- 1Ottawa Research and Development Centre, Agriculture and Agri-Food Canada, Ottawa, ON, Canada
- 2Department of Soil Science, University of Saskatchewan, Saskatoon, SK, Canada
Evidence-based decisions governing sustainable agricultural land management practices require a mechanistic understanding of soil organic matter (SOM) transformations and stabilization of carbon in soil. Large amounts of carbon from organic fertilizers, root exudates, and crop residues are input into agricultural soils. Microbes then catalyze soil biogeochemical processes including carbon extracellular transformation, mineralization, and assimilation of resources that are later returned to the soil as metabolites and necromass. A systems biology approach for a holistic study of the transformation of carbon inputs into stable SOM requires the use of soil “omics” platforms (metagenomics, metatranscriptomics, metaproteomics, and metabolomics). Linking the data derived from these various platforms will enhance our knowledge of structure and function of the microbial communities involved in soil carbon cycling and stabilization. In this review, we discuss the application, potential, and suitability of different “omics” approaches (independently and in combination) for elucidating processes involved in the transformation of stable carbon in soil. We highlight biases associated with these approaches including limitations of the methods, experimental design, and soil sampling, as well as those associated with data analysis and interpretation.
Introduction and Background
Soil organic matter (SOM) underpins the health and productivity of soil. Representing the largest carbon (C) pool in terrestrial ecosystems, SOM plays a pivotal role in the global C cycle and climate regulation. Invested with the sun’s energy by photosynthesis, the continual influx of C-rich plant litter and exudates to the soil drives the C cycle as nutrients are released during decay and eventually absorbed by growing plants or released/sequestered to the environment (Pelletier et al., 2011; Haberl et al., 2014; Amundson et al., 2015). The ability of the soil microbiome to access this energy source depends on several factors, many of which are inter-related. For example, the chemical composition of plant litter entering soil, or the SOM itself, affects the structure and activity of the microbiome. The physical environment of where the SOM is located within the soil matrix affects the soil water content and gas exchange, which in turn regulate microbial growth and activity. Also, the degree to which soil physically protects SOM (e.g., within small pores or by chemical bonding to metals and mineral surfaces) may restrict access to it by the microbiome. Therefore, soil type, cropping sequence, soil management, and the nutritional complexity of inputs to soil affect the fate and turnover of C in soil. The turnover rate of this cycle affects many ecosystem processes and properties: soil biodiversity—by delivery of solar energy; plant growth—by providing soil nutrients; water quality—by release of soluble nitrogen and phosphorus; climate change—by exchange of CO2 and other greenhouse gases; and soil resilience—by effects on SOM stocks (Handa et al., 2014). Many of these influences are critical, especially now in the face of global stresses such as growing food demands, changing climate, and loss of biodiversity (Lin, 2014). Improved knowledge of the processes that govern SOM stability and long-term C storage under different land use, management practices, and climates will be important to identify practices that enhance and preserve soil health and productivity and the ongoing recycling and supply of nutrients.
The persistence and stability of soil organic C depends on its biotic and abiotic environment. In terrestrial ecosystems, SOM represents a continuum of organic compounds, from fresh inputs like plant litter to progressively decomposed compounds. Biological, physical, and chemical transformation processes convert plant root exudates and intact residues into derivative products that form complex and intimate associations with soil minerals (Lehmann and Kleber, 2015). These transformative processes of SOM are determined by interdependent factors that include: compound chemistry, spatial arrangement and interaction with mineral surfaces, temperature and moisture conditions, soil acidity and redox state, and the proximity, biomass, and community composition of microbial degraders (Schmidt et al., 2011; Lehmann and Kleber, 2015). SOM plays a key role in forming a stable physical structure (aggregates and biopores) within the soil matrix. This structure promotes aeration and the infiltration and holding capacity of plant-available water as nutrients and energy are released during decomposition, to promote soil fertility (Janzen, 2015; Lehmann and Kleber, 2015; Liang et al., 2017).
Within soil (Figure 1), microbial degradation of plant residues promotes the release of C to the atmosphere through catabolism and at the same time, stabilizes C into forms of SOM that are not easily decomposed (Schimel and Schaeffer, 2012; Liang et al., 2017). The accumulation of fungal and bacterial necromass via biomass turnover is one of the main drivers of C-stabilization of the SOM reservoir (Kindler et al., 2006; Schweigert et al., 2015; Kallenbach et al., 2016). Through microbial anabolism, accessible organic compounds are re-synthesized into molecules that are relatively more chemically stable (i.e., microbial cells, cell debris, and biofilms); fungal residues are thought to be more persistent in soils compared to bacterial residues (Six et al., 2006; Liang et al., 2017). In time, over the course of decomposition, the distinct chemistries of initial litter types (organic inputs) slowly converge following assimilation into microbial biomass and subsequent microbial turnover, into chemical compounds that serve as indicators of plant inputs (e.g., lignin phenols) and microbial metabolites and necromass (e.g., amino sugars) (Wickings et al., 2011; Kallenbach et al., 2016; Liang et al., 2017).
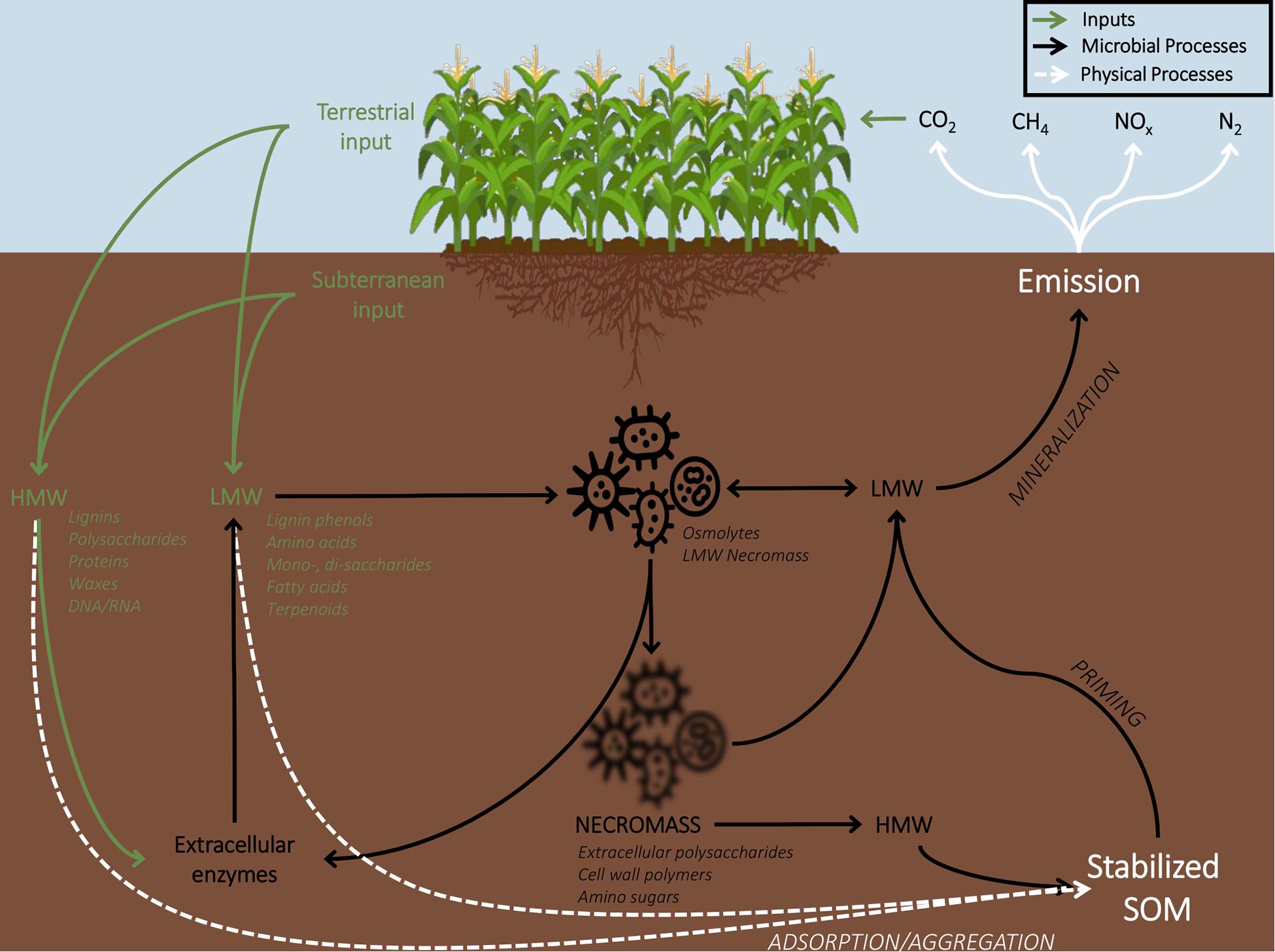
Figure 1. Nutrient cycling schematic demonstrating pathways for stabilization of soil organic matter (SOM). Plant inputs in the form of litter and exudates condition the physiology of the soil microbial community. Soil microbes directly assimilate some low molecular weight (LMW) molecules and produce extracellular enzymes to degrade high molecular weight (HMW) plant inputs, undergoing respiration (and mineralization of SOM) and biomass accumulation, ultimately resulting in a net increase of stabilized C in the form of microbial necromass.
Evidence-based decisions governing sustainable agricultural land management practices require a mechanistic understanding of SOM transformations and C-stabilization in soil. Our current knowledge of microbial population diversity and dynamics of soil communities is, in part, derived from the application of modern molecular biological tools. Significant gaps in our knowledge of microbial-mediated stabilization of soil C include a comprehensive knowledge of the specific compounds involved, their turnover rates, and the nature of their stabilization mechanisms (Liang et al., 2017). Application of a systematic and biological approach has been proposed to study the soil environment as a whole, employing “omics” tools to link metabolic pathways and gene expression of microbial populations into structure–function relationships regarding soil C cycling and stabilization (Schmidt et al., 2011). Our aim in this review is to discuss the suitability of different “omics” approaches (independently and in combination) in soil C transformation studies. We also highlight potential methodological biases associated with these approaches, from limitations of the methods to experimental design and soil sampling to data analysis and interpretation.
Soil Metagenomics and Its Applications for Soil Carbon Stabilization
Metagenomics—General Context and Current Applications
Culture-based approaches used in the study of microbial population dynamics substantially underestimate the diversity of the soil microbial community, the majority of which remain uncharacterized (Fierer, 2017). Although most soil microbes cannot be cultured or grown in the lab outside of the soil matrix (i.e., on/in defined growth media), application of culture-independent molecular approaches such as metagenomics (Figures 2A,B) has uncovered an extraordinary diversity of microorganisms associated with the soil environment (Nesme et al., 2016). Modern sequencing techniques have dramatically improved our taxonomic understanding of soil microorganisms and have helped answer the question: “Who is there?,” but the next critical question of “Who’s doing what?” in terms of soil carbon stabilization has not been resolved. Combining phylogenetic markers with functional measurements begins to address this problem (Cadotte et al., 2009). New approaches employing stable isotopes (e.g., 13C and 18O) to simultaneously evaluate soil processes and microbial community structures at the genomic level are beginning to resolve the relationships between phylogeny and function. For example, stable isotope probing using 18O can be used to label DNA in bacterial cultures and distinguish between newly grown cells and microorganisms that have not grown during incubation (Schwartz et al., 2014).
