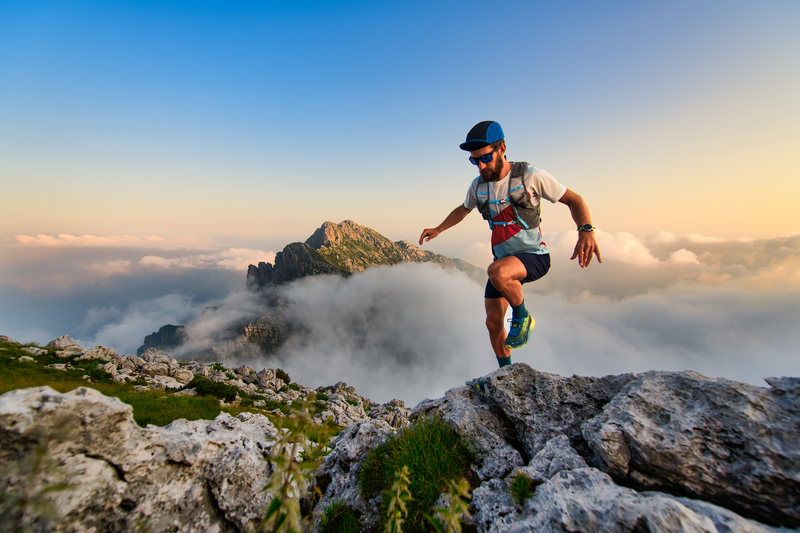
94% of researchers rate our articles as excellent or good
Learn more about the work of our research integrity team to safeguard the quality of each article we publish.
Find out more
ORIGINAL RESEARCH article
Front. Environ. Sci. , 27 August 2020
Sec. Environmental Informatics and Remote Sensing
Volume 8 - 2020 | https://doi.org/10.3389/fenvs.2020.00135
This article is part of the Research Topic Frontiers in Environmental Science – Editor’s Picks 2021 View all 25 articles
Malaria is a vector-borne disease of significant public health concern. Despite widespread success of many elimination initiatives, elimination efforts in some regions of the world have stalled. Barriers to malaria elimination include climate and land use changes, such as warming temperatures and urbanization, which can alter mosquito habitats. Socioeconomic factors, such as political instability and regional migration, also threaten elimination goals. This is particularly relevant in areas where local elimination has been achieved and consequently surveillance and control efforts are dwindling and are no longer a priority. Understanding how environmental change, impacts malaria elimination has important practical implications for vector control and disease surveillance strategies. It is important to consider climate change when monitoring the threat of malaria resurgence due to socioeconomic influences. However, there is limited assessment of how the combination of climate variation, interventions and socioeconomic pressures influence long-term trends in malaria transmission and elimination efforts. In this study, we used Bayesian hierarchical mixed models and malaria case data for a 29-year period to disentangle the impacts of climate variation and malaria control efforts on malaria risk in the Ecuadorian province of El Oro, which achieved local elimination in 2011. We found shifting patterns of malaria between rural and urban areas, with a relative increase of Plasmodium vivax in urbanized areas. Minimum temperature was an important driver of malaria seasonality and the association between warmer minimum temperatures and malaria incidence was greater for Plasmodium falciparum compared to P. vivax malaria. There was considerable heterogeneity in the impact of three chemical vector control measures on both P. falciparum and P. vivax malaria. We found statistically significant associations between two of the three measures [indoor residual spraying (IRS) and space spraying] and a reduction in malaria incidence, which varied between malaria type. We also found environmental suitability for malaria transmission is increasing in El Oro, which could limit future elimination efforts if malaria is allowed to re-establish. Our findings have important implications for understanding environmental obstacles to malaria elimination and highlights the importance of designing and sustaining elimination efforts in areas that remain vulnerable to resurgence.
Malaria is the most important vector-borne disease worldwide, with 228 million cases reported in 2018 (WHO, 2019). Despite global elimination and eradication efforts, progress toward elimination is stalling in some endemic countries. In particular, increases in malaria incidence have been observed in some South American countries since 2014 including Venezuela, Peru, Colombia, and Ecuador, where movement of infected individuals between neighboring countries in the region can result in a resurgence of cases in local populations, threatening progress toward malaria reduction for other countries (Grillet et al., 2019; WHO, 2019). Malaria is highly sensitive to environmental conditions, including climate variability and land use practices (Moreno, 2006; Caminade et al., 2014), which along with socioeconomic influences can act as significant barriers to elimination. In addition, the lapse in control and surveillance efforts combined with global environmental changes also poses a threat to malaria elimination efforts in South America (Alimi et al., 2015; Conn et al., 2018).
Variation in meteorological conditions, particularly temperature, and rainfall, determines the spatiotemporal patterns of malaria through their effects on both the Plasmodium parasite and the Anopheles vector. Warmer temperatures can decrease the extrinsic incubation period (EIP), the time taken for the malaria parasite to complete its development inside the mosquito. A shorter EIP increases the transmission intensity of malaria by allowing mosquitoes to become infectious more quickly. The EIP for the Plasmodium falciparum parasite is longer (∼10 days) than that for Plasmodium vivax (∼8 days) and P. vivax can also develop at relatively cooler temperatures (minimum 15°C), compared to P. falciparum (minimum 18°C) (Patz and Olson, 2006). Temperature also impacts the mosquito vector, by affecting both larval and adult survival and longevity (Beck-Johnson et al., 2013), mosquito development (Bayoh and Lindsay, 2003), and vector population dynamics (Beck-Johnson et al., 2013). However, the effect of temperature on malaria transmission is non-linear, with the optimal temperature for malaria transmission estimated to be 25°C with transmission declining above 30°C (Paaijmans et al., 2009; Mordecai et al., 2013). Rainfall is also important for malaria transmission as it determines the availability of mosquito breeding habitat and thus mosquito abundance, although heavy and persistent rainfall can wash out larval habitats (Galardo et al., 2009; Olson et al., 2009; Prussing et al., 2019; Wolfarth-Couto et al., 2019).
Anthropogenic activities, such as urbanization and agricultural land use can also change malaria transmission patterns over longer time scales by creating more or less favorable microclimatic conditions and habitats for malaria vectors, depending on the species (Conn et al., 2002; Wayant et al., 2010; Tucker Lima et al., 2017). For example, urban development can eliminate mosquito habitat subsequently decreasing malaria incidence or in some cases increases in peri-urban malaria can occur as a result of economic migration and the creation of novel breeding habitats (Padilla et al., 2015). In addition, deforestation can facilitate malaria transmission due to the creation of new mosquito habitats on forest fringes (Vittor et al., 2006; Stefani et al., 2013). Increasing environmental suitability of malaria transmission, associated with climatic change and land use modifications, has important implications for malaria elimination efforts in South America, as these changes could compromise elimination efforts (Recht et al., 2017).
Previous studies have identified several environmental risk factors for malaria transmission that may impede elimination efforts in South America. For example, large-scale climate patterns such as the El Niño Southern Oscillation have been associated with large outbreaks of malaria in Peru, due to flooding conditions and in Colombia and Venezuela due to drought conditions (Gagnon et al., 2002). In highland areas of Colombia, warmer years were associated with an increased number of malaria cases occurring at higher altitudes (Siraj et al., 2014). Studies assessing spatial heterogeneity in malaria risk in South America have found deforestation and agricultural development have altered anopheline vector ecology in the Amazon region through creation of new Anopheles habitats and increased human-mosquito interactions (Moreno et al., 2007; Vittor et al., 2009; Hahn et al., 2014; Burkett-Cadena and Vittor, 2017).
When assessing the role of environmental factors on malaria incidence it is important to simultaneously consider the relative impact of control interventions, especially in elimination settings. Studies that evaluate the effectiveness of malaria control programs often consider environmental factors, most commonly rainfall and vegetation indices. Some studies used geostatistical modeling and after adjusting for climate conditions estimate the contribution and effectiveness of malaria control measures, including indoor residual spraying (IRS) in sub-Saharan Africa, relative to the impacts of climate variability (Graves et al., 2008; Giardina et al., 2014). Other studies often treat climate and environmental variables as confounders and do not apportion variation in malaria incidence due to climate (Aregawi et al., 2011; Bennett et al., 2014). There has been a limited assessment of how the combination of environmental factors, elimination efforts and socioeconomic pressures influences long-term trends in malaria and the route toward elimination. Understanding how malaria elimination efforts might be hindered by environmental changes has implications for targeting of vector control and disease surveillance, and is important especially given the possibility of shifting vector distributions and increasing climate suitability in South America (Pinault and Hunter, 2012).
