et al., 2012). As a response, the “United States Cornbelt” encompassing the states of Iowa, Illinois, Indiana, southern Michigan, western Ohio, eastern Nebraska, and south Minnesota has received most of the attention for habitat restoration efforts. The main argument for this restoration approach is that the United States Cornbelt provides the natal habitat for most Monarchs overwintering in Mexico (Pleasants and Oberhauser, 2012; Flockhart et al., 2013; Green et al., 2018), is the region with the highest concentration of GMO farmland, and it has the least amount of remaining native plant composition (Holt, 2017).
In this paper, we examine two current assumptions considered in the design of Monarch conservation strategies. First, following classic exponential growth, we hypothesize that first-generation breeding success across the southern part of the range may be more critical to the demographics of subsequent generations leading to the final pre-overwintering population, compared with the mid-section of the breeding range as it is commonly assumed (Crewe et al., 2019). Mortality and lack of reproductive activity while overwintering (L. P. Brower and Missrie, 1999) translate into the lowest Monarch abundance during the early spring, i.e., when Monarchs arrive at the southern edge of their breeding range from the overwintering grounds. Not disregarding central North America’s importance as a critical Monarch breeding region, that early-spring Monarch’s low abundance suggests that the southern region could play a pivotal role in the cycle’s success. Second, recovery strategies must consider that Monarchs will not use the entirety of the stems provided, either due to limiting factors such as droughts and temperature changes or by idiosyncratic factors related to the stem’s appeal to the Monarch. In other words, it is crucial to understand the difference between available and total stems and that a successful strategy will need to provide a considerably larger number of total stems to reach the Monarch’s required available stems. With that in mind, we hypothesize that the number of 1.3–1.6 billion new milkweed stems per annum needed to reach a minimum viable population size falls short of the actual number the Monarch needs to establish a self-sustaining population successfully (Pleasants, 2017; Thogmartin et al., 2017b).
To test our hypotheses, we developed the Monarch Butterfly Systems Dynamics Model (MOBU-SDyM). The MOBU-SDyM is a full-migration, temperature-dependent systems dynamics model of the Monarch butterfly. Through a Bayesian-driven estimation approach, we used this model to test the hypotheses presented above and estimate the yearly number of stems that would need to be added across the breeding region to reach a self-sustainable long-term population of six overwintering hectares. Throughout the analysis, we highlight the differences between considering total versus available milkweed stems. To our knowledge, three simulation models of the Monarch’s full migration exist (Yakubu et al., 2004; Flockhart et al., 2015; Oberhauser et al., 2017) but none of these models explicitly incorporate tightly coupled biotic and abiotic components of the system. Innovatively, we investigate the effects of temperature and humidity on milkweed production and the temperature-dependent behavior of Monarch reproductive capacity and intergenerational time across the landscape, all critical factors affecting Monarch population dynamics.
2. Methods
We first describe how the MOBU-SDyM captures the population dynamics of the Monarch’s migration, including the model’s initial setup and the main equations and assumptions. Next, we focus on performance metrics to assess the model’s capacity to capture the real-life system. After that, we present a sensitivity analysis that tests the leverage1 that variations in the number of milkweed stems have over the overwintering colonies’ geographic area. Finally, we describe our optimization-driven process to estimate the most significant yearly milkweed allocation across the breeding regions. The last two steps underscore the importance of differentiating total and available milkweed stems. The TRACE documentation (Grimm et al., 2014) of this document (Supplementary Appendix A1) describes the model in full detail, parameters, equations, code, description, and discussion of all the assumptions, and Table 1 includes all model parameters and nomenclature.
2.1. Overview of the MOBU-SDyM
The MOBU-SDyM is a spatially explicit stage-structured model with four geographic regions (Breeding regions in the South, Central, and North and one Overwintering region in Mexico) and three Monarch stage classes (Immature, Breeding Adults, and Overwintering Adults)2. The breeding range is divided latitudinally in the model from 30°-35°, 35.1°-40°, and 40.1°-50° for the South, Central and North regions, respectively and longitudinally from 75°-105° (Figure 1). A system of coupled partial differential equations described briefly in the following sections, and detailed in Supplementary Appendix A1, generates the model’s dynamics.
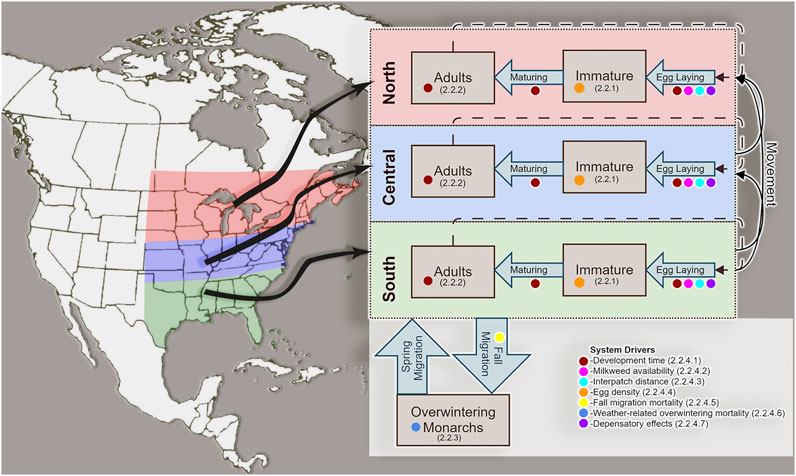
FIGURE 1. Spatial (vertical dimension) and stage-class (horizontal dimension) structure of the Monarch Butterfly System Dynamics Model (MOBU-SDyM). Simulated Monarchs reproduce and disperse across the three breeding regions during the breeding months and mobilize to a non-breeding overwintering region during the overwintering season. The colored dots give the key to the system drivers that affect MOBU-SDyM dynamics. Numbers in parentheses provide sections in the paper where a description of that driver can be found.
Monarch development time is temperature-dependent and typically measured in Degree-Days (°D). As such, spatio-temporal variation in temperature and milkweed habitat drive Monarch population dynamics in the MOBU-SDyM through a temperature variable based on historical records. That variable is converted into °D to calculate the expected total development time specific to each region at each time step. The model transitions individuals from the Immature to the Adult class using the Monarch’s requirement of °D, bracketed by the Monarch’s heat impairment threshold and developmental zero temperatures (Zalucki, 1982). Additionally, the adult’s requirement of °D (Zalucki, 1981) dictates the average lifespan of Adults. Temperature, along with soil moisture, also drives milkweed habitat in the model, which, in turn, is essential for the reproductive success of the Monarch (Veihmeyer and Hendrickson, 1927; Jeffery and Robison, 1971; Flanagan and Johnson, 2005; Pleasants and Oberhauser, 2012).
The MOBU-SDyM initializes in January 1994, with all individuals concentrated in the Overwintering class and remaining there until mid-March. The initial value model represents the 6.23 ha of overwintering colonies recorded in 1994 multiplied by a density conversion factor
A breeding season’s success is dictated in the model mainly by the number of individuals arriving from the Overwintering class, density-dependent effects, temperature, and habitat availability. The south migration begins around mid-August with individuals transitioning from the North to Central regions and then mid-September to the South region. Finally, when the migration trigger is set off in the South region (around early October), individuals take an average of 15 simulation days to transition to the Overwintering class. Individuals that return to the Overwintering class do not reproduce and reduce in numbers due to background and weather-related mortality. By mid-December, all the individuals within the simulation concentrate in the Overwintering class, remaining there until mid-March to start a new cycle.
2.2. Population Dynamics
Here we detail how the model captures the Monarch’s lifecycle dynamics by presenting the stock equations that define the number of Immatures and Adults across the different sections of the model. These descriptions are further explained as the flows that integrate across the stock equations3. The life cycle dynamics outlined here are repeated for each of the Breeding regions, with any differences in the Adult classes of different regions highlighted. The remaining section, Drivers, focuses on defining the factors that drive the life cycle dynamics and how they are explicitly incorporated into the model.
2.2.1. Immature Individuals
The number of Immature individuals (including eggs, larvae, and pupae) found in region x at the end of time step4t (
From Eq. 1, the number of eggs laid per Adult female multiplied by the total number of females in time t determines the number of new individuals joining the Immature class (
Previous Monarch models have assumed a constant development rate for the Monarchs (Flockhart et al., 2015; Oberhauser et al., 2017). However, this does not capture development time’s variation related to the number of Degree-Days (°D) to which individuals are exposed. The MOBU-SDyM captures this variation by calculating the number of individuals transitioning from the Immature to the Adult class at a time step (
The number of new individuals that die daily within the Immature class (
2.2.2. Adult Individuals
We estimated the number of individuals within the Adults classes (
The movement of any one individual across Breeding regions is assumed to be instantaneous (Eq. 8), and the model uses lookup tables to describe the monthly proportion of individuals moving across the four regions
2.2.3. Migration and Overwintering
A Sun angle of 52° at solar noon is assumed to be a cue for Monarch migratory behavior, although little peer-reviewed research exists in this regard (Perez and Taylor, 2004; Taylor et al., 2019). However, the migratory behavior does not appear precisely the same day every year as it would happen if the Sun angle were the only cue. Evidence suggests that temperature and host plant senescence additionally influence the reproductive diapause before migration (Goehring and Oberhauser, 2002), although the relationship between Sun angle, plant senescence, and weather variables is still unknown. The model explores this uncertainty by assuming a non-fixed migratory trigger
Once migration is triggered within each region
The Central region migrating Adults, already merged with individuals from the North, converge in the South until the migration trigger is activated there. Then, the South region transitions all the Adults toward the Overwintering region. Since the distance from the south to the overwintering region is considerably more than the distance among breeding regions, the MOBU-SDyM assumes that migration will take 15 days for an individual beginning its migration from the South region (
To accommodate for the 15 days between departure from the south and arrival to the overwintering grounds, along with the mortality associated with it, we added a stock variable (
During that migration time, many factors not sufficiently studied but broadly assumed to be present
The Overwintering region holds all the individuals within the system for approximately five months (
2.2.4. Drivers
2.2.4.1. Development Time
Temperature directly affects the time a Monarch takes to develop (Zalucki, 1982); in theory, at low or high temperatures, a Monarch could take as many as 30 days or as few as ten to reach maturity respectively. Moreover, research on Monarchs (Zalucki, 1981) and other butterfly species (Gossard and Jones, 1977) suggests that the adult lifespan is also negatively related to temperature. The MOBU-SDyM captures that variability in development time (
2.2.4.2. Milkweed Availability
In addition to its effect on Monarch development time, milkweed growth and subsequent habitat availability are also affected by temperature. Most experts agree that habitat availability across the breeding regions is the primary driver of Monarch population size (Pleasants and Oberhauser, 2012; Pleasants, 2017; Thogmartin et al., 2017a; Stenoien et al., 2018). Previous studies used the estimated total number of milkweed stems that a region can support as a proxy for habitat availability (Flockhart et al., 2015; Thogmartin et al., 2017b). However, it is essential to consider that the actual number of stems available to the Monarch is highly dependent on weather conditions, e.g., a drought can reduce the number of stems across the landscape. Considering that butterflies are an R-selected species (Pianka, 1970) with highly fluctuating population sizes, models should capture the factors behind these fluctuations, such as dynamic milkweed availability across the landscape. However, no peer-reviewed studies exist linking weather and milkweed growth to the necessary level of detail.
