- 1EnviroCore, Dargan Research Centre, Institute of Technology Carlow, Carlow, Ireland
- 2Departamento de Biología, Facultad de Ciencias, Universidad Autónoma de Madrid, Madrid, Spain
- 3MicroGen Biotech Ltd., Enterprise, Research and Innovation Centre, Green Road, Carlow, Ireland
The soil microbiome consists of a vast variety of microorganisms which contribute to essential ecosystem services including nutrient recycling, protecting soil structure, and pathogen suppression. Recalcitrant organic compounds present in soils contaminated with fuel oil can lead to a decrease in functional redundancy within soil microbiomes. Ecopiling is a passive bioremediation technique involving biostimulation of indigenous hydrocarbon degraders, bioaugmentation through inoculation with known petroleum-degrading consortia, and phytoremediation. The current study investigates the assemblage of soil microbial communities and pollutant-degrading potential in soil undergoing the Ecopiling process, through the amplicon marker gene and metagenomics analysis of the contaminated soil. The analysis of key community members including bacteria, fungi, and nematodes revealed a surprisingly diverse microbial community composition within the contaminated soil. The soil bacterial community was found to be dominated by Alphaproteobacteria (60–70%) with the most abundant genera such as Lysobacter, Dietzia, Pseudomonas, and Extensimonas. The fungal community consisted mainly of Ascomycota (50–70% relative abundance). Soil sequencing data allowed the identification of key enzymes involved in the biodegradation of hydrocarbons, providing a novel window into the function of individual bacterial groups in the Ecopile. Although the genus Lysobacter was identified as the most abundant bacterial genus (11–46%) in all of the contaminated soil samples, the metagenomic data were unable to confirm a role for this group in petrochemical degradation. Conversely, genera with relatively low abundance such as Dietzia (0.4–9.0%), Pusillimonas (0.7–2.3%), and Bradyrhizobium (0.8–1.8%) did possess genes involved in aliphatic or aromatic compound degradation.
Introduction
Soil contamination is an ongoing and increasing issue that is of great concern from societal, economic, and environmental perspectives. Contaminants of the greatest concern are petroleum hydrocarbons, heavy metals, pesticides, herbicides, and chlorinated hydrocarbons (Devatha et al., 2019). Due to worldwide demand, population growth, and extensive use of petroleum products, the petrochemical industry has continued to grow and continues to pollute both aquatic and terrestrial environments. This can happen during production, transport, usage, or disposal of the oil (Ramadass et al., 2018; Baoune et al., 2019). Contamination of soil by petroleum hydrocarbons is especially problematic for a variety of physical and chemical reasons and is detrimental to ecosystems due to their lipophilic, mutagenic, and carcinogenic effects of its constituents. When the soil or sediment is contaminated with oil, liquid transfer and air diffusion within the porous spaces of the soil are hindered. This impacts the physical properties of soil such as permeability and moisture content, in addition to chemical properties such as pH and the availability of nutrients such as nitrates, phosphates, and sodium. These physical and chemical changes result in reduced growth of microorganisms and plant life within the soil (Baoune et al., 2019; Devatha et al., 2019). The physical and chemical changes caused by pollution of a pristine ecosystem occur rapidly, greatly impacting the functionality of ecosystem services in that environment. Some sensitive autochthonous microbes are quickly killed or inhibited by the petroleum hydrocarbons, reducing the robustness of the microbial community (Hou et al., 2018; Xu et al., 2018). In addition to this, plants are directly affected by factors such as contaminant toxicity, access to light, and an inability to acquire nutrients and water blocked by oil contamination. These factors significantly impact the plants’ ability to grow. Without a functional microbial community and healthy plant life, the environment is unlikely to support much higher-order lifeforms (Girsowicz et al., 2018; Truskewycz et al., 2019).
Bioremediation can remove large percentages of petroleum hydrocarbons for a relatively low cost and with much less ecological impact than the chemical and physical alternatives. Bioremediation utilizes microorganisms such as bacteria and fungi, often combined with plants, to remove contaminants from the environment (Quintella et al., 2019). The nature and scale of contamination are environment specific, and bioremediation treatments may not be suitable in all cases. As some ecosystems are contaminated by weathered hydrocarbons, which are less bioavailable, it is important to devise functional and efficient microbial consortia for remediation of these sites as microbes are not guaranteed to respond to the fresh and weather hydrocarbons in the same way (Khan et al., 2018; Ramadass et al., 2018).
Total petrochemical hydrocarbon (TPH) concentrations can be a poor indicator of the actual risk associated with toxicity of the bioavailable fraction of the hydrocarbons. Due to their prevalence and intimate contact with the soil, the abundance and diversity of eukaryotic organisms such as fungi and nematodes can provide valuable insights into the toxicity of the contaminants in soils. Nematodes are the most abundant and species-rich metazoan taxon of terrestrial environments. Nematode assemblages typically comprise species with different feeding types and life strategies, as well as different levels of tolerance to changes in environmental conditions (Ekschmitt and Korthals, 2006; Wang et al., 2009). Because of their limited mobility, nematodes are unable to escape pollution events and are in direct contact with their surrounding microhabitat, both through ingestion and due to the permeability of their cuticle to many chemicals, and may react in diverse ways to contaminants. All those reasons justify the use of nematodes as sentinels for toxicity impact studies. The diversity and abundance of nematodes in soil can be a very good indicator of the degree of toxicity associated with hydrocarbon contaminants (Urzelai et al., 2000).
Metagenomics data can provide a huge amount of information on the genetic potential of microbial communities in soil to degrade a pollutant, allowing researchers to determine which community members are potentially degrading the pollutants at the time of sampling. Detection and characterization of key microbial species pinned to specific physiological pollutant-degrading processes will allow us to discover new metabolic pathways and identify previously unknown or ignored microbes that play critical supporting roles in the metabolism and cometabolism of toxic hydrocarbons in soil (Devarapalli and Kumavath, 2015).
The site used in the current study was a former industrial site in Ireland (latitude, 52.8523; longitude, −6.9270). The site was a former food manufacturing site on which pockets of mineral oil-contaminated subsoil (2–4 m below the surface) were discovered. Ecopiles had been used previously on this site in 2009 to successfully remediate TPH-contaminated soil (Germaine et al., 2015). Further site investigations in 2019 resulted in the discovery of additional areas of contamination. Approximately 6,600 m3 of contaminated soil was excavated and stockpiled in preparation for remediation. The source of the hydrocarbons was suspected to be heavy fuel oil used in the heat generators on the site. It is likely that, over time, weathering (evaporation, leaching, and biodegradation) would have removed most of the low molecular components. The remediation target levels were set based on soil guideline values (SGV) using the United Kingdom Environment Agency’s Contaminated Land Exposure Assessment (CLEA) methodology for category 4 screening levels (C4SLs). This sets a target level for various aliphatics and aromatics fractions. The site risk assessment showed that there was a possible source–pathway–receptor linkage in relation to a river running adjacent to the site (classified as a good ecological status), and therefore, the site required remediation. Ecopiling was again chosen as the desired technology to remediate the soil.
The aim of the work described here is to determine the microbial (bacterial and fungal) and nematode community composition within TPH-impacted soil at a former food industry site in Ireland. We also wanted to evaluate the hydrocarbon degradation potential of the indigenous bacterial populations by defining the genes and pathways that are present in Ecopiled soil and their taxonomic assignment. The purpose of this analysis is to use these data as a baseline to determine any temporal changes in community composition as the soil was being remediated.
Materials and Methods
Seven samples of the stockpiled soil were analyzed for TPH and polycyclic aromatic hydrocarbon (PAH) concentration. Analysis and speciation were carried out by an accredited external commercial laboratory (ALS Environmental, United Kingdom). Briefly, TPH extraction was carried out on 10 g of soil sample using a hexane:acetone (50:50) solvent, and the extracts were analyzed using gas chromatography-flame ionization detection (GCxGC-FID). For PAH speciation, 5 g of soil samples was subjected to microwave extraction using a hexane:acetone:trimethylamine (50:45:5) solvent mixture and analyzed by GC-mass spectroscopy (GC-MS). The contaminated soil was amended with chemical fertilizer (24:5 N:P to achieve a 100:10:1 C:N:P ratio). The soil was used to construct seven Ecopiles (Figure 1) as described in Germaine et al., 2015.
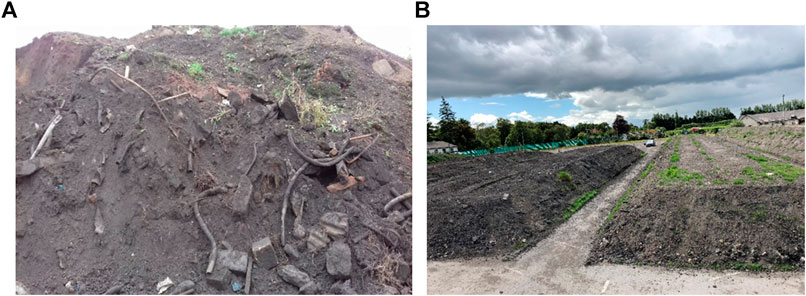
FIGURE 1. (A) Stockpiled soil contaminated with TPH (February 2019) at a former industry site in Ireland. (B) Ecopiles constructed to passively remediate the soil (June 2019).
Ecopile Sampling
For each Ecopile, nine soil samples (∼200 g) were collected (3 from either side and 3 from the top). These soil samples were mixed to create one composite sample. From this composite sample, 2 subsamples were collected for analysis. Each subsample was sieved using a 2 mm sieve and analyzed for aerobic heterotrophic bacterial counts, TPH degrader counts, nitrate and phosphate content, soil pH and conductivity, and % organic matter. Samples were taken for DNA extraction and subsequent microbiome and metagenome analysis.
Processing of Soil and Total DNA Extraction
Soil samples from Ecopiles were first sieved and manually homogenized. Total DNA extraction was achieved using the FastDNA® Spin Kit for Soil (mpbio) following the kit specifications with some modifications. Briefly, 2 g of soil was first resuspended in 5 ml NaCl 0.85% followed by 15 min of vortex. The sample was then centrifuged at 300 × g for 30 s to pellet soil debris. The supernatant was centrifuged again at 8.000 × g for 10 min, and the pellet was resuspended in 1 ml of PBS. An additional incubation with 60 μL/ml of lysozyme (1 mg/ml) at 37°C for 1 h was carried out before continuing with the extraction as described by the manufacturer. The homogenization step in the FastPrep Instrument was repeated four times, reducing the speed of the machine to 5.5.
Sequencing, Filtering of Reads, and Assembly
For bacteria, fungal, and nematode microbiome analyses of the soil DNA samples were sent to Novogene (United Kingdom) for sequencing. For bacterial 16S and fungal analysis community analysis, Novogene standard primer sets were used. For bacterial analysis, the V3-V4 region was amplified using primers 341F 5′-CCTAYGGGRBGCASCAG-3′ and 806 R 5′-GGACTACNNGGGTATCTAAT-3′. For fungal 18S analysis, the V4 region was amplified with a primer set: 528 F 5′-GCGGTAATTCCAGCTCCAA-3′ 706 R 5′-AATCCRAGAATTTCACCTCT-3′. Finally, for the nematode community analysis, primer sets MN18 F 5′-CGCGAATRGCTCATTACAACAGC-3′ and 22(R) –5′-GCCTGCTGCCTTCCTTGGA-3′ were used, as described in Bhadury et al., (2006).
For metagenomic analysis, total DNA extracted from the soil was sequenced at Parque Científico de Madrid (Spain) using the Illumina MiSeq 2x300 system and TruSeq kit for the shotgun library preparation, which were also carried out by the sequencing company. Raw reads were quality filtered using Trimmomatic software v0.39 (Bolger et al., 2014) to remove sequencing adapters and those reads with less than 150 nt, remaining parameters as default. Filtered and trimmed paired reads were assembled with metaSPAdes software v3.13 (Nurk et al., 2017) default parameters. Assembly quality and overall statistics were assessed using QUAST v5.0.2 (Gurevich et al., 2013).