According to the Pan-American Health Organization (PAHO), Ecuador is in the malaria pre-elimination phase, but has fallen short of its target to achieve elimination by 2020 (WHO, 2018). Since 2016 malaria has increased significantly in the country, mainly in the Amazon region, with more cases being detected than expected for a country on the verge of malaria elimination (PAHO, 2017; Sáenz et al., 2017). Recent surges of malaria in other South American countries, primarily Venezuela, are threatening current elimination efforts in Ecuador due to regional migration (Daniels, 2018; Grillet et al., 2019). This study focuses on Ecuador’s southern province, El Oro, a high-risk region, which previously eliminated malaria but recently recorded indigenous cases, in addition to being vulnerable to the introduction of infections via imported cases due to high rates of human movement associated with its location on a strategic migration route (Jaramillo-Ochoa et al., 2019). Here we seek to identify threats to malaria elimination, with a view to prevent lapses in control efforts and the re-establishment of malaria in an area currently considered malaria-free but historically endemic for malaria. We advance the existing knowledge of the malaria elimination success in El Oro (Krisher et al., 2016) by quantifying the associations between malaria, climate and control interventions in a region vulnerable to resurgence.
El Oro province (latitude: 3°5′45.20′′S-4°11′3.06′′S, longitude:-79°43′10.92′′W-80°50′37.96′′W) is located in southern Ecuador on the Pacific Coast and shares a border with the Tumbes region of northern Peru (Figure 1A). El Oro covers 5,870 km2 and is divided into 14 cantons, which range in size from approximately 70 km2 up to 900 km2. Population densities per canton range from 7 people per km2 in rural areas and up to 760 people per km2 in the province capital, Machala.
Figure 1. Malaria cases in El Oro 1990–2018. (A) Location of El Oro province (red), Ecuador in relation to neighboring countries in South American; Colombia, Peru, and Venezuela. (B) Total number of malaria cases (gray), cases of P. falciparum (pink), and P. vivax malaria (blue) recorded in El Oro 1990–2018.
Plasmodium vivax and P. falciparum are the malaria-causing parasites in the region (WHO, 2019) and the primary mosquito vectors transmitting malaria in El Oro are Anopheles albimanus and Anopheles punctimacula (Ryan et al., 2017).
During the mid-1990s public health authorities in El Oro and neighboring Tumbes unified to implement an effective binational collaboration to tackle the surge in malaria transmission in the region (Krisher et al., 2016). El Oro has been free of locally acquired malaria infections since 2011 although malaria has been increasing elsewhere in Ecuador (WHO, 2019). In 2018 seven malaria cases were recorded in El Oro (6/7 were imported cases) during a period of elevated migration of Venezuelan citizens associated with the social and economic crisis in their country (Jaramillo-Ochoa et al., 2019). El Oro’s border location, along the Pan American highway, a route used by many migrants, makes it particularly vulnerable to malaria resurgence. In addition, El Oro is one of the most hazardous coastal zones in Latin America and the Caribbean (Calil et al., 2017). Vulnerable populations are susceptible to the effects of the El Niño Southern Oscillation, which intensifies annual flooding events during the rainy season (Lowe et al., 2017; Tauzer et al., 2019). Lapses in surveillance and control efforts and reductions in funding following local elimination also means malaria resurgence is likely (PAHO, 2017; Sáenz et al., 2017).
Monthly counts of blood smear confirmed cases of P. falciparum and P. vivax malaria for each canton in El Oro, January 1990–December 2018, were provided by the Ecuadorian Ministry of Health, where malaria is a mandatory notifiable disease. Cases were recorded at local clinics across El Oro and collated by the Ministry of Health. Population data, available for each canton, were sourced from the national census in Ecuador, from the Instituto Nacional de Estadística y Censos (INEC1) for 1990, 2001, and 2010. Data for 2011–2018 were provided by INEC as annual population projections. Population values between census years 1990, 2001, and 2010 were estimated by interpolating assuming linear growth, to provide annual population estimates for each canton. To estimate the proportion of the population in poverty per canton in El Oro, we used Unmet Basic Needs (UBN) sourced from the 2010 census, an indicator based on measures including housing quality, education and access to water and sanitation. We used UBN data from 2010 as a socioeconomic covariate in all our models.
Between 2001 and 2015 an intensive period of vector control was carried out across El Oro following a resurgence of malaria cases and monthly canton-level data for three control measures were available from the Ministry of Health. Monthly estimates per canton of the number of households treated by IRS with insecticides (deltamethrin 5% concentrated suspension, deltamethrin 2.5%, malathion 50%, alphacypermethrin 10% concentrated suspension, and betacypermethrin 2.5%) were available for January 2001–September 2013. The number of neighborhoods that were treated with insecticide via ultra-low volume (ULV) fumigation, which is performed by spraying entire neighborhoods with 96% malathion from trucks were available between January 2004 and May 2015. Finally, the number of households space-sprayed with 2.5% deltamethrin concentrated emulsion, using a backpack fogger that creates a fog insecticide to treat both inside and outside the home, were available between January 2004 and May 2015 (Supplementary Figure S1). For each control measure we tested for lagged relationships, up to 3 months, in order to account for delays between implementation and impact on measurable malaria cases (Supplementary Table S1). Other malaria control interventions including fumigation with DDT (until banned in 1996), elimination of larval habitats, and bed net provision occurred during the study period, but no detailed data for control measures were available prior to 2001.
Monthly climate data, for temperature and precipitation, between January 1990 and December 2018 were sourced from TerraClimate, a high-spatial resolution (∼4 km) dataset that uses climatically aided interpolation to produce monthly estimates (Abatzoglou et al., 2018). To obtain climate estimates for each canton in El Oro, TerraClimate variables were aggregated by taking mean grid cell values using the raster package in R.
Annual land cover maps at a 300 m spatial resolution generated by the European Space Agency (ESA) Climate Change Initiative (CCI) were compiled for 1990–20182 (European Space Agency, 2019). For each annual map, the number of grid cells in each canton that were classified in the urban category were extracted, and from this the proportion of urban grid cells per canton were calculated to give a continuous variable of urbanization (Supplementary Figure S3). All continuous variables were scaled by subtracting the variable mean from each value and dividing this by the standard deviation. This transformation allowed for the direct comparison of continuous variables and enabled their relative importance to be determined in the model.
Bayesian hierarchical mixed models are powerful statistical models that can be used to estimate the marginal posterior distributions of multiple covariates whilst simultaneously accounting for multiple sources of uncertainty that can arise from disease count data. Here, spatiotemporal models were constructed, for each malaria parasite, P. falciparum and P. vivax, to explore the contributions of climate and control interventions to variations in monthly malaria incidence in El Oro between January 1990 and December 2018 (348 months).
We fitted zero-inflated negative binomial models that take into account excess zeros that cannot be explained by a standard negative binomial distribution (Supplementary Appendix S1.1). Where μst is the mean number of malaria cases in each canton (s = 1,…,14) for each timestep (t = 1,…,348), the hierarchical spatiotemporal model is defined as:
Annual population estimates per 1,000 inhabitants for each canton in El Oro, log(Pst), were included as an offset in the model to account for canton-level differences in the annual parasite incidence (API). The estimated API, log(ρst) is then made up of a combination of climate covariates, including mean monthly minimum temperature and precipitation for each canton. Other explanatory variables considered included socioeconomic factors (poverty rates), level of urbanization and vector control interventions (IRS, ULV fumigation and space-spraying).
To account for the seasonality in malaria incidence, a monthly random effect was introduced using a first order autoregressive prior (mt), which allows malaria in 1 month to depend on incidence in the previous month. Independent random effects for each year (yt), 1990–2018, were included to allow for additional sources of variation due to unobserved confounding factors such as variations in healthcare access, case reporting and diagnostics.
Spatially structured random effects, υs, were introduced into the model to allow for correlated heterogeneity in malaria incidence across cantons in El Oro. This spatial dependency structure was accounted for by assuming a conditional intrinsic Gaussian autoregressive (CAR) model prior distribution for the spatial effects, which takes into account the neighborhood structure of the area (Besag et al., 1991). We also included spatially unstructured random effects in the model to allow for additional uncorrelated spatial variation across cantons, νs, which were assigned independent diffuse Gaussian exchangeable prior distributions (Lowe et al., 2016). Separate models were constructed for both P. falciparum and P. vivax malaria due to their intrinsic differences, which include diagnostic potential, development time inside the mosquito vector (extrinsic incubation period, EIP), the differential impact of vector control on transmission, infection reservoirs of P. vivax hypnozoites and the presence of asymptomatic cases.
