- 1Department of Civil and Environmental Engineering, University of California, Davis, CA, United States
- 2Tahoe Environmental Research Center, University of California, Davis, CA, United States
- 3Office of Information Management and Analysis, California State Water Resources Control Board, Sacramento, CA, United States
- 4Department of Land, Air and Water Resources, University of California, Davis, CA, United States
Harmful algal blooms of cyanobacteria are increasing in magnitude and frequency globally, degrading inland and coastal aquatic ecosystems and adversely affecting public health. Efforts to understand the structure and natural variability of these blooms range from point sampling methods to a wide array of remote sensing tools. This study aims to provide a comprehensive view of cyanobacterial blooms in Clear Lake, California — a shallow, polymictic, naturally eutrophic lake with a long record of episodic cyanobacteria blooms. To understand the spatial heterogeneity and temporal dynamics of cyanobacterial blooms, we evaluated a satellite remote sensing tool for estimating coarse cyanobacteria distribution with coincident, in situ measurements at varying scales and resolutions. The Cyanobacteria Index (CI) remote sensing algorithm was used to estimate cyanobacterial abundance in the top portion of the water column from data acquired from the Ocean and Land Color Instrument (OLCI) sensor on the Sentinel-3a satellite. We collected hyperspectral data from a handheld spectroradiometer; discrete 1 m integrated surface samples for chlorophyll-a and phycocyanin; multispectral imagery from small Unmanned Aerial System (sUAS) flights (∼12 cm resolution); Autonomous Underwater Vehicle (AUV) measurements of chlorophyll-a, turbidity, and colored dissolved organic matter (∼10 cm horizontal spacing, 1 m below the water surface); and meteorological forcing and lake temperature data to provide context to our cyanobacteria measurements. A semivariogram analysis of the high resolution AUV and sUAS data found the Critical Scale of Variability for cyanobacterial blooms to range from 70 to 175 m, which is finer than what is resolvable by the satellite data. We thus observed high spatial variability within each 300 m satellite pixel. Finally, we used the field spectroscopy data to evaluate the accuracy of both the original and revised CI algorithm. We found the revised CI algorithm was not effective in estimating cyanobacterial abundance for our study site. Satellite-based remote sensing tools are vital to researchers and water managers as they provide consistent, high-coverage data at a low cost and sampling effort. The findings of this research support continued development and refinement of remote sensing tools, which are essential for satellite monitoring of harmful algal blooms in lakes and reservoirs.
Introduction
Harmful algal blooms of toxin-producing cyanobacteria (cyanoHABs) are increasing in magnitude and frequency globally, both degrading aquatic ecosystems and posing a risk to public health (Havens, 2008; Cheung et al., 2013; Taranu et al., 2015; Huisman et al., 2018; Ho et al., 2019). Monitoring these cyanoHABs is necessary to track their development and mitigate their effects in the context of a changing climate (Paerl et al., 2016; Visser et al., 2016). Monitoring cyanoHABs is challenging because they exhibit substantial spatial and temporal variability (Kutser, 2009; Carey et al., 2014) making it difficult to link complex bloom dynamics with the underlying drivers for these systems (Paerl et al., 2011; Ho and Michalak, 2015).
CyanoHABs demonstrate high spatial variability, which complicates bloom measurement (Kutser, 2009). Drivers of this spatial patchiness include winds and currents, with higher concentrations of cyanobacteria typically observed at downwind sites (Carey et al., 2014; Liu et al., 2019a). Additionally, some cyanobacterial species have gas vacuoles, which allow cells to control their buoyancy and rise and fall in the water column creating spatial variability with depth (Oliver et al., 2012). Even though spatial variability of cyanoHABs is a known issue with regards to remote sensing of blooms as measurements can vary substantially within a satellite pixel (Kutser, 2009), satellite remote sensing tools remain one of the key tools for monitoring cyanoHABs because of their global coverage.
Remote sensing methods have the benefit of offering high spatial and temporal coverage across large scales. Remote sensing of cyanobacteria is possible due to the differences in spectral signatures for cyanobacteria and other types of phytoplankton. Phytoplankton contains chlorophyll-a as the main photosynthetic pigment. This pigment demonstrates absorption features in its spectral profile at 440 and 675 nm (Ruiz-Verdú et al., 2008; Stumpf et al., 2016). Cyanobacteria contain the secondary photosynthetic pigment phycocyanin in addition to chlorophyll-a (Bryant, 1982; Matthews, 2011). Due to its unique spectral signature (including an absorption peak ∼620 nm and a reflectance peak ∼650 nm) and limitation to cyanobacteria and rhodophytes, phycocyanin concentrations are often used to distinguish cyanobacteria from freshwater phytoplankton (Kutser, 2009).
Many satellite remote sensing tools for monitoring harmful algal blooms have been developed (see reviews by Kutser, 2009; Matthews, 2011; and Gholizadeh et al., 2016). In this study we use the Cyanobacterial Index (CI) remote sensing tool, which is a spectral shape algorithm based on the tendency of Microcystis (a common genus of cyanobacteria) to demonstrate weak chlorophyll-a fluorescence compared to other phytoplankton (Wynne et al., 2008; Wynne et al., 2010; Stumpf et al., 2016). This results in an observed reflectance sag in the satellite data at wavelengths of 681 nm. The CI algorithm uses the multispectral MERIS sensor mounted on the Envisat satellite and the Ocean and Land Color Instrument (OLCI) sensor on Sentinel-3 because they have the correct spectral resolution to differentiate cyanobacteria from other phytoplankton. While satellite remote sensing allows for repeated sub-weekly observation of the conditions at the same location, the spatial resolution is usually coarse, ranging from 30 to 1,000 m (Kutser, 2009; Hunter et al., 2017), with most cyanobacteria-specific algorithms utilizing the MERIS and OLCI sensors, which have a resolution of 300 m. This issue is further exacerbated by pixel contamination close to the shoreline. Additionally, cloud coverage is problematic, creating instances where no useful data are obtainable from a satellite pixel. Finally, coarse resolution satellites are not well suited to detect spatial variability of cyanobacteria as the bloom density can vary by up to two orders of magnitude within one satellite pixel (Kutser, 2009).
Given the coarse spatial resolution of satellite tools aimed at detecting cyanobacteria, other high spatial resolution sampling methods are more apt at measuring the spatial variability of cyanoHABs. Many field deployable platforms such as Autonomous Underwater Vehicles and small Unmanned Aerial Systems, can collect data at high spatial resolution. AUVs equipped with fluorescence sensors and/or on-board samplers have been used to measure algal and cyanobacterial blooms, (e.g. Robbins et al., 2006; Blackwell et al., 2008). sUAS carrying Red-Green-Blue, multispectral, and sometimes hyperspectral cameras have also been used for detecting blooms (see review paper by Kislik et al., 2018). These sampling methods have clear benefit due to their high spatial resolution, but do not offer the repeat high temporal measurements offered by satellite platforms.
When satellite tools are combined with other high resolution monitoring tools, they augment the observer’s ability to both monitor and study blooms (Vander Woude et al., 2019). Additionally, the high spatial resolution methods, (e.g. AUV and sUAS) may be used to quantify the spatial heterogeneity of cyanoHABs, specifically to determine their Critical Scale of Variability (CSV). CSVs (as defined by Blackwell et al., 2008) are the length scales necessary to resolve the spatial variability of a bloom. The CSV of a cyanobacterial bloom defines the distance required between samples to observe the spatial “patchiness” or changes in bloom concentration across space. The CSV also helps define the spatial extent at which biological and physical processes may occur (Fraschetti et al., 2006). The CSV is important for designing sampling plans by selecting the sampling resolution necessary to adequately characterize a bloom (Vander Woude et al., 2019).
In this study, we measure cyanobacterial bloom density using several methods at varying spatial scales and spatial resolutions to understand how bloom density changes across scales. We collected discrete water samples; hyperspectral measurements from a handheld spectroradiometer; coarse spatial resolution multispectral reflectance data from the OLCI sensor on the Sentinel-3a satellite; high spatial resolution multispectral reflectance data collected using a sUAS; and high spatial resolution scattering and fluorescence data from an AUV. This multiplatform approach provides a synoptic view of cyanobacterial density across multiple spatial scales. The collected data were used to 1) understand the spatial and temporal trends at our study site; 2) quantify the CSV for cyanobacteria blooms; and 3) evaluate the accuracy of the CI algorithm. Key recommendations stemming from this work are consideration of the CSV of cyanobacteria blooms when designing sampling plans and future satellite remote sensing sensors, and continued refinement of the CI algorithm for improved detection of low concentration blooms.
Methods
Study Site
This study was conducted at Clear Lake, California, a large (approximately 160 km2 in surface area) and shallow lake (average depth 8 m, maximum depth 15 m). Clear Lake is comprised of three basins–the Upper Arm, Oaks Arm, and Lower Arm (Figure 1). All three basins are deep enough to be thermally stratified but shallow enough to vertically mix fully several times a year and partially mix almost daily, (i.e. polymictic) (Rueda and Schladow, 2003). Clear Lake is naturally eutrophic and supports large fish and waterfowl populations. Algal blooms have been documented to occur primarily in the late spring, summer, and fall (Winder et al., 2010). Past research has identified the phytoplankton community to be cyanobacteria dominated for most of the year (Horne, 1975). CyanoHABs have increased in frequency at Clear Lake since the mid-1900s to create nuisance scums and odors, which cause public and environmental health concerns (Richerson et al., 1994).
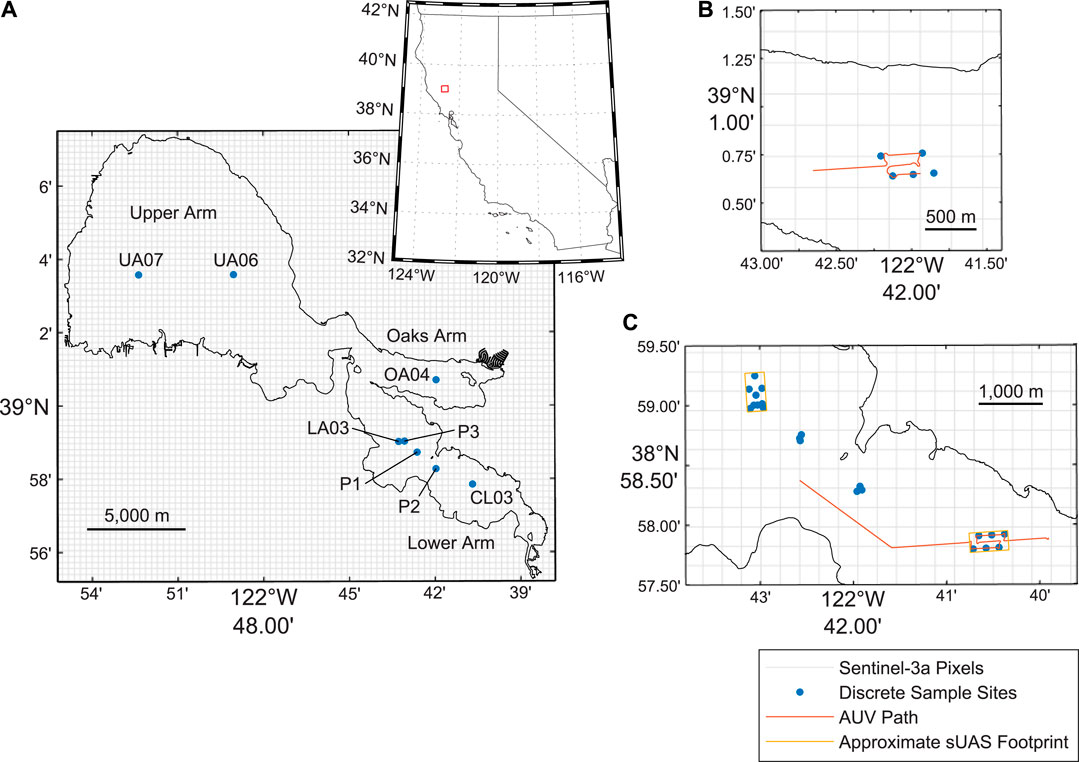
FIGURE 1. Sample site locations in Clear Lake (A) is an overview map of Clear Lake (B) shows the sampling locations in the Oaks Arm (C) shows the sampling locations in the Lower Arm. The 300 × 300 m outlines of the Sentinel-3a pixels are also shown.
Data Collection and Data Processing
Data were collected in Clear Lake using several methods (Table 1; Figure 1). The in situ data collection methods include collection of discrete samples, hyperspectral data measurements using a handheld spectroradiometer, multispectral imaging from a sUAS, and fluorescence and scattering meter measurements from an AUV. Additionally, cyanobacteria index (CI) was calculated from Level 3 OLCI sensor data from the Sentinel-3a satellite. Finally, meteorological and lake temperature data were obtained to provide context to our cyanobacteria measurements.
