- 1Environmental Change Institute, University of Oxford, Oxford, United Kingdom
- 2School of Geography and the Environment, University of Oxford, Oxford, United Kingdom
- 3Sussex Sustainability Research Programme/School of Global Studies, University of Sussex, Brighton, United Kingdom
- 4Department of Aquatic Sciences and Assessment, Swedish University of Agricultural Sciences, Uppsala, Sweden
Concerns have been raised about rising trends in surface water dissolved organic carbon (DOC) concentrations in UK upland catchments over the past decades. Several mechanisms have been proposed to explain these trends, including changes in climate and declines in sulfate deposition across Europe. Drier summers and wetter winters are projected in the UK, and there is an increasing interest in whether the rising trends of DOC would be continued or stabilized. In this paper, the INCA (INtegrated CAtchment) water quality model was applied to the upland catchment of the River Severn at Plynlimon in Wales and used to simulate the effects of both climate and sulfate deposition on surface water DOC concentrations. We introduced new parameter sets of INCA to explain enzymatic latch effect in peatlands during droughts. The model was able to simulate recent past (1995–2013) rising trends in DOC in Plynlimon. Climatic projections were employed to estimate the future trends on DOC in the uplands and to consider potential impacts on catchment management. The model was run with climatic scenarios generated using the weather@home2 climate modeling platform and with sulfate deposition scenarios from the European Monitoring and Evaluation Programme (EMEP) for 1975–2100. The modeling results show that the rising DOC trends are likely to continue in the near future (2020–2049) and the level of DOC concentrations is projected to stabilize in the far future (2070–2099). However, in the far future, the seasonal patterns of DOC concentrations will change, with a post-drought DOC surge in autumn months.
Introduction
While the peatlands cover a relatively small amount of the Earth's terrestrial area, they contain approximately one-third of the world's soil carbon pool (Gorham, 1991; Pastor et al., 2003) and about 40% of the UK's total soil carbon storage (UK National Ecosystem Assessment, 2011). Release of carbon from peatlands includes dissolved organic carbon (DOC) and carbon dioxide (CO2) in rivers, and direct release of CO2 and methane (CH4) to the atmosphere. Rising trends of DOC concentrations in freshwater system have been reported across northern, central Europe and eastern North America, particularly in the industrial areas (Monteith et al., 2007). The changes in the flux of DOC from the land to surface waters have been attracting much interest from academics (Freeman et al., 2001a, Evans, 2015) and water companies due to the problematic for water treatment (Ritson et al., 2014). Several mechanisms have been proposed to explain these trends including changes in climate and declines in sulfate deposition across Europe (Evans et al., 2005; Monteith et al., 2007). Fluvial DOC export determines a significant proportion of the peatland carbon balance, and widespread increases in DOC fluxes suggest that this carbon balance may be altering, with major implications for terrestrial carbon budgets. These increases appear to be driven by regional or global-scale environmental changes, although the key driving mechanisms have yet to be conclusively identified.
Major DOC increases have been observed over the timescales that have seen reductions in the acidity of precipitation, therefore, sulfur deposition is thought to have a significant effect by altering microbial populations and suppressing the formation of DOC under high deposition loading (Evans et al., 2006, 2012). Monteith et al. (2007) reported that the increasing DOC concentrations are in proportion to the rates at which atmospheric deposition of anthropogenic sulfur and sea salt are declining. As sulfur deposition controls soil water acidity, it leads to changes in DOC solubility in the soil (Evans et al., 2012). A recent study by Kang et al. (2018) investigated biogeochemical linkages between pH increase and DOC production in peatlands and demonstrated that increases of pH stimulate key enzyme activities, which involved the decomposition of peat, resulting in the enhancement of DOC concentrations in the pore water. This experiment supports the hypothesis that the recovery from acidification could cause the increase in DOC concentrations in freshwater ecosystems. Clark et al. (2005) discovered that DOC concentrations and sulfate dynamics in soil solution have a strong relationship under drought conditions in peat soils, which are a major source of DOC to surface waters. Climate-related mechanisms present a particular concern as they would imply that increasing atmospheric CO2 could lead to an increase in peatland DOC release (Freeman et al., 2004). Conversion of this DOC to CO2, by microbial or photochemical processes, could therefore create a positive feedback effect, raising atmospheric CO2 concentrations and stimulating the further release of DOC from wetlands. Other proposed drivers include factors related to the increase of air temperature (Freeman et al., 2001b), changes in precipitation and runoff (Tranvik and Jansson, 2002), and changes in land use (Yallop and Clutterbuck, 2009).
Under future climate conditions, drier summers and wetter winters are projected in the UK (Lowe et al., 2018). Changes in climatic conditions will affect catchment hydrology and river water quality (Whitehead et al., 2009). DOC production rates and mineralisation processes in pore water are sensitive biochemical processes directly affected by these changes (Bell et al., 2018). Drought conditions will consequently affect the carbon storage in peatlands and fluvial DOC concentrations caused by the “enzymatic latch” process (Freeman et al., 2001b, Fenner and Freeman, 2011). Drought events introduce oxygen into the system and change the redox conditions in peatlands, which controls DOC solubility. Organic carbon is not released during the drought, but subsequent re-wetting and re-flooding accelerate carbon losses from the peat to the atmosphere and the receiving waters.
The long-term monitoring of surface water quality networks in the UK has also revealed increasing DOC concentration trends in upland catchments since the late 1980s. DOC may be now stabilizing in catchments where reduced sulfur deposition has reduced ionic strength in soils. However, this would not be the case for peatlands that continue to leak stored sulfur. One upland catchment, Plynlimon, in the upper Severn catchment, is dominated by organomineral soils, with smaller areas of blanket peat so that the upward movement of DOC concentrations could continue for some years. In addition, extreme weather events could trigger occasional releases of DOC, flushing DOC in late summer and autumn storm events (Delpla et al., 2015). A DOC surge could be expected during storm events which could create problems downstream. Peatlands supply over a quarter of drinking water in the UK (ONS, 2019) and higher DOC concentrations in drinking water can cause human health issues as DOC reacts with the chlorine used in some drinking water treatment processes to produce potentially carcinogenic trihalomethanes (Chow et al., 2003). It affects watercolor, transparency, and pH, which all directly link to stream ecology and increases in DOC represent a change in the quality and ecology of streams, thereby creating difficulties from a Water Framework Directive (WFD) perspective (Whitehead et al., 2006). Additionally, the removal of DOC in raw water in the treatment plant is costly for water utility companies (Ritson et al., 2014, Ritson et al., 2016). There has been considerable debate about the changes in DOC concentration in the upland UK, and the Acid Water Monitoring Network report rising trends in many upland peat catchments across the UK.
Several DOC simulation models have been developed for both terrestrial and aquatic environments (de Wit et al., 2016). The INtegrated CAtchments model for Carbon (INCA-C) has been widely used to simulate seasonal and long-term patterns in soil and surface water DOC (Futter et al., 2007). Changes in climate, sulfate deposition and land management contribute to variability in surface water DOC concentrations, and INCA-C is capable of simulating complex, interdependent processes in catchment-scale peatland as a multi-parameter process-based model. The initial model development and application was to catchments in Canada (Futter et al., 2007) and further applications including Norway (Futter and de Wit, 2008), Finland (Futter et al., 2009), Ireland (O'Driscoll et al., 2018) and several UK catchments (Xu et al., 2020).
Whether or not rising trends of DOC in upland catchments will continue, or will stabilize, is an important consideration for peatland catchment responses to future drought and acidic deposition, in addition to the implications for downstream drinking water treatment and supply. The aim of this paper is to present an assessment of potential future climatic and acidic deposition impacts on river water quality in peatland catchments in the upper Severn that has been showing large rising trends of DOC over the last decades. The INCA-C model was first used to simulate current flow and DOC concentrations and fluxes. Here, we introduce new parameter sets of INCA-C to simulate the enzymatic latch effect in peatlands during severe droughts. A large ensemble of climate scenarios generated by weather@home2 project and acidic deposition scenarios produced by Emissions Monitoring and Evaluation Programme (EMEP) were then projected to drive INCA-C model simulations.
Study Area
The study area, Plynlimon sub-catchments, are headwater catchments of the River Severn in the UK. Two sub-catchments are the Hafren and the Hore at between 300 and 700 m above sea level (Figure 1). They are 5.5 and 3.2 km2 in area, respectively. Peatland, semi-natural grassland, areas of plantation conifer forest (mainly Sitka spruce with some Norway spruce), are all represented within Plynlimon catchments (Neal et al., 2011). The upper parts of the Hafren and the Hore comprise hilltop plateau dominated by up to 2 m of deep blanket peat whereas the valley bottoms include areas of seasonally saturated peat and gley soil. The Upper and Lower Hafren represent a single main channel system with a clear divide between the upstream blanket peat and the downstream forested peaty podzols areas. The upper and lower parts of the Hore were harvested in 1985–1988 and 2006–2009, respectively. Logging residue was left on the site after clear-felling, and then the area was subsequently replanted with conifer forest. The catchment soils were plowed and drained by creating ditches in the between harvest periods, so consequently “flashy” catchments were created with rapid responses to rainfall and enhanced sediment transport rates.
