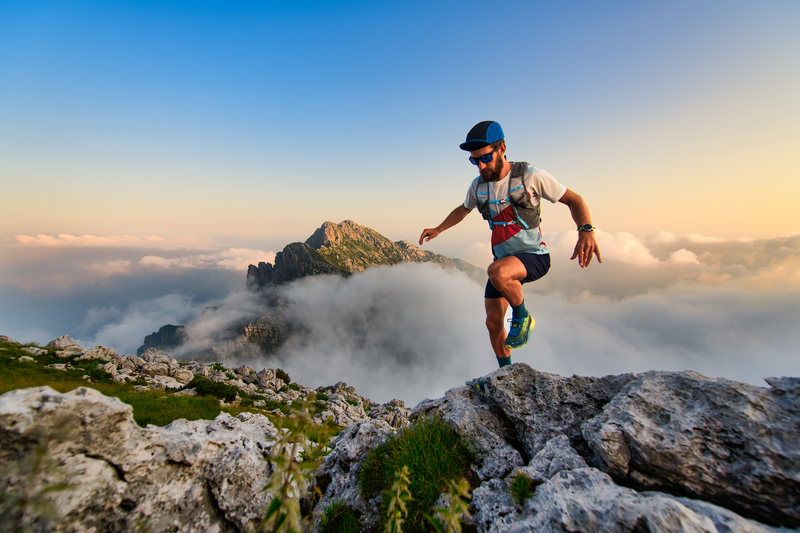
94% of researchers rate our articles as excellent or good
Learn more about the work of our research integrity team to safeguard the quality of each article we publish.
Find out more
SYSTEMATIC REVIEW article
Front. Environ. Sci. , 21 August 2020
Sec. Toxicology, Pollution and the Environment
Volume 8 - 2020 | https://doi.org/10.3389/fenvs.2020.00131
This article is part of the Research Topic Microplastics in the Marine Environment: Sources, Distribution, Biological Effects and Socio-Economic Impacts View all 24 articles
Background: Ecological impacts of micro- and nanoplastics particles (MNP) are among the most discussed environmental concerns. In algae, MNP are commonly hypothesized to reduce growth, which is a standard ecotoxicological endpoint. However, the reported test outcomes vary, with both growth inhibition and stimulation being observed. Due to this conflict of information, a data synthesis for MNP potential to cause growth inhibition in toxicity testing is needed.
Methods: We performed a meta-analysis study to assess the effect of MNP exposure on algal growth. Twenty studies published between 2010 and 2020 and representing 16 algal species and five polymer materials administered as particles in size range 0.04–3,000 μm were included in this meta-analysis. A random-effect model was used to estimate the effect size in three datasets: (1) Low concentration range (<100 mg/L), (2) High concentration range (≥100 mg/L), and (3) Full range model (0.004–1,100 mg/L), which encompassed all studies using the combination of experimental settings (test species, MNP concentration, polymer material, and particle size) yielding the highest effect size within a study.
Results: The exposure to MNP was not significantly associated with growth inhibition in any of the models tested. However, a high heterogeneity between the studies was found in all three models. Neither MNP concentration nor polymer material contributed significantly to the heterogeneity, whereas polymer density had a significant moderating effect, with a higher risk of growth inhibition at lower densities. We also identified a publication bias, with small studies that reported significant inhibition being overrepresented in our dataset.
Conclusions: The meta-analysis found limited evidence for MNP effect on microalgal growth in the standard algal growth inhibition test. The heterogeneity and varying methodological quality of studies limited the interpretation and the confidence in the findings. For hazard assessment, standardization and controlled exposure are needed as well as more sensitive endpoints that can inform us about the effect mechanisms. Finally, using particle-free controls in such tests cannot account for the presence of inert particulates in the test system, and, hence, does not allow to attribute observed effects to the test polymers.
The pollution by plastic litter is ubiquitous in aquatic environments, both freshwater (Rodrigues et al., 2018) and marine (Anderson et al., 2016). Once released in the environment, plastics undergo weathering and fragmentation due to chemical, mechanical, and biological degradation. These processes generate smaller particles collectively known as secondary micro- (MP; <5 mm) and nanoplastics (NP; <0.1 μm), the main contributors to an environmental load of plastics (Andrady, 2011). Primary MP (e.g., microbeads) manufactured for use in industry, personal care products, and medicine are also contributing to the plastic pollution worldwide, although to a lesser extent than secondary MP and NP.
Given the global nature of the plastic littering, accurate characterization of the impacts of these environmental contaminants is essential for risk assessment. The current concern is that both MP and NP (MNP) are harmful to aquatic biota. However, while some studies report adverse effects (Casado et al., 2013; Della Torre et al., 2014; Au et al., 2015), no-effect observations in laboratory tests with plants and animals are also common (Watts et al., 2016; Long et al., 2017; Chae et al., 2018). Moreover, the effect mechanisms specific for MNP in microorganisms, plants, and animals are largely unknown. Due to this conflict of information, more studies on environmental fate and biological effects of these emerging environmental contaminants in various organisms are needed.
The exposure to anthropogenic polymer particles is particularly relevant for lower trophic levels within food webs (Yokota et al., 2017), because (1) this is the entry point into the food web affecting the baseline load of MNP in consumers, (2) negative impacts on primary producers and primary consumers may change food resources and translate into adverse effects on higher consumers and food web functions, and (3) interactions of MNP with bacterio- and microplankton may have implications for biogeochemical cycling in ecosystems (Rogers et al., 2020). Moreover, similar to other particulates in the water, MNP form aggregates with detritus and microorganisms, which facilitate sedimentation and transfer of these contaminants in the food web but can also affect microbial growth and physiology (Ward and Kach, 2009; Long et al., 2017; Mao et al., 2018) and downward fluxes in the system (Rogers et al., 2020). Therefore, exposure of algae and other microorganisms to MNP should be a part of the risk assessment for plastic litter.
It has been shown that exposure to MNP can inhibit algal growth (Casado et al., 2013; Besseling et al., 2014; Bergami et al., 2017), chlorophyll content (Besseling et al., 2014; Zhang et al., 2017), and photosynthetic activity (Bhattacharya et al., 2010). For example, Besseling et al. (2014) reported a typical dose-response for the freshwater alga Scenedesmus obliquus exposed to polystyrene (PS). However, the particle concentrations inducing significant growth inhibition are much higher than MP concentrations reported from the aquatic environments (Eerkes-Medrano et al., 2015; Li et al., 2018). When the test concentrations resemble the levels of suspended solids that are typical for surface waters, the observed effects on algal growth and physiology appear to be limited (e.g., Long et al., 2017; Prata et al., 2018) or even positive, i.e., growth stimulation can be observed (e.g., Yokota et al., 2017; Canniff and Hoang, 2018). Also, the observed responses may vary during the exposure; for instance, for Chlorella pyrenoidosa, growth inhibition was observed in the exponential phase of the growth curve, whereas during the stationary phase, this trend was reversed, and growth exceeded that in control (Mao et al., 2018). Such biphasic responses indicate that algal populations are able to adapt to the presence of particulates, including MNP, and sustain high productivity (Sjollema et al., 2016; Long et al., 2017; Yokota et al., 2017; Zhang et al., 2017; Mao et al., 2018; Prata et al., 2018). What is more important for the risk assessment is that it is not always clear whether the observed effects can be attributed, at least partially, to changes in the experimental conditions related to the presence of particulate material in the test system, such as high turbidity, nutrient limitation, and poor light penetration (Besseling et al., 2014; He et al., 2017), which are not necessarily specific to the polymers as test materials.
When testing MNP effects on biota, it is surprising that the general effects of suspended solids in the exposure systems are largely ignored, although there is a consensus that suspended particles of a particular geochemistry and size range induce various responses in aquatic organisms (Gordon and Palmer, 2015). In algae, sediment particles, and other organic and inorganic solids can cause growth inhibition (Cahoon et al., 1999). Consequently, the concentration of suspended solids in effluents is recognized as an important parameter that should be under control in toxicity testing (Hatch and Burton, 1999; He et al., 2017). Levels of suspended solids that cause adverse effects vary depending on the algal species and other components in the test system (Bilotta and Brazier, 2008). With respect to setting thresholds beyond which impacts on biota would be negligible, the estimates vary from 20 to 100 mg/L (Bilotta and Brazier, 2008; Gordon and Palmer, 2015; Chapman et al., 2017). A threshold value of 100 mg/L suggested by U.S. EPA (2015), has been used in the comparative analysis of the fossil-based polymers and natural particles (Ogonowski et al., 2018). In the latter study, it was found that median toxic concentrations were very similar between natural particles (such as clay and cellulose) and MP; moreover, these concentrations were within environmentally acceptable levels for suspended solids (Gordon and Palmer, 2015).