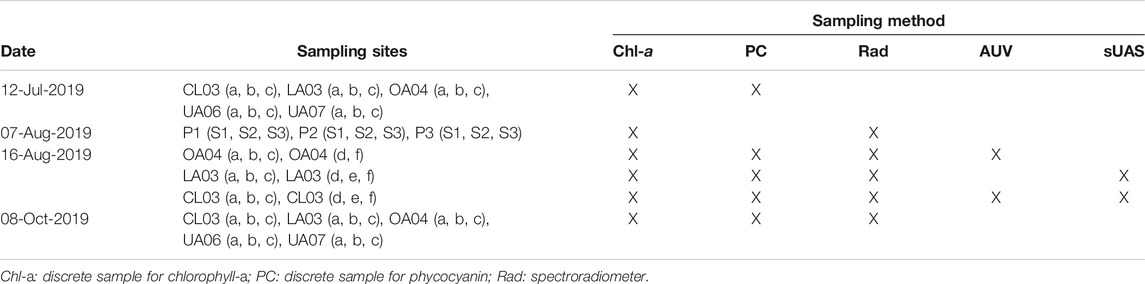
TABLE 1. Summary of data collection. Sampling sites CL03 (a, b, c, d, e, f), LA03 (a, b, c, d, e, f), P1 (S1, S2, S3), P2 (S1, S2, S3), and P3 (S1, S2, S3) are in the Lower Arm of Clear Lake, OA04 (a, b, c, d, f) is in the Oaks Arm, and UA06 (a, b, c) and UA07 (a, b, c) are in the Upper Arm.
Discrete Samples
Discrete water samples were collected across 32 sampling sites on Clear Lake (Table 1; Figure 1), generally located near existing sampling sites in routine water quality monitoring programs. These existing sampling sites were selected to allow for future comparison between the data collected in this study with data collected in routine water quality monitoring programs; those comparisons were not included with this research. To enable comparisons between discrete samples and satellite CI values, the sample sites are located within 19 separate Sentinel-3a pixels near the centers of each Clear Lake basin to avoid shoreline contamination of the satellite pixels. The samples were planned such that three sample sites would be located within one corresponding Sentinel-3a pixel, (e.g. CL03a, CL03b, and CL03c were planned within the same Sentinel-3a pixel and are all located nearby the routine CL03 water quality monitoring site). However, due to an unknown projection issue associated with the Sentinel-3a CI product obtained from the San Francisco Estuary Institute (SFEI) and the National Oceanic and Atmospheric Administration (NOAA) HAB Tool (see https://fhab.sfei.org/; Stumpf et al., 2015) at the time of planning, the sampling sites did not all necessarily occur in the same pixel and more pixels were sampled than originally planned (19 pixels sampled vs. 10 pixels planned). This projection issue was corrected in data processing and analysis.
Discrete water samples were collected during four sampling events in July, August, and October 2019 (Table 1). The sampling dates were chosen to correspond with Sentinel-3a satellite overpasses and with expected summer and fall algal blooms in Clear Lake. The first sampling event in July 2019 included grab sampling from 0.1 m depth. The sampling method was later refined for the remaining sampling events in August and October 2019 to include collection of an integrated depth sample of the top 1 m of the water column. No correction was made to the data despite the differences in sampling method depth. Surface scums were not present at our study sites during sampling so errors arising from the difference in sampling method depth are believed to be minimal. Discrete samples were filtered through pre-combusted (500°C for 2 h) Whatman GF/F filters (0.7 μm nominal pore size). The filters from all four sampling events were analyzed for chlorophyll-a by sample processing by extraction in 90% acetone at −20°C for 24 h following a modified EPA method 445.0 (Arar and Collins, 1997) and sample analysis on a Turner Designs 10 AU fluorometer. The filters from three sampling events (July 12, 2019, August 16, 2019, and October 08, 2019) were analyzed for phycocyanin following modified methods developed by Kasinak et al. (2015); Konopko (2007); Siegelman and Kycia (1978) with sample processing by extraction in 10 mM phosphate buffer (pH 6.8) with three freeze-thaw cycles and then sample analysis using a Turner Designs TD700 fluorometer. The sampling event on August 07, 2019 did not include analysis for phycocyanin because this sample was collected with a different sampling program that does not include sampling and analysis for phycocyanin.
Due to unforeseen complications, only one set of sample filters from the July 12, 2019 and August 16, 2019 sampling events was available for laboratory analysis. These filters were cut in half to analyze both chlorophyll-a and phycocyanin from a single filter. Prior to analysis of the Clear Lake samples, a test was run on split filters vs. whole filter analysis with five replicate samples from another site (surface samples collected from Pinto Lake, CA on October 24, 2019). Three sets of filters were prepared from each sample, with one set of filters analyzed for chlorophyll-a, one set of filters analyzed for phycocyanin, and one set of filters cut in half, with one half analyzed for chlorophyll-a and one half analyzed for phycocyanin. A paired-sample t-test on the whole and half filter data resulted in p-values of 0.42 and 0.77 for the chlorophyll-a and phycocyanin data, respectively. Additionally, the means of the replicates were found to be within a standard deviation of one another. Given the statistically insignificant difference between whole and half-filters, the half-filter method was decided to be not ideal but acceptable in the absence of other measurements.
Spectroscopy Data
High resolution spectral measurements were collected at eight sampling sites on Clear Lake during three sampling events in August and October 2019 (Table 1; Figure 1). Spectral measurements were not made during the July 2019 sampling event because the sampling equipment was not available on that date. The sampling locations were selected such that one sampling site out of every cluster of three discrete sample sites had spectroscopy measurements completed. We could not collect spectral measurements at every discrete sample site due to time constraints. We used a Malvern Panalytical FieldSpec Handheld two Pro spectroradiometer with a 7.5° fore optic angular field of view. The spectroradiometer has a spectral resolution of <3 nm across a range of wavelengths from 375 to 1,075 nm, with a total of 575 spectral bands (discrete wavelengths). At each site, three to four measurements were taken following standard methods (Tomlinson et al., 2016). Measurements over a 10% calibrated spectralon reflectance plate and at the sky were also collected to convert the radiance measurements to dimensionless reflectance during post-processing (Mobley, 1999; Tomlinson et al., 2016).
Sentinel-3a Data
Satellite-derived cyanobacteria index (CI) was calculated from Level 3 data obtained from NOAA for the multispectral OLCI sensor on the Sentinel-3a satellite. Level 3 data products include
where,
Using the approach in Tomlinson et al. (2016), a locally tuned equation comparing chlorophyll-a and phycocyanin to CI was determined for our study site. Previous proposed equations relating CI to chlorophyll-a are shown below (Eq. 3 from Tomlinson et al., 2016 and Eq. 4 from Stumpf et al., 2015).
A least-squares linear regression approach was used to model the relationship between CI and both chlorophyll-a and phycocyanin specific to Clear Lake.
Small Unmanned Aerial System Data
Very high spatial resolution multispectral imagery was collected from the two Lower Arm sites (CL03 and LA03) on August 16, 2019 (Table 1; Figure 1). The sampling date was chosen as a larger effort sampling event when coincident discrete samples, satellite measurements, sUAS, and AUV data could be collected. The sUAS and AUV tools were only available on this sampling date due to resource limitations. The intention was to collect measurements at three locations (two in the Lower Arm and one in the Oaks Arm). These basins were selected for sampling by the sUAS and AUV because at the time of mission planning, they demonstrated the most cyanobacterial activity. However, due to vehicle piloting difficulties only the two Lower Arm sites could be sampled by the sUAS.
We used a MicaSense RedEdge multispectral camera mounted on a Matrice 100 sUAS and operated using DJI GS Pro software using standard methods (Liu et al., 2019b). The MicaSense RedEdge camera, like other commercial multispectral cameras, has individual lenses for each band with discrete exposure times that optimize the radiometric range depending on the target (Kim et al., 2020). Aerial flights were conducted with this sUAS at an average height of 120 m above ground level, resulting in images at a resolution of 8.2 cm/pixel. The flight lines had front and side overlaps of 60%. Before and after each sUAS flight, standard reflectance panel images were captured by the MicaSense camera for later calibration of the data. Individual images were georectified and stitched into a single orthomosaic of multispectral reflectance (true geometrically correct and mosaicked image) using Pix4Dfields software. The planned sUAS flights were intended to cover an area aligned with and equivalent two Sentinel-3a pixels (300 m × 300 m each). However, as previously mentioned, due to an unknown projection issue with the data used in planning, the sUAS flights overlapped with several Sentinel-3a pixels, but none of them were fully covered.
The MicaSense multispectral camera captures reflectance data at five bands: blue (center λ = 475 nm with 20 nm bandwidth Full Width at Half Maximum or FWHM), green (center λ = 560 nm with 20 nm bandwidth FWHM), red (center λ = 668 nm with 10 nm bandwidth FWHM), near infrared (center λ = 840 nm with 40 nm bandwidth FWHM), and red edge (center λ = 717 nm with 10 nm bandwidth FWHM). Chlorophyll-a was derived from the reflectance data using the approach in Ha et al. (2017). Ha et al. (2017) found an exponential equation using a green-red band ratio yielded the best results for their study site (Eq. 5, where B3 = green band and B4 = red band).
We attempted to determine a locally tuned band ratio equation computing chlorophyll-a for our study site by using the coincident discrete sample results (n = 6); however, a clear relationship was not found and the published equation (Eq. 5) was used instead. The chlorophyll-a concentrations determined from Eq. 5 were scaled to the discrete sample chlorophyll-a measurements, where the scaling factor was equal to the ratio of the average of the discrete sample chlorophyll-a results to the average of the sUAS-derived chlorophyll-a values. See Supplementary Section S1 for more information on sUAS data processing.
Autonomous Underwater Vehicle Data
High resolution data were collected using an Autonomous Underwater Vehicle from one Lower Arm site (CL03) and one Oaks Arm site (OA04) on August 16, 2019 (Table 1; Figure 1). The sampling date and sampling locations are discussed in the sUAS data section above. Additionally, the AUV sample locations intended to include two Lower Arm sites and one Oaks Arm site. However, due to vehicle deployment difficulties and time constraints, only one Lower Arm site and the Oaks Arm site could be sampled.
The AUV used is a small Gavia-class AUV. The instruments mounted on the AUV include a Seabird Electronics SBE-49 CTD (Conductivity, Temperature, Depth) sensor and a WETLabs ECO Triplet BBFL2 combination scattering meter and fluorometer. The BBFL2 determines turbidity through measurement of red light scattering at 700 nm; colored dissolved organic matter (CDOM) through fluorescence with excitation at 370 nm and response measurement at 460 nm; and chlorophyll-a through fluorescence with excitation at 470 nm and response measurement at 695 nm. The detection ranges for the BBFL2 sensors are 0–50 μg/L for chlorophyll-a, 0–375 ppb for CDOM, and 0–5 m−1 for the scattering meter. The AUV is also equipped with a navigation system including a combined Teledyne RD Instruments workforce navigator Doppler velocity log (DVL) and a Kearfott inertial navigation system (INS). The AUV was deployed at a cruising speed of 1.5–2 m/s. The AUV mission was designed to have the vehicle run at a constant depth of 0.5 m below the lake surface. A tolerance of three standard deviations from the mean depth was allowed for each mission and all data outside this set tolerance was disregarded during post-processing (Yu et al., 2002; Blackwell et al., 2008; Forrest et al., 2008). The CTD sampled at 16 Hz, the combination scattering meter and fluorometer sampled at approximately 0.9 Hz, and the navigation system records data at 1 Hz. The three datasets were merged with data points interpolated using a linear approach and the fastest sampling rate. The data were then bin averaged to a bin size greater than the slowest sampled dataset. The recorded scattering and fluorescence measurements were converted to turbidity (NTU), CDOM (ppb), and chlorophyll-a (μg/L) using the factory determined dark count offsets (false positives read by the sensor in absolute zero light) and scaling factors. The [back] scattering meter was not calibrated in turbidity (NTU) units using a turbidity solution. An approximate conversion from the sensor single-angle scattering scale factor (m−1 sr−1) to a turbidity value (NTU) was used to report the turbidity measurements. The chlorophyll-a data were further scaled to the results of the adjacent discrete samples to account for differences in the field fluorometry measurements and the laboratory chlorophyll-a extraction measurements. Although scaling the AUV chlorophyll-a measurements by the discrete samples does not ensure accuracy of the AUV results, it allows for easier comparison of the two datasets. As the main purpose of this study is to evaluate the variability of cyanobacteria blooms rather than the magnitude, we feel this approach is acceptable. See Supplementary Section S1 for more information on AUV data processing.