According to the UK National River Flow Archive (NRFA), the Plynlimon receives an average annual rainfall of 2,653 mm (computed in the period of 1961–2015, with a minimum of 1,855 mm in 1976 and a maximum of 3,801 mm in 2000). The annual average temperature is 9.2°C (1995–2015, a minimum of 7.9°C in 1996 and a maximum of 9.8°C in 2014). The average summer temperature is 14.7°C, and the average winter temperature is 3.8°C. The land uses of the Plynlimon catchments were categorized as forest (both managed and unmanaged), unimproved grassland (i.e., unfertilised grassland), and peatland. The Hore catchment was divided into two reaches, upper Hore and lower Hore. The land use of Hore catchment is predominantly forest cover with 60% of forest, 31% of unimproved grassland and 9% of peatland in upper Hore. Lower Hore has a similar land use to upper Hore. The Hafren catchment was divided into three reaches, upper Hafren, lower Hafren and Severn at Plynlimon flume where the confluence of Hore and Hafren and an inflow of river Severn and includes Tanllwyth stream. The land use of upper Hafren is 90% of peatland and 10% of unimproved grassland. Forest cover dominates from lower Hafren and Plynlimon flume. Figure 1 shows the catchment area and land use proportions for the Plynlimon catchments.
The trend of DOC concentrations in the upper Severn catchments is shown in Figure 2, showing a gradually increasing trend since the late 1990s with high peak concentrations in 2009 (Table 1). The monitoring infrastructure was established initially to investigate the impacts of upland conifer plantations on the hydrological cycle in the 1960s. With growing awareness and concern about acid deposition and the effect of forest management practices on river chemistry, hydro-chemical measurements have generated a 30-year uninterrupted record from weekly to monthly which includes pH, alkalinity, nutrients, major cations, anions, trace metals, dissolved organic carbon and dissolved organic nitrogen. The Plynlimon catchment experiment is one of the longest-running studies in Europe, producing extensive environmental data (Neal et al., 2011; Robinson et al., 2013) with several decades of high-quality climate, flow and water quality data. As an extensive data set, it has helped to improve the understandings of many aspects of hydrology, water chemistry and the consequences of changing the land cover in the catchment (Robinson et al., 2013).
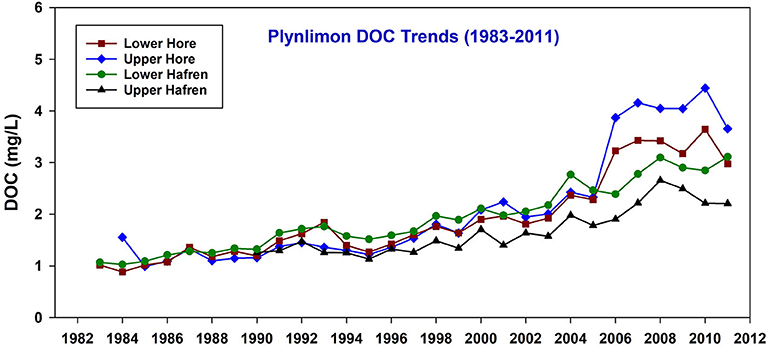
Figure 2. Plynlimon DOC Trends (1983–2011) with annual mean DOC (Neal et al., 2013 and Norris et al., 2017).
Materials and Methods
Model Data
Table 2 shows the data used in this study for model application with temporal and spatial coverage. The meteorological data, daily precipitation and temperature, were obtained from the UK Met office (Met Office, 2012). Records of continuous daily water discharge at the Hore and the Hafren were obtained from the National River Flow Archive (NRFA). Discharge records after 2009 were obtained from the Plynlimon experimental site in the UK Center for Ecology and Hydrology (CEH). Weekly water quality data from the Plynlimon experimental site were also provided by CEH (Neal et al., 2013, Norris et al., 2017).
The Integrated Catchments Model for Carbon (INCA-C)
The INCA-C model was employed to reproduce and simulate carbon dynamics in the Plynlimon catchment. INCA-C is a dynamic, semi-distributed catchment scale process-based model of DOC concentrations and fluxes in surface waters (Futter et al., 2007). The INCA model was initially developed to simulate nitrogen (Whitehead et al., 1998a,b) and phosphorus (Wade et al., 2002a) at a catchment scale. Several sub-models were later added, including carbon (Futter et al., 2007), sediment transport and soil erosion (Lazar et al., 2010), pathogens (Whitehead et al., 2016), an organic contaminant model (Lu et al., 2016), and microplastics (Nizzetto et al., 2016).
The INCA-C model simulates daily DOC concentrations, flux and water flow in streams. Fluxes and concentrations of DOC are simulated by solving mass balance equations for terrestrial processes while simultaneously solving flow equations. INCA-C requires a daily time series of soil moisture deficit (SMD), hydrological effective rainfall (HER), air temperature and precipitation as inputs. The latter two input datasets were estimated using a semi-distributed rainfall-runoff model, PERSiST (Precipitation, Evapotranspiration and Runoff Simulator for Solute Transport model, Futter et al., 2014). PERSiST simulates water fluxes in a catchment scale, with a series of user-defined buckets indicating different hydrological processes such as snowmelt, surface runoff generation, soil water storage, groundwater and streamflow. It is particularly designed to provide input time series for the INCA models. In addition, spatial data describing catchment land cover and soil properties, the location of point sources, abstraction and effluent discharge are required to run INCA-C. INCA-C then provides daily time series of flow, DOC and DIC (dissolved inorganic carbon) concentrations at each reach boundary, as well as profiles along with descriptive statistics of these variables at selected sites. INCA-C is calibrated using the time series of observed and modeled daily discharge and point estimates of in-stream DOC concentrations to minimize the differences between observed and modeled values.
Highlighted Processes for Acidic Deposition and Enzymatic Latch
A detailed description and model equations of INCA-C terrestrial and in-stream carbon processing routines, hydrological routing, and climate controls are well-documented by Futter et al. (2007). The initial version of the model has been further modified, and this section will present the highlighted processes related to the analysis in this study.
In the INCA-C model, all carbon procession in soils and surface waters are modeled as a series of first-order differential equations. All rate coefficients in soil layers are dependent on moisture saturation status and soil temperature. INCA-C simulates in-soil temperature by using observed air temperature. The rate coefficient for soil moisture effect is a linear function of soil moisture content. For example, in-soil carbon transformation operates at a maximum rate when the simulated SMD is at zero, and cease when the simulated SMD is greater than SMDmax. SMDmax is a calibrated threshold of the maximum soil moisture deficit at which carbon transformations may occur.
The following simplified equations explain the effect of sulfate concentrations and the enzymatic latch on organic carbon sorption and desorption in soil solution. The modification of the sulfate effect to INCA-C has been initially introduced by Futter et al. (2009) and successfully applied to a boreal catchment in Finland. The enzymatic latch process is an innovative additional application in this paper. The same processes occur in the organic and mineral soil horizons with different rate constants.
As mentioned above, the change in mass of DOC is controlled as a combination of soil moisture (kM, d−1), soil temperature (kT, d−1) effects and the mass of organic carbon sorbed (c2DOC, kg C d−1 and desorbed (c1SOC, kg C d−1. The base rates of the sorption(c2) and desorption(c1) coefficients are estimated during the model calibration. To simulate soil water sulfate concentrations ([]), atmospheric deposition is used as a surrogate in soil solution. Equations 1, 2 are simplified equations of the changes in the mass of DOC and SOC (solid organic carbon) with functions of soil chemistry controls on organic carbon sorption and desorption rates.
Equation 1
Equation 2
The sulfate effect () is added on the rate at which DOC is transformed to SOC. At high sulfate concentrations, the transformation from DOC to SOC will be more rapid. Increasing sulfate concentrations should increase the rate at which DOC is transformed to SOC, and hence lower the mass of DOC in solution (Equation 1). The equation for the change in mass of SOC is effectively the reverse of that for DOC (Equation 2). When b1 is at zero, the effect of is turned off in simulation where organic matter changes from the dissolved to the solid phase.
The “enzymic latch” mechanism (Freeman et al., 2001b) has been proposed as a mechanism to explain an increase of DOC concentrations observed following a severe drought in peatlands (Worrall and Burt, 2004). The mechanism is simulated in INCA-C as an increase in the rate of SOC to DOC transformation when the soil moisture deficit drops below a critical threshold.