A recent review and meta-analysis by Bucci et al. (2019) based on 66 studies evaluating microplastic effects in aquatic organisms suggested that the crucial parameters influencing the test outcome are particle concentration, their shape, and size. In particular, fibers were found to be more hazardous than beads and fragments; moreover, for smaller particles, the adverse effects were more likely to be detected (Bucci et al., 2019). This meta-analysis provided a useful synthesis of the experimental data; unfortunately, only six studies examining effects in algae were included in the data set. Other critical reviews for various effects of MP in biota (Foley et al., 2018) and the effect concentrations (Ogonowski et al., 2018) also indicate a high variability across the reports and a lack of comprehensive data sets for algae compared to invertebrates and fish (Bucci et al., 2019). As effect mechanisms are likely to differ between the producers and consumers, it is necessary to conduct a comparative analysis focusing on specific groups of biota.
Given the variability of the responses, it has been recommended to use meta-analysis to support the risk assessment of plastic litter and the development of the appropriate test systems (Bucci et al., 2019). Indeed, single trials with often contradicting reports, small sample sizes, variable exposure conditions, and a diversity of species used in the experiments are difficult to synthesize. Meta-analysis permits an evaluation of the effect sizes across different studies by increasing the statistical power and analyzing the variability between studies. In toxicology, the meta-analysis techniques allow generalizing tendencies, resolving uncertainties, and identifying promotors of the effects. Based on the outcome of the meta-analysis, new hypotheses, and experimental designs can be generated (Fagard et al., 1996) to improve mechanistic understanding of the hazard potential of the plastic litter.
To critically evaluate the current experimental evidence for adverse effects of MNP in algae, we reviewed available studies and conducted a meta-analysis. The aim was to determine the associations between the exposure to MNP and the risk of growth inhibition in unicellular freshwater and marine algae, with a particular focus on the responses observed below and above the threshold concentration of 100 mg/L adopted by US EPA. Based on the current literature suggesting inhibitory effects of MNP on algal growth, we put forward the following hypotheses: (H1) exposure to plastic particles causes growth inhibition, which is concentration-dependent, (H2) particle size, shape, and polymer material moderate to the variations in the effect size, (H3) the inhibitory effect decreases with increasing exposure duration; and (H4) freshwater and marine species respond similarly to the exposure. When testing these hypotheses, we focused on both nano- and microplastics, owing to their global relevance, public concerns, and ecologically plausible interactions with algae in both freshwater and marine ecosystems. Also, from the ecological perspective, there is NP-MP size continuum that organisms are exposed to in situ and, therefore, considering the entire spectrum in the meta-analysis is environmentally relevant.
Studies examining the response of algae to nano- and microplastic exposure were retrieved from Web of Knowledge and Google Scholar databases. We used broad-range search combining keywords: microplastic, nanoplastic, exposure, algae, and experiment, and targeting article title, keywords, abstract, and subject headings. When screening the retrieved studies, we paid attention to the experimental design, including information on the physicochemical properties of the test particles reported in the paper. In some cases, when the polymer material was specified but its density was not, we used a polymer-specific value obtained from Omnexus Plastics Database1.
The publication date was not restricted; however, the last search was conducted on January 14, 2020. Further studies were sought by manually searching reference lists of the selected articles. In addition, given the paucity of the published reports, we included one study deposited in the bioRxiv2 manuscript repository (Gorokhova et al., 2020) and one M.Sc. thesis (Rogstad, 2019); both studies met the selection criteria.
The completed search yielded 28 studies, which were further screened according to the following criteria: (i) effects of exposure were quantified in a controlled experiment, (ii) experimental results were reported with basic statistical measures, including sample size, mean, and variance for the endpoint measured, (iii) primary information on the polymer material used in the exposure experiments, test concentrations of the particle suspension, and particle size were presented within the paper or in its Supplementary Materials, and (iv) the endpoints used in the experiment were relevant for standard growth inhibition test with the growth measured either by cell count or fluorescence (OECD, 2011). We used growth inhibition because it is a standard endpoint in current toxicity testing with microalgae. This screening resulted in 20 studies that met the selection criteria and, hence, were included in the meta-analysis; see Figure 1, Table 1, and Supplementary Table S1 for an overview of the studies included in the final data set and those that failed to meet the selection criteria. In most cases, the grounds for not including a study in the final selection were related to a lack of appropriate growth measurements, insufficient reporting of primary results (e.g., missing variance metrics), and insufficient description of the experimental setup (Supplementary Table S1).
Figure 1. Workflow for data selection and grouping. To investigate effects at the low and high levels of MNP in the tests, the studies were assigned to three models: Low concentration model (<100 mg/L), High concentration model (≥100 mg/L), and Full range model (0.004–1,100 mg/L). The threshold of 100 mg/L was used following the recommendations for suspended solid concentrations in stormwater (U.S. EPA, 2015).
Table 1. Overview of studies testing micro- and nanoplastic effects on algal growth that were included in the meta-analysis.
From each study, we extracted the following data: (1) authors and year of publication, (2) test species, (3) observed response in the treatment (exposure to the test material in the culture medium) and control (no exposure to any suspended solids, culture medium only), (4) method used to measure a change in algal population size by cell counting or fluorescence-based techniques, (5) a set of exposure system characteristics (salinity and exposure duration), and (6) microplastic characteristics (polymer material, particle size, concentration, shape, and density). For the continuous responses representing a change in the algal population abundance, values for mean, variance (standard deviation, SD), and sample size (number of experimental units in treatment and control) were used. When possible, the mean and variances were extracted from tables and text of the publication; however, when these results were presented only as graphs, they were retrieved using ImageJ version 1.52a (Abramoff et al., 2004). If SD in control was set to zero, the SD of the treatment was used together with both control and treatment mean (Wiebe et al., 2006).
Studies included in this analysis were conducted to measure algal growth inhibition following—at least to a large extent—the OECD guidelines (OECD, 2011) (Table 1). Multiple experiments per study were used when they were conducted independently, e.g., with different polymer materials (e.g., Bergami et al., 2017; Rogstad, 2019; Gorokhova et al., 2020) or different algal species (e.g., Long et al., 2017). Given the focus of our analysis, this was considered appropriate as long as the exposure effect was measured in independent trials within a study.
The study entries were grouped in relation to the threshold for exposure concentration that was set to 100 mg/L, the level below which no adverse effects on aquatic biota would be expected; this value was based on the regulations for the total suspended solid concentration in stormwater and various effluents (U.S. EPA, 2015). Based on the particle concentration in the experimental system, we generated three datasets: (1) Low concentration model (<100 mg/L); here, no growth inhibition due to non-specific effects of suspended solids, such as shading or nutrient sorption, was expected to occur, (2) High concentration model (≥100 mg/L); under these conditions, the non-specific effects were likely and, thus, growth inhibition was expected. Therefore, we expected to observe a more pronounced inhibition in the High concentration model compared to the Low concentration model. In both datasets, when study design included multiple test concentrations and exposure durations, we aimed at a conservative estimate from the environmental perspective and used experimental settings that yielded the most considerable effect size for a given polymer material or a species tested. In addition, a dataset that included all studies was used as (3) Full range model (0.004–1,100 mg/L), which included entries with the combination of the experimental settings (MNP concentration, polymer material, particle size, etc.) yielding the highest effect size for a specific study. In this model, we expected to see a stronger evidence for the effect size being affected by MNP concentration and particle size because of the broader span of values for these parameters in the dataset.
The effect size (Cohen's d; Equation 1) was calculated using observations for treatment (i.e., exposed algae) and control (i.e., algae that were grown in pure culture medium):
where nT and nC are sample sizes in treatment and control, respectively; SDT and SDC are standard deviations in treatment and control, respectively; and df is degrees of freedom.
The calculated Cohen's d values were adjusted using a correction factor J (Equation 2):
This correction accounts for a small sample size (Nakagawa and Cuthill, 2007; Borenstein et al., 2009; Hedges and Olkin, 2014); the resulting estimate is referred to as Hedge's g (Equation 3).
