- 1UK Centre for Ecology & Hydrology, Wallingford, United Kingdom
- 2Cardiff School of Biosciences, University of Cardiff, Cardiff, United Kingdom
Because it is only possible to test chemicals for effects on a restricted range of species and exposure scenarios, ecotoxicologists are faced with a significant challenge of how to translate the measurements in model species into predictions of impacts for the wider range of species in ecosystems. Because of this challenge, within ecotoxicology there is no more fundamental aspect than to understand the nature of the traits that determine sensitivity. To account for the uncertainties of species extrapolations in risk assessment, “safety factors” or species sensitivity distributions are commonly used. While valuable as pragmatic tools, these approaches have no mechanistic grounding. Here we highlight how mechanistic information that is increasingly available for a range of traits can be used to understand and potentially predict species sensitivity to chemicals. We review current knowledge on how toxicokinetic, toxicodynamic, physiological, and ecological traits contribute to differences in sensitivity. We go on to discuss how this information is being used to make predictions of sensitivity using correlative and trait-based approaches, including comparisons of target receptor orthologs. Finally, we discuss how the emerging knowledge and associated tools can be used to enhance theoretical and applied ecotoxicological research through improvements in mechanistic modeling, predictive ecotoxicology, species sensitivity distribution development, mixture toxicity assessment, chemical design, biotechnology application and mechanistically informed monitoring.
Introduction
Chemicals are the business of some of the largest industrial sectors. Whether as raw materials from mining, oil and gas, as agrochemicals, textile, plastics, cosmetics, personal care, cleaning or through use in the pharmaceutical industry, chemicals pervade every aspect of modern life. When in widespread use, it becomes almost inevitable that chemicals will find their way into the environment (Rockstrom et al., 2009). Routes may be through direct application and run-off of agrochemicals (Kohler and Triebskorn, 2013; Elias et al., 2018), intended or accidental industrial releases (Batty and Hallberg, 2010), or via waste and wastewater infrastructure, such as for down the drain products passing through wastewater treatment (Munoz et al., 2008; Gardner et al., 2012), or in leachates from landfills (Masoner et al., 2014; Kummerer et al., 2019). As well as their recognized health effects (Landrigan et al., 2018), pollutant chemicals can also affect wildlife species and ecological communities (Hayes et al., 2018; Johnson et al., 2020), placing real costs on ecosystem functions and services (Wang et al., 2019). For example, Pretty et al. (2000) identified external costs of £120 M and £16 M for pesticide and fertilizer applications to the United Kingdom drinking water supply chain. Demonstrated, expected and even perceived impacts on ecosystem widely shape public opinion on chemical safety and use, even in those cases where no impacts may actually exist (i.e., where a source-pathway-receptor link cannot be established). Given the scale, diversity and potential costs, effective management of chemicals through risk assessment is critical for environmental management (Jenck et al., 2004).
To tackle issues in risk assessment, science-based chemical management faces the fundamental problem of how to extrapolate data produced for a small number of “standard” test species to predict impacts for a large number of species from different phyla present in an ecosystem that may differ in their physiology, diet and life-history traits. This challenge is relevant both in cases of prospective assessments to assess the likelihood of future hazard and risk (e.g., during the registration of a new substance, such as a pesticide) or for retrospective assessments of the impact of known chemical releases (e.g., through industrial emissions or an accidental release) on ecosystem structure and function (Faber et al., 2019). With >80,000 chemicals produced at >1 ton volume in Europe, testing even for a “representative” subset of “standard” species cannot be achieved in any sensible timescale. Defining “safe” contaminant concentrations for ecosystems, therefore, often relies on the use of an arbitrary “safety factor” (usually division of a toxicity metric, such as an LCx, ECx or NOEC by 100 or 1,000) placed on data available often for only a small number of species (e.g., in aquatic risk assessment often only Daphnia, fish, algae) or at best species sensitivity distributions (SSDs) constructed with at most 10s of species (Posthuma et al., 2019).
This assessment factor approach is a pragmatic solution to address the uncertainties relating to lack of knowledge on the basis and range of species sensitivity. The approach has notable technical flaws, not just in that the size of assessment factor used is largely arbitrary, but also that the derivation of any value for regulatory application (e.g., a predicted no-effect concentration) is driven by the most sensitive species. As a consequence, hazard values derived using generic safety factors may sometime be over-precautionary, but in others may fail to protect vulnerable species (Lau et al., 2014). Failures of protection from the application of assessment factors have previously been found to occur in cases where a given chemical has an impact on a specific physiological trait, such as behavior, immuno-competence, genotoxicity and physiology that is not commonly measured in standard regulatory toxicity tests. Such unforeseen effects account for some of the most well-known impacts of chemicals on populations, such as tributyltin on marine mollusks (Matthiessen and Law, 2002); neonicotinoid pesticides for bees (Jin et al., 2015; Woodcock et al., 2016; Douglas et al., 2020); DDT on birds of prey (Newton and Wyllie, 1992); and the anti-inflammatory drug diclofenac on vulture populations (Oaks et al., 2004).
For the chemicals of highest concern, such as some trace metals, high volume use pesticides, selected pharmaceuticals and more recently some nanomaterials, specific research has resulted in the development of a richer ecotoxicological effects database covering a greater range of species (Posthuma et al., 2001; Semenzin et al., 2015). The additional data available for these well-studied chemicals almost always comprises results from short-term laboratory toxicity tests for an extended range of species. Even for the most investigated chemicals, the number of species for which toxicity has been assessed remains relatively small. For example, Mebane (2010) identified a data-set of 27 species within 21 genera for cadmium; while Zhao and Chen (2016) extracted sensitivity data for 207 freshwater and saltwater species for the organophosphate insecticide chlorpyrifos in what is probably the largest ecotoxicological effect database currently available for any substance. In those relatively few cases where the ecotoxicological effects literature is large and taxonomically broad, then the distribution of toxicity metrics (e.g., LCxs, ECxs, NOECs etc.) can be modeled as a statistical distribution to assess the pattern and order of species sensitivities. Such analyses have become the basis for sensitivity distribution (SSD) approaches in ecotoxicology (Posthuma et al., 2001).
The initial conceptualization of species sensitivity distributions as descriptive tools to summarize ecotoxicological data was founded on principles that species sensitivity varies in a symmetrical distribution on a log scale (Kooijman, 1987). This concept was later formulated into an approach for deriving ecosystem protection goals for chemical exposure by selecting a protection goal for accepted effect on a given percentage of species (Van Straalen and Denneman, 1989). Conventionally the threshold for effects routinely used with SSDs has been to protect 95% of species and, thereby, to accept an adverse effect on 5%. This protection goal is known as the hazardous concentration for 5% of species (the “HC5”). By using systematically collated toxicity data from multiple studies to gain a more complete estimation of response than achieved by a single study, SSD represents an early example of the application of meta-analysis approaches to environmental management problems. In the thirty years since their initial development, SSDs have passed into routine use in chemical policy and management.
In the period immediately after SSD conceptualization and integration into policy, a series of concerns with their technical and ecological bases were raised. An initial methodological issue concerned the shape of the distribution of sensitivity between species. Doubts were expressed on the choice of the log-logistic distribution as the best model to describe the pattern of rank order for sensitivity, even by Kooijman (1987) in the founding model paper. A comparison of SSD model fits has suggested that a model based on a Burr III distribution may provide a better fit to data than either log-normal or log-logistic fits (He et al., 2014). However, models using a Burr III distribution have computation drawbacks in their processing complexity, meaning that the log-logistic fit remains the dominant model form used in SSD studies (Xu et al., 2015).
A more fundamental issue with the use of SSDs for setting environmental protection goals concerns the selection of acceptable thresholds and the potential for effects on keystone species (Hopkin, 1993). The framework for assessment assumes that the range of sensitivity of the tested species used to generate the SSD matches those of the species in the focus ecosystem. This is not a justified assumption, based on the fact that the majority of test data comes from laboratory species presenting similar traits of short generational time, rapid growth and high fecundity that are not replicated in many species in natural communities (Beaudouin and Pery, 2013). Evidence that some types of chemicals may strongly affect species in specific groups that undertake key ecosystem processes (e.g., herbicide effects on primary producers) have resulted in the development of species sensitivity distribution for only species from those taxa (King et al., 2015; Wang et al., 2015; Baillard et al., 2020). This selection, which can be based on taxonomy or function, avoids setting protection goals that disproportionately leave key functional species among the affected 5%, even when the protection goals for 95% of species is met (Hopkin, 1993; Forbes and Calow, 2002).
A further concern raised on the value of SSDs is whether the sensitivity of organisms tested in the laboratory matches that found among field species. Again aware of this issue, Kooijman (1987) identified disagreement between biologists on whether the species selected for testing were likely to be on average hardier or more susceptible than field species given their amenability for laboratory culturing and experimental use. To make this comparison, SSDs derived from laboratory toxicity data have been compared to community impacts in the field. Results have been found to both corroborate SSD predictions (e.g., Posthuma and DeZwart, 2006) and also to suggest that SSDs may underestimate the effect (e.g., Smetanova et al., 2014). Because of this remaining uncertainty, current risk assessment schemes, such as that for pesticides in the EU, do not use the unamended HC5 derived from an SSD as the protection goal (Brock et al., 2006). Instead, assessment factors scaled according to the amount of data used for SSD development are placed on the HC5. The need to include an additional assessment factor as a blunt tool to support precautionary analysis indicates that, while undoubtedly useful, descriptive SSD models provide only a partial solution to the pan-species problem in chemical risk assessment.
Understanding the Basis of Differential Sensitivity
The basis of species sensitivity is that chemical exposure at a sufficient concentration imparts negative impacts on the physiology, behavior and ultimately life-history and population dynamics of an exposed species. This interaction of the chemical with the physiology of species has been recognized through the development of the adverse outcome pathways (AOPs) concept, an approach that combines existing knowledge of xenobiotic interactions with a specific biomolecule as the “molecular initiating event” (MIE) to apical endpoints across different levels of biological organization (Ankley et al., 2010). The AOP concept provides an approach to support more mechanistically driven toxicology including by the identification and analysis of conserved toxicokinetics (TK) and toxicodynamics (TD) pathways. Toxicokinetic pathways underlie the Adsorption-Distribution-Metabolism-Excretion (ADME) traits that determine chemical concentration in organisms, and ultimately reaching target sites (McCarty and Mackay, 1993; Nyman et al., 2012). Toxicodynamics covers the processes of interaction between the chemical and the target and the resulting cascade of events leading to phenotypic change within the AOP (McCarty and Mackay, 1993; Nyman et al., 2012). An understanding of species TK and TD traits have a significant potential to contribute to support species read-across (Rivetti et al., 2020). Consequently, an understanding of the range of biochemical pathways that contribute to TK and TD traits can be informative for understanding the nature and severity of species response to chemical exposures (see Figure 1 for trait organization and integration).
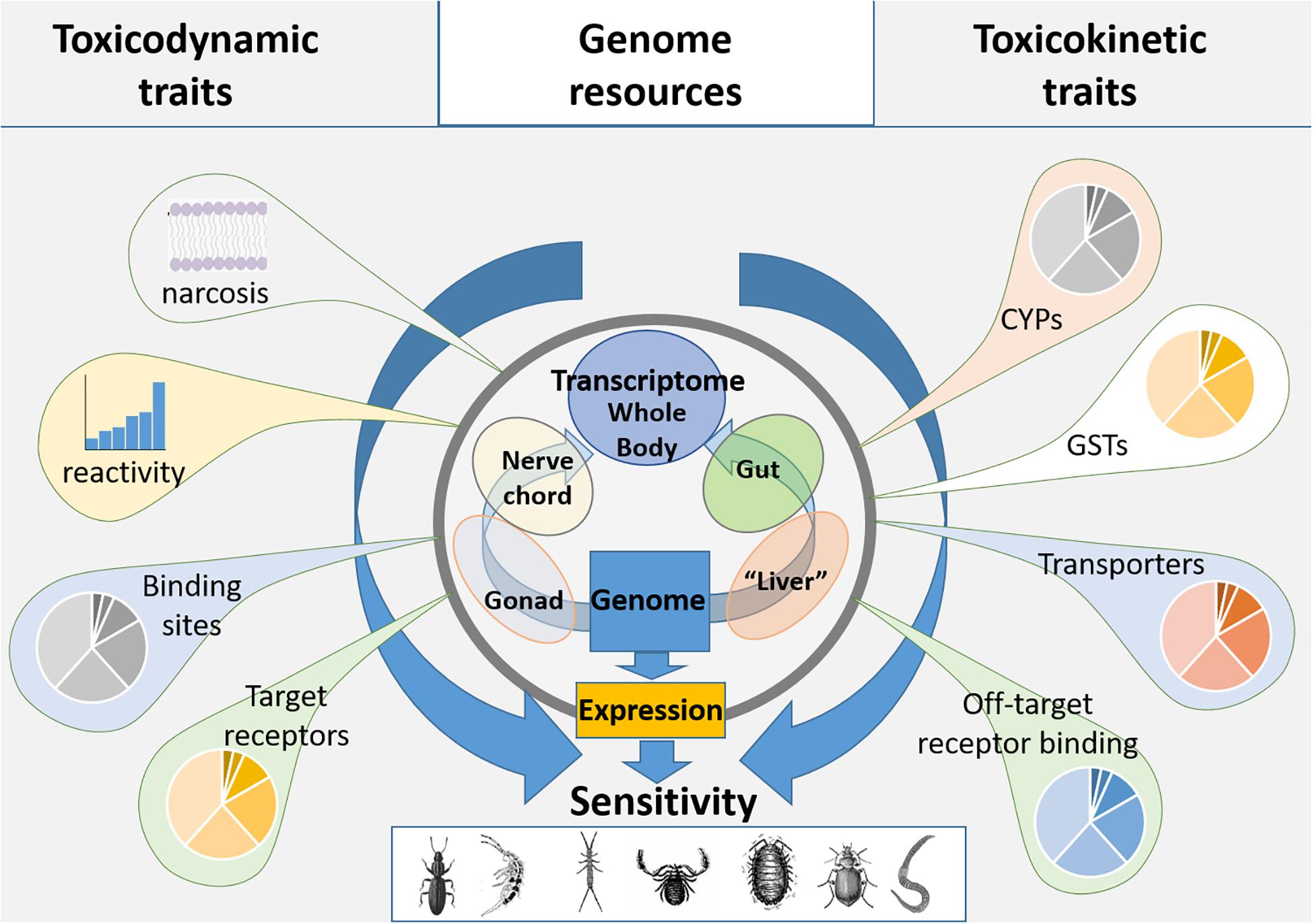
Figure 1. Summary of the TK and TD traits that may be linked to species sensitivity, traits can be inherent or derived from a range of available genome resources.
AOPs currently exist in a range of forms, from putative and partially characterized to well−described and fully validated (Coady et al., 2019). They range from qualitative to semi-quantitative and quantitative AOP (qAOP) models (Spinu et al., 2020). A fully developed qAOP can help guide the interpretation of comparative effects between species, as the link between exposure level and the degree of response of the adverse outcome is established. However, for the purposes of predicting species sensitivity, the application of qAOPs may be challenged by the very differences that they seek to investigate. In some cases, comparative analyses may be able to reliably attribute a mechanism and a scale of toxicant effects across species based on binding to the highly conserved MIE target. For example, a qAOP for an organophosphate could be plausibly transferred across species, due to the conservation of nervous system and of the target acetylcholinesterase receptors (Brockmeier et al., 2017), although even in this context variation may remain depending on species specific processes such as the relative irreversibility of acetylcholinesterase inhibition (Wallace and Herzberg, 1988). However, generalizing a qAOP to understand chemical sensitivity for species outside of the taxonomic range for which it has been formulated needs to be considered within different evolutionary and physiological contexts (Gunnarsson et al., 2008; Verbruggen et al., 2018). For example, for compounds that mimic estrogen, the target estrogen receptor sequences show more variance and may not even be present in some taxa (e.g., for Daphnia). Thus, qAOPs for mechanisms of effect applied to species with a different receptor structure may lead to highly erroneous sensitivity prediction for a chemical working through this pathway. Understanding the contribution of evolutionary processes and traits to sensitivity is, thus, needed to understand the contexts into which qAOPs can or cannot be applied.
The Contribution of Evolutionary Process to Species Sensitivity
Microevolutionary or macroevolutionary changes that impact on TK and TD traits would be anticipated to modify sensitivity by changing either target site exposure or chemical-target site interactions (Klerks et al., 2011). There are some well-established examples of known microevolutionary changes that can modify sensitivity. The development of antibiotic resistance in microbial populations is such a case. Antibiotic resistance can arise from exposure to specifically designed antimicrobials or through cross tolerance to other contaminants (Bell et al., 2014; Xie et al., 2018). Indeed there is growing evidence of a co-evolutionary process of antimicrobial resistance arising from environmental exposure to organic pollutants and even metals leading to the development of clinically relevant resistance (Pal et al., 2015). Because of its obvious therapeutic relevance, antibiotic resistance is one of the most widely understood microevolutionary processes for any group of toxicants. The many mechanisms identified leading to resistance include changes in both TK traits, such as acquisition or modification of cellular chemical transport systems that remove chemicals from cells, and also TD processes such as modifications to the structural confirmation and functional characteristics of cellular targets (Davies and Davies, 2010). These widely observed and well understood trajectories establish a dominant paradigm of TK and TD change as causes of differences in population sensitivity to toxicants.
Understanding the microevolution for TK and TD traits also provides a rationale for characterizing pesticide resistance. The development of insecticide resistance was initially documented for inorganic pesticides even during their early use (Melander, 1914). In the intervening years, the diversification of pesticides has been associated with expansion in the range of species exhibiting resistance. Studies that have addressed the causes of resistance identified two major non-competing mechanisms: increased metabolic detoxification and decreased sensitivity of the target-site that represent, respectively, TK and TD changes (Van Leeuwen et al., 2010; Liu, 2015; Siegwart et al., 2017). Increased metabolic detoxification arises following mutational changes that increase the expression or efficiency of one or more enzymes involved in endogenous pesticide metabolism or excretion. Typically esterases are the main enzymes involved, although resistance has also been linked to changes in glutathione-s-transferase expression and activity (Hemingway et al., 2004). Target site insensitivity also has multiple mechanistic bases, including point mutations that change structural confirmation and, thereby, ligand-receptor affinity (Hemingway et al., 2004). There is obvious value in identifying the dominant mechanisms of resistance as this can support mitigation. However, as TK associated changes to metabolism and TD associated changes to target site interactions are effectively independent, many cases of insecticide resistance show characteristics of both types of response (Reyes et al., 2007; Siegwart et al., 2017).
Microevolutionary processes leading to the development of tolerance have also been shown to shape population responses to inorganic chemicals, e.g., trace metal exposures. There is a significant body of evidence for the development of population tolerances to metals in long-term exposed invertebrates, plants and microbial populations (see review of Posthuma and Van Straalen, 1993; Meharg, 2005; Singh et al., 2016; Chandrangsu et al., 2017). Tolerance to metal exposure in animals has been associated with microevolutionary processes that change the expression of metallothioneins and in plants to changes in phytochelatins synthases that together alter the TK processes of metal uptake, transport and detoxification in the adapted species (Dallinger and Hockner, 2013; Weng and Wang, 2014; Zanella et al., 2016; Shaw et al., 2019; Lwalaba et al., 2020; Mekawy et al., 2020). For example, in populations of the Collembola O. cincta, Costa et al. (2012) showed that a metal-tolerant phenotype was mainly influenced by cis-genetic factors acting on metallothionein expression, with specific promoter genotypes found dominantly in cadmium tolerant populations. Observations in a further springtail species Folsomia candida also supported a role of constitutive metallothionein expression in cadmium tolerance, influenced by both trans and cis regulatory variation (Costa et al., 2012).
While mechanisms of adaptation can become hard wired into the genome, more transient changes in the epigenome have also been associated with the capacity of organisms to respond to chemicals. Such epigenetic changes appear to be associated with downstream modification of both TK and TD processes (Bruce et al., 2007; Mirbahai and Chipman, 2014; Brander et al., 2017; Horemans et al., 2019). Components of the epigenome, such as DNA methylation, are emerging as the interface between the static genome and the dynamic and changing environment in which species live and persist (Marczylo et al., 2016; Horemans et al., 2019). Evidence that epigenetic marks induced by adverse conditions encountered by the parents, such as stressor exposure can be partly stable across generations is beginning to build in plants, vertebrates and more recently in invertebrates (against prior expectations) leading to transgenerational heritable changes in offspring (Greer et al., 2011; Pecinka and Scheid, 2012; Uller et al., 2015; Klosin et al., 2017; Stassen et al., 2018). Examples include the observation of the transfer of stress tolerance associated with gene expression changes linked with altered trimethylation of histone H3 lysine 9, identified as a mechanisms for transgenerational inheritance of the maintenance of stressor responses in C. elegans (Klosin et al., 2017) and heritable changes in a DNA methylation in Arabadopsis in response to nickel exposure (Li et al., 2015). A role of epigenetic mechanisms in heritable effects has also been identified in Daphnia magna in response to ionizing radiation (Jeremias et al., 2018; Trijau et al., 2018), silver nanoparticles (Ellis et al., 2020), uranium (Dias et al., 2008; Massarin et al., 2011; Dutilleul et al., 2014; Goussen et al., 2015) and a DNA methylation inhibitor (Lindeman et al., 2019). How these epigenetic mechanisms are linked to sensitivity is currently unknown. However, evidence of altered DNA methylation in an arsenic tolerant populations of the earthworm L. rubellus (Kille et al., 2013) that also express high levels of phytochelatins (Liebeke et al., 2013) suggests a role in regulating detoxification mechanisms.
