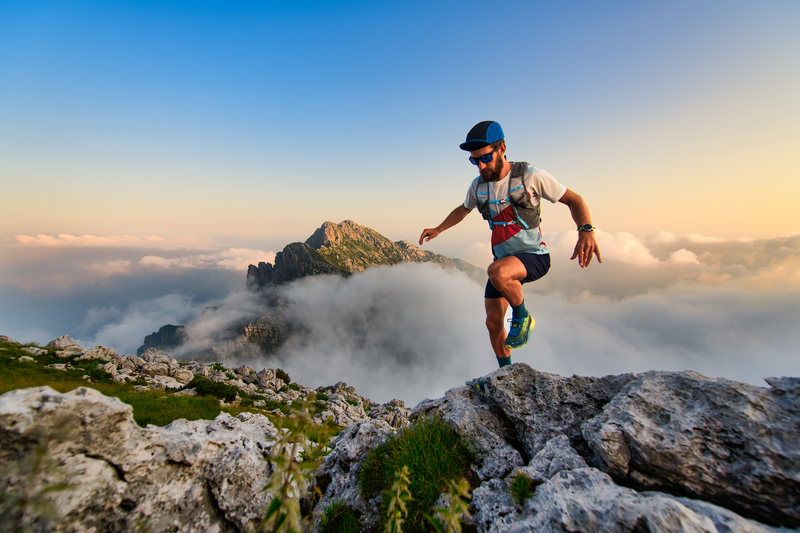
94% of researchers rate our articles as excellent or good
Learn more about the work of our research integrity team to safeguard the quality of each article we publish.
Find out more
PERSPECTIVE article
Front. Environ. Sci. , 23 December 2020
Sec. Interdisciplinary Climate Studies
Volume 8 - 2020 | https://doi.org/10.3389/fenvs.2020.584690
This article is part of the Research Topic Drought and Water Scarcity: Addressing Current and Future Challenges View all 19 articles
With ongoing climate change, we are staring at possibly longer and more severe droughts in the future. Therefore, monitoring and understanding duration and intensity of droughts, and how are they evolving in space and time is imperative for global socio-economic security. Satellite remote sensing has helped us a lot in this endeavor, but most of the satellite missions observe only near-surface properties of the Earth. A recent geodetic satellite mission, GRACE, measured the water storage change both on and beneath the surface, which makes it unique and valuable for drought research. This novel dataset comes with unique problems and characteristics that we should acknowledge before using it. In this perspective article, I elucidate important characteristics of various available GRACE products that are important for drought research. I also discuss limitations of GRACE mission that one should be aware of, and finally I shed some light on latest developments in GRACE data processing that may open numerous possibilities in near future.
Drought is one of the extremes of water availability and one of the biggest threat to sustainable socio-economic development of a region (Nagarajan, 2010; West et al., 2019). In 2019, a quarter of the world's population was reported under severe water stress by water resource institute, and by 2050 this fraction is predicted to double itself (Schlosser et al., 2014). These future predictions are based on models, and they are only as good as our contemporary understanding of the spatiotemporal characteristics of droughts. Therefore, numerous research groups are investigating ongoing and past drought events to obtain novel insights.
Droughts can be classified into four categories that are linked to each other (Mishra and Singh, 2010; Nagarajan, 2010; West et al., 2019). Meteorological drought is driven by a dry weather, usually triggered by less than normal precipitation, high temperature, wind, and sunshine duration. This condition over a prolonged period leads to a drop in soil moisture that cannot support the vegetation, triggering agricultural drought. This usually leads to exploitation of water resources on and beneath the surface of the Earth, which when combined with low runoff and recharge announces the arrival of hydrological drought. Another category is socio-economic drought, defined as the gap between supply and demand of water, which can be a consequence of agricultural or hydrological drought for an agriculture based economy, or due to an unsustainable increase in water demand due to rise in population or changes in lifestyle. Furthermore, onset of drought, its duration, and its impact varies from region to region based on its climate zone, human intervention, socio-economic structure, and impact of climate change and natural variability on the regional water availability (Van Loon et al., 2016). Therefore, monitoring, modeling, and mitigating drought at continental to global scales are few of the biggest challenges in drought research.