Detailed data on interventions was only available for the period January 2001–December 2015. Therefore, we formulated separate sub-models for each malaria type to investigate the relative impact of the control measures implemented between 2001 and 2015 (see Supplementary Appendix S2), herein referred to as “intervention models.” The intervention models, fitted to data for the period 2001–2015 included the same explanatory variables and random effects as the “full” models (fitted to data for the period 1990–2018) with the addition of the three control measures. We examined differences between the random effects structures for the full models and the intervention models, to see how much variation in malaria due to interventions could be accounted for by random effects in the absence of intervention data for the entire time period.
Posterior distributions of model parameters were estimated using Integrated Nested Laplace approximations (INLA) (Rue et al., 2009). INLA provides a computationally more efficient alternative to Markov Chain Monte Carlo (MCMC) methods, by using numerical approximations of model parameters (see Supplementary Appendix S1.2). Covariate time lags and the most parsimonious models were selected using the deviance information criterion (DIC) (Spiegelhalter et al., 2002) and the Watanabe–Akaike information criterion (WAIC) (Watanabe, 2010), which are Bayesian methods of model comparison that trade off model adequacy against model complexity. Models with lower DIC and WAIC indicate a more parsimonious model. We also used the logarithmic score to assess model fit, which is based on the conditional predictive ordinate (CPO) leave-one-out cross-validation score, where a smaller value indicates a greater predictive power of the model (Gneiting and Raftery, 2007).
Covariates were added iteratively to the models starting with a baseline model, which included spatial and temporal structured and unstructured random effects and retained if model fit was improved, assessed through a decrease in DIC and WAIC. The most appropriate temperature variable, maximum or minimum temperature was selected using DIC and WAIC (Supplementary Table S2). We tested different time lags (from 0 to 3 months) to take into account the lagged effect of climate factors on malaria transmission, including development time of mosquitoes and parasites as well as the time between malaria diagnosis and case recording (Supplementary Table S2). We also tested non-linear functions of temperature and precipitation (Supplementary Table S3). We used the root mean square error (RMSE) to assess the extent to which models of malaria incidence for 2001–2015 were improved by the inclusion of each control measure in turn. RMSE is a measure of the standard deviation of the model residuals, with smaller values indicating better model fit to the observed data.
Between 1990 and 2018, 62,120 cases of malaria were recorded in El Oro, with 54% of infections resulting from P. falciparum malaria and 46% from P. vivax. Malaria transmission in El Oro was historically high, reaching up to 2,000 total annual cases in 1999 and an API of 61 cases of P. falciparum and 35 P. vivax cases per 1,000 people recorded in Huaquillas canton along the Peruvian border. Between 1998 and 2002 large outbreaks, particularly of P. falciparum malaria occurred across the province before declining to low levels of incidence after the period of more intensive vector control (Figure 1B). A small outbreak of P. vivax malaria occurred between 2007 and 2009 before malaria incidence was dramatically reduced and remained at a low and stable level (Figure 1B). The highest malaria incidence was concentrated in the western part of the province, along the Peru-Ecuador border (Supplementary Figure S4).
Between 1990 and 2018, outbreaks of both P. falciparum and P. vivax malaria occurred roughly at the same time. Prior to the decline in cases in 2001–2015 there was a greater difference between P. falciparum and P. vivax incidence in rural areas, compared to urbanized areas (Supplementary Figure S5). During the elimination phase (2001–2010) the incidence of P. falciparum and P. vivax malaria in rural areas was reduced much more than malaria in urbanized areas, where outbreaks of P. vivax were still observed. There was also a greater overall reduction of P. falciparum malaria in rural and urbanized areas.
In the models of malaria incidence in El Oro between 1990 and 2018, we found that areas that were more urbanized were associated with greater incidences of P. falciparum malaria (Figure 2A). To examine how the epidemiology of malaria in El Oro had changed with the three vector control interventions, we tested whether there was a difference in the relationship between malaria and level of urbanization in El Oro, before and after the interventions were implemented. We found a statistically significant interaction between level of urbanization in El Oro and the period the control measures were implemented (2001–2015) for P. vivax malaria and no significant interaction for P. falciparum malaria (Supplementary Figure S6). Prior to 2001, more urbanized areas of El Oro were associated with decreased P. vivax malaria and after 2001 this relationship reversed with urbanized areas associated with more P. vivax malaria.
Figure 2. Parameter estimates for explanatory variables of P. falciparum and P. vivax malaria in El Oro 1990–2018. (A) Posterior mean and 95% credible intervals for minimum temperature and precipitation, included as linear terms, level of urbanization and poverty covariates for P. falciparum (pink) and P. vivax (blue) malaria. (B) Relationships between P. falciparum and P. vivax relative risk, on the log scale defined as the annual parasite incidence (API), log(ρst), and minimum temperature, lagged by 3 months, included in the model as a function to allow for non-linearities.
During the period of malaria decline (post 2001) there was also a reduction in the seasonal pattern of both P. falciparum and P. vivax malaria in El Oro especially for P. vivax malaria incidence, which ceased to peak between June and July (Supplementary Figure S7). For P. falciparum a seasonal peak in incidence during this period was evident between June and July, but less distinct than before 2001.
Minimum temperature was the best temperature variable in the full models (Supplementary Table S2) and in the 3 months prior to case reporting was an important predictor of the spatio-temporal distribution of P. falciparum and P. vivax malaria in El Oro (Figure 2A). Warmer temperatures in El Oro between 1990 and 2018 were associated with increases in malaria incidence and greater increases in P. falciparum malaria than P. vivax malaria (Figure 2A). Precipitation lagged by 3 months for P. falciparum and 1 month for P. vivax were selected as the best time lags although when included in the full model, precipitation was not significant in driving malaria incidence in El Oro (Figure 2A). Introducing a non-linear relationship between malaria and climatic variables improved model fit by decreasing model DIC and RMSE for P. vivax but not P. falciparum malaria incidence (Table 1 and Supplementary Figure S8). We also found that higher temperatures were associated with larger increases in malaria incidence (Figure 2B).
Table 1. Adequacy results, deviance information criterion (DIC), Watanabe–Akaike Information Criterion (WAIC) and cross-validated log score for full models of P. falciparum and P. vivax malaria in El Oro 1990–2018.
We also investigated the influence of minimum temperature on the unexplained variation in P. falciparum and P. vivax incidence in El Oro between 1990 and 2018. We compared the monthly and interannual random effects of models with and without temperature to determine the extent to which temperature accounted for the seasonal pattern of malaria incidence. The monthly random effects of the model for P. falciparum incidence that included minimum temperature were near zero, showing that temperature accounted for all of the seasonal variation in P. falciparum malaria in El Oro 1990–2018 (Figure 3A). In contrast there was minimal reduction in the random effects for the P. vivax model with minimum temperature, indicating the seasonal pattern was driven by other factors (Figure 3B). The proportion of the variation accounted for by the yearly random effects in a model without minimum temperature was greater than the proportion of the variation accounted for by the monthly random effects in a model without minimum temperature. This suggests that temperature is the main driver of malaria seasonality and other interannual signatures as well as temperature contribute to the interannual variability of malaria in El Oro. For example, for some years the proportion of variation accounted for by the yearly random effects decreased when minimum temperature was included into models of malaria incidence 1990–2018. A reduction in the value of the random effects can be seen for 1998, when a strong El Niño event occurred (Supplementary Figure S9). Reductions were also particularly apparent for P. falciparum malaria between 2001–2004 and 2009–2011, suggesting that some additional variation was explained by minimum temperature. In contrast for some years there was no reduction for example in 2008, when an outbreak of P. vivax malaria occurred suggesting the outbreak was not driven by a climate event.
Figure 3. Effect of minimum temperature (Tmin) on the annual cycle of malaria in El Oro 1990–2018. Difference in the monthly random effect marginal posterior distributions for models of (A) P. falciparum and (B) P. vivax malaria that include minimum temperature (orange), lagged by 3 months and exclude minimum temperature (gray). Relative risk, on the log scale, is defined as the annual parasite incidence (API), log(ρst).
We explored how climate suitability for malaria transmission had changed over the time period 1990–2018 in El Oro, specifically for minimum temperature since it was an important predictor. To do this, we calculated the number of months that had suitable temperature conditions for transmission of each malaria parasite. We used the lower temperature limits of the range for which the development of the malaria parasites inside the mosquito vector is considered most suitable for transmission, as an indicator of this suitability.