Paired-end reads were merged using FLASH (V1.2.7) (Magoč and Salzberg, 2011). Quality filtering on the raw tags was performed under specific filtering conditions to obtain the high quality clean tag according to the QIIME (V1.7.0) quality control process (Bokulich et al., 2013; Caporaso et al., 2010). The tags were compared with the reference database (Gold database) using the UCHIME algorithm (UCHIME Algorithm) (Edgar et al., 2011) to detect the chimera sequences, and then, the chimera sequences were removed (Haas et al., 2011). Sequences analyses were performed by Uparse software (Uparse v7.0.10) (Edgar et al., 2011) using all the effective tags. Sequences with ≥97% similarity were assigned to the same OTUs. Representative sequence for each OTU was screened for further annotation. For each representative sequence, Mothur software was performed against the SSUrRNA database of SILVA database (version 132) (Wang et al., 2007) for species annotation at each taxonomic rank (threshold: 0.8∼1) (Quast and Pruesse, 2013) (kingdom, phylum, class, order, family, genus, and species). To obtain the phylogenetic relationship of all OTUs representative sequences, MUSCLE (version 3.8.31) (Edgar, 2004) can compare multiple sequences rapidly. OTUs abundance information were normalized using a standard of sequence number corresponding to the sample with the least sequences. Subsequent analyses of alpha diversity and beta diversity were all performed based on this output normalized data.
Metagenome Analyses
Contigs longer than 500 bp were submitted to the SqueezeMeta v1.1.0 (Tamames and Puente-Sanchez, 2019) pipeline for functional annotation. Briefly, gene prediction was achieved with prodigal (Hyatt et al., 2010) and rRNAs using barnap (Seemann, 2014). The resulting rRNA sequences were classified using the RDP classifier (Wang et al., 2007). Diamond (Buchfink et al., 2015) searches against the taxonomic and functional databases GenBank nr NCBI database, eggNOG (Huerta-Cepas et al., 2016), and KEGG (Kanehisa and Goto, 2000) were performed. HMMER3 (Eddy, 2009) was also used for classification against the PFAM database (Finn et al., 2014). Taxonomic assignments of genes were performed using the LCA algorithm and the diamond results against the GenBank nr database. Cutoff identity values were identified for the assignment of taxonomic ranks as previously described (Luo et al., 2014; Tamames and Puente-Sanchez, 2019). Coverage and abundance estimations for each gene were calculated by mapping reads into genes using Bowtie2 (Langmead and Salzberg, 2012) and further processed with BEDTools (Quinlan and Hall, 2010) and SqueezeMeta scripts (Tamames and Puente-Sanchez, 2019) to compute average coverage and normalized TPM (transcripts per kilobase million) values for genes.
The taxonomic profiling of the metagenome was based on the number of reads mapped against classified CDSs (coding DNA sequences), as reported by the SqueezeMeta analysis. Sankey network visualization was achieved using Pavian web application (Breitwieser and Salzberg, 2016). Bar plots of abundance at specific taxonomic ranks were visualized using ggplot2 R package (Wickham, 2011).
Metagenome coverage and sequence-based diversity were determined with the redundancy-based approach implemented in Nonpareil 3 software (Rodriguez-R et al., 2018). Forward filtered reads were used to calculate metagenome coverage and diversity, establishing a similarity threshold of 95%, a minimum overlapping percentage of 50%, and a maximum number of query sequences of 10,000.
Sequence Accession Numbers
The bacterial, fungal, and nematode microbiome sequence data generated in this study are deposited in the National Center for Biotechnology Information (NCBI) under the Bioproject number PRJNA664743. Bacterial 16S datasets are archived under biosample numbers SAMN16231369–16231382, fungal 18S datasets under SAMN16233626–16233639, and nematode 18S datasets under SAMN16234236–16234249. Metagenomic sequence data have been deposited in the NCBI Sequence Read Archive (SRA) under the accession number SRR12703469.
Results
Soil Conditions and Ecopile Construction
The hydrocarbon-impacted soil was generally found to be granular in nature, fine to medium sandy loam (sand 84%, silt 11%, and clay 5%), and contained 5–15% organic matter (mean = 7.94 ± 3.6%) (Table 1). The soil was found to have relatively high pH (average pH 8.9) due to the presence of lime waste from the industrial processes that had taken place on the site. This high pH, coupled with the petroleum contaminants, may explain the relatively low numbers of culturable bacteria present in the soil samples (106–107 g−1 soil). Analysis of the soils before Ecopiling showed average TPH levels (C10–C40) of 9,294 mg·kg−1 (Table 2). The wide range of TPH levels (∼2,000–26,700 mg·kg−1) detected in the seven soil samples demonstrated the heterogeneous dispersal of the hydrocarbons in the soil. TPH analysis showed that the vast majority of the hydrocarbons present were high molecular weight compounds (C16-C35 aliphatics and aromatics, but mostly aromatics).
Microbiome Analysis
Seven Ecopiles were constructed from the contaminated soil. Soil samples were taken from each of the seven Ecopiles, and DNA was extracted from two 0.25 g subsamples per Ecopile (2 technical replicates per Ecopile, 14 samples in total). These were analyzed for bacteria, fungi, and nematode microbiome analysis.
The sequence quality metrics for the 16S marker gene data are given in Supplementary Table S1. In the bacteria microbiomes, nine phyla represented 99% of the bacterial community. The remaining 1% consisted of more than 36 different phyla. Bacteria in the Proteobacteria phylum made up 60–75% of the reads. The Actinobacteria phylum was the next largest group (12–24%, an average of 17%), while Firmicutes made up 3–8% of the community. 734 different bacterial genera were identified in the TPH-contaminated soil with 489 ± 42 identified genera per Ecopile. These made up (on average) 50% of the total sequencing reads per Ecopile sample. At the genus level, the 14 samples had similar bacterial communities (Figure 2). Given that all these Ecopiles are constructed from the same contaminated soil, this similarity in bacterial community is to be expected. 10 genera made up between 39% and 55% of the identified genera found in soil samples (an average of 46%). Lysobacter, a gram-negative genus, was the most identified individual genus across all 14 Ecopile samples (14–39%). Lysinomonas, Luteimonas, Mycobacterium, Pseudomonas, and Extensimonas typically made up 1–6% of the bacterial communities. A total of 1850 different bacterial OTUs were identified across the 14 samples, of which 1,115 OTUs (60%) were common to all 14 samples. Ecopile 5 had the most unique bacterial microbiome with 236 OTUs (12%) that were specific to it. Analysis of the alpha diversity of the seven Ecopiles showed that there was a significant difference between the observed OTUs, Simpson, ACE, and PD whole tree indices of Ecopile 2 and Ecopile 7. There were statistically significant differences between some of the other Ecopiles. However, as there were only two replicates per Ecopile, inference of trends and differences between Ecopiles is not possible. The value of the data is only scientifically valid when looking at overall trends across all 14 samples.
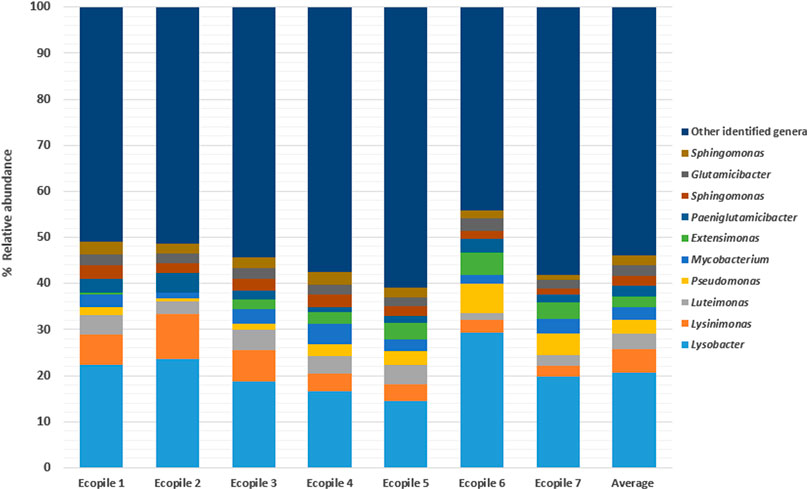
FIGURE 2. The top ten most abundant bacterial genera (relative abundance) in each of the Ecopiles (n = 2). The last bar represents the average bacterial composition across all Ecopile soil samples (n = 14).
The sequence quality metrics for the fungal 18S marker gene data are given in Supplementary Table S2. Analysis of the fungal microbiome of Ecopile soils based on 18S rDNA sequencing found that, on average, 55% (range: 48–64%) of the sequence reads could be assigned to the kingdom Fungi. The remaining sequences were assigned to other eukaryotic soil organisms such as protists, plants, and animals. The fungal microbiome of the Ecopiles identified 7 fungal phyla in the soil. Unidentified fungal reads accounted for 5–50% of the fungal reads (an average of 17%). Reads assigned to the phyluma Ascomycota made up the vast majority (an average of 70%) of the reads in all but not in one Ecopile (Ecopile 5, 37%). At the genus level, 145 different fungal genera were identified in the TPH-contaminated soil with 66–90 different genera per sample. Figure 3 shows the most abundant fungal genera in the Ecopile soil samples. 66 of these genera belonged to the phylum Ascomycota, 39 belonged to Basidiomycota, 17 were unassignable at the phylum level, 12 belonged to Chytridiomycota, 4 belonged to Glomeromycota, 3 belonged to Entomophthoromycota, and 2 belonged to Cryptomycota. The top ten most abundant fungal genera across all Ecopile samples were Ochroconis, Fusarium, Pseudallescheria, Cochliobolus, Boeremia, Cladosporium, Powellomyces, Gaertneriomyces, Spizellomycetales, and Paramicrosporidium.
The sequence quality metrics for the nematode 18S marker gene data are given in Supplementary Table S3. On average, 6-7 different nematode genera could be identified in each Ecopile sample. Figure 4 shows the distribution of the nematode sequence reads assigned at the genus level. On average, 60% of the nematode reads were assigned to the genus Rhabditida, 25% to Diplgasteridae, and 10% to Tylenchida. With the exception of genus Monhysterida in Ecopile 1 (22% of nematode reads), the remaining genera combined made up less than 2% of the nematode reads. Sequences representing 15 individual species of nematode were identified in these soil samples and included Poikilolaimus oxycercus, Rhabditida sp., Ditylenchus destructor, Panagrolaimus detritophagus, Paratylenchus dianthus, Paralamyctes sp., Ditylenchus dipsaci, Acrostichus sp., Prismatolaimus dolichurus, Demaniella sp., Ceroglossini sp., and Aphelenchus avenae.
Metagenome Sequencing Overview and Taxonomic Profiling
A combined sample from different Ecopiles was used for DNA extraction and sequencing. After filtering and trimming, 5,477,721 paired reads (90, 11% of total raw reads) that range from 150 nt to 301 nt were maintained for subsequent assembly. The assembly resulted in 394,033 contigs longer than 500 bp (summing a total length of 362 Mbp). The longest contig was 550,098 bp in length, and the N50 parameter was 870. A total of 656,748 ORFs were predicted, of which 392,806 were annotated against KEGG database, 515,483 against COG database, and 318,861 against Pfam database. 226 rRNAs, 59 of them corresponding to 16S rRNAs, and 3,132 tRNAs were found.