Different type of droughts are characterized by different hydrological variables, for example, Meteorological drought is assessed by precipitation, hydrological drought by runoff or reservoir level, and agricultural drought by soil moisture (West et al., 2019). Therefore, to study droughts we require long uninterrupted hydrological observations. Recording and sharing in-situ observations require a strong political will and financial commitment, which is missing. A partial solution to this problem has been offered by satellite remote sensing, but it brings several other challenges with it. For example, remote sensing observations and required hydrological variable are not the same, and requires various assumptions to approximate the latter from the former (e.g., altimetry records river height changes which can then be related to runoff) (Tourian et al., 2013). Another issue is the difference in the spatio-temporal scales of remote sensing observations and the hydrological variable of interest. Therefore, how to use remote sensing observations efficiently for drought research is one of the most important challenges being tackled at various fronts (Liu Q. et al., 2020).
Nevertheless, fueled by decades of scientific developments, several satellite based products are being used. For example, soil moisture time series from SMOS, SMAP, and Sentinel 1, precipitation products from TRMM and GPM missions, snow volume from MODIS, and Lansat based NDVI have been used to study droughts (West et al., 2019; Modanesi et al., 2020). These products were able to provide great insights into meteorological and agricultural drought, but struggled with hydrological drought because of their ability to sense surface processes only. Gravity Recovery And Climate Experiment (GRACE), a geodetic satellite mission launched in 2002, was the first remote sensing platform to provide an estimate of ground water storage change as well (Wouters et al., 2014; Frappart and Ramillien, 2018; Tapley et al., 2019). Therefore, GRACE attracted a lot of interest from the hydrology community to map extremes of water availability (Ramillien et al., 2008; Houborg et al., 2012; Long et al., 2013; Vishwakarma et al., 2013; Thomas et al., 2014; Forootan et al., 2019; Kvas et al., 2019; Liu X. et al., 2020). However, complicated geo-physical inversion behind GRACE products, its post-processing, its debated spatial resolution, a short GRACE time-series, and its disagreement with hydrology models, have prevented us from taping the full potential of GRACE mission.
GRACE mission consisted of two satellite launched in the same near-polar orbit, one following the other with a distance of 220 km between them, at an altitude of 500 km from the surface of the Earth. By measuring changes in the inter-satellite distance with micrometer precision, we are able to map the gravity field of the Earth, which varies in space due to Earth's interior density distribution and topography, and also varies in time due to mass redistribution (Ramillien et al., 2008; Wouters et al., 2014; Tapley et al., 2019). Water being adequately dense and mobile, constitutes the majority of the time-variable signal in GRACE. The second largest signal comes from the visco-elastic response of the solid Earth to past glacial cycles, known as Glacial Isostatic Adjustment (GIA) (Wouters et al., 2014; Peltier et al., 2015). Various GRACE products are freely available to users. We can categorize them into:
1. Level 2, Spherical Harmonic fields: these are dimensionless spherical harmonic coefficients truncated up to a maximum degree, for example lmax = 96. They are made available by science data centers: GFZ, CSR, and JPL (Dahle et al., 2018; Save, 2018). These coefficients, representing full gravity field of the Earth, must be reduced to residual coefficients by subtracting a mean gravity field. To separate hydrology from GIA, a forward model based GIA trends are also subtracted. Then less accurate low degree coefficients (degree 1, C2,0, C3,0) are replaced by those estimated from other sources Then using the relations in Wahr et al. (1998), we can obtain global water mass change estimates in terms of Equivalent Water Height (EWH); an imaginary uniform water (density = 1000 kg/m3) layer of thickness h over a grid cell, which represents the Total Water Storage (TWS) anomaly in that region. It should be pointed out that level 2 GRACE spherical harmonic fields are noisy, hence filtering is essential to extract meaningful information (Swenson and Wahr, 2006; Kusche, 2007; Rangelova et al., 2007). Filtering is known to damage signal amplitude and spatial resolution, therefore, one must apply a correction method to obtain more accurate results (Klees et al., 2007; Vishwakarma et al., 2016, 2017). Since, there are a number of GIA models, filters and corrections method that one can choose from, the final output can vary depending on user's choice.