Meteorology and Lake Temperature Data
Meteorological forcing and lake temperature data were acquired for Clear Lake for our sampling dates at multiple locations across the lake’s basins (Cortés and Schladow, 2020). To measure meteorological conditions, a network of seven Davis Instruments Wireless Vantage Pro2 Plus stations installed at the shoreline around the perimeter of Clear Lake measured air temperature, relative humidity, and wind speed and direction every 15 min (see station map in UC Davis Tahoe Environmental Research Center, 2020). Lake surface temperature was determined from a network of Onset Water Temp Pro loggers installed along the near-shore and adjacent to all but one of the meteorological stations (∼0.5 m below lake water level) and also installed on three permanent offshore navigation markers (within the top 2 m of the water column). The thermistors sample every 10 min with 0.2°C accuracy and 0.02°C resolution. Lake water clarity was characterized at each sampling site (Table 1; Figure 1) using a Secchi disk.
Data Analysis
Critical Scales of Variability
CSVs of cyanobacteria were determined for this study using a semivariogram analysis of the AUV and sUAS data using the GeoR Package in R following similar methods as Blackwell et al. (2008) and as described in Diggle and Ribeiro (2007). The CSV is considered the “apparent range” (Blackwell et al., 2008) or the “practical range” (Diggle and Ribeiro, 2007) at which the semivariance levels off, forming the “sill” of the semivariogram. This value was determined by visual examination of the log-log plot of the semivariogram at the point where there is a noticeable change in the slope of the line (see Figure 2 of Moses et al., 2016), which corresponds to the point of leveling off in the semivariogram. The semivariograms were computed for the AUV-acquired chlorophyll-a measurements and the sUAS-derived chlorophyll-a measurements. Two semivariograms were produced for the AUV missions in the Lower Arm and in the Oaks arm. Initially semivariograms were examined for every 500th pixel row and column, (i.e. lines) of sUAS data subsampled from the mosaic of pixel values. A subset of data (every 100th pixel row of the lines 2,500–4,500) were further examined to determine the CSV from the sUAS data. The pixel column data did not produce meaningful results to determine the CSV, likely because the columns are shorter that the rows for our data set. See Supplementary Section S1 for more information on the data processing for the CSV data analysis.
Coefficients of Variation
We calculated the coefficients of variation of the datasets as an additional metric to quantify the variability of the bloom. The coefficient of variation is equal to the ratio of the standard deviation to the mean.
Results
We measured cyanobacteria over four sampling dates in the Summer and Fall 2019 at different sampling locations in Clear Lake, CA (Table 1; Figure 1). The central aim of this work was to characterize the CSV of cyanobacteria. We used five methods to quantify cyanobacteria in the water: 1) laboratory analysis of discrete samples for chlorophyll-a and phycocyanin; 2) hyperspectral radiance measurements from a handheld spectroradiometer; 3) coarse spatial resolution multispectral reflectance data from the OLCI sensor on Sentinel-3a; 4) high spatial resolution multispectral reflectance data collected using a sUAS; and, 5) high spatial resolution scattering and fluorescence data of turbidity, CDOM, and chlorophyll-a collected using an AUV.
Discrete Sampling
The average chlorophyll-a concentration of all the samples was approximately four times larger than the average phycocyanin value, with mean ± standard deviation of 18.7 ± 12.0 μg/L and 4.60 ± 4.55 μg/L for chlorophyll-a and phycocyanin, respectively. For individual samples, maximum values of phycocyanin (25.6 μg/L) were about half the maximum chlorophyll-a concentrations (46.1 μg/L). The mean of the ratio of phycocyanin to chlorophyll-a was 0.57 ± 1.04. The correlation coefficients for the relationship between chlorophyll-a and phycocyanin are 0.54, 0.36, and 0.87 for the July 12, 2019, August 16, 2019, and October 08, 2019 sampling dates, respectively.
As time progressed over the three sampling dates, we observed increasing chlorophyll-a concentrations relative to the concentration of phycocyanin (Figure 2). Chlorophyll-a concentrations were highest on October 08, 2019 (31.5 ± 6.16 μg/L), while the July 12, 2019 and August 16, 2019 concentrations were similar, 11.6 ± 11.6 μg/L and 13.8 ± 5.93 μg/L, respectively. The August 16, 2019 and October 08, 2019 sampling events had comparable phycocyanin concentrations of 1.89 ± 1.14 μg/L and 3.37 ± 1.95 μg/L, respectively. The largest phycocyanin concentrations were obtained on July 12, 2019 (8.88 ± 5.70 μg/L). As a result, the July 12, 2019 sampling event observed a higher average phycocyanin to chlorophyll-a ratio of 1.48 compared to average ratios of 0.19 and 0.10 for the August 16, 2019 and October 08, 2019 events, respectively. Only chlorophyll-a was measured on August 07, 2019 and the mean ± standard deviation was 23.0 ± 6.54 μg/L (n = 9). The coefficients of variation for chlorophyll-a are 100.0, 28.4, 42.9, and 19.5% for July 12, 2019, August 07, 2019, August 16, 2019, and October 08, 2019, respectively. The coefficient of variation for chlorophyll-a for just the CL03 sites in the Lower Arm on August 16, 2019, where coincident AUV and sUAS were taken, is 33%. The coefficients of variation for phycocyanin are 64.2, 60.4, and 57.9% for July 12, 2019, August 16, 2019, and October 08, 2019, respectively. In addition to the chlorophyll-a and phycocyanin measurements on August 16, 2019 and October 08, 2019, we also identified the dominant genera of cyanobacteria as Dolichospermum, Gleotrichia, and Microcystis. The authors would like to note that the results from the July 12, 2019 and August 16, 2019 sampling dates are believed to be underestimated. This is because the sample filters from those dates arrived at room temperature after transfer from lab to another.
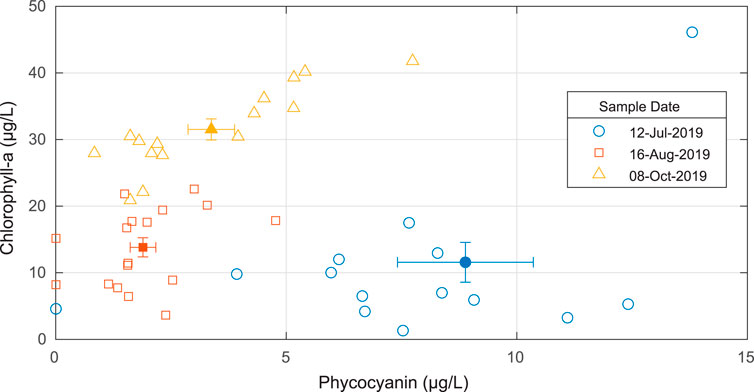
FIGURE 2. Phycocyanin and chlorophyll-a concentrations in discrete samples collected on July 12, 2019, August 16, 2019, and October 08, 2019. The July 12, 2019 sampling event observed a higher average phycocyanin to chlorophyll-a ratio compared to the other sampling dates. The averages for each sampling date are shown as the solid symbols with the vertical error bars representing the standard error of the chlorophyll-a measurements and the horizontal error bars representing the standard error of the phycocyanin measurements. There is an outlier (phycocyanin = 25.6 μg/L and chlorophyll-a = 27.2 μg/L) from July 12, 2019 that is not shown in this plot for visualization purposes.
To provide context to our measurements of cyanobacteria, we measured meteorological forcing and lake surface temperature data. Meteorological variables and lakes surface temperatures were variable between the different basins (Table 2). Generally, the Lower and Oaks arms were windier than the Upper Arm. During our sampling dates, wind direction was generally from the Northwest direction. The air and lake surface temperature results are varied but there appears to be some tendency of the Lower and Oaks Arms to have warmer air temperature than the Upper Arm. The relative humidity results also do not show a clear trend. Finally, each basin has similar Secchi depths, but the Oaks Arm consistently had the lowest clarity.
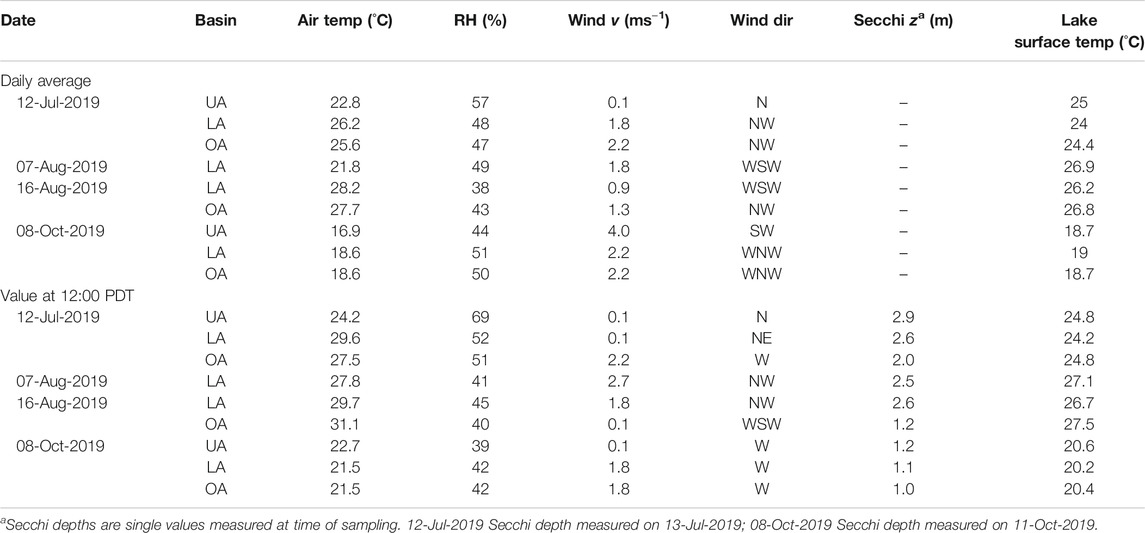
TABLE 2. Meteorological forcing and lake surface temperature data results. Data provided (columns from left to right) include sampling date, basin sampled, air temperature, relative humidity, wind speed, wind direction, Secchi depth, and lake surface temperature. Generally, the Lower and Oaks arms were windier and have warmer air temperature than the Upper Arm during our sampling events. The Oaks Arm consistently has the lowest clarity based on the Secchi depths.
In Situ and Satellite-Based Spectra and Cyanobacteria Index Values
We calculated hyperspectral reflectance data from spectroradiometer measurements at several locations on August 07, 2019, August 16, 2019, and October 08, 2019 along with concurrent multispectral satellite reflectance data for all sampling locations and times. Paired hyperspectral/multispectral reflectance data from the spectroradiometer measurements and corresponding Sentinel-3a pixels on August 07, 2019, August 16, 2019 and October 08, 2019 were examined across the spectrum 600–750 nm, where most of the spectral features for phycocyanin and chlorophyll-a occur (Figure 3). The in situ measurements yield a much smoother curve with 150 bands of spectroradiometer data across the range of wavelengths (600–750 nm). Sentinel-3a captured reflectance across 21 bands, and thus, yielded coarser curves. The spectra are presented across the full visible spectrum (400–750 nm) in Supplementary Figure S1.