With no quantitative studies linking abiotic parameters to milkweed growth, the MOBU-SDyM includes a hypothesized relationship between temperature, soil moisture, and milkweed availability (
2.2.4.3. Interpatch Distance
Recently, the discussion of milkweed as the main driver of Monarch decline has expanded from considering absolute stem numbers to including their distribution across the landscape (Cutting and Tallamy, 2015; Kasten et al., 2016; Zalucki et al., 2016; Stenoien et al., 2018). We decided to account for interpatch distance since it is an element with considerable impact on the Monarch’s egg-laying success (Zalucki et al., 2016). We included this effect by estimating the average interpatch distance across the landscape
The MOBU-SDyM assumes that each patch is circular and estimates the interpatch distance (
Based on the authors’ empirical observations, the MOBU-SDyM assumes that, a female will lay its eggs only if no other adult individuals are on that same stem. So, the available stems result from subtracting the number of Adults within a region from the total number of stems in that same region. Finally, the area not suitable for egg-laying is defined as the total area of region x
2.2.4.4. Egg Density
According to Flockhart et al. (2012), there is a marked decrease in egg survival when the egg density on a milkweed stem exceeds a threshold. Considering that the milkweed is, arguably, the most critical limiting factor for Monarch success, the density-dependent death rate (
2.2.4.5. Adult Density
Problems that arise at small population size, e.g., inbreeding depression, difficulty in finding a mate, and protection against predators, are grouped within the broad category of depensatory or negative density-dependent effects (also known as Allee effects), all of which can hinder a population’s potential to recover from a perturbation (Freckleton et al., 2005). Currently, no studies have linked the Monarch directly with any of these phenomena. However, these effects are present in other butterfly species (Murphy et al., 1990; Kuussaari et al., 1998). Here, the MOBU-SDyM assumes an individual’s reproductive success logistically decreases once it reaches the lower threshold of approximately 0.5 individuals per hectare (Eq. 32). We employed a Powell hill-climbing optimization algorithm to estimate this parameter (Powell, 1971; Burns and Janamanchi, 2007), using as a start search parameter the estimated Monarch density across the breeding regions during the lowest overwintering season recorded (2014) (Rendón-Salinas et al., 2019). We did not opt to use Markov Chain posterior sampling as we did with the other uncertain parameters in the model since it would considerably complicate the parameter search space, and it proved to have almost a negligible effect within the current reported size of the colonies (Supplementary Appendix A1).
2.2.4.6. Fall Migration Mortality
Multiple demographic drivers exist during the fall migration that could strongly alter the mortality during this stage, e.g., availability of nectar sources (L. P. Brower et al., 2006), road mortality, mosquito-controlling pesticides (Tracy et al., 2019), “sequestration of migrants” by tropical milkweed (Asclepias curassavica) in the southern region (Batalden and Oberhauser, 2015), and climatic adversities such as Atlantic hurricanes (Ries et al., 2018) and droughts. Moreover, some authors propose that some of these drivers may not be solely detrimental but could improve migration success (Ries et al., 2018; Saunders et al., 2019); however, there is an ongoing debate about their magnitude and impact. The MOBU-SDyM indirectly incorporates these arguments by including a fall migration mortality parameter (named “Hurricanes Estimate” in the model) parameterized with its estimated posterior distribution.
The MOBU-SDyM assumes that all the factors comprising the fall migration mortality are constant over time, except for the hurricane’s season intensity, expected to increase in the future (Villarini and Vecchi, 2013). The model uses the historical records of the Power Dissipation Index8 and its forecasted behavior (Villarini and Vecchi, 2013) as a proxy of mean hurricane intensity. The posterior distribution of the fall migration death rate gives a general sense of the possible magnitude of mortality during the fall migration but does not discern which of all those elements are the main contributors to that value. Eq. 13 in Section 2.2.3 section above describes the way the “Hurricanes estimate”
2.2.4.7. Weather-Related Overwintering Mortality
Most of the eastern Monarch population gather in just a few forest patches from early November to mid-March (Urquhart and Urquhart, 1978). This elevated concentration of individuals makes the overwintering sites extremely vulnerable to perturbations, where a localized threat can be devastating (Oberhauser et al., 2008). Although predation by birds and rodents is a constant pressure, the greatest threat to the overwintering Monarchs is extreme weather events such as winter storms (Calvert et al., 1983). Some such events have killed up to 30% of the entire population at a time (L. P. Brower et al., 2004). Exposure to open air due to forest canopy degradation, driven mainly by illegal logging, can exacerbate such casualties (Anderson and Brower, 1996; Saunders et al., 2019).
In the model, the number of individuals dying within the Overwintering region (
2.3. Model Performance
We assessed the MOBU-SDyM’s performance through posterior predictive checks (Meng, 1994), comparing the posterior predictive distribution of the overwintering colonies simulated area on January 20th of every simulation year with the actual data provided by Rendón-Salinas et al. (2019). Then, we estimated the observed population’s growth rate mean and variance of the standard deviates (McCarthy and Broome, 2000) using the yearly area from Rendón-Salinas et al. (2019) and calculated the simulated growth rate for posterior predictive data of the whole posterior sample (39,980 samples). We compared the standard deviates’ means from both datasets using a One-Sample t-test and their variances with a chi-squared test. Complete cycle migratory models of the Monarch have used this same measure of performance in the past (Flockhart et al., 2015).
A model’s accuracy depends on how it can recreate the same mean and variance of the observed data and recreate the same type of oscillations. As a final test of model performance, we used a Receiver Operating Characteristic (ROC) curve (Hanley and McNeil, 1982) to evaluate the model’s capacity to recreate the same oscillation pattern as the pattern of the observed data. The MOBU-SDyM performance metrics were estimated using R (R Core Team, 2019); the “bayesplot,” “stats,” and “pROC” packages were used to estimate the posterior predictive checks, standard deviates metrics, and ROC curve, respectively.
2.4. Model Calibration
There are not enough empirical data in most ecological systems for full parameterization without using highly-aggregated variables that ignore their underlying dynamics and impede emergent behavior within the system (Yates, 2012). Based on sound ecological theory, the structural hypothesis that guided our system suggested that seven parameters necessary to the model were either highly uncertain or had inexistent empirical data. The parameters whose posterior distributions were sampled to calibrate the model belong to one of three groups: 1) Milkweed growth: ideal temperature and humidity for milkweed growth and a moisture estimate scaling factor, 2) Fall migration: Temperature migration threshold and hurricanes estimate, and 3) Overwintering: Monarchs density, and exposure to open sky while overwintering.
We used a Bayesian inference approach (Tulsyan et al., 2016) through the VENSIM’s built-in Markov Chain Monte Carlo & Simulated Annealing method to sample the posterior distributions of these parameters and estimate the most likely vector of values for all of them (Rahmandad et al., 2015). Supplementary Appendix A3 displays the convergence metrics. We also used the sampling of the parameters’ posterior distributions to set confidence bounds around the model’s output and establish confidence limits to our policy recommendations (Gelman et al., 2013).
2.5. Region’s Leverage and Total vs. Available Milkweed Stems
One of our research objectives was to examine how varying the number of milkweed stems affects the overwintering colonies size. Furthermore, we wanted to test the difference in the resulting area of the overwintering colonies when the milkweed restoration strategy’s objective assumes, or not, that every stem planted will be available and used by Monarchs, as currently is accepted. We accomplished these two objectives by setting up an experimental design to “artificially” increase and decrease the total number of stems by 3 × 106, 141 × 106, 723 × 106, 2.06 × 109, and 4.5 × 109 and then allocating them either to one region, equally divided over two regions only, or equally divided across the three regions. This design, consisting of 700 individual runs, ran once per 100 vectors of parameter values drawn from the posterior distribution previously obtained (7,000 runs in total). Finally, we ran the same experiment but increasing or decreasing the available stems instead of total stems, essentially bypassing any assumption of the resource’s incomplete use. For this set of experiments, we used a stable version of the model with all the dynamic variables affecting the model over time fixed to their 2019 values, e.g., temperature, precipitation, sex ratio, PDI. For the analysis of these experiments, we performed a simple linear regression in R (R Core Team, 2019) of the mean simulated overwintering area, dependent variable, versus the number of milkweeds supplemented to each region (South, Central, and North), and their interactions. We averaged the best and most parsimonious candidate models and used them to explain the differences between using total and available stems.