For P. falciparum a lower temperature limit of 18°C was used and for P. vivax 15°C (Gilles, 1999; Watts et al., 2019). Between 1990 and 2018, the number of months with suitable temperatures for P. falciparum transmission showed an increasing trend (Figure 4), with noticeable peaks of around 8 months of suitable temperature conditions occurring between 1997 and 1998 and between 2014 and 2016. This trend of increasing temperatures for P. falciparum transmission coincides with warmer temperatures throughout the year in El Oro and increasing temperatures between 1990 and 2018 (Supplementary Figure S10). In contrast, temperature conditions for P. vivax malaria transmission showed no increasing trend in El Oro between 1990 and 2018 (Figure 4), although similar peaks in suitability were observed in 1997–1998 and in 2015–2016.
Figure 4. Suitable temperature conditions for malaria transmission in El Oro 1990–2018. Mean (solid curve) number of months per year where minimum temperature exceeds 18°C (i.e., considered suitable for P. falciparum malaria transmission; pink) and where minimum temperature exceeds 15°C (i.e., considered suitable for P. vivax malaria transmission; blue), logistic regression line (dashed curve) and 95% confidence intervals (gray shading).
The most parsimonious models of malaria in El Oro 1990–2018 included minimum temperature and precipitation as linear terms for P. falciparum and as non-linear functions for P. vivax malaria, poverty rates, level of urbanization including an interaction with the period when the vector control measures were implemented, and spatial and temporal random effects (Table 1). Although in these models the credible intervals for precipitation and poverty rates contained zero, the addition of these covariates to the models decreased DIC values and increased model fit for P. falciparum and P. vivax malaria incidence in El Oro between 1990 and 2018. Accounting for poverty rates (socioeconomic effects) across El Oro also decreased DIC and WAIC for models of P. falciparum and P. vivax malaria. Including meteorological variables (as linear terms for P. falciparum and as non-linear functions for P. vivax malaria) decreased the uncertainty of the model posterior distributions, in comparison to distributions from models that excluded meteorological variables (Figure 5). In particular, model uncertainty was reduced more for P. falciparum malaria, especially during the large outbreaks that occurred in 1992–1994 and 1998–2002. Interestingly during the later years of the study period, model posterior distributions show an upsurge in malaria incidence, particularly for P. vivax incidence between 2015 and 2018 although this upsurge was not observed in case reports.
Figure 5. Model posterior distributions with and without climate information for P. falciparum and P. vivax malaria in El Oro 1990–2018. Observed (gray solid line), posterior mean (blue dashed line) and 95% credible intervals (blue shading) for annual parasite incidence (API) for (A) models that include minimum temperature, lagged by 3 months and precipitation, lagged by 3 months for P. falciparum models and 1 month for P. vivax models and (B) without climate information. For models of P. falciparum malaria minimum temperature and precipitation were included as linear terms. For P. vivax malaria models, model fit improved when including non-linear functions of minimum temperature and precipitation.
We fitted separate sub-models for incidence of P. falciparum and P. vivax malaria in El Oro between 2001 and 2015, to evaluate the impact of the three vector control measures (IRS, space spraying and ULV fumigation) that were implemented during this period. These intervention models included the same covariates as the full models (linear and non-linear climate information and socioeconomic effects) and random effects but with the addition of the intervention data that were available for 2001–2015.
By comparing the differences in the covariate parameter estimates from the full models for the entire time series 1990–2018 and the intervention models for the period 2001–2015, we found that posterior mean estimates were roughly similar across the different models (Figure 6 and Supplementary Tables S4, S5). However, there was more uncertainty in the estimates for the environmental covariates in the intervention models compared to the 1990–2018 models, particularly for minimum temperature. We also found that between 2001 and 2015 areas in El Oro with greater levels of poverty were associated with higher incidences of P. vivax malaria. In the intervention models, IRS implemented in El Oro 2001–2015, 3 months prior to case detection was associated with decreased incidence of P. falciparum malaria but was not associated with significant decreases in incidence of P. vivax malaria. Space spraying was associated with decreased P. vivax malaria incidence in the following 3 months but not P. falciparum malaria in the following 2 months. ULV fumigation, also lagged by 3 months was not statistically significant (i.e., the credible intervals contained zero).
Figure 6. Posterior mean estimates for environmental, socioeconomic and malaria vector control covariates for models of (A) P. falciparum and (B) P. vivax malaria from full models (dark blue) and intervention models (green). All interventions were lagged by 3 months, apart from space spraying in the P. falciparum model, which was lagged by 2 months.
We also assessed which vector control measure implemented in El Oro between 2001 and 2015 provided more valuable information to the intervention models in explaining malaria incidence across the province. We found there was considerable heterogeneity in the model improvement for each control measure, as measured by RMSE difference (Figure 7). IRS improved the model for P. falciparum malaria in the coastal northwest of El Oro and in cantons along the Ecuador-Peru border, improving the model by up to 14%. In contrast, there was little improvement in model fit for P. vivax. Space spraying improved models of both P. falciparum and P. vivax in the province capital, Machala, with a greater improvement for P. vivax. ULV fumigation provided minimal or no model improvement for either parasite.
Figure 7. Model improvement for each vector control measure in El Oro 2001–2015. Model improvement, calculated as percentage change in root mean square error (RMSE) between models of P. falciparum and P. vivax malaria excluding each control measure, indoor residual spraying, ULV fumigation, and space spraying, and models including each measure. Positive values (green) show where the addition of the control measure reduces RMSE and negative values (purple) show where including the intervention does not improve the model. Gray areas show missing data.
Finally, we explored if the variation in malaria incidence in El Oro attributed to the vector control measures in the intervention model could be captured in the random effects structure of the full models. We compared the yearly random effects of the full models (fitted to data from 1990 to 2018), which did not include intervention data, to the yearly random effects of the intervention models (fitted to data from 2001 to 2015. We found that for some years (2001–2008) there was a reduction in the magnitude of the random effects for the 2001–2015 models, suggesting the control measures accounted for some of the unexplained variation in malaria incidence in the model for 1990–2018 during those years. However, a considerable portion of the random variation was likely due to other unmeasured factors. Between 2012 and 2015 the random effects of the P. falciparum models are close to zero, indicating that variation in malaria incidence for those years was well captured by factors included in the model (Figure 8). We found a similar pattern for P. vivax malaria, with a reduction in the value of the random effects for the intervention model, compared to the full model during the early years of the interventions 2001–2009. This reduction indicates that the control measures influenced the interannual variation of malaria risk during these years.
Figure 8. Interannual random effects for malaria risk in El Oro 1990–2018. Difference in the interannual random effect marginal posterior distributions for (A) P. falciparum malaria models and (B) P. vivax malaria models. Distributions from the intervention model are shown in green, which include intervention data. Distributions from the full model are shown in gray, which does not include intervention data. Relative risk, on the log scale, is defined as the annual parasite incidence (API), log(ρst).
For regions approaching malaria elimination and where funding and disease surveillance are limited, it is important to understand the drivers of malaria incidence in order to prevent disease re-establishment and to ensure that elimination efforts are sustained. In this study, we developed a statistical modeling framework to disentangle the relative role of multiple explanatory variables in driving variation in malaria incidence, while accounting for unobserved heterogeneity. We found that minimum temperature was an important driver of malaria incidence in El Oro. We also found that control measures had a differential impact on the two types of malaria. After the intensive period of vector control in El Oro between 2001 and 2015 malaria incidence in rural areas declined more than malaria in urbanized areas and P. vivax malaria became more dominant. Incidence of P. falciparum malaria was also reduced more than P. vivax malaria and relatively more P. vivax cases were reported in urbanized areas during this time period. The greater reduction in P. falciparum malaria is expected given that malaria control measures deployed during this time, are primarily designed for use in rural settings and target mosquito vectors that are exophagic such as An. albimanus, a dominant malaria vector in El Oro.
Indoor residual spraying has largely been shown to be effective in reducing malaria transmission in settings with high prevalence and when applied on a large scale, especially in African countries (Pluess et al., 2010). In this study we found that IRS was effective at reducing the incidence of P. falciparum malaria in El Oro between 2001 and 2015, but not P. vivax malaria. Conventional control methods are less effective in reducing transmission of P. vivax compared to P. falciparum malaria because of the ability of P. vivax to cause multiple relapsing malaria episodes after the initial infection, from the activation of dormant liver stages (hypnozoites). In addition the P. vivax parasite develops more rapidly in the mosquito than P. falciparum and so control methods such as IRS deployed in El Oro, which aim to shorten the mosquito lifespan are less effective (Mendis et al., 2001; Bassat et al., 2016). The rapid development of P. vivax and hypnozoite stage also means that drug treatments, which are often of a longer duration are less effective in reducing transmission as mosquitoes can become infected during a pre-symptomatic period, allowing for onward transmission before drug treatment is initiated (White, 2008; McCarthy et al., 2013).