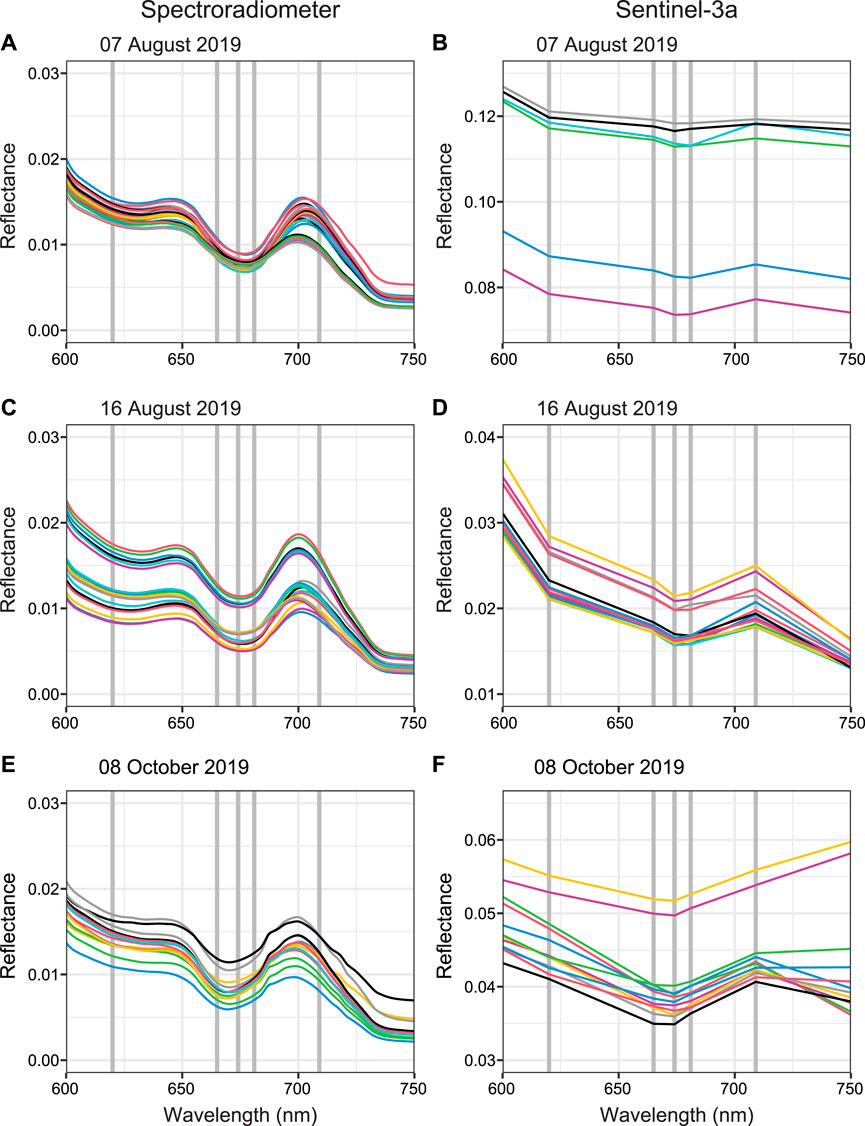
FIGURE 3. Spectra from in situ spectroradiometer (A, C, E) and Sentinel-3 pixel data (B, D, F) for different sampling dates (A–B) August 07, 2019 (C–D) August 16, 2019, and (E–F) October 08, 2019. The field spectra demonstrate spectral features for phycocyanin and chlorophyll-a which are less discernable in the coarser satellite data. Each colored line represented the results from a different discrete sample site (n = 61 total over three sampling dates) for the spectroradiometer data and a different pixel (n = 42 total over three sampling dates) for satellite data. The vertical gray lines represent the spectral band centers of Sentinel-3a. Note the difference in the y-axis range between the reflectance plots. Reflectance is dimensionless.
The hyperspectral measurements demonstrate spectral signatures expected from algal and cyanobacteria-laden waters including strong chlorophyll-a absorption of red light at ∼675 nm (Figures 3A,C,E). A slight phycocyanin absorption feature (characterized by reflectance trough) is observed at 615–630 nm as well as the reflectance peak at ∼650 nm. The absorption feature at 615–630 nm is more prominently observed in the data for the two August sampling dates.
The coarser reflectance plots (Figures 3B,D,F) of the multispectral satellite data do not show the absorption and reflectance features specific to phycocyanin and chlorophyll-a as strongly as the hyperspectral reflectance plots. The absorption feature by chlorophyll-a of red light (674 nm) is present, while the phycocyanin spectral features at 620 and 650 nm are absent. The reflectance values from the satellite data are higher than the reflectance values from the spectroradiometer data particularly for the August 07, 2019 sampling date, which has the highest satellite reflectance values of any of the sampling dates.
The CI calculated from the spectroradiometer measurements (Field CI) were compared to the corresponding CI from satellite measurements (Satellite CI) (Figure 4). CI was calculated both using the original equation defined by Wynne et al. (2008 and 2010) and the revised equation which includes an exclusionary criterion for the spectral shape around wavelength 665 nm defined by Matthews et al. (2012) and validated for lakes in the eastern US by Lunetta et al. (2015). CI without the exclusionary criterion shows some seasonal trend with the highest CI observed on August 07, 2019 with decreasing CI until the lowest values on October 08, 2019 (Figure 4A). This trend is consistent between the Field CI and the Satellite CI. We obtained the highest CI values using satellite measurements, which were four-fold larger than the overall mean Satellite CI, and only two-fold larger when using field measurements. The Field CI are generally higher than the Satellite CI for August 07, 2019 whereas the reverse is true for October 08, 2019. The standard deviation was 1.04 × 10−3 for all the calculated CI values. All field reflectance data failed to exceed the SS{665} exclusionary criterion threshold, resulting in Field CI values of zero (Figure 4B). The same is true for the October 08, 2019 satellite data.
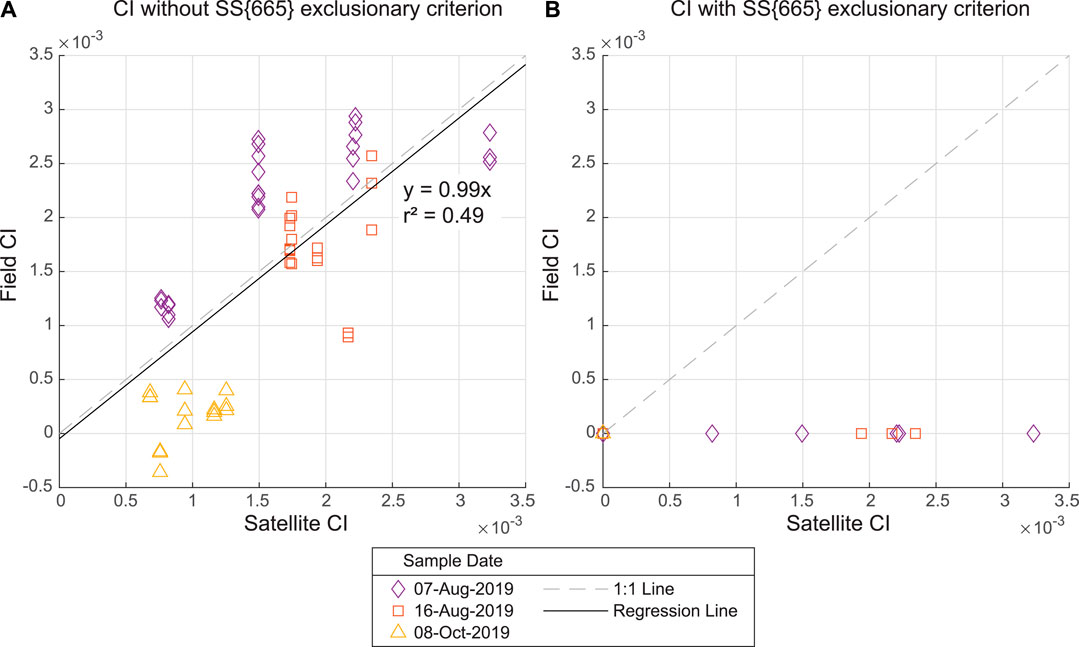
FIGURE 4. (A) CI without the SS{665} exclusionary criterion for field spectroradiometer measurements (y-axis) and the Sentinel-3 measurements (x-axis) for the same sampling dates (B) CI with the SS{665} exclusionary criterion for field spectroradiometer measurements (y-axis) and the Sentinel-3 measurements (x-axis) for the same sampling dates. The CI calculated without the SS{665} exclusionary criteria demonstrates good correlation between the field CI and satellite CI. The CI calculated with the exclusionary criteria for the field measurements resulted in CI equal to zero in all cases. The CI calculated with the exclusionary criteria for the satellite measurements resulted in CI equal to zero for all of October 08, 2019 data.
Relationship Between Cyanobacteria Index, Chlorophyll-a, and Phycocyanin
The relationship between Field CI and chlorophyll-a was significant (p < 0.05) on only one sampling day (August 07, 2019). For Satellite CI and chlorophyll-a, the relationship was significant for three sampling days, and there was a significantly positive relationship across all sampling days (Figures 5A,B). The October 08, 2019 did not fit the same trend as the other dates for Field CI vs. chlorophyll-a. For this date, we observe the highest chlorophyll-a concentrations but also the lowest Field CI (Figure 5A). The Satellite CI to chlorophyll-a does not show this same trend with the October 08, 2019 Satellite CI values similar to the other sampling dates (Figure 5B). The CI to phycocyanin relationship is less clear, although there is a positive linear trend evident in the July 12, 2019 data (only Satellite CI is available for this date) (Figures 5C,D).
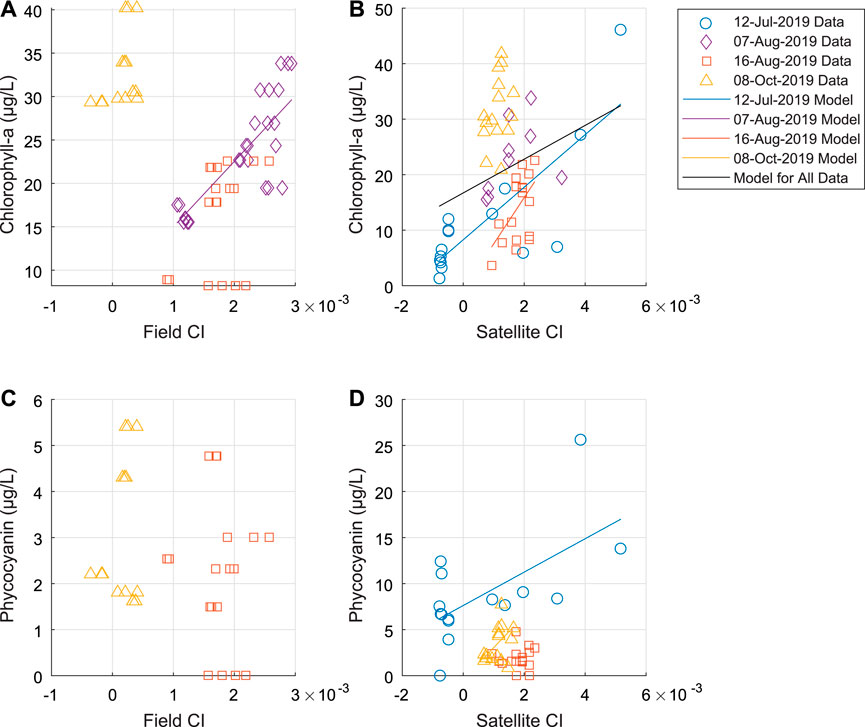
FIGURE 5. Relationship of (A) CI from field spectroradiometer data to chlorophyll-a concentration (B) CI from satellite data to chlorophyll-a concentration (C, D) as (A, B) except for to phycocyanin concentration. Note the difference in axes ranges between each plot. Linear models are shown for those relationships that are significant (corrected p-value < 0.05, where p-value is corrected using Benjamini and Hochberg False Discovery Rate procedure). Few of the modeled relationships are significant.
We found significant relationships (corrected p-value < 0.05, where p-value is corrected using Benjamini and Hochberg False Discovery Rate procedure) for only six out of sixteen evaluated linear models between Field and Satellite CI to chlorophyll-a and phycocyanin for all the available data as well as each individual sampling date. Of those significant relationships, we found moderately low to moderate correlation (r2 values between 0.08–0.39) for most of the linear models (Table 3). The linear model between Field CI and chlorophyll-a for August 07, 2019 and Satellite CI and chlorophyll-a for July 12, 2019 both demonstrated moderately high correlation with r2 values of 0.68 and 0.65, respectively (Table 3).
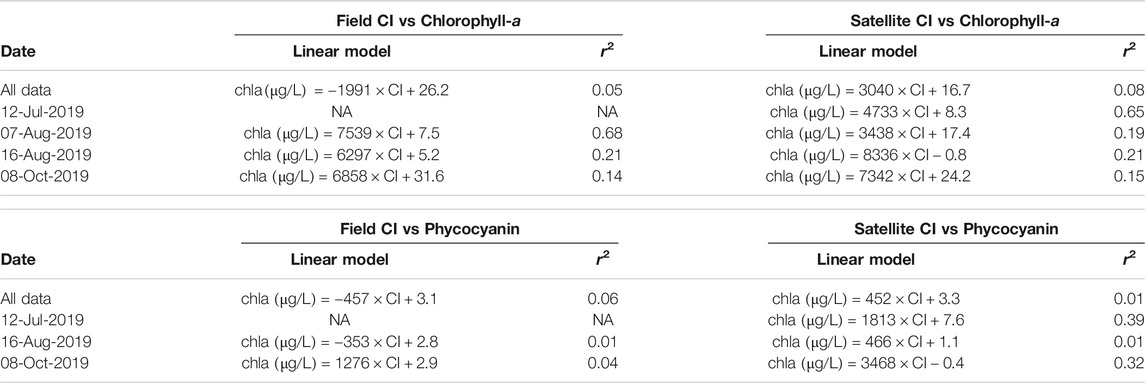
TABLE 3. Linear model parameters as well as the model performances of results of least-squares linear regression analysis relating the calculated CI to observed chlorophyll-a and phycocyanin concentrations. Only the linear models Field CI vs Chlorophyll-a (07-Aug-2019), Satellite CI vs Chlorophyll-a (All Data, 12-Jul-2019, and 16-Aug-2019), and Phycocyanin vs Satellite CI (12-Jul-2019 and 8-Oct-2019) are significant (corrected p-value < 0.05, where p-value is corrected using Benjamini and Hochberg False Discovery Rate procedure). The significant models have positive linear slopes. The significant models have moderately low to moderately high correlation.