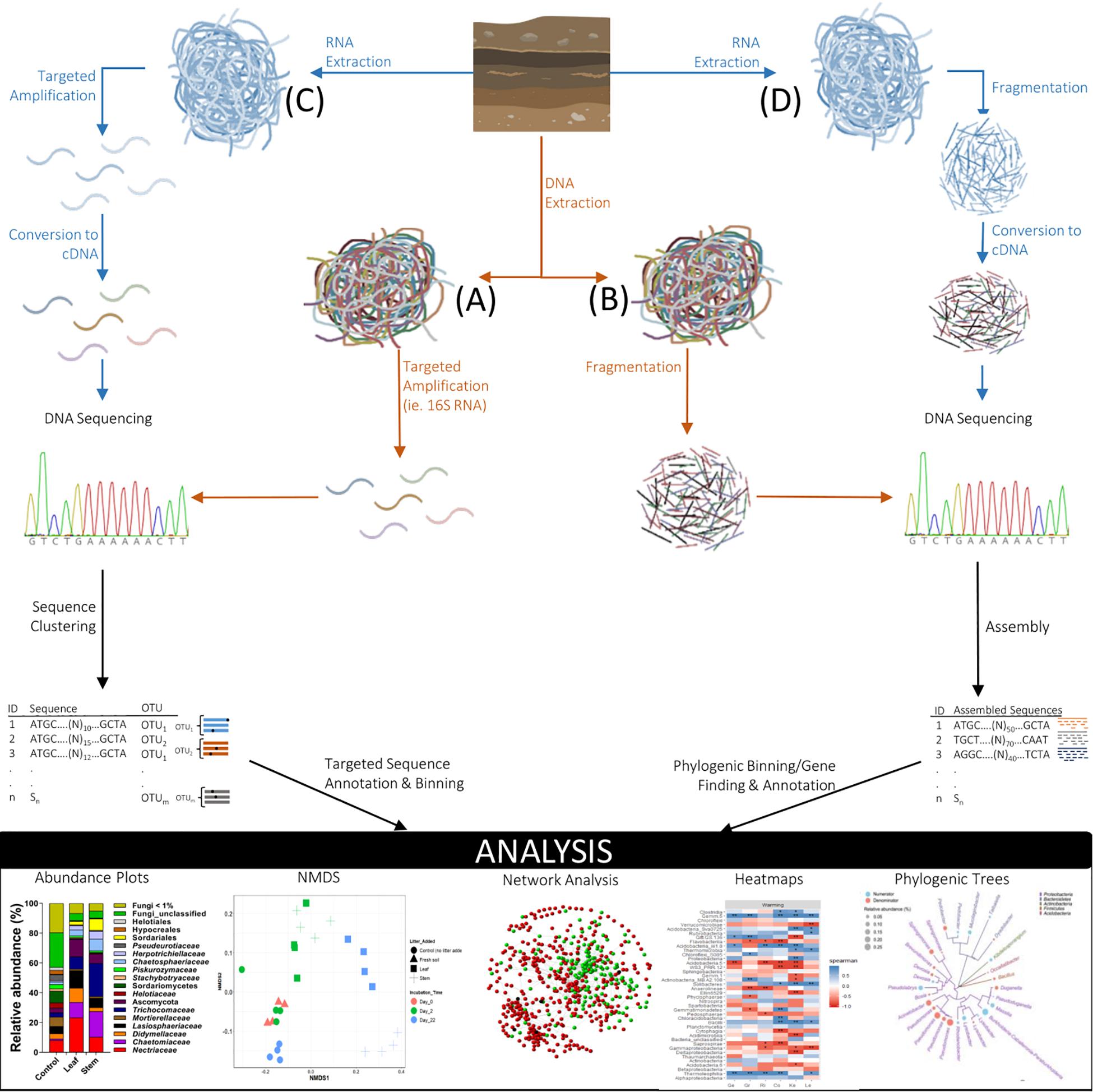
Figure 2. Soil metagenomics and metatranscriptomic studies provide insight into population diversity and functional dynamics of soil microbial communities. Targeted gene sequencing (A) or shotgun sequencing of gene fragments (B) are common soil metagenomics approaches used to examine microbial population composition and can focus on particular gene sequences encoding for enzymes and other proteins involved with functional ecosystem processes. Metatranscriptomics involves the extraction of RNA, targeted amplification (C) or fragmentation (D), and conversion to cDNA prior to DNA sequencing, to provide contemporaneous information of community functional processes (such as SOM stabilization) actively occurring in soil.
In terms of soil carbon stabilization, microbial communities are understood to contain specific keystone taxa that exhibit functional redundancy and thus have strong positive associations during C cycling (Banerjee et al., 2016). In a rigorous metagenomics study, Bahram et al. (2018) sequenced approximately 160 million unique genes/gene fragments (with only 0.5% overlap with published genomes) from 189 topsoil samples collected from representative regions/biomes across the globe. They concluded that bacterial taxonomic diversity, composition, richness, and biomass correlate with soil pH, nutrient concentration, and to a lesser extent climatic variables (Bahram et al., 2018). In comparison, similar characteristics in fungi were more strongly correlated with soil C/N ratios as a predictor for fungal biomass and relative abundance and composition of gene functions; compared to bacteria, fungal global distribution was influenced by substrate/resource availability and energy demand variables (Bahram et al., 2018).
Metagenomic approaches have been successfully applied to investigate the role of C-cycling genes associated with various management practices (e.g., addition of organic matter and/or fertilizers, and tillage practices) to predict C loss or storage in agricultural soils (Cline and Zak, 2015; Nivelle et al., 2016; Zhao et al., 2016). Field experiments have shown that, in response to frequent fertilizer and organic material additions, shifts of the copiotrophic communities within the soil microbiome coincide with respective changes in soil biochemical processes (Carbonetto et al., 2014). Conventional tillage and crop rotation management practices impact soil microbial communities and are associated with an increase in abundance carbohydrate metabolism-related gene fragments (Souza et al., 2015). Carbon-cycling enzymes involved in biopolymer degradation (such as glycosyltransferases, glycoside hydrolases, carbohydrate esterases, carbohydrate-binding modules, and polysaccharide lyases) are characteristically associated with biogeochemical transformations in soil (Howe et al., 2016). Although differences in the relative abundance of these genes have been observed in various soil types, the fact that representative gene fragments were detected in multiple soil samples from a fertilized tall grass prairie indicates the ubiquity of the microbial potential to drive C cycling in soils (Howe et al., 2016).
Current Metagenomics Limitations
Targeted gene sequencing or shotgun sequencing of gene fragments are common approaches used in soil metagenomics studies (Nesme et al., 2016). Using phylogenetic barcoding genes, targeted soil metagenomics applications examine microbial population composition at a resolution of taxonomic order or higher (some studies attempt to annotate to the genus or species levels). But in this context, primer and PCR biases need to be considered because they can often skew population predictions (Sipos et al., 2007) and indeed important soil taxa (e.g., Verrucomicrobia) have been previously overlooked by commonly used “universal” bacterial 16S ribosomal ribonucleic acids (rRNA) gene primers (Bergmann et al., 2011). Moreover, the use of barcoding genes only provides information regarding microbial population diversity/composition and does not provide resolution toward population function. Here, targeted sequencing analyses that focus on particular gene sequences encoding for enzymes and other proteins involved with functional ecosystem processes are required. The future of metagenomics applications toward the prediction of the full functional potential of a soil microbial population will require deep sequencing efforts done at the full genome level; however, reconstruction of “population genomes” from biologically complex samples such as soil is currently difficult as genome closure remains a challenge (Sharon and Banfield, 2013; Prosser, 2015).
Soil metagenomics has proven to be useful for characterizing the soil microbiome, but like all tools, there are inherent limitations and biases. Several of these limitations and biases are common to all molecular techniques associated with cell lysis, efficient nucleic acid extraction and stability, and sequencing errors that affect reliable gene annotation and quantification of shotgun environmental gene sequences. Moreover, the assignment of observed sequences to specific taxa is difficult and not always possible (Prosser, 2015). To address some of these limitations, data analysis pipelines (e.g., MEGAN, Mothur, Qiime, DADA2, and Deblur) are available that can selectively remove or correct erroneous sequences (Huson et al., 2007; Schloss et al., 2009; Caporaso et al., 2010; Callahan et al., 2016; Amir et al., 2017). Also common to high-throughput sequencing approaches is the challenge that the data generated are compositional. That is, the total number of sequences generated is arbitrary and influenced by sequencing instrument, which imposes constraints for data analysis and interpretation (Gloor et al., 2017).
Other limitations involved in using metagenomics in soil research are related to working with a physically heterogeneous material. For example, efficient extraction of DNA requires complete dispersion of soil aggregates and detachment of cells from a broad range of soil types and textures. Hence, repeated extractions of a given soil sample may be necessary to maximize cell yield (Williamson et al., 2011). The cells obtained through repeated extractions may capture unique assemblages of microbes that would otherwise have been missed in single-pass extractions. Despite homogenization of soil samples prior to nucleic acid extraction, most DNA extractions are performed on a small sub-sample (less than 1 g). Even with the use of technical replicates, it is possible that bias occurs as a result of the small sample mass.
Soil microbial communities are dynamic and active members of a population fluctuate, where at any one point in time, a significant proportion of the community may be dead or dormant (Blagodatskaya and Kuzyakov, 2013). As amplicon-based or shotgun metagenomics cannot differentiate between active, dead/dormant, and relic DNA (Carini et al., 2016) (qualitatively, the presence of a functional gene is not evidence of its activity; Prosser, 2015), a complete portrayal of active soil functions at the time of sampling is not possible (rather such techniques provide a prediction of potential soil function—past, present, and future). Caution is required when assuming links between microbial activity and relative gene abundance (Prosser, 2015). Accurate separation of functional variation from taxonomic variation within metagenomics data is also necessary, if deeper insight into microbial community assembly and mechanistic soil microbial ecology is to be achieved (Louca et al., 2016). However, despite these limitations, metagenomics approaches can be used to make hypotheses regarding how microbial populations respond as environmental and soil management conditions change.
Soil Metatranscriptomics and Its Applications for Soil Carbon Stabilization
Metatranscriptomics—General Context and Current Applications
Growth and metabolism of soil microbial communities involve the transcription of genes into ribonucleic acids (RNA); molecules that are physically involved with protein assembly in the cell (e.g., rRNA and tRNA) or carry specific template information for protein translation [messenger RNA (mRNA)]. Soil metatranscriptomics strives to represent the total RNA in soil (Figures 2C,D) and RNA expression and transcription is typically an indicator of the live and active members of a microbial community (Blagodatskaya and Kuzyakov, 2013). Therefore, application of soil metatranscriptomics tools to the study of carbon stabilization in soil provides contemporaneous community population and functional information about processes that are actively occurring in soil.
In comparison to metagenomics studies, there are relatively few metatranscriptomics studies in the literature that investigate soil carbon cycling in agricultural soils. One study of note investigated the effects of adding wood ash amendments to agricultural soil. Wood ash amendment can affect soil physicochemical parameters and influence soil microbial community composition and functional expression, primarily related to soil chemical properties (increased pH, electrical conductivity, dissolved organic carbon, and phosphate) (Bang-Andreasen et al., 2020). Addition of the wood ash amendments caused an increase in genes associated with copiotrophic microbial subpopulations (rRNA); in general, more functional genes (mRNA) were observed to be upregulated than downregulated, with the majority originating from four functional categories: “post-translation modification, protein turnover and chaperones”; “transcription”; “replication, recombination, and repair”; and “carbohydrate transport and metabolism”—genes that are involved with carbon cycling (i.e., metabolism and cell growth) (Bang-Andreasen et al., 2020). The researchers concluded that increases in pH, bioavailable dissolved organic carbon, and nutrients (induced by the wood ash ammendments) allow copiotrophic groups to thrive at the expense of oligotrophic groups directly following amendment application.