Taxonomic profiling of the metagenome based on reads mapped against classified CDSs is represented in Figure 5A–C. Eukaryota and Archaea domains are irrelevant in the metagenome when comparing their abundances (1.26 and 0.92 thousand reads, respectively) to the number of reads belonging to the Bacteria domain (7.8 million reads). The relative abundance of reads assigned to the class level shows that the metagenome is clearly dominated by Gammaproteobacteria (29.90%), Actinobacteria (19.43%), Betaproteobacteria (17.12%), and Alphaproteobacteria (15.16%). The most abundant family is Xanthomonadaceae (20.70%), followed by Alcaligenaceae (12.26%) and Dietziaceae (7.21%), but other relevant families include Microbacteriaceae, Sphingomonadaceae, and Nocardioidaceae (4.63%, 3.56%, and 2.28%, respectively). Lysobacter (10.34%), Dietzia (7.20%), and Pusillimonas (4.69%) are the most represented genera. On the other hand, the estimated metagenome coverage based on sequence diversity is roughly 51%, with an estimate sequencing effort of 142 Gbp to achieve at least 95% of coverage (Figure 5B). This was not unexpected, as similar coverages are common in metagenomes derived from environmental samples (Rodriguez-R and Konstantinidis, 2014; Johnston et al., 2016).
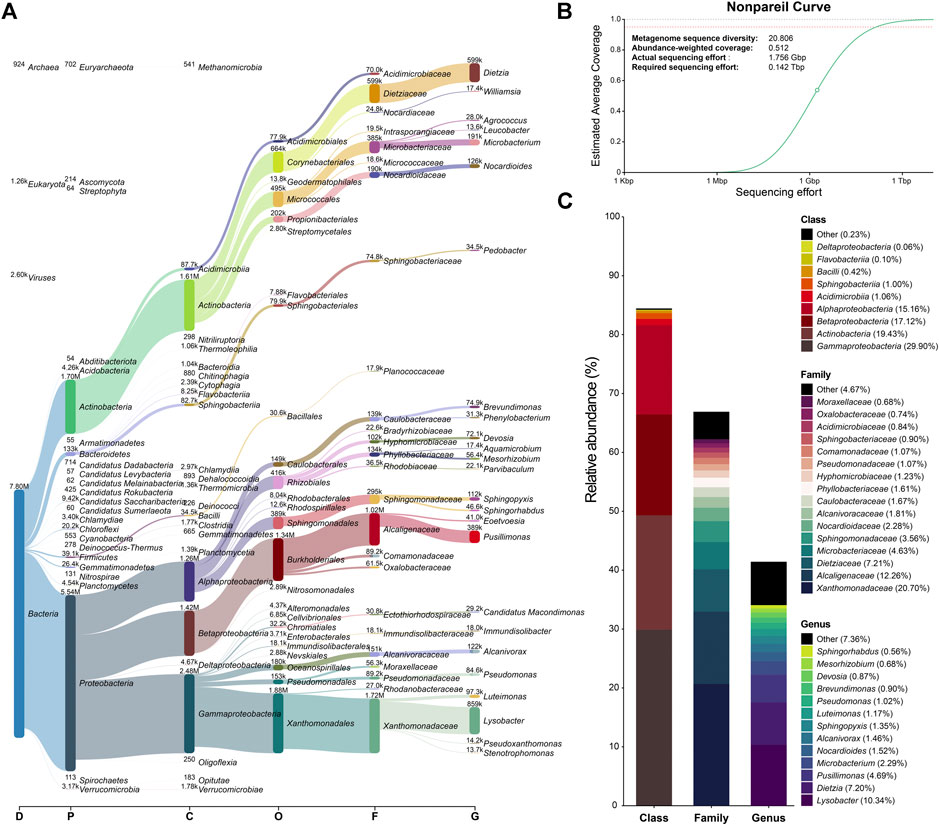
FIGURE 5. Taxonomic profiling of the metagenome based on reads mapped against classified CDSs and metagenome diversity. (A) Sankey network of reads visualized with Pavian. The heights of the rectancles indicate the number of read assigned per taxa and rank, also indicated above/next to each taxa. The scale shows the Domain (D), phyllum (P), class (C), order (O), family (F) and genus (G) taxonomic ranks. Only a maximum of 23 taxa per rank is shown. (B) Nonpareil curve of the average metagenome coverage as a function of sequencing effort. The circle represents the coverage obtained. The line following the circle represents the projection of the fitted model. Horizontal dotted lines represent 100% (gray) and 95% (red) coverage. The model was calculated using only the forward sister of the reads pair, a similarity threshold of 95%, a minimum overlapping percentage of 50% and a maximum number of query sequences of 10,000. (C) Stacked barplot of relative abundance of reads mapping CDSs assigned to class, family and genus ranks. Taxa with a relative abundance lower than 0.05 for class and lower than 0.5 for family and genus are grouped under the “other” category.
Metagenome Functional Characterization
An assessment of the metagenome functional potential was based on the distribution of KEGG metabolic pathways in the predominant families of the metagenome (Figure 6). The most abundant categories according to the number of genes include those of central metabolic pathways, such as carbohydrate and amino acid metabolism, replication and repair, membrane transport, and signal transduction. Interestingly, two categories with a prevalent representation among the dominant families are xenobiotic biodegradation and metabolism and lipid metabolism pathway categories, which, respectively, include aromatic compounds degradation and central lipid metabolism, in which the degradation of alkanes is funneled. Aliphatic and aromatic compounds are the main components of petroleum hydrocarbons. Therefore, we searched the metagenome to identify genes and pathways involved in the degradation of these compounds and assign them to specific populations.
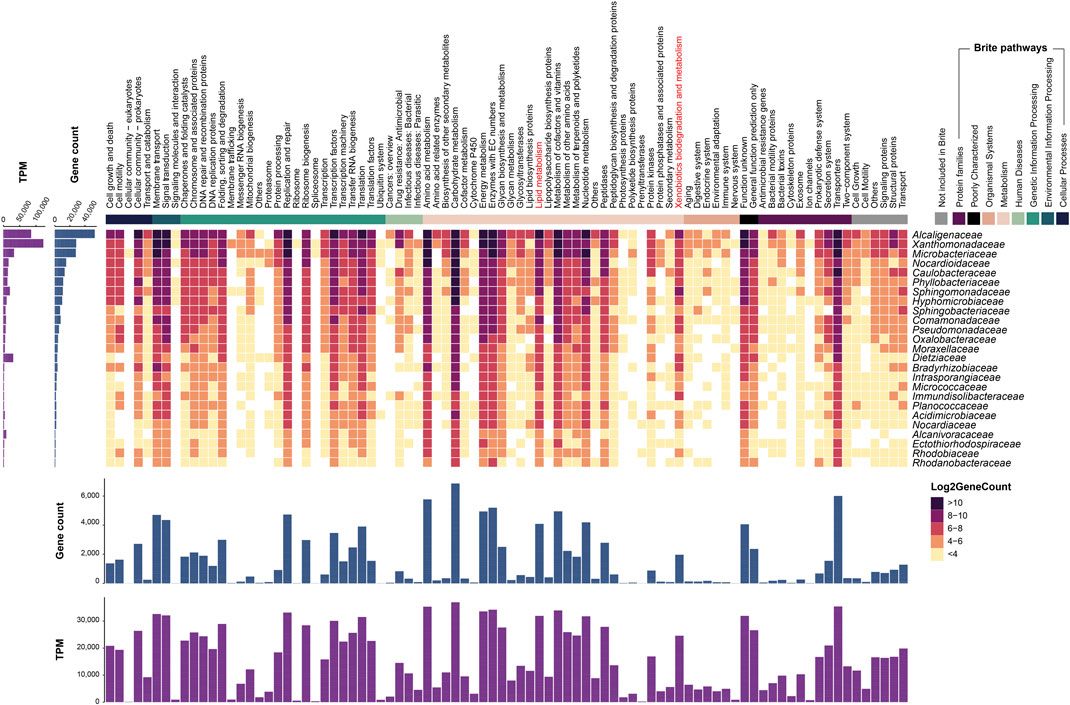
FIGURE 6. Distribution of pathways in the predominant families of the metagenome. Heatmap showing log2 of gene count in families with a relative abundance >0.2%. Pathways according to the Brite classification based on KEGG metabolic pathways. Empty tiles indicate genes of pathways not appearing in the shown families. Sum of gene counts and TPM per family and per pathway is displayed at the left and bottom of the heatmap, respectively. Pathways not assigned to the selected families and genes not assigned to any pathway are not shown.
Metabolism of Alkanes
The results of the identification of genes encoding alkane-degrading enzymes in the metagenome are summarized in Figure 7 and Supplementary Table S4. AlkB (alkane 1-monooxygenase) is a nonheme iron integral membrane protein that carries out the initial hydroxylation of C5-C12n-alkanes, oxidizing them to 1-alkanols (Kok et al., 1989; Van Beilen et al., 1994; Van Beilen et al., 2003). Although almost half of the 55 copies of alkB identified within the metagenome have not been assigned to the family level, the ones which have been classified belong predominantly to Nocardioidaceae (eight copies predominantly assigned to the genus Nocardioides), Immundisolibacteraceae (five copies assigned to the genus Immundisolibacter), Moraxellaceae (five copies), and Nocardiaceae (four copies assigned to the genus Williamsia). LadA (long-chain alkane monooxygenase) also participates in the terminal oxidation pathway of n-alkanes. LadA is a flavoprotein monooxygenase that utilizes dioxygen to insert an oxygen atom into long-chain alkanes (Li et al., 2008; Ji et al., 2013). Seventeen of the 42 copies of ladA identified in the metagenome belong to the Microbacteriaceae family, with seven of them assigned to the genus Microbacterium. On the other hand, short-chain alkanes (<C5) are usually metabolized by methane monooxygenases (MMOs), which are responsible for the first step in their catabolism. Genes encoding the three polypeptides that constitute the pMMO (pmoABC) have been found in the metagenome, while only the α subunit of the hydroxylase component (mmoX) of sMMO has been identified (Figure 7). Moreover, mmoX has been found three times in the metagenome (two of them assigned to the Methylocystaceae family), while copies of the pmoABC cluster (six of pmoA, nine of pmoB, and 13 of pmoC) are distributed mainly within Ectothiorhodospiraceae (three pmoA, three pmoB, and seven pmoC), Nitrosomonadaceae (one pmoA, two pmoB, and two pmoC), and Methylocystaceae (two pmoC) families. The limited presence of sMMO genes compared to pMMO is in agreement with previous works, since whereas pMMO is ubiquitous in methanotrophic bacteria, sMMO has been isolated from only certain strains of methanotrophs (Hanson and Hanson, 1996). In addition, the prmABCD gene cluster encodes the components of a dinuclear iron-containing multicomponent monooxygenase that plays an essential role in propane oxidation via subterminal oxidation to 2-propanol and then further to acetone (Kotani et al., 2003; Kotani et al., 2007). This prmABCD gene cluster has a discrete presence in the metagenome, with a single copy of all the subunits (except prmB, three copies), most of which are only classified at the class level, belonging to Actinobacteria (Figure 7A). Finally, another gene cluster, acmAB, encodes a Baeyer–Villiger monooxygenase and esterase, involved in the metabolism of the acetone derived from propane oxidation (Kotani et al., 2007). Their abundance is also limited to five and ten copies, respectively, assigned mainly to Actinobacteria, Gammaproteobacteria, and Alphaproteobacteria.