We found that IRS only reduced the incidence of P. falciparum malaria and in the west of the province. IRS is most effective against endophilic mosquitoes, which rest indoors following a blood meal. The two main malaria in vectors in El Oro, A. albimanus and A. punctimacula, are most commonly observed biting outdoors and mainly rest outdoors (Ryan et al., 2017). As a result, IRS and other conventional control methods may not be suitable for reducing malaria transmitted by these mosquitoes.
In contrast we found that space spraying was effective in reducing incidence of P. vivax in El Oro, especially in the capital, Machala and by a larger amount than IRS. Evidence for the success of space spraying is limited and it is currently only recommended during outbreaks. In our study, space-spraying is possibly targeting outdoor resting mosquitoes, such as A. albimanus, especially in peri-urban areas where housing conditions are poor and outdoor exposure is higher. There is evidence of a positive effect of space-spraying on malaria incidence in India, but the current evidence base is limited (Pryce et al., 2018).
The P. vivax malaria parasite is able to develop at lower temperatures than P. falciparum (Nikolaev, 1935; Moshkovsky, 1946; Olliaro et al., 2016; Ohm et al., 2018), enabling its persistence in less favorable environmental conditions (Mendis et al., 2001). We found a stronger association between minimum temperature and P. falciparum malaria than P. vivax, and all of the seasonality in P. falciparum malaria was explained by temperature. A greater association between minimum temperature and P. falciparum malaria compared to P. vivax has been previously identified in China (Bi et al., 2013), but to our knowledge this difference has not been previously quantified in South America. The stronger effect of temperature on P. falciparum malaria is due to the greater dependence of P. falciparum transmission on the mosquito vector, which is sensitive to climate conditions such as temperature. In contrast P. vivax transmission is less influenced by the mosquito vector and can be sustained by unpredictable relapsing infections, which have been suggested to be explained by other factors such as systemic illness in humans (White, 2011). Similarly, between 2001 and 2015 we found that areas in El Oro with higher levels of poverty were associated with increased incidences of P. vivax malaria, which suggests that socioeconomic conditions, such as limited access to healthcare, may have influenced relapsing P. vivax infections during this time.
Warmer minimum temperatures in El Oro were associated with increases in P. falciparum and P. vivax malaria incidence 3 months later. This finding is in agreement with other studies in South America that have found a similar relationship between higher temperatures and malaria incidence (Poveda et al., 2000; Basurko et al., 2011; Laneri et al., 2019). This relationship can be attributed to the physiological effects of temperature on both the mosquito vector and parasite that increases transmission. For example warmer temperatures shorten the development time of the parasite inside the mosquito, the EIP and increase mosquito larval reproduction thus increasing disease transmission (Bayoh and Lindsay, 2003; Blanford et al., 2013; Mordecai et al., 2013). We also found a trend of rising minimum temperatures in El Oro between 1990 and 2018, with an increase in the number of months that have suitable minimum temperature conditions for P. falciparum malaria transmission, which suggests a lengthening of the malaria transmission season. Large peaks in the transmission suitability of both P. falciparum and P. vivax malaria are evident between 1997–1998 and 2014–2016, when major El Niño events occurred. El Niño conditions bring warmer and wetter conditions to southern Ecuador, conditions that are favorable for malaria transmission and have previously been suggested to have caused a peak in cases observed during this time (Krisher et al., 2016). Increasingly suitable temperature conditions and large-scale climate events such as El Niño pose a real threat for the re-establishment of malaria in El Oro if cases are allowed to return, surveillance is not maintained, and interventions are not deployed appropriately. Our model posterior distributions for 2012–2018 show increases in malaria incidence despite zero or few cases being reported, which is possibly due to warmer temperatures and the large El Niño event that occurred during this time. This highlights how incidence could rise if risks, such as relaxing control efforts are not mitigated.
Rainfall is essential for providing suitable habitats for mosquito breeding (Thomson et al., 2005; Parham and Michael, 2010) and is thus considered to be a dominant factor in driving malaria transmission. Studies in the Amazon and Argentina have found that rainfall was important in determining malaria incidence (Dantur Juri et al., 2009; Olson et al., 2009). In this study, rainfall was not a statistically significant explanatory variable for malaria incidence in El Oro, possibly because ample larval habitat was already available. Two to three months prior to the peak malaria season (March–June), monthly rainfall accumulation reaches 80 mm across El Oro, which is considered to be suitable conditions for malaria transmission (Watts et al., 2019), and up to 530 mm rainfall in the southeast of the province. In addition, much of El Oro is rural with extensive mangroves in the northwest coastal regions, which provide habitat suited to A. albimanus mosquitoes (Pinault and Hunter, 2012). Therefore, our results suggest that rainfall is not a limiting factor for malaria transmission in El Oro.
The addition of temporal random effects for each year in the full models for 1990–2018 accounted for some of the variation in malaria incidence due to the control measures that were explicitly accounted for in the intervention model for 2001–2015, particularly for P. falciparum malaria, which appeared more sensitive to interventions. The use of temporal random effects also allowed us to pick up variation in malaria in El Oro due to other unobserved factors. For example, the large El Niño events mentioned previously likely caused an increased number of cases and a reduction in the unexplained yearly variation during 1997–1998 and between 2014 and 2016. However, we expect that the temperature and precipitation variables included in the models to pick up some variation to due climatic anomalies from El Niño events. The Cenepa War, a period of political instability across the Ecuador-Peru border (Krisher et al., 2016) likely hindered malaria control efforts and case reporting during 1995, and there is evidence of reduced malaria risk during this time in the interannual random effects, which is likely explained by reduced reporting. In addition we found that the outbreak of P. vivax in 2008 was not driven by a climatic event and may instead be driven by a political event or lapse in control efforts.
Despite the considerable length of the dataset, there are some limitations to consider. In Ecuador a high number of malaria cases are asymptomatic. Low levels of parasitemia, which act as a reservoir of transmission, are difficult to diagnose (Sáenz et al., 2017). It is likely that many cases of P. vivax malaria were missed and not reported during the study period. In addition, many P. vivax cases reported between 1990 and 2018 are likely to be relapses from the same initial infection, which could mask the true climate-malaria relationship. We also found that urban malaria was approximately 70% higher than incidence of malaria in rural areas. However, this may be a product of migration from neighboring countries and cases being imported from rural areas and subsequently recorded as originating in urban areas, as was found in Colombia (Molina Gómez et al., 2017). We could not quantify the amount of malaria variation explained by migration owing to the lack of detailed migration data for the whole time period. Due to El Oro’s location on a key migratory route, including for Venezuelan refugees, it is likely that human movements are influential in driving case reporting and malaria incidence. In addition, outbreaks in northern Peru can increase the risk of malaria transmission in El Oro due to cross border human movement. Other factors that likely contributed to the spatiotemporal variation in malaria incidence in El Oro that were accounted for through the use of random effects include variations in case reporting and surveillance, as well as health seeking behavior in El Oro.
The incompleteness of the intervention data for the study period is also a limiting factor in this study. We were only able to evaluate the role of the three control measures implemented between 2001 and 2015 although there were other important elimination efforts that were carried out in El Oro between 1990 and 2018. For example, the use of DDT was widely used until 1996 and a campaign to distribute insecticide-treated bed nets (ITNs) was initiated in 2004, although no detailed data exist for this in El Oro, or for other forms of personal protection that would have contributed to local elimination. However, by examining the interannual random effects of our models we observe a reduction in P. falciparum malaria risk between 2004 and 2006, which could be attributed to the use of ITNs. In 2005, the Ministry of Health suspended the use of chloroquine for treatment of P. falciparum malaria, adopting the recommended change to artemisinin-based combination therapy (ACT) amid reports of drug resistance, which local partners reported led to a major decline in transmission (Krisher et al., 2016). The introduction of ACT in Colombia and Peru at similar times contributed to significant reductions in malaria case numbers (Rodríguez et al., 2011; Quispe et al., 2016). ACT introduction is also likely to have contributed to local malaria elimination El Oro as we observed declining malaria risk from this time through the use of interannual random effects in our models. At the same time in El Oro, a new shorter treatment regime for P. vivax malaria was adopted to ensure patients completed their treatment course, which was also reported to have contributed to declining transmission (Krisher et al., 2016).