Carbon cycling in soils is also affected by differences in oxygen availability—which is of particular relevance in paddy soil microbiomes. Kim and Liesack (2015) compared microbiome structure and functional succession in the oxic and anoxic layers of paddy soils using metatranscriptomics. Their results suggested that the taxonomic composition of paddy soil microbiomes is governed by the prevailing habitat conditions (such as oxygen availability); differences in the composition and succession of the microbiome between the two oxygen zones were related to the differential expression of transcripts of highly conserved and ubiquitous genes essential for metabolic activity in the respective zones. Fungi and Xanthomonadales appeared to cooperate in decomposing plant materials in the oxic zone, whereas most of the dominant groups in the anoxic zone collaboratively transformed organic matter. Another study using shotgun sequencing of total extracted RNA from rice paddy soils showed that anaerobic biodegradation of SOM and methanogenesis were the predominate C transformations (Masuda et al., 2018). They also provided strong evidence that Anaeromyxobacter plays a key role in not only in the C and N cycles, but also in Fe reduction in the paddy soil environment.
Current Metatranscriptomics Limitations
RNA gene transcripts are transient intermediate molecules, and therefore transcript profiles can vary over short periods of time even in stable conditions. Hence, obtaining RNA gene transcripts that accurately reflect a snapshot of the microbial community in highly dynamic environments like agricultural soils can be particularly challenging. Ribosomal RNA (rRNA) accounts for the largest proportion of soil RNA (Urich et al., 2008; Turner et al., 2013; Orellana et al., 2018), whereas mRNA represents only a very small fraction (<5%) of the soil transcriptome (He et al., 2010). Owing to the low proportion of mRNA in the total soil transcriptome and the interests of many studies to investigate specific soil functions (e.g., carbon and/or nitrogen transformations), enrichment of mRNA prior to downstream analysis is often conducted (He et al., 2010; Carvalhais et al., 2012). Annotation of gene transcripts generally requires the use of a metagenome scaffold as the identification of transcripts benefits from the availability of genome sequences obtained from pure cultures of microorganisms that are present in the soil sample (Prosser, 2015). Lastly, interpretation of metatranscriptomics data requires a link between a transcript and the activity of its associated enzyme which necessitates assumptions regarding transcript and protein stability and turnover. This link may be difficult to make because of the dynamic nature of agricultural soils. Soil environmental (temperature and moisture) and physical/chemical properties are dynamic at both daily and seasonal time scales because field operations such as plowing, disking, planting, fertilizing, spraying, and harvesting operations all affect soil conditions and microbial activity.
Similar technical issues that are encountered in metagenomic studies are associated with metatranscriptomics, with further potential bias introduced during the construction of cDNA libraries and a requirement for rapid inactivation of samples to prevent mRNA turnover (Prosser, 2015). The short half-life of RNA molecules requires RNA stabilizing reagents or flash-freezing samples at the site using liquid nitrogen. This can lead to significant challenges in applying transcriptomics in field-based research where experiments are far from research infrastructure. Commercially available stabilization protocols are used as alternatives to the use of liquid nitrogen (e.g., LifeGuard Soil Preservation Solution, Qiagen); however, caution should be noted as stabilization protocol induced biases have been reported (Tatangelo et al., 2014; McCarthy et al., 2015). Some of the major challenges in extracting RNA from soil include the presence of coextractants (mainly organic acids; Peršoh et al., 2008), RNA adsorption to soil minerals, low yield of RNA, and the predominance of rRNA in the total extract (Wang et al., 2012). Although there are bioinformatics tools to specifically identify mRNA and rRNA sequences, low-expressed mRNA genes may be absent in the final sequence reads due to various biases (e.g., incomplete coverage as primers are database-dependent, preferential amplifications, and variable efficiency due to varied annealing temperature for different organisms; Brooks et al., 2015; García-Ortega and Martínez, 2015).
Soil Metaproteomics and Its Applications for Soil Carbon Stabilization
Metaproteomics—General Context and Current Applications
Soil metaproteomics tools are used to study the total protein content in a soil sample to provide information on specific proteins, such as enzymes, that are related to microbial community functionality and are persistent in the environment, unlike RNA which is transient. Metaproteome studies of different environments all use similar, or closely related, analytical methods and instruments (Benndorf et al., 2007; Williams et al., 2010; Wang et al., 2011; Keiblinger et al., 2012; Grob et al., 2015; Hultman et al., 2015; Liu et al., 2017). Prior to proteomics analysis, soil-extracted proteins are usually separated by using 1D or 2D SDS polyacrylamide gel electrophoresis. Then, separated gel bands of proteins are digested into smaller peptides and further separated using different liquid chromatographic systems and characterized using mass spectrometry (MS). Fine tuning of protein extraction and sample preparation methods, and technological advances in mass spectrometers are extremely relevant for the advancement of the field of soil metaproteomics.
Metaproteomics studies have proven essential for providing fundamentally new insights into the role played by microbes in soil biogeochemical processes involving soil carbon stabilization (such as plant residue decomposition), where protein expression is linked together with microbial origin and temporal distribution (Schneider et al., 2012). Decomposition of plant residues plays an initial and integral step in carbon cycling in agricultural soils. More recalcitrant biopolymers such as lignin, cutin, and suberin persist in soils as they take longer to degrade, where degradation requires specific enzymes produced by unique members of the microbial population, typically attributed fungi (Kramer et al., 2016; Arcand et al., 2017; Müller et al., 2017). Sidibé et al. (2016) conducted an incubation study and characterized the soil metaproteome to evaluate whether addition of recalcitrant suberin to the soil can enrich specific bacterial members of soil microbial communities. Counts of protein spectral features indicated a decline in the proportion of fast-growing bacteria and enrichment of particular bacterial constituents of the soil microbial community that can specifically degrade suberin (as suggested by the observation of putative bacterial lipases and other proteins linked to lipid metabolism), providing new insight into this fundamental process in carbon-cycling in soils (Sidibé et al., 2016).
Tracing the flow of carbon in soil using stable isotope-labeling of the soil metaproteome helps to link microbial species to a specific substrate in situ (Seifert et al., 2012; von Bergen et al., 2013; Grob et al., 2015). Kleiner et al. (2018) developed a direct protein stable isotope fingerprint method that links microbial species in communities to the carbon source they consume by determining their stable carbon isotope signature. The method involves preparation of peptide mixtures, measuring their stable C and N isotope ratios using 1D or 2D LC-MS/MS, database searching of the peptide spectra, and using the spectral scores and raw MS data as inputs to provide single, robust, averaged stable C and N isotope ratios per species.
Soil metaproteomics have also been successfully applied to characterize the structure of metabolically-active microorganisms in rhizosphere soils (Wang et al., 2011; Mattarozzi et al., 2017; Bona et al., 2019). The rhizosphere encompasses the soil environment situated in proximity to root hairs of growing root tips, where plant exudates (carbon inputs) are exchanged with microbial populations, often in exchange for non-organic nutrients, essential for plant growth and health. Wang et al. (2011) detected numerous proteins with potential functions in energy metabolism, protein turnover and amino acid biosynthesis, secondary metabolism, nucleotide metabolism, signal transduction, and resistance mechanisms in crop rhizosphere soils. Although 43% of the proteins were unknown, about 23% of the remaining proteins were from the rhizosphere, and from microorganisms in which Proteobacteria have been identified as the most predominant in the metaproteomics (44%) and T-RFLP (48%) libraries. Another study by Mattarozzi et al. (2017) successfully applied soil metaproteomics to rhizosphere soils obtained from plants that were metal-tolerant and metal hyperaccumulators. The authors identified up to 294 unique bacterial proteins using liquid chromatography (LC)-high-resolution MS (HRMS) analysis of the soil metaproteome.
Current Metaproteomic Limitations
The unbiased extraction of proteins directly from soil is difficult because soil is one of the most complex and heterogeneous of all microbial environments. There are numerous methods reported for direct protein extraction from the soil, all of which involve: cell lysis, stabilization of proteins (often by using reducing agents), precipitation of proteins, and a subsequent cleanup step (Benndorf et al., 2007; Chourey et al., 2010; Hultman et al., 2015). Major challenges associated with the direct isolation of proteins from soil include: co-extraction of organic acids (which impact the downstream analysis), binding of proteins to metal ions, soil particles, and organic matter, denaturation of proteins by the extraction conditions (e.g., chemicals, temperature, ionic strength, and pH), the low abundance of some important proteins, and the heterogeneous nature of the soil matrix (Bastida et al., 2009). Protein recovery is highly dependent on extractant and soil type, suggesting that no single or universal extractant is capable of complete protein recovery. This may limit direct comparison of studies using different recovery methods, especially if no extraction controls are used (Greenfield et al., 2018). Finally, both the high diversity of microorganisms in soil and the interaction of environmental conditions likely play a role in the specific response of the microbial community to drivers of microbial functioning, such as land use and management practices.
These challenges make characterizing the soil metaproteome a daunting task. A study by Nicora et al. (2013) demonstrated enhanced recovery of proteins from sediment soil samples by blocking protein binding sites in the soil using a mixture of polar positive amino acids prior to in situ lysis and by enhancing the release of proteins from the soil using an optimized desorption buffer. As these amino acids may pose interferences on the MS analysis, the authors optimized the concentrations to be small enough to avoid detection by the MS. Parallel or sequential extraction of soil proteins from a soil sample using different extraction methods and combining the spectral data may improve the detection capability. For instance, Mattarozzi et al. (2017) combined protein spectral features obtained from three different extraction methods, and showed that up to 1.5% of the total proteins (∼294 unique proteins) from each sample could be detected by using the methods. Extraction efficiency of proteins may also be improved following separation and isolation of microorganisms from the soil by differential centrifugation; however, in such circumstances, extra-cellular proteins (e.g., polymer-degrading enzymes) in the soil solution are lost (Chourey et al., 2010).
Soil microbial communities are heterogeneous, dominated in density by a few species, while the remainder of the microbial diversity are present in low abundances. Deciphering the presence of specialized functional proteins from complex protein profiles dominated by proteins of ubiquitous function (such as “house-keeping” proteins involved with cellular respiration, energy metabolism, DNA replication, etc.) is therefore a considerable challenge, especially when produced by low abundance community members (Starke et al., 2019). Further complications arise during the annotation stage, where protein identification relies upon successful database matching. Multiple protein databases are available; however, these databases favor intensively-investigated and well-characterized model organisms (i.e., Escherichia coli, Saccharomyces cerevisiae, and Caenorhabditis elegans), and therefore annotation of functional proteins from community members of “unknown” identity is difficult and tenuous (Starke et al., 2019).
Soil-Metabolomicsand Its Applications for Soil Carbon Stabilization
Metabolomics—General Context and Current Applications
Soil metabolomics characterizes the composition of small metabolites (100–2000 Da range) in the soil (by-products, intermediates, and end products of cellular processes) and provides information directly on carbon transformation and stabilization as well as the constituents of SOM therein (Figure 3). Untargeted metabolomics (metabolite profiling) is a global analysis that characterizes as much of small molecule chemistry of the SOM as possible, whereas semi-targeted analyses focus on a specific molecular class or range of classes (e.g., N containing compounds, or fatty acids). Untargeted metabolite profiling is intended to have maximal coverage of chemical space; however, regardless of the extraction method chosen, some molecules will be missed because they are present in trace amounts, strongly adsorbed on to mineral surfaces, or because they are not soluble with the extract used, and/or incompatible with the instrument methods used for profiling. Semi-targeted extractions are comparably less complex and easier to analyze, but important cross-class metabolite interactions may be missed. Untargeted metabolite profiling aims to generate hypotheses to explain difference in molecular patterns observed, while targeted analyses are often used to test hypotheses as they are more quantitative and are therefore used to answer questions related to specific classes of compounds.