Small Unmanned Aerial System Measurements
Conditions on the day of sUAS deployment were clear and calm, with sunny skies and low to no cloud cover. The sUAS-derived chlorophyll-a ranged from 0 to 39.7 μg/L. For image presentation of the data the upper and lower bounds were set to the 99.7 percentile (3σ = 18.2 μg/L) and 0.3 percentile (−3σ = 11.7 μg/L), respectively (Figure 6). The chlorophyll-a concentrations are variable throughout the site with higher concentrations observed near the northern portions and through an area in the eastern portion of the site. The average calculated chlorophyll-a value was 15.3 mg/L ± 0.90 μg/L. The coefficient of variation for the sUAS-derived chlorophyll-a is 5.92%.
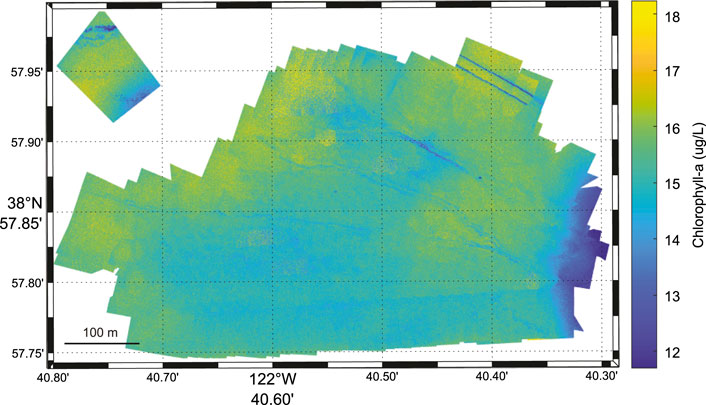
FIGURE 6. sUAS-derived chlorophyll-a (μg/L) image from August 16, 2019. Spatial variability is observed in the sUAS-derived chlorophyll-a image with higher concentrations observed near the northern portions and through an area in the eastern portion of the site.
Autonomous Underwater Vehicle Measurements
Concurrent with the sUAS flights, an AUV was deployed on August 16, 2019 in the Lower and Oaks Arms of Clear Lake. Sensors mounted on the AUV collected continuous measurements of turbidity, chlorophyll-a, and CDOM during deployment at an average depth below the free water surface of ∼0.6 m in the Lower Arm and ∼0.9 m in the Oaks Arm (Figure 7). Conditions were mild on the day of deployment with low winds (<5 kph) and low wave heights (<10 cm). Lake clarity was low, similar to other observations made for our other sampling events. Due to technical difficulties during operations, data were only obtained from the Lower Arm site CL03 and the Oaks Arm site OA04. Additionally, AUV and sensor hardware issues during deployment may have resulted in strings of zero values, which were subsequently removed from the dataset (Figure 7).
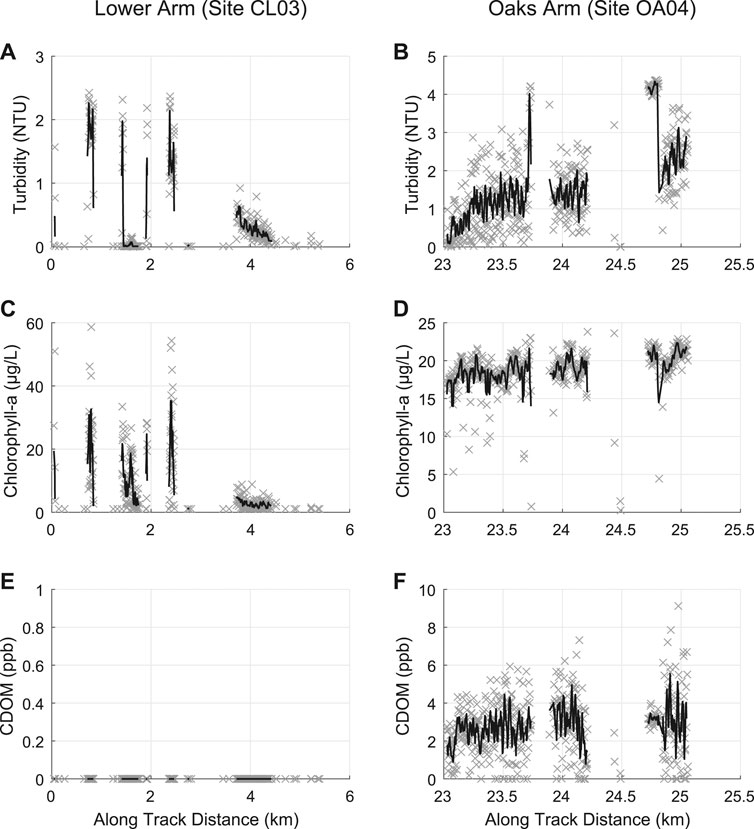
FIGURE 7. AUV data results for (A, B) turbidity (NTU) (C, D) chlorophyll-a (μg/L), and (E, F) CDOM (ppb) for (A, C, E) the Lower Arm and (B, D, F) the Oaks Arm. Running averages (5 consecutive results) are shown for each plot in black. Turbidity is higher in the Oaks Arm than the Lower Arm. The maximum chlorophyll-a concentrations are higher in the Lower Arm while the average concentrations are roughly twice as high in the Oaks Arm. CDOM values were zero in the Lower Arm and averaged 2.7 ppb in the Oaks Arm.
The turbidity, chlorophyll-a, and CDOM results from the AUV mission showed spatial variability varied among the basins. Turbidity was higher in the Oaks Arm than in the Lower Arm, with averages of 1.58 ± 1.13 NTU and 0.57 ± 0.72 NTU, respectively, and maximum values approximately double in the Oaks Arm (Figures 7A,B). The coefficients of variation for turbidity are 127% in the Lower Arm and 71.4% in the Oaks Arm. The chlorophyll-a results had higher maxima in the Lower Arm than in the Oaks Arm (58.6 μg/L and 23.8 μg/L, respectively), but higher average chlorophyll-a concentrations in the Oaks Arm compared to the Lower Arm (18.7 μg/L and 9.26 μg/L, respectively) (Figures 7C,D). The coefficients of variation for chlorophyll-a are 119% in the Lower Arm and 15.2% in the Oaks Arm. The CDOM concentrations in the Lower Arm were below detection. This occurs because the factory determined offset and scaling factor can result in some CDOM values calculated from the fluorescence counts to be below the detection limit, which we interpret as zero values. The CDOM concentrations ranged from 0 to 9.12 ppb in the Oaks Arm and averaged of 2.78 ± 1.55 ppb (Figures 7E,F). The coefficient of variation for CDOM in the Oaks Arm is 56.0%.
Co-Located and Coincident Measurements
We used the concurrent and co-located measurements to obtain a more complete characterization of a cyanobacteria bloom in the Lower Arm of Clear Lake on August 16, 2019 (Figure 8). There is observed variability in the measurements between the different sensors and platforms employed at the site. As seen in the southwest corner of the site, the lowest discrete sample result is observed (8.21 μg/L chlorophyll-a), whereas the adjacent AUV fluorometry results indicate chlorophyll-a levels with concentrations as high as 54.3 μg/L. The range of average ± standard deviation of chlorophyll-a values for this site on this sampling date were 15.3 ± 5.02 μg/L for the discrete samples, 9.26 ± 11.1 μg/L for the AUV fluorometer results, and 15.3 ± 0.90 μg/L for the sUAS-derived chlorophyll-a results. In addition to variance between platforms, high spatial heterogeneity is also observed inside each satellite pixel. At sampling site (CL03) on August 16, 2019, the discrete sample chlorophyll-a results range from 8.21 to 22.6 μg/L; the AUV chlorophyll-a results range from 1.06 to 58.6 μg/L; and the sUAS-derived chlorophyll-a results range from 0 to 39.7 μg/L.
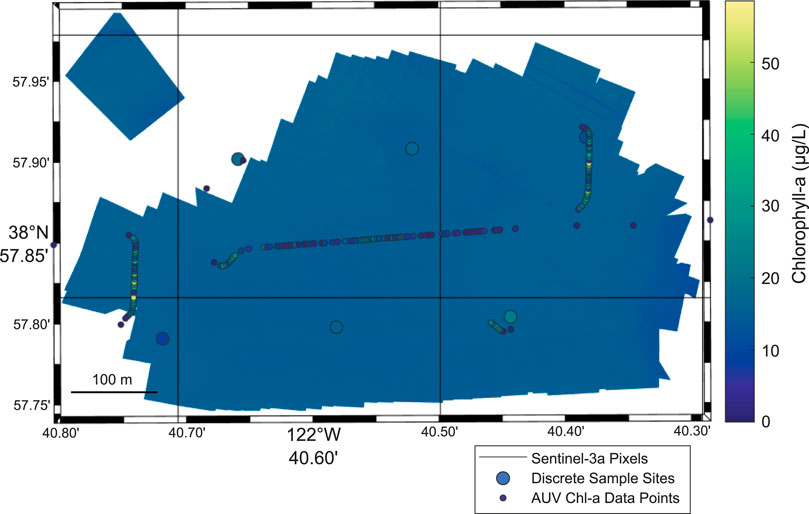
FIGURE 8. Concurrent measurements of cyanobacteria bloom in the Lower Arm of Clear Lake (site CL03) on August 16, 2019. Variability is observed in the chlorophyll-a measurements between the different sampling platforms. Also, there is high intra-pixel variability of the chlorophyll-a measurements. Image includes grab sample chlorophyll-a observations (large circles), AUV chlorophyll-a measurements (small circles), and sUAS-derived chlorophyll-a as background image. Sentinel-3a satellite pixels shown as dark grey outlines.
Critical Scales of Variability
Chlorophyll-a concentrations are spatially autocorrelated in the AUV and sUAS data. The semivariograms have a mostly asymptotic shape with the value of semivariance increasing with distance and then leveling off (Figure 9). The spatial autocorrelation is stronger in the AUV data than the sUAS, and especially in the AUV mission in the Lower Arm where the variation overall is very low between measurements. From the semivariograms of the AUV-acquired chlorophyll-a measurements (Figures 9A,B) and also considering their log-log plots, we find the CSV occurs at distances of 70–100 m. From the semivariograms and the log-log plots of the sUAS-derived chlorophyll-a measurements, we find the CSV occurs at roughly 175 m on average with the CSV ranging from 70 to 300 m for the 21 lines evaluated (Figures 9C,D).