2. Level 3, gridded EWH fields: these are processed level 2 data with a specific GIA model, filter and correction method. Their spatial resolution and accuracy is dependent on the filter and correction method used (Vishwakarma et al., 2018). They are available from various data centers in a ready to use format that requires minimum effort on the user side, but offers minimum flexibility.
3. Mascons: they are equivalent to level 3 products, but with a different processing strategy. They predict mass changes (in terms of EWH) in concentrated blocks on the surface of the Earth, which would lead to the observed satellite orbit and inter-satellite range rate (Luthcke et al., 2013). The method uses a-prior signal information, physical boundary assumptions (such as continents and oceans), and GRACE variance co-variance information to solve a regularized least squares problem (Luthcke et al., 2013; Watkins et al., 2015; Save et al., 2016). There is no need for an additional filtering and the method claims to tackle signal leakage better than other approaches. These products are available from three centers: JPL, CSR, and GSFC, at a grid sampling of ≤ 1°. However, it should be made clear that GRACE cannot resolve signals at spatial scales smaller than ≈3° on the surface of the Earth (Luthcke et al., 2013; Watkins et al., 2015; Devaraju and Sneeuw, 2016; Vishwakarma, 2017; Vishwakarma et al., 2018; Tapley et al., 2019). These high resolution products are interpolated products sampled at ≤ 1° grid, and should be aggregated to capture mass change signal accurately. In other words, Mascon products should not be used at single grid cell scale. If we want to observe mass change at higher resolution, the satellites should be placed in a lower altitude, which will affect the mission's life-time due to atmospheric drag (Wouters et al., 2014).
4. Level 4 time series: there are several institutes that provide GRACE time series for a region/catchment. The philosophy behind providing these products is that GRACE is more accurate and meaningful at catchment scale (Vishwakarma, 2017). Therefore, researchers aggregate GRACE EWH estimates over a minimum spatial area before carrying out hydrological investigations. The accuracy of GRACE products depend on the post-processing method employed, but as a general rule it decreases with the catchment area (Long et al., 2015; Vishwakarma et al., 2018).
The EWH fields from GRACE represents the total hydrological mass change in a region, also referred to as the TWS anomaly that is a sum of change in groundwater, surface water, soil moisture, snow mass, and canopy water. Therefore, estimating change in one component is not simple, and we require model-based estimates or in-situ observations of other components (Long et al., 2013; Sun, 2013; Li B. et al., 2019). Since model uncertainties vary in space and time and in situ information is scarce, using GRACE for hydrological studies concerning one component of TWS is a challenge (Zaitchik et al., 2008; Sun, 2013; Sneeuw et al., 2014).