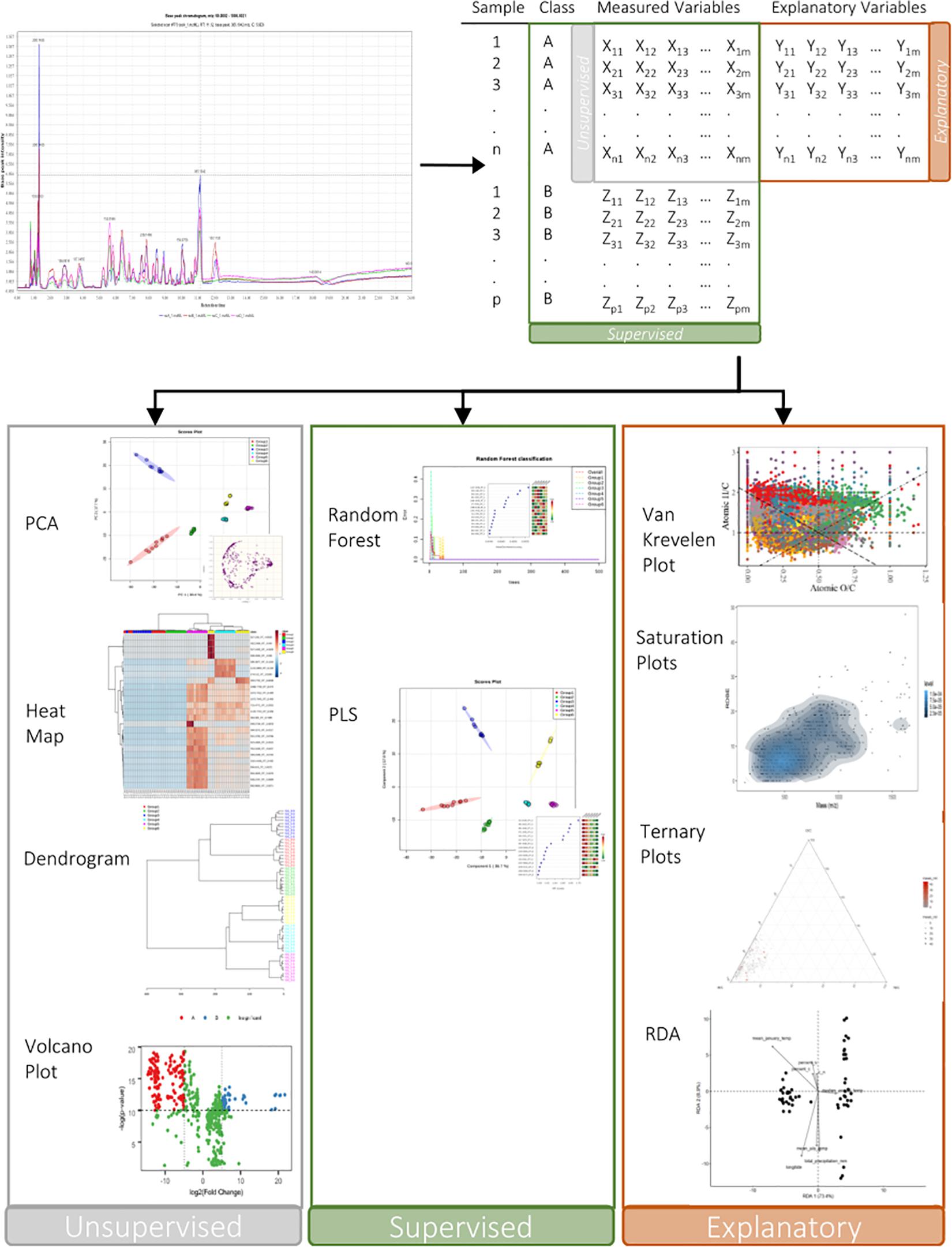
Figure 3. Application of untargeted or semi-targeted metabolomics analysis of SOM provides insight into physiochemical and biological drivers of SOM stabilization and mineralization. Untargeted metabolite profiling is intended to have maximal coverage of chemical space; whereas semi-targeted analyses focus on a specific molecular class or range of classes (e.g., N containing compounds, or fatty acids). During data processing (i.e., of an LC-MS dataset), mass spectral chromatograms [three-dimensional data: retention time, m/z range (50–2000 Da), relative signal abundance] are converted into two-dimensional data matrices through data preprocessing software, followed by multivariate and univariate statistical analysis (unsupervised, supervised, and molecular fingerprinting methods).
There are a number of excellent untargeted analyses of small molecules and SOM composition by biogeochemists and geologists (Pautler et al., 2013; Pisani et al., 2016; Seifert et al., 2016; Grewer et al., 2018; Li et al., 2018; Wu et al., 2018), in which the term “molecular characterization” has been used rather than “metabolomics”—as the term “metabolomics” infers small molecules associated with metabolic processes. This discrepancy in semantics is an important consideration, in that, the soil environment is complex and SOM chemistry is a reflection of both biotic and abiotic effects upon small organic molecules, wherein not all small molecules in SOM are directly derived from metabolism.
Metabolomics has been successfully applied in several experimental models to characterize the molecular composition of SOM. Clemente et al. (2013) compared the composition of SOM after incubation with different parts of the maize plant. They found that after 36 weeks of incubation, soil amended with maize roots had the greatest microbial SOM contribution, compared to soil amended with stems or leaves. This is consistent with other studies showing that the “quality” of plant inputs affects the soil microbial community. Rochfort et al. (2015) used nuclear magnetic resonance (NMR) spectroscopy-based soil metabolomics and compared metabolite profiles in soils under different land use and found that lipid- and sugar-related metabolites were distinctly abundant in an agricultural soil, whereas soil from a remnant site was dominated by terpene metabolites. The abundance of easily degradable carbon substrates in the agricultural soils may be related to the larger inputs of organic carbon. Pisani et al. (2015) assessed the SOM composition across a large temperature gradient with changes in land use. Using NMR, they were able to determine that soil cultivation resulted in increased microbial contributions at higher temperatures, had lower plant contributions, and increased suberin and lignin degradation. They concluded that land-use changes associated with cultivation may become sources of atmospheric CO2.
Untargeted SOM analyses have attempted to link together SOM compositional knowledge with microbial analyses to investigate SOM stabilization. The terms “soil dissolved organic matter” (DOM), “water extractable organic matter” (WEOM), or “water extractable organic carbon” (WEOC) are commonly used to describe water extracts from soil. Linked soil metabolomics has been used in a few instances to determine soil metabolite profiles during wetting/drying (Swenson et al., 2018), in ecological assessments (Beale et al., 2017, 2018), to study permafrost carbon storage (Ward and Cory, 2015), with soil amendments (Mitchell et al., 2015), and for developing improved growth media for the soil microbiome (Jenkins et al., 2017). Some studies link to microbial activity more generally. Jenkins et al. (2017) used LC-MS and gas chromatography (GC)-MS to identify 96 metabolites in soils and quantify 25 of them, which showed that the latter metabolites were unevenly distributed. This allowed them to formulate two soil defined media, one containing 23 metabolites and another containing 46 metabolites; medium formulations that were focused on the cultivation of previously uncultured microorganisms. Ward and Cory (2015) demonstrated linking soil DOM to characterize bacterial growth and activity parameters such as microbial respiration, production, and growth efficiency. Warren (2014) proposed a method using LC-MS to target fatty acids as indicators of microbial activities, to infer specific microbial contributions to the formation of SOM. Similarly, Mitchell et al. (2015) used untargeted NMR paired with a targeted analysis of phospholipid fatty acids (PLFAs) to link the effects of biochar amendment to impacts on the soil microbial community and the WEOM fraction of SOM. This technique paired with untargeted NMR of the DOM suggested that the microbial community shifted from fungal- to more bacterial-dominated and from Gram-negative bacteria to more Gram-positive bacteria within 16 weeks after biochar amendment. In the WEOM, they also observed increased concentrations of short-chain carboxylic acids but decreased carbohydrates and peptides and concluded that biochar amendments increased soil microbial activity and thus CO2 emissions.
Current Metabolomics Limitations
Selection of an appropriate extraction system is critical for unbiased characterization of soil metabolites. The diverse nature of chemical constituents within soil limits the use of a single solvent to extract and obtain all available molecules. Thus, global-untargeted studies require parallel or sequential extraction techniques (using different extractants) on a single soil sample (Tfaily et al., 2015, 2017), and to combine data obtained from different analytical tools (Jenkins et al., 2017). Swenson et al. (2015) profiled metabolites using different solvents and demonstrated that water ranked as the best extractant for soil exometabolites because no significant differences were observed when compared with other extractants which included methanol, K2SO4, and NH4HCO3. By using water as an extractant, potential artifacts created by the other chemical extractants may be avoided (Sauerschnig et al., 2017), but non-polar metabolites such as lipophilic molecules (that are also important components of SOM stabilization) are excluded. Chloroform fumigation is commonly used to lyze cells prior to extraction in order to increase both the diversity and abundance of extractable soil metabolites (with a particular bias toward nucleosides, nucleotides, and amino acids; Warren, 2014); however, chloroform fumigation does not inhibit all exoenzyme activity, and care should be taken following cell lysis during sample processing as active enzymatic degradation of organic matter can continue for the duration of the extraction process (Blankinship et al., 2014).
Metabolites of various sources are usually a mixture of different molecular compounds of varying abundance and chemical properties (e.g., carbohydrates, amino acids, peptides, lipids, nucleic acids, and organic acids). Different analytical tools are available for the identification and quantification of soil metabolites (Zhang et al., 2012; Alonso et al., 2015); however, NMR and MS are the most commonly used. Both tools have advantages and drawbacks. NMR provides direct relationships to the amount of each molecular constituent in a mixture and yields interpretable information on molecular structures; while MS provides information about the mass and charge of individual molecules. NMR is considerably less sensitive than MS and therefore, MS is more suited for the detection of low-abundance metabolites (Zhang et al., 2012). Annotation of the individual constituents of an extract is difficult and often requires some degree of chromatographic separation prior to detection for proper annotation. However, regardless of the method used, separation comes at a cost, as only metabolites that are soluble in solvent systems used for injection and elution (in LC) and derivatization and thermostability (in GC) are observed, introducing a bias or complexity reduction of metabolic coverage in global-untargeted metabolomics analyses. In such a case, multiple chromatographic systems or technologies will often be used to profile soil extracts to maximize molecular coverage (i.e., GC-MS and LC-MS profiling, or reverse phase and HILIC chromatography). In addition, we have found that high salt contents naturally present in soil or from SOM extraction procedures can damage sensitive chromatography and MS instrumentation and also increases the complexity of the resulting spectra via adduct formation.