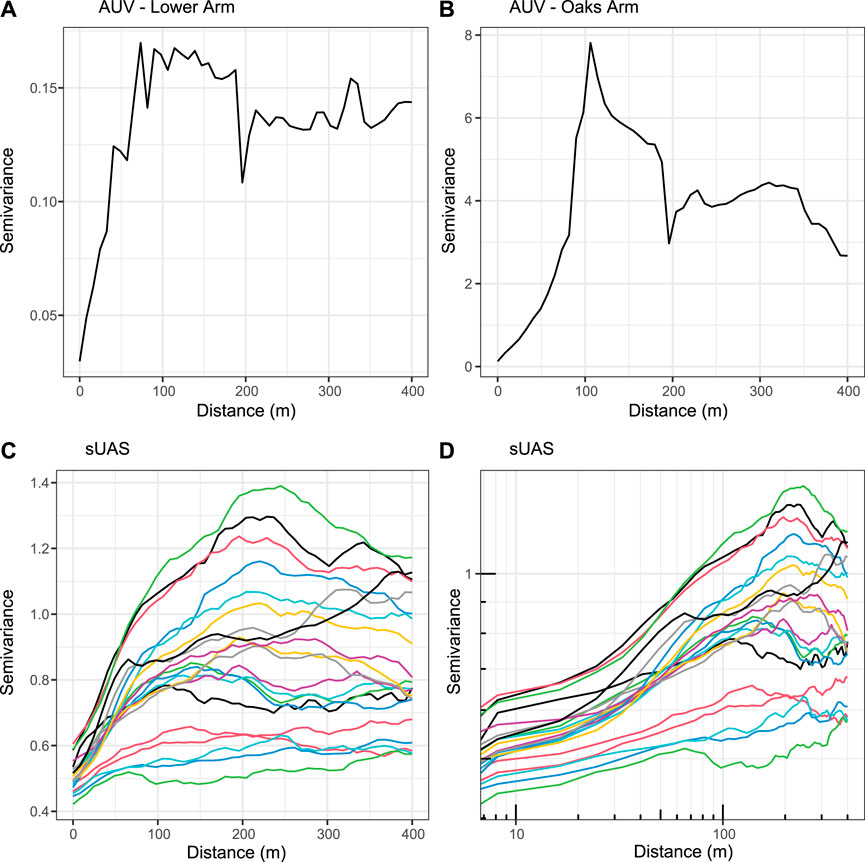
FIGURE 9. Semivariograms of chlorophyll-a measurements from (A) AUV Missions in Lower Arm (B) AUV Mission in Oaks Arm; and (C) lines extracted from sUAS data in Lower Arm. Panel (D) shows the log-log scale of the sUAS semivariograms shown in panel (C), which is used for determining the CSV. Semivariograms demonstrate spatial autocorrelation with close data points having less variation. The semivariograms level off at the CSV at roughly 70–100 m for the AUV data and at 175 m on average for the sUAS data, with values ranging from 70 to 300 m for the 21 lines evaluated.
A semivariogram analysis was also completed on the AUV turbidity and CDOM data. The semivariogram analysis on the CDOM data did not yield any meaningful results for the Lower Arm and the Oaks Arm mostly demonstrated a leveling off pattern (see Supplementary Figure S2). The CSV for the CDOM is estimated to be ∼100 m based on the semivariogram for the Oaks Arm data. The semivariogram for the turbidity data were similar to those for chlorophyll-a (see Supplementary Figure S3). The CSV for Turbidity is estimated to be between 90 and 100 m.
Discussion
While satellite tools have clear advantages with early detection of cyanoHABs, which has quantifiable socioeconomic benefits (Stroming et al., 2020), they do not adequately characterize bloom spatial variability (Kutser, 2009). This study used a multiplatform sampling approach to measure cyanoHABs to characterize spatial variability of the blooms. Each sampling method provides a perspective of a cyanobacteria bloom from a different vantage point. Satellite imagery provides high coverage and temporal resolution of the bloom. sUAS-acquired imagery provides good coverage and high spatial resolution of the surface of the bloom. Measurements from the AUV provides high spatial resolution measurements of the blooms underwater. Finally, the discrete samples and spectroradiometer measurements provide context and validation of the other methods as well as an understanding of the surface forcing conditions.
Synoptic View of Cyanobacterial Blooms
Each of cyanobacteria sampling methods employed was a compromise of the sampling scale and spatial, temporal, and, in some cases, spectral resolution. While previous studies have used AUV, (e.g. Robbins et al., 2006; Blackwell et al., 2008) and sUAS platforms, (e.g. Kislik et al., 2018), a more complete picture of cyanobacterial blooms is obtained if these high resolution platforms are deployed concurrently, which, to our knowledge, has not been done to date. When comparing the measurements from the discrete samples, AUV, and sUAS, we find the discrete samples fail to capture the spatial variability in chlorophyll-a concentrations (Figure 8). Based on their coefficients of variation, the discrete samples only capture 28% of the variability measured by the AUV. The discrete samples are limited to six disparate locations and only represent the chlorophyll-a concentration for each discrete point. However, these sample results can be scaled up to the higher spatial resolution provided by the AUV and sUAS measurements by providing accurate measurements to scale the AUV results to (Figures 7C,D) and by informing models relating the sUAS measurements to chlorophyll-a concentrations (Figure 6). These higher resolution measurements demonstrate the inherent spatial variability of these blooms and how discrete sampling under resolves bloom dynamics.
The high spatial variability is also observed within each satellite pixel. The intra-pixel variability is reflected in the standard deviation of the AUV chlorophyll-a measurements within each pixel, which was on average 5.37 μg/L (n = 10, only pixels with a minimum of 20 AUV points were included). Using this average standard deviation, we find at least 28 discrete samples are needed to estimate the chlorophyll-a concentrations of a bloom (95% confidence interval with 2 μg/L margin of error) within a 300 × 300 m pixel (Israel, 1992). Collecting this many samples is impractical for many research and monitoring programs, which highlights the need for calibrated multi-platform measurement programs to more precisely measure and track bloom densities.
The inter-basin variability is observed with respect to the meteorological data. Generally, and although the observed differences are slight, the meteorological and lake surface temperature data (Table 2) near the time of sampling (values at 12:00 local time) show the air and lake water surface temperatures were warmer and the wind speeds were calmer (thus lower mixing) for the basins that demonstrated the highest ratio of phycocyanin to chlorophyll-a for that sampling date, which indicates dominance by cyanobacteria over phytoplankton. These include the Upper Arm for July 12, 2019, Oaks Arm for August 16, 2019, and the Upper Arm for October 08, 2019. These observations are consistent with the understanding that cyanobacteria favor warm temperatures and calm, stratified lake conditions, and in such conditions will outcompete phytoplankton (Paerl and Huisman, 2008). Our results support the concern that cyanobacteria blooms are expected to increase with increasing global temperatures associated with climate change (Paerl and Huisman, 2009).
Critical Scales of Variability of Cyanobacterial Blooms
CSVs of cyanobacterial blooms are the length scales necessary for detecting the spatial variability or “patchiness” observed in blooms. Previous phytoplankton and cyanobacteria bloom CSVs have been less than the spatial resolution for Sentinel-3a (300 m). Wrigley and Horne (1974) visually identified length scales on the orders of meters for detecting the microstructure variation of cyanoHABs in Clear Lake. They further identified complex patterns of cyanoHABs as not being detectable by conventional boat-based sampling techniques. Blackwell et al. (2008) computed the CSV for fluorescence measurements from an AUV-platform at sub-kilometer scales ranging from 23 to 170 m in coastal systems. While the work of Blackwell et al. (2008) was not in a freshwater system, they used a similar vehicle and instrumentation as in this study. Moses et al. (2016) evaluated scales of variability of ocean color parameters (including phytoplankton) in coastal systems by determining the distance at a critical change in slope for the plot of distance vs. coefficient of variance using ship-based, airborne, and satellite data. They found scales of variability between 75 and 600 m, with an average distance of 200 m. Finally, Vander Woude et al. (2019) identified scales of variability for cyanoHABs in the Great Lakes from hyperspectral data using decorrelation scales. They found scales of variability ranged from 8 to 335 m.
The CSV is necessary to improve sampling plans by selecting the sampling resolution necessary to adequately characterize a bloom (Vander Woude et al., 2019). We find the AUV fluorometer results correspond to a CSV of 70–100 m and the sUAS-derived chlorophyll-a concentrations correspond to a CSV of approximately 175 m. These length scales of variability are less than the 300 m pixel size of the OLCI-derived CI. The CSV found by this and other studies (e.g. Blackwell et al., 2008; Moses et al., 2016; Vander Woude et al., 2019) should be used to inform how distant to sample for cyanobacteria to ensure the bloom is adequately resolved. Additionally, the CSV may inform sensor specifications for future satellite development to have spatial resolutions equal to or finer than this CSV while maintaining similar temporal and spectral scales to Sentinel-3 to adequately monitor cyanobacterial blooms (such as those found by Moses et al., 2016 for sensor design over coastal targets).
Challenges of Multimodal Platform Sampling
Despite being able to characterize cyanobacteria blooms, there are significant challenges associated with each method detailed in this work. Firstly, as shown in the CSV analysis, one of the biggest disadvantages of using satellite imagery is that the CSV for cyanobacteria blooms are finer than the Sentinel-3a pixel size of 300 m. Secondly, the results of this research also indicate poor performance of the revised CI algorithm for Clear Lake. Despite these challenges, this and other satellite-based remote sensing tools are invaluable for water managers and researchers because of their repeat measurements at high temporal frequency and spatial coverage, which provides data on cyanoHABs where monitoring programs are currently lacking (Matthews, 2011). Furthermore, even without calibration and validation, we speculate the CI algorithm still provides valuable information on development and trends of cyanoHABs.
As shown in Figure 4A, the CI algorithm without the exclusionary criterion demonstrates good performance as the results of the CI follow a 1:1 line on the plot. In contrast, the revised CI algorithm using the exclusionary criterion, as suggested for best practice (Stumpf et al., 2015; Wynne et al., 2018), produces false negatives. The CI with the exclusionary criterion calculated from the spectroradiometer measurements results in all CI values equal to zero (Figure 4B). Additionally, the CI with the exclusionary criterion calculated from the satellite measurements from October 08, 2019 results in all CI values equal to zero. Visual observations in the field and microscopy confirmed that cyanobacteria were present on the sampling dates, in contradiction to the CI results equal to zero.
The exclusionary criteria of SS{665} is less sensitive than the original SS{681} CI algorithm of spectral shape around 681 nm, and thus does not adequately capture low-level cyanobacteria blooms (Urquhart et al., 2017). The spectral shape around 665 nm (SS{665}) is influenced by increased absorption of the 620 nm band by phycocyanin compared to chlorophyll-a (Lunetta et al., 2015). Our results show an absorption feature at 615–630 nm (Figures 3A,C,E), however, the depression is not significant enough to change SS{665} from negative to positive in order for the revised CI algorithm with the exclusionary criterion to identify cyanobacteria. Although the cyanobacteria blooms during our sampling dates were not as large as has been observed on Clear Lake in other years, there were still cyanobacteria present despite not being detected using the CI algorithm with the exclusionary criterion. The SS{665} exclusionary criteria is designed to reduce the rate of false-positives (Lunetta et al., 2015), however, as seen in our results, it also increases the rate of false-negatives by failing to detect low-concentration blooms. These low detections of cyanobacteria are still valuable (Matthews et al., 2012) as they allow water managers to observe and prepare for the onset of blooms. We recommend continued data collection and research to tune the exclusionary criterion for improved performance at Clear Lake, and likely other lakes and reservoirs. The exclusionary criterion could be tuned by adjusting the zero threshold so that low level blooms would result in a SS{665} greater than the revised threshold. This adjustment could improve the algorithm results for detection of low level cyanobacteria blooms.
In addition to evaluating the performance of the CI algorithm, we evaluated the relationship of CI to chlorophyll-a and phycocyanin. Previous research has shown mixed success when comparing CI to chlorophyll-a and to phycocyanin. Poor correlations between CI and phycocyanin and chlorophyll-a have been reported by Kudela et al. (2015) and Xu et al. (2019), respectively. However, there has been better success by others (Tomlinson et al., 2016 found an r2 = 0.95 for the relationship of CI to chlorophyll-a). Additionally, on a continental scale, the CI has shown good correlation to cyanobacterial abundance (cells/mL) (Lunetta et al., 2015; Clark et al., 2017). We feel the mixed success of establishing a relationship of CI to chlorophyll-a and phycocyanin in this study may be due to limitations, both in scope and robustness, of our dataset. Our dataset does not validate nor disprove the CI algorithm, rather our work shows that more research is needed.
Inland waters are optically complex (Ortiz et al., 2019) meaning the application of remote sensing tools is challenging. Use of spectral decomposition methods aimed at identifying the specific components of a bloom may prove more useful for some inland waters, where spectral shape algorithms such as the CI are unsuccessful. This is shown by Avouris and Ortiz (2019) with their use of varimax-rotated principal component analysis to partition the spectral components of a bloom, although they also acknowledge that further research is needed. The confounding factors for use of remote sensing methods in optically complex waters and our findings in the variation in the CI to chlorophyll-a and phycocyanin relationship emphasize the need to better understand how CI performs on specific waterbodies of interest when making decisions based on CI values.
On a finer scale, one of the main challenges associated with using a fluorometer sensor on an AUV is the effect of non-photochemical quenching (NPQ) on chlorophyll-a measurements. NPQ is the process by which plants and algae dissipate excess light energy than is needed for photosynthesis (Müller et al., 2001). NPQ is also known to occur in cyanobacteria and reduces chlorophyll-a fluorescence (Humbert and Törökné, 2017). Therefore, ground truthing data using fluorometry for comparison to satellite remote sensing products must account for the of decrease in daytime chlorophyll-a fluorescence due to NPQ (Carberry et al., 2019). In situ data collected for comparison to satellite products is ideally collected near the surface of the water and close to the time of daytime satellite overpass, which in the case of Sentinel-3a for Clear Lake is approximately 12:00 pm (local time). This directly conflicts with collection of chlorophyll-a data using fluorometry to avoid the impacts of NPQ, which occur closer to the water surface and follow the diurnal parabolic pattern of shortwave radiation (see the results of Austin, 2019). Further research into alleviating and/or accounting for the impacts of NPQ on fluorescence measurements for satellite validation is needed. However, even without this NPQ correction applied to fluorescence datasets, we find AUV-acquired fluorescence data are useful for determining the CSV of the blooms even if the relative magnitude of chlorophyll-a concentrations remains poorly quantified.
