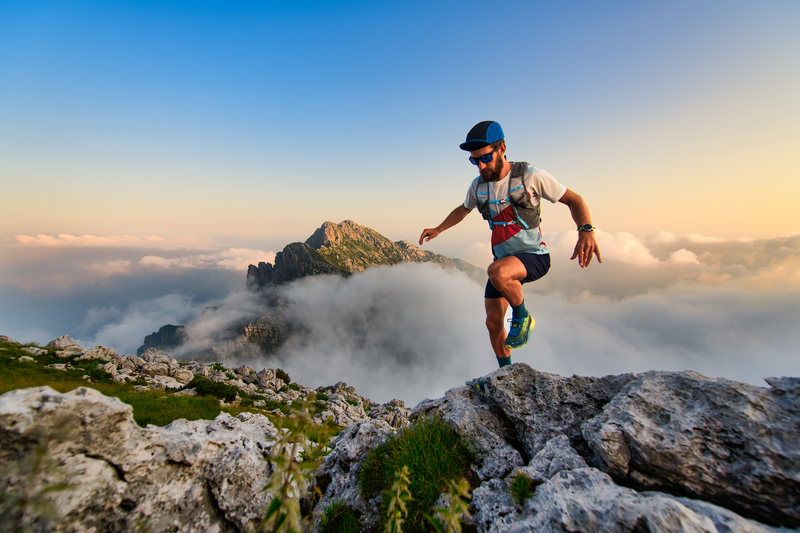
94% of researchers rate our articles as excellent or good
Learn more about the work of our research integrity team to safeguard the quality of each article we publish.
Find out more
ORIGINAL RESEARCH article
Front. Environ. Sci. , 04 March 2021
Sec. Conservation and Restoration Ecology
Volume 9 - 2021 | https://doi.org/10.3389/fenvs.2021.597971
This article is part of the Research Topic Grassland Conservation in Asia: Sustainability Under Climate Change View all 28 articles
Large-scale climate oscillations, particularly the Atlantic Multidecadal Oscillation (AMO) and the Pacific Decadal Oscillation (PDO), have widespread influences on climate systems across the Tibetan Plateau (TP). It is understudied how the temporal changes in AMO and PDO affected growth of vegetation through modifying the local climatic factors in different areas across the TP. We used the AMO and PDO indices, gridded growing season mean temperature (TGS), cumulative precipitation (PGS), and normalized difference vegetation index (NDVIGS) data from 1982 to 2015 to investigate the temporal trends of these variables and the correlations of the TGS and PGS with each of the AMO and PDO indices as well as their correlations with the NDVIGS. The results showed that the warming of the TGS over the TP and the increases of the PGS in western, central, and northeastern areas of the TP may have been related to an increase of the AMO index and a decrease of the PDO index. Combining those relationships with the spatial patterns of the TGS-NDVIGS and PGS-NDVIGS correlations suggested that the changes of the AMO and PDO may have indirectly increased the NDVIGS in the central and northeastern areas of the TP by increasing TGS and PGS, in most parts of the southwestern TP by increasing PGS, and in the eastern and south-central regions of the TP by increasing TGS. In contrast, the decrease of the NDVIGS in some areas of the southeastern and southwestern TP may have been associated with a negative effect of warming as a result of changes in the AMO and PDO. These results highlight the indirect impacts of changes in large-scale climate oscillations on the growth of vegetation through modification of local climatic factors across the TP, and they suggest the substantial spatial heterogeneity of these impacts largely depends on the responses of vegetation to local climatic factors.
The Tibetan Plateau (TP), known as the roof of the world, is the largest and highest highland in the world, with an average elevation of over 4,000 m and an area of about 2.5 × 106 km2 (Tang et al., 2009). The TP has experienced environmental degradation aggravated by rapid climate changes (Kang et al., 2010; Duan and Xiao, 2015). The Atlantic Multidecadal Oscillation (AMO), a major source of temperature variability in the Northern Hemisphere (Li et al., 2013; Delworth et al., 2016) has been shown to modulate TP summer temperatures at interannual to multidecadal time scales (R2 as high as 0.36–0.50, P < 0.001) (Shi et al., 2019). Most precipitation that falls on the vegetated portion of the TP during the growing season is attributable to the South Asian Summer Monsoon (SASM) (Conroy and Overpeck, 2011), while a recent study claimed 63% water vapor of TP precipitation is provided by local moisture recycling (Curio et al., 2015). Both the AMO and SASM are controlled by sea surface temperature (SST) over the North Pacific region [e.g., the Pacific Decadal Oscillation (PDO)] through alternating SST of the tropical Indian Ocean and hence by the thermal contrast between the TP and tropical Indian Ocean (Li and Yanai, 1996; Krishnamurthy and Krishnamurthy, 2014). Other large-scale climate variabilities, such as the ENSO (El Niño Southern Oscillation) signal, are detected in the SASM as well, but the impacts are highly variable (Kumar et al., 1999) and dependent on the phases of the PDO (Yoon and Yeh, 2010; Feng et al., 2014), and the Indian Ocean Dipole Mode (Ashok et al., 2001). The changes of the AMO and PDO may therefore have major impacts on climate over areas of the TP where the growth of vegetation is sensitive to climate change.
Mean annual temperature ranges from −15 to +10°C from the high to low elevations, and cumulative annual precipitation ranges from less than 100 mm to more than 1,000 mm from the northwestern to southeastern TP (You et al., 2013; Maussion et al., 2014), whereas the potential evapotranspiration, which ranges from about 700 mm year−1 to about 1,500 mm year−1 (Zhang et al., 2007), is substantially higher than precipitation in most areas of the TP. The vegetation types from the southeast to northwest are mainly forest, alpine meadow, alpine steppe, and alpine desert; alpine shrubland and alpine cushion vegetation are found in some areas (Geng et al., 2012). The growth of vegetation in the TP is known to be sensitive to climate change (Shen et al., 2015a; Shen et al., 2015b; Gao et al., 2016; Shen et al., 2016; Dorji et al., 2018; Hopping et al., 2018; Wang et al., 2020). The responses of the growth of TP vegetation to climate changes have major impacts on carbon cycles and terrestrial surface water, regulating climate both within and beyond the TP (Zeng et al., 2008; Shang et al., 2013; Shen et al., 2015c; Zhao et al., 2016; Ma et al., 2018; Zhao et al., 2019; Fu et al., 2020). Moreover, the changes in the growth of vegetation can also influence ecosystem services in the TP (Klein et al., 2008; Tang et al., 2015; Huang et al., 2016b; Hopping et al., 2018; Kan et al., 2018). Determining how the growth of vegetation responds to climate changes should therefore enhance our understanding of how ecosystems within the TP respond to climate change and allow for a more realistic representation of relevant processes in land surface models, which are essential for the simulation and management of ecosystems within the TP.