The enzymatic latch effect is triggered by a drought event by increasing the rate at which SOC is transformed to DOC. Drought is defined in INCA-C when simulated SMD is exceeding a critical threshold (l2, mm). The maximum effect of the enzymatic latch is immediately following a drought event and gradually declines to zero. The decay in enzymatic latch effect is simulated as a linear function of the number of days (n) since the drought threshold was crossed (tCrit) divided by the duration of the latching effect in days (365 x l0). l0 indicates a return period (years) of the enzymatic latch. In the event that another drought event occurs in which the SMD exceeds the critical threshold (l2), the number of days since crossing the drought threshold (tCrit) is reset to 0. The enzymatic latch multiplier (lMult) is defined in Equation 3. It should be initialized to a value of 0.
Equation 3
Since both sulfate effect and enzymatic latch effect are associated with the same process, the changes in the mass of DOC and SOC, Equations 1, 2 can be updated accordingly to Equations 4, 5, respectively. The rate of production of DOC from SOC is controlled by the desorption(c1) coefficient and the enzymatic latch effect (lMultl1), where is the enzymatic latch rate coefficient. The rate of DOC transformation to SOC is controlled by the sorption(c2) coefficient and the sulfate effect (). The range of parameters is achieved during the calibration process.
Equation 4
Equation 5
Model Parameterisation and Generalized Sensitivity Analysis
The Calibration Procedure
The INCA-C model has been applied to the whole upper Severn Catchment at Plynlimon using a long run of data from 1995 to 2013 to calibrate and validate the model. The period was split in two: 2000–2013 for calibration, and 1995–1999 for validation. The period of model evaluation was set according to the availability of daily meteorological inputs. Given that the two catchments have quite a different land uses, separate INCA-C models were set up for the two catchments to compare catchment responses under a changing climate. Reach boundaries were drawn at Plynlimon experimental monitoring stations where long-term flow and water quality data were available. Manual calibrations were carefully conducted in which land phase hydrology and carbon processing parameters were allowed to vary so as to observe the correspondence between modeled and observed stream flows and DOC. The initial values of parameters and those ranges were found from the literature (Futter et al., 2007). The manual calibration was repeated and conducted until the Nash-Sutcliffe (NS) statistics (Nash and Sutcliffe, 1970) showed a reasonable agreement between simulated and observed values of flow and DOC. The parameter sets derived from manual calibrations were used as the starting point for a Monte Carlo analysis in which selected carbon processing and hydraulic parameters which could affect modeled DOC concentrations were allowed to vary by ± 20%. The Monte Carlo simulation produced an ensemble of 10,000 model runs, and those results were assessed to find the best performing parameter sets. Model performance was assessed using the sum of Nash-Sutcliffe statistics representing correspondence between modeled and observed DOC in upper and lower Hafren and upper and lower Hore, respectively. Best performing model runs were selected and used for further analysis.
General Sensitivity Analysis
A General Sensitivity Analysis (Hornberger and Spear, 1980; Spear and Hornberger, 1980) using Monte Carlo Simulations was applied in order to investigate model complexity and understand parameter influence on model simulations. The method is also conversely to inform those parameters that appear to exert little influence on model results as well. The main strategy is to incorporate uncertainty in the model simulation by specifying the parameter values from probability distribution functions, rather than point values. The Monte Carlo simulations are employed with chosen parameter values from the specified distributions, which should reflect the feasible parameter ranges. The results of Monte Carlo simulations are classified into those that are considered behavioral and non-behavior with respect to the pre-defined criteria set. The definition of behavior and non-behavior is problem-dependent, and the criteria can be for general trends or extreme events. The each of model results from the Monte Carlo parameter sets is compared with the observed values via the pre-defined behavior criteria algorithm (in this study, increasing trends in DOC concentrations) in order to determine the occurrence or non-occurrence of the required behavior. Then, the parameter sets will be classified to the one associated with the occurrence of behavior (B) and one with the non-behavior(N). Similar methodologies have been applied to provide a probabilistic procedure for model calibration and to identify critical parameter uncertainties in phytoplankton models (Whitehead and Hornberger, 1984), in a model application of phosphorus and macrophyte dynamics in River Kennet (Wade et al., 2002b), and in new model development of dissolved oxygen, Q2 (Cox and Whitehead, 2005).
The Hornberger and Spear's generalized sensitivity analysis looks for the difference between the behavioral and non-behavioral sets for each parameter. It does so by comparing the cumulative distribution of that parameter in each set. Where there is a strong difference between the two distributions for a parameter, it may be concluded that the simulations are sensitive to those parameters. If the two distributions are very similar, it may be concluded that the simulations are not very sensitive to those parameters. A quantitative measure of the difference between two distributions can be calculated using the non-parametric Kolmogorov–Smirnov d statistic (dm,n). The Kolmogorov-Smirnov two sample test is computed as following Equation 6:
Equation 6
where Sn and Sm are the sample distribution functions corresponding to the behavior(m) and non-behavior(n) values of a given model parameter and this statistic is the same as that used in the Spear and Hornberger (1980). The value of dm,n, d statistic, can be used as an index of relative difference for model parameters. The highest dm,n value among all parameter set is the most influent model parameter. This approach is a nonparametric method of sensitivity analysis in that it makes no prior assumptions about the variation or covariation of different parameter values, but only evaluates sets of parameter values in terms of their performance.
In this paper, the aim of the behavior analysis is specific to understand which particular parameters will have a significant influence on the rising trends of modeled DOC concentrations. The general sensitivity analysis was applied to the Hore and Hafren INCA carbon models, respectively. Based on a prior general sensitive analysis of the INCA Carbon model (Futter et al., 2007, Futter and de Wit, 2008), following carbon and non-carbon processing parameters were identified as the most significantly influential on model behaviors and considered to the analysis:
- In Forest cover, organic layer: DOC mineralisation rate, the rate of change in mass of DOC to SOC, the rate of change in mass of SOC to DOC, retention volume of organic layer, minimum organic layer flow
- In Forest cover, both organic and mineral layer: soil temperature rate multiplier, the rate of change in mass of SOC to DOC
- In Peatland cover, organic layer: retention volume of organic layer, minimum organic layer flow
- In Peatland cover, mineral layer: the rate of change in mass of DOC to SOC
- In Peatland cover, both organic and mineral layer: the rate of change in mass of SOC to DOC, and maximum SMD at which carbon processing can occur.
In addition, parameters related to the enzymatic latch process and sulfate multipliers were also included in the analysis. The feasible space of model parameters was drawn and sampled randomly to generate 10,000 different parameter sets. The calibrated INCA-C model was then run with each of these parameter sets. The 2000 best performing model runs were retained for further sensitivity analysis. Model performance was assessed using the sum of the NS statistics representing correspondence between modeled and observed DOC in the Upper and Lower reaches for the Hafren and Hore.
Projected Future Climate
In this study, climate scenarios generated by the weather@home system (Massey et al., 2015, Guillod et al., 2018) were applied to explore the potential impacts of climate change on hydrology, the carbon in catchments. The modeling system- weather@home (hereafter, w@h) consists of a global climate model (GCM) with a nested regional climate model (RCM) driven by sea surface temperatures as well as other forcings. It enables the production of a large number of ensembles of weather events with the help of volunteers distributed computing across the world. In this model application, weather@home2 (hereafter w@h2, which includes an improved land surface scheme) was used to generate 30-year-long weather time series of rainfall and temperature projections for three different periods. The data set is specifically designed to support a risk-based approach for the study of extreme events such as drought and heavy precipitation, both of which require the spatial consistency of hydro-meteorological data sets (Guillod et al., 2018). The scenarios in each future time slice all follow the Representative Concentration Pathway 8.5 (RCP8.5) and sample the range of sea surface temperatures and sea ice changes from CMIP5 (Coupled Model Intercomparison Project Phase 5) models. A number of daily and (or) monthly output variables are available from the RCM including temperature, precipitation, surface air humidity, mean sea level pressure, and potential evaporation. To assess the worst possible future condition of water quality, the RCP8.5 was selected as it is the most severe scenario among the current available future scenarios. Of particular relevance for water quality modeling is the changes in intense rainfall, which can affect leaching and drought, which influences chemical retention times and river flows.
From a 25 × 25 km grid over Europe, 100-time series are available for each of time slices which represent baseline (BL, 1975–2004), near future (NF, 2020–2049), and far future (FF, 2070–2099) climatic scenarios. Outputs from grid cells include Plynlimon catchment in Wales were averages to generate input time series for water quality modeling application (Figure 3).