Physiological Traits as Determinants of Species Sensitivity
Multiple studies have identified how species biological traits can lead to differences in responses to chemical exposure. An understanding of the evolutionary background of species and especially their shared and distinct TK and TD traits can, thus, contribute greatly to our understanding of their role as drivers of sensitivity. The role of TK/TD and AOP traits in toxicity can be seen as orthogonal (Figure 2). TK traits related to adsorption distribution, metabolism and excretion determine the concentration of the chemical entering into the organisms, as well as the distribution to and removal from the target site at which the MIE occurs. Examples for traits that may affect TK rates leading to changes in exposure include species behavior (e.g., feeding traits), anatomical traits (e.g., surface area-to-body mass scaling relationship), metabolic capacities (e.g., direct via CYP divergence or indirect via the AH-receptor that activates CYP expression), stress-response capacities (e.g., Metallothionein/phytochelatin expression patterns) and microbiome differences (e.g., presence of gut bacteria known to degrade xenobiotic chemicals). The specific nature of the TD interactions cover mechanisms through which chemicals interact at target sites to result, triggering a MIE that leads to the biochemical, cellular, tissue organism that together comprise the toxicant AOP (Figure 2). Examples of traits that may affect TK rates include lipid content variations affecting the nature of narcotic interactions, presence/absence of target receptors to determine whether species experience toxicity through specific trigger AOPs, gene duplication and loss varying receptor ortholog compliment and target receptor sequence leading to binding site differences that affect ligand-receptor interaction strengths. Evidence relating to the importance of such changes affecting sensitivity are detailed below.
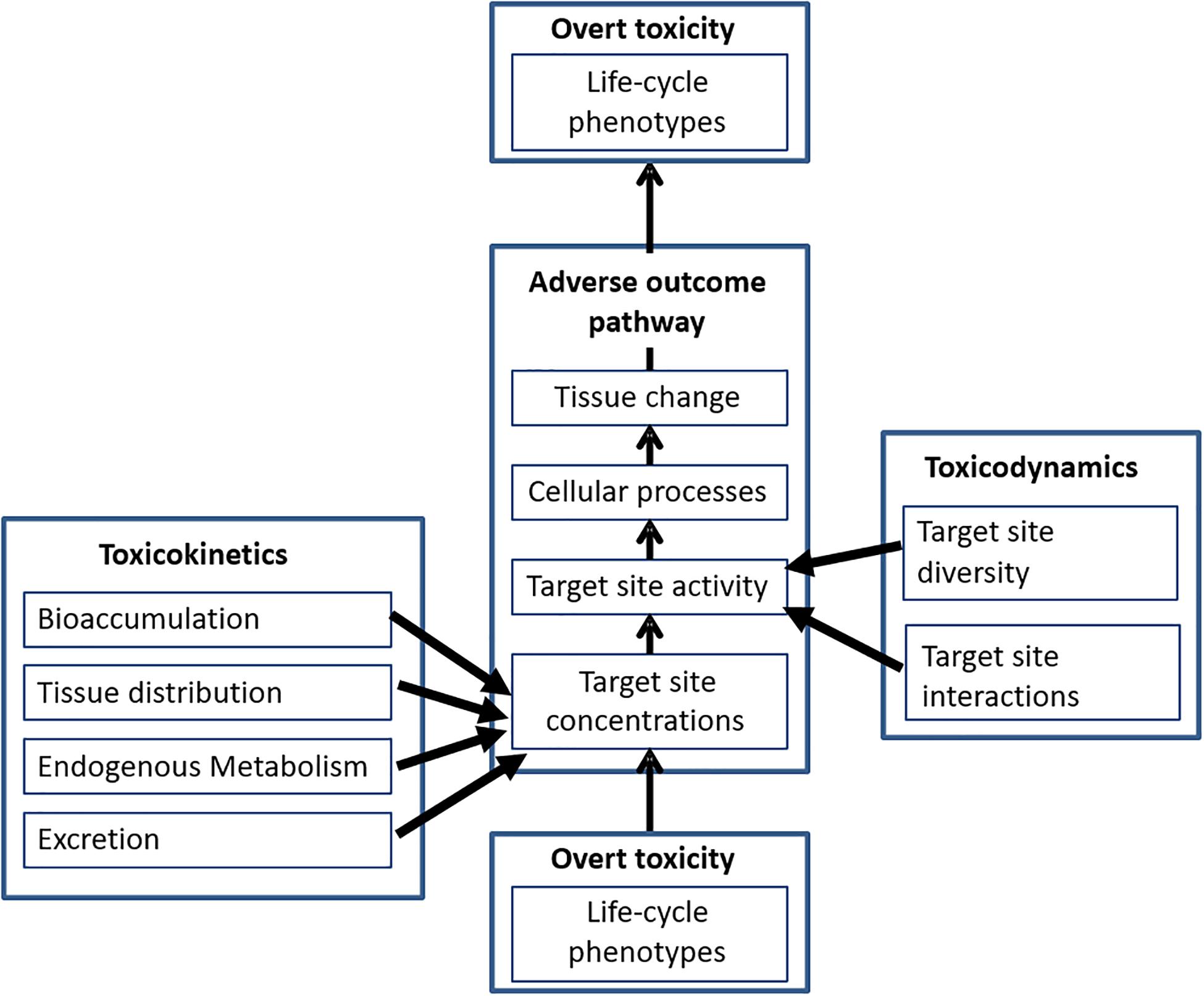
Figure 2. The integration of TK and TD traits within the AOP framework, TK traits determine the concentration of the chemical reaching the site, TD traits determine the nature of the target site and influence downstream AOP processes leading ultimately to overt toxicity.
Toxicokinetic Traits and Species Sensitivity
Species traits can govern the extent to which exposure may occur. At the simplest level, the movement of species through the landscape and dietary choices can influence exposure. For example, Woodcock et al. (2016) identified that bee species foraging on oilseed rape treated with systemic neonicotinoids showed greater population declines than non-oilseed rape feeding species not similarly subject to insecticide exposure. In this case there was no indication that different species population trajectories were driven by inherent sensitivity, but rather by feeding traits that governed exposure. A somewhat analogous situation exists for other established cases of species vulnerability, such as top predator (e.g., predatory birds) exposure to persistent, bioaccumulative and toxic chemicals through food chain biomagnification. However, within the highly exposed species groups, differences in vulnerability may still occur as the product of physiological traits leading to differences in inherent sensitivity (Farmahin et al., 2013; Heard et al., 2017).
A number of studies have attempted to link variations in species anatomical or physiological traits to chemical sensitivity (Baird and Van den Brink, 2007; Rubach et al., 2012, 2010a; Qiu et al., 2014; Gergs et al., 2015; Rico and Van den Brink, 2015; Van den Berg et al., 2019; Dalhoff et al., 2020). Most commonly the traits available and used have been either taxonomic, anatomical (e.g., body size and weight, presence of external respiratory surfaces), life-history (e.g., life-span, reproductive strategy) or ecological (life-stage specific habitat, feeding guild), although Rubach et al. (2012) and Van den Berg et al. (2019) both included measured TK traits and Dalhoff et al. (2020) included TK and TD parameters derived from modeling. Relationships that link both single and also multiple traits to sensitivity have been identified. For example, Gergs et al. (2015) found a relationship between sensitivity and body size, which has been linked to possible effects on TK traits for Triphenyltin hydroxide through a surface area to body mass scaling relationship (Rubach et al., 2010a). Dalhoff et al. (2020) also found a strong correlation between surface area/volume and TK parameters (sorption and uptake rate constants and the resulting BCF) for a pyrethroid insecticide. However, in this case, there was no correlation between any TK parameter and sensitivity. Instead sensitivity was correlated with “killing rate,” which is the major TD parameter derived from the General Unified Theory Reduced Stochastic Death (GUTS-RED-SD) TK-TD model. This finding suggests that species variations at the target site, or associated with different AOP components, may act as the major drivers of arthropod pyrethroid sensitivity rather than any ADME parameter. Despite some progress, not all trait-based assessments have succeeded in identifying links between specific species characteristics and sensitivity. The absence of such simple relationships highlights the complexity of potential predictive model development due to factors such as multiple trait contributions (Qiu et al., 2014), lack of relevant trait data for key intrinsic characteristics (Rubach et al., 2010a; Van den Berg et al., 2019), and the need to better consider TK (and TD) traits within models (Gergs et al., 2015).
Although trends across a wide range of taxa have not been established, more focussed species specific comparisons have identified cases in which TK traits have been shown to contribute to sensitivity difference. Such an example is the exposure of two cryptic species of Capitella to the PAH, fluoranthene (Selck et al., 2003; Li et al., 2004). In this polychaete annelid, higher fluoranthene biotransformation capability was found in Capitella sp. I than in its closely related sibling species Capitella sp. S. (Selck et al., 2003). Between the two polychaetes, Li et al. (2004) identified two novel cytochrome p450s present in Capitella sp. I (CYP331A1 and CYP4AT1), both potentially capable of PAH biotransformation, that were not found in Capitella sp. S. These two enzymes supported a higher rate of fluoranthene biotransformation in Capitella sp. I that could in turn explain the lower sensitivity of the species for fluoranthene compared to Capitella sp. S. (Bach et al., 2005). For lead, Andre et al. (2010) identified differences in the potential for two cryptic species of the earthworm L. rubellus to persist in polluted soils could be linked to species difference in calcium metabolism. Specifically, sequencing of the SERCA Ca-transport gene in the two cryptic clades revealed mutations in the protein cytosolic domain likely to contribute to differences in lead accumulation and internal sequestration, differences expected to influence sensitivity under different soil pH conditions.
A further set of examples of the role of TK trait differences for sensitivity comes from studying the responses of bird species to dioxin exposure. The initial mechanisms of dioxin congener interactions leading to toxicity are comparatively well known. Initially dioxins act as a ligand and bind to the aryl-hydrocarbon receptor (AH-receptor). This results in co-chaperone dissociation and heterodimerization with the AhR nuclear translocator (ARNT) which acts as a transcription factor, most notably for biotransformation enzymes such as cytochrome P450 1a1 (Denison et al., 2011). Gene expression changes resulting from AH-receptor mediated transcriptional activation in birds has been convincingly related to a range of pathologies linked to DNA damage, changes in hormone cycling, teratogenicity, neurotoxicity and carcinogenesis (Mandal, 2005). Binding studies have indicated that ligands with a low inhibitory constant induce cytochrome P450 1A1 expression at lower concentrations and this expression and activity is associated with higher potency for these compounds through the production of reactive metabolites (Hestermann et al., 2000). Based on this mechanism, expectations are that high ligand binding to species specific AH-receptor sequences result in higher cytochrome P450 1A1 expression and activity, that would result in greater toxicity through metabolite production and downstream effects.
The link between AH-receptor sequence variations and dioxin sensitivity has been convincingly illustrated in comparative studies with bird species. Observations that some species of piscivorous birds (e.g., the common tern Sterna hirundo) are highly exposed to 2,3,7,8-tetrachlorodibenzo-p-dioxin (TCDD) and related halogenated compounds but show limited effects have been linked to their lower sensitivity (up to 250-fold) to these chemicals compared to chicken (Gallus gallus), a species commonly used for toxicity testing. The expressed AH-receptor from Sterna hirundo was found to show lower TCDD binding affinity and exhibits a reduced ability to support TCDD-dependent transactivation as compared to chicken AH-receptors (Farmahin et al., 2013). A further analysis of avian AH-receptors revealed particular amino acid sequence polymorphisms in the ligand-binding domain at sites 324 and 380 that were strongly linked to the reduced activation potential (Farmahin et al., 2013). This model of AH-receptor sequence mediated variation in sensitivity was further tested through sequence analysis and in ovo exposure using a luciferase reporter gene assay to measure cytochrome P4501A induction (Farmahin et al., 2013). Although further polymorphisms between species were found for different AH-receptor residues, only those at the two sites identified from the initial study (324 and 380) affected luciferase activation as linked to toxicity (Farmahin et al., 2013). Indications of a relationship between AH-receptor mediated gene expression and dioxin and halogenated organic toxicity in fish species suggests the link between binding domain sequence variation and sensitivity may extend beyond bird species (Doering et al., 2018).
The potential vulnerability of bee species to xenobiotic exposure, as illustrated by the recent cases of neonicotinoid impacts (Rundlof et al., 2015; Woodcock et al., 2017; Main et al., 2020), has been linked to the constrained metabolic potential of bee species for these insecticides. Relative to most other insects, sequenced bee genomes have a deficit of detoxification genes from the Phase I, II, and III gene families (Berenbaum and Johnson, 2015). For example, the honey bee Apis mellifera has 46 identified cytochrome P450 genes and the bumblebee Bombus huntii 44 compared to 52–92 for a range of other insect species (e.g., 85 for Drosophila melanogaster). The reasons for the reduced complement of metabolic enzymes in honey bees is not established. However, it is suggested that detoxification in eusocial bees may be supplemented by behaviors that produce a “social detoxification system.” These include forager discrimination, dilution by pollen mixing, and food processing through microbial fermentation, all of which might lower the number or quantity of ingested chemicals that require detoxification (Berenbaum and Johnson, 2015). The reduced enzymatic potential for biotransformation in bees has been proposed as a key trait that may engender higher sensitivity to some individual xenobiotics (Berenbaum and Johnson, 2015). Such comparative sensitivity of bees compared to other insects is supported by meta-analysis showing the sensitivity for honey bees across commonly tested insecticide classes (Hardstone and Scott, 2010).
The potential role of specific cytochrome P450s in bee sensitivity to xenobiotics has been shown in comparative toxicity studies for the neonicotinoids imidacloprid and thiacloprid for Apis mellifera and Bombus terrestris. Of the two insecticides, thiacloprid has the lowest toxicity to both species. In Apis mellifera, this compound is more rapidly metabolized by the single Cytochrome P450 (CYP9Q3), identified as the major Phase I metabolic enzymes for this class of insecticide (Manjon et al., 2018). Mining of an available Bombus terrestris genome identified five orthologs of Apis mellifera CYP9Q3, of which two (CYP9Q4, CYP9Q5) were found to metabolize thiacloprid, but not imidacloprid (Manjon et al., 2018). This was consistent with a lower toxicity of thiacloprid than imidacloprid in bumblebees. The affinity of the family of cytochrome P450 enzymes for the varoicides tau-fluvalinate and coumaphos has also been linked to the low sensitivity of Apis mellifera to these compounds compared to their mite targets (Mao et al., 2011). Further, recent work has identified functionally conserved metabolic enzymes in the solitary bee Osmia bicornis that play a critical role in the biotransformation of pesticides including neonicotinoids, which in turn determines the sensitivity of this species to exposure (Beadle et al., 2019).
The functional role of TK traits in chlorpyrifos sensitivity was investigated across a range of 15 taxonomically varied freshwater arthropod species (Rubach et al., 2010b). Differences in TK traits influencing both uptake and elimination rates were found. Those TK traits relating to uptake, elimination and bioconcentration were compared to sensitivity (expressed as log EC50). Significant negative relationships were found for the kinetic parameters, but not for bioconcentration factor values. Perhaps most surprising was the significant negative relationship of elimination rate and sensitivity. Expectation may have been that higher biotransformation leading to elimination would be linked to lower sensitivity, as for insecticides and adapted Capitella populations exposed to PAHs (Selck et al., 2003; Bach et al., 2005). However, key to understanding this relationship may be the requirement for chlorpyrifos to be metabolized to chlorpyrifos-oxon, a more potent acetylcholinesterase inhibitor, to initiate toxicity. Hence, species with high metabolism may increase internal chlorpyrifos-oxon levels leading to greater inhibition and toxicity. Separately, uptake and elimination traits accounted for 38 and 28% of the differences in species sensitivity indicating that, although important, the predictive power of such TK trait measurements was not high (Rubach et al., 2012). The limited value of TK traits for sensitivity prediction has also been demonstrated in a study for cypermethrin (Dalhoff et al., 2020), further questioning the importance of their role.
Metallothioneins, other metal binding proteins and small molecular weight metabolites (e.g., phytochelatins and glutathione) can all play a role as determinants of TK traits for metal exposures. The induction of metallothionein and other metal chaperones following exposure has been widely demonstrated (Scott-Fordsmand and Weeks, 1998; Weeks et al., 2004; Amiard et al., 2006), and as discussed above, basal metallothionein expression has also been linked to metal tolerance (Deeds and Klerks, 1999; Costa et al., 2012). Hence, there is expectation that basal metallothionein expression levels and induction potential could be linked to sensitivity (Shaw et al., 2019). Although systematic studies are lacking, small scale studies have highlighted a potential role for metal binding systems in species response to exposure. For example, a role for metal binding proteins to sequester accumulated metals in a quasi-inert fraction has been suggested to play a role in earthworm species sensitivity to cadmium (Fourie et al., 2007). Further, studies in three marine species demonstrated a higher sensitivity to copper for the common limpet (Patella vulgarta) could be explained by lower metallothionein expression as compared to the relatively insensitive shore crab (Carcinus maenas) and blue mussel (Mytilus edulis) (Brown et al., 2004). Differences in the extent of production, oligomer length and granular binding of phytochelatins has also been linked to sensitivity in two algal species (Chlamydomonas reinhardtii and Pseudokirchneriella subcapitata) to cadmium (Lavoie et al., 2009). In contrast to these positive results, studying the promoter region of fish species, Olsson and Kille (1997) found that metallothionein locus induction was not quantitatively linked to sensitivity, indicating that this is not a ubiquitous trend.
A final emerging area of research relevant to understanding the contribution of TK traits to sensitivity is the potential contribution of the host associated microbiome. There is a growing understanding that a multiplicity of interactions linking host organisms to their microbiomes (McFall-Ngai et al., 2013) through consortia involvement in a range of physiological processes (Lee and Hase, 2014). Bacteria in the microbiome have been associated with a number of processes that influence xenobiotic TK fate and exposure for the host. For example, perturbation of the mammalian gut microbiome has been shown to alter the concentrations of inorganic arsenic metabolites present in urine in laboratory studies (Lu et al., 2013). These changes in arsenic speciation in individuals with altered gut microbiomes have been linked to an increased sensitivity to arsenic toxicity (Chi et al., 2019). At present relatively little is known of the role of the gut microbiome in modifying arsenic speciation in natural populations. However, observations of the presence of methylated and organoarsenic forms in a range of aquatic and terrestrial species points to a role of bacteria, perhaps in conjunction with host metabolic capacity, in arsenic handling (Langdon et al., 2002; Rahman et al., 2012; Liebeke et al., 2013).
The host associated microbiome has also been shown to be involved in the degradation of assimilated organic chemicals. There is growing evidence that in some cases, removal of the host microbiome through treatment with antibiotics can change biotransformation, potentially resulting in significant changes in host pesticide susceptibility (Daisley et al., 2018; Fernandez et al., 2019). Bacterial species isolated from the gut of the diamondback moth Plutella xylostella have been shown to degrade a range of xenobiotic chemicals (Ramya et al., 2016), suggesting mechanisms through which species and populations may vary in sensitivity based on their potential to harbor such degradative isolates. Further, atrazine exposure has been found to result in heritable changes in the wasp gut microbiome that result in increased rates of metabolism (Wang G. H. et al., 2020). The potential for populations to develop tolerance to pesticide exposure, has been linked to an increased metabolic capacity of the gut flora, findings further indicating how possession of a specific microbiome may alter susceptibility (Kikuchi et al., 2012; Cheng et al., 2017). This is an emerging area and given the critical role of bacteria species in chemical biotransformation in environmental media, it seems likely that the capacity of species to harbor different symbionts may emerge as key determinants of TK traits (Gressel, 2018). Hence the functioning of the gut microbiome may remain an important area for ecotoxicological research (Evariste et al., 2019).
Toxicodynamic Traits and Species Sensitivity
A second set of physiological components that can contribute to species sensitivity are related to TD traits. As the first critical interface of the chemical with the AOP, the nature and strength of the MIE has the potential to act as a key determinant of a species response to exposure, with the caveat that the receptors involved may not be relevant for all species and cases. For chemicals with non-specific mechanisms of action, toxicity may result from chemical interactions with a range of targets, such as multiple protein thiol binding sites for metals or more generalized membrane interactions for non-polar narcotics. Conversely, for some specifically acting substances (e.g., pesticides, pharmaceuticals) the MIE may be associated with more specific interactions identifiable from knowledge of the substance’s known mechanism of action. These more specific TD interactions may have a greater potential to vary between species.