In summary, we have developed a modeling framework for exploring the barriers to malaria elimination in a low-transmission setting. Accounting for unobserved interannual variation via yearly random effects is useful for investigating malaria variation in many different contexts where detailed data, such as intervention information, may not be available. Here, we used available intervention data to explore the relative impact of three vector control measures on P. falciparum and P. vivax malaria in El Oro. We also provide an assessment of where control measures improved models of P. falciparum and P. vivax incidence, which will prove useful for targeting future malaria control efforts in the region. We have shown that warmer temperatures increase incidence of P. falciparum malaria, which is important to consider in light of global environmental change and increasing climate suitability for malaria transmission (Laporta et al., 2015; Watts et al., 2019). In addition, with a global warming of 1.5°C above pre-industrial levels it is predicted that the western coast of South America, including southern Ecuador, will experience temperatures between 2 and 3°C warmer and precipitation could increase by up to 20% (IPCC, 2018), providing climate conditions that are highly suitable for malaria transmission. In contrast, we show that P. vivax malaria is less sensitive to temperature variations and shows more complex transmission patterns, making it particularly challenging to eliminate (Feachem et al., 2010). An assessment of the environmental obstacles to elimination efforts in Ecuador is timely and important. Lapses in control efforts should be avoided, especially as it becomes harder to prevent and detect cases due to limited funding for surveillance and control. Recently, indigenous cases of malaria have been detected in El Oro and re-introduction of malaria parasites along with warming temperatures threaten current elimination progress.
The data and code used to produce the analysis are available on GitHub via the following link: https://github.com/isabelfletcher/malaria_eloro.
IF and RL conceived the study. IF collated the data, fitted the models, analyzed the results, and wrote the manuscript. AS-I helped conceive the study, provided information on the vector control measures, and contributed to discussion of the study results. RS provided insight on vector-borne diseases and surveillance in El Oro. GC-E helped with the environmental data sources and extraction. MS, EB-A, TO, and JA provided the malaria case data and intervention data for analysis. FS provided insight on the malaria situation in Ecuador, including treatments, and diagnostics. KJ provided input on model formulation. KJ and CD provided input on interpretation of model results. RL and AS-I edited the manuscript. All authors critically reviewed and approved the final version of the manuscript. Special recognition of MS, posthumously (1959–2020), a leader in the field of medical entomology and tireless advocate for community public health in El Oro. All authors contributed to the article and approved the submitted version.
IF was supported by the Biotechnology and Biological Sciences Research Council (grant BB/M009513/1). RL was supported by a Royal Society Dorothy Hodgkin Fellowship. RL and GC-E were supported by the National Socio-Environmental Synthesis Center (SESYNC) under funding from the National Science Foundation (DBI-1639145).
The authors declare that the research was conducted in the absence of any commercial or financial relationships that could be construed as a potential conflict of interest.
We thank all those at the Ministerio de Salud Pública, Machala, Ecuador for their kind hospitality and support for this work, Leonardo Ortega-López for his thoughtful discussion on malaria in Ecuador, members of KJ Lab Group at UCL for their discussion on data collation and analysis, and finally members of the Planetary Health and Infectious Diseases group at LSHTM for their support and discussion on this work.
The Supplementary Material for this article can be found online at: https://www.frontiersin.org/articles/10.3389/fenvs.2020.00135/full#supplementary-material
Abatzoglou, J. T., Dobrowski, S. Z., Parks, S. A., and Hegewisch, K. C. (2018). TerraClimate, a high-resolution global dataset of monthly climate and climatic water balance from 1958–2015. Sci. Data 5:170191. doi: 10.1038/sdata.2017.191
Alimi, T. O., Fuller, D. O., Qualls, W. A., Herrera, S. V., Arevalo-Herrera, M., Quinones, M. L., et al. (2015). Predicting potential ranges of primary malaria vectors and malaria in northern South America based on projected changes in climate, land cover and human population. Paras. Vect. 8:431. doi: 10.1186/s13071-015-1033-9
Aregawi, M. W., Ali, A. S., Al-mafazy, A., Molteni, F., Katikiti, S., Warsame, M., et al. (2011). Reductions in malaria and anaemia case and death burden at hospitals following scale-up of malaria control in Zanzibar, 1999-2008. Malaria J. 10:46. doi: 10.1186/1475-2875-10-46
Bassat, Q., Velarde, M., Mueller, I., Lin, J., Leslie, T., Wongsrichanalai, C., et al. (2016). Key knowledge gaps for plasmodium vivax control and elimination. Am. J. Trop. Med. Hyg. 95(Suppl. 6), 62–71. doi: 10.4269/ajtmh.16-0180
Basurko, C., Hanf, M., Han-Sze, R., Rogier, S., Heritier, P., Grenier, C., et al. (2011). Influence of climate and river level on the incidence of malaria in Cacao. French Guiana. Malaria J. 10:26. doi: 10.1186/1475-2875-10-26
Bayoh, M. N., and Lindsay, S. W. (2003). Effect of temperature on the development of the aquatic stages of Anopheles gambiae sensu stricto (Diptera: Culicidae). Bull. Entomol. Res. 93, 375–381. doi: 10.1079/BER2003259
Beck-Johnson, L. M., Nelson, W. A., Paaijmans, K. P., Read, A. F., Thomas, M. B., and Bjørnstad, O. N. (2013). The effect of temperature on Anopheles mosquito population dynamics and the potential for malaria transmission. PLoS One 8:e79276. doi: 10.1371/journal.pone.0079276
Bennett, A., Yukich, J., Miller, J. M., Vounatsou, P., Hamainza, B., Ingwe, M. M., et al. (2014). A methodological framework for the improved use of routine health system data to evaluate national malaria control programs: evidence from Zambia. Populat. Health Metrics 12:30. doi: 10.1186/s12963-014-0030-0
Besag, J., York, J., and Mollié, A. (1991). Bayesian image restoration, with two applications in spatial statistics (with discussion). Ann. Instit. Statist. Math. 43, 1–59.
Bi, Y., Yu, W., Hu, W., Lin, H., Guo, Y., Zhou, X.-N., et al. (2013). Impact of climate variability on Plasmodium vivax and Plasmodium falciparum malaria in Yunnan Province, China. Paras. Vect. 6:357. doi: 10.1186/1756-3305-6-357
Blanford, J. I., Blanford, S., Crane, R. G., Mann, M. E., Paaijmans, K. P., Schreiber, K. V., et al. (2013). Implications of temperature variation for malaria parasite development across Africa. Sci. Rep. 3:1300. doi: 10.1038/srep01300
Burkett-Cadena, N. D., and Vittor, A. Y. (2017). Deforestation and vector-borne disease: forest conversion favors important mosquito vectors of human pathogens. Basic Appl. Ecol. 26, 101–110. doi: 10.1016/j.baae.2017.09.012
Calil, J., Reguero, B. G., Zamora, A. R., Losada, I. J., and Méndez, F. J. (2017). Comparative Coastal Risk Index (CCRI): a multidisciplinary risk index for Latin America and the Caribbean. PLoS One 12:e0187011. doi: 10.1371/journal.pone.0187011
Caminade, C., Kovats, S., Rocklov, J., Tompkins, A. M., Morse, A. P., Colón-González, F. J., et al. (2014). Impact of climate change on global malaria distribution. Proc. Natl. Acad. Sci. U.S.A. 111, 3286–3291. doi: 10.1073/pnas.1302089111
Conn, J. E., Grillet, M. E., Correa, M., and Sallum, M. A. M. (2018). Malaria Transmission in South America—Present Status and Prospects for Elimination, Towards Malaria Elimination. London: IntechOpen Limited.