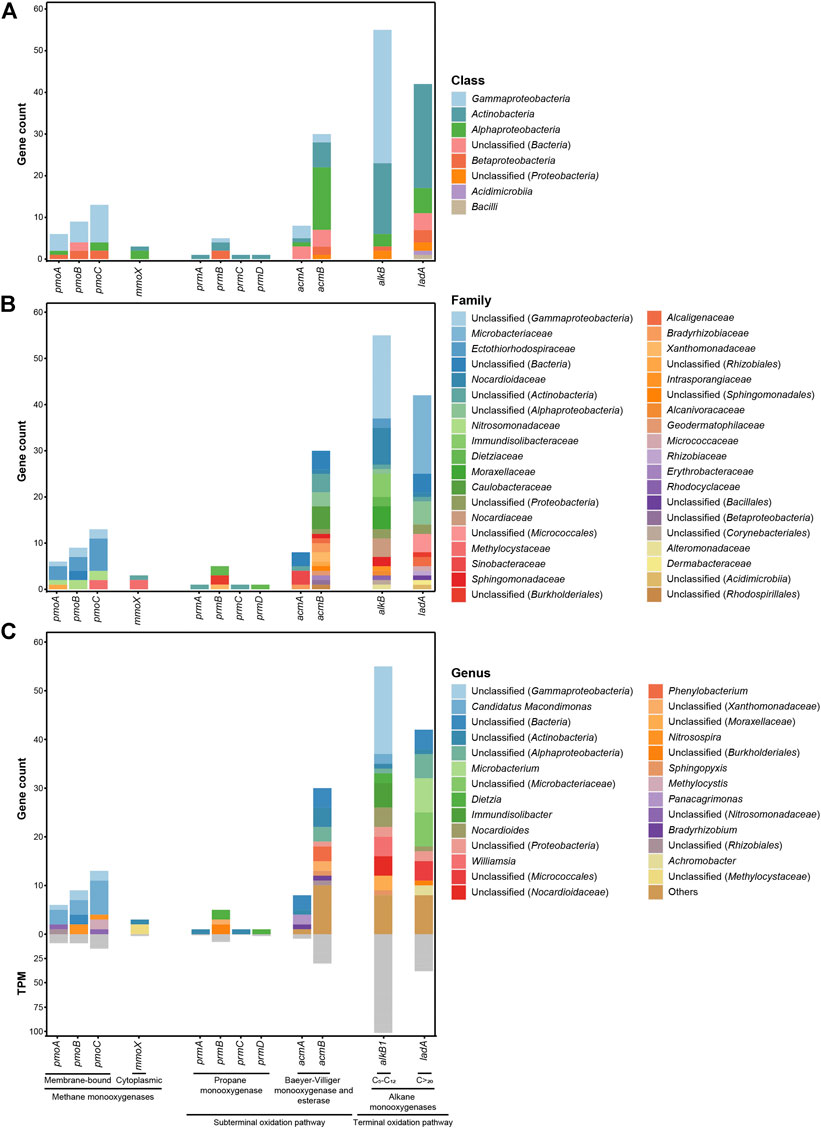
FIGURE 7. Abundance, in terms of number of genes and TPM values, of genes encoding alkane-degrading enzymes identified in the metagenome and classified at class (A), family (B), and genus (C) levels.
Metabolism of Aromatic Compounds
Ring-hydroxylating dioxygenases and monooxygenases identified within the metagenome are shown in Figure 8 and Supplementary Table S5. Biphenyl 2,3-dioxygenase (encoded by bphAa, found nine times in the metagenome, three of them belonging to the genus Immundisolibacter) and toluene monooxygenase (encoded by tmoA, found two times) are involved in the conversion of biphenyl and toluene, respectively, to benzoate. The benzoate ring is further oxidized by benzoate 1,2-dioxygenase (encoded by benA, with 102 copies identified, 21 of them belonging to the genus Immundisolibacter, five to genus Pusillimonas, and four to genus Sphingopyxis) and hydroxybenzoate 3-monooxygenase (encoded by pobA, with 66 copies identified with eight of them assigned to Microbacteriaceae family, six to Alcaligenaceae, and five to Hyphomicrobiaceae). Naphthalene 1,2-dioxygenase (encoded by nahAc) was found to be present only once in the metagenome. This enzyme is implicated in a wide range of different aromatic substrates degradative reactions (Resnick et al., 1996; Ferraro et al., 2017). These reactions include the conversion of naphthalene to salicylate, which is further hydroxylated by salicylate hydroxylases (18 copies of nagG and 80 copies of nahG were found in the metagenome, principally belonging to Alcaligenaceae, Comamonadaceae, Hyphomicrobiaceae, Microbacteriaceae, Phyllobacteriaceae, and Sphingomonadaceae families). Other genes encoding relevant ring-hydroxylating enzymes also found in the metagenome include those involved in the degradation of ethylbenzene (21 copies etbA), phenanthrene (three copies of nidA), phenol (one copy of poxA), and terephthalate (seven copies of tphA2, five of them belonging to Comamonadaceae family). Furthermore, ring cleavage dioxygenases involved in the peripheral degradation pathways of the abovementioned compounds have also been found (Figure 8; Supplementary Table S5).
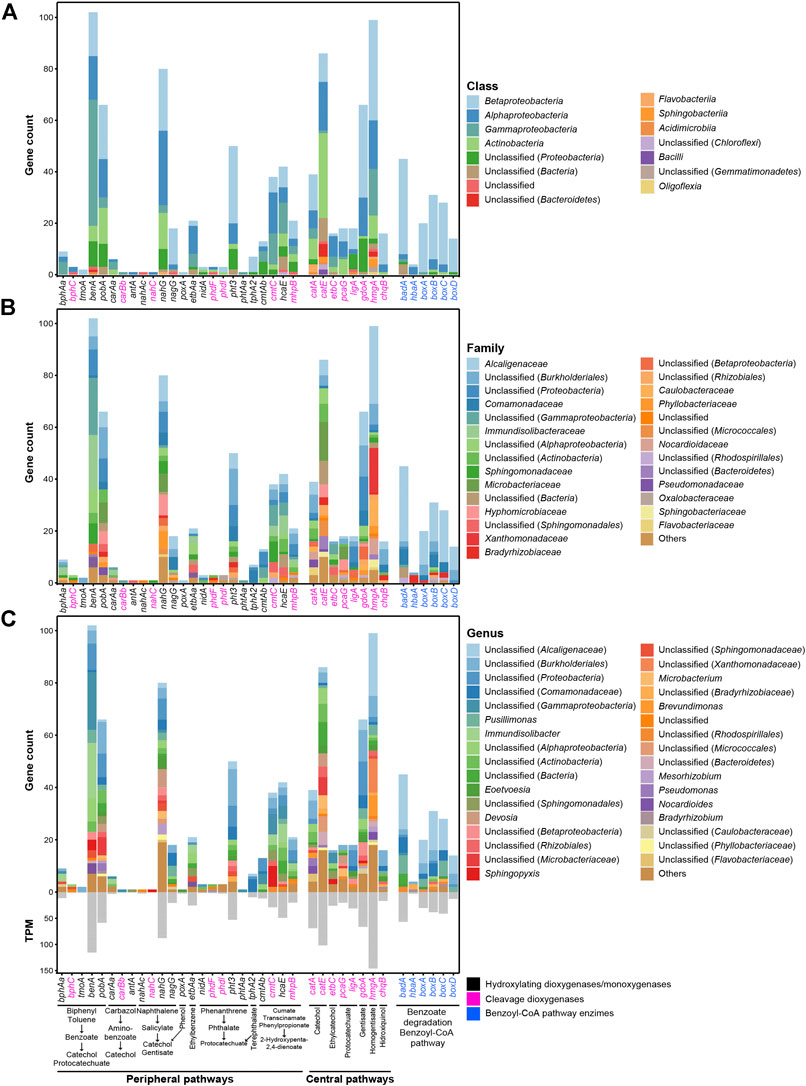
FIGURE 8. Abundance, in terms of number of genes and TPM values, of genes encoding enzymes involved in aromatic compounds degradation pathways identified in the metagenome and classified at class (A), family (B), and genus (C) levels.
In regard to central metabolism of the intermediates derived from peripheral metabolism of aromatic compounds, the most abundant ring cleavage dioxygenases found in the metagenome were catechol 1,2-dioxygenase (catA), catechol 2,3-dioxygenase (catE), gentisate 1,2-dioxygenase (gdoA), and homogentisate 1,2-dioxygenase (hmgA) with 39, 86, 66, and 99 CDSs, respectively (Figure 8; Supplementary Table S5). Protocatechuate 3,4 dioxygenase (pcaG), protocatechuate 4,5-dioxygenase (ligA), ethylbenzene 1,2-dioxygenase (etbC), and hydroxyquinol 1,2-dioxygenase (chqB) were also found in the metagenome (18, 18, 16, and 16 CDSs, respectively). Most of these central aromatic degradation enzymes that have been taxonomically classified at the family level belong to Alcaligenaceae family (especially Pusillimonas and Eoetvoesia genera), with other families such as Microbacteriaceae (Microbacterium genus), Comamonadaceae, Xanthomonadaceae, Caulobacteraceae (Brevundimonas genus), Hyphomicrobiaceae (Devosia genus), and Nocardioidaceae (Nocardioides genus) being also representatives.
Benzoate aerobic mineralization via the benzoyl-CoA pathway first involves the ligation of acetyl-CoA to benzoate by benzoate-CoA ligase (encoded by badA) and 4-hydroxybenzoate-CoA ligase (encoded by hbaA gene), which is further epoxidated by benzoyl-CoA 2,3 epoxidase (encoded by boxABCD gene cluster) (Rather et al., 2010). The enzymes responsible for this benzoate degradation pathway have been identified in the metagenome (45 CDSs of badA, 4 of hbaA, 20 of boxA, 31 of boxB, 28 of boxC, and 14 of boxD) (Figure 8; Supplementary Table S5), with most of the genes belonging to Alcaligenaceae and Comamonadaceae families.
Figure 9 summarizes the implication of the main families and genera in the different pathways for the degradation of TPHs according to the metagenomic data. It is interesting to note that several families that are relatively abundant do not seem to participate in TPHs degradation, since no genes implicated in these pathways have been found within these families. These families include Alcanivoracaceae, Sphingobacteriaceae, Acidimicrobiaceae, Oxalobacteraceae, Rhodobiaceae, Rhodanobacteraceae, Intrasporangiaceae, Micrococcaceae, and Planococcaceae. Since many of these families are among the major constituents of soils microbiota (Delgado-Baquerizo et al., 2018), they should be performing other soil functions not related with TPHs degradation.
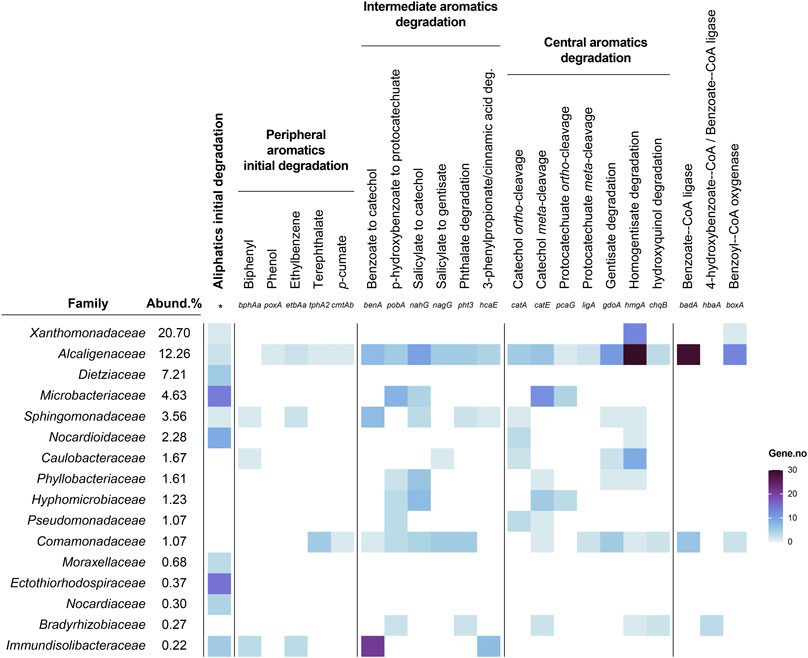
FIGURE 9. Summary of aliphatics, peripheral, intermediate, and central aromatic compounds biodegradation pathways in the most abundant families present in the metagenome. Color scale bar represents number of genes assigned to each family. *Aliphatics initial degradation consists in the sum of alkB, ladA, and pmo and prm genes.