If only standard error (SE) and sample size (n) were reported, we calculated SD for each group as:
The sampling variances corresponding to the observed outcome (Hedges' g) were calculated as:
The standardized unbiased effect size estimated as Hedges' g and the related sampling variance were used in the meta-analysis.
The meta-analysis was conducted with the R package metafor version 2.1.0 (Viechtbauer, 2010) using the R studio version 3.6.0 (R Core Team, 2019) and the computed Hedges' g and V values. Because study design and test species varied across the studies, a random-effects analysis was used to allow for heterogeneity of the true effect sizes among the studies (Borenstein et al., 2010). The impact of each entry was weighted based on sample size and illustrated by the size of the symbol in the figures. Pooled estimates of the outcomes were reported as a forest plot depicting the mean effect size and the associated 95% confidence intervals (95% CI). Prediction interval (95% PI) was calculated to estimate the interval that a new observation would fall into (IntHout et al., 2016) as opposed to the uncertainty estimated by the 95% CI of the effect size. The mean effect size, 95% CI and 95% PI were estimated for each model.
Exploratory random-effects meta-regression analysis was used to investigate the moderating role of exposure time, MNP concentration, particle size, polymer density, and temperature. In addition to the particle size, the ratio between the longest linear dimension of the algal cell (Supplementary Table S2) and the nominal size of the test particle (cell-to-particle size ratio) as a continuous predictor of the effect sizes was evaluated. The effect was considered significant when the regression slope was significantly non-zero. To estimate the percentage of variance accounted for by the moderator, an R2 index was calculated (López-López et al., 2014). While the R2 index has a range of 0–100%, it is possible for sampling error to yield a value of R2 that falls outside of this range. In that case, the value is truncated to either 0% (if the estimate falls below 0%) or to 100% (if it falls above 100%). Categorical parameters, such as polymer material (PS vs. PE vs. PVC), particle shape (spherical vs. non-spherical), particle size category (MP vs. NP), and origin of the test algae (freshwater vs. saltwater species), were also explored as a source of heterogeneity. Finally, we tested whether different approaches for growth measurement (cell count by light microscopy or electronic means vs. fluorescence-based methods) contributed to the heterogeneity because these approaches have different precision and sensitivity; they also address different aspects of algal growth (Butterwick et al., 1982). The effect of a categorical predictor was considered significant when the 95% CI range did not include zero.
Funnel plots were used to assess publication bias by plotting the effect size vs. the standard error (Sterne and Egger, 2001; Peters et al., 2008). Using these plots, we tested whether there is a relation between the effect size of a study and its precision. First, the Egger's test was applied to evaluate the asymmetry of the plot (Egger et al., 1997), which may result from excluding non-significant studies or presence of studies with small sample sizes. Further, we applied the trim-and-fill method, which is an iterative rank-based method, to estimate the number of missing studies in the dataset and their effect on the meta-analysis outcome (Duval and Tweedie, 2000). Lastly, a sensitivity analysis was applied to assess the importance of bias.
Between-study heterogeneity in study-specific effects sizes was evaluated with the Q statistic (Higgins et al., 2002). The Q-test is based on the chi-square distribution and provides a measure of the sum of the squared differences between the observed and the expected results in each study under the assumption that each study estimates the same treatment effect (i.e., growth inhibition). When all studies share the same effect size, the expected Q value is equal to the degrees of freedom (the number of studies minus 1), whereas a higher Q value indicates heterogeneity. The I2 statistics was calculated as a measure to assess the percentage of variation due to heterogeneity between the studies rather than chance alone (Higgins and Thompson, 2002).
The set of studies used for meta-analysis included 20 publications, with 67 data entries (Figure 1) allocated to three datasets: (1) Low concentration model (<100 mg/L; 0.004–99 mg/L; 27 entries, 19 studies), (2) High concentration model (≥100 mg/L; 100–1,100 mg/L; 12 entries; 10 studies), and (3) Full range model (0.004–1,100 mg/L; 28 entries; 20 studies). The majority of entries (65%) presented tests with saltwater algae, among which Dinophyceae and Prymnesiophyceae were the most common (13 and 9%, respectively), whereas Chlorophyceae were the most frequent species (36%) used in the studies with freshwater algae; only four out of 16 species were used in more than a single study (Supplementary Figure S8).
Across the selected studies, the particle size and MNP concentration ranged from 0.04 to 3,000 μm and 0.004 to 1,100 mg/L, respectively (Figure 2). The mean and median values for the particle size were 213 and 2 μm, respectively. Only six studies used particles in the nano-range, whereas 70% of studies used particles larger than 0.1 μm. The mean and median values for MNP concentration used in the exposure experiments were 182 and 50 mg/L, respectively.
Figure 2. Variability of the main parameters in the global dataset used in the meta-analysis: (A) MNP concentration (mg/L), (B) particle size (μm), (C) polymer density (g/cm3), and (D) particle shape. The mean and median values are shown by solid and dotted lines, respectively. The effects on algal growth reported by the authors in each study are indicated by the color coding; red, negative effect; black, no effect; and green, positive effect. Note logarithmic scale on the x axes for (A,B).
Many studies used polystyrene (PS; 45%) followed by polyethylene (PE; 25%) and polyvinyl chloride (PVC; 20%); others used polyethylene terephthalate (PET), polyester (PES) and polyamide (PA). The polymer densities ranged between 0.99 and 1.40 g/cm3. In most cases, the plastics were virgin, and only two studies used weathered PET and PVC; moreover, one study used functionalized PS. With regard to the particle shape, 85% of studies used spherical particles. Due to the limited number of studies that used fibers and irregular fragments and the similarity of the effects observed in these studies, these entries were grouped as non-spherical particles for moderation analysis.
To assess changes in algal growth, the majority of the studies used cell count (75%; either microscopy-based or using electric counters), whereas fluorometry was less common (25%). Moreover, the fluorometry-based methods varied among the studies and included flow cytometry, in vivo fluorometry of Chlorophyll a, and fluorescence microscopy with image analysis.
In the Low concentration model, the effect size was overlapping with zero in 66% cases, whereas significant growth inhibition and stimulation were observed in 26 and 7% cases, respectively (Figure 3, upper panel). The mean effect size of the MNP exposure was −0.22, indicating decreased algal growth compared to the particle-free controls; however, the 95% CI for the mean effect size overlapped with zero [−0.90–0.45]. Therefore, mean growth in the exposed algae was not significantly different from that in controls. The 95% PI ranged from −3.20 to 2.75, indicating that both positive and negative effect sizes are nearly equally plausible for a future test employing similar design.
Figure 3. Forest plot of the random-effect models for effect sizes estimated as Hedges' g and the associated 95% confidence interval (95% CI) in the Low concentration model (upper panel; 20 entries) and the High concentration model (lower panel; 11 entries). The negative and positive values for Hedges' g correspond to growth inhibition and stimulation, respectively. In each model, the overall effect (i.e., pooled estimate) is depicted as a diamond. The prediction interval shown by lines extending from the overall effect diamond captures the expected true effect for a future study. Symbol size is proportional to the study weight. Multiple entries for some studies correspond to independent trials for different polymers and/or different species. In each model, studies that employed cell count and fluorescence methods for measuring growth are shown separately.
The heterogeneity was high and significant (Q = 100.20; I2 = 75.8%; p < 0.001), indicating divergent responses among the studies that cannot be attributed to chance alone. Results of the exploratory meta-regression showed that polymer density was a significant positive predictor (p < 0.03; Figure 4A), i.e., with increasing density, the growth inhibition was less likely. The moderator explained 37% of the heterogeneity in the model. Particle size showed a similar trend, albeit explaining only 14% (p > 0.07; Supplementary Figure S2A), implying that growth inhibition was more likely when algae were exposed to smaller particles. By contrast, neither MNP concentration nor exposure time nor cell-to-particle size ratio were significant as predictors (Supplementary Figures S1A, S3A, S4A). Among the categorical predictors, a significant influence on the effect size was only found for the particle shape (Figure 5A): non-spherical particles (fibers and fragments) were more likely to induce growth stimulation compared to the spherical particles (Figure 5A). Of the three polymer materials (PS, PE, and PVC) that were sufficiently represented to allow for the comparison, none were found to cause significant growth inhibition (Supplementary Figure S5A). NP had slight adverse effects on growth compared to MP, albeit, not significant (Supplementary Figure S6A). The test outcome was not affected by the freshwater or saltwater origin of the test species (Supplementary Figure S7A).