The TP has experienced significant climatic warming over the past five decades (Lu and Liu, 2010; Cai et al., 2017; Zhong et al., 2019), and the warming has accelerated since the 1980s (Lu and Liu, 2010; Zhong et al., 2019). The mean annual temperature has increased faster in the northeastern and northwestern areas than in the southeastern areas of the TP over the past five decades (Li et al., 2010; Deng et al., 2017). Annual precipitation has increased substantially in the western, central, and northeastern areas but has decreased slightly along the southeastern and southwestern edges of the TP over the past 40 years (Zhang et al., 2017). Climatic change has resulted in substantial changes in the growth of vegetation across the TP (Piao et al., 2012; Lehnert et al., 2016; Zhu et al., 2016; Zhang et al., 2018; Zhong et al., 2019). For instance, climate warming not only advanced vegetation green-up date but also extended the length of the growing season across the TP (Liu et al., 2006; Piao et al., 2011; Dong et al., 2012) Shen et al. (2016) have reported that nighttime warming advanced vegetation green-up date in most area across the TP, likely nighttime warming reduced low temperature constraints. Meanwhile, increasing temperatures are the main factors for the increase in net primary productivity of vegetation on the TP (Gao et al., 2013). However, climate warming has a negative impact on the net primary productivity in arid regions, mainly due to increasing temperatures leading to decline in water availability (Fu et al., 2013). However, the manner by which the growth of vegetation has responded to climatic factors shows considerable spatial heterogeneity (Piao et al., 2012; Huang et al., 2016a; Hua and Wang, 2018; Li et al., 2018; Zhong et al., 2019). Increasing temperatures are recognized to have enhanced vegetation growth in central and southeastern areas of the TP, but they have depressed vegetation growth in southwestern and northeastern areas, likely by causing drought stress (Du et al., 2015; Hua and Wang, 2018; Li et al., 2018). Moreover, when we focused on the areas where higher temperatures enhanced the growth of vegetation, we found that the growth of vegetation was more sensitive to temperature in alpine meadow than in alpine steppe (Zhang et al., 2014). This is mainly because the alpine meadow is distributed in the eastern and southern TP with more precipitation. Therefore, increasing temperatures are the main factor to enhance the growth of vegetation (Zheng et al., 2020). The effects of temporal changes in precipitation on the growth of vegetation have also varied spatially. An increase of precipitation had positive impacts on the growth of vegetation in the northeast and southwest of the TP (Du et al., 2015; Li et al., 2018; Li et al., 2020) but negative impacts in the southeast of the TP (Du et al., 2015; Hua and Wang, 2018). In addition, in both alpine steppe and alpine meadow, higher precipitation had positive impacts on the growth of vegetation, but the vegetation growth of alpine steppe was more sensitive to precipitation than that of alpine meadow. This is mainly because the alpine steppe is distributed in arid regions of the TP, where precipitation plays a primarily regulating role in the growth of vegetation (Zheng et al., 2020). Overall, there was high spatial heterogeneity in terms of the correlation between the growth of vegetation and local climatic factors as well as in the sensitivities of the growth of vegetation to temperature and precipitation.
However, a few studies have assessed the direct correlation between the growth of vegetation in the TP and large-scale climate oscillations (Shi et al., 2018; Yu et al., 2018; Cheng et al., 2019). For example, Cheng et al. (2019) have calculated the correlation coefficient between four ENSO indices and tree growth in the southern TP and have reported that tree growth has been sensitive to the ENSO since the 1970s. On the basis of a correlation coefficient between the spring Arctic Oscillation index and the start of the vegetation growing season, Yu et al. (2018) have reported that the changes in the start of the vegetation growing season in the eastern area of the Three-River Source Region were influenced by the Arctic Oscillation from 2000 to 2013. Shi et al. (2018) have calculated the correlation coefficients between the growth of vegetation in the TP and the PDO or the North Atlantic Oscillation. Their results show considerable spatial variations in both the sign and magnitude of those correlations. The oversimplified correlations between large-scale climate oscillations and vegetation growth have ignored the high spatial heterogeneity of the vegetation growth response to local climatic conditions and the complex teleconnections between local climatic conditions and large-scale climate oscillations. Numerous studies have shown that the PDO and AMO are key factors that drive the thermal and hydrological conditions over the TP (Gou et al., 2014; Li and Li, 2017; Shi et al., 2017; Shi et al., 2019). We believe exploring the effects of PDO and AMO on vegetation growth required detailed inspections of the teleconnections of local and large scale climatic factors, and the response pattern of vegetation growth to local climate.
In this study, we investigated how temporal changes in the AMO and PDO impact the growth of vegetation through modifying local climatic conditions in different areas across the TP. We first determined the correlation coefficients between either the AMO or PDO index and one or the other of the growing season mean temperature (TGS) or cumulative precipitation (PGS). We also calculated the correlation coefficients between the growing season–normalized difference vegetation index (NDVIGS) and either the TGS or PGS across the TP during the period 1982–2015. We then examined the temporal trends of those variables (AMO index, PDO index, TGS, PGS, and NDVIGS). Finally, we explained how the interaction between temporal variations of the large-scale climate oscillations and local climatic factors led to variations of the growth of vegetation across the TP.
The AMO index was defined as the average of monthly SST over the extra-tropical North Atlantic (25–60°N, 7–75°W) minus the linear trend of global mean temperature (van Oldenborgh et al., 2009). This approach can remove the influence of ENSO at tropical latitudes and reduce the influence of global warming on the average SST. The PDO index, which was first developed by Mantua et al. (1997), is defined as the leading principal component of monthly SST anomalies in the North Pacific Ocean (poleward of 20°N). These data were obtained from the Koninklijk Nederlands Meteorologisch Instituut climate explorer at https://climexp.knmi.nl/. Seasonally averaged AMO and PDO indices from January to August and from April to July (Shi et al., 2010; Shi et al., 2019), respectively, for every year from 1982 to 2015 were used to study the impacts of large-scale climate oscillations on local climatic factors.
Gridded monthly mean temperature and cumulative precipitation data over the period 1982–2015 were taken from the Climatic Research Unit, University of East Anglia (http://data.ceda.ac.uk, accessed on 11 June 2019). The Climatic Research Unit Time-Series version 4.03 (CRU TS4.03) has a spatial resolution of 0.5° by 0.5° (Harris et al., 2014). For each pixel, TGS and PGS were calculated as the mean temperature and cumulative precipitation, respectively, during the growing season (May–September) (Shen et al., 2015c) for every year.
Growth of vegetation across the TP over the period 1982–2015 was quantified by the NDVI. Calculation of the NDVI makes use of the spectral signature of chlorophyll absorption and mesophyll scattering on reflected radiation (Tucker, 1979; Shen et al., 2008) and has been widely used as a surrogate of the growth and productivity of vegetation at regional and global spatial scales (Beck and Goetz, 2011; Gonsamo et al., 2016; Huang et al., 2016a; Pan et al., 2018). We used the third-generation NDVI derived from the Advanced Very High Resolution Radiometer (AVHRR) produced by the Global Inventory Modeling and Mapping Studies (GIMMS) group at a spatial resolution of 1/12° and a temporal resolution of 15 days (https://ecocast.arc.nasa.gov/data/pub/gimms/3g.v1/, accessed in November 2017). Multiple measures were taken to minimize the errors caused by the update of sensors, atmospheric effects, orbital drift, and sensor attenuation (Pinzon and Tucker, 2014). To match the gridded temperature and precipitation data, the NDVI data were aggregated to 0.5° by averaging the gridded NDVI values. Pixels with mean annual NDVIs less than 0.10 were considered to contain sparse vegetation and were excluded in this study (Cong et al., 2017). The NDVIGS was then calculated as the mean of the maximum NDVI for each month from May to September (An et al., 2018).