Metabolites in soils are highly dynamic and vary widely spatially and temporally. They are produced as a result of changes substrate (e.g., amendments to soil), temperature, and moisture. Therefore, proper study design (e.g., with a sufficient number of biological replicates and including control experiments and method blanks), and well-planned sampling and sample preparation are critical for untargeted SOM studies. Sample collection and storage duration influence the results as the passage of time can shift the quantity and quality of metabolites available in the soil of improperly stored samples. Thus, unbiased characterization of metabolites at a specific time of the study requires an immediate quenching of the microbial/enzymatic activity in the soil samples, and reduced exposure to light and oxygen. The easiest method of quenching microbial metabolism is using liquid nitrogen and flash freezing the soil; this is particularly important when samples need to be transported from a study site to the lab. If the soil samples are collected from a lab study, it may be possible to immediately store in a −80°C freezer or flash freeze with liquid N2 prior to −80°C storage. Ideally, the extractions are also performed at low temperatures to slow enzymatic reactions or else microbes and exoezymes will continue to modify the native SOM leading to methodological-derived biases in the results.
Linking Omics Technologies—For Soil Carbon Stabilization
To develop a deeper understanding of the process of carbon stabilization in soil, the connections between the composition of the microbial community and its functional response to the environment (e.g., resources available) need to be characterized. But redundancies in the functional response by different members of the microbial community increase the complexity of any assessment of this process. Many common microbial enzymes and metabolites involved in carbon cycling in soils are produced from ubiquitous gene clusters, all playing specific roles as inputs/outputs from one type of microbe are actively used by a different group of the soil microbial community.
Current “omics” approaches have successfully modeled various aspects of these processes; however, using a single “omics” tool only allows for a single perspective of this heterogeneous environment, generating a partial, or fragmented, representation of a complex network of interactions. But combining observations from multiple “omics” platforms links the genetic potential and community structure to functional relationships with gene, protein, and metabolite, thereby providing a more holistic vision of soil “metaphenomics” (Jansson and Hofmockel, 2018) (Figure 4). For example, a multi-omics approach could involve targeted 16S rRNA gene sequencing to determine the microbial community composition; total metagenomic DNA sequencing to determine the complement of phylogenetic and functional genes; total metatranscriptome RNA sequencing to determine which genes were expressed; and untargeted MS-based metaproteomics to determine which proteins were produced (Hultman et al., 2015). This type of approach could help to identify the dominant heterotrophic pathways for carbon metabolism and examine how microbial physiology influences the relative importance of carbon cycling pathways in response to environmental conditions.
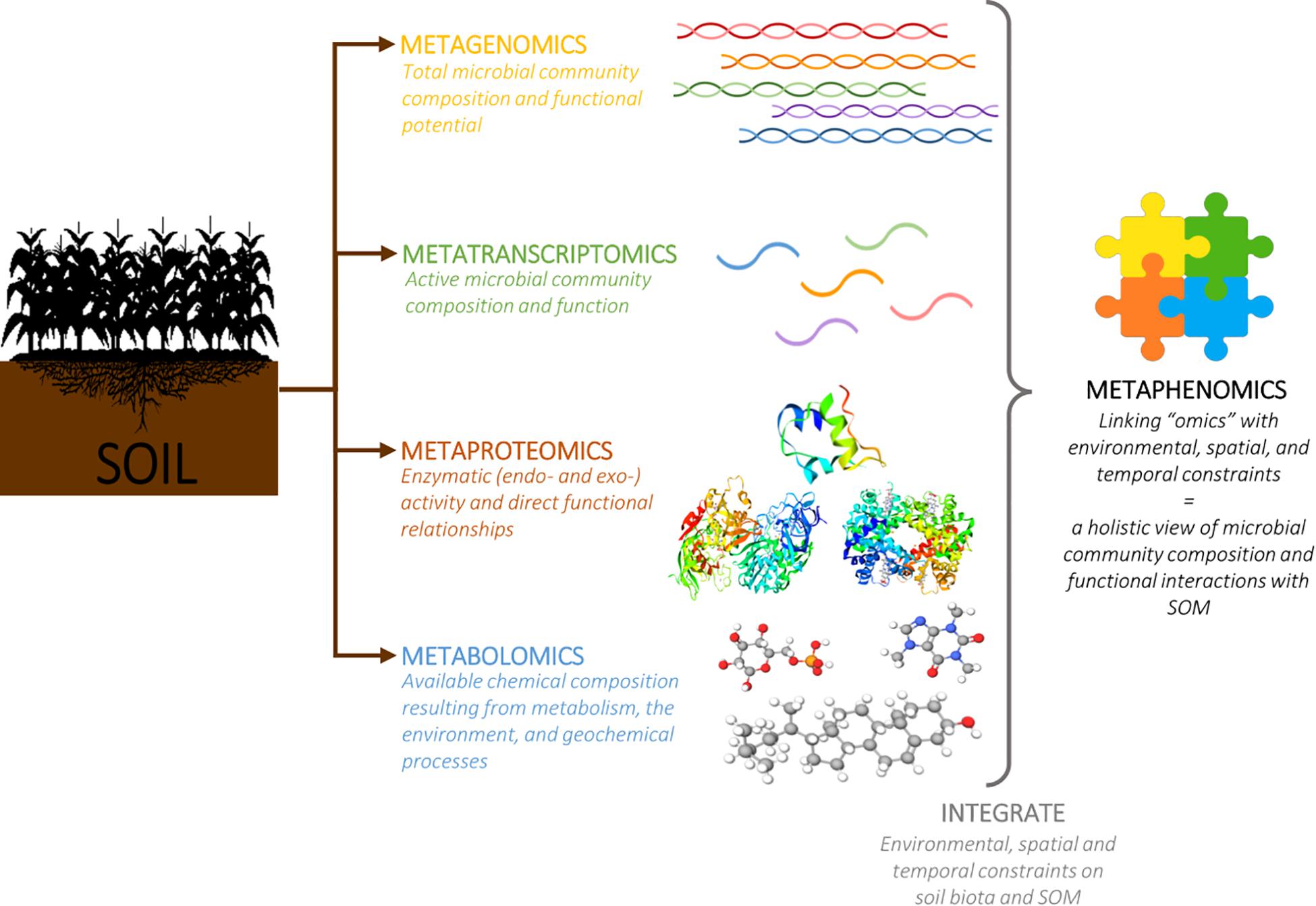
Figure 4. Linking data resulting from parallel analysis of soil samples using various “omics” platforms (metagenomics, metatranscriptomics, metaproteomics, and metabolomics) provides a more holistic view (metaphenomics) of microbial community composition and understanding of functional interactions associated with biogeochemical processes involved in SOM stabilization.
There are a few examples of research to link soil “omics” techniques in the scientific literature. Recently, Beale et al. (2017, 2018) combined untargeted GC-MS metabolomics with bacterial metagenomics. Beale et al. (2018) characterized metabolite profiles and microbial community structure in sediments during dry and wet seasonal conditions to account for biological and physicochemical variance due to non-rainfall-based abiotic stresses. The authors identified significant metabolic features (e.g., 3,5-dihydroxyphenylglycine, beta-alanine, L-allothreonine, and numerous unknown metabolites) that increase (2.2- to 13.2-fold) during the dry season that were linked with a transition in bacterial community composition toward organisms that utilize more complex organic energy sources, such as carbohydrates and fatty acids, and anaerobic redox processes (Beale et al., 2018). Swenson et al. (2018) also combined metagenomics with GC-MS metabolomics to provide a high-resolution assessment of the soil microbial community. They used biocrusts as a model system to demonstrate the feasibility of integrating metagenomics with soil metabolomics to hypothesize food webs that develop after wetting dry soils. They also discussed building databases that can contribute to more sophisticated models for carbon flux predictions. Such models would be invaluable for future data miners and modelers interested in climate change or modeling state changes in agricultural soils following a change in cropping history or soil management practices.
A good example of a metabolomics approach to characterize changes in SOM chemistry in terms of major functional classes of metabolites was conducted by Kallenbach et al. (2016). They added a soil-derived microbial seed inoculum to different sterile, mineral soils (kaolinite or montmorillonite clay mixed with quartz sand—containing little to no SOM). Each mineral soil was amended weekly with additions of different C-substrate inputs over a 15-month period and then left without additions for another 3 months. Temporal changes in microbial population composition were characterized using PLFA biomarkers, and proxy measurements of C use efficiency were made to estimate microbial residue production. Over the 18-month period, convergence in SOM chemistry (and in turn SOM stabilization) was observed regardless of C-input or clay/soil type as a direct result of microbial activity, biomass accumulation, and stabilization into microbial necromass. Using this approach as a model and expanding it to include other “omics” platforms will provide relevant functional information in terms of which particular transformative processes were initiated and when (i.e., metatranscriptomics), what enzymatic reactions were occurring (i.e., metaproteomics), and how microbial population dynamics (i.e., metagenomics) fluctuate over time to cause a convergence in SOM chemistry between the different soils.
Each of the different “omics” platforms discussed in this review has been successfully applied to model various aspects of soil C stabilization; however, harmonization of each platform toward a common understanding remains challenging. Going forward, soil microbial metagenomics needs to move beyond simple descriptions of community diversity (Fierer, 2017) to characterize and evaluate important soil processes (such as C cycling). Community metabolic function is strongly influenced by nutritional, energetic, and stoichiometric constraints, while taxonomic variation within these communities is only poorly explained by environmental conditions (Raes et al., 2011; Louca et al., 2016; Nelson et al., 2016). Linking “omics” experiments through genome-centric metagenomics and metatranscriptomics will provide insight into the metabolic basis and mechanism for phylotype involvement (Prosser, 2015); however, consideration of temporal dynamics when setting up an experiment of merged “omics” studies is imperative to link gene cluster potential with transcript abundance and metabolic activity.
When designing a set of linked “omics” experiments (and more importantly, when interpreting data derived from those experiments), several caveats of each “omics” approach need to be considered. First, amplification of environmental DNA is indiscriminate and can represent dead, dormant, or relic members of the microbial community (Levy-Booth et al., 2007; Carini et al., 2016; Lennon et al., 2018), potentially skewing interpretations of the relative abundance of different taxa. Second, RNA transcripts are transient and rapidly degrade following transcription making them less subject to similar bias; however, due to their short temporal nature, coordination with metaproteomic and metabolomics experiments is essential. Changes in cellular metabolism and exoenzymatic degradation of SOM can only be detected after transcription of their associated proteins has occurred and metabolite products have accumulated, decoupling SOM breakdown from population estimates based on nucleic acid analysis. Without knowledge of the temporal dynamics, erroneous assumptions can be made regarding community structure (genomic predictions of functional potential) and the expression of a functional response. Experiments that account for temporal change by repeated sampling over time will elucidate trends in gene transcription, translation, and metabolite fluxes. Hence, conducting experiments at an appropriate time scale will provide deeper insight into the associations of genome potential and the functional expression of a microbial population. As abiotic soil properties are a major driver of soil microbiomes, a minimum dataset is also required, which needs to be analyzed and implemented independently from the research question(s). A basic suite of these should consist of soil texture, pH, and organic C and N. Appropriate contextual information is critical to interpret most omics information. For example, at agricultural sites, current and past management practices should be listed. These include time of fertilization, tillage, harvest, as well as crop growth and yield, crop sequence, and biomass. For unmanaged sites, the land use and/or vegetation type should be characterized.
Soil Sampling Considerations for Linked “Omics”
Soils vary laterally across the landscape and vertically through the profile and therefore comprise diverse microhabitats, even at fine (cm) scales (O’Brien et al., 2016). The degree of heterogeneity strongly depends on: (i) texture, which influences extraction efficiency; (ii) soil structure and related properties (e.g., aggregation and drainage); (iii) vegetation type and proximity to growing plants (e.g., the rhizosphere); (iv) season; and (v) specific site characteristics such as topographic location (slope and depressions) and groundwater table. Taking this heterogeneity into account, the typical 0.5–2 g soil sample used for an “omics” extraction does not reflect a single microsite, but a mixture of different microhabitats with different chemical, physical, and biological properties. The effects of spatial heterogeneity can be reduced by increasing the amount of soil and number of samples used for an extraction. For example, regarding DNA extraction from soil, Penton et al. (2016) observed that sample size significantly affected overall bacterial and fungal community structure, and the number of operational taxonomic units retrieved. They found that recovery of bacterial and fungal diversity improved substantially as the mass of soil samples increased from 0.25 to 10 g.