Figure 4. Meta-regressions for the effect size as a function of polymer density (g/cm3) across the models: (A) Low concentration model (<100 mg/L), (B) High concentration model (≥100 mg/L), and (C) Full range model (0.004–1,100 mg/L). Circle size indicates the study weight in the effect size calculation; note that the scale for the weights varies among the models. Solid line represents model prediction and dashed line is the corresponding 95% CI.
Figure 5. Subgroup analysis for the particle shape as a predictor for the effect size across the models: (A) Low concentration model (<100 mg/L) and (B) Full range model (0.004–1,100 mg/L). Crosses represent the median, the boxes are the 95% CI of the median, and whiskers show the overall 95% CI. The black dots indicate outliers. The horizontal dashed line indicates no effect. N values on the x axis indicate number of entries in each subgroup. The asterisk indicates statistical significance at α = 0.05.
In the High concentration model, the effect size was overlapping with zero in 41% cases, whereas significant growth inhibition and stimulation were observed in 41 and 17%, respectively (Figure 3, lower panel). The mean effect size was −1.11, with the 95% CI overlapping with zero [−3.17, 0.95]. The 95% PI was also wide, ranging from −8.01 to 5.79; thus, both positive and negative effects will be observed in future tests employing similar design.
The heterogeneity was high and significant (Q = 68.73; I2 = 89.9%; p < 0.001). However, none of the continuous predictors (i.e., concentration, exposure duration, polymer density, particle size, and cell-to-particle size ratio; Supplementary Figures S1B, S2B, S3B, S4B; Figure 4B, respecti-vely) were significant (p > 0.07 in all cases). MNP concentration explained 29% of the heterogeneity in the effect size, with a higher probability of growth inhibition at higher MNP levels; the effect was not, however, significant (p > 0.07; Supplementary Figure S1B). Also, neither polymer material nor particle size category nor species origin were significant as predictors (Supplementary Figures S5B–S7B). The effect of particle shape could not have been evaluated due to the lack of observations for the non-spherical particles.
The Full range model was based on 28 entries from all 20 studies selected for the meta-analysis, 42% of which had the effect size overlapping with zero, whereas significant growth inhibition and stimulation were observed in 32 and 17% cases, respectively. The mean effect size was negative (−1.34), with the 95% CI [−2.88, 0.20]. The prediction interval was very broad, ranging from −8.94 to 6.26, indicating that both negative and positive effects will be observed in the future tests even if only maximal effect sizes across the treatments in each study are considered.
The heterogeneity was high and significant (Q =156.88 I2 = 94.1%, p < 0.001), reflecting the variability in the data. None of the continuous predictors (MNP concentration, exposure duration, polymer density, polymer size, and cell-to-particle size ratio) were significant in explaining the heterogeneity (p > 0.09 in all cases; Supplementary Figures S1–S3C, S4C; Figure 4C). Among these predictors, the cell-to-particle size ratio contributed most to the observed heterogeneity (17%, p > 0.09; Supplementary Figure S4C), with a higher probability of growth inhibition at higher cell-to-particle size ratio. Particle shape was a significant predictor; we found that exposure to fibers and fragments was associated with growth stimulation (Figure 5B). Neither polymer type nor particle size category nor species origin contributed significantly to the observed heterogeneity (Supplementary Figures S5C–S7C).
This dataset was also used to assess the effect of the growth measurement method, i.e., cell count (22 entries) vs. fluorescence (6 entries) on the effect size (Figure 6). The mean effect size was not significantly different from zero regardless of the method used, with the mean effect size for the cell count model and the fluorescence model being −1.26 [−2.82, 0.29] and −1.68 [−6.62, 3.27], respectively. The prediction interval for the true treatment effect was broader in the fluorescence model (−14.22–10.86) compared to that based on cell counts (−8.05–5.53), which at least partly was related to the fewer entries in the former.
Figure 6. Forest plot of the random-effect model for effect sizes estimated as Hedges' g and the associated 95% confidence interval (95% CI) in Forest plot for the Full range model (28 entries). Symbol size for individual entries is proportional to the study weight. The overall effect (pooled estimate) on growth according to the random-effect model is depicted as a diamond. The prediction interval (PI) is shown with lines extending from the diamond. Multiple entries for some studies correspond to different polymers tested within a study or several species used in the tests. Studies that employed cell count and fluorescence methods for measuring growth are shown separately.
Funnel plots revealed some low-precision studies (Figure 7). Moreover, low precision studies that reported significant inhibitory effects were present, whereas those reporting stimulatory effects were not. All studies reporting stimulatory effects of the exposure had high precision and most of them were significant, whereas the negative effect studies had greater effect size but lower precision. In all three models (Figure 7), a significant asymmetry was found using Egger's test, with z-scores of −4.79 (p < 0.0001), −3.09 (p < 0.002), and −5.17 (p < 0.0001) for the Low Concentration, High concentration and the Full range models, respectively (Supplementary Table S3). Thus, the negative effect sizes appeared to be reported by studies with smaller sample sizes (Figure 7). This asymmetry indicates that a bias toward the publication of large negative effects may stem from the sample size of the study.
Figure 7. Funnel plots estimating probability of a publication bias for the studies used in the meta-analysis for (A) Low concentration model, (B) High concentration model, and (C) Full range model. In the panels on the left side, the standard error is plotted against the effect size (Hedges' g), and in the panels on the right side, the sample size is plotted against the effect size. Each dot represents an entry in the model. The hollow symbols in the funnel plots are the studies filled by the trim-and-fill procedure, which would balance out the asymmetry of the funnel. The white area in the funnel plots depicts the significance with 0.05 < p ≤ 1.00 and the gray area is for 0.00 < p ≤ 0.05.
With high between-sample heterogeneity (as in all three models), the use of funnel plot for publication bias detection may yield false-positive results, i.e., detect publication bias when none is present. To address this concern, we first inspected the funnel plot distribution. Large moderating effects, i.e., effects of the moderators that were not tested, should result in a bimodal distribution, which did not occur (Figure 7). Therefore, the existence of the publication bias and small study effect was accepted, albeit with caution.
Second, the trim-and-fill method was applied to estimate the number and impact of missing studies in the dataset (Figure 7). Five studies were identified to be missing in the Low concentration model and seven in the Full range model on the right side of the funnel, and none in the High concentration model. The imputation changed the model results to the mean effect size and 95% CI of 0.10 [−2.09, 2.29] and PI [−10.09, 10.9] for the Low concentration model and −0.41[−1.74, 2.55] with PI [−11.71,12.52] for the Full range model. In both cases, the corrected values were similar to the original output; thus, no significant effect of the exposure across the studies was found even when the results were corrected for the publication bias.
Using meta-analysis, we found that exposure to micro- or nanoplastic was not associated with significant growth inhibition in unicellular algae. In the 20 studies based on standard growth inhibition assay, all possible outcomes were reported, ranging from inhibition (up to 57%) to stimulation (up to 56%) of growth rate. Neither Low concentration model nor High concentration model nor Full range model produced a significant mean effect size; moreover, prediction intervals indicate that tests following this type of experimental design are likely to yield both inhibitory and stimulatory responses. Thus, our meta-analysis lends limited support to the hypothesized growth inhibition of algae by exposure to micro- and nanoplastic (hypothesis H1). However, caution should be taken when interpreting these results owing to substantial heterogeneity within the models and a relatively low number of studies.
Notably, we found no clear indication that the exposure effect is related to MNP concentration (H1). The exposure to MNP concentrations of <100 mg/L resulted in mean inhibition by 0.22 standard deviations (Low concentration model), whereas at the concentration ≥100 mg/L (High concentration model), the average inhibition was more pronounced (1.11 standard deviations). Although the High concentration model suggested more adverse effects compared to the Low concentration model, which was in line with our expectations, neither mean effect sizes were significantly different from zero (Figure 3; Supplementary Figures S1A,C). The only weak indication of a possible concentration-dependent response was found for the High concentration model, where the negative trend indicated that at higher MNP concentrations, growth inhibition might be more likely (p > 0.07; Supplementary Figure S1B). However, the High concentration model had the lowest number of entries; therefore, to investigate this trend and establish effect concentrations, more studies would be needed.