GRACE is sensitive to a change in TWS that includes groundwater, soil moisture, and surface water, which means it should be able to detect hydrological drought (including groundwater drought) and severe agricultural drought (Ramillien et al., 2008; Thomas et al., 2014; Frappart and Ramillien, 2018; Li B. et al., 2019). This motivated researchers to develop GRACE based drought indicators, such as Total Storage Deficit Index (TSDI), GRACE-drought severity index (DSI), water storage deficit index (WSDI), GRACE-based Hydrological Drought Index (GHDI), and so on. Most of these indices are derived by following the concept behind conventional drought indices (such as PDSI, SPI, SPEI, SMDI) (Zhao et al., 2017; Hosseini-Moghari et al., 2019). For example, Yirdaw et al. (2008) proposed TSDI, written as
Where TWSi,j is the TWS from GRACE for year i and month j, is the mean TWS value for month j. p and q parameters are obtained from cumulative TSD time series. c is the TSDI value obtained from the best-fit line for the period of dryness, m is the slope and b is the intercept of the cumulative TSD time series. Zhao et al. (2017) introduced GRACE-DSI, defined as
where σj is the standard deviation for month j. GRACE-DSI normalizes the difference between TWS for a time epoch and mean TWS for the corresponding month, with standard deviation of TWS for that month. Similarly there is Water Storage Deficit Index (WSDI) from Sun et al. (2018):
Here μ is the standard deviation of WSD. Another index called total water deficit is written as Leblanc et al. (2009):
Here TWS(t) is the TWS anomaly at current epoch and TWS(t0) is the TWS anomaly observed at time t0 when a drought threshold was observed. The concept behind these indices relies on the assumption that the short GRACE time series is able to capture the climatology signal represented by , which is not true. Therefore, efficacy of GRACE based drought indicators has been questioned. Nevertheless, they have been compared with traditional drought indices and over many regions they have shown exciting potential (Zhao et al., 2017). A recent study showed that GRACE based drought indices when computed with detrended GRACE time series can help us capture meteorological and agricultural droughts (Liu X. et al., 2020). The assumption in this study is that the linear trend is completely anthropogenic, which can be a reasonable assumption in some cases, and removing the linear part will help us get rid of the anthropogenic component and target meteorological and agricultural droughts due to climatic variability. However, such analysis should be carried out with caution as decadal climatic variability could appear to be a linear trend in a short time series (Parker et al., 2007), such as from GRACE.
Drought is a complex phenomenon and its signature can be seen in various hydrological variables. Since the water availability varies in space and in time, using different hydrological observations in a robust framework could help us characterize drought. This is the reason, more than 100 drought indices have been proposed till now (Zargar et al., 2011). Each one of them have been shown to characterize/analyze drought with excellent efficacy for a case study, but their global performance is questioned from time to time. GRACE added a unique dimension to our observational capability by monitoring TWS anomaly, which led to several GRACE based drought indices. There are a few things to note, (a) GRACE has a coarse spatial resolution and it is more accurate at catchment scale, which means large scale droughts are more likely to be efficiently studied using GRACE. (b) Mascons and Level 3 GRACE TWS fields are heavily post-processed, and based on the method chosen, the output quality varies. Hence, users should carefully interpret these products. (c) GRACE was launched in 2002 and we do not have data for several months here and there, which means the time series has gaps and is not long enough (less than 20 years) to compute TWS climatology, hence, available GRACE based drought index should be used carefully. (d) The trade-off between temporal resolution and the spatial resolution of GRACE fields is a big obstacle in using GRACE for real-time regional applications.
The above mentioned issues with GRACE products are currently being investigated by researchers, for example, (a) spatial downscaling of GRACE by assimilating it with other hydrological observations to produce higher spatial resolution TWS products (Zaitchik et al., 2008; Miro and Famiglietti, 2018). (b) With the launch of GRACE Follow On mission, we are expecting another decade long GRACE data, and several efforts are going on to reconstruct GRACE TWS for filling the data gaps and reconstructing TWS prior to 2002 (Humphrey and Gudmundsson, 2019; Li W. et al., 2019; Li et al., 2020), and (c) novel daily and weekly GRACE products have been developed and shown to detect short-lived extreme events, such as floods (Kvas et al., 2019). Therefore, ongoing improvements will make GRACE even more effective for drought research. There is no doubt that GRACE based drought indices are an excellent tool to study hydrological and agricultural drought, but comparing them with other traditional drought indices to seek validation is probably not a right approach. Can we expect a perfect match between Palmer Drought Severity Index (PDSI) and Soil Moisture Index (SMI) or between Standardized Precipitation Index (SPI) and standardized runoff index (SRI)? The answer is “no” because these indices deal with different variable that are related to drought differently (Zargar et al., 2011). Hence, GRACE should be used as an independent or a complementary indicator for droughts (Zhao et al., 2017).