In addition to the challenges associated with fluorometry measurements on the AUV, we found difficulties with the other remote sensing methods employed in this research. Although aerial imaging with sUAS flights provides a larger coverage and higher spatial resolution view of a cyanobacterial bloom, there are challenges associated with the sUAS multispectral imaging method. One challenge is that there may be uncertainty in the sUAS measurements due to a potentially lower signal to noise ratio of the MicaSense camera over a water body, due to the lower radiance level than typical terrestrial targets (Kim et al., 2020). Additionally, the coarser spectral resolution of the typical multiband multispectral camera limits the ability to capture the fine and narrower absorption features observed for phycocyanin and chlorophyll-a in the hyperspectral spectroradiometer data (Figure 3). The MicaSense camera used for our study has very few bands at limited wavelengths, and this did not allow for calculation of the CI from the sUAS data, although it did allow for calculation of chlorophyll-a from a known band ratio relationship (Ha et al., 2017). A locally tuned chlorophyll-a band ratio equation could not be determined for Clear Lake because there were not enough coincident measurements (n = 6). There are consequences of using a published equation tuned to another study site (Lake Ba Be in Vietnam in the case of Ha et al., 2017). We speculate that a locally tuned relationship would yield more accurate results. In the case of this study, the unscaled sUAS-derived chlorophyll-a measurements using the published equation were much lower than the discrete sample chlorophyll-a results. For this reason, we scaled the sUAS-derived chlorophyll-a results so that the average equals the average of the adjacent discrete samples. This allowed for easier comparison of the datasets. Additionally, the main purpose of this study is to evaluate the variability of cyanobacteria blooms and not necessarily the magnitude of the chlorophyll-a results. Thus, we acknowledge that the sUAS-derived chlorophyll-a results without a locally tuned equation, although scaled to the discrete sample results, should not be used to directly consider the magnitude of the concentrations individually. Further research with additional discrete sampling coincident to sUAS flights is needed to develop a locally tuned equation relating chlorophyll-a to a reflectance band ratio for this site in order for the magnitudes of the sUAS chlorophyll-a results to be meaningful. Finally, we encountered challenges with photomosaicing the sUAS images over water because there are limited static reference points for image matchups and further challenges due to wave action. Due to these challenges, we were not successful in photomosaicing the images from the second sUAS site (LA03) and that data is not presented in this paper.
Conclusion
This research used a multiplatform sampling approach to evaluate the spatial variability including the CSV of cyanobacteria blooms. We find the CSV for cyanobacteria blooms is on the order of 70–175 m, which should be considered when planning sampling efforts. A multiplatform approach provides a more holistic view of a cyanobacteria bloom as each sampling method is completed at different sampling scales and resolutions. We found high intra-pixel variability and also variability between methods at discrete sampling locations. Based on intra-pixel variability of our measurements, we determined a sample size of 28 discrete samples per 300 × 300 m pixel is necessary to adequately characterize the variability of a bloom. Finally, we find low sensitivity of the revised CI algorithm with exclusionary criteria, which failed to detect cyanobacteria at Clear Lake during our sampling events. As such, the exclusionary criterion should be tuned for Clear Lake and potentially for all lakes across California, with the zero threshold adjusted to improve the algorithm results for low level cyanobacteria blooms.
With many lakes across the globe experiencing an increase in the frequency and severity of harmful algal blooms of cyanobacteria (Taranu et al., 2015; Ho et al., 2019), there is a need to develop of tools for water managers to understand and predict their inception. Satellite-based remote sensing tools have emerged as a solution for water managers to monitor the onset and development of harmful algal blooms (Coffer et al., 2020). This research provides data for ground-truthing and algorithm validation, which is essential before widespread use and data interpretation of these satellite products can take place. However, this higher resolution data from autonomous platforms also demonstrates that satellite measurements under-resolve the spatial variability of cyanoHABs. Therefore, strategies will need to be used to scale data between these different platforms. Validation of remote sensing tools will also allow for high temporal resolution cyanobacteria data to be easily accessible by water managers which will aid as a decision support tool. This data will reveal daily, seasonal, and interannual trends, which will be useful to researchers with understanding the drivers of cyanobacteria blooms and determining appropriate engineering solutions to manage large scale harmful algal blooms.
Data Availability Statement
The raw data supporting the conclusion of this article will be made available by the authors, without undue reservation.
Author Contributions
SLS conceived the presented idea with contributions from AF and SGS. SLS, AF, and KG planned and conducted the field work, and YJ provided support. SLS lead the data analysis under the supervision of AF with additional feedback from KG and AC. SLS took the lead in writing the manuscript. All authors provided critical feedback and helped shape the research, analysis, and manuscript.
Funding
This study was made possible by funding from the California Department of Fish and Wildlife through California State Assembly Bill 707 (2017), the UC Davis Tahoe Environmental Research Center, and the State Water Resources Control Board. Samantha Sharp is funded through a NASA OSE graduate student fellowship awarded in 2020 (Grant Number 80NSSC20K1458).
Conflict of Interest
The authors declare that the research was conducted in the absence of any commercial or financial relationships that could be construed as a potential conflict of interest.
Acknowledgments
The authors would like to acknowledge contribution to this research by Ben Daniels, Nick Framsted, Matthew Krause, Doug Kubota, Drew Stang, Micah Swann, and Andy Wong and for data collection, Erica Kono and Lindsay Vaughan for sampling preparation and sample filtering, Brandon Berry and Andy Wong for AUV and sUAS mission planning and data processing, Randy Turner for technical assistance, and to Sarah Ryan and the Big Valley Band of Pomo Indians for sharing their cyanobacteria data results collected during our sampling events. The authors acknowledge the Clear Lake Blue Ribbon Committee for their leadership role in the efforts to restore Clear Lake. The authors acknowledge the two reviewers for their thorough and thoughtful review.
Supplementary Material
The Supplementary Material for this article can be found online at: https://www.frontiersin.org/articles/10.3389/fenvs.2021.612934/full#supplementary-material.
References
Arar, E. J., and Collins, G. B. (1997). Method 445.0: in vitro determination of chlorophyll a and pheophytin a in marine and freshwater algae by fluorescence (Ohio, United States: United States Environmental Protection Agency, Office of Research and Development, National Exposure Research Laboratory).
Austin, J. A. (2019). Observations of radiatively driven convection in a deep lake. Limnol. Oceanogr. 64 (5), 2152–2160. doi:10.1002/lno.11175
Avouris, D. M., and Ortiz, J. D. (2019). Validation of 2015 Lake Erie MODIS image spectral decomposition using visible derivative spectroscopy and field campaign data. J. Great Lakes Res. 45 (3), 466–479. doi:10.1016/j.jglr.2019.02.005
Blackwell, S. M., Moline, M. A., Schaffner, A., Garrison, T., and Chang, G. (2008). Sub-kilometer length scales in coastal waters. Continental Shelf Res. 28 (2), 215–226. doi:10.1016/j.csr.2007.07.009
Bryant, D. A. (1982). Phycoerythrocyanin and phycoerythrin: properties and occurrence in cyanobacteria. Microbiology 128 (4), 835–844. doi:10.1099/00221287-128-4-835
Carberry, L., Roesler, C., and Drapeau, S. (2019). Correcting in situ chlorophyll fluorescence time‐series observations for nonphotochemical quenching and tidal variability reveals nonconservative phytoplankton variability in coastal waters. Limnol. Oceanogr. Methods 17 (8), 462–473. doi:10.1002/lom3.10325
Carey, C. C., Weathers, K. C., Ewing, H. A., Greer, M. L., and Cottingham, K. L. (2014). Spatial and temporal variability in recruitment of the cyanobacterium Gloeotrichia echinulatain an oligotrophic lake. Freshw. Sci. 33 (2), 577–592. doi:10.1086/675734
Cheung, M. Y., Liang, S., and Lee, J. (2013). Toxin-producing cyanobacteria in freshwater: a review of the problems, impact on drinking water safety, and efforts for protecting public health. J. Microbiol. 51 (1), 1–10. doi:10.1007/s12275-013-2549-3
Clark, J. M., Schaeffer, B. A., Darling, J. A., Urquhart, E. A., Johnston, J. M., Ignatius, A., et al. (2017). Satellite monitoring of cyanobacterial harmful algal bloom frequency in recreational waters and drinking source waters. Ecol. Indic. 80, 84–95. doi:10.1016/j.ecolind.2017.04.046
Coffer, M. M., Schaeffer, B. A., Darling, J. A., Urquhart, E. A., and Salls, W. B. (2020). Quantifying national and regional cyanobacterial occurrence in US lakes using satellite remote sensing. Ecol. Indic. 111, 105976. doi:10.1016/j.ecolind.2019.105976
Cortés, A., and Schladow, S. G. (2020). Lake temperature, dissolved oxygen and meteorological data in Clear Lake, CA, USA (2019–2020). Knowledge Network for Biocomplexity. doi:10.5063/F1C827P7
Diggle, P. J., and Ribeiro, P. J. (2007). Model-based geostatistics. New York, NY, United States: Springer.
Forrest, A. L., Laval, B. E., Pieters, R., and Lim, D. S. S. (2008). Convectively driven transport in temperate lakes. Limnol. Oceanogr. 53 (5part2), 2321–2332. doi:10.4319/lo.2008.53.5_part_2.2321
Fraschetti, S., Terlizzi, A., Bevilacqua, S., and Boero, F. (2006). The distribution of hydroids (Cnidaria, Hydrozoa) from micro- to macro-scale: spatial patterns on habitat-forming algae. J. Exp. Mar. Biol. Ecol. 339 (2), 148–158. doi:10.1016/j.jembe.2006.07.007
Gholizadeh, M. H., Melesse, A. M., and Reddi, L. (2016). A comprehensive review on water quality parameters estimation using remote sensing techniques. Sensors (Basel) 16 (8), 1298. doi:10.3390/s16081298
Ha, N. T. T., Thao, N. T. P., Koike, K., and Nhuan, M. T. (2017). Selecting the best band ratio to estimate chlorophyll-a concentration in a tropical freshwater lake using sentinel 2A images from a case study of Lake Ba Be (Northern Vietnam). Ijgi 6 (9), 290. doi:10.3390/ijgi6090290
Havens, K. (2008). Cyanobacteria blooms: effects on aquatic ecosystems, Adv. Exp. Med. Biol. 619, 733–747. doi:10.1007/978-0-387-75865-7_33
Ho, J. C., Michalak, A. M., and Pahlevan, N. (2019). Widespread global increase in intense lake phytoplankton blooms since the 1980s. Nature 574 (7780), 667–670. doi:10.1038/s41586-019-1648-7
Ho, J. C., and Michalak, A. M. (2015). Challenges in tracking harmful algal blooms: a synthesis of evidence from Lake Erie. J. Great Lakes Res. 41 (2), 317–325. doi:10.1016/j.jglr.2015.01.001
Horne, A. J. (1975). The ecology of Clear Lake phytoplankton. Clear Lake Algal Research Unit, 1–116.
Huisman, J., Codd, G. A., Paerl, H. W., Ibelings, B. W., Verspagen, J. M. H., and Visser, P. M. (2018). Cyanobacterial blooms. Nat. Rev. Microbiol. 16 (8), 471–483. doi:10.1038/s41579-018-0040-1
Humbert, J. F., and Törökné, A. (2017). “New tools for the monitoring of cyanobacteria in freshwater ecosystems,” in Handbook of Cyanobacterial Monitoring and Cyanotoxin Analysis. Editors J. Meriluoto, L. Spoof, and G. A. Codd (Chichester, United Kingdom: John Wiley and Sons), 84–88. doi:10.1002/9781119068761.ch8
Hunter, P. D., Matthews, M. W., Kutser, T., and Tyler, A. N. (2017). “Remote sensing of cyanobacterial blooms in inland, coastal, and ocean waters,” in Handbook of Cyanobacterial Monitoring and Cyanotoxin Analysis. Editors J. Meriluoto, L. Spoof, and G. A. Codd (Chichester, United Kingdom: John Wiley and Sons), 89–99. doi:10.1002/9781119068761.ch9
Israel, G. D. (1992). Determining sample size. IFAS extension, PEOD-6. Gainesville, FL, United States: University of Florida.