2.6. Milkweed Improvement
Finally, we wanted to calculate the minimum yearly increase of total milkweed stems needed across the three predefined regions to produce a long-term mean size of the Overwintering colonies of 6 ha9. To meet this goal, we set an optimization objective that would reduce the residuals between the expected long-term mean area of 6 ha until the year 2050 and the simulated data by modifying the yearly increase in the number of total milkweed stems across the three breeding regions, while also penalizing the total number of stems needed to achieve that goal. We used a Powell optimization algorithm (hill-climbing optimization), which is the built optimizer in VENSIM. To reduce the computational burden, we set the optimization step to 1 million stems, i.e., the optimizer modified the search values in increments of 1 million. We ran four optimizations for each of a 10% random draw taken from the posterior distribution sample (∼5.3*106 simulations) that we estimated earlier and used the best payoff as the “optimal” solution, given the parameters. Since the actual policy objective set by policymakers is the total number of stems, this was the number we used as the independent variable in this analysis while, at the same time, we recorded the resulting available stems on each run.
We obtained and used climate projections from Villarini and Vecchi (2013) and the B1 scenario from the SRES experiment done by the Canadian Center for Climate Modeling Analysis model with T47 resolution (Flato, 2007) to parameterize the weather variables (PDI, soil moisture, and mean temperature) during the forecasting period to ensure our analysis was relevant under climate change conditions.
3. Results
The results section first presents the obtained posterior distributions of the parameters described in Section 2.3 for the calibration of the MOBU-SDyM, then transitions to describe the model’s performance, followed by an examination between total and available milkweed stems and the different leverage that the three Breeding regions have to changes in milkweed on the Overwintering colonies’ modeled size. Lastly, we provide results on the milkweed stems’ number needed to reach restoration goals.
3.1. Model Performance
The model showed a remarkably good performance in estimating the observed overwintering population’s size, trend, and oscillations under the metrics we used. The posterior predictive checks of the models’ data, also known as “Bayesian p-value” (Meng, 1994), showed 96% accuracy. In other words, the size of the overwintering colonies posterior distribution generated by the model included 96% of the times the data points from the observed colonies’ size. The empirical cumulative distribution function’s visual overlay, another step of the posterior predictive checks, also accurately resembled the observed data’s cumulative distribution function (Supplementary Appendix A1). The ROC curve evaluated the model’s accuracy to predict the direction of observed data’s year-to-year growth, i.e., if the population grows or shrinks. This metric yielded a level of accuracy of 67.91% (CI 95% [67.82–68.94]). The One-Sample t-test to the standard deviates mean, which tested differences between the observed and simulated growth rates, failed to reject the null hypothesis that it was different from 0 (95% CI:[-3.87, 0.54], t = -1.56). The standard deviates variance, evaluated via a Chi-squared test, yielded a variance of 0.1215, rejecting the null hypothesis that it was equal to one, suggesting that the patterns obtained from the MOBU-SDyM tended to vary more than the observed data. The system’s variance has been a problematic element to capture, and previous models have not been able to do so, either (Flockhart et al., 2015).
3.2. Model Calibration
The posterior distribution of all seven parameters subject to Bayesian inference, i.e., ideal temperature and humidity for milkweed growth, moisture estimate scaling factor, temperature migration threshold, hurricanes estimate, Monarchs density, and exposure to open sky while overwintering, fell within credible ranges (Figure 2). The joint posterior distribution for the first group of three variables representing milkweed growth reveals that, even at optimal conditions, stem availability is not greater than 31.37% (Figure 3). The parameter posteriors belonging to the fall migration group showed a considerably narrow distribution, which denotes the system’s high sensitivity to temperature changes during the fall, migration delays, and mortality events during the migration. Finally, with the overwintering group’s parameters, the exposure parameter’s posterior was considerably skewed toward zero while the posterior for the density per hectare of overwintering Monarchs had a wide distribution ranging from 20 to 140 million Monarchs/hectare.
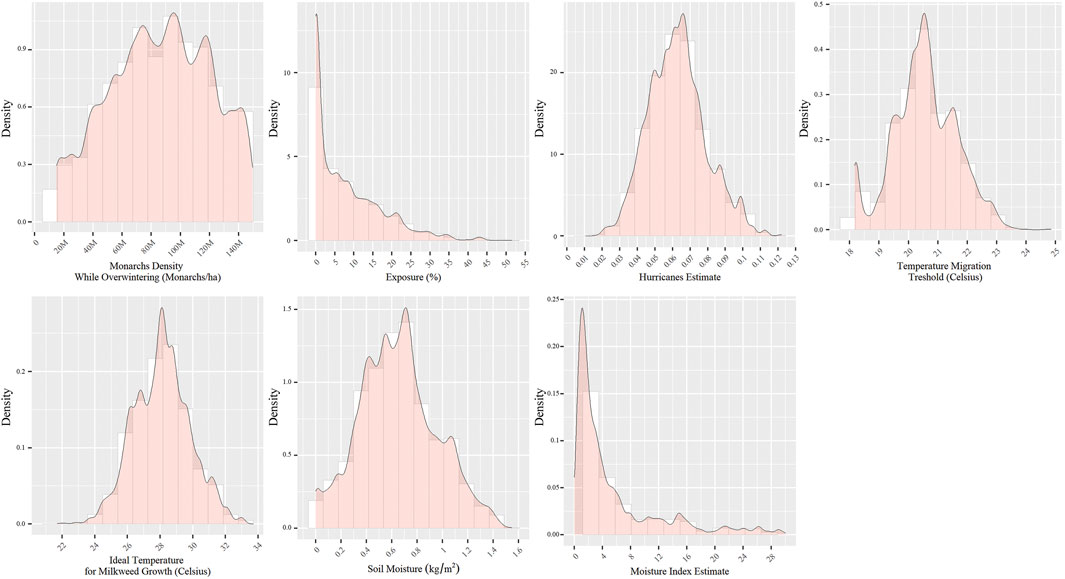
FIGURE 2. Posterior distribution sample (n = 35,603) of parameters used to calibrate the model. For the Moisture Index Estimate, to show in more detail most of the sample and, since 90% of the posterior sample has a value below 30, the figure omits the upper 10% of values (>30, max = 189).
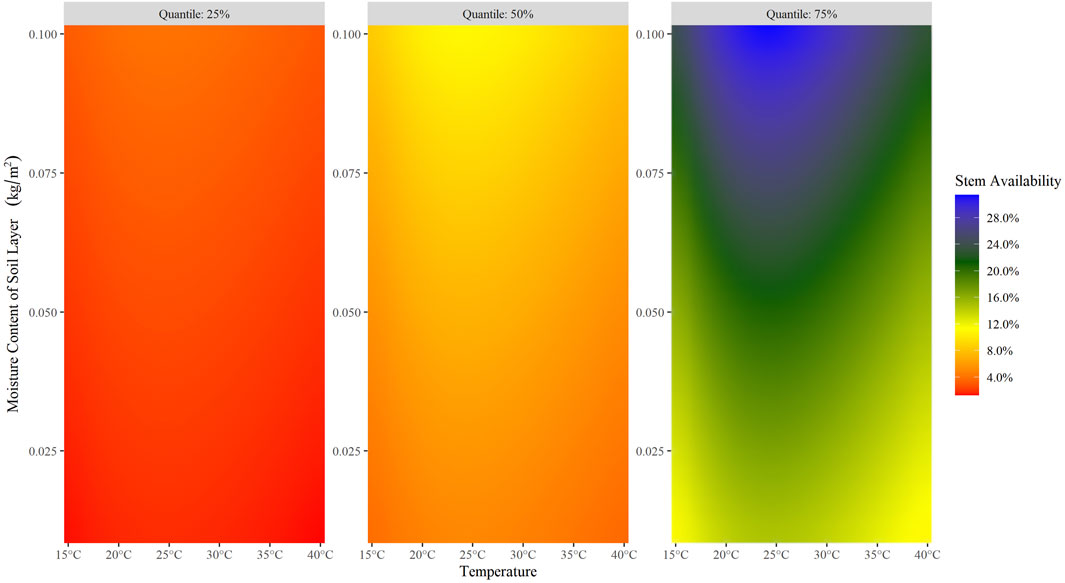
FIGURE 3. Effect of the Soil Moisture, Temperature, and Moisture Estimate joint posterior distribution on the Stem Availability. The stem availability (color of the graph) is estimated via Eq. 23, using as input the mean temperature (x-axis), moisture content of the soil layer (y-axis) and scaled by the moisture estimate. The 25, 50, and 75% quantiles for the resultant posterior predictive data are shown on the left, center, and right panels, respectively.
3.3. Region’s Leverage and Total Vs. Available Milkweed Stems
We compared the differences between modifying the number of total and available stems, i.e., between assuming a perfect use of all milkweed or not due to weather conditions and Monarch’s idiosyncrasy. Under the conditions of the model, an increase of available stems affected the size of the overwintering colonies, almost one order of magnitude more than the same addition of total stems. We also tested the relative contribution (leverage) to the Overwintering colonies’ size of changes of milkweed stems on each of the three Breeding regions. The top regression models concur that one stem of milkweed in the Central region translates into a greater number of Monarchs for the next overwintering season and more than one stem in South, and considerably more than one stem the North region (Table 2). However, each region’s relative contribution substantially changed if the measure used was either total or available stems. More specifically, while adding total milkweed stems in the Central region showed an effect 1.61 times larger than adding this same number of stems in the South, the difference dampened to 0.96 times more if considering available stems instead (Figure 4). This dampening effect is most likely due to climate change affecting milkweed availability and consequent Monarch productivity more in the Central region than in the South (Supplementary Appendix A2).