The effect concentrations reported in the experimental studies are very variable and often biphasic. Dose-response relationships using growth as the endpoint (Besseling et al., 2014; Sjollema et al., 2016; Gambardella et al., 2018; Wu et al., 2019; Zhao et al., 2019) indicate that the lowest concentrations which induced inhibition (LOEC) varied 0.001–50 mg/L, i.e., within the range of our Low concentration model. Also, similar concentrations of 1-μm PVC (≤50 mg/L) caused significant growth inhibition (Zhang et al., 2017). However, in some cases, PVC induced less inhibition at higher concentrations (≥100 mg/L) compared to 10 mg/L (Fu et al., 2019). Moreover, for unplasticized PVC, Guo and co-workers showed growth stimulation at 50 g/L compared to significant inhibition (37%) at 0.05 mg/L (Guo et al., 2020). The main reason for these counter-intuitive observations could be a reduced exposure during the test because of the uncontrolled particle aggregation and settling, which are enhanced at higher concentrations. These methodological shortcomings in the experimental design and uncertainties with regard to the stability of the exposure would preclude meaningful evaluation of the concentration effects not only across the studies but also within a study unless sufficient measures were taken to prevent particle sedimentation.
An indication of the particle size influence on algal growth was found in the Low concentration model, with growth inhibition being more likely in trials with smaller particles (p > 0.07; Supplementary Figure S2A). In the High concentration and Full range models, the regression slopes for the particle size effects were also positive, albeit non-significant and with low explanatory power (Supplementary Figures S2B,C), which might also be related to the less pronounced effect due to particle aggregation at higher MNP concentrations. The subgroup analysis contrasting MP and NP effects corroborated the meta-regression outcome as growth inhibition in algae exposed to NP appeared to be more pronounced compared to that in MP exposures, albeit neither effect size was significant (Supplementary Figure S6). In algae and filter-feeders, particle size has been considered a crucial driver of the adverse effects (Cole et al., 2013; Lee et al., 2013; Besseling et al., 2014; Davarpanah and Guilhermino, 2015; Jeong et al., 2016). However, even in a controlled experimental system with appropriate mixing, particle size is affected by aggregation during the exposure (Gorokhova et al., 2020), which makes it a challenging parameter to consider in the risk assessment. In algae, particles of 0.05–1 μm were reported to cause growth inhibition (Sjollema et al., 2016; Zhang et al., 2017; Gambardella et al., 2018), whereas those of ~200 μm had a stimulatory effect (Chae et al., 2019). Moreover, the cell size of the test algae has also been suggested to contribute, with adverse effects on MNP observed at higher cell-to-particle size ratio (Chae et al., 2019). The mechanisms of these effects have been linked to particle adsorption to algal cell walls leading to compromised permeability, inhibition of growth, and photosynthetic activity (Bhattacharya et al., 2010; Mao et al., 2018). Although we found no significant influence of the cell-to-particle ratio on the effect size within the cell size range used in the dataset (1–78 μm; Supplementary Table S2), the observed negative trend was in agreement with the suggested effect direction and the mechanisms (Chae et al., 2019), and in the Full range model, this moderator explained 17% of the model heterogeneity.
Of the three polymers used in the subgroup analysis, none was found to induce a significant growth inhibition; thus, no support to the hypothesized effect of polymer material as a significant predictor of the effect size was obtained (H2). PS was the most commonly used polymer (45% of studies) followed by PE (25%) and PVC (20%). In experimental studies, the high toxicity of styrene for algae and invertebrates has been related to the adverse effects of PS (Sussarellu et al., 2016; Mueller et al., 2020). Moreover, PS with anionic and cationic groups can undergo strong aggregation, which was suggested to mediate embryotoxicity in sea urchin embryos (Della Torre et al., 2014). Similar mechanisms of the adverse effects in alga Dunaliella tertiolecta were suggested by the same team (Bergami et al., 2017). However, the same polymer (PS-COOH) at comparable concentrations (40–50 mg/L) had differing effects on algal growth, ranging from no-effect (Bergami et al., 2017) to strong inhibition (Nolte et al., 2017), which suggests that other exposure characteristics, such as media and species, may alter the outcome. In particular, higher aggregation potential for positively charged polymer particles was suggested to affect biological availability and, thus, exerted effects, which is in line with the higher toxicity observed for cationic amino-modified particles (Bhattacharya et al., 2010).
Across the studies, PE and PVC were found to have both inhibitory and stimulatory effects resulting in the non-significant mean effects. These polymers have relatively low (≤1 g/cm3) and high (~1.4 g/cm3) density, respectively, which makes them more likely to either float on the surface (low-density PE) or sink to the bottom (PVC) during the experiment. Therefore, static tests are not likely to provide a controlled exposure and, hence, comparable results for polymers with negative or positive buoyancy (Karami, 2017), emphasizing the need for methodological developments and search for adequate exposure systems.
Support for the hypothesized effect of the polymer material (H2) was provided by the meta-regression showing that polymer density was a significant predictor of the effect size in the Low-concentration model but not in the other two models encompassing higher exposure concentrations. At MNP concentrations <100 mg/L, low-density polymers are more likely to inhibit algal growth compared to high-density polymers, which is most likely due to the lower sedimentation of the former. At higher MNP concentrations, the enhanced contact between the polymer particles could facilitate aggregation and sedimentation, thus masking the effect of the polymer density.
Non-spherical particles, such as fibers and irregular fragments, were found to have stimulating effects on algal growth, unlike spherical particles that tended to exert inhibition. However, these findings must be interpreted with caution because studies using non-spherical particles are very scarce, and in our meta-analysis, all entries for such particles originated from only three studies (Yokota et al., 2017; Rogstad, 2019; Gorokhova et al., 2020). Although fragments and fibers are the most commonly observed MP in the field (Hidalgo-Ruz et al., 2012; Eriksen et al., 2014; Avio et al., 2015), spherical beads are used in the vast majority of the experiments, which has been broadly criticized (Wagner et al., 2014; Ogonowski et al., 2018). Given the observed differences in the effects caused by spherical and non-spherical particles, studies on MNP shaped as fibers and fragments are particularly needed, if we are to obtain environmentally relevant hazard assessment. More testing is also needed to evaluate weathering effects on the hazard potential of MNP because both stronger inhibition of growth (Fu et al., 2019) and no-effect outcomes (Gorokhova et al., 2020) have been reported for weathered particles compared to virgin ones. Plastic weathering changes the particle shape and size, surface area, and near-surface functional groups leading to the increasing frequency of hydroxyl groups (Liu et al., 2020). Unfortunately, the low number of studies that employed weathered plastics precluded evaluation of this factor as a predictor of the effect size for growth inhibition.
The hypothesized positive effect of the prolonged exposure on the effect size (i.e., lower inhibition at longer exposure duration; hypothesis H3) was not statistically significant. In the Full range model, however, 92% less inhibition was observed when exposure time increased from 72 to 168 h (p > 0.13). For such time-related effects, there are several possible explanations, related to algae adaption to the experimental conditions and changes in the exposure levels as well as culture conditions with time. Under prolonged incubation, different trade-offs and adaptations can occur in the exposure systems with algae (Andriukonis and Gorokhova, 2017) in response to deficiencies in light and nutrient regimes due to increased turbidity and concomitant nutrient depletion. If mixing is not adequate, particle aggregation and sedimentation will decrease the exposure and, hence, the response. Indeed, several studies reported the inhibition effect to be more severe in shorter incubations (e.g., ≤ 4 vs. >5 d; Fu et al., 2019; Zhao et al., 2019). Linking this pattern to the growth curve, Mao et al. (2018) explained growth inhibition during the exponential phase with compensatory growth as a sign of adaptation in the populations approaching the stationary phase. Moreover, changes in cell morphology and lipid accumulation can be indicative of such adaptations. For example, Su et al. (2019) observed an increase of cell density over a 10-d period, with a concomitant reduction in the cell size suggestive of nitrogen limitation (Kilham et al., 1997). Also, no growth inhibition has been reported after a 4-d exposure but a significant inhibition and lipid accumulation after 9-d, which could be explained by nutrient depletion due to algal growth but also sorption to the polymers (Guo et al., 2020). Therefore, time-related changes in the growth conditions may mask true response to the exposure, and the OECD-recommended duration of 72–96 h for the algal growth inhibition test design must be followed.
The effect sizes were not significantly different between the tests with fresh- and saltwater algae, thus supporting the hypothesized similarity (H4); however, freshwater algae had more negative effects in all three models (Supplementary Figure S7). The saltwater algae were used in 65% of the studies, which means that we have less information for conducting MNP hazard assessment in freshwaters compared to marine environments, where the plastic pollution has been studied more actively (Barboza and Gimenez, 2015; Eerkes-Medrano et al., 2015). Moreover, the taxonomic diversity of the used test species is relatively low (16 species), with the majority of species belonging to Dinophyceae, Prymnesiophyceae and Chlorophyceae. Yet, the similarity of the observed effects suggests that the test results are transferable between the freshwater and marine algae; thus, all available information can be used for risk assessment of plastic debris in lakes and rivers until more data for freshwater microalgae become available.