The precipitation data was bias-corrected in order to reproduce the patterns and distributions of observed data better, and a linear approach was applied (Guillod et al., 2018). The bias correction method was conducted by using spatially-variable monthly bias-correction factors, obtained by the ratio of the modeled and observed monthly average precipitation. However, very little bias was observed from temperature, so temperature bias was not explicitly corrected. More details about weather@home2 data and bias-correction method can be found in Guillod et al. (2017) and Guillod et al. (2018). Figure 4 shows the comparison of bias-corrected precipitation (purple) and raw precipitation from w@h2 (gray) with observed precipitation. A dry bias during summer months on precipitation was well-corrected.
Sulfate Deposition Scenarios
Deposition scenarios from the Emissions Monitoring and Evaluation Programme (EMEP) were applied for this study to include the impacts of historical, present, and future acidic deposition. Historical sulfate deposition time series from 1970 to 2010 on a 50 × 50 km grid resolution was taken from the output of the EMEP/MSC-W model, as well as the future projection on the depositions until 2030 was also provided under the Gothenburg Protocol Currently Legislated Emissions (CLE) scenario (Posch et al., 2007). The chemical transport model developed at the Meteorological Synthesizing Center–West (MSC-W) is called the EMEP/MSC-W model. After 2030, the deposition is assumed to remain constant until 2090. More information on EMEP data can be found in Schöpp et al. (2003).
Reduction in acidic depositions is believed to have helped acidification recovery of European rivers and lakes (Skjelkvåle et al., 2003). As explained in the previous section, organic matter solubility in the INCA-C model is controlled by the concentrations of strong acid anions in soil solution (Futter et al., 2009, 2011). As soil solution data are not readily available, sulfate deposition data are assumed to be a reasonable surrogate for soil solution strong acid anions concentrations. Deposition for the forest land cover type was applied as an input to the modeling study. A smoothed sulfate deposition time series was created using estimates of average monthly wet sulfate deposition. The linearly interpolated monthly sulfate loadings were multiplied to daily precipitation depth to produce monthly average deposition values. These monthly deposition estimates were then assumed to be representative of the soil solution concentration throughout the soil profile. Estimated deposition showed that the peak was in the 1980s and rapidly declined since then. For the calibration, a single deposition series was provided. For the climate projections, 300 deposition time series were produced according to each input precipitation for each climate realizations for each of w@h2 time slices (baseline, near future and far future).
Results and Discussions
Model Evaluation
The Monte Carlo simulation produced an ensemble of 10,000 model runs and those results were assessed based on observed values of flow and water quality (DOC) at reach two for both the Hore and Hafren catchments (Lower Hore and Lower Hafren). The best model parameter set was selected for the Hore and Hafren model and used for the rest of the study. A combination of model evaluation measures was used to determine the best performance model including (a) visual assessment of time series, (b) model performance statistics and (c) total DOC loads estimation, and selected model performance was described.
Figure 5 shows the calibration and validation results of flow and DOC concentrations at Lower Hafren and Lower Hore. It is seen that the models are successfully representing a temporal pattern of DOC concentration and flow. The model simulations did not always capture the magnitude of peaks in DOC concentration. However, the models were able to capture the timing of peaks and seasonal patterns of DOC concentrations.
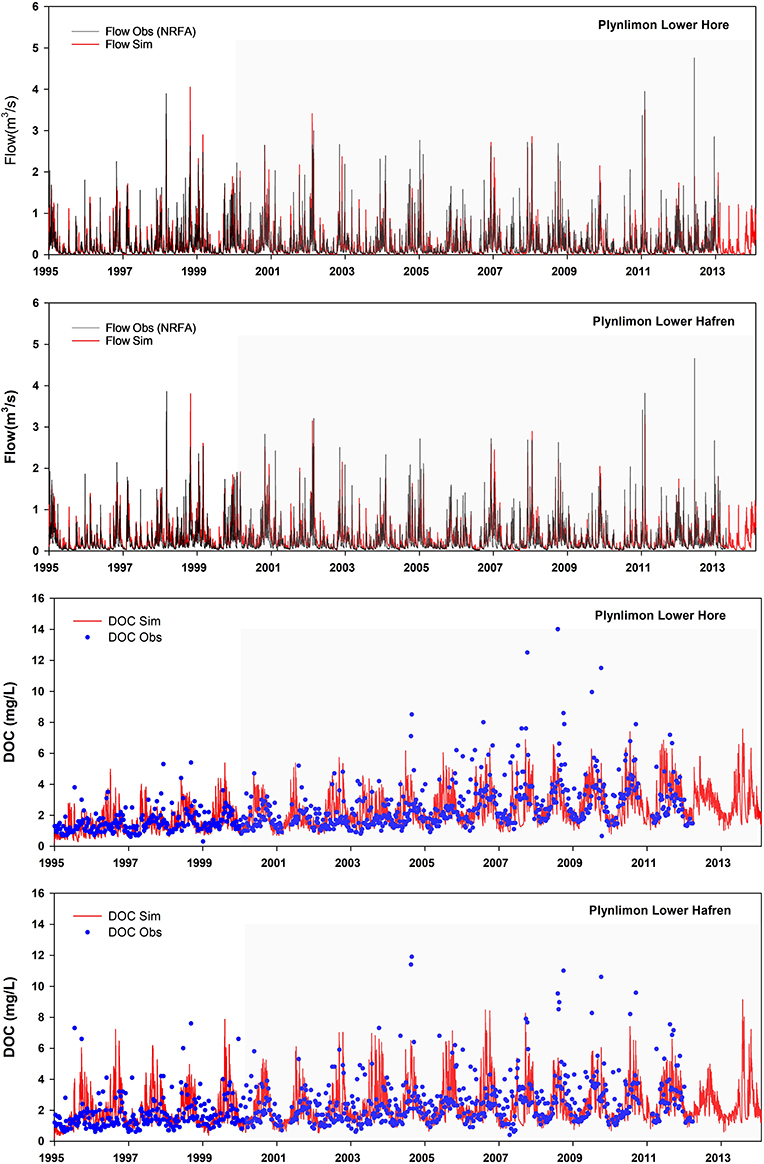
Figure 5. INCA-C calibration and validation results (Flow and DOC) at two locations in Plynlimon catchment. The gray-shaded area is the time period used for calibration. The unshaded area is used for validation.
Table 3 shows the performance indices of the INCA model in Plynlimon catchments (1995–2013). The performances were measured by Nash-Sutcliffe Efficiency (NSE), log NSE for streamflow and percent bias (PBIAS) for DOC concentration. Perfect matching was represented by the value of one for NSE. A negative value of NSE means that the average observed value is a better predictor than the simulated values of model simulation. NSE is one of the most popular indices for evaluating models, but it is sensitive to high or low extreme values. Therefore, log-transformed discharge (log NSE) was evaluated to consider the variability balanced at low values (low flows). The NSE values of daily simulated streamflow in both the Hore and Hafren catchments show acceptable ranges for a whole period of model evaluation (1995–2013), 0.68 and 0.66, respectively. The log NSE values were improved from the values of NSE in this study, which is 0.73 and 0.68, respectively, for the Hore and Hafren catchments. The simulated daily flow provided an acceptable reproduction of the observed flow, including a low flow period, particularly given the flow in Plynlimon is generally small.
Concerning the DOC simulation, PBIAS is <10% bias for both catchments, and INCA-C results can be considered satisfactory. PBIAS measures the average tendency of the modeled values to be larger or smaller than observed counterparts. The optimum value is 0, and negative values indicate a model overestimation bias while positive values mean an underestimation of modeled values. As suggested by Moriasi et al. (2007), satisfactory simulation can be indicated by PBIAS from ±25% up to ±70% for water quality variables. The sulfate deposition data application was helpful to the model calibrations. Model calibrations without the sulfate deposition data were shown −42% and −16% of PBIAS values for the Hore and Hafren, respectively.
The monthly in-stream DOC flux (kg/day) was calculated to demonstrate whether the model reproduced similar seasonal loads. Figure 6 showed the simulated and observed monthly DOC loads, which yielded similar results and well-reproduced seasonal patterns. The precipitation-driven annual DOC fluxes showed a great agreement between observed and simulated DOC fluxes (Figure 7). The degree of fit in terms of fluxes load with an R2 of 0.71 (for Hore catchments) and 0.77 (for Hafren) are acceptable.