Kasinak, J.-M. E., Holt, B. M., Chislock, M. F., and Wilson, A. E. (2015). Benchtop fluorometry of phycocyanin as a rapid approach for estimating cyanobacterial biovolume. J. Plankton Res. 37 (1), 248–257. doi:10.1093/plankt/fbu096
Kim, W., Jung, S., Moon, Y., and Mangum, S. C. (2020). Morphological band registration of multispectral cameras for water quality analysis with unmanned aerial vehicle. Remote Sensing 12 (12), 2024. doi:10.3390/rs12122024
Kislik, C., Dronova, I., and Kelly, M. (2018). UAVs in support of algal bloom research: a review of current applications and future opportunities. Drones 2 (4), 35. doi:10.3390/drones2040035
Konopko, E. A. (2007). Development of a flow-through fluorometric system for the detection of phycocyanin in the lower Great Lakes. Syracuse, NY, United Staes: State University of New York College of Environmental Science and Forestry.
Kudela, R. M., Palacios, S. L., Austerberry, D. C., Accorsi, E. K., Guild, L. S., and Torres-Perez, J. (2015). Application of hyperspectral remote sensing to cyanobacterial blooms in inland waters. Remote Sensing Environ. 167, 196–205. doi:10.1016/j.rse.2015.01.025
Kutser, T. (2009). Passive optical remote sensing of cyanobacteria and other intense phytoplankton blooms in coastal and inland waters. Int. J. Remote Sensing 30 (17), 4401–4425. doi:10.1080/01431160802562305
Liu, H., Dahlgren, R., Larsen, R., Devine, S., Roche, L., O’ Geen, A., et al. (2019a). Estimating rangeland forage production using remote sensing data from a small unmanned aerial system (sUAS) and PlanetScope satellite. Remote Sensing 11 (5), 595. doi:10.3390/rs11050595
Liu, H., Zheng, Z. C., Young, B., and Harris, T. D. (2019b). Three-dimensional numerical modeling of the cyanobacterium Microcystis transport and its population dynamics in a large freshwater reservoir. Ecol. Model. 398, 20–34. doi:10.1016/j.ecolmodel.2019.01.022
Lunetta, R. S., Schaeffer, B. A., Stumpf, R. P., Keith, D., Jacobs, S. A., and Murphy, M. S. (2015). Evaluation of cyanobacteria cell count detection derived from MERIS imagery across the eastern United States. Remote Sensing Environ. 157, 24–34. doi:10.1016/j.rse.2014.06.008
Matthews, M. W. (2011). A current review of empirical procedures of remote sensing in inland and near-coastal transitional waters. Int. J. Remote Sensing 32 (21), 6855–6899. doi:10.1080/01431161.2010.512947
Matthews, M. W., Bernard, S., and Robertson, L. (2012). An algorithm for detecting trophic status (chlorophyll-a), cyanobacterial-dominance, surface scums and floating vegetation in inland and coastal waters. Remote Sensing Environ. 124, 637–652. doi:10.1016/j.rse.2012.05.032
Mobley, C. D. (1999). Estimation of the remote-sensing reflectance from above-surface measurements. Appl. Opt. 38 (36), 7442–7455. doi:10.1364/AO.38.007442
Moses, W. J., Ackleson, S. G., Hair, J. W., Hostetler, C. A., and Miller, W. D. (2016). Spatial scales of optical variability in the coastal ocean: implications for remote sensing and in situ sampling. J. Geophys. Res. Oceans 121 (6), 4194–4208. doi:10.1002/2016JC011767
Müller, P., Li, X. P., and Niyogi, K. K. (2001). Non-photochemical quenching. A response to excess light energy. Plant Physiol. 125 (4), 1558–1566. doi:10.1104/pp.125.4.1558
Oliver, R. L., Hamilton, D. P., Brookes, J. D., and Ganf, G. G. (2012). “Physiology, blooms and prediction of planktonic cyanobacteria,” in Ecology of cyanobacteria II. Editor B. A. Whitton (Dordrecht, Netherlands: Springer), 155–194. doi:10.1007/978-94-007-3855-3_6
Ortiz, J. D., Avouris, D. M., Schiller, S. J., Luvall, J. C., Lekki, J. D., Tokars, R. P., et al. (2019). Evaluating visible derivative spectroscopy by varimax-rotated, principal component analysis of aerial hyperspectral images from the western basin of Lake Erie. J. Great Lakes Res. 45 (3), 522–535. doi:10.1016/j.jglr.2019.03.005
Paerl, H. W., Gardner, W. S., Havens, K. E., Joyner, A. R., McCarthy, M. J., Newell, S. E., et al. (2016). Mitigating cyanobacterial harmful algal blooms in aquatic ecosystems impacted by climate change and anthropogenic nutrients. Harmful Algae 54, 213–222. doi:10.1016/j.hal.2015.09.009
Paerl, H. W., Hall, N. S., and Calandrino, E. S. (2011). Controlling harmful cyanobacterial blooms in a world experiencing anthropogenic and climatic-induced change. Sci. Total Environ. 409 (10), 1739–1745. doi:10.1016/j.scitotenv.2011.02.001
Paerl, H. W., and Huisman, J. (2009). Climate change: a catalyst for global expansion of harmful cyanobacterial blooms. Environ. Microbiol. Rep. 1, 27–37. doi:10.1111/j.1758-2229.2008.00004.x
Paerl, H. W., and Huisman, J. (2008). Climate. Blooms like it hot. Science 320, 57–58. doi:10.1126/science.1155398
Richerson, P. J., Suchanek, T. H., and Why, S. J. (1994). The causes and control of algal blooms in Clear Lake. Final report, 1–182.
Robbins, I. C., Kirkpatrick, G. J., Blackwell, S. M., Hillier, J., Knight, C. A., and Moline, M. A. (2006). Improved monitoring of HABs using autonomous underwater vehicles (AUV). Harmful Algae 5 (6), 749–761. doi:10.1016/j.hal.2006.03.005
Rueda, F. J., and Schladow, S. G. (2003). Dynamics of large polymictic lake. II: numerical simulations. J. Hydraul. Eng. 129 (2), 92–101. doi:10.1061/(ASCE)0733-942910.1061/(asce)0733-9429(2003)129:2(92)
Ruiz-Verdú, A., Simis, S. G. H., de Hoyos, C., Gons, H. J., and Peña-Martínez, R. (2008). An evaluation of algorithms for the remote sensing of cyanobacterial biomass. Remote Sensing Environ. 112 (11), 3996–4008. doi:10.1016/j.rse.2007.11.019
Siegelman, H. W., and Kycia, H. H. (1978). “Algal biliproteins,” in Handbook of phycological methods: Physiological and biochemical methods. Editors J. A. Hellebust, and J. S. Craigie (Cambridge, United Kingdom: Cambridge University Press), 71–80.
Stroming, S., Robertson, M., Mabee, B., Kuwayama, Y., and Schaeffer, B. (2020). Quantifying the human health benefits of using satellite information to detect cyanobacterial harmful algal blooms and manage recreational advisories in United States lakes. GeoHealth 4, e2020GH000254. doi:10.1029/2020GH000254
Stumpf, R. P., Davis, T. W., Wynne, T. T., Graham, J. L., Loftin, K. A., Johengen, T. H., et al. (2016). Challenges for mapping cyanotoxin patterns from remote sensing of cyanobacteria. Harmful Algae 54, 160–173. doi:10.1016/j.hal.2016.01.005
Stumpf, R. P., Tomlinson, M. C., Meredith, A., Briggs, T., and Wynne, T. T. (2015). Application of MERIS satellite data to cyanobacterial blooms in California. Report to the San Francisco Estuary Institute from the National Oceanic and Atmospheric Administration National Centers for Coastal Ocean Science.
Taranu, Z. E., Gregory-Eaves, I., Leavitt, P. R., Bunting, L., Buchaca, T., Catalan, J., et al. (2015). Acceleration of cyanobacterial dominance in north temperate-subarctic lakes during the Anthropocene. Ecol. Lett. 18 (4), 375–384. doi:10.1111/ele.12420
Tomlinson, M. C., Stumpf, R. P., Wynne, T. T., Dupuy, D., Burks, R., Hendrickson, J., et al. (2016). Relating chlorophyll from cyanobacteria-dominated inland waters to a MERIS bloom index. Remote Sensing Lett. 7 (2), 141–149. doi:10.1080/2150704X.2015.1117155
UC Davis Tahoe Environmental Research Center (2020). Data Archive TERC Clear Lake Research. Available at: https://terc-clearlake.wixsite.com/cldashboard/dataarchive
Urquhart, E. A., Schaeffer, B. A., Stumpf, R. P., Loftin, K. A., and Werdell, P. J. (2017). A method for examining temporal changes in cyanobacterial harmful algal bloom spatial extent using satellite remote sensing. Harmful Algae 67, 144–152. doi:10.1016/j.hal.2017.06.001
Vander Woude, A., Ruberg, S., Johengen, T., Miller, R., and Stuart, D. (2019). Spatial and temporal scales of variability of cyanobacteria harmful algal blooms from NOAA GLERL airborne hyperspectral imagery. J. Great Lakes Res. 45 (3), 536–546. doi:10.1016/j.jglr.2019.02.006
Visser, P. M., Ibelings, B. W., Bormans, M., and Huisman, J. (2016). Artificial mixing to control cyanobacterial blooms: a review. Aquat. Ecol. 50 (3), 423–441. doi:10.1007/s10452-015-9537-0
Winder, M., Reuter, J., and Schladow, G. (2010). Clear Lake historical data analysis. Clear Lake final report for Lake County. Davis, CA, United States: University of California, 1–51.
Wrigley, R. C., and Horne, A. J. (1974). Remote sensing and lake eutrophication. Nature 250 (5463), 213–214. doi:10.1038/250213a0
Wynne, T. T., Meredith, A., Briggs, T., Litaker, W., and Stumpf, R. P. (2018). Harmful algal bloom forecasting branch ocean color satellite imagery processing guidelines. NOAA Tech. Memorandum NOS NCCOS 252, 48. doi:10.25923/twc0-f025
Wynne, T. T., Stumpf, R. P., Tomlinson, M. C., and Dyble, J. (2010). Characterizing a cyanobacterial bloom in western Lake Erie using satellite imagery and meteorological data. Limnol. Oceanogr. 55 (5), 2025–2036. doi:10.4319/lo.2010.55.5.2025
Wynne, T. T., Stumpf, R. P., Tomlinson, M. C., Warner, R. A., Tester, P. A., Dyble, J., et al. (2008). Relating spectral shape to cyanobacterial blooms in the Laurentian Great Lakes. Int. J. Remote Sensing 29 (12), 3665–3672. doi:10.1080/01431160802007640
Xu, M., Liu, H., Beck, R., Lekki, J., Yang, B., Shu, S., et al. (2019). Regionally and locally adaptive models for retrieving chlorophyll-a concentration in inland waters from remotely sensed multispectral and hyperspectral imagery. IEEE Trans. Geosci. Remote Sensing 57 (7), 4758–4774. doi:10.1109/TGRS.2019.2892899
Keywords: cyanobacteria, harmful algal blooms (HABs), remote sensing, Clear Lake, critical scales of variability (CSV), autonomous underwater vehicles (AUV), small unmanned aerial systems (sUAS), inland waters
Citation: Sharp SL, Forrest AL, Bouma-Gregson K, Jin Y, Cortés A and Schladow SG (2021) Quantifying Scales of Spatial Variability of Cyanobacteria in a Large, Eutrophic Lake Using Multiplatform Remote Sensing Tools. Front. Environ. Sci. 9:612934. doi: 10.3389/fenvs.2021.612934
Received: 01 October 2020; Accepted: 02 February 2021;
Published: 15 April 2021.
Edited by:
Sherry L. Palacios, California State University, Monterey Bay, United StatesReviewed by:
Mark Harrison Myer, United States Environmental Protection Agency (EPA), United StatesJoseph Daniel Ortiz, Kent State University, United States
Copyright © 2021 Sharp, Forrest, Bouma-Gregson, Jin, Cortés and Schladow. This is an open-access article distributed under the terms of the Creative Commons Attribution License (CC BY). The use, distribution or reproduction in other forums is permitted, provided the original author(s) and the copyright owner(s) are credited and that the original publication in this journal is cited, in accordance with accepted academic practice. No use, distribution or reproduction is permitted which does not comply with these terms.
*Correspondence: Samantha L. Sharp, ssharp@ucdavis.edu