Given that plastic litter has become a hot topic in the current environmental research fuelled by keen public interest, discovering indications of the publication bias was not particularly surprising. The magnitude of the bias was, however, not sufficiently large to invalidate the meta-analysis outcome. Nevertheless, the publication bias in testing MNP effects indicates that a study reporting inhibitory effects is more likely to be published, even though the results would be less reliable, and the sample size is small. There are several potential causes of this bias, including the fact that we used entries representing treatments with the largest effect size when compiling the datasets. However, all studies were treated equally, and small effect studies were well-represented in all three models. Thus, the bias appears to be related to the selection of an effect size associated with statistical significance for reporting. It is also a possibility that variables that have not been accounted for as moderators, particularly methodological parameters, such as elements of test design, physicochemical properties of the particles, or different levels of measurement error across the studies, might explain some of the observed funnel plot asymmetries. To alleviate some of these concerns, improve the comparability of the test results and, thus, their suitability for meta-analysis, it is critical to standardize the test protocols and report all the details of the experimental design as well as any outcomes of the testing, both positive and negative, regardless of their significance and sample size.
Since adverse effects can occur in turbid environments with a variety of particulates, both natural, such as sediment, and anthropogenic, such as microplastic, it is relevant to compare responses between the microplastic and other suspended solids present at ecologically relevant concentrations. Our findings, including model heterogeneity and lack of significant effects rated to the variation of the nominal particle concentrations, strongly suggest that analysis of the particle size distribution in the test systems should be included in the experimental design to control for the aggregation and sedimentation as potential drivers of algal growth. Needless to say, that these processes occur in mixtures of any suspended solids, both natural and anthropogenic.
We hope that our study would stimulate a debate on the methodology of microplastic hazard assessment and possibly influence further research efforts toward this goal. Following the critical analysis of the recent publications on the subject (Table 2), we propose practical recommendations for researchers for conducting transparent and methodologically sound test protocols and reporting routines. This should help to consolidate the search for convergent assays informing the risk assessment of plastic litter in aquatic ecosystems. Current standard toxicity testing is mostly developed for chemicals, not particulates with complex behavior resulting in particle aggregation and physical interactions with test organisms (Ogonowski et al., 2018; Gerdes et al., 2019). As a standard ecotoxicological endpoint, algal growth alone might be insufficient to provide insights into the ecological impacts of MP (Gambardella et al., 2019). Therefore, more sensitive endpoints, such photosynthetic efficiency (e.g., Wu et al., 2019), electron transport (e.g., Mao et al., 2018), and biochemical changes (e.g., Bhattacharya et al., 2010), together with reliable test protocols, are needed to evaluate how microalgae might be affected by polymer particles. At present, it was not possible to include these subcellular responses in the meta-analysis, because of the low number of such studies and considerable variation in the methods employed. One should also keep in mind that subcellular-level responses in these tests must be linked to those at the higher levels of biological organization (Martyniuk, 2018) if we are to protect wild populations exposed to environmental contaminants, including plastic litter.
Table 2. Practical recommendations for standardization of algal bioassays with micro- and nanoplastic and reporting routines based on the outcome of the meta-analysis.
The raw data supporting the conclusions of this article will be made available by the authors, without undue reservation.
EG conceived the study idea. EG and SR designed the study and wrote the manuscript. SR collected data and performed the analysis. Both authors contributed to the data interpretation and writing.
This research was supported by the Joint Programming Initiative Healthy and Productive Seas and Oceans (JPI Oceans, WEATHER-MIC project, grant number 942-2015-1866)—EG; The Swedish Innovation Agency (VINNOVA) and the joint Baltic Sea research and development programme (BONUS, Blue Baltic) for MICROPOLL project [grant number 2017-00979]—EG; The Swedish Environmental Protection Agency (Naturvårdsverket) for MIxT project [grant number 802-0160-18]—EG, and Swedish Research Council for Environment, Agricultural Sciences and Spatial Planning (FORMAS) [grant number 2019-00388].
The authors declare that the research was conducted in the absence of any commercial or financial relationships that could be construed as a potential conflict of interest.
Eric McGivney (ACES, Stockholm University) is thanked for suggestions on the study design and data acquisition.
The Supplementary Material for this article can be found online at: https://www.frontiersin.org/articles/10.3389/fenvs.2020.00131/full#supplementary-material
Abramoff, M., Magalhães, P., and Ram, S. J. (2004). Image processing with ImageJ. Biophotonics Int. 11, 36–42.
Anderson, J. C., Park, B. J., and Palace, V. P. (2016). Microplastics in aquatic environments: implications for Canadian ecosystems. Environ. Pollut. 218, 269–280. doi: 10.1016/j.envpol.2016.06.074
Andrady, A.L. (2011). Microplastics in the marine environment. Mar. Pollut. Bull. 62, 1596–1605. doi: 10.1016/j.marpolbul.2011.05.030
Andriukonis, E., and Gorokhova, E. (2017). Kinetic N-15-isotope effects on algal growth. Sci. Rep. 7, 2533–2545. doi: 10.1038/srep44181
Au, S. Y., Bruce, T. F., Bridges, W. C., and Klaine, S. J. (2015). Responses of Hyalella azteca to acute and chronic microplastic exposures. Environ. Toxicol. Chem. 34, 2564–2572. doi: 10.1002/etc.3093
Avio, C.G., Gorbi, S., and Regoli, F. (2015). Experimental development of a new protocol for extraction and characterization of microplastics in fish tissues: first observations in commercial species from Adriatic Sea. Mar. Environ. Res. Part. Oceans Impl. Safe Mar. Environ. 111, 18–26. doi: 10.1016/j.marenvres.2015.06.014
Barboza, L. G. A., and Gimenez, B. C. G. (2015). Microplastics in the marine environment: current trends and future perspectives. Mar. Pollut. Bull. 97, 5–12. doi: 10.1016/j.marpolbul.2015.06.008
Bergami, E., Pugnalini, S., Vannuccini, M. L., Manfra, L., Faleri, C., Savorelli, F., et al. (2017). Long-term toxicity of surface-charged polystyrene nanoplastics to marine planktonic species Dunaliella tertiolecta and Artemia franciscana. Aquat. Toxicol. 189, 159–169. doi: 10.1016/j.aquatox.2017.06.008
Besseling, E., Wang, B., Lürling, M., and Koelmans, A. A. (2014). Nanoplastic affects growth of S. obliquus and reproduction of D. magna. Environ. Sci. Technol. 48, 12336–12343. doi: 10.1021/es503001d
Bhattacharya, P., Lin, S., Turner, J. P., and Ke, P. C. (2010). Physical adsorption of charged plastic nanoparticles affects algal photosynthesis. J. Phys. Chem. C 114, 16556–16561. doi: 10.1021/jp1054759
Bilotta, B. S., and Brazier, R. E. (2008). Understanding the influence of suspended solids on water quality and aquatic biota. Water Res. 42, 2849–2861. doi: 10.1016/j.watres.2008.03.018
Borenstein, M., Hedges, L. V., Higgins, J. P. T., and Rothstein, H. R. (2009). Introduction to Meta-Analysis. Hoboken: John Wiley and Sons.