Discussion
The introduction of inorganic and organic pollutants such as heavy metals or petroleum hydrocarbons can adversely affect both the diversity of microorganisms present in the soil and their ability to carry out these essential ecosystem roles. Organic compounds, which dominate the complex mixture of molecules represented by petroleum hydrocarbons, can provide certain soil microbes with a source of carbon for their respiration (Sima et al., 2019). Consequently, microbial communities in soils are essential in the degradation of contaminants, and the key to successful microbial bioremediation is to harness the naturally occurring catabolic capability of microbes to catalyze transformations of soil pollutants. Microbial bioremediation is a cost-effective and ecofriendly technology that provides sustainable ways to clean up contaminated soils (Ying and Wei 2019).
The current study utilized molecular tools to profile the bacterial, fungi, and nematode microbiomes present in soil contaminated with petrochemicals. High throughput sequencing of microbial communities allows for the detection of both culturable and unculturable microorganisms. This, in turn, allows for the possibility of identifying rarely studied microbes which are actively involved in decontamination of the soil and which can be further used to optimize the existing bioremediation strategies and to develop new ones (Stenuit et al., 2008).
16S rDNA analysis of the soils used in our study found high abundances of bacteria classified to Proteobacteria, Actinobacteria, and Firmicutes, and this is similar to previous reports on the bacterial microbiome studies of petrochemical-contaminated soils (Jung et al., 2016; Siles and Margesin, 2018 and Borowik et al., 2019). Lysobacter made up the largest individual genus across all 14 Ecopile samples (14–39%). This taxon has previously been reported as the most dominant genus in diesel-contaminated soils (Borowik et al., 2019), where it is observed that Lysobacter brunescens comprised as much as 29.9% relative abundance in soil samples. While we were unable to link this genus with any pollutant degradation ability, it has been suggested that abundant taxa play a dominant role in the stability and maintenance of soil microbial communities, while rare taxa served as a diverse pool to enhance both bacterial resilience and resistance under environmental disturbances (Jiao et al., 2019). With the exception of commonly reported genera such as Pseudomonas, Sphingomonas, and Mycobacteria, many of the other genera present in the Ecopile soil (taxa such as Glutamicibacter, Paeniglutamicibacter, Extensimonas, Luteimonas, and Lysinimonas) are rarely reported as dominant members of the soil community. The genus Extensimonas, for example, is a member of the family Comamonadaceae, which belongs to the Betaproteobacteria class. This family contains numerous genera, including Acidovorax, Brachymonas, and Comamonas, which are widely reported in contaminated soil microbiomes. Extensimonas, on the other hand, has rarely been reported in soil microbiomes, and there has only been a handful of studies on their involvement in hydrocarbon degradation (Zhang et al., 2013; Gauchotte-Lindsay et al., 2019). This soil therefore potentially presents a rich source of unusual species with potentially novel hydrocarbon-degrading abilities.
The mycobiome structure in the Ecopile soil mainly consisted of members of Ascomycota and Basidiomycota and a high proportion of unclassified fungal reads, which is consistent with studies of similar contaminated soils. Unsurprisingly, Ascomycota was by far the most dominant fungal phylum in each of the Ecopile soil samples. Ascomycota has been reported as the largest fungal phylum with over 64,000 identified species in almost 6400 genera and the most dominant fungal phylum in soils. Siles and Margesin (2018) reported a fungal profile in which Ascomycota made up 0.6–95.3% and Basidiomycota made up 0.7–62.7% of the soil mycobiome. Consistent with the findings in the current study, they also reported a very high incidence of unclassified fungal reads. Wang et al., (2018) also found that Ascomycota was the dominant fungal phylum (∼71%) in crude oil-contaminated soil and that the abundance of this phylum increased in the presence of oily sludge. The dominant fungal genera found in the Ecopile soil were Ochroconis (∼15%), Pseudallescheria (9%), Gaertneriomyces (9%), and Fusarium (7%), many of which have been reported to be present in contaminated soils. Borowik et al., (2017) showed that hydrocarbon-polluted soil was dominated by fungi from the genera Fusarium (37.9%), Candida (13.8%), Microsporum (13.8%), and Penicillium (13.8%), while Ogbonna et al., (2020) identified a number of hydrocarbon-degrading fungi in oil-contaminated soil including some species of Fusarium, Penicillium, Kodamaea, and Lentinus.Wu et al., (2020a) and Wu et al., (2020b) reported the presence of Ochroconis species in two different petroleum-contaminated soils in China at relative abundances of 1–5%. Aranda et al., (2015) reported the ability of Pseudallescheria isolates to degrade PAHs, and Covino et al., (2015) found that a species of Pseudallescheria was highly efficient (71% removal rates) at degrading aliphatic hydrocarbons from clayey soils. The presence of these groups of fungi in the Ecopile soil suggests that they have a possible role in hydrocarbon degradation.
Nematode communities offer a potent ecological tool for assessing soil disturbances and for the development of a biomonitoring system. There are many records of specific nematode species showing a preference for certain environmental factors as well as many studies on changes of nematode community composition in response to soil pollution (Urzelai et al., 2000). In our analysis, the primer set used to analyze the nematode communities was not specific to the phylum Nematoda. On average, just 41% of the reads were assigned to this phylum, although the percentage of reads assigned differed significantly from sample to sample (12–69%). The presence of 15 different nematode species in the Ecopile soil samples was identified, with representative species that were bacteriovorous, fungivorous, insect parasitic, and plant parasitic. Blakely et al., (2002) also observed nematodes from all tropic levels in soils that were contaminated with PAHs. Contrary to their predictions, PAHs affected nematodes communities indirectly by altering the physical habitat of the nematodes rather than the direct chemical toxicity of PAHs. Increased bulk density of soil and hydrophobic properties of PAHs decrease the amount of habitable space within soil pores affecting the mobility and hence the distribution of nematodes. Monteiro et al., (2018) studied the effect of crude, diesel, or motor oil on the survival of free-living nematodes in soil. They observed that most species exhibited moderate to extreme mortality levels in oil treatments and experienced an increased mortality with time. Species sensitivities to oil did not follow patterns of taxonomic relatedness, contradicting the idea that closely related species should intrinsically respond similarly to pollution. Out of 6 species of Rhabditidae tested, only Bursilla monhystera was highly tolerant, while C. elegans and cryptic species of Litoditis marina were among the most sensitive taxa. In contrast to their study, 60% of the nematode reads in the Ecopile samples were from the genus Rhabditida. The dominance of this group of nematodes in the Ecopile soils samples may be due to the fact that the hydrocarbon contaminants mainly consisted of aged high molecular weight compounds which tend to be less toxic than freshly contaminated soil. Monteiro et al., (2018) recommended that future effect studies do not focus on a single model species but instead incorporate multiple species for a better and more robust assessment of pollutant effects.
Metagenomic analysis presented here has shown that bacteria are the main constituents of the Ecopiles soil community, representing most of the biomass. The composition of the bacterial community at the Ecopiles soil, according to metagenomic analysis, is consistent with the composition determined in different Ecopiles by analyzing 16S amplicons, and the same genera and families were detected. Furthermore, the major genera identified are bacteria previously shown to participate in the degradation of organic pollutants. Members of the genus Lysobacter, the most abundant genus in both analyses, have been previously isolated from pesticide sludge (Ye et al., 2015), chlorothalonil-contaminated soil (Wang et al., 2011), and oil-contaminated soil (Chaudhary et al., 2017). Bacteria affiliated to other abundant genera, such as the genus Dietzia, have also been isolated from petroleum-contaminated soils (Von der Weid et al., 2007; Bihari et al., 2010) and oil reservoirs worldwide (Bødtker et al., 2009; Wang et al., 2011), and several Dietzia strains described to date have been shown to degrade a wide variety of aliphatic hydrocarbons with chain lengths that range from C6 to C40 (Bihari et al., 2010; Wang et al., 2011), aromatic compounds (Von der Weid et al., 2007; Fathi et al., 2016), and conventional crude oil (Wang et al., 2011). Oil-degrading bacteria belonging to the genus Pusillimonas have been found in petroleum-contaminated sites (Cao et al., 2011; Obi et al., 2016), and the ability of some members of this genus to metabolize alkanes and aromatic compounds has been reported (Stolz et al., 2005; Li et al., 2013; Obi et al., 2016).
The identification and phylogenetic assignation of genes encoding the main enzymes participating in each of the pathways have allowed us to model the main degradation routes for aliphatic and aromatic compounds. Regarding aliphatic hydrocarbons degradation, the main role in degradation appears to be performed by bacteria from the Ectothiorhodospiraceae, Microbacteriaceae, and Nocardioidaceae families and to some extent by the Immundisolibacteraceae, Nocardiaceae, Moraxellaceae, and Sphingomonadaceae families. The relevance of Ectothiorhodospiraceae can be attributed to the degradation of short chain alkanes since most of the pmoABC genes found in the metagenome were classified to the Ectothiorhodospiraceae family and have been further assigned to “Candidatus Macondimonas” at the genus level. “Candidatus Macondimonas” was proposed by Karthikeyan et al., (2019) as a novel genus within Gammaproteobacteria. The type strain, “Ca. Macondimonas diazotrophica” KTK01T, was isolated from crude oil-contaminated sediments. The limited presence of sMMO genes compared to pMMO is in agreement with previous works, since whereas pMMO is ubiquitous in methanotrophic bacteria, sMMO has been isolated from only certain strains of methanotrophs (Hanson and Hanson, 1996). The other families seem to participate in the degradation of medium to long chain alkanes. Their role in alkane degradation is not unexpected. The isolation of bacterial strains with hydrocarbon-degrading capabilities from oil-contaminated sites has been reported for Nocardioides (Jung et al., 2002), Williamsia (Obuekwe et al., 2009), and Microbacterium (Ali et al., 2020) genera. Moreover, regarding the genus Nocardioides, genes encoding alkane monooxygenases have been previously identified in several strains (Kimbrel et al., 2013; Brown et al., 2017). On the other hand, the Immundisolibacter genus was first described by Corteselli et al., (2017), and I. cernigliae T3.2T was isolated from a PAH-contaminated bioreactor-treated soil and described as a high-molecular-weight PAH-degrading bacterium. Although the presence of genes encoding alkane monooxygenases in I. cernigliae has not been reported before, the analysis of a metagenome, derived from a polluted sediment dominated by I. cernigliae, showed several copies of putative n-alkane-degrading genes alkB and ladA (Gacesa et al., 2018).
Regarding the degradation of aromatic compounds, only five families appear to be participating in peripheric (upper) pathways. These families are Alcaligenaceae, Sphingomonadaceae, Caulobacteraceae, Comamonadaceae, and Immundisolibacteraceae, all of them belonging to the Proteobacteria phylum. The majority of the formally described genera of aromatic hydrocarbon-degrading bacteria belong to the large Proteobacteria phylum (Prince et al., 2019). Within Betaproteobacteria, Alcaligenaceae and Comamonadaceae are the most representative families harboring aromatic compound-degrading genes identified in the metagenome. Regarding the Alcaligenaceae family, the classification of several copies of different genes belonging to both peripheral and central pathways and the benzoyl-CoA pathway to the genera Pusillimonas and Eoetvoesia are significant (Figure 8). Moreover, the genus Pusillimonas is one of the most represented genera within the general taxonomic profile of the metagenome, and its aromatic hydrocarbon-degrading capability has been previously described (Stolz et al., 2005). The presence of the genus Eoetvoesia in petroleum-contaminated soil has also been reported (Liu et al., 2020). On the other hand, most genes assigned to the Comamonadaceae family have not been further classified at the genus level, but it is worth mentioning that several copies of different genes involved in the three types of pathways represented belong to this family. Some of the most common genera within Betaproteobacteria which are known to degrade hydrocarbons and related substituted molecules under aerobic conditions belong to Comamonadaceae family, including Acidovorax, Comamonas, Delftia, and Polaromonas (Tan and Parales, 2019). Furthermore, in a study reported by Mukherjee et al., (2017), Comamonadaceae was found to make significant contribution to the abundance of hydrocarbon degradation genes in oil sand tailing ponds samples.