To assess the impacts of temporal changes of the climate oscillations on local climatic factors, we calculated the Pearson correlation coefficient between the time series (1982 to 2015) of each of the AMO and PDO indices and the time series of each of the TGS and PGS for each pixel. Then, to assess the impact of TGS or PGS on NDVIGS, we also calculated the Pearson correlation coefficient between the NDVIGS and TGS or PGS, respectively. Finally, the temporal trends of these variables (AMO index, PDO index, TGS, PGS, and NDVIGS) over the period 1982–2015 were assessed on the basis of the slopes of the ordinary least-squares regression lines relating year (independent variable) to the variable (dependent variable). All statistical significance levels reported in this study were determined on the basis of two-tailed Student’s t-tests.
The correlation coefficients between each of the large-scale climate oscillation indices and the annual mean TGS or PGS for each pixel showed that both the TGS and PGS were influenced by the AMO and PDO indices across the TP (Figure 1). Regionally averaged TGS values were significantly related to AMO indices (R = 0.57, P < 0.01). Almost throughout the vegetated portion of the TP (98.7% of the pixels), AMO indices and TGS values were significantly (P < 0.05; hereafter, “significant” means P < 0.05 unless otherwise stated), positively correlated (Figure 1A). The correlation coefficient between regionally averaged PGS values and AMO indices was also significant and positive (R = 0.41). In 93.4% of all pixels, PGS values were positively correlated with AMO indices, and the positive correlations were significant in 24.4% of the pixels, most of which were distributed in the northeastern and central portion of the TP and in the region between 84 and 90°E (Figure 1B). Negative correlations between PGS values and AMO indices were found only at the eastern and southwestern edges of the TP, and none were significant.
FIGURE 1. Spatial pattern of correlation coefficients (R) between each of the AMO and PDO indices and each of the annual TGS and PGS metrics across the TP over the period 1982–2015. R = ±0.44, R = ±0.34, R = ±0.29, and R = ±0.23 correspond to significance levels P = 0.01, P = 0.05, P = 0.10, and P = 0.20, respectively. Pixels with dots were statistically significant (P < 0.05). Bottom-left inset in each map shows the percentage of pixels in each interval of correlation coefficient with the interval value indicated by the color in the legend in the right. The pixels with mean annual NDVI lower than 0.10 were excluded (see method for detail).
In contrast, regional averages of both TGS (R = −0.42, P < 0.05) and PGS (R = −0.49, P < 0.01) were negatively correlated with the PDO index. The TGS was negatively correlated with the PDO index in all the pixels. The correlations were significant in 55.2% of the pixels, mainly in the center-northeast and northwest areas of the TP (Figure 1C). The correlations between the PGS and the PDO were negative in 85.8% of the pixels. Those correlations were significant in 36.0% of the pixels, mainly in the center of the TP and in the region between 84 and 92°E (Figure 1D). The areas where there were positive correlations between the PGS and the PDO index were in the eastern and northwestern edges; all of those correlations were insignificant. In the remaining areas, most of the correlations were negative and insignificant.
When the data were averaged over regions, the interannual variations of NDVIGS were significantly related with TGS (R = 0.41) but not with PGS (R = 0.18, P > 0.05). For 73.0% of all pixels, the NDVIGS was positively correlated with TGS, and the correlations were significant for 23.7% of all pixels, most of which were distributed in the northeast, center, and center-east areas of the TP (Figure 2A). Negative correlations between NDVIGS and TGS occurred mainly in the southwestern quarter of the TP and were significant in only 2.7% of the pixels. The correlations between NDVIGS and PGS were positive in 71.6% of the pixels and were significant for 18.5% of the pixels, most of which were in the southwestern quarter and northeast TP (Figure 2B). The negative correlations between NDVIGS and PGS were associated with pixels located mostly in the eastern and center-south areas of the TP, and the negative correlations were significant in only 1.4% of the pixels. The partial correlation between NDVIGS and TGS (or PGS) controlling PGS (or TGS) produced similar results (Figure S1).
FIGURE 2. Spatial pattern of correlation coefficients between the NDVIGS and annual TGS(A) or PGS(B). R = 0.44, R = ±0.34, R = ±0.29, and R = ±0.23 correspond to significance levels P = 0.01, P = 0.05, P = 0.10, and P = 0.20, respectively. Pixels with dots were statistically significant (P < 0.05). Bottom-left inset in each map shows the percentage of pixels in each interval of correlation coefficient with the interval value indicated by the color in the legend in the right.
There was a significant (P < 0.01), positive temporal trend in the AMO index, whereas the PDO index decreased significantly over the period (Figure 3A). The regionally averaged TGS increased substantially at a rate of 0.22°C decade−1 (P < 0.01) over the period (Figure 3B). In most areas, the trends were 0.10–0.30°C decade−1 (Figure 4A). The temporal trend of the TGS in most (96.8%) of the pixels was significant and positive, except for a few pixels to the south of the center of the TP, where the trends were positive but insignificant. In contrast, the temporal trend of the regional average of the PGS was positive but not significant (P > 0.10) (Figure 3B). This lack of significance was associated with the spatial inconsistency of the PGS trends at the pixel level. The PGS decreased insignificantly by less than 10 mm decade−1 in the southeastern area and along the southwestern edge of the TP (Figure 4B). In the remaining areas, the temporal trends were mostly 0–20 mm decade−1, except for the center-south area, where the trends were mostly 20–40 mm decade−1. The trends of PGS were significantly positive in only 9.5% of the pixels, in the center-north and in the northeast of the TP.
FIGURE 3. Temporal trends of AMO and PDO indices (A); regionally averaged TGS and PGS(B); and NDVIGS(C).
FIGURE 4. Spatial pattern of temporal trends in TGS(A), PGS(B), and NDVIGS(C). Pixels with dots were statistically significant (P < 0.05). Bottom-left inset in each map shows the percentage of pixels in each trend interval. The trend interval values are indicated by the color scale to the right.
The temporal trend of the regional average of the NDVIGS was positive, 2.67 × 10–3 decade−1, but marginally significant (P < 0.10) (Figure 3C). The temporal trends of the NDVIGS were positive in 75.6% of all the pixels, which were widely distributed geographically, with the exception of the southeast area of the TP (Figure 4C). In most areas with positive trends, the trends were 0–10 × 10–3 decade−1; trends larger than 10 × 10–3 decade−1 were mostly in the northeast of the TP. The pixels where the trends of the NDVIGS were significant and positive accounted for 29.0% of all the pixels and were distributed mainly in the northeast and north of the center; some were scattered in areas south of the center and along the southwestern edge of the TP. NDVIGS decreased significantly in only 4 pixels.