Borenstein, M., Hedges, L. V., Higgins, J. P. T., and Rothstein, H. R. (2010). A basic introduction to fixed-effect and random-effects models for meta-analysis. Res. Synth. Methods 1, 97–111. doi: 10.1002/jrsm.12
Bucci, K., Tulio, M., and Rochman, C. M. (2019). What is known and unknown about the effects of plastic pollution: a meta-analysis and systematic review. Ecol. Appl. 30:e02044. doi: 10.1002/eap.2044
Butterwick, C., Heaney, S. I., and Talling, J. F. (1982). A comparison of eight methods for estimating the biomass and growth of planktonic algae. Br. Phycol. J. 17, 69–79. doi: 10.1080/00071618200650091
Cahoon, L. B., Nearhoof, J. E., and Tilton, C. L. (1999). Sediment grain size effect on benthic microalgal biomass in shallow aquatic ecosystems. Estuaries 22, 735–741. doi: 10.2307/1353106
Canniff, P. M., and Hoang, T. C. (2018). Microplastic ingestion by Daphnia magna and its enhancement on algal growth. Sci. Total Environ. 633, 500–507. doi: 10.1016/j.scitotenv.2018.03.176
Casado, M. P., Macken, A., and Byrne, H. J. (2013). Ecotoxicological assessment of silica and polystyrene nanoparticles assessed by a multitrophic test battery. Environ. Int. 51, 97–105. doi: 10.1016/j.envint.2012.11.001
Chae, Y., Kim, D., and An, Y.-J. (2019). Effects of micro-sized polyethylene spheres on the marine microalga Dunaliella salina: focusing on the algal cell to plastic particle size ratio. Aquat. Toxicol. 216:105296. doi: 10.1016/j.aquatox.2019.105296
Chae, Y., Kim, D., Kim, S. W., and An, Y.-J. (2018). Trophic transfer and individual impact of nano-sized polystyrene in a four-species freshwater food chain. Sci. Rep. 8, 1–11. doi: 10.1038/s41598-017-18849-y
Chapman, P. M., Hayward, A., and Faithful, J. (2017). Total suspended solids effects on freshwater lake Biota other than fish. Bull. Environ. Contam. Toxicol. 99, 423–427. doi: 10.1007/s00128-017-2154-y
Cole, M., Lindeque, P., Fileman, E., Halsband, C., Goodhead, R., Moger, J., et al. (2013). Microplastic ingestion by Zooplankton. Environ. Sci. Technol. 47, 6646–6655. doi: 10.1021/es400663f
Davarpanah, E., and Guilhermino, L. (2015). Single and combined effects of microplastics and copper on the population growth of the marine microalgae Tetraselmis chuii. Estuar. Coast. Shelf Sci. 167, 269–275. doi: 10.1016/j.ecss.2015.07.023
Della Torre, C., Bergami, E., Salvati, A., Faleri, C., Cirino, P., Dawson, K.A., et al. (2014). Accumulation and embryotoxicity of polystyrene nanoparticles at early stage of development of sea Urchin Embryos Paracentrotus lividus. Environ. Sci. Technol. 48, 12302–12311. doi: 10.1021/es502569w
Duval, S., and Tweedie, R. (2000). Trim and fill: a simple funnel-plot–based method of testing and adjusting for publication bias in meta-analysis. Biometrics 56, 455–463. doi: 10.1111/j.0006-341X.2000.00455.x
Eerkes-Medrano, D., Thompson, R. C., and Aldridge, D. C. (2015). Microplastics in freshwater systems: a review of the emerging threats, identification of knowledge gaps and prioritisation of research needs. Water Res. 75, 63–82. doi: 10.1016/j.watres.2015.02.012
Egger, M., Smith, G.D., Schneider, M., and Minder, C. (1997). Bias in meta-analysis detected by a simple, graphical test. BMJ 315, 629–634. doi: 10.1136/bmj.315.7109.629
Eriksen, M., Lebreton, L. C. M., Carson, H. S., Thiel, M., Moore, C. J., Borerro, J. C., et al. (2014). Plastic pollution in the World's Oceans: more than 5 trillion plastic pieces weighing over 250,000 tons afloat at sea. PLoS ONE 9:e111913. doi: 10.1371/journal.pone.0111913
Fagard, R. H., Staessen, J. A., and Thijs, L. (1996). Advantages and disadvantages of the meta-analysis approach. J. Hypertens. 14, S9–S13. doi: 10.1097/00004872-199609002-00004
Foley, C. J., Feiner, Z. S., Malinich, T. D., and Höök, T. O. (2018). A meta-analysis of the effects of exposure to microplastics on fish and aquatic invertebrates. Sci. Total Environ. 631, 550–559. doi: 10.1016/j.scitotenv.2018.03.046
Fu, D., Zhang, Q., Fan, Z., Qi, H., Wang, Z., and Peng, L. (2019). Aged microplastics polyvinyl chloride interact with copper and cause oxidative stress towards microalgae Chlorella vulgaris. Aquat. Toxicol. 216:105319. doi: 10.1016/j.aquatox.2019.105319
Gambardella, C., Morgana, S., Bramini, M., Rotini, A., Manfra, L., Migliore, L., et al. (2018). Ecotoxicological effects of polystyrene microbeads in a battery of marine organisms belonging to different trophic levels. Mar. Environ. Res. 141, 313–321. doi: 10.1016/j.marenvres.2018.09.023
Gambardella, C., Piazza, V., Albentosa, M., Bebianno, M. J., Cardoso, C., Faimali, M., et al. (2019). Microplastics do not affect standard ecotoxicological endpoints in marine unicellular organisms. Mar. Pollut. Bull. 143, 140–143. doi: 10.1016/j.marpolbul.2019.04.055
Garrido, S., Linares, M., Campillo, J. A., and Albentosa, M. (2019). Effect of microplastics on the toxicity of chlorpyrifos to the microalgae Isochrysis galbana, clone t-ISO. Ecotoxicol. Environ. Saf. 173, 103–109. doi: 10.1016/j.ecoenv.2019.02.020
Gerdes, Z., Hermann, M., Ogonowski, M., and Gorokhova, E. (2019). A novel method for assessing microplastic effect in suspension through mixing test and reference materials. Sci. Rep. 9, 1–9. doi: 10.1038/s41598-019-47160-1
Gordon, A. K., and Palmer, C. G. (2015). Defining an exposure–response relationship for suspended kaolin clay particulates and aquatic organisms: work toward defining a water quality guideline for suspended solids. Environ. Toxicol. Chem. 34, 907–912. doi: 10.1002/etc.2872
Gorokhova, E., Ek, K., and Reichelt, S. (2020). Algal growth at environmentally relevant concentrations of suspended solids: implications for microplastic hazard assessment. bioRxiv 2020.04.11.036889. doi: 10.1101/2020.04.11.036889
Guo, Y., Ma, W., Li, J., Liu, W., Qi, P., Ye, Y., et al. (2020). Effects of microplastics on growth, phenanthrene stress, and lipid accumulation in a diatom, Phaeodactylum tricornutum. Environ. Pollut. 257:113628. doi: 10.1016/j.envpol.2019.113628
Hatch, A. C., and Burton, G. A. (1999). Sediment toxicity and stormwater runoff in a contaminated receiving system: consideration of different bioassays in the laboratory and field. Chemosphere 39, 1001–1017. doi: 10.1016/S0045-6535(99)00023-5
He, Q., Qiu, Y., Liu, H., Sun, X., Kang, L., Cao, L., et al. (2017). New insights into the impacts of suspended particulate matter on phytoplankton density in a tributary of the Three Gorges Reservoir, China. Sci. Rep. 7:13518. doi: 10.1038/s41598-017-13235-0
Hidalgo-Ruz, V., Gutow, L., Thompson, R.C., and Thiel, M. (2012). Microplastics in the marine environment: a review of the methods used for identification and quantification. Environ. Sci. Technol. 46, 3060–3075. doi: 10.1021/es2031505
Higgins, J., Thompson, S., Deeks, J., and Altman, D. (2002). Statistical heterogeneity in systematic reviews of clinical trials: a critical appraisal of guidelines and practice. J. Health Serv. Res. Policy 7, 51–61. doi: 10.1258/1355819021927674
Higgins, J. P. T., and Thompson, S. G. (2002). Quantifying heterogeneity in a meta-analysis. Stat. Med. 21, 1539–1558. doi: 10.1002/sim.1186
IntHout, J., Ioannidis, J. P. A., Rovers, M. M., and Goeman, J. J. (2016). Plea for routinely presenting prediction intervals in meta-analysis. BMJ Open 6. doi: 10.1136/bmjopen-2015-010247
Jeong, C.-B., Won, E.-J., Kang, H.-M., Lee, M.-C., Hwang, D.-S., Hwang, U.-K., et al. (2016). Microplastic size-dependent toxicity, oxidative stress induction, and p-JNK and p-p38 activation in the Monogonont Rotifer (Brachionus koreanus). Environ. Sci. Technol. 50, 8849–8857. doi: 10.1021/acs.est.6b01441