Within Alphaproteobacteria, Sphingomonadaceae and Hyphomicrobiaceae are the most representative families. Members of Sphingomonadaceae have been known for many years as degraders of aromatic hydrocarbons (many belonging to Sphingomonas, Sphingobium, Novosphingobium, and Sphingopyxis genera) and particularly of PAHs in contaminated soil environments (Kertesz et al., 2019). Specifically, Sphingopyxis was found to be one of the genera with a greater number of genes encoding aromatic-degrading enzymes identified in the metagenome (Figure 8). The genetic potential for the degradation of aromatic compounds of Sphingopyxis has been previously analyzed (Yang et al., 2020). The genes assigned to Hyphomicrobiaceae family mostly belong to the genus Devosia. The growth of some strains of Devosia with aromatic compounds as the sole carbon and energy source has been previously reported, for instance, with naphthalene (Chen et al., 2019) and fluoranthene (Zhao et al., 2016).
Within Gammaproteobacteria, Immundisolibacter is the most representative genus regarding aromatic compound degradation found in the metagenome, although it should be noted that all the genes belonging to this genus are only involved in peripheral pathways. The PAH-degrading capability of Immundisolibacter has been previously described (Corteselli et al., 2017).
In addition to the Proteobacteria phylum, another relevant taxon in the metagenome is Actinobacteria and within this phylum, the Microbacteriaceae family. Specifically, Microbacterium is one of the genera present in the metagenome with more aromatic hydrocarbon-degrading genes. The degradation of different PAHs by Microbacterium members has been reported (Salam et al., 2014; Jin et al., 2017). The presence of these genes within the soil microbiome suggests that the indigenous soil microflora have significant potential for degrading the petroleum-derived contaminants.
Conclusion
Microbe-assisted phytoremediation promises to be the most sustainable and environmentally friendly approach for removing organic contaminants from polluted soils/sediments. Ecopiling utilizes both biostimulation of the indigenous pollutant-degrading microbial community and microbe-assisted phytoremediation to remove petroleum hydrocarbons and PAHs from contaminated soils. Our analysis of the initial Ecopile soil found that it contained diverse bacterial and fungal communities, but these communities were dominated by a small number of genera. Lysobacter was the single most abundant bacterial genus across all 14 soil samples (based on 16S microbiome data). This was also observed in the metagenome data where 10% of the CDSs were assigned to this genus. Although our ability to draw conclusions is limited due to the low genome coverage, this group does not seem to have a major role in hydrocarbon degradation as no genes involved in aliphatic or aromatic catabolic pathways were assigned to this group. On the other hand, less abundant genera of bacteria such as Pusillimonas, Dietzia, and Bradyrhizobium were found to be associated with hydrocarbon degradation genes in the metagenome. These groups represent possible novel targets for isolation and development into efficient consortia for bioaugmentation of hydrocarbon-contaminated soil.
Data Availability Statement
The datasets presented in this study can be found in online repositories. The names of the repository/repositories and accession number(s) can be found at: <br>https://www.ncbi.nlm.nih.gov/. Sequence data generated in this study are deposited in the National Centre for Biotechnology Information (NCBI) under the bioproject number PRJNA664743. Bacterial 16S data sets are archived under biosample numbers SAMN16231369-16231382, fungal 18S data sets under SAMN16233626-16233639, and nematode 18S data sets under SAMN16234236-16234249. Metagenomic sequence data have been deposited in the NCBI Sequence Read Archive (SRA) under the accession number SRR12703469. </b>.
Funding
This project has received funding from the European Union’s Horizon 2020 research and innovation programme “GREENER” under grant agreement number 826312 and the Institute of Technology Carlow Postgraduate Presidents Awards.
Author Contributions
MW, DG-S, PS-L, and MR-N carried out the microbiome and metagenome analysis. RC and RM preformed the physicochemical analysis. XL and KG set up the Ecopiling experiment. MM, DD, RR, XL, and KG were involved in the experimental design and preparation of the manuscript.
Conflict of Interest
RM and XL were employed by Microgen Biotech Ltd.
The remaining authors declare that the research was conducted in the absence of any commercial or financial relationships that could be construed as a potential conflict of interest.
Microgen Biotech Ltd. was remunerated by the owners of the industrial site for the services of designing and monitoring the Ecopiles described in this manuscript. The project had oversight from the Irish Environmental Protection Agency as part of the industrial sites remedial action plan under its waste management license.
Supplementary Material
The Supplementary Material for this article can be found online at: https://www.frontiersin.org/articles/10.3389/fenvs.2021.624070/full#supplementary-material.
References
Ali, N., Dashti, N., Khanafer, M., Al-Awadhi, H., and Radwan, S. (2020). Bioremediation of soils saturated with spilled crude oil. Sci. Rep. 10, 1–9. doi:10.1038/s41598-019-57224-x
Aranda, E., Godoy, P., Reina, R., Badia-Fabregat, M., Rosell, M., Marco-Urrea, E., et al. (2015). Isolation of Ascomycota fungi with capability to transform PAHs: insights into the biodegradation mechanisms of Penicillium oxalicum. Int. Biodeteriora. Biodegrad. 112, 141–150. doi:10.1016/j.ibiod.2017.05.015
Baoune, H., Aparicio, J. D., Acuña, A., El Hadj-khelil, A. O., Sanchez, L., Polti, M. A., et al. (2019). Effectiveness of the Zea mays-Streptomyces association for the phytoremediation of petroleum hydrocarbons impacted soils. Ecotoxicol. Environ. Saf. 184, 109591. doi:10.1016/j.ecoenv.2019.109591
Bhadury, P., Austen, M., Bilton, D., Lambshead, P., Rogers, A., and Smerdon, G. (2006). Development and evaluation of a DNA-barcoding approach for the rapid identification of nematodes. Mar. Ecol. Prog. Ser. 320, 1–9. doi:10.3354/meps320001
Bihari, Z., Szabó, Z., Szvetnik, A., Balázs, M., Bartos, P., Tolmacsov, P., et al. (2010). Characterization of a novel long-chain n-alkane-degrading strain, Dietzia sp. E1. Z. Naturforsch, C, J. Biosci. 65, 693–700. doi:10.1515/znc-2010-11-1210
Blakely, J. K., Neher, D. A., and Spongberg, A. L. (2002). Soil invertebrate and microbial communities, and decomposition as indicators of polycyclic aromatic hydrocarbon contamination. Appl. Soil Ecol. 21, 71–88. doi:10.1016/s0929-1393(02)00023-9
Bødtker, G., Hvidsten, I. V., Barth, T., and Torsvik, T. (2009). Hydrocarbon degradation by Dietzia sp. A14101 isolated from an oil reservoir model column. Antonie Van Leeuwenhoek 96, 459–469. doi:10.1007/s10482-009-9359-y
Bokulich, N. A., Subramanian, S., Faith, J. J., Gevers, D., Gordon, J. I., Knight, R., et al. (2013). Quality-filtering vastly improves diversity estimates from Illumina amplicon sequencing. Nat. Methods 10 (1), 57–59. doi:10.1038/nmeth.2276
Bolger, A. M., Lohse, M., and Usadel, B. (2014). Trimmomatic: a flexible trimmer for Illumina sequence data. Bioinformatics 30 (15), 2114–2120. doi:10.1093/bioinformatics/btu170
Borowik, A., Wyszkowska, J., and Oszust, K. (2017). Functional diversity of fungal communities in soil contaminated with diesel oil. Front. Microbiol. 8, 1862. doi:10.3389/fmicb.2017.01862
Borowik, A., Wyszkowska, J., Kucharski, M., and Kucharski, J. (2019). Implications of soil pollution with diesel oil and BP petroleum with active technology for soil health. Ijerph 16, 2474. doi:10.3390/ijerph16142474
Breitwieser, F. P., and Salzberg, S. L. (2016). Pavian: interactive analysis of metagenomics data for microbiomics and pathogen identification. Bioinformatics 36 (4), 1303–1304. doi:10.1093/bioinformatics/btz715
Brown, L. M., Gunasekera, T. S., and Ruiz, O. N. (2017). Draft genome sequence of Nocardioides luteus strain BAFB, an alkane-degrading bacterium isolated from JP-7-polluted soil. Genome. Announc. 5 (4), e01529–16. doi:10.1128/genomeA.01529-16
Buchfink, B., Xie, C., and Huson, D. H. (2015). Fast and sensitive protein alignment using diamond. Nat. Methods 12 (1), 59–60. doi:10.1038/nmeth.3176
Cao, B., Ma, T., Ren, Y., Ren, Y., Li, G., Li, P., et al. (2011). Complete genome sequence of Pusillimonas sp. T7-7, a cold-tolerant diesel oil-degrading bacterium isolated from the Bohai Sea in China. J. Bacteriol. 193, 4021–4022. doi:10.1128/JB.05242-11
Caporaso, J. G., Kuczynski, J., Stombaugh, J., Bittinger, K., Bushman, F. D., Costello, E. K., et al. (2010). QIIME allows analysis of high-throughput community sequencing data. Nat. Methods 7 (5), 335–336. doi:10.1038/nmeth.f.303
Chaudhary, D. K., Lee, S. D., and Kim, J. (2017). Lysobacter olei sp. nov., isolated from oil-contaminated soil. Int. J. Syst. Evol. Microbiol. 67, 4660–4666. doi:10.1099/ijsem.0.002348
Chen, Y., Zhu, S., Lin, D., Wang, X., Yang, J., and Chen, J. (2019). Devosia naphthalenivorans sp. nov., isolated from East Pacific Ocean sediment. Int. J. Syst. Evol. Microbiol. 69, 1974–1979. doi:10.1099/ijsem.0.003410
Corteselli, E. M., Aitken, M. D., and Singleton, D. R. (2017). Description of Immundisolibacter cernigliae gen. nov., sp. nov., a high-molecular-weight polycyclic aromatic hydrocarbon-degrading bacterium within the class Gammaproteobacteria, and proposal of Immundisolibacterales ord. nov. and Immundisolibacteraceae fam. nov. Int. J. Syst. Evol. Microbiol. 67, 925. doi:10.1099/ijsem.0.001714
Covino, S., D’Annibale, A., Stazi, S. R., Cajthaml, T., Čvančarová, M., Stella, T., et al. (2015). Assessment of degradation potential of aliphatic hydrocarbons by autochthonous filamentous fungi from a historically polluted clay soil. Sci. Total Environ. 505, 545–554. doi:10.1016/j.scitotenv.2014.10.027
Delgado-Baquerizo, M., Oliverio, A. M., Brewer, T. E., Benavent-González, A., Eldridge, D. J., Bardgett, R. D., et al. (2018). A global atlas of the dominant bacteria found in soil. Science 359 (6373), 320–325. doi:10.1126/science.aap9516
Devarapalli, P., and Kumavath, R. N. (2015). “Metagenomics- a technological drift in bioremediation,” in Advances in bioremediation of wastewater and polluted soil. Editor N. Shiomi (Europe: InTech). doi:10.5772/60749
Devatha, C. P., Vishnu Vishal, A., and Purna Chandra Rao, J. (2019). Investigation of physical and chemical characteristics on soil due to crude oil contamination and its remediation. Appl. Water Sci. 9 (4), 89. doi:10.1007/s13201-019-0970-4
Eddy, S. R. (2009). A new generation of homology search tools based on probabilistic inference. Genome Inform. 23, 205–211. doi:10.1142/9781848165632_0019
Edgar, (2004). MUSCLE: multiple sequence alignment with high accuracy and high throughput. Nucleic Acids Res. 32 (5), 1792–1797. doi:10.1093/nar/gkh340
Edgar, R. C., Haas, B. J., Clemente, J. C., Quince, C., and Knight, R. (2011). UCHIME improves sensitivity and speed of chimera detection. Bioinformatics 27, 2194–2200. doi:10.1093/bioinformatics/btr381
Ekschmitt, K., and Korthals, G. W. (2006). Nematodes as sentinels of heavy metals and organic toxicants in the soil. J. Nematol. 38 (1), 13–19.