Using a longer TWS time series for obtaining TWS climatology should be explored. Existing TWS reconstructions for period before GRACE, such as from Humphrey and Gudmundsson (2019), are not useful as they are able to reconstruct only the inter-annual variability in TWS and the seasonal part is derived from short GRACE time series (Humphrey and Gudmundsson, 2019). GRACE can already tell us about the water mass loss in a period of time (Tapley et al., 2019), we must create a framework that can use this information along with other hydro-meteorological observations and forecasts to predict the probability of drought. This is challenging because both natural variability and human intervention are responsible for drought (Van Loon et al., 2016), and separating their magnitude requires excellent understanding of: (a) relation between climatic variability and regional hydrology, (b) how this relation is affected by change in climate and land use land cover, and (c) anthropogenic response to dry conditions. Using surface observations alone or relying on models will only provide us limited insight. GRACE, although poor in its spatio-temporal resolution, provides us additional information, i.e., TWS including groundwater storage change, affected by both natural variability and human interventions signal. Therefore, carefully integrating GRACE with other hydro-climatic observations and models can greatly benefit drought studies, as was recently shown by Yang et al. (2020). A few projects (such as EGSIEM and GlobalCDA) have been undertaken with this objective, but we need more collaborative efforts between research communities engaged in drought and GRACE to obtain novel insights into prediction of drought, assessing its impact on water resources, calculating recovery time, and predicting its socio-economic cost in a changing climate.
The original contributions presented in the study are included in the article/supplementary material, further inquiries can be directed to the corresponding author/s.
The author confirms being the sole contributor of this work and has approved it for publication.
BV was supported by the Marie Skłodowska-Curie Individual Fellowship (msca-if) under Grant Agreement 841407 (CLOSeR).
The author declares that the research was conducted in the absence of any commercial or financial relationships that could be construed as a potential conflict of interest.
Dahle, C., Flechtner, F., Murböck, M., Michalak, G., Neumayer, H., Abrykosov, O., et al. (2018). Grace Geopotential gsm Coefficients gfz rl06. v. 6.0. doi: 10.5880/GFZ.GRACE_06_GSM
Devaraju, B., and Sneeuw, N. (2016). On the spatial resolution of homogeneous isotropic filters on the sphere,” in VIII Hotine-Marussi Symposium on Mathematical Geodesy: Proceedings of the Symposium in Rome, ed N. Sneeuw, P. Novák, M. Crespi, and F. Sansò (Rome: Springer International Publishing), 67-73. doi: 10.1007/1345_2015_5
Forootan, E., Khaki, M., Schumacher, M., Wulfmeyer, V., Mehrnegar, N., Van Dijk, A., et al. (2019). Understanding the global hydrological droughts of 2003–2016 and their relationships with teleconnections. Sci. Tot. Environ. 650, 2587–2604. doi: 10.1016/j.scitotenv.2018.09.231
Frappart, F., and Ramillien, G. (2018). Monitoring groundwater storage changes using the gravity recovery and climate experiment (grace) satellite mission: a review. Remote Sens. 10:829. doi: 10.3390/rs10060829
Hosseini-Moghari, S.-M., Araghinejad, S., Ebrahimi, K., and Tourian, M. J. (2019). Introducing modified total storage deficit index (mtsdi) for drought monitoring using grace observations. Ecol. Indic. 101, 465–475. doi: 10.1016/j.ecolind.2019.01.002