The inherent heterogeneity of soil is a primary driving factor when developing a sampling strategy for a soil “omics” experiment. A preliminary assessment of the spatial variation of soil properties is a prime requisite for developing a sound soil sampling strategy. In agricultural soils, where factors such as tillage practices and application of chemical and organic fertilizers influence the distribution of resources with depth in the profile, the vertical heterogeneity may be of interest and must be accounted for Sun et al. (2018). In this case, the sampling strategy should consider the depth of plowing as well as the stratification of horizons with varying depths, to avoid the mixing of different soil horizons. One soil sample taken from a given site is an inadequate representation; therefore, true soil sample replicates (multiple individual samples taken, rather than a single sample split into “replicates”) need to be collected and analyzed. Moreover, to limit the influence of soil heterogeneity within a given sample, a representative sampling strategy, involving the harvesting and subsequent pooling of subsamples, should be used.
Overlaid on these spatial factors are temporal factors that need to be taken into account because soil microhabitats and organisms therein change in response to management practices like fertilization, tillage, harvesting, plant growth stage, weather, and season. Likewise, the stabilization and accumulation of proteins and metabolites are strongly influenced by the local conditions prior to sampling. Therefore, sampling at one time is a brief “snapshot” of nucleic acids, proteins, and metabolites, each of which change at a different rate. Monitoring and evaluating antecedent local conditions (e.g., soil temperature and water content) is therefore necessary to correctly interpret omics data. Hence, a snapshot of metagenomic data will also require evaluation of temperature and moisture conditions over a period of days, weeks, or months. The issue of spatial and temporal heterogeneity is more pronounced when analyzing RNA because the stability of mRNA in cells is often in the order of minutes to hours. Thus, one sampling gives only a brief glimpse of the microbial community, one that depends very much on the environmental conditions at the time of sampling. By taking account of the strong spatial and temporal dynamics of soil microbial communities, a sound sampling strategy needs to be driven by a clear research hypothesis.
Conclusion and Future Perspective
“Omics” technologies encompass a broad suite of techniques that are currently being applied to diverse scientific fields to understand complex environmental processes. These technologies provide opportunities to advance our understanding of how and which microorganisms transform SOM inputs into stable compounds that enhance soil health and productivity. The real potential of applying “omics” in this area of soil science will be realized by linking “omics” technologies together in well-designed experiments (taking spatial/temporal changes into consideration) to generate relevant and testable hypotheses. Integration of linked “omics” approaches with traditional methods used in soil science is relatively recent but will become more prevalent in the near future. Modern technological advances are constantly occurring in all fields of “omics.” With increased sequencing depth and decreasing costs, sequencing of environmental DNA and RNA is making the assembly of “population genomes” feasible. Application of high-resolution mass spectrometers in environmental studies is providing greater accuracy and resolution for peak deconvolution and annotation in metabolomics and proteomic studies. These technological advances aid to provide greater resolution and insight into microbial populations and how these populations change and interact with their environment. “Omics” platforms are multi-disciplinary and require expensive technological infrastructure. Often a single lab is not able to integrate more than one “omics” tool from their toolbox; therefore, more collaboration will likely be required to achieve a linked “omics” approach (e.g., soil metaphenomics approach) to studying C-dynamics/stabilization in soil, as well as in other areas of environmental sciences.
Author Contributions
DO: conceptualization, writing—original draft, writing—review and editing, supervision, and funding acquisition. MB: writing—original draft, writing—review and editing, and visualizations. JH: writing—original draft and writing—review and editing. BH: writing—review and editing. EG: conceptualization, supervision, writing—original draft, writing—review and editing, and funding acquisition. All authors contributed to the article and approved the submitted version.
Funding
This work was supported by the Canadian Agricultural Partnership—Agrilnnovate Program from Agriculture and AgriFood Canada (Project ID# J-001756: “Understanding mechanisms in the biological stabilization of carbon in soil”).
Conflict of Interest
The authors declare that the research was conducted in the absence of any commercial or financial relationships that could be construed as a potential conflict of interest.
References
Alonso, A., Marsal, S., and JuliÃ, A. (2015). Analytical methods in untargeted metabolomics: state of the art in 2015. Front. Bioeng. Biotechnol. 3:23.
Amir, A., McDonald, D., Navas-Molina, J. A., Kopylova, E., Morton, J. T., and Zech, et al. (2017). Deblur rapidly resolves single-nucleotide community sequence patterns. mSystems 2:e000191-16.
Amundson, R., Berhe, A. A., Hopmans, J. W., Olson, C., Sztein, A. E., and Sparks, D. L. (2015). Soil and human security in the 21st century. Science 348:1261071.
Arcand, M. M., Levy-Booth, D. J., and Helgason, B. L. (2017). Resource legacies of organic and conventional management differentiate soil microbial carbon use. Front. Microbiol. 8:2293.
Bahram, M., Hildebrand, F., Forslund, S. K., Anderson, J. L., Soudzilovskaia, N. A., Bodegom, P. M., et al. (2018). Structure and function of the global topsoil microbiome. Nature 560, 233–237. doi: 10.1038/s41586-018-0386-6
Banerjee, S., Kirkby, C. A., Schmutter, D., Bissett, A., Kirkegaard, J. A., and Richardson, A. E. (2016). Network analysis reveals functional redundancy and keystone taxa amongst bacterial and fungal communities during organic matter decomposition in an arable soil. Soil Biol. Biochem. 97, 188–198. doi: 10.1016/j.soilbio.2016.03.017
Bang-Andreasen, T., Zohaib Anwar, M., Lanzén, A., Kjøller, R., Rønn, R., Ekelund, F., et al. (2020). Total RNA sequencing reveals multilevel microbial community changes and functional responses to wood ash application in agricultural and forest soil. FEMS Microbiol. Ecol. 96:fiaa016.
Bastida, F., Moreno, J. L., Nicolás, C., Hernández, T., and García, C. (2009). Soil metaproteomics: a review of an emerging environmental science. significance, methodology and perspectives. Eur. J. Soil Sci. 60, 845–859. doi: 10.1111/j.1365-2389.2009.01184.x
Beale, D. J., Crosswell, J., Karpe, A. V., Ahmed, W., Williams, M., Morrison, P. D., et al. (2017). A multi-omics based ecological analysis of coastal marine sediments from Gladstone, in Australia’s Central Queensland, and Heron Island, a nearby fringing platform reef. Sci. Total Environ. 609, 842–853. doi: 10.1016/j.scitotenv.2017.07.184
Beale, D. J., Crosswell, J., Karpe, A. V., Metcalfe, S. S., Morrison, P. D., Staley, C., et al. (2018). Seasonal metabolic analysis of marine sediments collected from Moreton Bay in South East Queensland, Australia, using a multi-omics-based approach. Sci. Total Environ. 631-632, 1328–1341. doi: 10.1016/j.scitotenv.2018.03.106
Benndorf, D., Balcke, G. U., Harms, H., and von Bergen, M. (2007). Functional metaproteome analysis of protein extracts from contaminated soil and groundwater. ISME J. 1, 224–234. doi: 10.1038/ismej.2007.39
Bergmann, G. T., Bates, S. T., Eilers, K. G., Lauber, C. L., Caporaso, J. G., Walters, W. A., et al. (2011). The under-recognized dominance of verrucomicrobia in soil bacterial communities. Soil Biol. Biochem. 43, 1450–1455. doi: 10.1016/j.soilbio.2011.03.012
Blagodatskaya, E., and Kuzyakov, Y. (2013). Active microorganisms in soil: critical review of estimation criteria and approaches. Soil Biol. Biochem. 67, 192–211. doi: 10.1016/j.soilbio.2013.08.024
Blankinship, J. C., Becerra, C. A., Schaeffer, S. M., and Schimel, J. P. (2014). Separating cellular metabolism from exoenzyme activity in soil organic matter decomposition. Soil Biol. Biochem. 71, 68–75. doi: 10.1016/j.soilbio.2014.01.010
Bona, E., Massa, N., Novello, G., Boatti, L., Cesaro, P., Todeschini, V., et al. (2019). Metaproteomic characterization of Vitis vinifera rhizosphere. FEMS Microbiol. Ecol. 95:fiy204. doi: 10.1093/femsec/fiy204
Brooks, J. P., Edwards, D. J., Harwich, M. D., Rivera, M. C., Fettweis, J. M., Serrano, M. G., et al. (2015). The truth about metagenomics: quantifying and counteracting bias in 16S rRNA studies. BMC Microbiol. 15:66.
Cadotte, M. W., Cavender-Bares, J., Tilman, D., and Oakley, T. H. (2009). Using phylogenetic, functional and trait diversity to understand patterns of plant community productivity. PLoS One 4:e0005695.
Callahan, B. J., McMurdie, P. J., Rosen, M. J., Han, A. W., Johnson, A. J. A., and Holmes, S. P. (2016). DADA2: high-resolution sample inference from Illumina amplicon data. Nat. Methods 13, 581–583. doi: 10.1038/nmeth.3869
Caporaso, J. G., Kuczynski, J., Stombaugh, J., Bittinger, K., Bushman, F. D., Costello, E. K., et al. (2010). QIIME allows analysis of high-throughput community sequencing data. Nat. Methods 7, 335–336.
Carbonetto, B., Rascovan, N., Álvarez, R., Mentaberry, A., and Vázquez, M. P. (2014). Structure, composition and metagenomic profile of soil microbiomes associated to agricultural land use and tillage systems in Argentine Pampas. PLoS One 9:e99949. doi: 10.1371/journal.pone.0099949
Carini, P., Marsden, P. J., Leff, J. W., Morgan, E. E., Strickland, M. S., and Fierer, N. (2016). Relic DNA is abundant in soil and obscures estimates of soil microbial diversity. Nat. Microbiol. 2:16242.
Carvalhais, L. C., Dennis, P. G., Tyson, G. W., and Schenk, P. M. (2012). Application of metatranscriptomics to soil environments. J. Microbiol. Methods 91, 246–251. doi: 10.1016/j.mimet.2012.08.011
Chourey, K., Jansson, J., VerBerkmoes, N., Shah, M., Chavarria, K. L., Tom, L. M., et al. (2010). Direct cellular lysis/protein extraction protocol for soil metaproteomics. J. Proteome Res. 9, 6615–6622. doi: 10.1021/pr100787q
Clemente, J. S., Simpson, M. J., Simpson, A. J., Yanni, S. F., and Whalen, J. K. (2013). Comparison of soil organic matter composition after incubation with maize leaves, roots, and stems. Geoderma 192, 86–96. doi: 10.1016/j.geoderma.2012.08.007
Cline, L. C., and Zak, D. R. (2015). Soil microbial communities are shaped by plant-driven changes in resource availability during secondary succession. Ecology 96, 3374–3385. doi: 10.1890/15-0184.1
Fierer, N. (2017). Embracing the unknown: disentangling the complexities of the soil microbiome. Nat. Rev. Microbiol. 15, 579–590. doi: 10.1038/nrmicro.2017.87
García-Ortega, L. F., and Martínez, O. (2015). How many genes are expressed in a transcriptome? estimation and results for RNA-Seq. PLoS One 10:e0130262. doi: 10.1371/journal.pone.0130262
Gloor, G. B., Macklaim, J. M., Pawlowsky-Glahn, V., and Egozcue, J. J. (2017). Microbiome datasets are compositional: and this is not optional. Front. Microbiol. 8:2224.