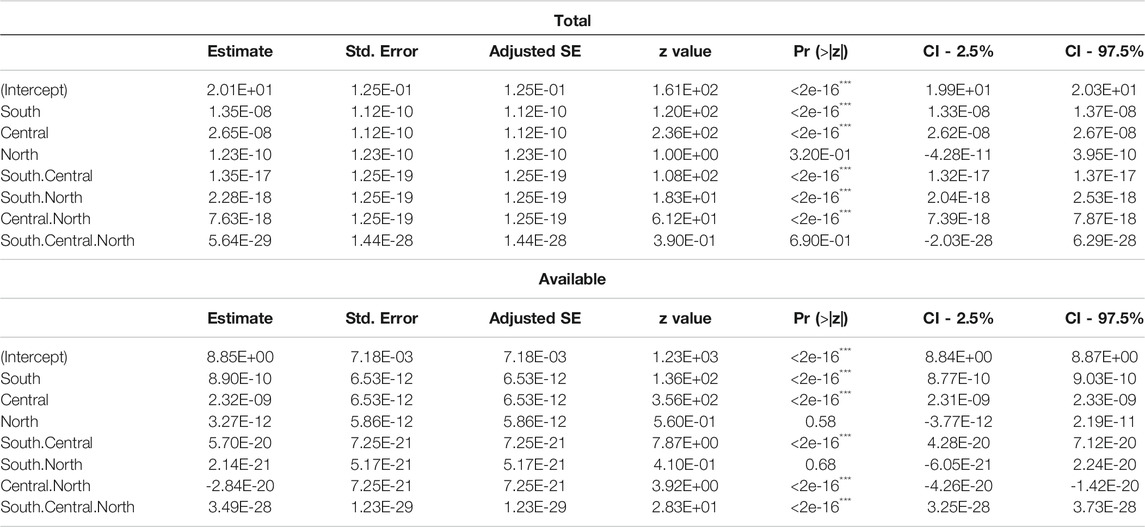
TABLE 2. Regression model for the sensitivity analysis of the effect of total and available milkweed on the size of the overwintering colonies. Model averaging using the 95% confidence set.
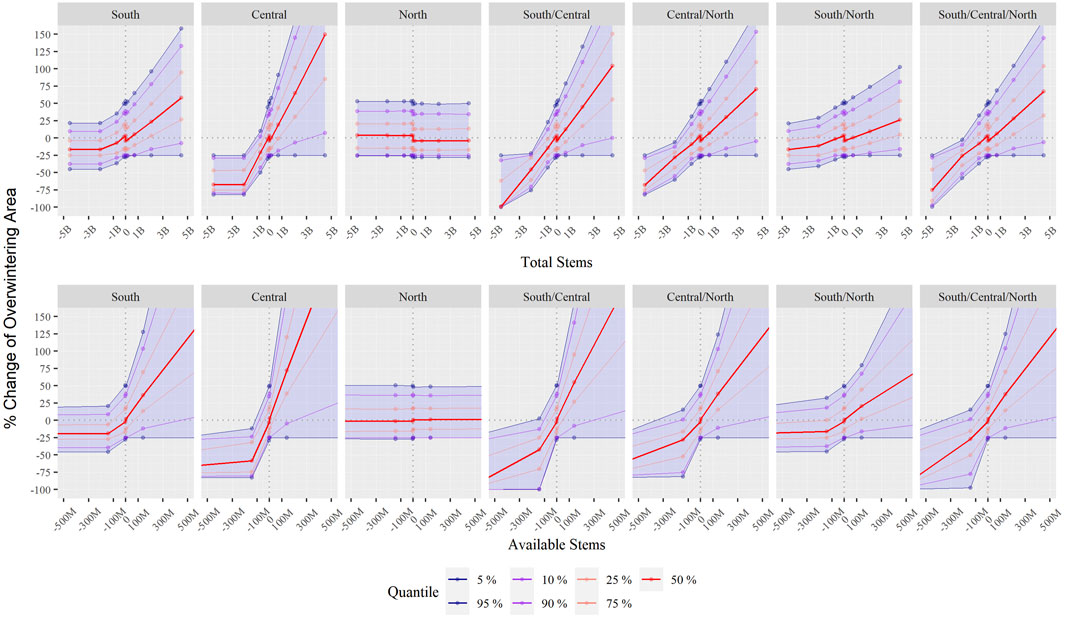
FIGURE 4. Sensitivity analysis to changes in total and available stems across the three breeding regions—confidence intervals obtained from the posterior distribution of the uncertain parameters. Note the difference in magnitude (y-axis) between the effect of changing total (top row) and available (bottom row) stems.
3.4. Milkweed Improvement
We explored different configurations of milkweed enhancement across the landscape in a search for its optimal allocation. In agreement with the previous step of the analysis, the payoff surface’s slope was considerably steeper for the Central breeding region, followed by South, and the shallowest for North region. We did not find any evidence of multiple optima. Under this optimization, the MOBU-SDyM generated posterior distributions for the best habitat rehabilitation strategy entailing mean yearly increases of 1.86*108, 2.41*109, and 5.49*106 total stems for the South, Central and North regions, respectively. These numbers translate into 3.09*107, 2.88*108, and 6.36*105 available stems. Figure 5 depicts the population’s long-term modeled trajectory under this optimization, and Figure 6, the optimal distribution of total and available number of stems given the posterior samples.
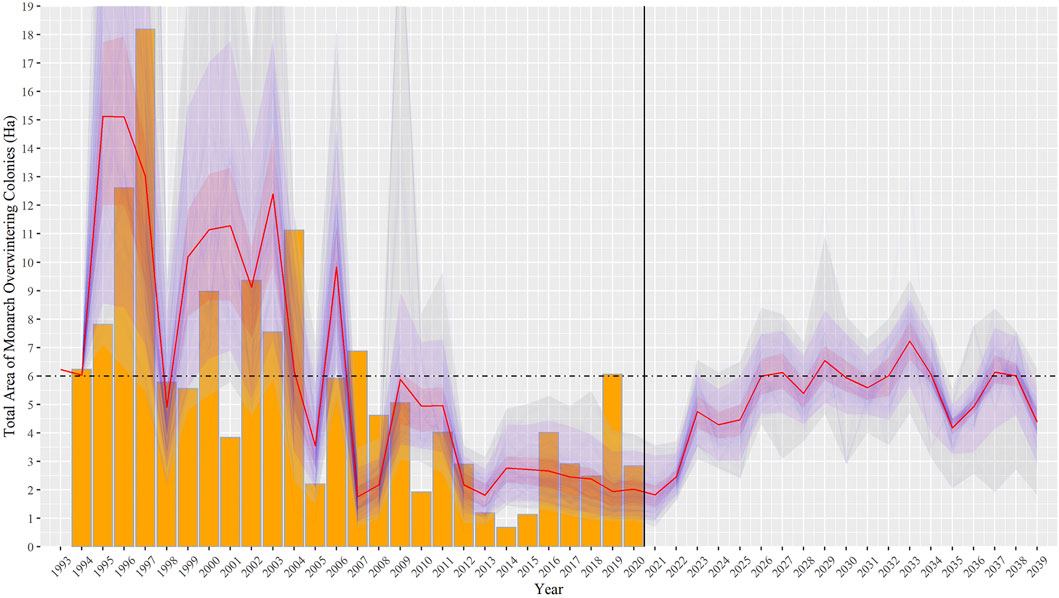
FIGURE 5. Expected recovery of Monarch overwintering counts following the amount and allocation of milkweed stems across the three Breeding regions prescribed by the optimization results. Each blue line represents one simulation using the specific optimum obtained given a specific parameter vector drawn from the posterior distribution. Orange bars represent the observed size of the overwintering colonies.
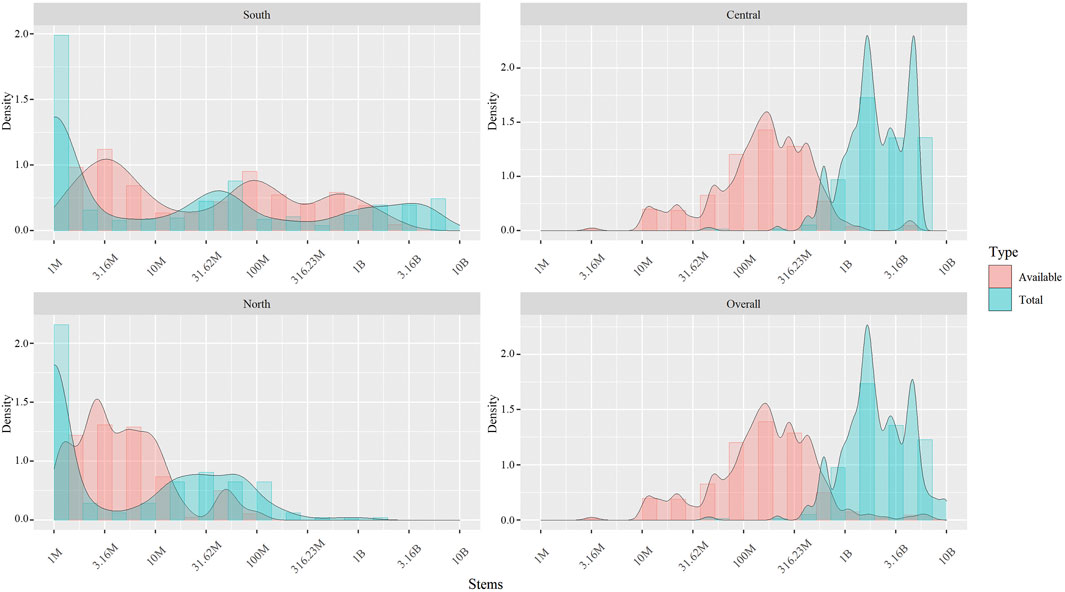
FIGURE 6. Required total number and allocation of milkweed restoration efforts given the posterior sampling as prescribed by the optimization results. The plot shows the difference between the numbers in the simulation as “prescribed by science,” considering perfect use and availability of the resource (Red) and the simulated total number of stems a policy objective should consider (Cyan).