We investigated the cross-correlations between the AMO/PDO and TGS/PGS as well as between the NDVIGS and TGS/PGS. We also investigated the temporal trends of these variables (AMO index, PDO index, TGS, PGS, and NDVIGS). The interpretation of those correlations and trends indicated that the increasing AMO index (the decreasing PDO index) indirectly enhanced the growth of vegetation in the northeast, central, and southwest marginal areas of the TP. Those areas accounted for 36.4% (32.3%) of the pixels associated with increasing trends of TGS and PGS (Figures 5A,B). In the east and south central areas of the TP, the increasing AMO index (decreasing PDO index) had indirect positive effects on the growth of vegetation in 24.6% (25.7%) of all pixels associated with increasing TGS. In most areas of the southwestern TP, the increasing AMO index (decreasing PDO index) could also have indirectly resulted in the increasing trend of the NDVIGS that occurred in 13.1% (13.1%) of the pixels associated with increasing PGS. In addition, for 12.0% (12.0%) of all pixels, the increasing AMO index (decreasing PDO index) had indirect negative effects on the growth of vegetation associated with increasing TGS, mainly in the areas southeast and southwest of the center of the TP. Using the partial correlation between NDVIGS and TGS (or PGS) gave similar results (Figure S2).
FIGURE 5. Different modes showing how the temporal changes of the AMO (A) and PDO (B) indices affected the NDVIGS by modifying the TGS or PGS. Bottom-left inset in each map shows the percentage of pixels in each mode. The modes are indicated by the color in the legend at the bottom of each map. The upward or downward arrow to the right of each variable indicates positive or negative trends of that variable. A positive or negative sign near the line between two variables indicates positive or negative correlations between them. If two variables connected by a line were positively (negatively) correlated, we considered only the case in which the trends of the two variables shared the same direction (opposite directions), and these pixels are colored in green and yellow on the map. Otherwise, the pixel was colored gray.
As has been indicated in previous studies, the growth of vegetation, including an extension of the growing season and increase of the NDVI and net primary production, has been substantially enhanced as a result of rapid warming and increased precipitation during the past 40 years (Liu et al., 2006; Chen et al., 2014; Zhang et al., 2018). Moreover, because the changes in large-scale climate oscillations have had a strong impact on local climatic conditions across the TP (Gou et al., 2014; Shi et al., 2017; Shi et al., 2019), some studies have asserted that these climate oscillations have affected the growth of vegetation based on the direct correlations between them (Shi et al., 2018; Yu et al., 2018). Our results differed from these conclusions. Instead, they suggested that the impacts of temporal changes of the AMO and PDO on the growth of vegetation were largely dependent on the responses of vegetation to local climatic factors that were affected by the large-scale climate oscillations.
Moreover, our results indicated that the impacts of the AMO and PDO on the growth of vegetation were spatially heterogeneous. The spatial pattern of such impacts depended mainly on the spatial characteristics of the responses of the growth of vegetation to local climate factors rather than on that of the impacts of large-scale climate oscillations on local climate factors. (Figure 5). Such pattern is substantially different from that for the direct correlation between AMO (PDO) index and NDVIGS (Figure 6). A number of studies have tried to assess the impacts of remote climate oscillations on the local growth of vegetation by using direct correlations between them on the TP (Shi et al., 2018) or elsewhere (Brown et al., 2010; Li et al., 2015; Zhu et al., 2017; Araghi et al., 2019). However, our study indicated that directly correlating the growth of vegetation with the AMO or PDO while ignoring the underlying mechanisms linking them could be misleading over the TP. Such correlations should be interpreted with caution.
FIGURE 6. Spatial pattern of correlation coefficients between the NDVIGS and annual AMO (A) or PDO (B) index. R = ±0.44, R = ±0.34, R = ±0.29, and R = ±0.23 correspond to significance levels P = 0.01, P = 0.05, P = 0.10, and P = 0.20, respectively. Pixels with dots were statistically significant (P < 0.05). Bottom-left inset in each map shows the percentage of pixels in each interval of correlation coefficient with the interval value indicated by the color in the legend in the right.
The oscillatory behavior between the warm and cold states of the AMO has largely modulated the large-scale climate variations in the northern hemisphere (Delworth et al., 2016). Our results suggest that the AMO variation might have been associated with changes of TGS throughout the TP and with the PGS in most areas of the TP during the study period. These results are consistent with the conclusions of several previous studies (Feng and Hu, 2008; Wang et al., 2013; Li and Li, 2017; Shi et al., 2017). A recent study has shown that half of the variability of TP summer temperatures can be explained by the AMO, and an atmospheric general circulation model simulation has suggested that a warm (cold) AMO leads to an anomalously high (low) surface pressure, a corresponding downward (upward) atmospheric motion over the TP, and hence high (low) TP summer temperatures (Shi et al., 2019). The in-phase teleconnection pattern of AMO and TP summer temperature reflects the fact that the warm phase of AMO can cause land surface heating over TP, which enhanced the thermal contrast between TP and surrounding tropical oceans, therefore increased summer monsoon intensity and concurrent precipitation (Feng and Hu, 2008; Wang et al., 2013; Li and Li, 2017). The indirect connection substantially explained the observed significant correlation between growing-season precipitation and AMO. While in the northern part of the TP where the precipitation was dominated by the westerlies, Huang et al. (2015) have found that the summer AMO index was significantly and positively correlated with summer precipitation (R = 0.77, P < 0.01) as well. The connection of Atlantic SST variability, westerlies intensity and precipitation of the none-monsoon region of central Asia was reported (Li et al., 2008; Yu et al., 2014; Zhang et al., 2016; Luo et al., 2018).
Shi et al. (2019) has reported that a warm (cold) AMO was associated with decreased (increased) summer precipitation, which is contradictory with our findings. However, the precipitation and AMO index data used by Shi et al. (2019) are completely different from ours in this study: the precipitation data of Shi et al. (2019) are model output instead of instrumental observation, and the AMO index was smoothed and normalized data.
Consistent with earlier findings over TP (Gou et al., 2013; Gou et al., 2014), the decreased PDO was apparently a key factor responsible for the PGS increase in most regions of the TP during the study period. PDO can regulate the intensity, persistence and onset time of the SASM and thus precipitation over the TP (Wu and Mao, 2019): A warm (cold) PDO induces a decreased (increased) SST over the tropical Indian Ocean (Yang et al., 2018), and therefore a reduced (enhanced) land-ocean thermal contrast and weakened (strengthened) SASM intensity (Dong and Xue, 2016), characterized by an earlier (delayed) onset of monsoon precipitation (Watanabe and Yamazaki, 2014). In contrast, the evidence for the impacts of the PDO on TGS is still limited.
We investigated the impacts of temporal changes of the AMO and PDO on local climate characteristics and subsequently on the growth of vegetation across the TP during the period 1982–2015. The increasing AMO (decreasing PDO) might have contributed to the increases of the TGS throughout the TP and of the PGS in most areas of the TP. The increase in the growth of vegetation in the northern areas of the TP was likely related to the increases of the TGS and PGS; in most areas of the southwestern TP to increases of the PGS; and in the south central areas and in some areas in the eastern TP to increasing TGS. The decrease in the growth of vegetation in some areas of the southeastern and southwestern TP was likely related to the increase of the TGS. Collectively, these results suggest that the increase of the AMO (decrease of the PDO) may have enhanced the growth of vegetation in the central and northeastern areas of the TP by increasing TGS and PGS, in most areas of the southwestern TP by increasing PGS, and in the east and south central regions by increasing the TGS. However, the increase of the AMO (decrease of the PDO) apparently had negative effects on the growth of vegetation in some areas of the southeastern and southwestern TP by increasing the TGS. The spatial pattern of the impacts of the AMO and PDO on the growth of vegetation was therefore dependent on the responses of growth of vegetation to local climatic factors. Our study also suggests that correlations between remote, large-scale climate oscillations and the local growth of vegetation should be interpreted with caution.