Greenfield, L. M., Hill, P. W., Paterson, E., Baggs, E. M., and Jones, D. L. (2018). Methodological bias associated with soluble protein recovery from soil. Sci. Rep. 8:11186.
Grewer, D. M., Lafrenière, M. J., Lamoureux, S. F., and Simpson, M. J. (2018). Spatial and temporal shifts in fluvial sedimentary organic matter composition from a high Arctic watershed impacted by localized slope disturbances. Org. Geochem. 123, 113–125. doi: 10.1016/j.orggeochem.2018.07.004
Grob, C., Taubert, M., Howat, A. M., Burns, O. J., Dixon, J. L., Richnow, H. H., et al. (2015). Combining metagenomics with metaproteomics and stable isotope probing reveals metabolic pathways used by a naturally occurring marine methylotroph: metagenomics, metaproteomics and methylotrophy. Environ. Microbiol. 17, 4007–4018. doi: 10.1111/1462-2920.12935
Haberl, H., Erb, K.-H., and Krausmann, F. (2014). Human appropriation of net primary production: patterns, trends, and planetary boundaries. Annu. Rev. Environ. Resour. 39, 363–391. doi: 10.1146/annurev-environ-121912-094620
Handa, I. T., Aerts, R., Berendse, F., Berg, M. P., Bruder, A., Butenschoen, O., et al. (2014). Consequences of biodiversity loss for litter decomposition across biomes. Nature 509, 218–221. doi: 10.1038/nature13247
He, S., Wurtzel, O., Singh, K., Froula, J. L., Yilmaz, S., Tringe, S. G., et al. (2010). Validation of two ribosomal RNA removal methods for microbial metatranscriptomics. Nat. Methods 7, 807–812. doi: 10.1038/nmeth.1507
Howe, A., Yang, F., Williams, R. J., Meyer, F., and Hofmockel, K. S. (2016). Identification of the core set of carbon-associated genes in a bioenergy grassland soil. PLoS One 11:e0166578. doi: 10.1371/journal.pone.0166578
Hultman, J., Waldrop, M. P., Mackelprang, R., David, M. M., McFarland, J., Blazewicz, S. J., et al. (2015). Multi-omics of permafrost, active layer and thermokarst bog soil microbiomes. Nature 521, 208–212. doi: 10.1038/nature14238
Huson, D. H., Auch, A. F., Qi, J., and Schuster, S. C. (2007). MEGAN analysis of metagenomic data. Genome Res. 17, 377–386. doi: 10.1101/gr.5969107
Jansson, J. K., and Hofmockel, K. S. (2018). The soil microbiome — from metagenomics to metaphenomics. Curr. Opin. Microbiol. 43, 162–168. doi: 10.1016/j.mib.2018.01.013
Janzen, H. H. (2015). Beyond carbon sequestration: soil as conduit of solar energy: soil carbon and energy. Eur. J. Soil Sci. 66, 19–32.
Jenkins, S., Swenson, T. L., Lau, R., Rocha, A., Aaring, A., Hazen, T. C., et al. (2017). Construction of soil defined media using quantitative exometabolomic analysis of soil metabolites. bioRxiv [preprint] doi: 10.1101/151282
Kallenbach, C. M., Frey, S. D., and Grandy, A. S. (2016). Direct evidence for microbial-derived soil organic matter formation and its ecophysiological controls. Nat. Commun. 7:13630.
Keiblinger, K. M., Wilhartitz, I. C., Schneider, T., Roschitzki, B., Schmid, E., Eberl, L., et al. (2012). Soil metaproteomics – Comparative evaluation of protein extraction protocols. Soil Biol. Biochem. 54, 14–24. doi: 10.1016/j.soilbio.2012.05.014
Kim, Y., and Liesack, W. (2015). Differential assemblage of functional units in paddy soil microbiomes. PLoS One 10:e0122221. doi: 10.1371/journal.pone.0122221
Kindler, R., Miltner, A., Richnow, H., and Kastner, M. (2006). Fate of gram-negative bacterial biomass in soil—mineralization and contribution to SOM. Soil Biol. Biochem. 38, 2860–2870. doi: 10.1016/j.soilbio.2006.04.047
Kleiner, M., Dong, X., Hinzke, T., Wippler, J., Thorson, E., Mayer, B., et al. (2018). Metaproteomics method to determine carbon sources and assimilation pathways of species in microbial communities. Proc. Natl. Acad. Sci. U. S. A. 115, E5576–E5584.
Kramer, S., Dibbern, D., Moll, J., Huenninghaus, M., Koller, R., Krueger, D., et al. (2016). Resource partitioning between bacteria, fungi, and protists in the detritusphere of an agricultural Soil. Front. Microbiol. 7:1524.
Lehmann, J., and Kleber, M. (2015). The contentious nature of soil organic matter. Nature 528, 60–68. doi: 10.1038/nature16069
Lennon, J. T., Muscarella, M. E., Placella, S. A., and Lehmkuhl, B. K. (2018). How, when, and where relic DNA affects microbial diversity. mBio 9:e000637-18.
Levy-Booth, D. J., Campbell, R. G., Gulden, R. H., Hart, M. M., Powell, J. R., Klironomos, J. N., et al. (2007). Cycling of extracellular DNA in the soil environment. Soil Biol. Biochem. 39, 2977–2991. doi: 10.1016/j.soilbio.2007.06.020
Li, H.-Y., Wang, H., Wang, H.-T., Xin, P.-Y., Xu, X.-H., Ma, Y., et al. (2018). The chemodiversity of paddy soil dissolved organic matter correlates with microbial community at continental scales. Microbiome 6:187.
Liang, C., Schimel, J. P., and Jastrow, J. D. (2017). The importance of anabolism in microbial control over soil carbon storage. Nat. Microbiol. 2:17105.
Lin, H. (2014). A new worldview of soils. Soil Sci. Soc. Am. J. 78, 1831–1844. doi: 10.2136/sssaj2014.04.0162
Liu, D., Keiblinger, K. M., Schindlbacher, A., Wegner, U., Sun, H., Fuchs, S., et al. (2017). Microbial functionality as affected by experimental warming of a temperate mountain forest soil—a metaproteomics survey. Appl. Soil Ecol. 117-118, 196–202. doi: 10.1016/j.apsoil.2017.04.021
Louca, S., Jacques, S. M. S., Pires, A. P. F., Leal, J. S., Srivastava, D. S., Parfrey, L. W., et al. (2016). High taxonomic variability despite stable functional structure across microbial communities. Nat. Ecol. Evol. 1:0015.
Masuda, Y., Itoh, H., Shiratori, Y., and Senoo, K. (2018). Metatranscriptomic insights into microbial consortia driving methane metabolism in paddy soils. Soil Sci. Plant Nutr. 64, 455–464. doi: 10.1080/00380768.2018.1457409
Mattarozzi, M., Manfredi, M., Montanini, B., Gosetti, F., Sanangelantoni, A. M., Marengo, E., et al. (2017). A metaproteomic approach dissecting major bacterial functions in the rhizosphere of plants living in serpentine soil. Anal. Bioanal. Chem. 409, 2327–2339. doi: 10.1007/s00216-016-0175-8
McCarthy, A., Chiang, E., Schmidt, M. L., and Denef, V. J. (2015). RNA preservation agents and nucleic acid extraction method bias perceived bacterial community composition. PLoS One 10:e0121659. doi: 10.1371/journal.pone.0121659
Mitchell, P. J., Simpson, A. J., Soong, R., and Simpson, M. J. (2015). Shifts in microbial community and water-extractable organic matter composition with biochar amendment in a temperate forest soil. Soil Biol. Biochem. 81, 244–254. doi: 10.1016/j.soilbio.2014.11.017
Müller, K., Marhan, S., Kandeler, E., and Poll, C. (2017). Carbon flow from litter through soil microorganisms: from incorporation rates to mean residence times in bacteria and fungi. Soil Biol. Biochem. 115, 187–196. doi: 10.1016/j.soilbio.2017.08.017
Nelson, M. B., Martiny, A. C., and Martiny, J. B. H. (2016). Global biogeography of microbial nitrogen-cycling traits in soil. Proc. Natl. Acad. Sci. 113, 8033–8040. doi: 10.1073/pnas.1601070113
Nesme, J., Achouak, W., Agathos, S. N., Bailey, M., Baldrian, P., Brunel, D., et al. (2016). Back to the future of soil metagenomics. Front. Microbiol. 7:73.
Nicora, C. D., Anderson, B. J., Callister, S. J., Norbeck, A. D., Purvine, S. O., Jansson, J. K., et al. (2013). Amino acid treatment enhances protein recovery from sediment and soils for metaproteomic studies. Proteomics 18-19, 2776–2785. doi: 10.1002/pmic.201300003
Nivelle, E., Verzeaux, J., Habbib, H., Kuzyakov, Y., Decocq, G., Roger, D., et al. (2016). Functional response of soil microbial communities to tillage, cover crops and nitrogen fertilization. Appl. Soil Ecol. 108, 147–155. doi: 10.1016/j.apsoil.2016.08.004
O’Brien, S. L., Gibbons, S. M., Owens, S. M., Hampton-Marcell, J., Johnston, E. R., Jastrow, J. D., et al. (2016). Spatial scale drives patterns in soil bacterial diversity. Environ. Microbiol. 18, 2039–2051. doi: 10.1111/1462-2920.13231
Orellana, L. H., Chee-Sanford, J. C., Sanford, R. A., Löffler, F. E., and Konstantinidis, K. T. (2018). Year-round shotgun metagenomes reveal stable microbial communities in agricultural soils and novel ammonia oxidizers responding to fertilization. Appl. Environ. Microbiol. 84:e001646-17.
Pautler, B. G., Sanborn, P. T., Simpson, A. J., and Simpson, M. J. (2013). Molecular characterization of organic matter in Canadian Arctic paleosols for paleoecological applications. Org. Geochem. 63, 122–138. doi: 10.1016/j.orggeochem.2013.08.009
Pelletier, N., Audsley, E., Brodt, S., Garnett, T., Henriksson, P., Kendall, A., et al. (2011). Energy intensity of agriculture and food systems. Annu. Rev. Environ. Resour. 36, 223–246.
Penton, C. R., Gupta, V. V. S. R., Yu, J., and Tiedje, J. M. (2016). Size matters: assessing optimum soil sample size for fungal and bacterial community structure analyses using high throughput sequencing of rRNA gene amplicons. Front. Microbiol. 7:824.