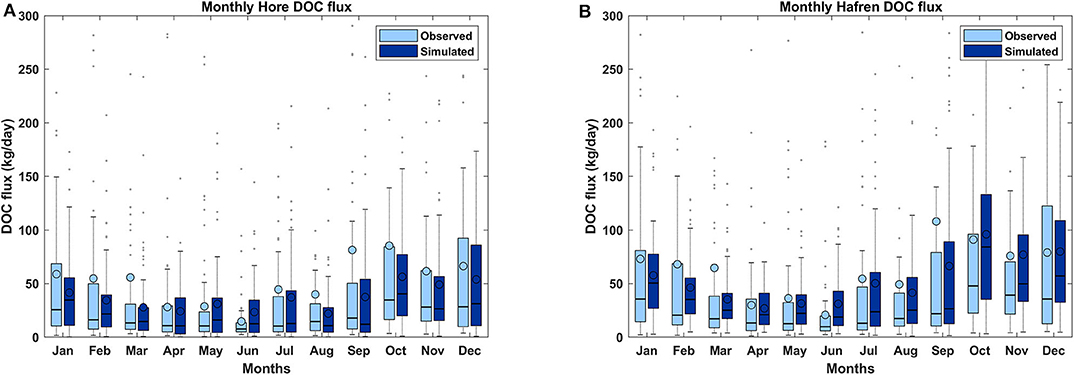
Figure 6. Simulated and Observed Monthly (in-stream) DOC load (kg/day) at Hore (A) and Hafren (B) catchments.
The many measures used for evaluating model performance showed generally satisfactory simulations, in terms of reproduction of the seasonal patterns of both streamflows and DOC concentrations over a long period, given the uncertainty that characterizes both model results and measurement data values. It suggests the model can be used with confidence in predicting future projections.
Model simulations were forced by a combination of gridded data for sulfate deposition and daily weather inputs and calibrated against point observations of streamflow and water chemistry. The gridded data used here provided complete, gap-free time series, which is a prerequisite for INCA modeling. The EMEP gridded data are widely used when modeling future trajectories of recovery from acidification (e.g., Posch et al., 2007, Futter et al., 2009). Within a region, e.g., the Severn River catchment, long-term temporal variation in sulfate deposition is higher than spatial variation. Thus, it was considered appropriate to use gridded EMEP data as an input to the model. Ledesma and Futter (2017) showed that gridded climate products could give as good or better simulations of streamflow when compared to observational weather data. This finding, combined with the difficulties in obtaining gap-free observational weather data and the need for consistency when applying model parameterizations based on the present-day to future conditions motivated the use of gridded climate data throughout this study. Point observations of streamflow and water quality are also spatially-integrated values as they are the result of processes occurring throughout the catchment.
Sensitivity Analysis –Sensitive Parameters
The criteria for behavior were defined by examining the observed data and identifying a range of values for monthly mean and maximum DOC concentration from 1995 to 2013. Behavior criteria were set to include an increasing trend of DOC concentration and reasonable ranges of monthly mean and maximum DOC concentrations for selected years and months. Summer months (August, July) were chosen and year of 1996, 2002, 2004, and 2008 were all selected to demonstrate increasing trends of DOC concentration. The top 2,000 model runs were retained to proceed with the general sensitivity analysis. Each simulation result consists of the parameter vector itself and the behavioral outcome, i.e., whether the particular parameter vector gave rise to the behavior or not. The criteria used to separate behavioral and non-behavioral parameter sets. Once the parameter set space was partitioned into two groups, the degree of difference between the two groups identified the model parameter influence. If the distribution of non-behavioral values of a model parameter does not separate from the behavioral one, this indicates that the parameter does not have a significant influence on model results. The Kolmogorov-Smirnov two-sample test was used to evaluate model parameter sensitivity. Following refinement of the parameter ranges, the 2,000 simulations for the Hore and the Hafren produce a mixture of behaviors (m) and non-behaviors (n) as expected.
In this study, 15 parameters for each type of land cover were applied to the sensitivity analysis, including parameters related to carbon processing, microclimate, hydraulic, enzymatic latch, and sulfate effects. As three land types (Forest, unimproved grassland, and peatland) were employed in this study, forty-five parameters in total were examined in the whole sensitivity analysis. The parameters and corresponding statistics (dm,n) that are significantly above the 95% level are ranked in order of importance and listed in Table 4. Eleven out of forty-five parameters are significant at the 95% level or greater in the Hore catchment. Nine for the Hafren catchment are found influential, and the relatively large separations denoted by the K-S test dm,n explains that these parameters are important for obtaining the correct behavior in a simulation. In this research, nine parameters contribute significantly to simulate an increasing trend of DOC concentrations in Plynlimon. Among the important parameters, the soil temperature response, sulfate effect (b1) multiplier, and organic layer hydraulic parameters (residence time and retention volume) have a significant impact on both catchments. It is not a surprise that the temperature effect has ranked as the most important parameter to influence model behavior and DOC concentrations. In soil process, sorption and desorption rates of organic carbon are dependent on soil temperature and moisture status, which decide the total mass of DOC and SOC in soil layers and in-stream DOC concentrations. Parameters associated with the effect of sulfate and enzymatic latch revealed to the influential parameters in Plynlimon (Table 4).
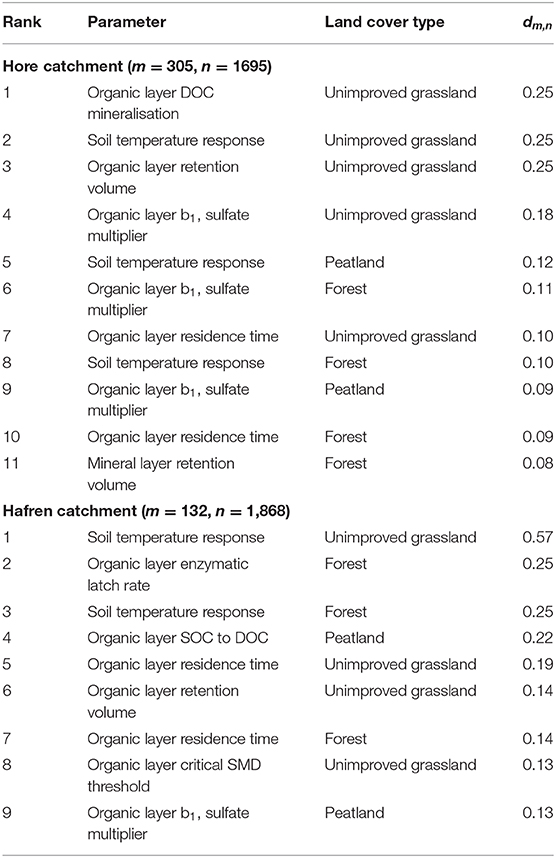
Table 4. Kolmogorov-Smirnov Statistics (significant at 95% level or greater) in the GSA of parameters influencing model behavior.
Climate Change in Plynlimon
According to the results of w@h2 climate scenarios, Plynlimon precipitation is expected to show a slight increase in winter months and a clear decrease in summer months (Figure 3). The trends are gradual from the near future to the far future time period. In the far future, the average increase of December precipitation is about 15% whilst a 43% average decrease is expected in July. The total annual average precipitation of the Plynlimon is expected to decrease by 3% in the near future and 6% in the far future. Projected changes in the seasonal patterns of precipitation are more significant than the total annual average precipitation. The average daily air temperature is projected to increase for all months for both future time periods (Figure 3). The mean temperature changes are from 9.01°C (8.76°C−9.28°C) as the baseline to 10.22°C (9.94°C−10.44°C) for the near future and to 12.28°C (12.02°C−12.48°C) for the far future scenario.
Simulated Flows and DOC With Projected Future Conditions
The calibrated INCA Carbon model was run with w@h2 climate scenarios and acidic deposition scenarios for the baseline, near future and far future for both Hore and Hafren catchments. The flows in Hore and Hafren show a clear seasonality, with low flows in summer months and high flows in winter months (Figure 8). The results of w@h2 driven by the INCA model, future climatic change will enhance this seasonal flow patterns. In particular, summer flows will decrease significantly, while changes in winter flows will be mild. This is probably because the projected lower summer rainfall and increased air temperatures in the Plynlimon area contribute to decrease flows, up to an average of 51% in July under the far future scenario. In winter months, projected flows are expected to increase the range of 1–5% in the near future, and 3–15% in the far future compared to the baseline. However, Plynlimon is a small stream with an average flow with 0.234 m3/s and 0.205 m3/s for Hafren and Hore, respectively (average between 1973 and 2009). It is quite difficult to represent in the model simulation, so the range of each future time slices is overlapping with the range of observed flow period. However, the median lines are showing the degree of changes in each time period (Figures 8A,B).
The INCA model results show a substantial increase in DOC concentrations in the near future and the far future from the baseline for both catchments. The DOC concentrations in the far future are stabilized and slightly reduced in the summer months after the near future. Figure 9 shows the DOC monthly average and maximum concentrations driven by the w@h2 climate scenarios. For the Hore catchment, the simulated baseline values slightly overestimate DOC concentrations from April to July compared to the observed values (Figures 9A,C). The rest of the months are represented in a reasonable range. It is worth noting that the time series of baseline condition started in 1975 and DOC monitoring has started since 1983, which may cause a slight discrepancy between baseline and observed values. The Hafren catchment, observed values lie within the range of the simulated values of baseline (Figures 9B,D).