4. Discussion
We developed the most comprehensive full life cycle model of the Monarch Butterfly biology to date, the MOBU-SDyM, which, despite its complexity, showed remarkable performance under several metrics. By including complex dynamic variables, along with the systems dynamics’ analytical and computational strengths, we created new avenues to investigate the complex biological system of the Monarch’s migration. Our model allowed us to test our original hypotheses, i.e., the South region’s importance, the relevance of considering incomplete use of milkweed in setting policy objectives, and the consequent underestimation of the number of currently recommended milkweed stems needed to bring back the Monarch population to a minimum self-sustaining size. In the following section, we discuss our results, the relevance and limitations of including novel dynamic variables, and the implications of our contribution to the overall Monarch’s conservation efforts moving forward.
We aimed to identify the Breeding region with the highest leverage (Senge and Forrester, 1980) on the following year’s Overwintering colonies’ size. Our sensitivity analysis showed substantial leverage of the milkweed availability across the Central region, slightly lower in the South region and almost negligible leverage in the North. Our results agree with previous researchers that identified the United States’s Midwest region (represented by the Central region in our model) as the restoration priority for Monarch conservation (Thogmartin et al., 2017a). This difference in leverage between the South and Central region refutes our initial hypothesis that the South should have more leverage than the Central Region, even when such leverage is substantially dampened when considering available instead of total milkweed stems. One possible explanation behind this result is that high temperatures surpassing the heat impairment threshold of 32°C in the South during several months in mid-summer halt Immature development and considerably decrease the available milkweed stems. If this presumption is correct, the high summer temperatures would have generated a yearly bimodal peak of butterflies in the South, which, indeed, happens in the wild (Inamine et al., 2016) and also occurred in the model (Supplementary Appendix A2). However, this result does not mean that the Central region is the only critical one. We showed through our sensitivity analysis a non-linear interaction between the South and Central regions in which, regardless of considering total or available stems, the reduction (not increasing) of milkweed stems in both regions simultaneously brought about catastrophic consequences (Figure 4). This result suggests that, even though the South may not play a key role in increasing the Overwintering colonies’ size to the desired area, it seems vital to keep the population above a critically small number.
Next, we estimated the mix of habitat restoration efforts across the three regions that would meet the safe minimum threshold of a long-term average of 6 ha of Overwintering colonies (Semmens et al., 2016). We showed that a significant focus should be habitat restoration in the Central breeding region, followed by the South and, to a lesser extent, in the North. The milkweed stem number recommended by our analysis brings the stem count considerably above the estimated number of stems across the breeding regions before 1995 (Flockhart et al., 2015), and it suggests a strategy with restoration efforts of one order of magnitude larger than previously determined (Pleasants, 2017). Our higher than previously suggested stem recommendation results from the imperfect use of the resource, meaning that not every single stem across the landscape will be visited by a Monarch throughout the season or be entirely usable when the Monarch visits. Interestingly, although our stems recommendation is considerably higher than previously suggested (Pleasants, 2017; Thogmartin et al., 2017b), when accounting only for available simulated stems over a 7-years simulation period10, the resulting number is appreciably close to the current recommendation but with a higher resolution in terms of those efforts being focused primarily on the Central region (Figure 5). In other words, previous research is correct regarding the number of stems used by the Monarch but failed to make the distinction that such number is the number used by the Monarch and not the number required to be planted.
Combining our continent-wide milkweed allocation recommendations with land-type specific research can be a powerful guide for policymaking. For example, there is a call for using roadside and railroad right-of-ways for planting milkweed and other nectar flowers to support the Monarch’s breeding and migration (Kasten et al., 2016). According to Pleasants (2017), there are 3.2 × 106 hectares of roadsides across the Midwest. To achieve the restoration requirement that we propose, using the milkweed stem density of 33,030 stems/ha (Ralph, 1977), the total roadside area required to be covered with milkweed would be 72,963 ha, i.e., 2.26% of roadsides across the Midwest. This is a feasible objective considering that roadsides are a relatively idle land where a mix of planting and strategic mowing (Knight et al., 2019) can be easily achieved, compared to other land types. However, Pitman et al. (2018) warns of the potentially increased mortality due to vehicle collisions. We recommend focusing more research on differential roadside mortality based on vehicle throughput and, probably, aiming roadside rehabilitation efforts on low throughput roads.
Conservation strategies need to consider metapopulation dynamics such as patch configuration and heterogeneity while applying our recommendations too (Hanski and Ovaskainen, 2003; Haddad et al., 2017). Even though spatially explicit models would be more suitable for including this kind of element and that analyzing the effect of interpatch distance was not the model’s primary objective, we decided to account for interpatch distance since it is an element with considerable impact over the Monarch’s egg-laying success (Zalucki et al., 2016). Regarding metapopulation dynamics, we also included depensatory effects as a potential population driver; however, at least at the current population size, that effect proved negligible (Supplementary Appendix A1).
We added a dynamic temperature variable affecting the whole system by simulating its influence on Monarch biology via direct effects such as the heat impairment threshold, developmental zero, temperature migration threshold, and subsequent impacts on Monarch’s developmental rate and longevity. Including this element becomes particularly relevant in the light of climate change, where other butterfly species’ first and peak flight times have shifted by as much as ten days per 1°C climate warming (Roy and Sparks, 2000), leading to potentially critical phenology mismatches with their host plants (Posledovich et al., 2015). Our model produces such phenology shifts and shows that temperature affects Monarch population dynamics directly by delaying the migration onset and reducing the intergenerational time when temperatures rise (Supplementary Appendix A1). For example, constant warm temperatures could lead to one whole extra generation of butterflies within the yearly cycle (Supplementary Appendix A2), which, for a species with such reproductive potential as the Monarch, would entail population changes of multiple orders of magnitude (Altermatt, 2010). This result parallels actual biological events, where development time and Monarchs’ longevity (and other insect species) strongly correlate with temperature (Gossard and Jones, 1977; Zalucki, 1981; Zalucki, 1981).
We also included indirect temperature effects as other variables’ drivers interacting with the Monarch’s biological system, namely its interaction with the soil layer moisture content and its impact on milkweed availability. Common knowledge assumes that plants strongly depend on temperature and soil moisture, and a deviation from an ideal mix of these parameters could have consequences on the quality and availability of milkweed at a landscape level. However, despite soil moisture and temperature’s importance on milkweed growth and, consequently, Monarch’s population success, literature documenting such dynamics is scarce. We generated a joint posterior distribution of this relation and its effect on the “ideal” milkweed availability revealing that optimal milkweed availability peaks with a temperature of 28.1°C and 0.65 kg/m2 of soil moisture (0–10 cm). However, even at this optimum, milkweed availability only reaches 31.37% because, being non-geographically explicit, the model assumes that an individual can utilize every stem available in the system, which is not the case. The soil moisture-temperature joint posterior captures the resource’s incomplete usage by making only a portion of the total number of stems available at optimal conditions. Indeed, data obtained from the Monarch Larvae Monitoring Program showed a highly variable weekly density of 0.091 immature individuals/stem during May–September of 2010–2018 (Monarch Larva Monitoring Project, 2019). This imperfect total availability highlights the importance of differentiating between total and available milkweed stems. Our model, as others (Flockhart et al., 2015; Oberhauser et al., 2017), considers only the availability of common milkweed (Asclepias syriaca), basing all our calculations regarding milkweed on rough approximations of the available number of stems in the ground (Flockhart et al., 2015) and leaving aside several other Asclepias species that Monarchs also use (Malcolm and Brower, 1986).
Previous research in other species has highlighted the importance of considering habitat availability as a dynamic variable subject to temporally explicit weather variables. Peterson (1997) analyzed the relationship between host-plant phenology and dispersal of the Dotted Blue butterfly (Euphylotes enoptes) in which the egg-laying behavior was uneven and correlated with their host plant’s artificially staggered phenology. Dennis et al. (2003) extended this idea by proposing a “Functional Resource-Based Concept” for habitat, drawing examples from several butterfly species. He argues that a patch’s size and internal habitat dynamics, such as resource synchronization with stadia, resource disturbance, complementary resources required, and abundance frequency of resources, drive butterflies’ meta-population dynamics.
We also accounted for weather-related mortality during the fall migration, another novel element of the MOBU-SDyM. The fall migration is, arguably, the Monarch’s migratory cycle stage that carries most uncertainty about the nature and intensity of drivers. The migratory Monarch life cycle’s importance has recently been heavily debated in the literature (Agrawal, 2019; Saunders et al., 2019). Unlike previous modeling efforts that, due to lack of data, opted to omit factors influencing the Monarch’s fall migration mortality, we included a mortality variable grouping all of those variables (and other unknown ones) into a single Bayesian posterior distribution. The posterior distribution showed that its effect in the overwintering colonies area is historically small, though slightly increasing towards the present, and substantially increasing into the future (Supplementary Appendixes A1, A2) due to an expected Atlantic hurricane intensity increase (Villarini and Vecchi, 2013). The lack of reproductive activity during migration and overwintering explains this parameter’s small effect over the overwintering colonies. More specifically, one fall-migrating Monarch’s death represents the loss of only one overwintering Monarch; in contrast, one individual’s death earlier in the season removes its expected potential demographic contribution to the next overwintering population. However, a factor not included in our model that could influence the effect of conditions during the fall migration is the loss of fat reserves during migration; current research is undergoing to bridge this knowledge gap.
5. Conclusion
The drivers behind the Monarch’s decline have been a matter of controversy for at least 20 years. Initially, that search pointed to illegal logging at the overwintering sites as the main reason (Oberhauser et al., 2008), later shifting to the well-supported milkweed limiting hypothesis (Pleasants and Oberhauser, 2012). Lately, alternative hypotheses have joined the discussion, such as the nectar-limiting (L. P. Brower et al., 2006) and the tropical milkweed-sequestration (Oberhauser et al., 2008) hypotheses.