All the data used in this study are publicly available through the internet. The sources of the data are listed in the Materials and Methods section.
MS and CS designed the research; LZ, FS, and NJ analyzed data; all authors interpreted results and wrote the paper.
This work was funded by the 2nd Scientific Expedition to the Qinghai-Tibet Plateau (Grants No. 2019QZKK0307 and 2019QZKK0405), the National Natural Science Foundation of China (Grants No. 41861134038 and 41830649), the Key Research Program of Frontier Sciences (Grant No. QYZDB-SSW-DQC025), the Youth Innovation Promotion Association (to Shen) of the Chinese Academy of Sciences, and the Top-Notch Young Talents Program of China (to Shen).
The authors declare that the research was conducted in the absence of any commercial or financial relationships that could be construed as a potential conflict of interest.
The Supplementary Material for this article can be found online at: https://www.frontiersin.org/articles/10.3389/fenvs.2021.597971/full#supplementary-material.
An, S., Zhu, X., Shen, M., Wang, Y., Cao, R., Chen, X., et al. (2018). Mismatch in elevational shifts between satellite observed vegetation greenness and temperature isolines during 2000-2016 on the Tibetan Plateau. Glob. Change Biol. 24, 5411–5425. doi:10.1111/gcb.14432
Araghi, A., Martinez, C. J., Adamowski, J., and Olesen, J. E. (2019). Associations between large-scale climate oscillations and land surface phenology in Iran. Agric. For. Meteorol. 278, 107682. doi:10.1016/j.agrformet.2019.107682
Ashok, K., Guan, Z., and Yamagata, T. (2001). Impact of the Indian Ocean dipole on the relationship between the Indian monsoon rainfall and ENSO. Geophys. Res. Lett. 28, 4499–4502. doi:10.1029/2001gl013294
Beck, P. S. A., and Goetz, S. J. (2011). Satellite observations of high northern latitude vegetation productivity changes between 1982 and 2008: ecological variability and regional differences. Environ. Res. Lett. 6, 045501. doi:10.1088/1748-9326/6/4/045501
Brown, M. E., de Beurs, K., and Vrieling, A. (2010). The response of African land surface phenology to large scale climate oscillations. Remote Sens. Environ. 114, 2286–2296. doi:10.1016/jrse.2010.05.005
Cai, D., You, Q., Fraedrich, K., and Guan, Y. (2017). Spatiotemporal temperature variability over the Tibetan plateau: altitudinal dependence associated with the global warming hiatus. J. Clim. 30, 969–984. doi:10.1175/jcli-d-16-0343.1
Chen, B., Zhang, X., Tao, J., Wu, J., Wang, J., Shi, P., et al. (2014). The impact of climate change and anthropogenic activities on alpine grassland over the Qinghai-Tibet Plateau. Agric. For. Meteorol. 189–190, 11–18. doi:10.1016/j.agrformet.2014.01.002
Cheng, X., Lyu, L., Buntgen, U., Cherubini, P., Qiu, H., and Zhang, Q. (2019). Increased El Niño-southern oscillation sensitivity of tree growth on the southern Tibetan Plateau since the 1970s. Int. J. Climatol. 39, 3465–3475. doi:10.1002/joc.6032
Cong, N., Shen, M., Yang, W., Yang, Z., Zhang, G., and Piao, S. (2017). Varying responses of vegetation activity to climate changes on the Tibetan Plateau grassland. Int. J. Biometeorol. 61, 1433–1444. doi:10.1007/s00484-017-1321-5
Conroy, J. L., and Overpeck, J. T. (2011). Regionalization of present-day precipitation in the greater monsoon region of Asia. J. Clim. 24, 4073–4095. doi:10.1175/2011JCLI4033.1
Curio, J., Maussion, F., and Scherer, D. (2015). A 12-year high-resolution climatology of atmospheric water transport over the Tibetan Plateau. Earth Syst. Dynam. 6, 109–124. doi:10.5194/esd-6-109-2015
Delworth, T. L., Zeng, F., Vecchi, G. A., Yang, X., Zhang, L., and Zhang, R. (2016). The north Atlantic oscillation as a driver of rapid climate change in the northern hemisphere. Nat. Geosci. 9, 509–512. doi:10.1038/ngeo2738
Deng, H., Pepin, N. C., and Chen, Y. (2017). Changes of snowfall under warming in the Tibetan Plateau. J. Geophys. Res. Atmos. 122, 7323–7341. doi:10.1002/2017jd026524
Dong, M., Jiang, Y., Zheng, C., and Zhang, D. (2012). Trends in the thermal growing season throughout the Tibetan Plateau during 1960–2009. Agric. For. Meteorol. 166−167, 201–206. doi:10.1016/j.agrformet.2012.07.013
Dong, X., and Xue, F. (2016). Phase transition of the Pacific decadal oscillation and decadal variation of the east Asian summer monsoon in the 20th century. Adv. Atmos. Sci. 33, 330–338. doi:10.1007/s00376-015-5130-7
Dorji, T., Hopping, K. A., Wang, S., Piao, S., Tarchen, T., and Klein, J. A. (2018). Grazing and spring snow counteract the effects of warming on an alpine plant community in Tibet through effects on the dominant species. Agric. For. Meteorol. 263, 188–197. doi:10.1016/j.agrformet.2018.08.017
Du, J., Zhao, C., Shu, J., Jiaerheng, A., Yuan, X., Yin, J., et al. (2015). Spatiotemporal changes of vegetation on the Tibetan Plateau and relationship to climatic variables during multiyear periods from 1982–2012. Environ. Earth Sci. 75, 77. doi:10.1007/s12665-015-4818-4
Duan, A., and Xiao, Z. (2015). Does the climate warming hiatus exist over the Tibetan Plateau? Sci. Rep. 5, 13711. doi:10.1038/srep13711
Feng, J., Wang, L., and Chen, W. (2014). How does the east asian summer monsoon behave in the decaying phase of El Niño during different PDO phases? J. Clim. 27, 2682–2698. doi:10.1175/JCLI-D-13-00015.1
Feng, S., and Hu, Q. (2008). How the north Atlantic multidecadal oscillation may have influenced the Indian summer monsoon during the past two millennia. Geophys. Res. Lett. 35, L01707. doi:10.1029/2007gl032484
Fu, G., Zhang, X., Zhang, Y., Shi, P., Li, Y., Zhou, Y., et al. (2013). Experimental warming does not enhance gross primary production and above-ground biomass in the alpine meadow of Tibet. J. Appl. Remote Sens. 7, 073505. doi:10.1117/1.jrs.7.073505
Fu, Y., Ma, Y., Zhong, L., Yang, Y., Guo, X., Wang, C., et al. (2020). Land-surface processes and summer-cloud-precipitation characteristics in the Tibetan Plateau and their effects on downstream weather: a review and perspective. Natl. Sci. Rev. 7, 500–515. doi:10.1093/nsr/nwz226