Non-polar organic chemicals are proposed to act through a narcotic mechanism based on interactions with lipid bilayers leading to changes in the cell membrane or its associated proteins (Escher et al., 2008). Since cell lipid bilayers have a conserved structure, such interactions are potentially generalized across species. The mechanism of action of narcotics through lipid membrane interactions underpins phase partitioning based theories such as the target lipid model. The approach is based on the assumption that toxicity occurs when the chemical concentration in target lipids in biological membranes reach a threshold concentration (Di Toro et al., 2000). A key model assumption is that this concentration is species specific, rather than universal. Traits based studies have established differences in species responses based on lipid content, with low lipid levels linked to higher sensitivity, such as for petroleum hydrocarbons in soil invertebrates (Gainer et al., 2018) and the organophosphate fenitrothion in freshwater phytoplankton species, in which this pesticide likely exhibits narcotic toxicity (Kent and Currie, 1995). However, lipid composition currently provides only a partial explanation for the sensitivity indicated by the range of species-specific critical target lipid concentrations (Di Toro et al., 2000). Further, traits such as the presence of lipid reserves may further complicate such assessments, because these can act as a reservoir for organic chemical accumulation that may reduce membrane concentrations and also provide protection from narcosis. As such, the interactions of the chemical with the other lipid pools may act as a TK mechanism to reduce exposure of the chemical to the membrane lipid that acts as the primary TD site of action for the narcotic effect.
For chemicals such as pesticides and pharmaceuticals that have a known mechanism of action, toxicity results from interactions with a target receptor to elicit downstream responses. For many, but far from all, pesticides, biocides and human and veterinary medicines, the nature of these interactions, at least for the target species (e.g., humans for medical pharmaceuticals, insect pests for insecticides etc.) is often known. As increasing genome resources become available1, it has become possible to screen non-target species for chemical receptor orthologs (Gunnarsson et al., 2008; LaLone et al., 2016; Santos et al., 2017; Verbruggen et al., 2018). The principal is that the toxicity of chemicals will be greater in those species possessing a receptor ortholog relevant to the mechanism of action compared to those species that do not. This provides a concept of response equivalence in different species resulting from a common receptor compliment that can be used for designing monitoring and testing programs based on in vitro methods for hazard identification (Bowes et al., 2012; LaLone et al., 2018; Blackwell et al., 2019).
For human pharmaceuticals, the taxonomic conservation of receptor orthologs in species has been explicitly linked to relative sensitivity. For example, for the statin drug target HMG-CoA reductase, orthologs were predicted in almost all eukaryotic species, archaea, and in certain true bacteria, consistent with inhibitory effects of statins on fungal species growth and on HMG-CoA reductase activity across different plant and invertebrate species (see Gunnarsson et al., 2008). For the sodium dependent serotonin transporter, orthologs are found in vertebrate and arthropod species consistent with specific responses of zebrafish, Drosophila and a flatworm to exposure to serotonin reuptake inhibitors, such as fluoxetine, that target this receptor (Gunnarsson et al., 2008). This contrasts with the apparent insensitivity of plants and bacteria to such chemicals, in which orthologs of this receptor are missing (see Gunnarsson et al., 2008).
The potential application of the ortholog approach can be extended beyond human pharmaceuticals to chemicals such as pesticides and biocides that also have known mechanisms of action targeting specific receptors. As an example, it has been shown that the nematode C. elegans has low sensitivity to pyrethroid insecticides (Svendsen et al., 2010). Analysis for this species indicates that, despite the presence of most major ion channel families, there are no predicted voltage-activated sodium channel orthologs (the known target for pyrethroids) in the C. elegans genome (Bargmann, 1998). Going beyond ortholog presence, the analysis of sequence can also highlight potential drivers of sensitivity. For example within species, comparative analysis of resistant strains can highlight key mutational changes in receptors leading to differences in population sensitivity increasing our knowledge about traits underlying differential sensitivity for between species sensitivity prediction.
As examples of ortholog and sequence analyses, sensitivity to organotin as an endocrine disruptor has be linked to the emergence of multiple peroxisome proliferation activation receptors (PPAR) orthologs early in the invertebrate lineage (named PPAR α, β, and γ). Specifically, the duplicated PPAR genes coincide with the acquisition of TBT sensitivity. In contrast, the single unduplicated PPAR copy in the invertebrate chordate amphioxus is irresponsive to TBT. This simple picture is slightly complicated by TBT insensitivity in some teleosts, but this is thought to be a consequence of ligand binding pocket mutations in specific species (Capitao et al., 2018). Similarly, comparative assessment of the potential for aromatase inhibition related to sequence variations has also been used to identify the range of potential sensitivities of fish species to endocrine disrupting chemicals (Doering et al., 2019a,b). Changes to the ligand binding domain of the nAChR have also been convincingly associated with altered sensitivity for species to neonicotinoid exposure. Specifically, homology modeling suggests that replacement of an arginine (associated with high binding) in a critical region of the ligand binding domain in various tick species may contribute to their lower imidacloprid sensitivity (Erdmanis et al., 2012). Furthermore, mutation of the equivalent arginine to threonine (associated with lower binding) is thought to underlie increased neonicotinoid resistance in a strain of the aphid Myzus persicae (Bass et al., 2011).
A complexity that may arise when using the presence of gene family representatives as simple determinants of sensitivity include cases where orthologs retain sequence homology but have evolved divergent roles outside the target function or tissue. As an example, earthworms have comparatively lower sensitivities to the organophosphate chlorpyrifos (Ma and Bodt, 1993) than soil arthropod species such as springtails (Broerse and van Gestel, 2010; Jegede et al., 2017; Kamoun et al., 2018). Earthworms do not lack the target acetylcholinesterases in the nervous system. Indeed, there is evidence that the gene family has expanded, with multiple orthologs present that show ligand binding domains conserved with those in susceptible insects. However, the chlorpyrifos-oxon binds and inhibits the activity of a range of esterases, including other cholinesterases (ChEs) and carboxylesterases (CbEs). Tissue specific analysis of earthworms has shown high ChE and CbE activity in a range of non-neural tissues, with notably high ChE and CbE activities in the pharynx and gut, respectively (Vejares et al., 2010). The activity of these non-neural CbE/ChEs are inhibited by organophosphates indicating their capacity to bind the chlorpyrifos-oxon (Sanchez-Hernandez, 2010; Vejares et al., 2010; Sanchez-Hernandez et al., 2018). This has led to the suggestion that the binding of the chlorpyrifos-oxon by these gut CbEs may prevent the molecule interacting with neural targets (Sanchez-Hernandez, 2010; Vejares et al., 2010). In this way, the gut system may act as a buffer against nervous system exposure, leading to lower sensitivity. As these non-neural receptors are thought to influence the extent of chlorpyrifos-oxon reaching the critical neural target, they could technically be classified as a TK, rather than a TD, trait. It is also worth noting that although the toxicity associated with non-neural CbE/ChE inhibition is lower than that associated with the neuronal receptor, inhibition of non-neural receptors still has non-trivial effects on the function of these non-neural tissues (Sanchez-Hernandez et al., 2018). Such characteristics mean that these gut esterase enzymes may bind chlorpyrifos and in doing so prevent the molecule interacting with neural targets. In this way, the gut system may act as a buffer against nervous system exposure, leading to lower sensitivity. Ultimately, through such mechanisms, what may be identified as a receptor involved in TD process, may also take a role in TK processes linked to chemical distribution that affects concentration at true target sites.
The expression of apparent receptors outside of the target tissue providing protection through binding the chemical away from primary target sites, as highlighted above for organophosphates in earthworms, may also have mirrors among other toxicants. Studies have shown clear differences in the sensitivity to neonicotinoids of relatively susceptible insects and tolerant arachnids. Although both these taxa possess the classical nicotinic acetylcholine receptor (nAChR) target, these two taxa have differences in off-target receptors, specifically the presence of acetylcholine binding proteins (AChBPs) in arachnids (Torkkeli et al., 2015; Bao et al., 2017). Unlike nAChRs, AChBPs are truncated and comprise the ligand binding domain but not the transmembrane domain of the target (Shahsavar et al., 2016) and have been found in molluscs (Banks et al., 2009; Saur et al., 2012), polychaetes (McCormack et al., 2010) and earthworms (Short et al., unpublished). AChBPs have been shown to bind neonicotinoid insecticides in vitro (Tomizawa et al., 2007) and could act as additional receptors for neonicotinoid binding at locations away from the critical synaptic nAChRs, thereby alleviating the toxic effects of neonicotinoid insecticides. Indeed, Short et al. (unpublished) have suggested the presence of specific amino acids in the AChBP ligand binding domains expressed by different earthworm species are linked to altered magnitudes of off-target stoichiometric binding and may underlie observed differences in sensitivity. Off-target binding has also been proposed as a mechanism to explain the development of resistance to pyrethroids in malarial mosquitos via increased expression of sensory appendage protein SAP2 (Ingham et al., 2020). These common findings suggest that the degree of off-target binding may make an important, but currently poorly understood, contribution to species sensitivity differences.
Using Our Knowledge to Move Beyond Description to Sensitivity Prediction
As knowledge has developed on the range of drivers that underlie species sensitivity, there has been an increasing interest in the development of predictive approaches. Critical to this has been both the expansion of the available ecotoxicological databases from which to identify differences and also the development of the AOP concept (Ankley et al., 2010). The assembly of the ecotoxicological data into searchable databases provides researchers the opportunity to look at sensitivity relationships between closely and distantly related species to develop evolutionary and correlative models. The AOP concept provides a structure within which to assess how the degree of conservation or divergence of species traits or toxicologically relevant biochemical pathways contribute to sensitivity (Rivetti et al., 2020). This includes through trait-based approaches and the further development of the ortholog concept by assessment of the gain or loss of molecular targets (Gunnarsson et al., 2008; Verbruggen et al., 2018) and their structural or functional variations (Celander et al., 2011; LaLone et al., 2013). These developments have supported the growth of two dominant types of approach for sensitivity prediction designed to address the current challenges for chemical risk assessment, challenges that arise from the need to use short-term bioassay data for a few species to predict long-term chemical risks for complex communities. The first type of approach is based primarily on the phylogenetic comparison of species and the use of this information to predict sensitivity. Methods following this approach include phylogenetic model development, interspecies correlation estimation (ICE) models for species pairs and trait-based predictions. The second major type of approach is based on the development of target ortholog assessments often based on the use of genomic, transcriptomic and/or metabolomic data. Approaches of this type include metabolic pathway assessments for TK analysis, receptor ortholog cataloging, receptor expression and functional determination and target receptor binding prediction analysis. The methods applicable within each of these approaches are described below.
Phylogenetic and Species Correlations Approaches for Predictive Ecotoxicology
As highlighted above, the genes and gene pathways contributing to TK (e.g., detoxification) traits, such as monooxygenases, glutathione-s-transferases, metallothioneins and phytochelatin synthases, and TD (AOP) traits, such as target receptors and repair mechanisms are commonly observed to underlie differential sensitivity both within and between species. Hence, knowledge of the evolution of these gene families can contribute to an understanding of species responses. Phylogenetic analyses conducted on genes associated with both TK and TD traits have identified taxon relevant phylogenetic structure. Seren et al. (2014) analyzed the metallothionein sequences available for vertebrate species. Distinct major metallothionein clades were found within the assembled sequence trees for fish, eutherians, amphibian/reptile/bird and amniotes, indicating that functional divergence and duplication has created a distinct metallothionein in the vertebrate lineage. A phylogenetic analysis of microorganisms, yeasts, algae and plant phytochelatins also indicated that plant variants had notably diverged from those of other organisms (Kolahi et al., 2018). For TD linked receptor targets, phylogenetic structure linked to taxonomy has been shown for acetylcholinesterase both across phyla (e.g., chordates arthropods and nematodes) and within phyla (e.g., between arthropods) (Bally et al., 2016). For the latter group, higher sequence similarity was found for ortholog sequences in more closely related species for each of the two known acetylcholinesterase genes (Kaur et al., 2015). The presence of a clear phylogenetic structure for such key genes increases the likely success of sensitivity predictions made using homology assessment.
The phylogenetic structure observed for genes in TK and TD pathways points to the likelihood of similar sensitivity in related species that share closely related metabolic systems and target structures. This phylogenetic signature for toxicity has been investigated in a small number of studies. For endosulfan, Jones et al. (2009) found up to 100-fold differences in the sensitivities of nine amphibian species. Within this variation a phylogenetic signature was observed, with the Bufonidae being least susceptible, Hylidae moderately susceptible, and Ranidae most susceptible. A phylogenetic signature of amphibian sensitivity has also been found for copper after the co-varying effects of exposure temperature were accounted for (Chiari et al., 2015). Fish species sensitivity to nitrite has also been shown to have a phylogenetic basis, specifically through the taxon specific distribution of chloride channels through which NO2– can be actively taken up. Thus, species that actively assimilated Cl– via gills, such as the channel catfish (Ictalurus punctatus), showed greater sensitive to NO2– than species such as killifish (Fundulus heteroclitus), European eel (Anguilla anguilla) and bluegill (Lepomis macrochirus) that access Cl– also through other (e.g., dietary) mechanisms (Brady et al., 2017). Further studies have also provided some support for the presence of a strong phylogenetic signature for some chemical classes, such as organophosphates for fish and other vertebrate species (Hylton et al., 2018; Moore et al., 2020), but only a weak signature for other classes such as organochlorines and metals (Hylton et al., 2018). In a study of energetic compound effects, the overlap of transcriptional network response showed a degree of conservation, but showed greater divergence in increasingly taxonomically distant species (Garcia-Reyero et al., 2011). This again indicates a phylogenetic basis in species responses to these chemical classes.
Building on the concept of phylogenetic conservation of critical pathways, Guenard et al. (2011) developed a predictive modeling approach that uses phylogeny to estimate sensitivity. These approaches use known molecular phylogenies to derive estimates of the taxonomic distance between species expressed as path length to the first common ancestor. This phylogenetic information was then regressed against known species sensitivities to individual pesticides in relation to overall chemical potency and species susceptibility to derive a predictive model. Within the analysis of Guenard et al. (2011), phylogeny explained up to 61–85% of among-species variations, with up to 83% of between species variation being phylogenetically structured. The high level of phylogenetic structure indicates a clear potential for the phylogenetic model to provide justifiable predictions of sensitivity for untested species (Guenard et al., 2014). Using the same approach, Malaj et al. (2016) developed models to explain species sensitivity for eight metals using combined phylogenetic and metal physicochemical properties. The models explained 70–80% of the variability in species sensitivity, with phylogeny, explaining >40% of variance, as the most important component.
The phylogenetic relationship between TK and TD pathway components, coupled with the potential for phylogeny alone to explain a significant proportion of sensitivity, indicates that species’ responses to chemical stress are not random. Instead, sensitivity can be viewed as a trait founded in the evolutionary relationships between species. As quantitative characteristics are partly explainable in terms of species evolution, there is potential to use chemical sensitivity data as an additional set of traits for the construction of species phylogenies and to compare and contrast physiologies in closely related or cryptic species. The potential to use chemical data to establish phylogenies can be demonstrated using effect data for multiple species and chemicals. For example, Baird and Van den Brink (2007) assessed the relationship of chemical sensitivity to a range of species morphology, life history, physiology and feeding ecology traits. Four traits explained 71% of species sensitivity variation. Using the same data matrix as input for phylogenetic assessment, a tree structure was found that closely mirror the conventionally understood taxonomic relationships between the tested species. Thus, species that are closely related, such as the three cladoceran species (Daphnia cucullata, Daphnia pulex, Daphnia magna) are closely grouped indicating a high similarity in their responses to the 15 chemicals tested. This is also the case for the two crustacean species (Gammarus pulex, Asellus aquaticus). The seven insect species included show a lower degree of relatedness based on sensitivity to the 15 chemicals, reflecting their lower taxonomic association to class level only (Figure 3). The capacity for reconstructing phylogenies based on toxicity information provides further evidence of the phylogenetic conservation of toxicity mediated through common pathways.
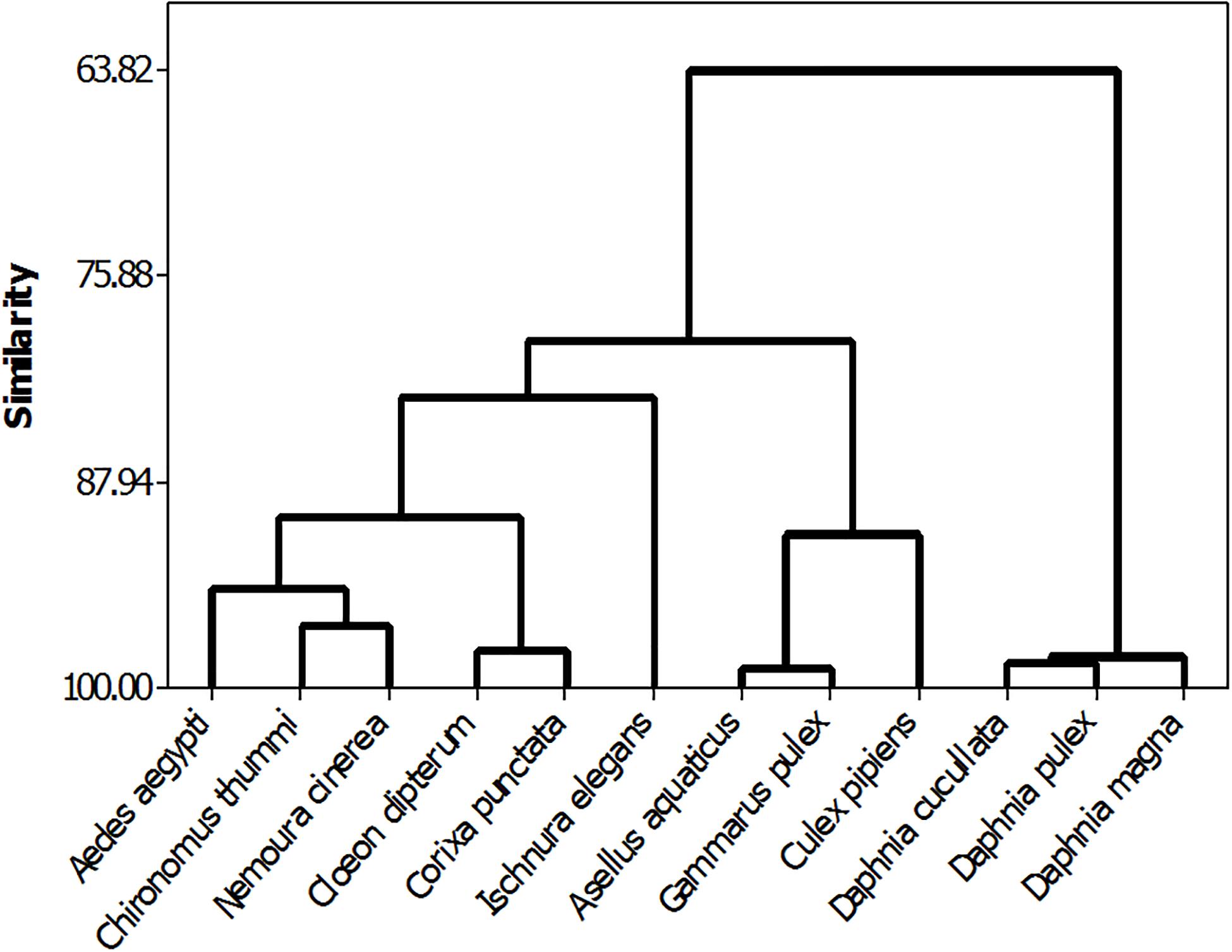
Figure 3. Phylogeny of aquatic invertebrate species reconstructed from the set of toxicity data for 15 chemicals assembled by Baird and Van Den Brink (Baird and Van den Brink, 2007), note the resulting phylogeny closely reflects the conventionally assumed taxonomic relationship between the different species.
The phylogenetic signature for toxicological responses has been used to justify the development of predictive correlative models designed to fill gaps in our knowledge of species sensitivity. The most commonly developed and widely used of such approaches are ICE models. These methods use the available toxicity data (e.g., as ECx or LCx values) for chemicals tested in two species to generate a log-linear least-squares regression-based ICE model specific to that species pair. Using species pair ICE models, toxicity measured for further chemicals in just a single taxon can be used to predict toxicity for the same chemical in the second untested taxon (Leblanc, 1984; Raimondo et al., 2010).