Houborg, R., Rodell, M., Li, B., Reichle, R., and Zaitchik, B. F. (2012). Drought indicators based on model-assimilated gravity recovery and climate experiment (GRACE) terrestrial water storage observations. Water Resour. Res. 48:W07525. doi: 10.1029/2011WR011291
Humphrey, V., and Gudmundsson, L. (2019). Grace-rec: a reconstruction of climate-driven water storage changes over the last century. Earth Syst. Sci. Data 11, 1153–1170. doi: 10.5194/essd-11-1153-2019
Klees, R., Zapreeva, E. A., Winsemius, H. C., and Savenije, H. H. G. (2007). The bias in GRACE estimates of continental water storage variations. Hydrol. Earth Syst. Sci. 11, 1227–1241. doi: 10.5194/hess-11-1227-2007
Kusche, J. (2007). Approximate decorrelation and non-isotropic smoothing of time-variable GRACE-type gravity field models. J. Geodesy 81, 733–749. doi: 10.1007/s00190-007-0143-3
Kvas, A., Gruber, C., Gouweleeuw, B., Güntner, A., Mayer-Gürr, T., and Flechtner, F. (2019). The EGSIEM near real-time service based on grace mission data–review and outlook. Montreal, QC. Avialable online at: https://graz.pure.elsevier.com/
Leblanc, M. J., Tregoning, P., Ramillien, G., Tweed, S. O., and Fakes, A. (2009). Basin-scale, integrated observations of the early 21st century multiyear drought in Southeast Australia. Water Resour. Res. 45:W04408. doi: 10.1029/2008WR007333
Li, B., Rodell, M., Kumar, S., Beaudoing, H. K., Getirana, A., Zaitchik, B. F., et al. (2019). Global grace data assimilation for groundwater and drought monitoring: advances and challenges. Water Resour. Res. 55, 7564–7586. doi: 10.1029/2018WR024618
Li, F., Kusche, J., Rietbroek, R., Wang, Z., Forootan, E., Schulze, K., et al. (2020). Comparison of data-driven techniques to reconstruct (1992–2002) and predict (2017–2018) grace-like gridded total water storage changes using climate inputs. Water Resour. Res. 56:e2019WR026551. doi: 10.1029/2019WR026551
Li, W., Wang, W., Zhang, C., Wen, H., Zhong, Y., Zhu, Y., et al. (2019). Bridging terrestrial water storage anomaly during GRACE/GRACE-FO gap using SSA method: a case study in China. Sensors 19:4144. doi: 10.3390/s19194144
Liu, Q., Zhang, S., Zhang, H., Bai, Y., and Zhang, J. (2020). Monitoring drought using composite drought indices based on remote sensing. Sci. Tot. Environ. 711:134585. doi: 10.1016/j.scitotenv.2019.134585
Liu, X., Feng, X., Ciais, P., Fu, B., Hu, B., and Sun, Z. (2020). Grace satellite-based drought index indicating increased impact of drought over major basins in China during 2002–2017. Agric. For. Meteorol. 291:108057. doi: 10.1016/j.agrformet.2020.108057
Long, D., Longuevergne, L., and Scanlon, B. R. (2015). Global analysis of approaches for deriving total water storage changes from GRACE satellites. Water Resour. Res. 51, 2574–2594. doi: 10.1002/2014WR016853
Long, D., Scanlon, B. R., Longuevergne, L., Sun, A. Y., Fernando, D. N., and Save, H. (2013). GRACE satellite monitoring of large depletion in water storage in response to the 2011 drought in Texas. Geophys. Res. Lett. 40, 3395–3401. doi: 10.1002/grl.50655
Luthcke, S. B., Sabaka, T., Loomis, B., Arendt, A., McCarthy, J., and Camp, J. (2013). Antarctica, Greenland and Gulf of Alaska land-ice evolution from an iterated GRACE global mascon solution. J. Glaciol. 59, 613–631. doi: 10.3189/2013JoG12J147
Miro, M. E., and Famiglietti, J. S. (2018). Downscaling GRACE remote sensing datasets to high-resolution groundwater storage change maps of California's Central Valley. Remote Sens. 10:143. doi: 10.3390/rs10010143