Gao, Q., Guo, Y., Xu, H., Ganjurjav, H., Li, Y., Wan, Y., et al. (2016). Climate change and its impacts on vegetation distribution and net primary productivity of the alpine ecosystem in the Qinghai-Tibetan Plateau. Sci. Total Environ. 554–555, 34–41. doi:10.1016/j.scitotenv.2016.02.131
Gao, Y., Zhou, X., Wang, Q., Wang, C., Zhan, Z., Chen, L., et al. (2013). Vegetation net primary productivity and its response to climate change during 2001-2008 in the Tibetan Plateau. Sci. Total Environ. 444, 356–362. doi:10.1016/j.scitotenv.2012.12.014
Geng, Y., Wang, Y., Yang, K., Wang, S., Zeng, H., Baumann, F., et al. (2012). Soil respiration in Tibetan alpine grasslands: belowground biomass and soil moisture, but not soil temperature, best explain the large-scale patterns. PLoS One 7, e34968. doi:10.1371/journal.pone.0034968
Gonsamo, A., Chen, J. M., and Lombardozzi, D. (2016). Global vegetation productivity response to climatic oscillations during the satellite era. Global Change Biol. 22, 3414–3426. doi:10.1111/gcb.13258
Gou, X., Deng, Y., Chen, F., Yang, M., Gao, L., Nesje, A., et al. (2014). Precipitation variations and possible forcing factors on the northeastern Tibetan Plateau during the last millennium. Quat. Res. 81, 508–512. doi:10.1016/j.yqres.2013.09.005
Gou, X., Yang, T., Gao, L., Deng, Y., Yang, M., and Chen, F. (2013). A 457-year reconstruction of precipitation in the southeastern Qinghai-Tibet Plateau, China using tree-ring records. Chin. Sci. Bull. 58, 1107–1114. doi:10.1007/s11434-012-5539-7
Harris, I., Jones, P. D., Osborn, T. J., and Lister, D. H. (2014). Updated high-resolution grids of monthly climatic observations—the CRU TS3.10 dataset. Int. J. Climatol. 34, 623–642. doi:10.1002/joc.3711
Hopping, K. A., Knapp, A. K., Dorji, T., and Klein, J. A. (2018). Warming and land use change concurrently erode ecosystem services in Tibet. Global Change Biol. 24, 5534–5548. doi:10.1111/gcb.14417
Hua, T., and Wang, X. (2018). Temporal and spatial variations in the climate controls of vegetation dynamics on the Tibetan Plateau during 1982–2011. Adv. Atmos. Sci. 35, 1337–1346. doi:10.1007/s00376-018-7064-3
Huang, K., Zhang, Y., Zhu, J., Liu, Y., Zu, J., and Zhang, J. (2016a). The influences of climate change and human activities on vegetation dynamics in the Qinghai-Tibet Plateau. Rem. Sens. 8, 876. doi:10.3390/rs8100876
Huang, W., Bruemmer, B., and Huntsinger, L. (2016b). Incorporating measures of grassland productivity into efficiency estimates for livestock grazing on the Qinghai-Tibetan Plateau in China. Ecol. Econ. 122, 1–11. doi:10.1016/j.ecolecon.2015.11.025
Huang, W., Chen, J., Zhang, X., Feng, S., and Chen, F. (2015). Definition of the core zone of the “westerlies-dominated climatic regime,” and its controlling factors during the instrumental period. Sci. China Earth Sci. 58, 676–684. doi:10.1007/s11430-015-5057-y
Kan, A., Li, G., Yang, X., Zeng, Y., Tesren, L., and He, J. (2018). Ecological vulnerability analysis of Tibetan towns with tourism-based economy: a case study of the Bayi District. J. Mt. Sci. 15, 1101–1114. doi:10.1007/s11629-017-4789-x
Kang, S., Xu, Y., You, Q., Flügel, W. A., Pepin, N., and Yao, T. (2010). Review of climate and cryospheric change in the Tibetan Plateau. Environ. Res. Lett. 5, 015101. doi:10.1088/1748-9326/5/1/015101
Klein, J. A., Harte, J., and Zhao, X. (2008). Decline in medicinal and forage species with warming is mediated by plant traits on the Tibetan Plateau. Ecosystems 11, 775–789. doi:10.1007/s10021-008-9160-1
Krishnamurthy, L., and Krishnamurthy, V. (2014). Influence of PDO on south Asian summer monsoon and monsoon-ENSO relation. Clim. Dynam. 42, 2397–2410. doi:10.1007/s00382-013-1856-z
Kumar, K. K., Rajagopalan, B., and Cane, M. A. (1999). On the weakening relationship between the Indian monsoon and ENSO. Science 284, 2156–2159. doi:10.1126/science.284.5423.2156
Lehnert, L. W., Wesche, K., Trachte, K., Reudenbach, C., and Bendix, J. (2016). Climate variability rather than overstocking causes recent large scale cover changes of Tibetan pastures. Sci. Rep. 6, 24367. doi:10.1038/srep24367
Li, C., and Yanai, M. (1996). The onset and interannual variability of the asian summer monsoon in relation to land-sea thermal contrast. J. Clim. 9, 358–375. doi:10.1175/1520-0442(1996)009<0358:TOAIVO>2.0.CO;2
Li, J., Fan, K., and Xu, Z. (2015). Links between the late wintertime north Atlantic oscillation and springtime vegetation growth over Eurasia. Clim. Dynam. 46, 987–1000. doi:10.1007/s00382-015-2627-9
Li, J., Sun, C., and Jin, F. (2013). NAO implicated as a predictor of northern hemisphere mean temperature multidecadal variability. Geophys. Res. Lett. 40, 5497–5502. doi:10.1002/2013gl057877
Li, J., Yu, R., and Zhou, T. (2008). Teleconnection between NAO and climate downstream of the Tibetan plateau. J. Clim. 21, 4680–4690. doi:10.1175/2008JCLI2053.1
Li, L., Yang, S., Wang, Z., Zhu, X., and Tang, H. (2010). Evidence of warming and wetting climate over the Qinghai-Tibet Plateau. Arctic Antarct. Alpine Res. 42, 449–457. doi:10.1657/1938-4246-42.4.449
Li, L., Zhang, Y., Liu, L., Wu, J., Wang, Z., Li, S., et al. (2018). Spatiotemporal patterns of vegetation greenness change and associated climatic and anthropogenic drivers on the Tibetan plateau during 2000–2015. Rem. Sens. 10, 1525. doi:10.3390/rs10101525
Li, P., Hu, Z., and Liu, Y. (2020). Shift in the trend of browning in southwestern Tibetan Plateau in the past two decades. Agric. For. Meteorol. 287, 107950. doi:10.1016/j.agrformet.2020.107950
Li, T., and Li, J. (2017). A 564-year annual minimum temperature reconstruction for the east central Tibetan Plateau from tree rings. Global Planet. Change 157, 165–173. doi:10.1016/j.gloplacha.2017.08.018