Theoretical ICE models can be developed for any species/taxa pair for which there is sufficient toxicological data available either across all chemicals or for a specific chemical class (Bejarano, 2019; Raimondo and Barron, 2020; Wang X. N. et al., 2020). In a quantitative comparison of 780 ICE models derived for 550 chemicals in 77 aquatic species, predictive power was found to be strongly governed by relatedness (Raimondo et al., 2010). For models derived from data for two species related at family level, ICE predicted sensitivities were within a factor of 5 of the measured value in 94% of cases and only 1% of predictions differed >50-fold from measured values. In contrast, for species pairs from different phyla, only 61% of model predictions were within a fivefold factor and 8% differed >50-fold (Raimondo et al., 2010). On the basis of their high predictive power, especially for closely related species pairs, ICE models have been proposed for the generation of in silico toxicity data for untested chemicals for SSD derivation in closely related species (Awkerman et al., 2014; Bejarano et al., 2017), for predicting hazards to untested endangered species (Willming et al., 2016) and for use in QSAR development (Raimondo and Barron, 2020).
Even though there is a clear signature of phylogenetic conservation, a close relationship alone cannot be taken as an absolute guarantee of similar sensitivities. For example, in an analysis of ICE models, Raimondo et al. (2010) found that species related at genus level still differed in sensitivity by >fivefold in 6% of cases and >10-fold in 4%. Further, studies of closely related “sibling” species, including known cases of species crypsis have reported different responses following exposure, including cases for copepods exposed to trace metals (Rocha-Olivares et al., 2004); different crustacean species exposed to nickel, a fungicide and an insecticide (Feckler et al., 2012); nematodes exposed to copper and lead (Monteiro et al., 2018); aquatic oligochaetes exposed to cadmium (Sturmbauer et al., 1999) cadmium, chromium and copper (Reynoldson et al., 1996); terrestrial oligochaetes to lead (Andre et al., 2010) and polychaetes exposed to different PAHs (Linke-Gamenick et al., 2000; Bach et al., 2005).
Trait-Based and Ortholog Approaches for Predictive Ecotoxicology
As sensitivity data for many species, including rare or difficult to keep organisms is challenging to obtain, trait-based and ortholog approaches have been proposed as a means to allow predictive assessment (Baird and Van den Brink, 2007). Traits potentially relevant for sensitivity prediction may occur at multiple levels of biological organization within the AOP, from the nature and dynamics of the energy budget to life-cycle characteristics and species-species interactions. Within any proposed trait-based approach, an obvious consideration is the nature of the traits to include for predictive assessment. Within the literature, a dichotomy has largely existed between mechanistic trait assessments based on AOPs and approaches that use higher tier physiological or ecological characteristics. For initial AOP development, the dominant paradigm is based on a bottom up approach centered on identifying the AOPs from gene regulation networks, often using high dimensional gene, protein or metabolite expression data (Perkins et al., 2019). This approach is established in human toxicology (Kimber et al., 2014; Abdul Hameed et al., 2019) and is transferable to ecotoxicological cases (Murphy et al., 2018; Gomes et al., 2019; Kim et al., 2020; Song et al., 2020). In contrast, ecological trait-based approaches are based on building correlative models that link physiological features or ecological habit (e.g., size, respiratory anatomy, trophic level, diet, reproductive strategy, habitat preference, generalized metabolic traits) (Baas et al., 2015; Berger et al., 2018; Van den Berg et al., 2019). Such approaches underpin techniques such as Species at Risk (SPEAR) assessment. This predictive model framework uses trait information on (i) sensitivity (e.g., to insecticidal compounds), (ii) generation time, (iii) presence of aquatic stages, and (iv) ability to migrate and recolonize; to provide and index of the severity of chemical exposure effects (Liess and Von der Ohe, 2005).
Full conceptual AOP development can provide a framework for sensitivity assessment that brings together the mechanistic approach within a wider trait concept. Such a merger can occur when the critical driver of toxicity is identified; the variation for these key processes quantified and their implications for sensitivity defined. This is not a simple process because at each stage of the AOP there is the potential for interspecific variation. Within the AOP, a particularly critical interaction is that between the chemical and its primary target that acts as the MIE. Differences in the nature and strength of this interaction has the potential to radically change sensitivity in a manner that is easier to conceptualize and which is likely to be more specific, and hence variable, than that for more downstream responses such as tissue, organ physiological, behavioral or life-cycle changes. Hence, to understand and predict sensitivity there has been a specific focus on critical receptors that represent MIEs. Such assessments have used an ortholog approach that covers both the receptor ortholog complement and sequence variation. Linkage of TK/TD approaches with resource allocation models have potential for understanding the development of ecotoxicological responses across multiple species (Ashauer and Jager, 2018; Baas et al., 2018).
The receptor complement hypothesis of sensitivity in ecotoxicology was initially proposed by Gunnarsson et al. (2008), who analyzed the sequenced genomes of 16 species for 1,318 human drug targets and linked ortholog complements to comparative species sensitivity. The work of Gunnarsson et al. (2008) has been expanded to cover a receptor analysis for 640 eukaryotic genomes (Verbruggen et al., 2018). These analyses have been assembled into the “ECOdrug” database which details the connection of orthologs to phylogeny for 1,194 active pharmaceuticals targeting 663 drug targets. The case studies presented by Verbruggen et al. (2018) highlight a range of potential predictive applications for ECOdrug. Examples include the fore selection of appropriate test species, such as for testing protein pump inhibitors in fish species based on the presence of the H+/K+-ATPase ortholog target in some, but not all teleosts (Castro et al., 2014); the selection of higher plants rather that algae for assessing the effects of cholesterol lowering drugs (e.g., statins), due to the presence of 3-hydroxy-3-methylglutaryl Coenzyme A Reductase (Verbruggen et al., 2018); and the identification of relevant model species for testing toxicity based on the presence of thyroid hormone receptors in some invertebrate species, but not in Daphnia the most commonly tested model species (Verbruggen et al., 2018).
Further building on the concept of known target conservation as an indicator of sensitivity, LaLone et al. (2016) developed the web-based Sequence Alignment to Predict Across Species Susceptibility tool (SeqAPASS)2. SeqAPASS provides a platform for assessing not just ortholog presence, but also gene structure against the known target to identify similarities. SeqAPASS analysis can be conducted at three levels to identify orthologs and compare their overall sequence similarity at the amino acid level between a species with a known sensitivity and a species under investigation. Thus, beyond ortholog identification, gene structures can be compared to identify homology in key motifs in ligand-binding domains or for individual amino acid residues at important positions for protein conformation and/or ligand-receptor interactions.
LaLone et al. (2016) used SeqAPASS analysis to investigate the differences in insect species responses to molt accelerating diacylhydrazines. Ortholog analysis showed the presence of target receptor orthologs of the ecdysteroid receptor with conserved ligand binding domains for insects and a range of other taxa, such as Merostomata, Malacostraca, Arachnida, Branchiopoda, Chilopoda, Maxillopoda, and Priapulidae. Ligand binding studies with the natural steroid ligand of the ecdysteroid receptor in the Lepidopteran Heliothis virescens indicated that sensitivity was strongly associated with the presence of a valine at residue 384. This amino acid was conserved among lepidopteran species which show sensitivity to this class of insecticide. Ecdysteroid receptor sequences in Hemiptera insensitive to diacylhydrazines were found to possess an isoleucine at residue 55 that prevents ligand interactions through steric hindrance. Furthermore, a lysine at 48, phenylalanine at 222, and glutamine at 227 also restricted ligand-receptor interactions in the Hemiptera (Amor et al., 2012). Hence the sensitivity of these two insect groups to diacylhydrazines could be explained by amino acid differences at key binding residues, rather than by the presence or absence of the ortholog alone.
In a further example of the use of SeqAPASS, LaLone et al. (2016) focused on differences for neonicotinoids among arthropod groups showing high (insects including honey bees) and low (arachnids including ticks) sensitivity. This example was more difficult to fully resolve than the ecdysteroid receptor case. As such, this assessment highlighted some advantages and limitations of the ortholog and homology approach. The synaptic nAChR target of neonicotinoids is known to comprise of multiple α- and β-subunits. Between these two types, differences in the percent similarity of all sequences from insects to arachnids for the β1 subunit provided a possible explanation for sensitivity. However, this observation alone may not be enough to resolve all aspects. The nicotinic acetylcholine receptor family is large, comprising multiple α- and β-subunit sequences (Jones et al., 2006; Jones and Sattelle, 2010). This evolutionary divergence across nAChR subunit gene families makes it difficult to resolve functional homology (Pedersen et al., 2019). Further, the total numbers of α- and β-subunit gene orthologs can vary significantly from lowers numbers in some taxa (e.g., 10 D. melanogaster, 11 A. mellifera, 16 human, 29 C. elegans) to a greatly expanded number (>100) in some Lophotrochozoan taxa (Jiao et al., 2019; Short et al., unpublished). Cases of multi-subunit receptors, in which subunits are encoded by large and divergent gene families, makes it difficult to identify which of the subunit orthologs should be analyzed for critical motif modifications and amino acid substitutions, a challenge that does not exist in cases where there is a single receptor target encoded by a single gene (LaLone et al., 2013).
Further limitations arise for ortholog (Verbruggen et al., 2018) and ligand binding analysis (LaLone et al., 2017) in cases where gene family expansion allows the functional divergence of members. Gunnarsson et al. (2008) already identified that “the presence of a drug target ortholog in a species does not guarantee that a functional interaction with the drug can occur” and that “Vice versa, functional interactions between a drug and other non-orthologous proteins are also possible.” The example given was of the molluscan estrogen receptors that, while expressed in reproductive tissues (e.g., in oyster Saccostrea glomerate), has been shown not to bind estrogen in vitro (Tran et al., 2016). The examples above detailing expression of the cholinesterase and carboxylesterase targets for organophosphates in non-neural earthworm tissues and of AChBPs that interact with neonicotinoids via their ligand binding domain, but possess no ion channel functionality, provide comparable examples. In such cases, ortholog analysis would identify an orthologous gene and sequence analysis a functional ligand binding domain containing the critical motifs and residues. However, any ligand-receptor interaction will take place spatially removed from the target site (e.g., at the synapse in the case of AChEs and nAChRs). This spatial separation means that any downstream links in the neurotoxic AOP will not be initiated. Therefore, there remains a need for the further development of approaches to include not just the presence and homology of orthologs, but also evidence that downstream AOP linkage will occur, through methods such as tissue specific expression analysis and target function assessments. Inclusion of these additional aspects would advance predictive sequence based approaches to their range of application and predictive capability.
Applications of Predictive Ecotoxicology in Chemical Research and Management
The current and future development of approaches for sensitivity assessment and prediction can support a number of emerging applications in theoretical and applied ecotoxicological research. These range from the refinement of modeling approaches to the development of alternative animal models, species orientated assessment and structure testing and assessment programs.
Integrating Mechanistic Insights Into TK and TD Models
The recognized importance of TK and TD in toxicity have underpinned the development of models to capture these aspects of internal exposure and accrued damage (Ashauer and Escher, 2010; Jager and Ashauer, 2018). By linking the TK and TD characteristics to resource allocation, it is possible to link exposure and damage to changes in energy fluxes (Kooijman, 1993).
Chemical exposure at target sites in TK-TD models for invertebrates are most commonly predicted using one compartment TK models (Jager and Ashauer, 2018), while for more physiologically complex organisms there is the potential to develop more complex TK models that include physiological based pharmacokinetic process that integrate a more complete set of physiological processes into a more integrated exposure modeling framework (Stadnicka et al., 2012; Brinkmann et al., 2016; Grech et al., 2017). These TK models can be coupled to physiological effect models to develop coupled TK-TD models, including those linked to dynamic energy budget approaches (Cropp et al., 2014). To provide insights into internal chemical fate, the characterization of genes critical to xenobiotic metabolism, including the phase I, II, and III pathways and metal binding protein pathways, all have the potential to ascribe physiological traits relating to kinetic rates to improve the basis for TK modeling.
The TD component of dynamic energy budget based models link internal exposure to effects on vital rates through five putative energetic or damage associated mechanisms, termed “physiological modes of action” (Kooijman and Bedaux, 1996; Alda Alvarez et al., 2006; Billoir et al., 2008; Swain et al., 2010; Ashauer and Jager, 2018). These five are: (1) Assimilation from food; (2) somatic and maturity maintenance; (3) costs for structure and maturation; (4) overhead costs for making an egg; and (5) Hazard during oogenesis (Jager and Zimmer, 2012; Ashauer and Jager, 2018). Measurement of traits relating to energy production (metabolic rate, mitochondrial activity, fatty acid metabolism, insulin signaling), cellular maintenance (protein synthesis and turnover, nervous system function), developmental change and reproduction (e.g., nuclear hormone receptor expression and cell death (e.g., caspases) have the potential to provide empirical support for these physiological impacts.
The development of quantitative relationships between physiological changes, TK and TD and parameters and sensitivity has the potential to support the development of more mechanistically informed models that can better ascribe sensitivity to key traits. For example, Baas et al. (2015) linked somatic maintenance, as a measure of metabolic rate, to the sensitivity of species to four pesticides, two requiring metabolic activation (chlorpyrifos and malathion), and two not (carbofuran and carbaryl). High sensitivity among smaller species was not linked simply to body size, but rather to the presence of high somatic maintenance costs in species linked to waste-to-hurry strategies under boom and bust population dynamics. This observation predicts high resource allocation to tissue cellular systems, measurable as a mechanistic trait that can be used to assess their contribution to sensitivity.
Predictive Ecotoxicology for the 3 Rs
As programs progress providing large-scale sequencing of animal, plant and microbial genomes, the potential to identify orthologs critical to sensitivity within and among species will increase. This growing availability of genome and other ‘omics (transcriptomics, proteomics, and metabolomics) data, when combined with other trait information, can support cross species assessment. Within toxicology there are significant ethical and regulatory drivers for the replacement of higher organisms as the main focus for testing. In the development of 3 Rs (Replacement, Reduction, Refinement) approaches, functional ’omics techniques have already shown their potential value in screening for specific pathway effects (Laroche et al., 2018). Given knowledge of TK characteristics and an AOP, an assessment of phylogenetic conservation through comparative genomics could be used to evaluate the appropriateness of potential surrogates for use in assessing the effects of specifically acting chemicals targeting this pathway. Assessment of TK pathways can be used to determine effect development and dose specific responses (Creton et al., 2012). Non-targeted metabolomics analysis can be used to support the assessment and development of rapid biochemical profiles that can provide evidence of species equivalence, while orthogonal omics datasets from different species can be used to identify components critical for conserved responses. In this manner it is possible to investigate the downstream response resulting from specific exposures in a replacement species, or even screen the potency of chemical effects through the specified AOP mechanism without the need to always proceed to higher vertebrate testing.
Endangered Species Protection
Just as there is potential to use mechanistic TK-TD information in predictive ecotoxicology for 3 Rs species replacement, it is also possible to use such information to predict risks for species of high conservation concern that cannot be directly tested (Ortiz-Santaliestra et al., 2018). For example in a given exposure scenario, resources may be better spent managing releases to water to protect a piscivorous bird possessing an AH-receptor with predicted high TCDD binding affinity, than on mitigating releases to land to protect insectivorous/vermivorous species possessing a AH-receptor with a predicted low TCDD affinity. For chemicals where bioaccumulation is a potential concern, knowledge of the specific capacity of metabolism or handling of different chemicals may help to identify trophic links within food chains that may lead to elevated exposure of predators. For example, earthworms are well known to accumulate cadmium due to the formation of detoxification granules (cadmosomes) (Stürzenbaum et al., 2004) and in doing so provide a route of exposure for worm eating species (Schipper et al., 2012). Past decisions on action for endangered species protection have been driven by observation of wide population declines (Newton and Wyllie, 1992; Woodcock et al., 2016). Combining analysis of the metabolic capacity of species and receptor orthologs (e.g., nAChRs and voltage-gated sodium channels in the case of neonicotinoids and pyrethroids) and competing off-target receptors (e.g., AChBPs and SAP2) distribution among species from “omic” analyses, with targeted biochemical pathway measurements has the potential to accurately predict the sensitivities among species. Such information can be used to identify potentially vulnerable species that can become a focus for future monitoring.
Risk Assessment—Better SSDs
While a goal may be to move toward a more predictive approach, for some time there is likely to remain species and chemicals for which reliable bottom up predictions of sensitivity will not be readily achievable. Cases where sensitivity can be linked to a few traits will be easiest to resolve. However, for species with multiple potential targets, full resolution to explain sensitivity may prove a daunting or even impossible task (LaLone et al., 2013). Protection of ecological communities is, therefore, likely to retain a statistical extrapolation component based on the use of species sensitivity distributions. Even though statistical models are likely to be retained as a key tool for ecological risk assessment, there is still the possibility to utilize mechanistic information to better inform such assessments. For example, it may be possible to evaluate the proportion of species present in an SSD that possesses one or more traits (e.g., absence of relevant detoxification pathway or presence of a receptor ortholog) linked to sensitivity. Soil SSDs are often dominated by earthworms. This is the case because Eisenia fetida is the main standard soil test species, but its substitution by other species is readily possible meaning that results are often available for multiple earthworm species (Ma and Bodt, 1993; Hartnik et al., 2008; Kreutzweiser et al., 2008; Römbke et al., 2017; Silva et al., 2017). Similarly, cladocerans are often over represented in aquatic SSDs based on the common use of Daphnia magna and related species for testing. Taxonomic biases may cause issues if the dominant species shows particular sensitivity or insensitivity. For example; earthworms are highly sensitive to copper exposure (Lofts et al., 2005) and cladocerans insensitive to neonicotinoids (Raby et al., 2018). Hence distributions for these chemicals, that contain high frequencies of data from these commonly tested taxa are often erroneous, representing a greater sensitivity for copper and a lower sensitivity for neonicotinoids, respectively.
The issue of sensitive and insensitive species representation in SSDs has been identified as an issue for chemicals such herbicides, fungicides and insecticides that are designed to target a specific taxon-relevant biochemical pathway. Thus, if a HC5 is derived from a taxonomically generalized SSD for these chemicals, then the species that fall in the 5% of the affected fraction would be overwhelmingly drawn from the known target species for these chemical classes (e.g., plants for herbicides etc.). Environmental quality criteria set for such chemicals would, as a result, likely not protect against ecosystem level effects, since all impacted species will be drawn from a specific taxa, especially if that group makes a major contribution to key ecosystem processes. The derivation of separate SSDs for sensitive target and non-target groups can protect against the risk of inadequate protection. Extending beyond such simple taxa based segregation, it is possible to envisage ecological, TK-TD or specific molecular traits used to segregate the tested species cohort to allow separate assessments for vulnerable groups. For example, an SSD may be derived only for species likely to bioaccumulate a chemical, that possess a receptor ortholog with the relevant ligand binding domain, or which lack competing off-target receptors for the chemical ligand that may play a protective role.
Mixture Toxicology and Sensitivity
In the field, organisms are almost always exposed to measureable levels of more than one chemical. The mixture effects for the combinations of similarly and dissimilarly acting chemicals are classically explained by the models of concentrations addition and independent action (Altenburger et al., 2000; Backhaus et al., 2000; Faust et al., 2001, 2003). However, although applicable to many chemical combinations, not all mixture studies show additive effects. Indeed, patterns of response indicating synergism (greater than additive) or antagonism (less than additive) may occur in a small, but nonetheless significant proportion of cases (Jonker et al., 2005; Cedergreen, 2014). The role of mechanistic toxicology in identifying which additive mixture model to apply, based on comparing chemical mode of action, has already been highlighted (Heijne et al., 2005; Vandenbrouck et al., 2010). However, an evolutionary perspective of the traits underlying sensitivity could also play a role. For example, it has been demonstrated in a number of species that pyrethroid insecticides can be synergized on simultaneous exposure with azole fungicides (Johnson et al., 2013; Cedergreen, 2014). Similar evidence is also emerging for azole fungicides when exposed with other groups of insecticides such as the neonicotinoids (Robinson et al., 2017; Tsvetkov et al., 2017). Mechanisms leading to synergy may occur at different stages of intoxication (Spurgeon et al., 2010; Figure 4). One chemical may impact on soil or gut microbiomes resulting in changes in the degradation rates of a second (Cheng et al., 2017) or one may affect species activity or feeding altering another’s bioavailability (Cedergreen et al., 2017). A major known TK based mechanism for synergism arises when chemicals alter Phase I, II, or III xenometabolism (Cedergreen, 2014; Figure 4). For example, changes to pesticide biotransformation are implicated as the cause of the synergism of insecticides by azoles. This occurs as the fungicide which targets CYP51 (lanosterol 14α-demethylase) in the target taxa, also inhibits other cytochrome P450s in other species, leading to reduced insecticide metabolism resulting in higher internal concentration and, hence, greater toxicity (Chen and Ying, 2015). There is potential to apply TK models to assess mixture effects for similar and dissimilar mixture combinations (Timchalk and Poet, 2008; Tebby et al., 2019). However, the striking lack of knowledge of xenometabolism in invertebrates, especially beyond Phase I enzymes to the Phase II (e.g., glutathione-s-transferases) and Phase III (e.g., p-glycoproteins) systems means there could be numerous unknown targets for such interactions.