Our results bring further evidence to support the milkweed-limiting hypothesis as the Monarch’s main population driver. Our results also acknowledge the importance of including alternative hypotheses and lesser drivers (e.g., depensatory effects, migration mortality, patch configuration, open sky exposure while overwintering) to provide more realistic modeling through a systemic view. Furthermore, our study advances the knowledge of the different breeding regions’ relative contributions to the next overwintering season’s success and, consequently, better informs the geographical allocation of milkweed in large-scale restoration efforts. We also highlight the importance of considering the incomplete use of milkweed in setting policy objectives, currently resulting in underestimating the milkweed stems needed to bring back the Monarch population to a minimum self-sustaining size.
Our results also underscore the need for a deeper understanding of fundamental variables to reduce uncertainty in our Monarch migratory population predictions. More specifically, there is a need for a better population metric at the overwintering sites and to expand, between Texas and the overwintering sites, the long-time standardized monitoring and reporting efforts found throughout the rest of the migratory flyway such as the Integrated Monarch Monitoring Program (Cariveau et al., 2019), Mission Monarch (mission-Monarch.org), The Monarch Larvae Monitoring Program (Kountoupes and Oberhauser, 2008), The TriNational Monarch Knowledge Network (birdscanada.org/birdmon/tmkn/main), and Monarch Watch (Taylor et al., 2019), to name a few. Also, in the light of climate change, modeling efforts not accounting for the dynamic and complex interdependencies between organisms and the changing climate are bound for failure. As such, research should focus intensely on getting empirical evidence to describe the soil moisture-temperature-milkweed growth system that we estimated here. Another important source of uncertainty that we found is the behavioral rules dictating how Monarchs interact with milkweed, i.e., all the cues at the micro and macro scale that determine individuals’ movement and behavior. Ethological research must elicit those rules to design habitat restoration strategies that maximize Monarch’s milkweed usage while reducing egg dumping and immature mortality.
Finally, the Monarch butterfly is a complex social-ecological system in which actions and perceptions from different stakeholders may play a determinant role in conservation strategies’ support and success. Research is undergoing to couple the MOBU-SDyM and the lessons we learned here with previous social research (Solis-Sosa et al., 2019) to give policymakers the necessary tools to conserve the Monarch Butterly’s eastern migratory phenomenon for generations to come.
Data Availability Statement
The raw data supporting the conclusions of this article will be made available by the authors, without undue reservation.
Author Contributions
RS conceived the original idea. RS designed and coded the model with input from CS. RS analyzed the model’s data and implemented a Bayesian approach with guidance from SC. RS wrote the manuscript, and it was revised by AM, CS, SC, with contributions of ML. TRACE documentation was written by RS and edited by CS and AM.
Funding
The RenewZoo program provided funding (Grant No. 481954) as part of the Collaborative Research and Training Experience (CREATE) Program of the Natural Sciences and Engineering Research Council of Canada (NSERC).
Conflict of Interest
The authors declare that the research was conducted in the absence of any commercial or financial relationships that could be construed as a potential conflict of interest.
Acknowledgments
We want to thank the Montreal Insectarium for hosting RS during a considerable part of the modeling process giving him access to the incredible expertize amassed there. Special thanks to all the Monarch Butterfly experts who gave shape to this multi-year effort through their example or guidance.
Supplementary Material
The Supplementary Material for this article can be found online at: https://www.frontiersin.org/articles/10.3389/fenvs.2021.634096/full#supplementary-material
Footnotes
1Places within a complex system where a small change in one parameter can have large effects system-wide (Meadows, 1999).
2To avoid confusing terms, we will not capitalize the physical regions and capitalize the systems classes and regions they represent, e.g., South, Central, North, Overwintering, Breeding.
3For a summary of Systems Dynamics modeling, including stock and flow equations see Meadows (2008).
4Our model had a time step of 0.25 days.
5Randomly generated using the temperature distribution backcasting provided by (Flato, 2007) for every region.
6Developmental Zero is the temperature below no measurable development occurs (Zalucki, 1982).
7Heat Impairment Threshold is the temperature above measurable development does not occur (Zalucki, 1982).
8The Power Dissipation Index, PDI, is defined as the sum of the maximum one-minute sustained wind speed cubed, at six-hourly intervals, for all periods when the cyclone is at least tropical storm strength. This measure can be used as a proxy for the intensity of the hurricane’s season (Villarini and Vecchi, 2013).
9A long-term mean area of the overwintering colonies of 6 ha is the safe-minimum threshold recommended by experts.
10Average simulation years it took the model to reach a stable minimum safe population.
References
Agrawal, A. A. (2019). Advances in Understanding the Long-Term Population Decline of Monarch Butterflies. Proc. Natl. Acad. Sci. USA 116 (17), 8093–8095. doi:10.1073/pnas.1903409116
Altermatt, F. (2010). Climatic Warming Increases Voltinism in European Butterflies and Moths. Proc. R. Soc. B. 277 (1685), 1281–1287. doi:10.1098/rspb.2009.1910
Anderson, J. B., and Brower, L. P. (1996). Freeze-protection of Overwintering Monarch Butterflies in Mexico: Critical Role of the Forest as a Blanket and an Umbrella. Ecol. Entomol. 21 (2), 107–116. doi:10.1111/j.1365-2311.1996.tb01177.x
Baskerville, G. L., and Emin, P. (1969). Rapid Estimation of Heat Accumulation from Maximum and Minimum Temperatures. Ecology 50 (3), 514–517. doi:10.2307/1933912
Batalden, R. V., and Oberhauser, K. S. (2015). Monarchs in a Changing World: Biology and Conservation of an Iconic Butterfly. Karen S. Oberhauser, Kelly R. Nail, and Sonia Altizer. Ithaca, New York: Cornell University Press. 215–224.
Brower, L. P., Fink, L. S., and Walford, P. (2006). Fueling the Fall Migration of the Monarch Butterfly. Integr. Comp. Biol. 46 (6), 1123–1142. doi:10.1093/icb/icl029
Brower, L. P., Kust, D. R., Rendon-Salinas, E., Serrano, E. G., Kust, K. R., Miller, J., et al. (2004). “Catastrophic Winter Storm Mortality of Monarch Butterflies in Mexico during January 2002,” in Monarch Butterfly Biology and Conservation, 151–166.
Brower, L. P., and Missrie, M. (1999). Para comprender la migración de la mariposa monarca, 1857-1995. México, D.F.: Instituto Nacional de Ecología.
Brower, L. P., Taylor, O. R., Williams, E. H., Slayback, D. A., Zubieta, R. R., and Ramírez, M. I. (2012). Decline of Monarch Butterflies Overwintering in Mexico: Is the Migratory Phenomenon at Risk? Insect Conservation Divers. 5 (2), 95–100. doi:10.1111/j.1752-4598.2011.00142.x
Burns, J. R., and Janamanchi, B. (2007). Optimal Control and Optimization of System Dynamics Models: Some Experiences and Recommendations. Proceedings of the 2007 Meeting of the Southwest Region (San Diego, CA: Decision Sciences Institute).
Calvert, W. H., Zuchowski, W., and Brower, L. P. (1983). The Effect of Rain, Snow and Freezing Temperatures on Overwintering Monarch Butterflies in Mexico. Biotropica 15, 42–47. doi:10.2307/2387997
Cariveau, A. B., Holt, H. L., Ward, J. P., Lukens, L., Kasten, K., and Thieme, J. (2019). The Integrated Monarch Monitoring Program: from Design to Implementation. Front. Ecol. Evol. 7, 167. doi:10.3389/fevo.2019.00167
Carlos Galindo-Leal, E. R.-S. (2005). Danaidas: Las Maravillosas Mariposas Monarca. México, D.F.: WWF-Telcel. Publicación Especial.
Chapman, J. W., Bell, J. R., Burgin, L. E., Reynolds, D. R., Pettersson, L. B., Hill, J. K., et al. (2012). Seasonal Migration to High Latitudes Results in Major Reproductive Benefits in an Insect. Proc. Natl. Acad. Sci. 109 (37), 14924–14929. doi:10.1073/pnas.1207255109
Crewe, T. L., Mitchell, G. W., and Larrivée, M. (2019). Size of the Canadian Breeding Population of Monarch Butterflies Is Driven by Factors Acting during Spring Migration and Recolonization. Front. Ecol. Evol. 7, 308. doi:10.3389/fevo.2019.00308
Cutting, B. T., and Tallamy, D. W. (2015). An Evaluation of Butterfly Gardens for Restoring Habitat for the Monarch Butterfly (Lepidoptera: Danaidae). Environ. Entomol. 44 (5), 1328–1335. doi:10.1093/ee/nvv111
David, G. C., Víctor Hugo, V. M., Mauricio, T. M., Ana Luisa, G. S., and Jorge, C. S. (2001). Programa de manejo de la Reserva de la Biosfera Mariposa Monarca. México.
Davis, A. K., and Rendón-Salinas, E. (2010). Are Female Monarch Butterflies Declining in Eastern North America? Evidence of a 30-year Change in Sex Ratios at Mexican Overwintering Sites. Biol. Lett. 6 (1), 45–47. doi:10.1098/rsbl.2009.0632
Dennis, R. L., Shreeve, T. G., and Van Dyck, H. (2003). Towards a Functional Resource-Based Concept for Habitat: a Butterfly Biology Viewpoint. Oikos 102, 417–426. doi:10.1034/j.1600-0579.2003.12492.x
Downhower, J. F. (1988). The Biogeography of the Island Region of Western Lake Erie. Columbus, OH: The Ohio State University Press.