Mishra, A. K., and Singh, V. P. (2010). A review of drought concepts. J. Hydrol. 391, 202–216. doi: 10.1016/j.jhydrol.2010.07.012
Modanesi, S., Massari, C., Camici, S., Brocca, L., and Amarnath, G. (2020). Do satellite surface soil moisture observations better retain information about crop-yield variability in drought conditions? Water Resour. Res. 56:e2019WR025855. doi: 10.1029/2019WR025855
Parker, D., Folland, C., Scaife, A., Knight, J., Colman, A., Baines, P., et al. (2007). Decadal to multidecadal variability and the climate change background. J. Geophys. Res. Atmos. 112:D18115. doi: 10.1029/2007JD008411
Peltier, W. R., Argus, D. F., and Drummond, R. (2015). Space geodesy constrains ice age terminal deglaciation: the global ICE-6GC (VM5a) model. J. Geophys. Res. Solid Earth 120, 450–487. doi: 10.1002/2014JB011176
Ramillien, G., Famiglietti, J. S., and Wahr, J. (2008). Detection of continental hydrology and glaciology signals from GRACE: a review. Surv.Geophys. 29, 361–374. doi: 10.1007/s10712-008-9048-9
Rangelova, E., van der Wal, W., Braun, A., Sideris, M. G., and Wu, P. (2007). Analysis of Gravity Recovery and Climate Experiment time-variable mass redistribution signals over North America by means of principal component analysis. J. Geophys. Res. Earth Surf. 112:F03002. doi: 10.1029/2006JF000615
Save, H., Bettadpur, S., and Tapley, B. D. (2016). High-resolution csr grace rl05 mascons. J. Geophys. Res. Solid Earth 121, 7547–7569. doi: 10.1002/2016JB013007
Schlosser, C. A., Strzepek, K., Gao, X., Fant, C., Blanc, É., Paltsev, S., et al. (2014). The future of global water stress: an integrated assessment. Earths Future 2, 341–361. doi: 10.1002/2014EF000238
Sneeuw, N., Lorenz, C., Devaraju, B., Tourian, M. J., Riegger, J., Kunstmann, H., et al. (2014). Estimating runoff using hydro-geodetic approaches. Surv. Geophys. 35, 1333–1359. doi: 10.1007/s10712-014-9300-4
Sun, A. Y. (2013). Predicting groundwater level changes using GRACE data. Water Resour. Res. 49, 5900–5912. doi: 10.1002/wrcr.20421
Sun, Z., Zhu, X., Pan, Y., Zhang, J., and Liu, X. (2018). Drought evaluation using the grace terrestrial water storage deficit over the yangtze river basin, China. Sci. Tot. Environ. 634, 727–738. doi: 10.1016/j.scitotenv.2018.03.292
Swenson, S., and Wahr, J. (2006). Post-processing removal of correlated errors in GRACE data. Geophys. Res. Lett. 33:L08402. doi: 10.1029/2005GL025285
Tapley, B. D., Watkins, M. M., Flechtner, F., Reigber, C., Bettadpur, S., Rodell, M., et al. (2019). Contributions of GRACE to understanding climate change. Nat. Clim. Change 9, 358–369. doi: 10.1038/s41558-019-0456-2
Thomas, A. C., Reager, J. T., Famiglietti, J. S., and Rodell, M. (2014). A GRACE-based water storage deficit approach for hydrological drought characterization. Geophys. Res. Lett. 41, 1537–1545. doi: 10.1002/2014GL059323
Tourian, M. J., Sneeuw, N., and Bárdossy, A. (2013). A quantile function approach to discharge estimation from satellite altimetry (ENVISAT). Water Resour. Res. 49, 4174–4186. doi: 10.1002/wrcr.20348
Van Loon, A. F., Gleeson, T., Clark, J., Van Dijk, A. I., Stahl, K., Hannaford, J., et al. (2016). Drought in the anthropocene. Nat. Geosci. 9:89. doi: 10.1038/ngeo2646
Vishwakarma, B. D. (2017). Understanding and repairing the signal damage due to filtering of mass change estimates from the GRACE satellite mission (Ph.D. thesis). University of Stuttgart, Stuttgart, Germany.