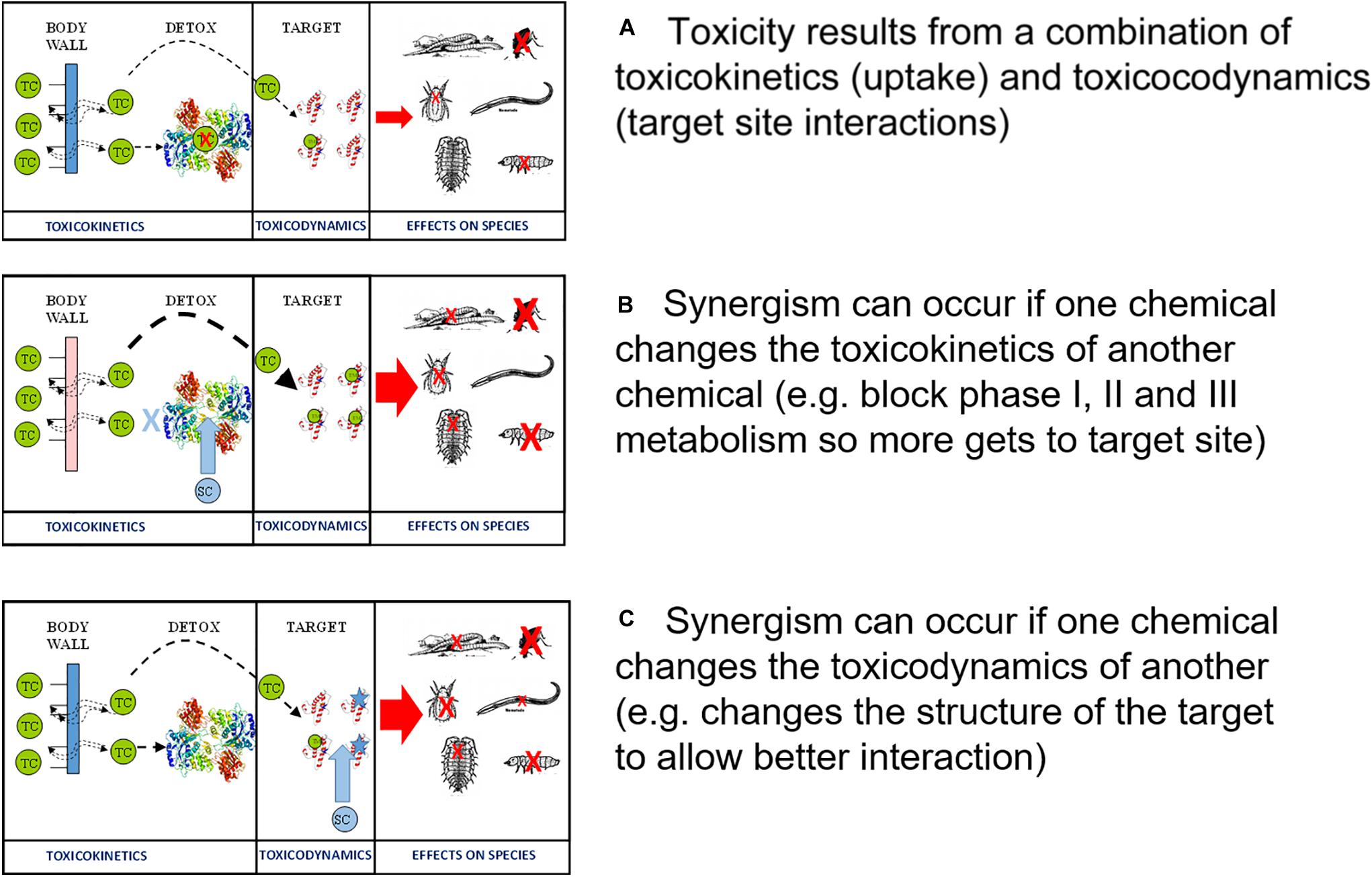
Figure 4. (A) Schematic representing the role of TK and TD traits in toxicity, following uptake the chemical may be detoxified by different enzymes or may escape detoxification to bind with the biological target, (B) interactive mixture effects (e.g., synergism can occur if one chemical changes the toxicokinetics of another chemical (e.g., block detoxification so more gets to target site) leading to greater effects on exposed species or (C) when one chemical changes the TD of another (e.g., changes the structure of the target to allow better interaction) again leading to greater effects.
Changes to TD in responses in mixtures could result from interactions associated with the MIE or at different points along the AOP. The dynamics of target site interactions have been shown to cause different effects depending on the sequential order in which species are exposed to chemicals in time (Ashauer et al., 2017; Figure 4). Further, when different chemicals affect different receptors in the same organism (e.g., different specific receptor targets), there is also a potential for interactions. Identification of the TK and TD traits could, thus, indicate the potential for synergism and contribute to improved mixture risk assessment.
Design of Effective and Benign Chemicals for Applications
The development of pharmaceuticals has refined the practice of receptor-ligand binding analysis and the identification of off-target effects using sequence analysis, biochemistry, ligand binding studies and in vitro and in vivo testing. With the increasing availability of genetic resources (Verbruggen et al., 2018) and functional testing approaches for ecotoxicological species (Kumagai et al., 2017; Roth et al., 2019; Maki et al., 2020), more options exist to extend such analyses to look at non-target chemical effects. Given the costs to develop new agrochemicals or biocides to market, a capacity to screen out molecules that may strongly affect a particular adverse outcome pathway in a species likely to be exposed is an appealing option. Tools such as EcoDrug and SeqAPASS may provide a basis for such screening, but will increasingly need updating as new information about the functional mediators of sensitivity become available, such as the reduction of exposure at classical target receptors due to the expression of alternative receptors associated with different functionality and/or expressed in non-target tissues.
Biotechnology Applications
Adaptation through evolution may result in predictable phenotypic traits conserved across species that determine sensitivity. For example, it has been commonly observed that insects exposed to pyrethroids evolve resistance through increased metabolic detoxification and decreased target sensitivity. The advent of methods for genetic editing using tools, such as CRISPR-Cas9, offer the possibility to change the metabolic capacity, target gene repertoire or expression of target sequences, with the potential to confer change in sensitivity. The concept of a gene drive for sterility have been advanced for the eradication of malarial mosquito populations (Gantz et al., 2015; Simoni et al., 2020). It is theoretically possible to imagine similar studies to introduce susceptible pests to eradicate an adapted population or the manipulation of the genes of vulnerable species to increase tolerance to a pervasive chemical threat.
Informed Species Monitoring
Assuming that we will not achieve sufficient understanding of all primary and secondary mechanisms of action to fully mitigate all chemical impacts, there is likely to remain a need for monitoring programs to track populations for any adverse chemical effects. This need underlies the concept of Pesticidovigilance (Milner and Boyd, 2017) as a future approach for post release impact assessment. For effects-based monitoring, any Pesticidovigilance framework will require the selection of focus species. Integration of data on ecological traits related to feeding preferences for certain pesticide-treated crops (Woodcock et al., 2016) or to particular TK or TD traits, relevant to the AOP for the chemical of concern, may be used to guide such a choice of species toward vulnerable taxa. Such approaches are complementary to currently used prospective risk-based frameworks for chemical management. As trait resources expand, the potential to make such decisions on focus species for retrospective chemical monitoring from a mechanistic viewpoint will increase in parallel.
Author Contributions
DS, SS, and PK conceived the original structure of the review and developed the overall structure and layout. DS and SS prepared the manuscript. All authors contributed specific knowledge of relevant literature to particular areas covered and edited the manuscript.
Funding
This article was written within research grants NE/S000224/2 and NE/M01438X/1 from the Natural Environment Research Council (now part of UK Research and Innovation) and a UK Individual Merit Promotion Award supported by the UK Centre for Ecology and Hydrology Institutional Fund project 06896 for DJS.
Conflict of Interest
The authors declare that the research was conducted in the absence of any commercial or financial relationships that could be construed as a potential conflict of interest.
Acknowledgments
We acknowledge Dr. Claus Svendsen, Dr. Jan Baas and Professor Andrew Johnson (all UK Centre for Ecology and Hydrology), Dr. Roman Ashauer (Syngenta International AG), Dr. Jacob Bundy (Imperial College, London) and the late Prof John Morgan for discussions on this subject.
Footnotes
References
Abdul Hameed, M. D. M., Pannala, V. R., and Wallqvist, A. (2019). Mining public toxicogenomic data reveals insights and challenges in delineating liver steatosis adverse outcome pathways. Front. Genet. 10:01007. doi: 10.3389/fgene.2019.01007
Alda Alvarez, O. A., Jager, T., Redondo, E. M., and Kammenga, J. E. (2006). Physiological modes of action of toxic chemicals in the nematode Acrobeloides nanus. Environ. Toxicol. Chem. 25, 3230–3237. doi: 10.1897/06-097r.1
Altenburger, R., Backhaus, T., Boedeker, W., Faust, M., Scholze, M., and Grimme, L. H. (2000). Predictability of the toxicity of multiple chemical mixtures to Vibrio fischeri: mixtures composed of similarly acting chemicals. Environ. Toxicol. Chem. 19, 2341–2347. doi: 10.1897/1551-5028(2000)019<2341:pottom>2.3.co;2
Amiard, J. C., Amiard-Triquet, C., Barka, S., Pellerin, J., and Rainbow, P. S. (2006). Metallothioneins in aquatic invertebrates: their role in metal detoxification and their use as biomarkers. Aquat. Toxicol. 76, 160–202. doi: 10.1016/j.aquatox.2005.08.015
Amor, F., Christiaens, O., Bengochea, P., Medina, P., Rouge, P., Vinuela, E., et al. (2012). Selectivity of diacylhydrazine insecticides to the predatory bug Orius laevigatus: in vivo and modelling/docking experiments. Pest Man. Sci. 68, 1586–1594. doi: 10.1002/ps.3353
Andre, J., King, R. A., Stürzenbaum, S. R., Kille, P., Hodson, M. E., and Morgan, A. J. (2010). Molecular genetic differentiation in earthworms inhabiting a heterogeneous Pb-polluted landscape. Environ. Pollut. 158, 883–890. doi: 10.1016/j.envpol.2009.09.021
Ankley, G. T., Bennett, R. S., Erickson, R. J., Hoff, D. J., Hornung, M. W., Johnson, R. D., et al. (2010). Adverse outcome pathways: a conceptual framework to support ecotoxicology research and risk assessment. Environ. Toxicol. Chem. 29, 730–741. doi: 10.1002/etc.34
Ashauer, R., and Escher, B. I. (2010). Advantages of toxicokinetic and toxicodynamic modelling in aquatic ecotoxicology and risk assessment. J. Environ. Mon. 12, 2056–2061. doi: 10.1039/c0em00234h
Ashauer, R., and Jager, T. (2018). Physiological modes of action across species and toxicants: the key to predictive ecotoxicology. Environ. Sci. Process. Impacts 20, 48–57. doi: 10.1039/c7em00328e
Ashauer, R., O’Connor, I., and Escher, B. I. (2017). Toxic mixtures in time—The sequence makes the poison. Environ. Sci. Technol. 51, 3084–3092. doi: 10.1021/acs.est.6b06163
Awkerman, J. A., Raimondo, S., Jackson, C. R., and Barron, M. G. (2014). Augmenting aquatic species sensitivity distributions with interspecies toxicity estimation models. Environ. Toxicol. Chem. 33, 688–695. doi: 10.1002/etc.2456
Baas, J., Augustine, S., Marques, G. M., and Dorne, J. L. (2018). Dynamic energy budget models in ecological risk assessment: from principles to applications. Sci. Total Environ. 628-629, 249–260. doi: 10.1016/j.scitotenv.2018.02.058
Baas, J., Sebastiaan, A. L., and Kooijman, M. (2015). Sensitivity of animals to chemical compounds links to metabolic rate. Ecotoxicology 24, 657–663. doi: 10.1007/s10646-014-1413-5
Bach, L., Palmqvist, A., Rasmussen, L. J., and Forbes, V. E. (2005). Differences in PAH tolerance between Capitella species: underlying biochemical mechanisms. Aquat. Toxicol. 74, 307–319. doi: 10.1016/j.aquatox.2005.06.002
Backhaus, T., Altenburger, R., Boedeker, W., Faust, M., Scholze, M., and Grimme, L. H. (2000). Predictability of the toxicity of a multiple mixture of dissimilarly acting chemicals to Vibrio fischeri. Environ. Toxicol. Chem. 19, 2348–2356. doi: 10.1897/1551-5028(2000)019<2348:pottoa>2.3.co;2
Baillard, V., Sulmon, C., Bittebiere, A. K., Mony, C., Couee, I., Gouesbet, G., et al. (2020). Effect of interspecific competition on species sensitivity distribution models: analysis of plant responses to chemical stress. Ecotox. Environ. Saf. 200:10.
Baird, D. J., and Van den Brink, P. J. (2007). Using biological traits to predict species sensitivity to toxic substances. Ecotox. Environ. Saf. 67, 296–301. doi: 10.1016/j.ecoenv.2006.07.001
Bally, J., McIntyre, G. J., Doran, R. L., Lee, K., Perez, A., Jung, H., et al. (2016). In-plant protection against Helicoverpa armigera by production of long hpRNA in chloroplasts. Front. Plant Sci. 7:9. doi: 10.3389/fpls.2016.01453
Banks, G., Kemenes, I., Schofield, M., O’Shea, M., and Korneev, S. A. (2009). Acetylcholine binding protein of mollusks is unlikely to act as a regulator of cholinergic neurotransmission at neurite-neurite synaptic sites in vivo. FASEB J. 23, 3030–3036. doi: 10.1096/fj.08-117135
Bao, H. B., Meng, X. K., and Liu, Z. W. (2017). Spider acetylcholine binding proteins: an alternative model to study the interaction between insect nAChRs and neonicotinoids. Insect Biochem. Mol. Biol. 90, 82–89. doi: 10.1016/j.ibmb.2017.09.014
Bargmann, C. I. (1998). Neurobiology of the Caenorhabditis elegans genome. Science 282, 2028–2033. doi: 10.1126/science.282.5396.2028
Bass, C., Puinean, A. M., Andrews, M., Cutler, P., Daniels, M., Elias, J., et al. (2011). Mutation of a nicotinic acetylcholine receptor beta subunit is associated with resistance to neonicotinoid insecticides in the aphid Myzus persicae. BMC Neuroscience 12:51.
Batty, L., and Hallberg, B. A. (2010). Ecology of Industrial Pollution. Cambridge: Cambridge University Press, 362.
Beadle, K., Singh, K. S., Troczka, B. J., Randall, E., Zaworra, M., Zimmer, C. T., et al. (2019). Genomic insights into neonicotinoid sensitivity in the solitary bee Osmia bicornis. PLoS Genet. 15:19.
Beaudouin, R., and Pery, A. R. R. (2013). Comparison of species sensitivity distributions based on population or individual endpoints. Environ. Toxicol. Chem. 32, 1173–1177. doi: 10.1002/etc.2148
Bejarano, A. C. (2019). Further development and refinement of Interspecies Correlation Estimation models for current-use dispersants. Environ. Toxicol. Chem. 38, 1682–1691.
Bejarano, A. C., Raimondo, S., and Barron, M. G. (2017). Framework for optimizing selection of interspecies correlation estimation models to address species diversity and toxicity gaps in an aquatic database. Environ. Sci. Technol. 51, 8158–8165. doi: 10.1021/acs.est.7b01493
Bell, B. G., Schellevis, F., Stobberingh, E., Goossens, H., and Pringle, M. (2014). A systematic review and meta-analysis of the effects of antibiotic consumption on antibiotic resistance. BMC Infect. Dis. 14:25.
Berenbaum, M. R., and Johnson, R. M. (2015). Xenobiotic detoxification pathways in honey bees. Curr. Opin. Insect Sci. 10, 51–58. doi: 10.1016/j.cois.2015.03.005
Berger, E., Haase, P., Schafer, R. B., and Sundermann, A. (2018). Towards stressor-specific macroinvertebrate indices: which traits and taxonomic groups are associated with vulnerable and tolerant taxa? Sci. Total Environ. 619, 144–154. doi: 10.1016/j.scitotenv.2017.11.022
Billoir, E., Delignette-Muller, M. L., Pery, A. R. R., Geffard, O., and Charles, S. (2008). Statistical cautions when estimating DEBtox parameters. J. Theor. Biol. 254, 55–64. doi: 10.1016/j.jtbi.2008.05.006
Blackwell, B. R., Ankley, G. T., Bradley, P. M., Houck, K. A., Makarov, S. S., Medvedev, A. V., et al. (2019). Potential toxicity of complex mixtures in surface waters from a nationwide survey of united states streams: Identifying in vitro bioactivities and causative chemicals. Environ. Sci. Technol. 53, 973–983. doi: 10.1021/acs.est.8b05304
Bowes, J., Brown, A. J., Hamon, J., Jarolimek, W., Sridhar, A., Waldron, G., et al. (2012). Reducing safety-related drug attrition: the use of in vitro pharmacological profiling. Nat. Rev. Drug Discov. 11, 909–922. doi: 10.1038/nrd3845
Brady, S. P., Richardson, J. L., and Kunz, B. K. (2017). Incorporating evolutionary insights to improve ecotoxicology for freshwater species. Evol. Appl. 10, 829–838. doi: 10.1111/eva.12507
Brander, S. M., Biales, A. D., and Connon, R. E. (2017). The role of epigenomics in aquatic toxicology. Environ. Toxicol. Chem. 36, 2565–2573. doi: 10.1002/etc.3930
Brinkmann, M., Schlechtriem, C., Reininghaus, M., Eichbaum, K., Buchinger, S., Reifferscheid, G., et al. (2016). Cross-species extrapolation of uptake and disposition of neutral organic chemicals in fish using a multispecies physiologically-based toxicokinetic model framework. Environ. Sci. Technol. 50, 1914–1923. doi: 10.1021/acs.est.5b06158
Brock, T. C. M., Arts, G. H. P., Maltby, L., and Van den Brink, P. J. (2006). Aquatic risks of pesticides, ecological protection goals, and common aims in European Union legislation. Int. Environ. Assess. Man. 2, E20–E46.
Brockmeier, E. K., Hodges, G., Hutchinson, T. H., Butler, E., Hecker, M., Tollefsen, K. E., et al. (2017). The role of omics in the application of adverse outcome pathways for chemical risk assessment. Tox. Sci. 158, 252–262.
Broerse, M., and van Gestel, C. A. M. (2010). Mixture effects of nickel and chlorpyrifos on Folsomia candida (Collembola) explained from development of toxicity in time. Chemosphere 79, 953–957. doi: 10.1016/j.chemosphere.2010.02.032
Brown, R. J., Galloway, T. S., Lowe, D., Browne, M. A., Dissanayake, A., Jones, M. B., et al. (2004). Differential sensitivity of three marine invertebrates to copper assessed using multiple biomarkers. Aquat. Toxicol. 66, 267–278. doi: 10.1016/j.aquatox.2003.10.001
Bruce, T. J. A., Matthes, M. C., Napier, J. A., and Pickett, J. A. (2007). Stressful “memories” of plants: evidence and possible mechanisms. Plant Sci. 173, 603–608. doi: 10.1016/j.plantsci.2007.09.002
Capitao, A. M. F., Lopes-Marques, M. S., Ishii, Y., Ruivo, R., Fonseca, E. S. S., Pascoa, I., et al. (2018). Evolutionary exploitation of vertebrate peroxisome proliferator-activated receptor gamma by organotins. Environ. Sci. Technol. 52, 13951–13959.
Castro, L. F. C., Goncalves, O., Mazan, S., Tay, B. H., Venkatesh, B., and Wilson, J. M. (2014). Recurrent gene loss correlates with the evolution of stomach phenotypes in gnathostome history. Proc. Royal Soc. B 281:20132669. doi: 10.1098/rspb.2013.2669
Cedergreen, N. (2014). Quantifying synergy: a systematic review of mixture toxicity studies within environmental toxicology. PLoS One 9:e96580. doi: 10.1371/journal.pone.0096580
Cedergreen, N., Dalhoff, K., Li, D., Gottardi, M., and Kretschmann, A. (2017). Can toxicokinetic and toxicodynamic modelling be used to understand and predict synergistic interactions between chemicals? Environ. Sci. Technol. 51, 14379–14389. doi: 10.1021/acs.est.7b02723
Celander, M. C., Goldstone, J. V., Denslow, N. D., Iguchi, T., Kille, P., Meyerhoff, R. D., et al. (2011). Species extrapolation for the 21ST centuary. Environ. Toxicol. Chem. 30, 52–63.
Chandrangsu, P., Rensing, C., and Helmann, J. D. (2017). Metal homeostasis and resistance in bacteria. Nat. Rev. Microbiol. 15, 338–350. doi: 10.1038/nrmicro.2017.15
Chen, Z. F., and Ying, G. G. (2015). Occurrence, fate and ecological risk of five typical azole fungicides as therapeutic and personal care products in the environment: A review. Environ. Int. 84, 142–153. doi: 10.1016/j.envint.2015.07.022
Cheng, D. F., Guo, Z. J., Riegler, M., Xi, Z. Y., Liang, G. W., and Xu, Y. J. (2017). Gut symbiont enhances insecticide resistance in a significant pest, the oriental fruit fly Bactrocera dorsalis (Hendel). Microbiome 5:12.