Peršoh, D., Theuerl, S., Buscot, F., and Rambold, G. (2008). Towards a universally adaptable method for quantitative extraction of high-purity nucleic acids from soil. J. Microbiol. Methods 75, 19–24. doi: 10.1016/j.mimet.2008.04.009
Pisani, O., Frey, S. D., Simpson, A. J., and Simpson, M. J. (2015). Soil warming and nitrogen deposition alter soil organic matter composition at the molecular-level. Biogeochemistry 123, 391–409. doi: 10.1007/s10533-015-0073-8
Pisani, O., Haddix, M. L., Conant, R. T., Paul, E. A., and Simpson, M. J. (2016). Molecular composition of soil organic matter with land-use change along a bi-continental mean annual temperature gradient. Sci. Total Environ. 573, 470–480. doi: 10.1016/j.scitotenv.2016.08.154
Prosser, J. I. (2015). Dispersing misconceptions and identifying opportunities for the use of “omics” in soil microbial ecology. Nat. Rev. Microbiol. 13, 439–446. doi: 10.1038/nrmicro3468
Raes, J., Letunic, I., Yamada, T., Jensen, L. J., and Bork, P. (2011). Toward molecular trait-based ecology through integration of biogeochemical, geographical and metagenomic data. Mol. Syst. Biol. 7, 473–473. doi: 10.1038/msb.2011.6
Rochfort, S., Ezernieks, V., Mele, P., and Kitching, M. (2015). NMR metabolomics for soil analysis provide complementary, orthogonal data to MIR and traditional soil chemistry approaches – a land use study. Magn. Reson. Chem. 53, 719–725. doi: 10.1002/mrc.4187
Sauerschnig, C., Doppler, M., Bueschl, C., and Schuhmacher, R. (2017). Methanol generates numerous artifacts during sample extraction and storage of extracts in metabolomics research. Metabolites 8:1. doi: 10.3390/metabo8010001
Schimel, J. P., and Schaeffer, S. M. (2012). Microbial control over carbon cycling in soil. Front. Microbiol. 3:348.
Schloss, P. D., Westcott, S. L., Ryabin, T., Hall, J. R., Hartmann, M., Hollister, E. B., et al. (2009). Introducing mothur: open-Source, platform-independent, community-supported software for describing and comparing microbial communities. Appl. Environ. Microbiol. 75, 7537–7541. doi: 10.1128/aem.01541-09
Schmidt, M. W. I., Torn, M. S., Abiven, S., Dittmar, T., Guggenberger, G., Janssens, I. A., et al. (2011). Persistence of soil organic matter as an ecosystem property. Nature 478, 49–56. doi: 10.1038/nature10386
Schneider, T., Keiblinger, K. M., Schmid, E., Sterflinger-Gleixner, K., Ellersdorfer, G., Roschitzki, B., et al. (2012). Who is who in litter decomposition? Metaproteomics reveals major microbial players and their biogeochemical functions. ISME J. 6, 1749–1762.
Schwartz, E., Van Horn, D. J., Buelow, H. N., Okie, J. G., Gooseff, M. N., Barrett, J. E., et al. (2014). Characterization of growing bacterial populations in McMurdo Dry Valley soils through stable isotope probing with 18O-water. FEMS Microbiol. Ecol. 89, 415–425. doi: 10.1111/1574-6941.12349
Schweigert, M., Herrmann, S., Miltner, A., Fester, T., and Kästner, M. (2015). Fate of ectomycorrhizal fungal biomass in a soil bioreactor system and its contribution to soil organic matter formation. Soil Biol. Biochem. 88, 120–127. doi: 10.1016/j.soilbio.2015.05.012
Seifert, A.-G., Roth, V.-N., Dittmar, T., Gleixner, G., Breuer, L., Houska, T., et al. (2016). Comparing molecular composition of dissolved organic matter in soil and stream water: influence of land use and chemical characteristics. Sci. Total Environ. 571, 142–152. doi: 10.1016/j.scitotenv.2016.07.033
Seifert, J., Taubert, M., Jehmlich, N., Schmidt, F., Völker, U., Vogt, C., et al. (2012). Protein-based stable isotope probing (protein-SIP) in functional metaproteomics. Mass Spectrom. Rev. 31, 683–697. doi: 10.1002/mas.21346
Sharon, I., and Banfield, J. F. B. (2013). Genomes from metagenomics. Science 342, 1057–1058. doi: 10.1126/science.1247023
Sidibé, A., Simao-Beaunoir, A.-M., Lerat, S., Giroux, L., Toussaint, V., and Beaulieu, C. (2016). Proteome analyses of soil bacteria grown in the presence of potato suberin, a recalcitrant biopolymer. Microbes Environ. 31, 418–426. doi: 10.1264/jsme2.me15195
Sipos, R., Székely, A. J., Palatinszky, M., Révész, S., Márialigeti, K., and Nikolausz, M. (2007). Effect of primer mismatch, annealing temperature and PCR cycle number on 16S rRNA gene-targeting bacterial community analysis. FEMS Microbiol. Ecol. 60, 341–350. doi: 10.1111/j.1574-6941.2007.00283.x
Six, J., Frey, S. D., Thiet, R. K., and Batten, K. M. (2006). Bacterial and fungal contributions to carbon sequestration in agroecosystems. Soil Sci. Soc. Am. J. 70, 555–569. doi: 10.2136/sssaj2004.0347
Souza, R. C., Hungria, M., Cantão, M. E., Vasconcelos, A. T. R., Nogueira, M. A., and Vicente, V. A. (2015). Metagenomic analysis reveals microbial functional redundancies and specificities in a soil under different tillage and crop-management regimes. Appl. Soil Ecol. 86, 106–112. doi: 10.1016/j.apsoil.2014.10.010
Starke, R., Jehmlich, N., and Bastida, F. (2019). Using proteins to study how microbes contribute to soil ecosystem services: the current state and future perspectives of soil metaproteomics. J. Proteomics 198, 50–58. doi: 10.1016/j.jprot.2018.11.011
Sun, R., Li, W., Dong, W., Tian, Y., Hu, C., and Liu, B. (2018). Tillage changes vertical distribution of soil bacterial and fungal communities. Front. Microbiol. 9:699.
Swenson, T. L., Jenkins, S., Bowen, B. P., and Northen, T. R. (2015). Untargeted soil metabolomics methods for analysis of extractable organic matter. Soil Biol. Biochem. 80, 189–198. doi: 10.1016/j.soilbio.2014.10.007
Swenson, T. L., Karaoz, U., Swenson, J. M., Bowen, B. P., and Northen, T. R. (2018). Linking soil biology and chemistry in biological soil crust using isolate exometabolomics. Nat. Commun. 9:19.
Tatangelo, V., Franzetti, A., Gandolfi, I., Bestetti, G., and Ambrosini, R. (2014). Effect of preservation method on the assessment of bacterial community structure in soil and water samples. FEMS Microbiol. Lett. 356, 32–38. doi: 10.1111/1574-6968.12475
Tfaily, M. M., Chu, R. K., Tolić, N., Roscioli, K. M., Anderton, C. R., Paša-Tolić, L., et al. (2015). Advanced solvent based methods for molecular characterization of soil organic matter by high-resolution mass spectrometry. Anal. Chem. 87, 5206–5215. doi: 10.1021/acs.analchem.5b00116
Tfaily, M. M., Chu, R. K., Toyoda, J., Tolić, N., Robinson, E. W., Paša-Tolić, L., et al. (2017). Sequential extraction protocol for organic matter from soils and sediments using high resolution mass spectrometry. Anal. Chim. Acta 972, 54–61. doi: 10.1016/j.aca.2017.03.031
Turner, T. R., Ramakrishnan, K., Walshaw, J., Heavens, D., Alston, M., Swarbreck, D., et al. (2013). Comparative metatranscriptomics reveals kingdom level changes in the rhizosphere microbiome of plants. ISME J. 7, 2248–2258. doi: 10.1038/ismej.2013.119
Urich, T., Lanzén, A., Qi, J., Huson, D. H., Schleper, C., and Schuster, S. C. (2008). Simultaneous assessment of soil microbial community structure and function through analysis of the meta-transcriptome. PLoS One 3:e2527. doi: 10.1371/journal.pone.0002527
von Bergen, M., Jehmlich, N., Taubert, M., Vogt, C., Bastida, F., Herbst, F.-A., et al. (2013). Insights from quantitative metaproteomics and protein-stable isotope probing into microbial ecology. ISME J. 7, 1877–1885. doi: 10.1038/ismej.2013.78
Wang, H.-B., Zhang, Z.-X., Li, H., He, H.-B., Fang, C.-X., Zhang, A.-J., et al. (2011). Characterization of metaproteomics in crop rhizospheric soil. J. Proteome Res. 10, 932–940. doi: 10.1021/pr100981r
Wang, Y., Hayatsu, M., and Fujii, T. (2012). Extraction of bacterial RNA from soil: challenges and solutions. Microbes Environ. 27, 111–121. doi: 10.1264/jsme2.me11304
Ward, C. P., and Cory, R. M. (2015). Chemical composition of dissolved organic matter draining permafrost soils. Geochim. Cosmochim. Acta 167, 63–79. doi: 10.1016/j.gca.2015.07.001
Warren, C. R. (2014). Response of osmolytes in soil to drying and rewetting. Soil Biol. Biochem. 70, 22–32. doi: 10.1016/j.soilbio.2013.12.008
Wickings, K., Stuart Grandy, A., Reed, S., and Cleveland, C. (2011). Management intensity alters decomposition via biological pathways. Biogeochemistry 104, 365–379. doi: 10.1007/s10533-010-9510-x
Williams, M. A., Taylor, E. B., and Mula, H. P. (2010). Metaproteomic characterization of a soil microbial community following carbon amendment. Soil Biol. Biochem. 42, 1148–1156. doi: 10.1016/j.soilbio.2010.03.021
Williamson, K. E., Kan, J., Polson, S. W., and Williamson, S. J. (2011). Optimizing the indirect extraction of prokaryotic DNA from soils. Soil Biol. Biochem. 43, 736–748. doi: 10.1016/j.soilbio.2010.04.017
Wu, X., Wu, L., Liu, Y., Zhang, P., Li, Q., Zhou, J., et al. (2018). Microbial interactions with dissolved organic matter drive carbon dynamics and community succession. Front. Microbiol. 9: 1234.
Zhang, A., Sun, H., Wang, P., Han, Y., and Wang, X. (2012). Modern analytical techniques in metabolomics analysis. Analyst 137, 293–300. doi: 10.1039/c1an15605e
Zhao, J., Ni, T., Li, J., Lu, Q., Fang, Z., and Huang, Q. (2016). Effects of organic–inorganic compound fertilizer with reduced chemical fertilizer application on crop yields, soil biological activity and bacterial community structure in a rice–wheat cropping system. Appl. Soil Ecol. 99, 1–12. doi: 10.1016/j.apsoil.2015.11.006
Keywords: carbon stabilization, metagenomics, metatranscriptomics, metaproteomics, metabolomics, metaphenomics
Citation: Overy DP, Bell MA, Habtewold J, Helgason BL and Gregorich EG (2021) “Omics” Technologies for the Study of Soil Carbon Stabilization: A Review. Front. Environ. Sci. 9:617952. doi: 10.3389/fenvs.2021.617952
Received: 15 October 2020; Accepted: 31 March 2021;
Published: 30 April 2021.
Edited by:
Philippe C. Baveye, AgroParisTech Institut des Sciences et Industries du Vivant et de L’Environnement, FranceReviewed by:
Yuntao Hu, Lawrence Berkeley National Laboratory, United StatesNa Li, Northeast Institute of Geography and Agroecology, Chinese Academy of Science, China
Copyright © 2021 Helgason B. L., and Her Majesty the Queen in Right of Canada, as represented by the Minister of Agriculture and Agri-Food Canada for the contribution of Overy D. P., Bell M. A., Habtewold J, Gregorich E. G. This is an open-access article distributed under the terms of the Creative Commons Attribution License (CC BY). The use, distribution or reproduction in other forums is permitted, provided the original author(s) and the copyright owner(s) are credited and that the original publication in this journal is cited, in accordance with accepted academic practice. No use, distribution or reproduction is permitted which does not comply with these terms.
*Correspondence: David P. Overy, david.overy@canada.ca; Edward G. Gregorich, ed.gregorich@canada.ca