Fathi, Z., Ebrahimipour, G., Kavyanifard, A., and Najmi, Z. (2016). Optimization of phenanthrene-degradation by Dietzia cinnamea AP for bioremediation applications. Res. Mol. Med. 4 (3), 22–28. doi:10.18869/acadpub.rmm.4.3.22
Ferraro, D. J., Okerlund, A., Brown, E., and Ramaswamy, S. (2017). One enzyme, many reactions: structural basis for the various reactions catalyzed by naphthalene 1,2-dioxygenase. IUCrJ 4 (5), 648–656. doi:10.1107/S2052252517008223
Finn, R. D., Bateman, A., Clements, J., Coggill, P., Eberhardt, R. Y., Eddy, S. R., et al. (2014). Pfam: the protein families database. Nucleic Acids Res. 42 (D1), D222–D230. doi:10.1093/nar/gkt1223
Gacesa, R., Baranasic, D., Starcevic, A., Diminic, J., Korlević, M., Najdek, M., et al. (2018). Bioprospecting for genes encoding hydrocarbon-degrading enzymes from metagenomic samples isolated from northern Adriatic sea sediments. Food Technol. Biotechnol. 56, 270–277. doi:10.17113/ftb.56.02.18.5393
Gauchotte-Lindsay, C., Aspray, T. J., Knappc, M., and Ijaz, U. Z. (2019). A Systems biology approach to elucidation of contaminant biodegradation in complex samples – integration of high-resolution analytical and molecular tools. Faraday Discuss. 218, 481–504. doi:10.1039/c9fd00020h
Germaine, K. J., Byrne, J., Liu, X., Keohane, J., Culhane, J., Lally, R. D., et al. (2015). Ecopiling: a combined phytoremediation and passive biopiling system for remediating hydrocarbon impacted soils at field scale. Front. Plant Sci. 5, 756. doi:10.3389/fpls.2014.00756
Girsowicz, R., Koryachenko, O., Sherman, C., Mayzlish-Gati, E., Doniger, T., and Steinberger, Y. (2018). Impact of oil-spill contamination on a soil bacterial community: a 40-year history of rehabilitation in the Arava valley. Soil Sediment. Contamination: Int. J. 27, 175–185. doi:10.1080/15320383.2018.1443427
Gurevich, A., Saveliev, V., Vyahhi, N., and Tesler, G. (2013). Quast: quality assessment tool for genome assemblies. Bioinformatics 29, 1072–1075. doi:10.1093/bioinformatics/btt086
Haas, B. J., Gevers, D., Earl, A. M., Feldgarden, M., Ward, D. V., Giannoukos, G., et al. (2011). Chimeric 16S rRNA sequence formation and detection in Sanger and 454-pyrosequenced PCR amplicons. Genome Res. 21, 494–504. doi:10.1101/gr.112730.110
Hanson, R. S., and Hanson, T. E. (1996). Methanotrophic bacteria. Microbiol. Rev. 60 (2), 439–471. doi:10.1128/mmbr.60.2.439-471.1996
Hou, N., Zhang, N., Jia, T., Sun, Y., Dai, Y., Wang, Q., et al. (2018). Biodegradation of phenanthrene by biodemulsifier-producing strain Achromobacter sp. LH-1 and the study on its metabolisms and fermentation kinetics. Ecotoxicol. Environ. Saf. 163, 205–214. doi:10.1016/j.ecoenv.2018.07.064
Huerta-Cepas, J., Szklarczyk, D., Forslund, K., Cook, H., Heller, D., Walter, M. C., et al. (2016). eggNOG 4.5: a hierarchical orthology framework with improved functional annotations for eukaryotic, prokaryotic and viral sequences. Nucleic Acids Res. 44 (D1), D286–D293. doi:10.1093/nar/gkv1248
Hyatt, D., Chen, G. L., LoCascio, P. F., Land, M. L., Larimer, F. W., and Hauser, L. J. (2010). Prodigal: prokaryotic gene recognition and translation initiation site identification. BMC bioinform. 11 (1), 119. doi:10.1186/1471-2105-11-119
Ji, Y., Mao, G., Wang, Y., and Bartlam, M. (2013). Structural insights into diversity and n-alkane biodegradation mechanisms of alkane hydroxylases. Front. Microbiol. 4, 58. doi:10.3389/fmicb.2013.00058
Jiao, S., Wang, J., WeiChen, G. W., Chen, W., and Lu, Y. (2019). Dominant role of abundant rather than rare bacterial taxa in maintaining agro-soil microbiomes under environmental disturbances. Chemosphere 235, 248–259. doi:10.1016/j.chemosphere.2019.06.174
Jin, J., Yao, J., Liu, W., Zhang, Q., and Liu, J. (2017). Fluoranthene degradation and binding mechanism study based on the active-site structure of ring-hydroxylating dioxygenase in Microbacterium paraoxydans JPM1. Environ. Sci. Pollut. Res. Int. 24, 363–371. doi:10.1007/s11356-016-7809-4
Johnston, E. R., Rodriguez-R, L. M., Luo, C., Yuan, M. M., Wu, L., He, Z., et al. (2016). Metagenomics reveals pervasive bacterial populations and reduced community diversity across the Alaska tundra ecosystem. Front. Microbiol. 7, 579. doi:10.3389/fmicb.2016.00579
Jung, C. M., Broberg, C., Giuliani, J., Kirk, L. L., and Hanne, L. F. (2002). Characterization of JP-7 jet fuel degradation by the bacterium Nocardioides luteus strain BAFB. J. Basic Microbiol. 42, 127–131. doi:10.1002/1521-4028(200205)42:2<127::AID-JOBM127>3.0.CO;2-C
Jung, J., Philippot, L., and Park, W. (2016). Metagenomic and functional analyses of the consequences of reduction of bacterial diversity on soil functions and bioremediation in diesel-contaminated microcosms. Sci. Rep. 6, 23012. doi:10.1038/srep23012
Kanehisa, M., and Goto, S. (2000). KEGG: kyoto encyclopedia of genes and genomes. Nucleic Acids Res. 28, 27–30. doi:10.1093/nar/28.1.27
Karthikeyan, S., Rodriguez-R, L. M., Heritier-Robbins, P., Kim, M., Overholt, W. A., Gaby, J. C., et al. (2019). "Candidatus Macondimonas diazotrophica," a novel gammaproteobacterial genus dominating crude-oil-contaminated coastal sediments. ISME J. 13, 2129–2134. doi:10.1038/s41396-019-0400-5
Kertesz, M. A., Kawasaki, A., and Stolz, A. (2019). “Aerobic hydrocarbon-degrading Alphaproteobacteria: sphingomonadales,” in Taxonomy, genomics and ecophysiology of hydrocarbon-degrading microbes. Editor T. J. McGenity (New York, NY: Springer), 105–124.
Khan, M. A. I., Biswas, B., Smith, E., Naidu, R., and Megharaj, M. (2018). Toxicity assessment of fresh and weathered petroleum hydrocarbons in contaminated soil- a review. Chemosphere 212, 755. doi:10.1016/j.chemosphere.2018.08.094
Kimbrel, J. A., Chang, J., Arp, D. J., and Sayavedra-Soto, L. A. (2013). The draft genome sequence of Nocardioides sp. Strain CF8 reveals the scope of its metabolic capabilities. Genome Announc. 1 (4), e00439-13. doi:10.1128/genomeA.00439-13
Kok, M., Oldenhuis, R., Van Der Linden, M. P., Raatjes, P., Kingma, J., van Lelyveld, P. H., et al. (1989). The Pseudomonas oleovorans alkane hydroxylase gene. Sequence and expression. J. Biol. Chem. 264 (10), 5435–5441. doi:10.1016/s0021-9258(18)83564-5
Kotani, T., Yamamoto, T., Yurimoto, H., Sakai, Y., and Kato, N. (2003). Propane monooxygenase and NAD+-dependent secondary alcohol dehydrogenase in propane metabolism by Gordonia sp. Strain TY-5. J. Bacteriol. 185, 7120–7128. doi:10.1128/jb.185.24.7120-7128.2003
Kotani, T., Yurimoto, H., Kato, N., and Sakai, Y. (2007). Novel acetone metabolism in a propane-utilizing bacterium, Gordonia sp. Strain TY-5. J. Bacteriol. 189, 886–893. doi:10.1128/JB.01054-06
Langmead, B., and Salzberg, S. L. (2012). Fast gapped-read alignment with Bowtie 2. Nat. Methods 9 (4), 357–359. doi:10.1038/nmeth.1923
Li, L., Liu, X., Yang, W., Xu, F., Wang, W., Feng, L., et al. (2008). Crystal structure of long-chain alkane monooxygenase (LadA) in complex with coenzyme FMN: unveiling the long-chain alkane hydroxylase. J. Mol. Biol. 376, 453–465. doi:10.1016/j.jmb.2007.11.069
Li, P., Wang, L., and Feng, L. (2013). Characterization of a novel Rieske-type alkane monooxygenase system in Pusillimonas sp. Strain T7-7. J. Bacteriol. 195, 1892–1901. doi:10.1128/JB.02107-12
Liu, H., Gao, H., Wu, M., Ma, C., Wu, J., and Ye, X. (2020). Distribution characteristics of bacterial communities and hydrocarbon degradation dynamics during the remediation of petroleum-contaminated soil by enhancing moisture content. Microb. Ecol. 1–10. doi:10.1007/s00248-019-01476-7
Luo, C., Rodriguez-R, L. M., and Konstantinidis, K. T. (2014). MyTaxa: an advanced taxonomic classifier for genomic and metagenomic sequences. Nucleic Acids Res. 42 (8), e73. doi:10.1093/nar/gku169
Magoč, T., and Salzberg, S. L. (2011). FLASH: fast length adjustment of short reads to improve genome assemblies. Bioinformatics 27, 2957–2963. doi:10.1093/bioinformatics/btr507
Monteiro, L., Traunspurger, W., RoeleveldLynen, K. F., Lynen, F., and Moens, T. (2018). Direct toxicity of the water-soluble fractions of a crude and a diesel-motor oil on the survival of free-living nematodes. Ecol. Indicators 93, 13–23. doi:10.1016/j.ecolind.2018.04.066
Mukherjee, A., Chettri, B., Langpoklakpam, J. S., Basak, P., Prasad, A., Mukherjee, A. K., et al. (2017). Bioinformatic approaches including predictive metagenomic profiling reveal characteristics of bacterial response to petroleum hydrocarbon contamination in diverse environments. Sci. Rep. 7, 1108–1122. doi:10.1038/s41598-017-01126-3
Nurk, S., Meleshko, D., Korobeynikov, A., and Pevzner, P. A. (2017). metaSPAdes: a new versatile metagenomic assembler. Genome Res. 27, 824–834. doi:10.1101/gr.213959.116
Obi, L. U., Atagana, H. I., and Adeleke, R. A. (2016). Isolation and characterisation of crude oil sludge degrading bacteria. SpringerPlus 5 (1), 1946. doi:10.1186/s40064-016-3617-z
Obuekwe, C. O., Al-Jadi, Z. K., and Al-Saleh, E. S. (2009). Hydrocarbon degradation in relation to cell-surface hydrophobicity among bacterial hydrocarbon degraders from petroleum-contaminated Kuwait desert environment. Int. Biodeterioration Biodegrad. 63, 273–279. doi:10.1016/j.ibiod.2008.10.004
Ogbonna, D. N., Douglas, S. I., and Awari, V. G. (2020). Characterization of hydrocarbon utilizing bacteria and fungi associated with crude oil contaminated soil. Mrji 30, 54–69. doi:10.9734/mrji/2020/v30i530221
Prince, R. C., Amande, T. J., and McGenity, T. J. (2019). “Prokaryotic hydrocarbon degraders,” in Taxonomy, genomics and ecophysiology of hydrocarbon-degrading microbes. Editor T. J. McGenity (New York, NY: Springer), 1–39.