Conn, J. E., Wilkerson, R. C., Segura, M. N. O., de Souza, R. T. L., Schlichting, C. D., Wirtz, R. A., et al. (2002). Emergence of a new neotropical malaria vector facilitated by human migration and changes in land use. Am. J. Trop. Med. Hygiene 66, 18–22. doi: 10.4269/ajtmh.2002.66.18
Daniels, J. P. (2018). Increasing malaria in Venezuela threatens regional progress. Lancet Infect. Dis. 18:257. doi: 10.1016/S1473-3099(18)30086-0
Dantur Juri, M. J., Zaidenberg, M., Claps, G. L., Santana, M., and Almiron, W. R. (2009). Malaria transmission in two localities in north-western Argentina. Malaria J. 8:18. doi: 10.1186/1475-2875-8-18
Feachem, R. G. A., Phillips, A. A., Hwang, J., Cotter, C., Wielgosz, B., Greenwood, B. M., et al. (2010). Shrinking the malaria map: progress and prospects. Lancet 376, 1566–1578. doi: 10.1016/S0140-6736(10)61270-6
Gagnon, A. S., Smoyer-Tomic, K. E., and Bush, A. B. G. (2002). The El Niño Southern oscillation and malaria epidemics in South America. Int. J. Biometeorol. 46, 81–89. doi: 10.1007/s00484-001-0119-6
Galardo, A. K. R., Zimmerman, R. H., Lounibos, L. P., Young, L. J., Galardo, C. D., Arruda, M., et al. (2009). Seasonal abundance of anopheline mosquitoes and their association with rainfall and malaria along the Matapi River, Amapa, [corrected] Brazil. Med. Veter. Entomol. 23, 335–349. doi: 10.1111/j.1365-2915.2009.00839.x
Giardina, F., Kasasa, S., Sie, A., Utzinger, J., Tanner, M., and Vounatsou, P. (2014). Effects of vector-control interventions on changes in risk of malaria parasitaemia in sub-Saharan Africa: a spatial and temporal analysis. Lancet Glob. Health 2, e601–e615. doi: 10.1016/S2214-109X(14)70300-6
Gneiting, T., and Raftery, A. E. (2007). Strictly proper scoring rules, prediction, and estimation. J. Am. Stat. Assoc. 102, 359–378. doi: 10.1198/016214506000001437
Graves, P., Osgood, D., Thomson, M., Sereke, K., Araia, A., Zerom, M., et al. (2008). Effectiveness of malaria control during changing climate conditions in Eritrea, 1998-2003. Trop. Med. Int. Health 13, 218–228. doi: 10.1111/j.1365-3156.2007.01993.x
Grillet, M. E., Hernández-Villena, J. V., Llewellyn, M. S., Paniz-Mondolfi, A. E., Tami, A., Vincenti-Gonzalez, M. F., et al. (2019). Venezuela’s humanitarian crisis, resurgence of vector-borne diseases, and implications for spillover in the region. Lancet Infect. Dis. 19, e149–e161. doi: 10.1016/S1473-3099(18)30757-6
Hahn, M. B., Gangnon, R. E., Barcellos, C., Asner, G. P., and Patz, J. A. (2014). Influence of deforestation, logging, and fire on malaria in the Brazilian Amazon. PLoS One 9:e85725. doi: 10.1371/journal.pone.0085725
IPCC (2018). Global Warming of 15°C. An IPCC Special Report on the Impacts of Global Warming of 15°C above Pre-industrial Levels and Related Global Greenhouse Gas Emission Pathways, in the Context of Strengthening the Global Response to the Threat of Climate Change. Geneva: IPCC.
Jaramillo-Ochoa, R., Sippy, R., Farrell, D. F., Cueva-Aponte, C., Beltrán-Ayala, E., Gonzaga, J. L., et al. (2019). Effects of political instability in venezuela on malaria resurgence at Ecuador–Peru Border, 2018. Emerg. Infect. Dis. J. 25, 834–836. doi: 10.3201/eid2504.181355
Krisher, L. K., Krisher, J., Ambuludi, M., Arichabala, A., Beltrán-Ayala, E., Navarrete, P., et al. (2016). Successful malaria elimination in the Ecuador–Peru border region: epidemiology and lessons learned. Malaria J. 15:573. doi: 10.1186/s12936-016-1630-x
Laneri, K., Cabella, B., Prado, P. I., Mendes Coutinho, R., and Kraenkel, R. A. (2019). Climate drivers of malaria at its southern fringe in the Americas. PLoS One 14:e0219249. doi: 10.1371/journal.pone.0219249
Laporta, G. Z., Linton, Y.-M., Wilkerson, R. C., Bergo, E. S., Nagaki, S. S., Sant’Ana, D. C., et al. (2015). Malaria vectors in South America: current and future scenarios. Paras. Vect. 8:426. doi: 10.1186/s13071-015-1038-4
Lowe, R., Cazelles, B., Paul, R., and Rodó, X. (2016). Quantifying the added value of climate information in a spatio-temporal dengue model. Stochas. Environ. Res. Risk Assess. 30, 2067–2078. doi: 10.1007/s00477-015-1053-1
Lowe, R., Stewart-Ibarra, A. M., Petrova, D., García-Díez, M., Borbor-Cordova, M. J., Mejía, R., et al. (2017). Climate services for health: predicting the evolution of the 2016 dengue season in Machala, Ecuador. Lancet Planet. Health 1, e142–e151. doi: 10.1016/S2542-5196(17)30064-5
McCarthy, J. S., Griffin, P. M., Sekuloski, S., Bright, A. T., Rockett, R., Looke, D., et al. (2013). Experimentally induced blood-stage Plasmodium vivax infection in healthy volunteers. J. Infect. Dis. 208, 1688–1694. doi: 10.1093/infdis/jit394
Mendis, K., Sina, B. J., Marchesini, P., and Carter, R. (2001). The neglected burden of Plasmodium vivax malaria. Am. J. Trop. Med. Hygiene 64(Suppl. 1–2), 97–106. doi: 10.1179/2047772413Z.000000000179
Molina Gómez, K., Caicedo, M. A., Gaitán, A., Herrera-Varela, M., Arce, M. I., Vallejo, A. F., et al. (2017). Characterizing the malaria rural-to-urban transmission interface: the importance of reactive case detection. PLoS Negl. Trop. Dis. 11:e0005780. doi: 10.1371/journal.pntd.0005780
Mordecai, E. A., Paaijmans, K. P., Johnson, L. R., Balzer, C., Ben-Horin, T., de Moor, E., et al. (2013). Optimal temperature for malaria transmission is dramatically lower than previously predicted. Ecol. Lett. 16, 22–30. doi: 10.1111/ele.12015
Moreno, A. R. (2006). Climate change and human health in Latin America: drivers, effects, and policies. Reg. Environ. Change 6, 157–164. doi: 10.1007/s10113-006-0015-z
Moreno, J. E., Rubio-Palis, Y., Paez, E., Perez, E., and Sanchez, V. (2007). Abundance, biting behaviour and parous rate of anopheline mosquito species in relation to malaria incidence in gold-mining areas of southern Venezuela. Med. Vet. Entomol. 21, 339–349. doi: 10.1111/j.1365-2915.2007.00704.x
Moshkovsky, S. (1946). On the dependency of the speed of development of plasmodia malaria on temperature. Med. Para Para Bol. 15, 19–32.
Nikolaev, B. P. (1935). On the influence of temperature on the development of malaria plasmodia inside the mosquito. Trans. Pasteur Inst. Epi. Bact. Leningrad. 2, 1–5.