Liu, X., Yin, Z., Shao, X., and Qin, N. (2006). Temporal trends and variability of daily maximum and minimum, extreme temperature events, and growing season length over the eastern and central Tibetan Plateau during 1961–2003. J. Geophys. Res. Atmos. 111, D19109. doi:10.1029/2005jd006915
Lu, H., and Liu, G. (2010). Trends in temperature and precipitation on the Tibetan Plateau, 1961–2005. Clim. Res. 43, 179–190. doi:10.3354/cr00909
Luo, F., Li, S., Gao, Y., Keenlyside, N., Svendsen, L., and Furevik, T. (2018). The connection between the Atlantic multidecadal oscillation and the Indian summer monsoon in CMIP5 models. Clim. Dynam. 51, 3023–3039. doi:10.1007/s00382-017-4062-6
Ma, L., Yao, Z., Zheng, X., Zhang, H., Wang, K., Zhu, B., et al. (2018). Increasing grassland degradation stimulates the non-growing season CO2 emissions from an alpine meadow on the Qinghai-Tibetan Plateau. Environ. Sci. Pollut. Res. Int. 25, 26576–26591. doi:10.1007/s11356-018-2724-5
Mantua, N. J., Hare, S. R., Zhang, Y., Wallace, J. M., and Francis, R. C. (1997). A Pacific interdecadal climate oscillation with impacts on salmon production. Bull. Am. Meteorol. Soc. 78, 1069–1080. doi:10.1175/1520-0477(1997)078<1069:APICOW>2.0.CO;2
Maussion, F., Scherer, D., Mölg, T., Collier, E., Curio, J., and Finkelnburg, R. (2014). Precipitation seasonality and variability over the Tibetan plateau as resolved by the high Asia reanalysis. J. Clim. 27, 1910–1927. doi:10.1175/jcli-d-13-00282.1
Pan, N., Feng, X., Fu, B., Wang, S., Ji, F., and Pan, S. (2018). Increasing global vegetation browning hidden in overall vegetation greening: insights from time-varying trends. Remote Sens. Environ. 214, 59–72. doi:10.1016/j.rse.2018.05.018
Piao, S., Cui, M., Chen, A., Wang, X., Ciais, P., Liu, J., et al. (2011). Altitude and temperature dependence of change in the spring vegetation green-up date from 1982 to 2006 in the Qinghai-Xizang Plateau. Agric. For. Meteorol. 151, 1599–1608. doi:10.1016/j.agrformet.2011.06.016
Piao, S., Tan, K., Nan, H., Ciais, P., Fang, J., Wang, T., et al. (2012). Impacts of climate and CO2 changes on the vegetation growth and carbon balance of Qinghai-Tibetan grasslands over the past five decades. Global Planet. Change 98–99, 73–80. doi:10.1016/j.gloplacha.2012.08.009
Pinzon, J. E., and Tucker, C. J. (2014). A non-stationary 1981–2012 AVHRR NDVI3g time series. Rem. Sens. 6, 6929–6960. doi:10.3390/rs6086929
Shang, Z., Feng, Q., Wu, G., Ren, G., and Long, R. (2013). Grasslandification has significant impacts on soil carbon, nitrogen and phosphorus of alpine wetlands on the Tibetan Plateau. Ecol. Eng. 58, 170–179. doi:10.1016/j.ecoleng.2013.06.035
Shen, M., Piao, S., Chen, X., An, S., Fu, Y. H., Wang, S., et al. (2016). Strong impacts of daily minimum temperature on the green-up date and summer greenness of the Tibetan Plateau. Global Change Biol. 22, 3057–3066. doi:10.1111/gcb.13301
Shen, M., Piao, S., Cong, N., Zhang, G., and Jassens, I. A. (2015a). Precipitation impacts on vegetation spring phenology on the Tibetan Plateau. Global Change Biol. 21, 3647–3656. doi:10.1111/gcb.12961
Shen, M., Piao, S., Dorji, T., Liu, Q., Cong, N., Chen, X., et al. (2015b). Plant phenological responses to climate change on the Tibetan Plateau: research status and challenges. Natl. Sci. Rev. 2, 454–467. doi:10.1093/nsr/nwv058
Shen, M., Piao, S., Jeong, S. J., Zhou, L., Zeng, Z., Ciais, P., et al. (2015c). Evaporative cooling over the Tibetan Plateau induced by vegetation growth. Proc. Natl. Acad. Sci. U.S.A. 112, 9299–9304. doi:10.1073/pnas.1504418112
Shen, M., Tang, Y., Klein, J., Zhang, P., Gu, S., Shimono, A., et al. (2008). Estimation of aboveground biomass using in situ hyperspectral measurements in five major grassland ecosystems on the Tibetan Plateau. J. Plant Ecol. 1, 247–257. doi:10.1093/jpe/rtn025
Shi, C., Masson-Delmotte, V., Daux, V., Li, Z., and Zhang, Q.-B. (2010). An unstable tree-growth response to climate in two 500 year chronologies, north eastern Qinghai-Tibetan Plateau. Dendrochronologia 28, 225–237. doi:10.1016/j.dendro.2009.12.002
Shi, C., Sun, C., Wu, G., Wu, X., Chen, D., Masson-Delmotte, V., et al. (2019). Summer temperature over the Tibetan Plateau modulated by Atlantic multidecadal variability. J. Clim. 32, 4055–4067. doi:10.1175/jcli-d-17-0858.1
Shi, F., Wu, X., Li, X., Chen, D., Liu, H., Liu, S., et al. (2018). Weakening relationship between vegetation growth over the Tibetan Plateau and large-scale climate variability. J. Geophys. Res. Biogeosci. 123, 1247–1259. doi:10.1002/2017jg004134
Shi, S., Li, J., Shi, J., Zhao, Y., and Huang, G. (2017). Three centuries of winter temperature change on the southeastern Tibetan Plateau and its relationship with the Atlantic multidecadal oscillation. Clim. Dynam. 49, 1305–1319. doi:10.1007/s00382-016-3381-3
Tang, L., Dong, S., Sherman, R., Liu, S., Liu, Q., Wang, X., et al. (2015). Changes in vegetation composition and plant diversity with rangeland degradation in the alpine region of Qinghai-Tibet Plateau. Rangel. J. 37, 107–115. doi:10.1071/RJ14077
Tang, Y., Wan, S., He, J., and Zhao, X. (2009). Foreword to the special issue: looking into the impacts of global warming from the roof of the world. J. Plant Ecol. 2, 169–171. doi:10.1093/jpe/rtp026
Tucker, C. J. (1979). Red and photographic infrared linear combinations for monitoring vegetation. Rem. Sens. Environ. 8, 127–150. doi:10.1016/0034-4257(79)90013-0
van Oldenborgh, G. J., te Raa, L. A., Dijkstra, H. A., and Philip, S. Y. (2009). Frequency- or amplitude-dependent effects of the Atlantic meridional overturning on the tropical Pacific Ocean. Ocean Sci. 5, 293–301. doi:10.5194/os-5-293-2009
Wang, H., Liu, H., Cao, G., Ma, Z., Li, Y., Zhang, F., et al. (2020). Alpine grassland plants grow earlier and faster but biomass remains unchanged over 35 years of climate change. Ecol. Lett. 23, 701–710. doi:10.1111/ele.13474
Wang, J., Yang, B., Ljungqvist, F. C., and Zhao, Y. (2013). The relationship between the Atlantic multidecadal oscillation and temperature variability in China during the last millennium. J. Quat. Sci. 28, 653–658. doi:10.1002/jqs.2658