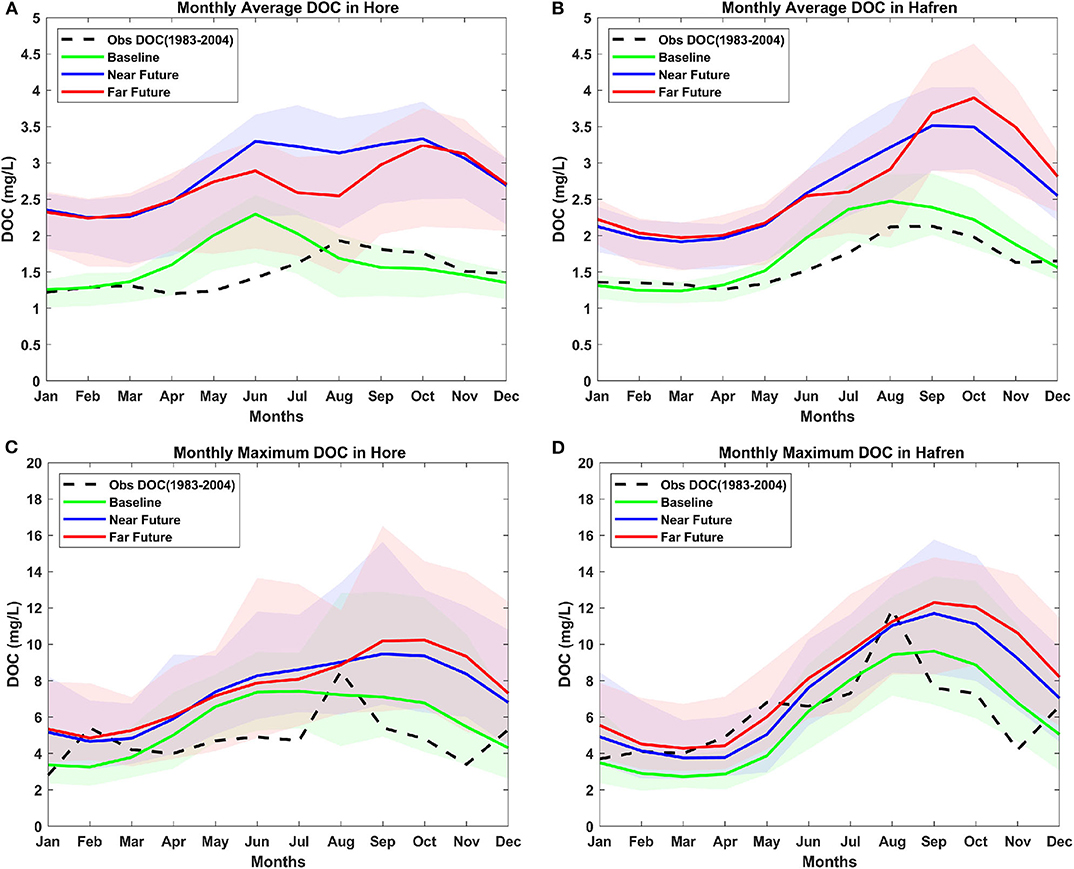
Figure 9. Impact of climate change and acidic deposition (up to 2030) on the Upper Severn catchments; the simulated mean monthly DOC concentrations at the Hore (A) and Hafren (B), the simulated monthly maximum DOC concentrations at the Hore (C) and Hafren (D).
In the near future for both catchments, there is a significant increase in later summer to autumn (August, September, and October). In the forest-dominant catchment at the lower Hore (Figure 9A), there is a slight reduction in DOC concentration the summer months in far future compared to the near future. However, there is still a big surge from the baseline level. Hafren (Peatland-dominant) catchment shows a different trend (Figure 9B). From September to November, there is an even greater increase in DOC in the far future than the near future while the summer months of DOC concentrations are slightly decreased.
Several droughts and associated low flows in summer months and subsequent re-wetting in early autumn in peatlands could destabilize peatland carbon stocks and enhance in-stream DOC production rates. This process is greatly affected by the combination of temperature and water availability in pore water as well. The w@h2 analyses projected less summer precipitation with warmer temperatures, which seems to cause an enzymatic latch effect in peatland soils. In the model simulations, the soil moisture deficit is a surrogate measure of soil dryness, and it is representative of the difference between the water currently in the soil and the water-holding capacity of the soil. The SMD is a key driver of INCA-C results as in-soil all carbon transformation rates are assumed to be soil moisture dependent. Additionally, in the INCA-C model application presented here, SMD is compared to a drought threshold below which an enzymatic latch effect is triggered. The SMD threshold of 31 mm was applied for this study in Plynlimon, and if SMD values were >31 mm depth, the enzymatic latch effect was triggered. The threshold was achieved from the calibration process, and it is consistent value with a measurement from Plynlimon experiment site (Hudson, 1988). Figure 10 shows the changes in the frequency and duration of soil moisture droughts (when SMD > 31 mm) for the two future scenarios that the enzymatic latch effect was triggered. The w@h2 future scenarios suggest a substantial increase in the duration of each soil moisture droughts (Figure 10B) than its frequency. It can be explained that during the drought, organic carbon is not mobilized. However, subsequent re-wetting after a long and severe drought, DOC production rates are accelerated from the peat. When the peatland soils become saturated and are mobilized, they flush DOC into the stream. Therefore post-drought surge of DOC concentrations become visible in autumn months (Figure 9B).
Maximum DOC concentrations show a substantial increase in the wet season, while a small increase in the summer months is projected at around 20% (Figures 9C,D). The projected sulfate deposition continues to decline until 2030 and remain constant after. The projected changes have inter-annual variability, but no intra-annual variability. Therefore, the sulfate decline could affect the stabilization of DOC concentrations in the far future period. However, the changes in seasonal variability of DOC concentrations are most likely driven by climatic changes and enzymatic latch processes during droughts. While this paper has only presented results for the Plynlimon in Wales, enzymatic latch simulations in peatlands can also be extended to understand the other peatlands catchments in the UK.
Conclusions
In this study, the impact of climate change and acidic deposition on flow and water quality was analyzed for an uplands catchment, Plynlimon in Wales, UK. This paper focused on the dynamics of droughts and sulfate deposition in peatlands by including those mechanisms in water quality modeling. Our study is the first to model enzymatic latch mechanisms in the UK catchments to explain a complex biochemical process in a multi-parameterised process-based model. The main findings are focused on assessing whether the rising trends of DOC concentrations would be continued or stabilized in the future. Taken across from our future climate, sulfate scenarios and the identified enzymatic latch parameters, the projected DOC concentrations in Plynlimon will continue to increase in the near future and then will be stabilized in the far future. However, in the far future, the seasonal patterns of DOC concentrations will change, with a post-drought DOC surge in autumn months.
Data Availability Statement
The original contributions presented in the study are included in the article. Publicly available datasets were analyzed in this study and this data can be found here: Land use map 2007 (UK Centre for Ecology and Hydrology, https://www.ceh.ac.uk/services/land-cover-map-2007#obtain), meteorology data (Met Office, https://catalogue.ceda.ac.uk/uuid/dbd451271eb04662beade68da43546e1), flow data (UK National River Flow Archive, https://nrfa.ceh.ac.uk/), and water quality data (UK Centre for Ecology and Hydrology, https://catalogue.ceh.ac.uk/documents/0392bf93-62b2-49f7-8c85-10038f22f0c0). The weather@home2 climate simulations are also available online (The Centre for Environmental Data Repository, https://catalogue.ceda.ac.uk/uuid/0cea8d7aca57427fae92241348ae9b03).
Author Contributions
All authors designed the research together. JL and MNF collected the data and set up the modeling. JL analysed the data. JL, PGW, MNF, and JWH wrote the paper.
Funding
This work was undertaken within the MaRIUS project: Managing the Risks, Impacts and Uncertainties of droughts and water Scarcity, funded by the Natural Environment Research Council (NERC), and undertaken by researchers from the University of Oxford (NE/L010364/1).
Conflict of Interest
The authors declare that the research was conducted in the absence of any commercial or financial relationships that could be construed as a potential conflict of interest.
Acknowledgments
The meteorological data (precipitation and air temperature) were provided by the UK Met Office. The river flow data were provided by the National River Flow Archive and Centre for Ecology and Hydrology. The Plynlimon water quality data were provided by the Centre for Ecology and Hydrology. We thank Dr Maximilian Posch (International Institute for Applied Systems Analysis, IIASA) for providing EMEP dataset. The authors would like to thank Dr Emma McIntosh (Arcadia Fund) and Dr Michael Bell (Forest Research) for valuable discussions on the earlier version of the paper.