Flanagan, L. B., and Johnson, B. G. (2005). Interacting Effects of Temperature, Soil Moisture and Plant Biomass Production on Ecosystem Respiration in a Northern Temperate Grassland. Agric. For. Meteorology 130 (3-4), 237–253. doi:10.1016/j.agrformet.2005.04.002
Flato, G. M. (2007). IPCC DDC AR4 CGCM3.1-T47_(med-Res) SRESB1 Run2. Retrieved from http://cera-www.dkrz.de/WDCC/ui/Compact.jsp?acronym=CGCM3.1_T47_SRESB1_2.
Flockhart, D. T. T., Pichancourt, J.-B., Norris, D. R., and Martin, T. G. (2015). Unravelling the Annual Cycle in a Migratory Animal: Breeding-Season Habitat Loss Drives Population Declines of Monarch Butterflies. J. Anim. Ecol. 84 (1), 155–165. doi:10.1111/1365-2656.12253
Flockhart, T., Martin, T., and Norris, R. (2012). Experimental Examination of Intraspecific Density-dependent Competition during the Breeding Period in Monarch Butterflies (Danaus plexippus). PLoS One 7 (9), e45080. doi:10.1371/journal.pone.0045080
Flockhart, T., Wassenaar, L., Martin, T., Hobson, K., Wunder, M., and Norris, R. (2013). Tracking Multi-Generational Colonization of the Breeding Grounds by Monarch Butterflies in Eastern North America. Proceedings of the Royal Society B: Biological Sciences, 280. 1768. doi:10.1098/rspb.2013.1087
Freckleton, R. P., Gill, J. A., Noble, D., and Watkinson, A. R. (2005). Large-scale Population Dynamics, Abundance-Occupancy Relationships and the Scaling from Local to Regional Population Size. J. Anim. Ecol. 74 (2), 353–364. doi:10.1111/j.1365-2656.2005.00931.x
Gelman, A., Carlin, J. B., Stern, H. S., Dunson, D. B., Vehtari, A., and Rubin, D. B. (2013). Bayesian Data Analysis. Cambridge, NY: CRC Press. doi:10.1201/b16018
Goehring, L., and Oberhauser, K. S. (2002). Effects of photoperiod, temperature, and host plant age on induction of reproductive diapause and development time in Danaus plexippus. Ecol. Entomol. 27 (6), 674–685.
Gossard, T. W., and Jones, R. E. (1977). The Effects of Age and Weather on Egg‐Laying in Pieris Rapae L. J. Appl. Ecol. 14, 65–71. doi:10.2307/2401827
Gotoh, K., Jodrey, W. S., and Tory, E. M. (1978). Average Nearest-Neighbour Spacing in a Random Dispersion of Equal Spheres. Powder Tech. 21 (2), 285–287. doi:10.1016/0032-5910(78)80097-7
Grant, T. J., Parry, H. R., Zalucki, M. P., and Bradbury, S. P. (2018). Predicting Monarch Butterfly (Danaus plexippus) Movement and Egg-Laying with a Spatially-Explicit Agent-Based Model: The Role of Monarch Perceptual Range and Spatial Memory. Ecol. Model. 374, 37–50. doi:10.1016/j.ecolmodel.2018.02.011
Green, T. R., Kipka, H., David, O., and McMaster, G. S. (2018). Where Is the USA Corn Belt, and How Is it Changing? Sci. Total Environ. 618, 1613–1618. doi:10.1016/j.scitotenv.2017.09.325
Grimm, V., Augusiak, J., Focks, A., Frank, B. M., Gabsi, F., Johnston, A. S. A., et al. (2014). others.Towards Better Modelling and Decision Support: Documenting Model Development, Testing, and Analysis Using TRACE. Ecol. Model. 280, 129–139. doi:10.1016/j.ecolmodel.2014.01.018
Haddad, N. M., Holt, R. D., Fletcher, R. J., Loreau, M., and Clobert, J. (2017). Connecting Models, Data, and Concepts to Understand Fragmentation’s Ecosystem-wide Effects.
Hanley, J. A., and McNeil, B. J. (1982). The meaning and use of the area under a receiver operating characteristic (ROC) curve. Radiology 143 (1), 29–36.
Hanski, I., and Ovaskainen, O. (2003). Metapopulation Theory for Fragmented Landscapes. Theor. Popul. Biol. 64 (1), 119–127. doi:10.1016/s0040-5809(03)00022-4
Hartzler, R. G., and Buhler, D. D. (2000). Occurrence of Common Milkweed (Asclepias Syriaca) in Cropland and Adjacent Areas. Crop Prot. 19 (5), 363–366. doi:10.1016/s0261-2194(00)00024-7
Hartzler, R. G. (2010). Reduction in Common Milkweed (Asclepias Syriaca) Occurrence in Iowa Cropland from 1999 to 2009. Crop Prot. 29 (12), 1542–1544. doi:10.1016/j.cropro.2010.07.018
Holt, H. (2017). Monitoring Monarch Butter_flies and Th_eir Habitat across North America: Inventory and Monitoring Protocols and Data Standards for Monarch Conservation. Montreal, Canada.
Howard, E., and Davis, A. K. (2015). Tracking the Fall Migration of Eastern Monarchs with Journey North Roost Sightings. Monarchs in a Changing World: Biology and Conservation of an Iconic Butterfly. Ithaca: Cornell Univ Press, 207–214.
Hristov, N. I., Nikolaidis, D., Hubel, T. Y., and Allen, L. C. (2019). Estimating Overwintering Monarch Butterfly Populations Using Terrestrial LiDAR Scanning. Front. Ecol. Evol. 7. doi:10.3389/fevo.2019.00266
Inamine, H., Ellner, S. P., Springer, J. P., and Agrawal, A. A. (2016). Linking the Continental Migratory Cycle of the Monarch Butterfly to Understand its Population Decline. Oikos 125 (8), 1081–1091. doi:10.1111/oik.03196
Jeffery, L. S., and Robison, L. R. (1971). Growth Characteristics of Common Milkweed. Weed Sci. 19. 193–196. doi:10.1017/s0043174500048682
Kasten, K., Stenoien, C., Caldwell, W., and Oberhauser, K. S. (2016). Can Roadside Habitat Lead Monarchs on a Route to Recovery?. J. Insect Conserv 20 (6), 1047–1057. doi:10.1007/s10841-016-9938-y
Knight, S. M., Norris, D. R., Derbyshire, R., and Flockhart, D. T. (2019). Strategic Mowing of Roadside Milkweeds Increases Monarch Butterfly Oviposition. Glob. Ecol. Conservation 19, e00678. doi:10.1016/j.gecco.2019.e00678
Kountoupes, D., and Oberhauser, K. (2008). Citizen Science and Youth Audiences: Educational Outcomes of the Monarch Larva Monitoring Project. J. Community Engagement Scholarship 1 (1), 10–20.
Kuussaari, M., Saccheri, I., Camara, M., and Hanski, I. (1998). Allee Effect and Population Dynamics in the Glanville Fritillary Butterfly. Oikos 82, 384–392. doi:10.2307/3546980
Malcolm, S. B., and Brower, L. P. (1986). Selective Oviposition by Monarch Butterflies(Danaus plexippus L.) in a Mixed Stand of Asclepias curassavica L. And A. Incarnata L. in South Florida. J. Lepidopterists Soc. 40 (4), 255–263.
Matthews, T. J., Cottee-Jones, H. E., and Whittaker, R. J. (2014). Habitat Fragmentation and the Species-Area Relationship: a Focus on Total Species Richness Obscures the Impact of Habitat Loss on Habitat Specialists. Divers. Distrib. 20 (10), 1136–1146. doi:10.1111/ddi.12227
McCarthy, M. A., and Broome, L. S. (2000). A Method for Validating Stochastic Models of Population Viability: a Case Study of the Mountain Pygmy-Possum (Burramys Parvus). J. Anim. Ecol. 69 (4), 599–607. doi:10.1046/j.1365-2656.2000.00415.x
Meadows, D. H. (1999). Leverage Points: Places to Intervene in a System. Hartland, VT: Sustainability Institute.
Meadows, D. H. (2008). Thinking in Systems: A Primer. chelsea green publishing. doi:10.1007/978-0-230-59393-0
Meng, X.-L. (1994). Posterior Predictive P-Values. Ann. Stat. 22 (3), 1142–1160. doi:10.1214/aos/1176325622
Monarch Larva Monitoring Project (2019). Monarch Larva Monitoring Project. Retrieved June 13, 2019, from http://www.mlmp.org.
Murphy, D. D., Freas, K. E., and Weiss, S. B. (1990). An Environment-Metapopulation Approach to Population Viability Analysis for a Threatened Invertebrate. Conservation Biol. 4 (1), 41–51. doi:10.1111/j.1523-1739.1990.tb00266.x
Oberhauser, K., Cotter, D., Davis, D., Decarie, R., Behnumea, A., and Galindo-Leal, C. (2008). North American Monarch Conservation Plan. MontrealCanada: Commission on Environmental Cooperation.
Oberhauser, K., Wiederholt, R., Diffendorfer, J. E., Semmens, D., Ries, L., Thogmartin, W. E., et al. (2017). A Trans-national Monarch Butterfly Population Model and Implications for Regional Conservation Priorities. Ecol. Entomol. 42 (1), 51–60. doi:10.1111/een.12351
Perez, S. M., and Taylor, O. R. (2004). The Monarch Butterfly: Biology and Conservation. In Karen S Oberhauser and Michelle J Solensky (Ed.). Ithaca, NY: Cornell University Press. 85–89.