Ohm, J. R., Baldini, F., Barreaux, P., Lefevre, T., Lynch, P. A., Suh, E., et al. (2018). Rethinking the extrinsic incubation period of malaria parasites. Paras. Vect. 11:178. doi: 10.1186/s13071-018-2761-4
Olliaro, P. L., Barnwell, J. W., Barry, A., Mendis, K., Mueller, I., Reeder, J. C., et al. (2016). Implications of Plasmodium vivax biology for control, elimination, and research. Am. J. Trop. Med. Hygiene 95(Suppl. 6), 4–14. doi: 10.4269/ajtmh.16-0160
Olson, S. H., Gangnon, R., Elguero, E., Durieux, L., Guegan, J. F., Foley, J. A., et al. (2009). Links between climate, malaria, and wetlands in the Amazon Basin. Emerg. Infect. Dis. 15, 659–662. doi: 10.3201/eid1504.080822
Paaijmans, K. P., Read, A. F., and Thomas, M. B. (2009). Understanding the link between malaria risk and climate. Proc. Natl. Acad. Sci. U.S.A. 106, 13844–13849. doi: 10.1073/pnas.0903423106
Padilla, J. C., Chaparro, P. E., Molina, K., Arevalo-Herrera, M., and Herrera, S. (2015). Is there malaria transmission in urban settings in Colombia? Malaria J. 14, 453. doi: 10.1186/s12936-015-0956-0
Parham, P. E., and Michael, E. (2010). Modeling the effects of weather and climate change on malaria transmission. Environ. Health Perspect. 118, 620–626. doi: 10.1289/ehp.0901256
Patz, J. A., and Olson, S. H. (2006). Malaria risk and temperature: influences from global climate change and local land use practices. Proc. Natl. Acad. Sci. U.S.A. 103, 5635–5636. doi: 10.1073/pnas.0601493103
Pinault, L. L., and Hunter, F. F. (2012). Characterization of larval habitats of Anopheles albimanus, Anopheles pseudopunctipennis, Anopheles punctimacula, and Anopheles oswaldoi s.l. populations in lowland and highland Ecuador. J. Vect. Ecol. 37, 124–136. doi: 10.1111/j.1948-7134.2012.00209.x
Pluess, B., Tanser, F. C., Lengeler, C., and Sharp, B. L. (2010). Indoor residual spraying for preventing malaria. Cochrane Database Syst. Rev. 2010:CD006657. doi: 10.1002/14651858.CD006657.pub2
Poveda, G., Graham, N. E., Epstein, P. R., Rojas, W., Quiñones, M. L., Vélez, I. D., et al. (2000). “Climate and ENSO variability associated with vector-borne diseases in Colombia,” in El Niño and the Southern Oscillation: Multiscale Variability and Global and Regional Impacts, eds H. F. Diaz and V. Markgraf (Cambridge: Cambridge University Press), 183–204. doi: 10.1017/cbo9780511573125.007
Prussing, C., Saavedra, M., Bickersmith, S., Alava, F., Guzmán, M., Manrique Valverde, E. J., et al. (2019). Malaria vector species in Amazonian Peru co-occur in larval habitats but have distinct larval microbial communities. PLoS Negl. Trop. Dis. 13:e0007412. doi: 10.1371/journal.pntd.0007412
Pryce, J., Choi, L., Richardson, M., and Malone, D. (2018). Insecticide space spraying for preventing malaria transmission. Cochrane Database Syst. Rev. 11:CD012689. doi: 10.1002/14651858.CD012689.pub2
Quispe, A. M., Llanos-Cuentas, A., Rodriguez, H., Clendenes, M., Cabezas, C., Leon, L. M., et al. (2016). Accelerating to zero: strategies to eliminate Malaria in the Peruvian Amazon. Am. J. Trop. Med. Hygiene 94, 1200–1207. doi: 10.4269/ajtmh.15-0369
Recht, J., Siqueira, A. M., Monteiro, W. M., Herrera, S. M., Herrera, S., and Lacerda, M. V. G. (2017). Malaria in Brazil, Colombia, Peru and Venezuela: current challenges in malaria control and elimination. Malaria J. 16:272. doi: 10.1186/s12936-017-1925-6
Rodríguez, J. C. P., Uribe, G. Á, Araújo, R. M., Narváez, P. C., and Valencia, S. H. (2011). Epidemiology and control of malaria in Colombia. Memorias Instit. Oswaldo Cruz. 106(Suppl. 1), 114–122. doi: 10.1590/s0074-02762011000900015
Rue, H., Martino, S., and Chopin, N. (2009). Approximate Bayesian inference for laten Gaussian models by using integrated nested Laplace approximations. J. R. Stat. Soc. Ser. B 71, 319–392. doi: 10.1111/j.1467-9868.2008.00700.x
Ryan, S. J., Lippi, C. A., Boersch-Supan, P. H., Heydari, N., Silva, M., Adrian, J., et al. (2017). Quantifying seasonal and diel variation in Anopheline and Culex human biting rates in Southern Ecuador. Malaria J. 16:479. doi: 10.1186/s12936-017-2121-4
Sáenz, F. E., Arévalo-Cortés, A., Valenzuela, G., Vallejo, A. F., Castellanos, A., Poveda-Loayza, A. C., et al. (2017). Malaria epidemiology in low-endemicity areas of the northern coast of Ecuador: high prevalence of asymptomatic infections. Malaria J. 16:300. doi: 10.1186/s12936-017-1947-0
Siraj, A. S., Santos-Vega, M., Bouma, M. J., Yadeta, D., Carrascal, D. R., and Pascual, M. (2014). Altitudinal changes in malaria incidence in highlands of Ethiopia and Colombia. Science 343, 1154–1158. doi: 10.1126/science.1244325
Spiegelhalter, D. J., Best, N. G., Carlin, B. P., and Van Der Linde, A. (2002). Bayesian measures of model complexity and fit. J. R. Stat. Soc. Ser. B 64, 583–639. doi: 10.1111/1467-9868.00353
Stefani, A., Dusfour, I., Corrêa, A. P. S. A., Cruz, M. C. B., Dessay, N., Galardo, A. K. R., et al. (2013). Land cover, land use and malaria in the Amazon: a systematic literature review of studies using remotely sensed data. Malaria J. 12:192. doi: 10.1186/1475-2875-12-192
Tauzer, E., Borbor-Cordova, M. J., Mendoza, J., De La Cuadra, T., Cunalata, J., and Stewart-Ibarra, A. M. (2019). A participatory community case study of periurban coastal flood vulnerability in southern Ecuador. PLoS One 14:e0224171. doi: 10.1371/journal.pone.0224171
Thomson, M. C., Mason, S. J., Phindela, T., and Connor, S. J. (2005). Use of rainfall and sea surface temperature monitoring for malaria early warning in Botswana. Am. J. Trop. Med. Hygiene 73, 214–221. doi: 10.4269/ajtmh.2005.73.214
Tucker Lima, J. M., Vittor, A., Rifai, S., and Valle, D. (2017). Does deforestation promote or inhibit malaria transmission in the Amazon? A systematic literature review and critical appraisal of current evidence. Philos. Trans. R. Soc. Lond. Ser. B Biol. Sci. 372:20160125. doi: 10.1098/rstb.2016.0125
Vittor, A. Y., Gilman, R. H., Tielsch, J., Glass, G., Shields, T., Lozano, W. S., et al. (2006). The effect of deforestation on the human-biting rate of Anopheles darlingi, the primary vector of Falciparum malaria in the Peruvian Amazon. Am. J. Trop. Med. Hygiene 74, 3–11. doi: 10.4269/ajtmh.2006.74.3
Vittor, A. Y., Pan, W., Gilman, R. H., Tielsch, J., Glass, G., Shields, T., et al. (2009). Linking deforestation to Malaria in the Amazon: characterization of the breeding habitat of the principal malaria vector, Anopheles darlingi. Am. J. Trop. Med. Hygiene 81, 5–12.
Watanabe, S. (2010). Asymptotic equivalence of bayes cross validation and widely applicable information criterion in singular learning theory. J. Mach. Learn. Res. 11, 3571–3594.
Watts, N., Amann, M., Arnell, N., Ayeb-Karlsson, S., Belesova, K., Boykoff, M., et al. (2019). The 2019 report of the lancet countdown on health and climate change: ensuring that the health of a child born today is not defined by a changing climate. Lancet 394, 1836–1878. doi: 10.1016/S0140-6736(19)32596-6
Wayant, N. M., Maldonado, D., Rojas, de Arias, A., Cousino, B., and Goodin, D. G. (2010). Correlation between normalized difference vegetation index and malaria in a subtropical rain forest undergoing rapid anthropogenic alteration. Geospatial Health 4, 179–190. doi: 10.4081/gh.2010.199
White, N. J. (2008). The role of anti-malarial drugs in eliminating malaria. Malaria J. 7:S8. doi: 10.1186/1475-2875-7-S1-S8
White, N. J. (2011). Determinants of relapse periodicity in Plasmodium vivax malaria. Malaria J. 10:297. doi: 10.1186/1475-2875-10-297
Keywords: malaria, environmental change, elimination, vector control, climate, spatio-temporal model
Citation: Fletcher IK, Stewart-Ibarra AM, Sippy R, Carrasco-Escobar G, Silva M, Beltran-Ayala E, Ordoñez T, Adrian J, Sáenz FE, Drakeley C, Jones KE and Lowe R (2020) The Relative Role of Climate Variation and Control Interventions on Malaria Elimination Efforts in El Oro, Ecuador: A Modeling Study. Front. Environ. Sci. 8:135. doi: 10.3389/fenvs.2020.00135
Received: 27 February 2020; Accepted: 16 July 2020;
Published: 27 August 2020.
Edited by:
Zed Zulkafli, University Putra Malaysia, MalaysiaReviewed by:
Lingxin Chen, Yantai Institute of Coastal Zone Research (CAS), ChinaCopyright © 2020 Fletcher, Stewart-Ibarra, Sippy, Carrasco-Escobar, Silva, Beltran-Ayala, Ordoñez, Adrian, Sáenz, Drakeley, Jones and Lowe. This is an open-access article distributed under the terms of the Creative Commons Attribution License (CC BY). The use, distribution or reproduction in other forums is permitted, provided the original author(s) and the copyright owner(s) are credited and that the original publication in this journal is cited, in accordance with accepted academic practice. No use, distribution or reproduction is permitted which does not comply with these terms.
*Correspondence: Isabel K. Fletcher, SXNhYmVsLkZsZXRjaGVyQGxzaHRtLmFjLnVr; Rachel Lowe, UmFjaGVsLkxvd2VAbHNodG0uYWMudWs=
Disclaimer: All claims expressed in this article are solely those of the authors and do not necessarily represent those of their affiliated organizations, or those of the publisher, the editors and the reviewers. Any product that may be evaluated in this article or claim that may be made by its manufacturer is not guaranteed or endorsed by the publisher.
Research integrity at Frontiers
Learn more about the work of our research integrity team to safeguard the quality of each article we publish.