References
Bell, M. C., Ritson, J. P., Verhoef, A., Brazier, R. E., Templeton, M. R., Graham, N. J. D., et al. (2018). Sensitivity of peatland litter decomposition to changes in temperature and rainfall. Geoderma 331, 29–37. doi: 10.1016/j.geoderma.2018.06.002
Chow, A. T., Tanji, K. K., and Gao, S. (2003). Production of dissolved organic carbon (DOC) and trihalomethane (THM) precursor from peat soils. Water Res. 37, 4475–4485. doi: 10.1016/S0043-1354(03)00437-8
Clark, J. M., Chapman, P. J., Adamson, J. K., and Lane, S. N. (2005). Influence of drought-induced acidification on the mobility of dissolved organic carbon in peat soils. Global Change Biol. 11, 791–809. doi: 10.1111/j.1365-2486.2005.00937.x
Cox, B. A., and Whitehead, P. G. (2005). Parameter sensitivity and predictive uncertainty in a new water quality model, Q2. J. Environ. Eng. 131, 147–157. doi: 10.1061/(ASCE)0733-9372(2005)131:1(147)
de Wit, H. A., Ledesma, J. L. J., and Futter, M. N. (2016). Aquatic DOC export from subarctic Atlantic blanket bog in Norway is controlled by seasalt deposition, temperature and precipitation. Biogeochemistry 127, 305–321. doi: 10.1007/s10533-016-0182-z
Delpla, I., Jones, T. G., Monteith, D. T., Hughes, D. D., Baurès, E., Jung, A.-V., et al. (2015). Heavy rainfall impacts on trihalomethane formation in Contrasting Northwestern European potable waters. J. Environ. Quality 44, 1241–1251. doi: 10.2134/jeq2014.10.0442
Evans, C. (2015). Biogeochemistry: old carbon mobilized. Nature Geosci. 8, 85–86. doi: 10.1038/ngeo2334
Evans, C. D., Chapman, P. J., Clark, J. M., Monteith, D. T., and Cresser, M. S. (2006). Alternative explanations for rising dissolved organic carbon export from organic soils. Global Change Biol. 12, 2044–2053. doi: 10.1111/j.1365-2486.2006.01241.x
Evans, C. D., Jones, T. G., Burden, A., Ostle, N., Zieliński, P., Cooper, M. D., et al. (2012). Acidity controls on dissolved organic carbon mobility in organic soils. Global Change Biol. 18, 3317–3331. doi: 10.1111/j.1365-2486.2012.02794.x
Evans, C. D., Monteith, D. T., and Cooper, D. M. (2005). Long-term increases in surface water dissolved organic carbon: observations, possible causes and environmental impacts. Environ. Pollut. 137, 55–71. doi: 10.1016/j.envpol.2004.12.031
Fenner, N., and Freeman, C. (2011). Drought-induced carbon loss in peatlands. Nat. Geosci. 4, 895–900. doi: 10.1038/ngeo1323
Freeman, C., Evans, C., Monteith, D., Reynolds, B., and Fenner, N. (2001a). Export of organic carbon from peat soils. Nature 412, 785–786. doi: 10.1038/35090628
Freeman, C., Fenner, N., Ostle, N. J., Kang, H., Dowrick, D. J., Reynolds, B., et al. (2004). Export of dissolved organic carbon from peatlands under elevated carbon dioxide levels. Nature 430, 195–198. doi: 10.1038/nature02707
Freeman, C., Ostle, N., and Kang, H. (2001b). An enzymic 'latch' on a global carbon store. Nature 409:149. doi: 10.1038/35051650
Futter, M., Erlandsson, M., Butterfield, D., Whitehead, P., Oni, S., and Wade, A. (2014). PERSiST: a flexible rainfall-runoff modelling toolkit for use with the INCA family of models. Hydrol Earth System Sci. 18, 855–873. doi: 10.5194/hess-18-855-2014
Futter, M. N., Butterfield, D., Cosby, B. J., Dillon, P. J., Wade, A. J., and Whitehead, P. G. (2007). Modeling the mechanisms that control in-stream dissolved organic carbon dynamics in upland and forested catchments. Water Resources Res. 43:W02424. doi: 10.1029/2006WR004960
Futter, M. N., and de Wit, H. A. (2008). Testing seasonal and long-term controls of streamwater DOC using empirical and process-based models. Sci. Total Environ. 407, 698–707. doi: 10.1016/j.scitotenv.2008.10.002
Futter, M. N., Forsius, M., Holmberg, M., and Starr, M. (2009). A long-term simulation of the effects of acidic deposition and climate change on surface water dissolved organic carbon concentrations in a boreal catchment. Hydrol. Res. 40, 291–305. doi: 10.2166/nh.2009.101
Futter, M. N., Lofgren, S., Kohler, S. J., Lundin, L., Moldan, F., and Bringmark, L. (2011). Simulating dissolved organic carbon dynamics at the swedish integrated monitoring sites with the integrated catchments model for carbon, INCA-C. Ambio 40, 906–919. doi: 10.1007/s13280-011-0203-z
Gorham, E. (1991). Northern peatlands: role in the carbon cycle and probable responses to climatic warming. Ecol. Applications 1, 182–195. doi: 10.2307/1941811
Guillod, B. P., Jones, R. G., Bowery, A., Haustein, K., Massey, N. R., Mitchell, D. M., et al. (2017). weather@home 2: validation of an improved global–regional climate modelling system. Geosci. Model Dev. 10, 1849–1872. doi: 10.5194/gmd-10-1849-2017
Guillod, B. P., Jones, R. G., Dadson, S. J., Coxon, G., Bussi, G., Freer, J., et al. (2018). A large set of potential past, present and future hydro-meteorological time series for the UK. Hydrol. Earth Syst. Sci. 22, 611–634. doi: 10.5194/hess-22-611-2018
Hornberger, G., and Spear, R. (1980). Eutrophication in Peel Inlet—I. The problem-defining behavior and a mathematical model for the phosphorus scenario. Water Res. 14, 29–42. doi: 10.1016/0043-1354(80)90039-1
Hudson, J. A. (1988). The contribution of soil moisture storage to the water balances of upland forested and grassland catchments. Hydrol. Sci. J. 33, 289–309. doi: 10.1080/02626668809491249
Kang, H., Kwon, M. J., Kim, S., Lee, S., Jones, T. G., Johncock, A. C., et al. (2018). Biologically driven DOC release from peatlands during recovery from acidification. Nat. Commun. 9:3807. doi: 10.1038/s41467-018-06259-1
Lazar, A. N., Butterfield, D., Futter, M. N., Rankinen, K., Thouvenot-Korppoo, M., Jarritt, N., et al. (2010). An assessment of the fine sediment dynamics in an upland river system: INCA-Sed modifications and implications for fisheries. Sci. Total Environ. 408, 2555–2566. doi: 10.1016/j.scitotenv.2010.02.030
Ledesma, J. L. J., and Futter, M. N. (2017). Gridded climate data products are an alternative to instrumental measurements as inputs to rainfall–runoff models. Hydrol. Processes 31, 3283–3293. doi: 10.1002/hyp.11269
Lowe, J. A., Bernie, D., Bett, P., Bricheno, L., Brown, S., Calvert, D., et al. (2018). UKCP18 Science Overview Report. Exeter: Met Office Hadley Centre. doi: 10.1017/CBO9781107415324
Lu, Q., Futter, M. N., Nizzetto, L., Bussi, G., Jürgens, M. D., and Whitehead, P. G. (2016). Fate and transport of polychlorinated biphenyls (PCBs) in the River Thames catchment – Insights from a coupled multimedia fate and hydrobiogeochemical transport model. Sci. Total Environ. 572, 1461–1470. doi: 10.1016/j.scitotenv.2016.03.029
Massey, N., Jones, R., Otto, F. E. L., Aina, T., Wilson, S., Murphy, J. M., et al. (2015). weather@home—development and validation of a very large ensemble modelling system for probabilistic event attribution. Quarterly J. Royal Meteorol. Soc. 141, 1528–1545. doi: 10.1002/qj.2455
Met Office (2012). Met Office Integrated Data Archive System (MIDAS) Land and Marine Surface Stations Data (1853-current). Centre for Environmental Data Analysis, Available online at: https://catalogue.ceda.ac.uk/uuid/dbd451271eb04662beade68da43546e1 (accessed October 8, 2020).