Peterson, M. A. (1997). Host Plant Phenology and Butterfly Dispersal: Causes and Consequences of Uphill Movement. Ecology 78 (1), 167–180. doi:10.1890/0012-9658(1997)078[0167:hppabd]2.0.co;2
Pianka, E. R. (1970). On R- and K-Selection. The Am. Naturalist 104 (940), 592–597. doi:10.1086/282697
Pitman, G. M., Flockhart, D. T. T., and Norris, D. R. (2018). Patterns and Causes of Oviposition in Monarch Butterflies: Implications for Milkweed Restoration. Biol. Conservation 217, 54–65. doi:10.1016/j.biocon.2017.10.019
Pleasants, J. (2017). Milkweed Restoration in the Midwest for Monarch Butterfly Recovery: Estimates of Milkweeds Lost, Milkweeds Remaining and Milkweeds that Must Be Added to Increase the Monarch Population. Insect Conserv Divers. 10 (1), 42–53. doi:10.1111/icad.12198
Pleasants, J., and Oberhauser, K. (2012). Milkweed Loss in Agricultural Fields Because of Herbicide Use: Effect on the Monarch Butterfly Population. Insect Conservation Divers. 6 (2), 135–144. doi:10.1111/j.1752-4598.2012.00196.x
Posledovich, D., Toftegaard, T., Wiklund, C., Ehrlén, J., and Gotthard, K. (2015). Latitudinal Variation in Diapause Duration and Post-winter Development in Two Pierid Butterflies in Relation to Phenological Specialization. Oecologia 177 (1), 181–190. doi:10.1007/s00442-014-3125-1
Powell, M. J. D. (1971). Recent Advances in Unconstrained Optimization. Math. Programming 1 (1), 26–57. doi:10.1007/bf01584071
R Core Team (2019). R: A Language and Environment for Statistical Computing. 3.6.0 ed. Vienna, Austria. Retrieved from https://www.R-project.org.
Rahmandad, H., Oliva, R., and Osgood, N. D. (2015). Analytical Methods for Dynamic Modelers. 1st ed. The MIT Press.
Ralph, C. P. (1977). Effect of Host Plant Density on Populations of a Specialzied, Seed-Sucking Bug, Oncopeltus Fasciatus. Ecology 58 (4), 799–809. doi:10.2307/1936215
Rawlins, J. E., and Lederhouse, R. C. (1981). Developmental Influences of Thermal Behavior on Monarch Caterpillars (Danaus plexippus): an Adaptation for Migration (Lepidoptera: Nymphalidae: Danainae). J. Kans. Entomol. Soc. 54, 387–408.
Rendón-Salinas, E., Martínez-Meza, F., Mendoza-Pérez, M., Cruz-Piña, M., Mondragon-Contreras, G., and Martínez-Pacheco, A. (2019). Superficie Forestal Ocupada por las Colonias de Mariposas Monarca en México Durante La Hibernación de 2018-2019.
Ries, L., Neupane, N., Baum, K. A., and Zipkin, E. F. (2018). Flying through Hurricane Central: Impacts of Hurricanes on Migrants with a Focus on Monarch Butterflies. Anim. Migration 5 (1), 94–103. doi:10.1515/ami-2018-0010
Roy, D. B., and Sparks, T. H. (2000). Phenology of British Butterflies and Climate Change. Glob. Change Biol. 6 (4), 407–416. doi:10.1046/j.1365-2486.2000.00322.x
Saunders, S. P., Ries, L., Neupane, N., Ramírez, M. I., García-Serrano, E., Rendón-Salinas, E., et al. (2019). Multiscale Seasonal Factors Drive the Size of Winter Monarch Colonies. Proc. Natl. Acad. Sci. USA 116 (17), 8609–8614. doi:10.1073/pnas.1805114116
Semmens, B. X., Semmens, D. J., Thogmartin, W. E., Wiederholt, R., López-Hoffman, L., Diffendorfer, J. E., et al. (2016). Quasi-extinction Risk and Population Targets for the Eastern, Migratory Population of Monarch Butterflies (Danaus plexippus). Scientific Rep. 6, 23265. doi:10.1038/srep23265
Senge, P. M., and Forrester, J. W. (1980). Tests for Building Confidence in System Dynamics Models. Syst. Dyn. TIMS Stud. Manag. Sci. 14, 209–228. doi:10.1016/0038-0121(80)90026-9
Solis-Sosa, R., Semeniuk, C., Fernandez-Lozada, S., Dabrowska, K., Cox, S., and Haider, W. (2019). Monarch Butterfly Conservation through the Social Lens: Eliciting Public Preferences for Management Strategies across Transboundary Nations. Front. Ecol. Evol. 7, 316. doi:10.3389/fevo.2019.00316
Stenoien, C., Nail, K. R., Zalucki, J. M., Parry, H., Oberhauser, K. S., and Zalucki, M. P. (2018). Monarchs in Decline: a Collateral Landscape-Level Effect of Modern Agriculture. Insect Sci. 25 (4), 528–541. doi:10.1111/1744-7917.12404
Taylor, O. R., Lovett, J. P., Gibo, D. L., Weiser, E. L., Thogmartin, W. E., Semmens, D. J., et al. (2019). Is the Timing, Pace, and Success of the Monarch Migration Associated with Sun Angle? Front. Ecol. Evol. 7, 442. doi:10.3389/fevo.2019.00442
Thogmartin, W. E., Diffendorfer, J. E., López-Hoffman, L., Oberhauser, K., Pleasants, J., Semmens, B. X., et al. (2017a). Density Estimates of Monarch Butterflies Overwintering in Central Mexico. PeerJ 5, e3221. doi:10.7717/peerj.3221
Thogmartin, W. E., López-Hoffman, L., Rohweder, J., Diffendorfer, J., Drum, R., and Semmens, D. (2017b). Restoring Monarch Butterfly Habitat in the Midwestern US: “All Hands on Deck. Environ. Res. Lett. 12 (7), 074005. doi:10.1088/1748-9326/aa7637
Tracy, J. L., Kantola, T., Baum, K. A., and Coulson, R. N. (2019). Modeling Fall Migration Pathways and Spatially Identifying Potential Migratory Hazards for the Eastern Monarch Butterfly. Landscape Ecol. 34 (2), 443–458. doi:10.1007/s10980-019-00776-0
Tulsyan, A., Bhushan Gopaluni, R., and Khare, S. R. (2016). Particle Filtering without Tears: A Primer for Beginners. Comput. Chem. Eng. 95, 130–145. doi:10.1016/j.compchemeng.2016.08.015
Urquhart, F. A., and Urquhart, N. R. (1978). Autumnal Migration Routes of the Eastern Population of the Monarch Butterfly (Danaus P. Plexippus L.; Danaidae; Lepidoptera) in North America to the Overwintering Site in the Neovolcanic Plateau of Mexico. Can. J. Zool. 56 (8), 1759–1764. doi:10.1139/z78-240
Urquhart, F., and Urquhart, N. (1976). The Overwintering Site of the Eastern Population of the Monarch Butterfly (Danaus P. Plexippus; Danaidae) in Southern Mexico. J. Lepidopterists’ Soc. 30, 153–158.
Veihmeyer, F. J., and Hendrickson, A. H. (1927). Soil-moisture Conditions in Relation to Plant Growth. Plant Physiol. 2 (1), 71–82. doi:10.1104/pp.2.1.71
Vidal, O., López-García, J., and Rendón-Salinas, E. (2013). Trends in Deforestation and Forest Degradation after a Decade of Monitoring in the Monarch Butterfly Biosphere Reserve in Mexico. Conservation Biol. 28 (1), 177–186. doi:10.1111/cobi.12138
Villarini, G., and Vecchi, G. A. (2013). Projected Increases in North Atlantic Tropical Cyclone Intensity from CMIP5 Models. J. Clim. 26 (10), 3231–3240. doi:10.1175/jcli-d-12-00441.1
WWF-Mexico (2019). Degradacion Forestal en la Zona Nucleo de la Reserva de la Biosfera Mariposa Monarca 2018-2019.
Yakubu, A.-A., Sáenz, R., Stein, J., and Jones, L. E. (2004). Monarch Butterfly Spatially Discrete Advection Model. Math. Biosciences 190 (2), 183–202. doi:10.1016/j.mbs.2004.03.002
Yates, F. E. (2012). Self-organizing Systems: The Emergence of Order. F. Eugene Yates, Alan Garfinkel, Donald O. Walter, and Gregory B. Yates (Ed.). New York, US: Springer Science & Business Media, 1–14.
Zalucki, M., Parry, H., and Zalucki, J. (2016). Movement and Egg Laying in Monarchs: To Move or Not to Move, that Is the Equation. Austral Ecol. 2 (41), 154–167. doi:10.1111/aec.12285
Zalucki, M. P. (1982). Temperature and Rate of Development in Danaus Plexippus L. And D. Chrysippus L. (Lepidoptera:nymphalidae). Aust. J. Entomol. 21 (4), 241–246. doi:10.1111/j.1440-6055.1982.tb01803.x
Keywords: Monarch Butterfly, resource allocation, population modelling, systems dynamics, metapopulation, milkweed, conservation, habitat restoration
Citation: Solis-Sosa R, Mooers AØ, Larrivée M, Cox S and Semeniuk CAD (2021) A Landscape-Level Assessment of Restoration Resource Allocation for the Eastern Monarch Butterfly. Front. Environ. Sci. 9:634096. doi: 10.3389/fenvs.2021.634096
Received: 27 November 2020; Accepted: 22 April 2021;
Published: 18 May 2021.
Edited by:
Martin Drechsler, Helmholtz Center for Environmental Research (UFZ), GermanyReviewed by:
Viorel Dan Popescu, Ohio University, United StatesAttila D Sándor, University of Agricultural Sciences and Veterinary Medicine of Cluj-Napoca, Romania
Copyright © 2021 Solis-Sosa, Mooers, Larrivée, Cox and Semeniuk. This is an open-access article distributed under the terms of the Creative Commons Attribution License (CC BY). The use, distribution or reproduction in other forums is permitted, provided the original author(s) and the copyright owner(s) are credited and that the original publication in this journal is cited, in accordance with accepted academic practice. No use, distribution or reproduction is permitted which does not comply with these terms.
*Correspondence: Rodrigo Solis-Sosa, cnNvbGlzQHNmdS5jYQ==