Quast, C., Pruesse, E., Yilmaz, P., Gerken, J., Schweer, T., Yarza, P., et al. (2013). The SILVA ribosomal RNA gene database project: improved data processing and web-based tools. Nucleic Acids Res. 41, D590–D596. doi:10.1093/nar/gks1219
Quinlan, A. R., and Hall, I. M. (2010). BEDTools: a flexible suite of utilities for comparing genomic features. Bioinformatics 26, 841–842. doi:10.1093/bioinformatics/btq033
Quintella, C., Mata, A. M. T., and Lima, L. C. P. (2019). Overview of bioremediation with technology assessment and emphasis on fungal bioremediation of oil contaminated soils. J. Environ. Manage. 241, 156–166. doi:10.1016/j.jenvman.2019.04.019
Ramadass, K., Mallavarapu, M., Kadiyala, , and Naidu, R. (2018). Bioavailability of weathered hydrocarbons in engine oil-contaminated soil: impact of bioaugmentation mediated by Pseudomonas spp. on bioremediation. Sci. Total Environ. 636, 968–974. doi:10.1016/j.scitotenv.2018.04.379
Rather, L. J., Knapp, B., Haehnel, W., and Fuchs, G. (2010). Coenzyme A-dependent aerobic metabolism of benzoate via epoxide formation. J. Biol. Chem. 285 (27), 20615–20624. doi:10.1074/jbc.M110.124156
Resnick, S., Lee, K., and Gibson, D. (1996). Diverse reactions catalyzed by naphthalene dioxygenase fromPseudomonas sp strain NCIB 9816. J. Ind. Microbiol. Biotechnol. 17, 438–457. doi:10.1007/bf01574775
Rodriguez-R, L. M., Gunturu, S., Tiedje, J. M., Cole, J. R., and Konstantinidis, K. T. (2018). Nonpareil 3: fast estimation of metagenomic coverage and sequence diversity. Msystems 3 (3), e00039-18. doi:10.1128/mSystems.00039-18
Rodriguez-R, L. M., and Konstantinidis, K. T. (2014). Estimating coverage in metagenomic data sets and why it matters. ISME J. 8 (11), 2349–2351. doi:10.1038/ismej.2014.76
Salam, L. B., Obayori, O. S., and Olatoye, N. O. (2014). Biodegradation of anthracene by a novel actinomycete, Microbacterium sp. Isolated from tropical hydrocarbon-contaminated soil. World J. Microbiol. Biotechnol. 30, 335–341. doi:10.1007/s11274-013-1437-7
Seemann, T. (2014). Prokka: rapid prokaryotic genome annotation. Bioinformatics 30 (14), 2068–2069. doi:10.1093/bioinformatics/btu153
Siles, J. A., and Margesin, R. (2018). Insights into microbial communities mediating the bioremediation of hydrocarbon-contaminated soil from an Alpine former military site. Appl. Microbiol. Biotechnol. 102 (10), 4409–4421. doi:10.1007/s00253-018-8932-6
Sima, N. A. K., Ebadia, A., Reiahisamania, N., and Rasekhb, B. (2019). Bio-based remediation of petroleum-contaminated saline soils: challenges, the current state-of-the-art and future prospects. J. Environ. Manage. 250, 109476. doi:10.1016/j.jenvman.2019.109476
Stenuit, B., Eyers, L., Schuler, L., Agathos, S. N., and George, I. (2008). Emerging high-throughput approaches to analyze bioremediation of sites contaminated with hazardous and/or recalcitrant wastes. Biotechnol. Adv. 26, 561–575. doi:10.1016/j.biotechadv.2008.07.004
Stolz, A., Bürger, S., Kuhm, A., Kämpfer, P., and Busse, H. J. (2005). Pusillimonas noertemannii gen. nov., sp. Nov., a new member of the family Alcaligenaceae that degrades substituted salicylates. Int. J. Syst. Evol. Microbiol. 55, 1077–1081. doi:10.1099/ijs.0.63466-0
Tamames, J., and Puente-Sánchez, F. (2018). SqueezeMeta, a highly portable, fully automatic metagenomic analysis pipeline. Front. Microbiol. 9, 3349. doi:10.3389/fmicb.2018.03349
Tan, W. A., and Parales, R. E. (2019). “Hydrocarbon degradation by Betaproteobacteria,” in Taxonomy, genomics and ecophysiology of hydrocarbon-degrading microbes. Editor T. J. McGenity (New York, NY: Springer), 125–141.
Truskewycz, A., Gundry, T. D., Khudur, L. S., Kolobaric, A., Taha, M., Aburto-Medina, A., et al. (2019). Petroleum hydrocarbon contamination in terrestrial ecosystems-fate and microbial responses. Molecules 24 (18), 3400. doi:10.3390/molecules24183400
Urzelai, A., Hernández, A. J., Jesus Pastor, J., and Pastor, J. (2000). Biotic indices based on soil nematode communities for assessing soil quality in terrestrial ecosystems. Sci. Total Environ. 247, 253–261. doi:10.1016/s0048-9697(99)00494-5
Van Beilen, J. B., Wubbolts, M. G., and Witholt, B. (1994). Genetics of alkane oxidation by Pseudomonas oleovorans. Biodegradation 5 (3–4), 161–174. doi:10.1007/BF00696457
Van Beilen, J. B., Li, Z., Duetz, W. A., Smits, T. H. M., and Witholt, B. (2003). Diversity of alkane hydroxylase systems in the environment. Oil Gas Sci. Tech.-Rev. IFP 58 (4), 427–440. doi:10.2516/ogst:2003026
Von der Weid, I., Marques, J. M., Cunha, C. D., Lippi, R. K., dos Santos, S. C., Rosado, A. S., et al. (2007). Identification and biodegradation potential of a novel strain of Dietzia cinnamea isolated from a petroleum-contaminated tropical soil. Syst. Appl. Microbiol. 30 (4), 331–339. doi:10.1016/j.syapm.2006.11.001
Wang, G. L., Wang, L., Chen, H. H., Shen, B., Li, S. P., and Jiang, J. D. (2011). Lysobacter ruishenii sp. Nov., a chlorothalonil-degrading bacterium isolated from a long-term chlorothalonil-contaminated soil. Int. J. Syst. Evol. Microbiol. 61 (3), 674–679. doi:10.1099/ijs.0.020990-0
Wang, H., Kuang, S., Lang, Q., and Yu, W. (2018). Effects of aged oil sludge on soil physicochemical properties and fungal diversity revealed by high-throughput sequencing analysis. Archaea 2018, 9264259. doi:10.1155/2018/9264259
Wang, Q., Garrity, G. M., Tiedje, J. M., and Cole, J. R. (2007). Naive Bayesian classifier for rapid assignment of rRNA sequences into the new bacterial taxonomy. Appl. Environ. Microbiol. 73 (16), 5261–5267. doi:10.1128/AEM.00062-07
Wang, Y., Chen, H., Chen, H., and Wu, J. (2009). Influences of chronic contamination of oil field exploitation on soil nematode communities at the Yellow River Delta of China. Front. Biol. China 4, 376–383. doi:10.1007/s11515-009-0030-9
Wickham, H. (2011). Product plots. IEEE Trans. Vis. Comput. Graph 17 (2), 2223–2230. doi:10.1109/TVCG.2011.227
Wu, M., Guo, X., Wu, J., and Chen, K. (2020a). Effect of compost amendment and bioaugmentation on PAH degradation and microbial community shifting in petroleum-contaminated soil. Chemosphere 256, 126998. doi:10.1016/j.chemosphere.2020.126998
Wu, M., Ma, C., Wang, D., Liu, H., Zhu, C., and Xu, H. (2020b). Nutrient drip irrigation for refractory hydrocarbon removal and microbial community shift in a historically petroleum-contaminated soil. Sci. Total Environ. 713, 136331. doi:10.1016/j.scitotenv.2019.136331
Xu, X., Liu, W., Tian, S., Wang, W., Qi, Q., Jiang, P., et al. (2018). Petroleum hydrocarbon-degrading bacteria for the remediation of oil pollution under aerobic conditions: a perspective analysis. Front. Microbiol. 9, 2885. doi:10.3389/fmicb.2018.02885
Yang, F., Feng, H., Massey, I. Y., Huang, F., Guo, J., and Zhang, X. (2020). Genome-wide analysis reveals genetic potential for aromatic compounds biodegradation of Sphingopyxis. Biomed. Res. Int. 2020, 5849123. doi:10.1155/2020/5849123
Ye, X. M., Chu, C. W., Shi, C., Zhu, J. C., He, Q., and He, J. (2015). Lysobacter caeni sp. nov., isolated from the sludge of a pesticide manufacturing factory. Int. J. Syst. Evol. Microbiol. 65 (3), 845–850. doi:10.1099/ijs.0.000024
Ying, T., and Wei, C. (2019). Soil microbiomes—a promising strategy for contaminated soil remediation: a review. Pedosphere 29, 283–297. doi:10.1016/S1002-0160(18)60061-X
Zhang, W. Y., Fang, M. X., Zhang, W. W., Xiao, C., Zhang, X. Q., Yu, Z. P., et al. (2013). Extensimonas vulgaris gen. nov., sp. nov., a member of the family Comamonadaceae. Int. J. Syst. Evol. Microbiol. 63, 2062–2068. doi:10.1099/ijs.0.038158-0
Keywords: Ecopiling, bioremediation, phytoremediation, microbiome, metagenomics
Citation: Wang M, Garrido-Sanz D, Sansegundo-Lobato P, Redondo-Nieto M, Conlon R, Martin M, Mali R, Liu X, Dowling DN, Rivilla R and Germaine KJ (2021) Soil Microbiome Structure and Function in Ecopiles Used to Remediate Petroleum-Contaminated Soil. Front. Environ. Sci. 9:624070. doi: 10.3389/fenvs.2021.624070
Received: 30 October 2020; Accepted: 01 February 2021;
Published: 15 March 2021.
Edited by:
Markus Puschenreiter, University of Natural Resources and Life Sciences Vienna, AustriaReviewed by:
Bhabananda Biswas, University of South Australia, AustraliaCristina Silva Pereira, New University of Lisbon, Portugal
Copyright © 2021 Wang, Garrido-Sanz, Sansegundo-Lobato, Redondo-Nieto, Conlon, Martin, Mali, Liu, Dowling, Rivilla and Germaine. This is an open-access article distributed under the terms of the Creative Commons Attribution License (CC BY). The use, distribution or reproduction in other forums is permitted, provided the original author(s) and the copyright owner(s) are credited and that the original publication in this journal is cited, in accordance with accepted academic practice. No use, distribution or reproduction is permitted which does not comply with these terms.
*Correspondence: K. J. Germaine, kieran.germaine@itcarlow.ie
†These authors have contributed equally to this work