Monteith, D. T., Stoddard, J. L., Evans, C. D., de Wit, H. A., Forsius, M., Hogasen, T., et al. (2007). Dissolved organic carbon trends resulting from changes in atmospheric deposition chemistry. Nature 450, 537–540. doi: 10.1038/nature06316
Moriasi, D. N., Arnold, J. G., Van Liew, M. W., Bingner, R. L., Harmel, R. D., and Veith, T. L. (2007). Model evaluation guidelines for systematic quantification of accuracy in watershed simulations. Trans. ASABE 50, 885–900. doi: 10.13031/2013.23153
Nash, J. E., and Sutcliffe, J. V. (1970). River flow forecasting through conceptual models part I — A discussion of principles. J. Hydrol. 10, 282–290. doi: 10.1016/0022-1694(70)90255-6
Neal, C., Kirchner, J., and Reynolds, B. (2013). Plynlimon research catchment hydrochemistry, NERC Environmental Information Data Centre, Available online at: https://doi.org/10.5285/44095e17-43b0-45d4-a781-aab4f72da025
Neal, C., Reynolds, B., Norris, D., Kirchner, J. W., Neal, M., Rowland, P., et al. (2011). Three decades of water quality measurements from the Upper Severn experimental catchments at Plynlimon, Wales: an openly accessible data resource for research, modelling, environmental management and education. Hydrological Processes, 25: 3818–3830. doi: 10.1002/hyp.8191
Nizzetto, L., Bussi, G., Futter, M. N., Butterfield, D., and Whitehead, P. G. (2016). A theoretical assessment of microplastic transport in river catchments and their retention by soils and river sediments. Environmental Science: Processes & Impacts, 18: 1050–1059. doi: 10.1039/C6EM00206D
Norris, D. A., Harvey, R., Winterbourn, J. M., Hughes, S., Lebron, I., Thacker, S. A., et al. (2017). Plynlimon research catchment hydrochemistry (2011-2016), NERC Environmental Information Data Centre. doi: 10.5285/794c609b-da62-4a42-a4c1-267219865bb1
O'Driscoll, C., Ledesma, J. L. J., Coll, J., Murnane, J. G., Nolan, P., Mockler, E. M., et al. (2018). Minimal climate change impacts on natural organic matter forecasted for a potable water supply in Ireland. Sci. Total Environ. 630, 869–877. doi: 10.1016/j.scitotenv.2018.02.248
Pastor, J., Solin, J., Bridgham, S. D., Updegraff, K., Harth, C., Weishampel, P., et al. (2003). Global warming and the export of dissolved organic carbon from boreal peatlands. Oikos 100, 380–386. doi: 10.1034/j.1600-0706.2003.11774.x
Posch, M., Aherne, J., Forsius, M., Fronzek, S., and Veijalainen, N. (2007). Modelling the impacts of European emission and climate change scenarios on acid-sensitive catchments in Finland. Hydrol. Earth System Sci. Discussions 4, 3209–3248. doi: 10.5194/hessd-4-3209-2007
Ritson, J. P., Bell, M., Brazier, R. E., Grand-Clement, E., Graham, N. J. D., Freeman, C., et al. (2016). Managing peatland vegetation for drinking water treatment. Sci. Reports 6:36751. doi: 10.1038/srep36751
Ritson, J. P., Graham, N. J. D., Templeton, M. R., Clark, J. M., Gough, R., and Freeman, C. (2014). The impact of climate change on the treatability of dissolved organic matter (DOM) in upland water supplies: a UK perspective. Sci. Total Environ. 473, 714–730. doi: 10.1016/j.scitotenv.2013.12.095
Robinson, M., Rodda, J. C., and Sutcliffe, J. V. (2013). Long-term environmental monitoring in the UK: origins and achievements of the Plynlimon catchment study. Trans. Institute British Geographers 38, 451–463. doi: 10.1111/j.1475-5661.2012.00534.x
Schöpp, W., Posch, M., Mylona, S., and Johansson, M. (2003). Long-term development of acid deposition (1880–2030) in sensitive freshwater regions in Europe. Hydrol. Earth Syst. Sci. 7, 436–446. doi: 10.5194/hess-7-436-2003
Skjelkvåle, B. L., Evans, C., Larssen, T., Hindar, A., and Raddum, G. G. (2003). Recovery from acidification in european surface waters: a view to the future. AMBIO 32, 170–175. doi: 10.1579/0044-7447-32.3.170
Spear, R., and Hornberger, G. (1980). Eutrophication in Peel Inlet—II. Identification of critical uncertainties via generalized sensitivity analysis. Water Res. 14, 43–49. doi: 10.1016/0043-1354(80)90040-8
Tranvik, L. J., and Jansson, M. (2002). Terrestrial export of organic carbon. Nature 415:861. doi: 10.1038/415861b
UK National Ecosystem Assessment (2011). The UK and National Ecosystem Assessment: Synthesis of the Key Findings. Unep-Wcmc Cambridge.
Wade, A. J., Whitehead, P. G., and Butterfield, D. (2002a). The Integrated Catchments model of Phosphorus dynamics (INCA-P), a new approach for multiple source assessment in heterogeneous river systems: model structure and equations. Hydrol. Earth System Sci. 6, 583–606. doi: 10.5194/hess-6-583-2002
Wade, A. J., Whitehead, P. G., Hornberger, G. M., and Snook, D. L. (2002b). On modelling the flow controls on macrophyte and epiphyte dynamics in a lowland permeable catchment: the River Kennet, southern England. Sci. Total Environ. 282-283, 375–393. doi: 10.1016/S0048-9697(01)00925-1
Whitehead, P., Futter, M., and Wilby, R. (2006). Impacts of Climate Change on Hydrology, Nitrogen and Carbon in Upland and Lowland Streams: Assessment of Adaptation Strategies to Meet Water Framework Directive Objectives. Proceedings Durham Meeting, British Hydrological Society.
Whitehead, P., and Hornberger, G. (1984). Modelling algal behaviour in the River Thames. Water Res. 18, 945–953. doi: 10.1016/0043-1354(84)90244-6
Whitehead, P., Wade, A., and Butterfield, D. (2009). Potential impacts of climate change on water quality and ecology in six UK rivers. Hydrol. Res. 40, 113–122. doi: 10.2166/nh.2009.078
Whitehead, P., Wilson, E., and Butterfield, D. (1998a). A semi-distributed Integrated Nitrogen model for multiple source assessment in Catchments (INCA): Part I—model structure and process equations. Sci. Total Environ. 210, 547–558. doi: 10.1016/S0048-9697(98)00037-0
Whitehead, P. G., Leckie, H., Rankinen, K., Butterfield, D., Futter, M. N., and Bussi, G. (2016). An INCA model for pathogens in rivers and catchments: model structure, sensitivity analysis and application to the River Thames catchment, UK. Sci. Total Environ. 572, 1601–1610. doi: 10.1016/j.scitotenv.2016.01.128
Whitehead, P. G., Wilson, E. J., Butterfield, D., and Seed, K. (1998b). A semi-distributed integrated flow and nitrogen model for multiple source assessment in catchments (INCA): part II — application to large river basins in south Wales and eastern England. Sci. Total Environ. 210, 559–583. doi: 10.1016/S0048-9697(98)00038-2
Worrall, F., and Burt, T. (2004). Time series analysis of long-term river dissolved organic carbon records. Hydrol. Proc. 18, 893–911. doi: 10.1002/hyp.1321
Xu, J., Morris, P. J., Liu, J., Ledesma, J. L. J., and Holden, J. (2020). Increased dissolved organic carbon concentrations in peat-fed UK water supplies under future climate and sulfate deposition scenarios. Water Res. Res. 56:e2019WR025592. doi: 10.1029/2019wr025592
Keywords: water quality, DOC (dissolved organic carbon), climate change, droughts, enzymatic latch, Plynlimon
Citation: Lee J, Whitehead PG, Futter MN and Hall JW (2020) Impacts of Droughts and Acidic Deposition on Long-Term Surface Water Dissolved Organic Carbon Concentrations in Upland Catchments in Wales. Front. Environ. Sci. 8:578611. doi: 10.3389/fenvs.2020.578611
Received: 30 June 2020; Accepted: 17 September 2020;
Published: 30 October 2020.
Edited by:
Ian Holman, Cranfield University, United KingdomReviewed by:
K. S. Rao, University of Delhi, IndiaXander Wang, University of Prince Edward Island, Canada
Copyright © 2020 Lee, Whitehead, Futter and Hall. This is an open-access article distributed under the terms of the Creative Commons Attribution License (CC BY). The use, distribution or reproduction in other forums is permitted, provided the original author(s) and the copyright owner(s) are credited and that the original publication in this journal is cited, in accordance with accepted academic practice. No use, distribution or reproduction is permitted which does not comply with these terms.
*Correspondence: Jaeyoung Lee, jae-young.lee@ouce.ox.ac.uk; Paul G. Whitehead, paul.whitehead@ouce.ox.ac.uk
†ORCID: Jaeyoung Lee orcid.org/0000-0002-3678-2433
Jim W. Hall orcid.org/0000-0002-2024-9191