Chi, L., Xue, J. C., Tu, P. C., Lai, Y. J., Ru, H. Y., and Lu, K. (2019). Gut microbiome disruption altered the biotransformation and liver toxicity of arsenic in mice. Arch. Toxicol. 93, 25–35. doi: 10.1007/s00204-018-2332-7
Chiari, Y., Glaberman, S., Seren, N., Carretero, M. A., and Capellini, I. (2015). Phylogenetic signal in amphibian sensitivity to copper sulfate relative to experimental temperature. Ecol. Appl. 25, 596–602. doi: 10.1890/14-0439.1
Coady, K., Browne, P., Embry, M., Hill, T., Leinala, E., Steeger, T., et al. (2019). When are adverse outcome pathways and associated assays “Fit for Purpose” for regulatory decision-making and management of chemicals? Int. Environ. Assess. Man. 15, 633–647. doi: 10.1002/ieam.4153
Costa, D., Marien, J., Janssens, T. K. S., van Gestel, C. A. M., Driessen, G., Sousa, J. P., et al. (2012). Influence of adaptive evolution of cadmium tolerance on neutral and functional genetic variation in Orchesella cincta. Ecotoxicology 21, 2078–2087. doi: 10.1007/s10646-012-0961-9
Creton, S., Saghir, S. A., Bartels, M. J., Billington, R., Bus, J. S., Davies, W., et al. (2012). Use of toxicokinetics to support chemical evaluation: Informing high dose selection and study interpretation. Regul. Toxicol. Pharmacol. 62, 241–247. doi: 10.1016/j.yrtph.2011.12.005
Cropp, R., Nash, S. B., and Hawker, D. (2014). A model to resolve organochlorine pharmacokinetics in migrating humpback whales. Environ. Toxicol. Chem. 33, 1638–1649. doi: 10.1002/etc.2603
Daisley, B. A., Trinder, M., McDowell, T. W., Collins, S. L., Sumarah, M. W., and Reid, G. (2018). Microbiota-mediated modulation of organophosphate insecticide toxicity by species-dependent interactions with lactobacilli in a Drosophila melanogaster insect model. Appl. Environ. Microbiol. 84:13.
Dalhoff, K., Hansen, A. M. B., Rasmussen, J. J., Focks, A., Strobel, B. W., and Cedergreen, N. (2020). Linking morphology, toxicokinetic, and toxicodynamic traits of aquatic invertebrates to pyrethroid sensitivity. Environ. Sci. Technol. 54, 5687–5699. doi: 10.1021/acs.est.0c00189
Dallinger, R., and Hockner, M. (2013). Evolutionary concepts in ecotoxicology: tracing the genetic background of differential cadmium sensitivities in invertebrate lineages. Ecotoxicology 22, 767–778. doi: 10.1007/s10646-013-1071-z
Davies, J., and Davies, D. (2010). Origins and evolution of antibiotic resistance. Microbiol. Mol. Biol. Rev. 74, 417–433.
Deeds, J. R., and Klerks, P. L. (1999). Metallothionein-like proteins in the freshwater oligochaete Limnodrilus udekemianus and their role as a homeostatic mechanism against cadmium toxicity. Environ. Pollut. 106, 381–389. doi: 10.1016/s0269-7491(99)00100-1
Denison, M. S., Soshilov, A. A., He, G. C., DeGroot, D. E., and Zhao, B. (2011). Exactly the same but different: promiscuity and diversity in the molecular mechanisms of action of the aryl hydrocarbon (dioxin) receptor. Tox. Sci. 124, 1–22. doi: 10.1093/toxsci/kfr218
Di Toro, D. M., McGrath, J. A., and Hansen, D. J. (2000). Technical basis for narcotic chemicals and polycyclic aromatic hydrocarbon criteria. I. Water and tissue. Environ. Toxicol. Chem. 19, 1951–1970. doi: 10.1002/etc.5620190803
Dias, V., Vasseur, C., and Bonzorn, J. M. (2008). Exposure of Chironomus riparius larvae to uranium: effects on survival, development time, growth, and mouthpart deformities. Chemosphere 71, 574–581. doi: 10.1016/j.chemosphere.2007.09.029
Doering, J. A., Villeneuve, D. L., Fay, K. A., Randolph, E. C., Jensen, K. M., Kahl, M. D., et al. (2019a). Differential sensitivity to in vitro inhibition of cytochrome P450 aromatase (cyp19) activity among 18 freshwater fishes. Tox. Sci. 170, 394–403. doi: 10.1093/toxsci/kfz115
Doering, J. A., Villeneuve, D. L., Poole, S. T., Blackwell, B. R., Jensen, K. M., Kahl, M. D., et al. (2019b). Quantitative response-response relationships linking aromatase inhibition to decreased fecundity are conserved across three fishes with asynchronous oocyte development. Environ. Sci. Technol. 53, 10470–10478. doi: 10.1021/acs.est.9b02606
Doering, J. A., Wiseman, S., Giesy, J. P., and Hecker, M. (2018). A cross-species quantitative adverse outcome pathway for activation of the aryl hydrocarbon receptor leading to early life stage mortality in birds and fishes. Environ. Sci. Technol. 52, 7524–7533. doi: 10.1021/acs.est.8b01438
Douglas, M. R., Sponsler, D. B., Lonsdorr, E. V., and Grozinger, C. M. (2020). County-level analysis reveals a rapidly shifting landscape of insecticide hazard to honey bees (Apis mellifera) on US farmland. Sci. Rep. 10:11.
Dutilleul, M., Bonzom, J.-M., Lecomte, C., Goussen, B., Daian, F., Galas, S., et al. (2014). Rapid evolutionary responses of life history traits to different experimentally-induced pollutions in Caenorhabditis elegans. BMC Evol. Biol. 14:252.
Elias, D., Wang, L. X., and Jacinthe, P. A. (2018). A meta-analysis of pesticide loss in runoff under conventional tillage and no-till management. Environ. Monitor. Assessm. 190:17.
Ellis, L. J. A., Kissane, S., Hoffman, E., Brown, J. B., Valsami-Jones, E., Colbourne, J., et al. (2020). Multigenerational exposures of Daphnia magna to pristine and aged silver nanoparticles: epigenetic changes and phenotypical ageing related effects. Small 16:2000301. doi: 10.1002/smll.202000301
Erdmanis, L., O’Reilly, A. O., Williamson, M. S., Field, L. M., Turberg, A., and Wallace, B. A. (2012). Association of neonicotinoid insensitivity with a conserved residue in the loop d binding region of the tick nicotinic acetylcholine receptor. Biochemistry 51, 4627–4629. doi: 10.1021/bi300522s
Escher, B. I., Ashauer, R., Dyer, S., Hermens, J. L. M., Lee, J.-H., Leslie, H. A., et al. (2008). Crucial role of mechanisms and modes of toxic action for understanding tissue residue toxicity and internal effect concentrations of organic chemical. Int. Environ. Assess. Man. 7, 28–49. doi: 10.1002/ieam.100
Evariste, L., Barret, M., Mottier, A., Mouchet, F., Gauthier, L., and Pinelli, E. (2019). Gut microbiota of aquatic organisms: a key endpoint for ecotoxicological studies. Environ. Pollut. 248, 989–999. doi: 10.1016/j.envpol.2019.02.101
Faber, J. H., Marshall, S., Van den Brink, P. J., and Maltby, L. (2019). Priorities and opportunities in the application of the ecosystem services concept in risk assessment for chemicals in the environment. Sci. Total Environ. 651, 1067–1077. doi: 10.1016/j.scitotenv.2018.09.209
Farmahin, R., Manning, G. E., Crump, D., Wu, D. M., Mundy, L. J., Jones, S. P., et al. (2013). Amino acid sequence of the ligand-binding domain of the aryl hydrocarbon receptor 1 predicts sensitivity of wild birds to effects of dioxin-like compounds. Tox. Sci. 131, 139–152. doi: 10.1093/toxsci/kfs259
Faust, M., Altenburger, R., Backhaus, T., Blanck, H., Boedeker, W., Gramatica, P., et al. (2001). Predicting the joint algal toxicity of multi-component s-triazine mixtures at low-effect concentrations of individual toxicants. Aquat. Toxicol. 56, 13–32. doi: 10.1016/s0166-445x(01)00187-4
Faust, M., Altenburger, R., Backhaus, T., Blanck, H., Boedeker, W., Gramatica, P., et al. (2003). Joint algal toxicity of 16 dissimilarly acting chemicals is predictable by the concept of independent action. Aquat. Toxicol. 63, 43–63. doi: 10.1016/s0166-445x(02)00133-9
Feckler, A., Thielsch, A., Schwenk, K., Schulz, R., and Bundschuh, M. (2012). Differences in the sensitivity among cryptic lineages of the Gammarus fossarum complex. Sci. Total Environ. 439, 158–164. doi: 10.1016/j.scitotenv.2012.09.003
Fernandez, M. D., Meeus, I., Billiet, A., Van Nieuwerburgh, F., Deforce, D., Vandamme, P., et al. (2019). Influence of microbiota in the susceptibility of parasitic wasps to abamectin insecticide: deep sequencing, esterase and toxicity tests. Pest Man. Sci. 75, 79–86. doi: 10.1002/ps.5195
Forbes, V. E., and Calow, P. (2002). Species sensitivity distributions revisited: a critical appraisal. Human Ecol. Risk Assess. 8, 473–492. doi: 10.1080/10807030290879781
Fourie, F., Reinecke, S. A., and Reinecke, A. J. (2007). The determination of earthworm species sensitivity differences to cadmium genotoxicity using the comet assay. Ecotox. Environ. Saf. 67, 361–368. doi: 10.1016/j.ecoenv.2006.10.005
Gainer, A., Cousins, M., Hogan, N., and Siciliano, S. D. (2018). Petroleum hydrocarbon mixture toxicity and a trait-based approach to soil invertebrate species for site-specific risk assessments. Environ. Toxicol. Chem. 37, 2222–2234. doi: 10.1002/etc.4164
Gantz, V. M., Jasinskiene, N., Tatarenkova, O., Fazekas, A., Macias, V. M., Bier, E., et al. (2015). Highly efficient Cas9-mediated gene drive for population modification of the malaria vector mosquito Anopheles stephensi. Proc. Natl. Acad. Sci. U.S.A. 112, E6736–E6743.
Garcia-Reyero, N., Habib, T., Pirooznia, M., Gust, K. A., Gong, P., Warner, C., et al. (2011). Conserved toxic responses across divergent phylogenetic lineages: a meta-analysis of the neurotoxic effects of RDX among multiple species using toxicogenomics. Ecotoxicology 20, 580–594. doi: 10.1007/s10646-011-0623-3
Gardner, M., Comber, S., Scrimshaw, M. D., Cartmell, E., Lester, J., and Ellor, B. (2012). The significance of hazardous chemicals in wastewater treatment works effluents. Sci. Total Environ. 437, 363–372. doi: 10.1016/j.scitotenv.2012.07.086
Gergs, A., Kulkarni, D., and Preuss, T. G. (2015). Body size-dependent toxicokinetics and toxicodynamics could explain intra- and interspecies variability in sensitivity. Environ. Pollut. 206, 449–455. doi: 10.1016/j.envpol.2015.07.045
Gomes, S. I. L., Roca, C. P., Scott-Fordsmand, J. J., and Amorim, M. J. B. (2019). High-throughput transcriptomics: Insights into the pathways involved in (nano) nickel toxicity in a key invertebrate test species. Environ. Pollut. 245, 131–140. doi: 10.1016/j.envpol.2018.10.123
Goussen, B., Pery, A. R. R., Bonzom, J. M., and Beaudouin, R. (2015). Transgenerational adaptation to pollution changes energy allocation in populations of nematodes. Environ. Sci. Technol. 49, 12500–12508. doi: 10.1021/acs.est.5b03405
Grech, A., Brochot, C., Dorne, J. L., Quignot, N., Bois, F. Y., and Beaudouin, R. (2017). Toxicokinetic models and related tools in environmental risk assessment of chemicals. Sci. Total Environ. 578, 1–15. doi: 10.1007/978-94-017-3437-0_1
Greer, E. L., Maures, T. J., Ucar, D., Hauswirth, A. G., Mancini, E., Lim, J. P., et al. (2011). Transgenerational epigenetic inheritance of longevity in Caenorhabditis elegans. Nature 479, 365–371. doi: 10.1038/nature10572
Gressel, J. (2018). Microbiome facilitated pest resistance: potential problems and uses. Pest Man. Sci. 74, 511–515. doi: 10.1002/ps.4777
Guenard, G., von der Ohe, P. C., de Zwart, D., Legendre, P., and Lek, S. (2011). Using phylogenetic information to predict species tolerances to toxic chemicals. Ecol. Appl. 21, 3178–3190. doi: 10.1890/10-2242.1
Guenard, G., von der Ohe, P. C., Walker, S. C., Lek, S., and Legendre, P. (2014). Using phylogenetic information and chemical properties to predict species tolerances to pesticides. Proc. Royal Soc. B 281:9.
Gunnarsson, L., Jauhiainen, A., Kristiansson, E., Nerman, O., and Larsson, D. G. J. (2008). Evolutionary conservation of human drug targets in organisms used for environmental risk assessments. Environ. Sci. Technol. 42, 5807–5813. doi: 10.1021/es8005173
Hardstone, M. C., and Scott, J. G. (2010). Is Apis mellifera more sensitive to insecticides than other insects? Pest Man. Sci. 66, 1171–1180. doi: 10.1002/ps.2001
Hartnik, T., Sverdrup, L. E., and Jensen, J. (2008). Toxicity of the pesticide alpha-cypermethrin to four soil nontarget invertebrates and implications for risk assessment. Environ. Toxicol. Chem. 27, 1408–1415. doi: 10.1897/07-385.1
Hayes, F., Spurgeon, D. J., Lofts, S., and Jones, L. (2018). Evidence-based logic chains demonstrate multiple impacts of trace metals on ecosystem services. J. Environ. Man. 223, 150–164. doi: 10.1016/j.jenvman.2018.05.053
He, W., Qin, N., Kong, X. Z., Liu, W. X., Wu, W. J., He, Q. S., et al. (2014). Ecological risk assessment and priority setting for typical toxic pollutants in the water from Beijing-Tianjin-Bohai area using Bayesian matbugs calculator (BMC). Ecol. Indicat. 45, 209–218. doi: 10.1016/j.ecolind.2014.04.008
Heard, M. S., Baas, J., Dorne, J. L., Lahive, E., Robinson, A. G., Rortais, A., et al. (2017). Comparative toxicity of pesticides and environmental contaminants in bees: are honey bees a useful proxy for wild bee species? Sci. Total Environ. 578, 357–365. doi: 10.1016/j.scitotenv.2016.10.180
Heijne, W. H. M., Kienhuis, A. S., van Ommen, B., Stierum, R. H., and Groten, J. P. (2005). Systems toxicology: applications of toxicogenomics, transcriptomics, proteomics and metabolomics in toxicology. Exp. Rev. Proteom. 2, 767–780. doi: 10.1586/14789450.2.5.767
Hemingway, J., Hawkes, N. J., McCarroll, L., and Ranson, H. (2004). The molecular basis of insecticide resistance in mosquitoes. Insect. Biochem. Mol. Biol. 34, 653–665. doi: 10.1016/j.ibmb.2004.03.018
Hestermann, E. V., Stegeman, J. J., and Hahn, M. E. (2000). Relative contributions of affinity and intrinsic efficacy to aryl hydrocarbon receptor ligand potency. Toxicol. Appl. Pharmacol. 168, 160–172. doi: 10.1006/taap.2000.9026
Hopkin, S. P. (1993). Ecological implications of ‘95% protection levels’ for metals in soils. Oikos 66, 137–141. doi: 10.2307/3545206
Horemans, N., Spurgeon, D. J., Lecomte-Pradines, C., Saenen, E., Bradshaw, C., Oughton, D., et al. (2019). Current evidence for a role of epigenetic mechanisms in response to ionizing radiation in an ecotoxicological context. Environ. Pollut. 251, 469–483. doi: 10.1016/j.envpol.2019.04.125
Hylton, A., Chiari, Y., Capellini, I., Barron, M. G., and Glaberman, S. (2018). Mixed phylogenetic signal in fish toxicity data across chemical classes. Ecol. Appl. 28, 605–611. doi: 10.1002/eap.1698
Ingham, V. A., Anthousi, A., Douris, V., Harding, N. J., Lycett, G., Morris, M., et al. (2020). A sensory appendage protein protects malaria vectors from pyrethroids. Nature 577, 376–380. doi: 10.1038/s41586-019-1864-1
Jager, T., and Ashauer, R. (2018). Modelling Survival Under Chemical Stress: A Comprehensive Guide to the GUTS Framework. Oakland, CA: Leanpub.
Jager, T., and Zimmer, E. I. (2012). Simplified dynamic energy budget model for analysing ecotoxicity data. Ecol. Model. 225, 74–81. doi: 10.1016/j.ecolmodel.2011.11.012
Jegede, O. O., Owojori, O. J., and Rombke, J. (2017). Temperature influences the toxicity of deltamethrin, chlorpyrifos and dimethoate to the predatory mite Hypoaspis aculeifer (Acari) and the springtail Folsomia candida (Collembola). Ecotox. Environ. Saf. 140, 214–221. doi: 10.1016/j.ecoenv.2017.02.046
Jenck, J. F., Agterberg, F., and Droescher, M. J. (2004). Products and processes for a sustainable chemical industry: a review of achievements and prospects. Green Chem. 6, 544–556. doi: 10.1039/b406854h
Jeremias, G., Barbosa, J., Marques, S. M., De Schamphelaere, K. A. C., Van Nieuwerburgh, F., Deforce, D., et al. (2018). Transgenerational inheritance of DNA hypomethylation in Daphnia magna in response to salinity stress. Environ. Sci. Technol. 52, 10114–10123. doi: 10.1021/acs.est.8b03225
Jiao, Y., Cao, Y. F., Zheng, Z., Liu, M., and Guo, X. M. (2019). Massive expansion and diversity of nicotinic acetylcholine receptors in lophotrochozoans. BMC Genom. 20:937.
Jin, X. W., Wang, Z. J., Wang, Y. Y., Lv, Y. B., Rao, K. F., Jin, W., et al. (2015). Do water quality criteria based on nonnative species provide appropriate protection for native species? Environ. Toxicol. Chem. 34, 1793–1798. doi: 10.1002/etc.2985
Johnson, A. C., Jin, X. W., Nakada, N., and Sumpter, J. P. (2020). Learning from the past and considering the future of chemicals in the environment. Science 367, 384–387. doi: 10.1126/science.aay6637
Johnson, R. M., Dahlgren, L., Siegfried, B. D., and Ellis, M. D. (2013). Acaricide, fungicide and drug interactions in Honey bees (Apis mellifera). PLoS One 8:e54092. doi: 10.1371/journal.pone.0054092
Jones, A. K., Raymond-Delpech, V., Thany, S. H., Gauthier, M., and Sattelle, D. B. (2006). The nicotinic acetylcholine receptor gene family of the honey bee, Apis mellifera. Gen. Res. 16, 1422–1430. doi: 10.1101/gr.4549206
Jones, A. K., and Sattelle, D. B. (2010). Diversity of insect nicotinic acetylcholine receptor subunits. Adv. Exp. Med. Biol. 683, 25–43. doi: 10.1007/978-1-4419-6445-8_3
Jones, D. K., Hammond, J. I., and Relyea, R. A. (2009). Very highly toxic effects of endosulfan across nine species of tadpoles: lag effects and family-level sensitivity. Environ. Toxicol. Chem. 28, 1939–1945. doi: 10.1897/09-033.1
Jonker, M. J., Svendsen, C., Bedaux, J. J. M., Bongers, M., and Kammenga, J. E. (2005). Significance testing of synergistic/antagonistic, dose level-dependent, or dose ratio-dependent effects in mixture dose-response analysis. Environ. Toxicol. Chem. 24, 2701–2713. doi: 10.1897/04-431r.1
Kamoun, I. J., Jegede, O. O., Owojori, O. J., Bouzid, J., Gargouri, R., and Rombke, J. (2018). Effects of deltamethrin, dimethoate, and chlorpyrifos on survival and reproduction of the collembolan Folsomia candida and the predatory mite Hypoaspis aculeifer in two African and two European soils. Int. Environ. Assess. Man. 14, 92–104. doi: 10.1002/ieam.1966
Kaur, K., Bakke, M. J., Nilsen, F., and Horsberg, T. E. (2015). Identification and molecular characterization of two acetylcholinesterases from the salmon louse, Lepeophtheirus salmonis. PLoS One 10:e0125362. doi: 10.1371/journal.pone.0125362
Kent, R. A., and Currie, D. (1995). Predicting algal sensitivity to a pesticide stress. Environ. Toxicol. Chem. 14, 983–991. doi: 10.1002/etc.5620140609
Kikuchi, Y., Hayatsu, M., Hosokawa, T., Nagayama, A., Tago, K., and Fukatsu, T. (2012). Symbiont-mediated insecticide resistance. Proc. Natl. Acad. Sci. U.S.A. 109, 8618–8622. doi: 10.1073/pnas.1200231109
Kille, P., Andre, J., Anderson, C., Ang, H. N., Bruford, M. W., Bundy, J. G., et al. (2013). DNA sequence variation and methylation in an arsenic tolerant earthworm population. Soil Biol. Biochem. 57, 524–532. doi: 10.1016/j.soilbio.2012.10.014
Kim, Y., Jeong, J., Lee, S., Choi, I., and Choi, J. (2020). Identification of adverse outcome pathway related to high-density polyethylene microplastics exposure: Caenorhabditis elegans transcription factor RNAi screening and zebrafish study. J. Hazardous Mat. 388:121725. doi: 10.1016/j.jhazmat.2019.121725
Kimber, I., Dearman, R. J., Basketter, D. A., and Boverhof, D. R. (2014). Chemical respiratory allergy: reverse engineering an adverse outcome pathway. Toxicology 318, 32–39. doi: 10.1016/j.tox.2014.02.001
King, G. K. K., Larras, F., Charles, S., and Delignette-Muller, M. L. (2015). Hierarchical modelling of species sensitivity distribution: Development and application to the case of diatoms exposed to several herbicides. Ecotox. Environ. Saf. 114, 212–221. doi: 10.1016/j.ecoenv.2015.01.022
Klerks, P. L., Xie, L. T., and Levinton, J. S. (2011). Quantitative genetics approaches to study evolutionary processes in ecotoxicology; a perspective from research on the evolution of resistance. Ecotoxicology 20, 513–523. doi: 10.1007/s10646-011-0640-2
Klosin, A., Casas, E., Hidalgo-Carcedo, C., Vavouri, T., and Lehner, B. (2017). Transgenerational transmission of environmental information in C. elegans. Science 356, 316–319.