Watanabe, T., and Yamazaki, K. (2014). Decadal-scale variation of south Asian summer monsoon onset and its relationship with the Pacific decadal oscillation. J. Clim. 27, 5163–5173. doi:10.1175/jcli-d-13-00541.1
Wu, X., and Mao, J. (2019). Decadal changes in interannual dependence of the bay of bengal summer monsoon onset on ENSO modulated by the Pacific decadal oscillation. Adv. Atmos. Sci. 36, 1404–1416. doi:10.1007/s00376-019-9043-8
Yang, L., Chen, S., Wang, C., Wang, D., and Wang, X. (2018). Potential impact of the Pacific decadal oscillation and sea surface temperature in the tropical Indian Ocean-western Pacific on the variability of typhoon landfall on the China coast. Clim. Dynam. 51, 2695–2705. doi:10.1007/s00382-017-4037-7
Yoon, J., and Yeh, S. W. (2010). Influence of the Pacific decadal oscillation on the relationship between El Niño and the Northeast Asian summer monsoon. J. Clim. 23, 4525–4537. doi:10.1175/2010jcli3352.1
You, Q., Fraedrich, K., Ren, G., Pepin, N., and Kang, S. (2013). Variability of temperature in the Tibetan Plateau based on homogenized surface stations and reanalysis data. Int. J. Climatol. 33, 1337–1347. doi:10.1002/joc.3512
Yu, J., Liu, Y., and Li, X. (2014). Connections between the dominant modes of westerly over the upstream region of Qinghai-Xizang Plateau and the regional precipitation of China and NAO in winter. Plateau Meteorol. 33, 877–886. [in Chinese] doi:10.7522/j.issn.1000-0534.2013.00108
Yu, S., Xia, J., Yan, Z., and Yang, K. (2018). Changing spring phenology dates in the three-rivers headwater region of the Tibetan Plateau during 1960–2013. Adv. Atmos. Sci. 35, 116–126. doi:10.1007/s00376-017-6296-y
Zeng, H., Ji, J., and Wu, G. (2008). An updated coupled model for land-atmosphere interaction. Part II: simulations of biological processes. Adv. Atmos. Sci. 25, 632–640. doi:10.1007/s00376-008-0632-1
Zhang, C., Tang, Q., and Chen, D. (2017). Recent changes in the moisture source of precipitation over the Tibetan Plateau. J. Clim. 30, 1807–1819. doi:10.1175/jcli-d-15-0842.1
Zhang, L., Guo, H., Wang, C., Ji, L., Li, J., Wang, K., et al. (2014). The long-term trends (1982–2006) in vegetation greenness of the alpine ecosystem in the Qinghai-Tibetan Plateau. Environ. Earth Sci. 72, 1827–1841. doi:10.1007/s12665-014-3092-1
Zhang, X., Jin, L., and Jia, W. (2016). Centennial-scale teleconnection between north Atlantic sea surface temperatures and the Indian summer monsoon during the Holocene. Clim. Dynam. 46, 3323–3336. doi:10.1007/s00382-015-2771-2
Zhang, Y., Liu, C., Tang, Y., and Yang, Y. (2007). Trends in pan evaporation and reference and actual evapotranspiration across the Tibetan Plateau. J. Geophys. Res. Atmos. 112, D12110. doi:10.1029/2006jd008161
Zhang, Z., Chang, J., Xu, C., Zhou, Y., Wu, Y., Chen, X., et al. (2018). The response of lake area and vegetation cover variations to climate change over the Qinghai-Tibetan Plateau during the past 30years. Sci. Total Environ. 635, 443–451. doi:10.1016/j.scitotenv.2018.04.113
Zhao, J., Xu, Z., Singh, V. P., Zuo, D., and Li, M. (2016). Sensitivity of potential evapotranspiration to climate and vegetation in a water-limited basin at the northern edge of Tibetan Plateau. Water Resour. Manag. 30, 4667–4680. doi:10.1007/s11269-016-1446-z
Zhao, Y., Duan, A., Wu, G., and Sun, R. (2019). Response of the Indian ocean to the Tibetan Plateau thermal forcing in late spring. J. Clim. 32, 6917–6938. doi:10.1175/jcli-d-18-0880.1
Zheng, Z., Zhu, W., and Zhang, Y. (2020). Seasonally and spatially varied controls of climatic factors on net primary productivity in alpine grasslands on the Tibetan Plateau. Glob. Ecol. Conserv. 21, e00814. doi:10.1016/j.gecco.2019.e00814
Zhong, L., Ma, Y., Xue, Y., and Piao, S. (2019). Climate change trends and impacts on vegetation greening over the Tibetan Plateau. J. Geophys. Res. Atmos. 124, 7540–7552. doi:10.1029/2019jd030481
Zhu, Z., Piao, S., Myneni, R. B., Huang, M., Zeng, Z., Canadell, J. G., et al. (2016). Greening of the Earth and its drivers. Nat. Clim. Change 6, 791–795. doi:10.1038/nclimate3004
Keywords: Alpine ecosystem, Atlantic Multidecadal Oscillation, Pacific Decadal Oscillation, precipitation, temperature, growth of vegetation
Citation: Zhang L, Shen M, Shi C, Shi F, Jiang N, Yang Z and Ji Z (2021) Local Climatic Factors Mediated Impacts of Large-Scale Climate Oscillations on the Growth of Vegetation Across the Tibetan Plateau. Front. Environ. Sci. 9:597971. doi: 10.3389/fenvs.2021.597971
Received: 23 August 2020; Accepted: 07 January 2021;
Published: 04 March 2021.
Edited by:
Yun Wang, Senckenberg Museum of Natural History Görlitz, GermanyReviewed by:
Xianzhou Zhang, Chinese Academy of Sciences, ChinaCopyright © 2021 Zhang, Shen, Shi, Shi, Jiang, Yang and Ji. This is an open-access article distributed under the terms of the Creative Commons Attribution License (CC BY). The use, distribution or reproduction in other forums is permitted, provided the original author(s) and the copyright owner(s) are credited and that the original publication in this journal is cited, in accordance with accepted academic practice. No use, distribution or reproduction is permitted which does not comply with these terms.
*Correspondence: Miaogen Shen, c2hlbi5taWFvZ2VuQGdtYWlsLmNvbQ==; Chunming Shi, Y2h1bm1pbmcuc2hpQGdtYWlsLmNvbQ==
Disclaimer: All claims expressed in this article are solely those of the authors and do not necessarily represent those of their affiliated organizations, or those of the publisher, the editors and the reviewers. Any product that may be evaluated in this article or claim that may be made by its manufacturer is not guaranteed or endorsed by the publisher.
Research integrity at Frontiers
Learn more about the work of our research integrity team to safeguard the quality of each article we publish.