Vishwakarma, B. D., Devaraju, B., and Sneeuw, N. (2016). Minimizing the effects of filtering on catchment scale GRACE solutions. Water Resour. Res. 52, 5868–5890. doi: 10.1002/2016WR018960
Vishwakarma, B. D., Devaraju, B., and Sneeuw, N. (2018). What is the spatial resolution of GRACE satellite products for hydrology? Remote Sens. 10:852. doi: 10.3390/rs10060852
Vishwakarma, B. D., Horwath, M., Devaraju, B., Groh, A., and Sneeuw, N. (2017). A data-driven approach for repairing the hydrological catchment signal damage due to filtering of GRACE products. Water Resour. Res. 53, 9824–9844. doi: 10.1002/2017WR021150
Vishwakarma, B. D., Jain, K., Sneeuw, N., and Devaraju, B. (2013). Mumbai 2005, Bihar 2008 flood reflected in mass changes seen by GRACE satellites. J. Indian Soc. Remote Sens. 41, 687–695. doi: 10.1007/s12524-012-0256-x
Wahr, J., Molenaar, M., and Bryan, F. (1998). Time variability of the Earth's gravity field: hydrological and oceanic effects and their possible detection using GRACE. J. Geophys. Res. Solid Earth 103, 30205–30229. doi: 10.1029/98JB02844
Watkins, M. M., Wiese, D. N., Yuan, D.-N., Boening, C., and Landerer, F. W. (2015). Improved methods for observing Earth's time variable mass distribution with GRACE using spherical cap mascons. J. Geophys. Res. Solid Earth 120, 2648–2671. doi: 10.1002/2014JB011547
West, H., Quinn, N., and Horswell, M. (2019). Remote sensing for drought monitoring & impact assessment: progress, past challenges and future opportunities. Remote Sens. Environ. 232:111291. doi: 10.1016/j.rse.2019.111291
Wouters, B., Bonin, J., Chambers, D., Riva, R. E. M., Sasgen, I., and Wahr, J. (2014). Grace, time-varying gravity, earth system dynamics and climate change. Rep. Prog. Phys. 77:116801. doi: 10.1088/0034-4885/77/11/116801
Yang, P., Zhang, Y., Xia, J., and Sun, S. (2020). Identification of drought events in the major basins of central asia based on a combined climatological deviation index from grace measurements. Atmos. Res. 244:105105. doi: 10.1016/j.atmosres.2020.105105
Yirdaw, S. Z., Snelgrove, K. R., and Agboma, C. O. (2008). Grace satellite observations of terrestrial moisture changes for drought characterization in the Canadian prairie. J. Hydrol. 356, 84–92. doi: 10.1016/j.jhydrol.2008.04.004
Zaitchik, B. F., Rodell, M., and Reichle, R. H. (2008). Assimilation of GRACE terrestrial water storage data into a land surface model: results for the Mississippi river basin. J. Hydrometeorol. 9, 535–548. doi: 10.1175/2007JHM951.1
Zargar, A., Sadiq, R., Naser, B., and Khan, F. I. (2011). A review of drought indices. Environ. Rev. 19, 333–349.doi: 10.1139/a11-013
Keywords: drought, grace, water storage change, drought index, remote sensing
Citation: Vishwakarma BD (2020) Monitoring Droughts From GRACE. Front. Environ. Sci. 8:584690. doi: 10.3389/fenvs.2020.584690
Received: 17 July 2020; Accepted: 04 December 2020;
Published: 23 December 2020.
Edited by:
Nevil Wyndham Quinn, University of the West of England, United KingdomReviewed by:
Meng Zhao, University of California, Irvine, United StatesCopyright © 2020 Vishwakarma. This is an open-access article distributed under the terms of the Creative Commons Attribution License (CC BY). The use, distribution or reproduction in other forums is permitted, provided the original author(s) and the copyright owner(s) are credited and that the original publication in this journal is cited, in accordance with accepted academic practice. No use, distribution or reproduction is permitted which does not comply with these terms.
*Correspondence: Bramha Dutt Vishwakarma, YmQudmlzaHdha2FybWFAYnJpc3RvbC5hYy51aw==
Disclaimer: All claims expressed in this article are solely those of the authors and do not necessarily represent those of their affiliated organizations, or those of the publisher, the editors and the reviewers. Any product that may be evaluated in this article or claim that may be made by its manufacturer is not guaranteed or endorsed by the publisher.
Research integrity at Frontiers
Learn more about the work of our research integrity team to safeguard the quality of each article we publish.