Kohler, H. R., and Triebskorn, R. (2013). Wildlife ecotoxicology of pesticides: can we track effects to the population level and beyond? Science 341, 759–765. doi: 10.1126/science.1237591
Kolahi, M., Yazdi, M., Goldson-Barnaby, A., and Tabandeh, M. R. (2018). In silico prediction, phylogenetic and bioinformatic analysis of SoPCS gene, survey of its protein characterization and gene expression in response to cadmium in Saccharum officinarum. Ecotox. Environ. Saf. 163, 7–18. doi: 10.1016/j.ecoenv.2018.07.032
Kooijman, S. A. L. M. (1987). A safety factor for LC50 values allowing for differences in the sensitivity among species. Ecotox. Environ. Saf. 18, 241–252.
Kooijman, S. A. L. M. (1993). Dynamic Energy Budget Models in Biological Systems: Theory and Application in Ecotoxicology. Cambridge: Cambridge University Press.
Kooijman, S. J., and Bedaux, J. M. (1996). Analysis of toxicity tests on Daphnia survival and reproduction. Wat. Res. 30, 1711–1723. doi: 10.1016/0043-1354(96)00054-1
Kreutzweiser, D. P., Good, K. P., Chartrand, D. T., Scarr, T. A., Holmes, S. B., and Thompson, D. G. (2008). Effects on litter-dwelling earthworms and microbial decomposition of soil-applied imidacloprid for control of wood-boring insects. Pest Man. Sci. 64, 112–118. doi: 10.1002/ps.1478
Kumagai, H., Nakanishi, T., Matsuura, T., Kato, Y., and Watanabe, H. (2017). CRISPR/Cas-mediated knock-in via non-homologous end-joining in the crustacean Daphnia magna. PLoS One 12:e0186112. doi: 10.1371/journal.pone.0186112
Kummerer, K., Dionysiou, D. D., Olsson, O., and Fatta-Kassinos, D. (2019). Reducing aquatic micropollutants – Increasing the focus on input prevention and integrated emission management. Sci. Total Environ. 652, 836–850. doi: 10.1016/j.scitotenv.2018.10.219
LaLone, C. A., Ankley, G. T., Belanger, S. E., Embry, M. R., Hodges, G., Knapen, D., et al. (2017). Advancing the adverse outcome pathway framework-an international horizon scanning approach. Environ. Toxicol. Chem. 36, 1411–1421. doi: 10.1002/etc.3805
LaLone, C. A., Villeneuve, D. L., Burgoon, L. D., Russom, C. L., Helgen, H. W., Berninger, J. P., et al. (2013). Molecular target sequence similarity as a basis for species extrapolation to assess the ecological risk of chemicals with known modes of action. Aquat. Toxicol. 144, 141–154. doi: 10.1016/j.aquatox.2013.09.004
LaLone, C. A., Villeneuve, D. L., Doering, J. A., Blackwell, B. R., Transue, T. R., Simmons, C. W., et al. (2018). Evidence for cross species extrapolation of mammalian-based high-throughput screening assay results. Environ. Sci. Technol. 52, 13960–13971. doi: 10.1021/acs.est.8b04587
LaLone, C. A., Villeneuve, D. L., Lyons, D., Helgen, H. W., Robinson, S. L., Swintek, J. A., et al. (2016). Sequence alignment to predict across species susceptibility (SeqAPASS): a web-based tool for addressing the challenges of cross-species extrapolation of chemical toxicity. Tox. Sci. 153, 228–245. doi: 10.1093/toxsci/kfw119
Landrigan, P. J., Fuller, R., Acosta, N. J. R., Adeyi, O., Arnold, R., Basu, N., et al. (2018). The lancet commission on pollution and health. Lancet 391, 462–512.
Langdon, C. J., Meharg, A. A., Feldmann, J., Balgar, T., Charnock, J., Farquhar, M., et al. (2002). Arsenic-speciation in arsenate-resistant and non-resistant populations of the earthworm, Lumbricus rubellus. J. Environ. Mon. 4, 603–608. doi: 10.1039/b201366p
Laroche, C., Aggarwal, M., Bender, H., Benndorf, P., Birk, B., Crozier, J., et al. (2018). Finding synergies for 3Rs-Toxicokinetics and read-across: report from an EPAA partners’. Forum. Regul. Toxicol. Pharmacol. 99, 5–21. doi: 10.1016/j.yrtph.2018.08.006
Lau, E. T. C., Yung, M. M. N., Karraker, N. E., and Leung, K. M. Y. (2014). Is an assessment factor of 10 appropriate to account for the variation in chemical toxicity to freshwater ectotherms under different thermal conditions? Environ. Sci. Pollut. Res. 21, 95–104. doi: 10.1007/s11356-013-1708-8
Lavoie, M., Le Faucheur, S., Fortin, C., and Campbell, P. G. C. (2009). Cadmium detoxification strategies in two phytoplankton species: Metal binding by newly synthesized thiolated peptides and metal sequestration in granules. Aquat. Toxicol. 92, 65–75. doi: 10.1016/j.aquatox.2008.12.007
Leblanc, G. A. (1984). Interspecies relationships in acute toxicity of chemicals to aquatic organisms. Environ. Toxicol. Chem. 3, 47–60. doi: 10.1002/etc.5620030107
Lee, W. J., and Hase, K. (2014). Gut microbiota-generated metabolites in animal health and disease. Nat. Chem. Biol. 10, 416–424. doi: 10.1038/nchembio.1535
Li, B., Bisgaard, H. C., and Forbes, V. E. (2004). Identification and expression of two novel cytochrome P450 genes, belonging to CYP4 and a new CYP331 family, in the polychaete Capitella capitata sp.I. Biochem. Biophys. Res. Commun. 325, 510–517. doi: 10.1016/j.bbrc.2004.10.066
Li, Z. G., Chen, X., Li, S. P., and Wang, Z. C. (2015). Effect of nickel chloride on Arabidopsis genomic DNA and methylation of 18S rDNA. Electron. J. Biotechnol. 18, 51–57. doi: 10.1016/j.ejbt.2014.12.004
Liebeke, M., Isabel, G.-P., Anderson, C. J., Lawlor, A. J., Bennett, M. H., Morris, C. A., et al. (2013). Earthworms produce phytochelatins in response to arsenic. PLoS One 8:e81271. doi: 10.1371/journal.pone.0081271
Liess, M., and Von der Ohe, P. C. (2005). Analyzing effects of pesticides on invertebrate communities in streams. Environ. Toxicol. Chem. 24, 954–965. doi: 10.1897/03-652.1
Lindeman, L. C., Thaulow, J., Song, Y., Kamstra, J. H., Xie, L., Asselman, J., et al. (2019). Epigenetic, transcriptional and phenotypic responses in two generations of Daphnia magna exposed to the DNA methylation inhibitor 5-azacytidine. Environ. Epigenet. 5:dvz016.
Linke-Gamenick, I., Forbes, V. E., and Mendez, N. (2000). Effects of chronic fluoranthene exposure on sibling species of Capitella with different development modes. Mar. Ecol. Prog. Ser. 203, 191–203. doi: 10.3354/meps203191
Liu, N. N. (2015). Insecticide Resistance in Mosquitoes: Impact, Mechanisms, and Research Directions. Palo Alto, CA: Annual Reviews, 537–559.
Lofts, S., Spurgeon, D., and Svendsen, C. (2005). Fractions affected and probabilistic risk assessment of Cu, Zn, Cd, and Pb in soils using the free ion approach. Environ. Sci. Technol. 39, 8533–8540. doi: 10.1021/es048121c
Lu, K., Cable, P. H., Abo, R. P., Ru, H. Y., Graffam, M. E., Schlieper, K. A., et al. (2013). Gut microbiome perturbations induced by bacterial infection affect arsenic biotransformation. Chem. Res. Toxicol. 26, 1893–1903. doi: 10.1021/tx4002868
Lwalaba, J. L. W., Louis, L. T., Zvobgo, G., Richmond, M. E. A., Fu, L. B., Naz, S., et al. (2020). Physiological and molecular mechanisms of cobalt and copper interaction in causing phyto-toxicity to two barley genotypes differing in Co tolerance. Ecotox. Environ. Saf. 187:109866. doi: 10.1016/j.ecoenv.2019.109866
Ma, W. C., and Bodt, J. (1993). Differences in toxicity of the insecticide chlorpyrifos to six species of earthworms (Oligochaeta, Lumbricidae) in standardized soil tests. Bull. Environ. Contam. Toxicol. 50, 864–870.
Main, A. R., Webb, E. B., Goyne, K. W., and Mengel, D. (2020). Reduced species richness of native bees in field margins associated with neonicotinoid concentrations in non-target soils. Agric. Ecosys. Environ. 287:106693. doi: 10.1016/j.agee.2019.106693
Maki, J. A., Cavallin, J. E., Lott, K. G., Saari, T. W., Ankley, G. T., and Villeneuve, D. L. (2020). A method for CRISPR/Cas9 mutation of genes in fathead minnow (Pimephales promelas). Aquat. Toxicol. 222:105464. doi: 10.1016/j.aquatox.2020.105464
Malaj, E., Guenard, G., Schafer, R. B., and von der Ohe, P. C. (2016). Evolutionary patterns and physicochemical properties explain macroinvertebrate sensitivity to heavy metals. Ecol. Applicat. 26, 1249–1259. doi: 10.1890/15-0346
Mandal, P. K. (2005). Dioxin: a review of its environmental effects and its aryl hydrocarbon receptor biology. J. Comp. Physiol. B Biochem. Sys. Environ. Physiol. 175, 221–230. doi: 10.1007/s00360-005-0483-3
Manjon, C., Troczka, B. J., Zaworra, M., Beadle, K., Randall, E., Hertlein, G., et al. (2018). Unravelling the molecular determinants of bee sensitivity to neonicotinoid insecticides. Curr. Biol. 28, 1137–1143. doi: 10.1016/j.cub.2018.02.045
Mao, W., Schuler, M. A., and Berenbaum, M. R. (2011). CYP9Q-mediated detoxification of acaricides in the honey bee (Apis mellifera). Proc. Natl. Acad. Sci. U.S.A. 108, 12657–12662. doi: 10.1073/pnas.1109535108
Marczylo, E. L., Jacobs, M. N., and Gant, T. W. (2016). Environmentally induced epigenetic toxicity: potential public health concerns. Crit. Rev. Toxicol. 46, 676–700. doi: 10.1080/10408444.2016.1175417
Masoner, J. R., Kolpin, D. W., Furlong, E. T. I, Cozzarelli, M., Gray, J. L., and Schwab, E. A. (2014). Contaminants of emerging concern in fresh leachate from landfills in the conterminous United States. Environ. Sci. Process Impacts 16, 2335–2354. doi: 10.1039/c4em00124a
Massarin, S., Beaudouin, R., Zeman, F., Floriani, M., Gilbin, R., Alonzo, F., et al. (2011). Biology-based modeling to analyze uranium toxicity data on Daphnia magna in a multigeneration study. Environ. Sci. Technol. 45, 4151–4158. doi: 10.1021/es104082e
Matthiessen, P., and Law, R. J. (2002). Contaminants and their effects on estuarine and coastal organisms in the United Kingdom in the late twentieth century. Environ. Pollut. 120, 739–757. doi: 10.1016/s0269-7491(02)00175-6
McCarty, L. S., and Mackay, D. (1993). Enhancing ecotoxicological modeling and assessment. Environ. Sci. Technol. 27, 1719–1728.
McCormack, T., Petrovich, R. M., Mercier, K. A., DeRose, E. F., Cuneo, M. J., Williams, J., et al. (2010). Identification and functional characterization of a novel acetylcholine-binding protein from the marine annelid Capitella teleta. Biochemistry 49, 2279–2287. doi: 10.1021/bi902023y
McFall-Ngai, M., Hadfield, M. G., Bosch, T. C. G., Carey, H. V., Domazet-Loso, T., Douglas, A. E., et al. (2013). Animals in a bacterial world, a new imperative for the life sciences. Proc. Natl. Acad. Sci. U.S.A. 110, 3229–3236.
Mebane, C. A. (2010). Relevance of risk predictions derived from a chronic species sensitivity distribution with cadmium to aquatic populations and ecosystems. Risk Anal. 30, 203–223. doi: 10.1111/j.1539-6924.2009.01275.x
Meharg, A. A. (2005). Mechanisms of plant resistance to metal and metalloid ions and potential biotechnological applications. Plant Soil 274, 163–174. doi: 10.1007/s11104-004-0262-z
Mekawy, A. M. M., Assaha, D. V. M., and Ueda, A. (2020). Constitutive overexpression of rice metallothionein-like gene OsMT-3a enhances growth and tolerance of Arabidopsis plants to a combination of various abiotic stresses. J. Plant Res. 133, 429–440. doi: 10.1007/s10265-020-01187-y
Melander, A. L. (1914). Can insects become resistant to sprays? J. Econ. Entomol. 7, 167–173. doi: 10.1093/jee/7.2.167
Milner, A. M., and Boyd, I. L. (2017). Toward pesticidovigilance: can lessons from pharmaceutical monitoring help to improve pesticide regulation? Science 357, 1232–1234.
Mirbahai, L., and Chipman, J. K. (2014). Epigenetic memory of environmental organisms: a reflection of lifetime stressor exposures. Mutat. Res. Gen. Toxicol. Environ. Mutagen. 764, 10–17.
Monteiro, L. C., Van Butsel, J., De Meester, N., Traunspurger, W., Derycke, S., and Moens, T. (2018). Differential heavy-metal sensitivity in two cryptic species of the marine nematode Litoditis marina as revealed by developmental and behavioural assays. J. Exp. Mar. Biol. Ecol. 502, 203–210.
Moore, D. R. J., Priest, C. D., Galic, N., Brain, R. A., and Rodney, S. I. (2020). Correcting for phylogenetic autocorrelation in species sensitivity distributions. Int. Environ. Assess. Man. 16, 53–65.
Munoz, I., Gomez, M. J., Molina-Diaz, A., Huijbregts, M. A. J., Fernandez-Alba, A. R., and Garcia-Calvo, E. (2008). Ranking potential impacts of priority and emerging pollutants in urban wastewater through life cycle impact assessment. Chemosphere 74, 37–44.
Murphy, C. A., Nisbet, R. M., Antczak, P., Garcia-Reyero, N., Gergs, A., Lika, K., et al. (2018). Incorporating suborganismal processes into dynamic energy budget models for ecological risk assessment. Int. Environ. Assess. Man. 14, 615–624.
Newton, I., and Wyllie, I. (1992). Recovery of a sparrowhawk population in relation to declining pesticide contamination. J. Appl. Ecol. 29, 476–484.
Nyman, A. M., Schirmer, K., and Ashauer, R. (2012). Toxicokinetic-toxicodynamic modelling of survival of Gammarus pulex in multiple pulse exposures to propiconazole: model assumptions, calibration data requirements and predictive power. Ecotoxicology 21, 1828–1840.
Oaks, J. L., Gilbert, M., Virani, M. Z., Watson, R. T., Meteyer, C. U., Rideout, B. A., et al. (2004). Diclofenac residues as the cause of vulture population decline in Pakistan. Nature 427, 630–633.
Olsson, P. E., and Kille, P. (1997). Functional comparison of the metal-regulated transcriptional control regions of metallothionein genes from cadmium-sensitive and tolerant fish species. Biochim. Biophys. Acta Gene Struct. Expression 1350, 325–334.
Ortiz-Santaliestra, M. E., Maia, J. P., Egea-Serrano, A., and Lopes, I. (2018). Validity of fish, birds and mammals as surrogates for amphibians and reptiles in pesticide toxicity assessment. Ecotoxicology 27, 819–833.
Pal, C., Bengtsson-Palme, J., Kristiansson, E., and Larsson, D. G. J. (2015). Co-occurrence of resistance genes to antibiotics, biocides and metals reveals novel insights into their co-selection potential. BMC Genomics 16:14. doi: 10.1186/s12864-015-2153-5
Pecinka, A., and Scheid, O. M. (2012). Stress-induced chromatin changes: a critical view on their heritability. Plant Cell Physiol. 53, 801–808. doi: 10.1093/pcp/pcs044
Pedersen, J. E., Bergqvist, C. A., and Larhammar, D. (2019). Evolution of vertebrate nicotinic acetylcholine receptors. BMC Evol. Biol. 19:21. doi: 10.1186/s12862-018-1341-8
Perkins, E. J., Gayen, K., Shoemaker, J. E., Antczak, P., Burgoon, L., Falciani, F., et al. (2019). Chemical hazard prediction and hypothesis testing using quantitative adverse outcome pathways, ALTEXAltern. Anim. Exp. 36, 91–102. doi: 10.14573/altex.1808241
Posthuma, L., and DeZwart, D. (2006). Predicted effects of toxicant mixtures are confirmed by changes in fish species assemblages in Ohio, USA, Rivers. Environ. Toxicol. Chem. 25, 1094–1105. doi: 10.1897/05-305R.1
Posthuma, L., Traas, T. P., and Suter, G. W. (2001). The Use of Species Sensitivity Distributions in Ecotoxicology. Boca Raton, Fl: Lewis Publishers. doi: 10.1201/9781420032314
Posthuma, L., van Gils, J., Zijp, M. C., van de Meent, D., and de Zwart, D. (2019). Species sensitivity distributions for use in environmental protection, assessment, and management of aquatic ecosystems for 12 386 chemicals. Environ. Toxicol. Chem. 38, 905–917. doi: 10.1002/etc.4373
Posthuma, L., and Van Straalen, N. M. (1993). Heavy-metal adaptation in terrestrial invertebrates: a review of occurrence, genetics, physiology and ecological consequences. Comparat. Biochem. Physiol. 106c, 11–38. doi: 10.1016/0742-8413(93)90251-F
Pretty, J. N., Brett, C., Gee, D., Hine, R. E., Mason, C. F., Morison, J. I. L., et al. (2000). An assessment of the total external costs of UK agriculture. Agricult. Syst. 65, 113–136. doi: 10.1016/S0308-521X(00)00031-7
Qiu, H., Wjgm, P., van Gestel, C. A. M., and Vijver, M. G. (2014). Can commonly measurable traits explain differences in metal accumulation and toxicity in earthworm species? Ecotoxicology 23, 21–32. doi: 10.1007/s10646-013-1147-9
Raby, M., Nowierski, M., Perlov, D., Zhao, X. M., Hao, C. Y., Poirier, D. G., et al. (2018). Acute toxicity of 6 neonicotinoid insecticides to freshwater invertebrates. Environ. Toxicol. Chem. 37, 1430–1445. doi: 10.1002/etc.4088
Rahman, M. A., Hasegawa, H., and Lim, R. P. (2012). Bioaccumulation, biotransformation and trophic transfer of arsenic in the aquatic food chain. Environ. Res. 116, 118–135. doi: 10.1016/j.envres.2012.03.014
Raimondo, S., and Barron, M. G. (2020). Application of Interspecies Correlation Estimation (ICE) models and QSAR in estimating species sensitivity to pesticides. SAR QSAR Environ. Res. 31, 1–18. doi: 10.1080/1062936X.2019.1686716
Raimondo, S., Jackson, C. R., and Barron, M. G. (2010). Influence of taxonomic relatedness and chemical mode of action in acute interspecies estimation models for aquatic species. Environ. Sci. Technol. 44, 7711–7716. doi: 10.1021/es101630b
Ramya, S. L., Venkatesan, T., Murthy, K. S., Jalali, S. K., and Varghese, A. (2016). Degradation of acephate by Enterobacter asburiae, Bacillus cereus and Pantoea agglonierans isolated from diamondback moth Plutella xylostella (L), a pest of cruciferous crops. J. Environ. Biol. 37, 611–618.
Reyes, P. F. M., Olivares, J., and Sauphanor, B. (2007). Genetic architecture in codling moth populations: comparison between microsatellite and insecticide resistance markers. Mol. Ecol. 16, 3554–3564. doi: 10.1111/j.1365-294X.2007.03410.x
Reynoldson, T. B., Rodriguez, P., and Madrid, M. M. (1996). A comparison of reproduction, growth and acute toxicity in two populations of Tubifex tubifex (Muller, 1774) from the North American Great Lakes and Northern Spain. Hydrobiologia 334, 199–206. doi: 10.1007/BF00017370
Rico, A., and Van den Brink, P. J. (2015). Evaluating aquatic invertebrate vulnerability to insecticides based on intrinsic sensitivity, biological traits, and toxic mode of action. Environ. Toxicol. Chem. 34, 1907–1917. doi: 10.1002/etc.3008
Rivetti, C., Allen, T. E. H., Brown, J. B., Butler, E., Carmichael, P. L., Colbourne, J. K., et al. (2020). Vision of a near future: Bridging the human health-environment divide. Toward an integrated strategy to understand mechanisms across species for chemical safety assessment. Toxicol. In Vitro 62:9. doi: 10.1016/j.tiv.2019.104692
Robinson, A., Hesketh, H., Lahive, E., Horton, A. A., Svendsen, C., Rortais, A., et al. (2017). Comparing bee species responses to chemical mixtures: Common response patterns? PLoS One 12:e0176289. doi: 10.1371/journal.pone.0176289
Rocha-Olivares, A., Fleeger, J. W., and Foltz, D. W. (2004). Differential tolerance among cryptic species: a potential cause of pollutant-related reductions in genetic diversity. Environ. Toxicol. Chem. 23, 2132–2137. doi: 10.1897/03-512
Rockstrom, J., Steffen, W., Noone, K., Persson, A., Chapin, F. S., Lambin, E., et al. (2009). Planetary boundaries: exploring the safe operating space for humanity. Ecol. Soc. 14:33. doi: 10.5751/ES-03180-140232
Römbke, J., Schmelz, R. M., and Pelosi, C. (2017). Effects of organic pesticides on enchytraeids (Oligochaeta) in agroecosystems: laboratory and higher-tier tests. Front. Environ. Sci. 5:20. doi: 10.3389/fenvs.2017.00020
Roth, A., Vleurinck, C., Netschitailo, O., Bauer, V., Otte, M., Kaftanoglu, O., et al. (2019). A genetic switch for worker nutrition-mediated traits in honeybees. PLoS Biol. 17:18. doi: 10.1371/journal.pbio.3000171
Rubach, M. N., Ashauer, R., Maund, S. J., Baird, D. J., and Van den Brink, P. J. (2010a). Toxicokinetic variation in 15 freshwater arthropod species exposed to the insecticide chlorpyrifos. Environ. Toxicol. Chem. 29, 2225–2234. doi: 10.1002/etc.273
Rubach, M. N., Baird, D. J., Boerwinkel, M. C., Maund, S. J., Roessink, I., and Van den Brink, P. J. (2012). Species traits as predictors for intrinsic sensitivity of aquatic invertebrates to the insecticide chlorpyrifos. Ecotoxicology 21, 2088–2101. doi: 10.1007/s10646-012-0962-8
Rubach, M. N., Baird, D. J., and Van den Brink, P. J. (2010b). A new method for ranking mode-specific sensitivity of freshwater arthropods to insecticides and its relationship to biological traits. Environ. Toxicol. Chem. 29, 476–487. doi: 10.1002/etc.55
Rundlof, M., Andersson, G. K. S., Bommarco, R., Fries, I., Hederstrom, V., Herbertsson, L., et al. (2015). Seed coating with a neonicotinoid insecticide negatively affects wild bees. Nature 521, 77–U162. doi: 10.1038/nature14420
Sanchez-Hernandez, J. C. (2010). Environmental applications of earthworm esterases in the agroecosystem. J. Pestic. Sci. 35, 290–301. doi: 10.1584/jpestics.R10-08
Sanchez-Hernandez, J. C., Rios, J. M., and Attademo, A. M. (2018). Response of digestive enzymes and esterases of ecotoxicological concern in earthworms exposed to chlorpyrifos-treated soils. Ecotoxicology 27, 890–899. doi: 10.1007/s10646-018-1914-8
Santos, R., Ursu, O., Gaulton, A., Bento, A. P., Donadi, R. S., Bologa, C. G., et al. (2017). A comprehensive map of molecular drug targets. Nat. Rev. Drug Discov. 16, 19–34. doi: 10.1038/nrd.2016.230
Saur, M., Moeller, V., Kapetanopoulos, K., Braukmann, S., Gebauer, W., Tenzer, S., et al. (2012). Acetylcholine-binding protein in the hemolymph of the planorbid snail Biomphalaria glabrata is a pentagonal dodecahedron (60 subunits). PLoS One 7:e43685. doi: 10.1371/journal.pone.0043685
Schipper, A. M., Wijnhoven, S., Baveco, H., and van den Brink, N. W. (2012). Contaminant exposure in relation to spatio-temporal variation in diet composition: A case study of the little owl (Athene noctua). Environ. Pollut. 163, 109–116. doi: 10.1016/j.envpol.2011.12.020
Scott-Fordsmand, J. J., and Weeks, J. M. (1998). Review of Selected Biomarkers in Earthworms. Pensacola, FL: SETAC Press, 173–190.
Selck, H., Palmqvist, A., and Forbes, V. E. (2003). Biotransformation of dissolved and sediment-bound fluoranthene in the polychaete Capitella sp I. Environ. Toxicol. Chem. 22, 2364–2374. doi: 10.1897/02-272
Semenzin, E., Lanzellotto, E., Hristozov, D., Critto, A., Zabeo, A., Giubilato, E., et al. (2015). Species sensitivity weighted distribution for ecological risk assessment of engineered nanomaterials: the n-TiO2 case study. Environ. Toxicol. Chem. 34, 2644–2659. doi: 10.1002/etc.3103
Seren, N., Glaberman, S., Carretero, M. A., and Chiari, Y. (2014). Molecular evolution and functional divergence of the metallothionein gene family in vertebrates. J. Mol. Evol. 78, 217–233. doi: 10.1007/s00239-014-9612-5
Shahsavar, A., Gajhede, M., Kastrup, J. S., and Balle, T. (2016). Structural studies of nicotinic acetylcholine receptors: using acetylcholine-binding protein as a structural surrogate. Basic Clin. Pharmacol. Toxicol. 118, 399–407. doi: 10.1111/bcpt.12528
Shaw, J. R., Colbourne, J. K., Glaholt, S. P., Turner, E., Folt, C. L., and Chen, C. Y. (2019). Dynamics of cadmium acclimation in Daphnia pulex: Linking fitness costs, cross-tolerance, and hyper-induction of metallothionein. Environ. Sci. Technol. 53, 14670–14678. doi: 10.1021/acs.est.9b05006
Siegwart, M., Thibord, J. B., Olivares, J., Hirn, C., Elias, J., Maugin, S., et al. (2017). Biochemical and molecular mechanisms associated with the resistance of the european corn borer (Lepidoptera: Crambidae) to lambda-cyhalothrin and first monitoring tool. J. Econ. Entomol. 110, 598–606. doi: 10.1093/jee/tow267
Silva, C. D. E., Brennan, N., Brouwer, J. M., Commandeur, D., Verweij, R. A., and van Gestel, C. A. M. (2017). Comparative toxicity of imidacloprid and thiacloprid to different species of soil invertebrates. Ecotoxicology 26, 555–564. doi: 10.1007/s10646-017-1790-7
Simoni, A., Hammond, A. M., Beaghton, A. K., Galizi, R., Taxiarchi, C., Kyrou, K., et al. (2020). A male-biased sex-distorter gene drive for the human malaria vector Anopheles gambiae. Nat. Biotechnol. 38, 1054–1060. doi: 10.1038/s41587-020-0508-1
Singh, S., Parihar, P., Singh, R., Singh, V. P., and Prasad, S. M. (2016). Heavy metal tolerance in plants: role of transcriptomics, proteomics, metabolomics, and lonomics. Front. Plant Sci. 6:1143. doi: 10.3389/fpls.2015.01143
Smetanova, S., Blaha, L., Liess, M., Schafer, R. B., and Beketov, M. A. (2014). Do predictions from species sensitivity distributions match with field data? Environ. Pollut. 189, 126–133. doi: 10.1016/j.envpol.2014.03.002
Song, Y., Xie, L., Lee, Y., Brede, D. A., Lyne, F., Kassaye, Y., et al. (2020). Integrative assessment of low-dose gamma radiation effects on Daphnia magna reproduction: toxicity pathway assembly and AOP development. Sci. Total Environ. 705:135912. doi: 10.1016/j.scitotenv.2019.135912
Spinu, N., Cronin, M. T. D., Enoch, S. J., Madden, J. C., and Worth, A. P. (2020). Quantitative adverse outcome pathway (qAOP) models for toxicity prediction. Arch. Toxicol. 94, 1497–1510. doi: 10.1007/s00204-020-02774-7
Spurgeon, D. J., Jones, O. A. H., Dorne, J.-L. C. M., Svendsen, C., Swain, S., and Stürzenbaum, S. R. (2010). Systems toxicology approaches for understanding the joint effects of environmental chemical mixtures. Sci. Total Environ. 408, 3725–3734. doi: 10.1016/j.scitotenv.2010.02.038
Stadnicka, J., Schirmer, K., and Ashauer, R. (2012). Predicting concentrations of organic chemicals in fish by using toxicokinetic models. Environ. Sci. Technol. 46, 3273–3280. doi: 10.1021/es2043728
Stassen, J. H. M., Lopez, A., Jain, R., Pascual-Pardo, D., Luna, E., Smith, L. M., et al. (2018). The relationship between transgenerational acquired resistance and global DNA methylation in Arabidopsis. Sci. Rep. 8:14761. doi: 10.1038/s41598-018-32448-5
Sturmbauer, C., Opadiya, G. B., Niederstatter, H., Riedmann, A., and Dallinger, R. (1999). Mitochondrial DNA reveals cryptic oligochaete species differing in cadmium resistance. Mol. Biol. Evol. 16, 967–974. doi: 10.1093/oxfordjournals.molbev.a026186
Stürzenbaum, S. R., Georgiev, O., Morgan, A. J., and Kille, P. (2004). Cadmium detoxification in earthworms: from genes to cells. Environ. Sci. Technol. 38, 6283–6289. doi: 10.1021/es049822c
Svendsen, C., Siang, P., Lister, L. J., Rice, A., and Spurgeon, D. J. (2010). Similarity, independence or interaction for binary mixture effects of nerve toxicants for the nematode Caenorhabditis elegans. Environ. Toxicol. Chem. 29, 1182–1191. doi: 10.1002/etc.140
Swain, S., Wren, J., Stürzenbaum, S. R., Kille, P., Jager, T., Jonker, M. J., et al. (2010). Linking toxicants mechanism of action and physiological mode of action in Caenorhabditis elegans. BMC Sys. Biol. 4:32. doi: 10.1186/1752-0509-4-32
Tebby, C., Brochot, C., Dorne, J. L., and Beaudouin, R. (2019). Investigating the interaction between melamine and cyanuric acid using a Physiologically-Based Toxicokinetic model in rainbow trout. Toxicol. Appl. Pharmacol. 370, 184–195. doi: 10.1016/j.taap.2019.03.021
Timchalk, C., and Poet, T. S. (2008). Development of a physiologically based pharmacokinetic and pharmacodynamic model to determine dosimetry and cholinesterase inhibition for a binary mixture of chlorpyrifos and diazinon in the rat. Neurotoxicology 29, 428–443. doi: 10.1016/j.neuro.2008.02.004
Tomizawa, M., Talley, T. T., Maltby, D., Durkins, K. A., Medzihradszky, K. F., Burlingame, A. L., et al. (2007). Mapping the elusive neonicotinoid binding site. Proc. Natl. Acad. Sci. U.S.A. 104, 9075–9080. doi: 10.1073/pnas.0703309104
Torkkeli, P. H., Liu, H. X., and French, A. S. (2015). Transcriptome analysis of the central and peripheral nervous systems of the spider Cupiennius salei reveals multiple putative cys-loop ligand gated ion channel subunits and an acetylcholine binding protein. PLoS One 10:e0138068. doi: 10.1371/journal.pone.0138068
Tran, T. K. A., MacFarlane, G. R., Kong, R. Y. C., O’Connor, W. A., and Yu, R. M. K. (2016). Potential mechanisms underlying estrogen-induced expression of the molluscan estrogen receptor (ER) gene. Aquat. Toxicol. 179, 82–94. doi: 10.1016/j.aquatox.2016.08.015
Trijau, M., Asselman, J., Armant, O., dam-Guillermin, C. A., De Schamphelaere, K. A. C., and Alonzo, F. (2018). Transgenerational DNA methylation changes in Daphnia magna exposed to chronic gamma irradiation. Environ. Sci. Technol. 52, 4331–4339. doi: 10.1021/acs.est.7b05695
Tsvetkov, N., Samson-Robert, O., Sood, K., Patel, H. S., Malena, D. A., Gajiwala, P. H., et al. (2017). Chronic exposure to neonicotinoids reduces honey bee health near corn crops. Science 356, 1395–1397. doi: 10.1126/science.aam7470
Uller, T., English, S., and Pen, I. (2015). When is incomplete epigenetic resetting in germ cells favoured by natural selection? Proc. Royal Soc. B 282:20150682. doi: 10.1098/rspb.2015.0682
Van den Berg, S. J. P., Baveco, H., Butler, E., De Laender, F., Focks, A., Franco, A., et al. (2019). Modeling the sensitivity of aquatic macroinvertebrates to chemicals using traits. Environ. Sci. Technol. 53, 6025–6034. doi: 10.1021/acs.est.9b00893
Van Leeuwen, T., Vontas, J., Tsagkarakou, A., Dermauw, W., and Tirry, L. (2010). Acaricide resistance mechanisms in the two-spotted spider mite Tetranychus urticae and other important Acari: a review. Insect Biochem. Mol. Biol. 40, 563–572. doi: 10.1016/j.ibmb.2010.05.008
Van Straalen, N. M., and Denneman, C. A. J. (1989). Ecotoxicological evaluation of soil quality criteria. Ecotox. Environ. Saf. 18, 241–251. doi: 10.1016/0147-6513(89)90018-3
Vandenbrouck, T., Jones, O. A. H., Dom, N., Griffin, J. L., and De Coen, W. (2010). Mixtures of similarly acting compounds in Daphnia magna: From gene to metabolite and beyond. Environ. Int. 36, 254–268. doi: 10.1016/j.envint.2009.12.006
Vejares, S. G., Sabat, P., and Sanchez-Hernandez, J. C. (2010). Tissue-specific inhibition and recovery of esterase activities in Lumbricus terrestris experimentally exposed to chlorpyrifos. Comp. Biochem. Physiol. C 151, 351–359. doi: 10.1016/j.cbpc.2009.12.008
Verbruggen, B., Gunnarsson, L., Kristiansson, E., Osterlund, T., Owen, S. F., Snape, J. R., et al. (2018). ECOdrug: a database connecting drugs and conservation of their targets across species. Nucleic Acids Res. 46, D930–D936. doi: 10.1093/nar/gkx1024
Wallace, K. B., and Herzberg, U. (1988). Reactivation and aging of phosphorylated brain acetylcholinesterase from fish and rodents. Toxicol. Appl. Pharmacol. 92, 307–314. doi: 10.1016/0041-008X(88)90390-0
Wang, G. H., Berdy, B. M., Velasquez, O., Jovanovic, N., Alkhalifa, S., Minbiole, K. P. C., et al. (2020). Changes in microbiome confer multigenerational host resistance after sub-toxic pesticide exposure. Cell Host Microbe 27, 213–224. doi: 10.1016/j.chom.2020.01.009
Wang, M. X., Liang, L. N., Siu, W. S., Fan, D., Sun, H. R., Zhao, H. H., et al. (2019). Loss accounting of environmental pollution within Pearl River Delta region, South China. Environ. Pollut. 249, 676–685. doi: 10.1016/j.envpol.2019.03.081
Wang, X. N., Fan, B., Fan, M., Belanger, S., Li, J., Chen, J., et al. (2020). Development and use of interspecies correlation estimation models in China for potential application in water quality criteria. Chemosphere 240:124848. doi: 10.1016/j.chemosphere.2019.124848
Wang, X. N., Liu, Z. T., Wang, W. H., Zhang, C., and Chen, L. H. (2015). Derivation of predicted no effect concentration (PNEC) for HHCB to terrestrial species (plants and invertebrates). Sci. Total Environ. 508, 122–127. doi: 10.1016/j.scitotenv.2014.11.079
Weeks, J. M., Spurgeon, D. J., Svendsen, C., Hankard, P. K., Kammenga, J. E., Dallinger, R., et al. (2004). Critical analysis of soil invertebrate biomarkers: a field case study in Avonmouth, UK. Ecotoxicology 13, 817–822. doi: 10.1007/s10646-003-4479-z
Weng, N. Y., and Wang, W. X. (2014). Improved tolerance of metals in contaminated oyster larvae. Aquat. Toxicol. 146, 61–69. doi: 10.1016/j.aquatox.2013.10.036
Willming, M. M., Lilavois, C. R., Barron, M. G., and Raimondo, S. (2016). Acute toxicity prediction to threatened and endangered species using interspecies correlation estimation (ICE) models. Environ. Sci. Technol. 50, 10700–10707. doi: 10.1021/acs.est.6b03009
Woodcock, B. A., Bullock, J. M., Shore, R. F., Heard, M. S., Pereira, M. G., Redhead, J., et al. (2017). Country-specific effects of neonicotinoid pesticides on honey bees and wild bees. Science 356, 1393–1395. doi: 10.1126/science.aaa1190
Woodcock, B. A., Isaac, N. J. B., Bullock, J. M., Roy, D. B., Garthwaite, D. G., Crowe, A., et al. (2016). Impacts of neonicotinoid use on long-term population changes in wild bees in England. Nature Comms. 7:12459. doi: 10.1038/ncomms12459
Xie, W. Y., Shen, Q., and Zhao, F. J. (2018). Antibiotics and antibiotic resistance from animal manures to soil: a review. Eur. J. Soil Sci. 69, 181–195. doi: 10.1111/ejss.12494
Xu, F. L., Li, Y. L., Wang, Y., He, W., Kong, X. Z., Qin, N., et al. (2015). Key issues for the development and application of the species sensitivity distribution (SSD) model for ecological risk assessment. Ecol. Indicat. 54, 227–237. doi: 10.1016/j.ecolind.2015.02.001
Zanella, L., Fattorini, L., Brunetti, P., Roccotiello, E., Cornara, L., D’Angeli, S., et al. (2016). Overexpression of AtPCS1 in tobacco increases arsenic and arsenic plus cadmium accumulation and detoxification. Planta 243, 605–622. doi: 10.1016/j.ecoenv.2015.11.039
Keywords: ecotoxicology, toxicokinetics, toxicodynamics, mechanism of action, predictive ecotoxicology
Citation: Spurgeon D, Lahive E, Robinson A, Short S and Kille P (2020) Species Sensitivity to Toxic Substances: Evolution, Ecology and Applications. Front. Environ. Sci. 8:588380. doi: 10.3389/fenvs.2020.588380
Received: 28 July 2020; Accepted: 04 November 2020;
Published: 01 December 2020.
Edited by:
Raul Perez Lejano, New York University, United StatesReviewed by:
Jianfeng Feng, Nankai University, ChinaRémy Beaudouin, French National Instiute for Industrial Environment and Risks, France
Copyright © 2020 Spurgeon, Lahive, Robinson, Short and Kille. This is an open-access article distributed under the terms of the Creative Commons Attribution License (CC BY). The use, distribution or reproduction in other forums is permitted, provided the original author(s) and the copyright owner(s) are credited and that the original publication in this journal is cited, in accordance with accepted academic practice. No use, distribution or reproduction is permitted which does not comply with these terms.
*Correspondence: David Spurgeon, dasp@ceh.ac.uk