Karami, A. (2017). Gaps in aquatic toxicological studies of microplastics. Chemosphere 184, 841–848. doi: 10.1016/j.chemosphere.2017.06.048
Kilham, S., Kreeger, D., Goulden, C., and Lynn, S. (1997). Effects of nutrient limitation on biochemical constituents of Ankistrodesmus falcatus. Freshw. Biol. 38, 591–596. doi: 10.1046/j.1365-2427.1997.00231.x
Lee, K.-W., Shim, W.J., Kwon, O.Y., and Kang, J.-H. (2013). Size-dependent effects of micro polystyrene particles in the marine copepod Tigriopus japonicus. Environ. Sci. Technol. 47, 11278–11283. doi: 10.1021/es401932b
Li, J., Liu, H., and Paul Chen, J. (2018). Microplastics in freshwater systems: a review on occurrence, environmental effects, and methods for microplastics detection. Water Res. 137, 362–374. doi: 10.1016/j.watres.2017.12.056
Liu, P., Zhan, X., Wu, X., Li, J., Wang, H., and Gao, S. (2020). Effect of weathering on environmental behavior of microplastics: properties, sorption and potential risks. Chemosphere 242:125193. doi: 10.1016/j.chemosphere.2019.125193
Long, M., Paul-Pont, I., Hégaret, H., Moriceau, B., Lambert, C., Huvet, et al. (2017). Interactions between polystyrene microplastics and marine phytoplankton lead to species-specific hetero-aggregation. Environ. Pollut. 228, 454–463. doi: 10.1016/j.envpol.2017.05.047
López-López, J. A., Marín-Martínez, F., Sánchez-Meca, J., Noortgate, W.V., and den Viechtbauer, W. (2014). Estimation of the predictive power of the model in mixed-effects meta-regression: a simulation study. Br. J. Math. Stat. Psychol. 67, 30–48. doi: 10.1111/bmsp.12002
Mao, Y., Ai, H., Chen, Y., Zhang, Z., Zeng, P., Kang, L., et al. (2018). Phytoplankton response to polystyrene microplastics: perspective from an entire growth period. Chemosphere 208, 59–68. doi: 10.1016/j.chemosphere.2018.05.170
Martyniuk, C. J. (2018). Are we closer to the vision? A proposed framework for incorporating omics into environmental assessments. Environ. Toxicol. Pharmacol. 59, 87–93. doi: 10.1016/j.etap.2018.03.005
Mueller, M.-T., Fueser, H., Trac, L. N., Mayer, P., Traunspurger, W., and Höss, S. (2020). Surface-related toxicity of polystyrene beads to nematodes and the role of food availability. Environ. Sci. Technol. 54, 1790–1798. doi: 10.1021/acs.est.9b06583
Nakagawa, S., and Cuthill, I. C. (2007). Effect size, confidence interval and statistical significance: a practical guide for biologists. Biol. Rev. Camb. Philos. Soc. 82, 591–605. doi: 10.1111/j.1469-185X.2007.00027.x
Nolte, T. M., Hartmann, N. B., Kleijn, J. M., Garnæs, J., van de Meent, D., Jan Hendriks, A., et al. (2017). The toxicity of plastic nanoparticles to green algae as influenced by surface modification, medium hardness and cellular adsorption. Aquat. Toxicol. 183, 11–20. doi: 10.1016/j.aquatox.2016.12.005
OECD (2011). Guidelines for the Testing of Chemicals, Guideline 201-Freshwater Alga and Cyanobacteria, Growth Inhibition Test. Organization for Economic Cooperation and DevelopmentTest No. 201: freshwater alga and cyanobacteria, growth inhibition test. Available online at: https://www.oecd-ilibrary.org/environment/test-no-201-alga-growth-inhibition-test_9789264069923-en (accessed July 1, 2020).
Ogonowski, M., Gerdes, Z., and Gorokhova, E. (2018). What we know and what we think we know about microplastic effects—a critical perspective. Curr. Opin. Environ. Sci. Health, Micro Nanoplast. 1, 41–46. doi: 10.1016/j.coesh.2017.09.001
Peters, J. L., Sutton, A. J., Jones, D. R., Abrams, K. R., and Rushton, L. (2008). Contour-enhanced meta-analysis funnel plots help distinguish publication bias from other causes of asymmetry. J. Clin. Epidemiol. 61, 991–996. doi: 10.1016/j.jclinepi.2007.11.010
Prata, J. C., Lavorante, B. R. B.O., Maria da Conceição, B. S. M., and Guilhermino, L. (2018). Influence of microplastics on the toxicity of the pharmaceuticals procainamide and doxycycline on the marine microalgae Tetraselmis chuii. Aquat. Toxicol. 197, 143–152. doi: 10.1016/j.aquatox.2018.02.015
R Core Team. (2019). R: A Language and Environment for Statistical Computing. Vienna: R Foundation for Statistical Computing.
Rodrigues, M. O., Abrantes, N., Gonçalves, F. J. M., Nogueira, H., Marques, J. C., and Gonçalves, A. M. A. (2018). Spatial and temporal distribution of microplastics in water and sediments of a freshwater system (Antuã River, Portugal). Sci. Total Environ. 633, 1549–1559. doi: 10.1016/j.scitotenv.2018.03.233
Rogers, K. L., Carreres-Calabuig, J. A., Gorokhova, E., and Posth, N. R. (2020). Micro-by-micro interactions: how microorganisms influence the fate of marine microplastics. Limnol. Oceanogr. Lett. 5, 18–36. doi: 10.1002/lol2.10136
Rogstad, D. T. (2019). Effekter av Tekstilfibre på den Marine Mikroalgen Isochrysis galbana (T-ISO). Trondheim.
Sjollema, S. B., Redondo-Hasselerharm, P., Leslie, H. A., Kraak, M. H. S., and Vethaak, A. D. (2016). Do plastic particles affect microalgal photosynthesis and growth? Aquat. Toxicol. 170, 259–261. doi: 10.1016/j.aquatox.2015.12.002
Sterne, J. A. C., and Egger, M. (2001). Funnel plots for detecting bias in meta-analysis: guidelines on choice of axis. J. Clin. Epidemiol. 54, 1046–1055. doi: 10.1016/S0895-4356(01)00377-8
Su, Y., Zhang, K., Zhou, Z., Wang, J., Yang, X., Tang, J., et al. (2019). Microplastic exposure represses the growth of endosymbiotic dinoflagellate Cladocopium goreaui in culture through affecting its apoptosis and metabolism. Chemosphere 244:125485. doi: 10.1016/j.chemosphere.2019.125485
Sussarellu, R., Suquet, M., Thomas, Y., Lambert, C., Fabioux, C., Pernet, M. E. J., et al. (2016). Oyster reproduction is affected by exposure to polystyrene microplastics. Proc. Natl. Acad. Sci. U.S.A. 113, 2430–2435. doi: 10.1073/pnas.1519019113
U.S. EPA (2015) U.S. EPA: United States Environmental Protection Agency (EPA) National Pollutant Discharge Elimination System (NPDES) Multisector Genereal Permit for Stormwater Discharges Associated With Industrial Activity (MSGP) [WWW Document]. US EPA. Available online at: https://www.epa.gov/npdes/final-2015-msgp-documents (accessed September 3 2020).
Viechtbauer, W. (2010). Conducting meta-analyses in {R} with the {metafor} package. J. Stat. Softw. 36, 1–48. doi: 10.18637/jss.v036.i03
Wagner, M., Scherer, C., Alvarez-Muñoz, D., Brennholt, N., Bourrain, X., Buchinger, S., et al. (2014). Microplastics in freshwater ecosystems: what we know and what we need to know. Environ. Sci. Eur. 26:12. doi: 10.1186/s12302-014-0012-7
Ward, J. E., and Kach, D. J. (2009). Marine aggregates facilitate ingestion of nanoparticles by suspension-feeding bivalves. Mar. Environ. Res. 68, 137–142. doi: 10.1016/j.marenvres.2009.05.002
Watts, A. J., Urbina, M. A., Goodhead, R., Moger, J., Lewis, C., and Galloway, T. S. (2016). Effect of microplastic on the gills of the shore crab Carcinus maenas. Environ. Sci. Technol. 50, 5364–5369. doi: 10.1021/acs.est.6b01187
Wiebe, N., Vandermeer, B., Platt, R. W., Klassen, T. P., Moher, D., and Barrowman, N. J. (2006). A systematic review identifies a lack of standardization in methods for handling missing variance data. J. Clin. Epidemiol. 59, 342–353. doi: 10.1016/j.jclinepi.2005.08.017
Wu, Y., Guo, P., Zhang, X., Zhang, Y., Xie, S., and Deng, J. (2019). Effect of microplastics exposure on the photosynthesis system of freshwater algae. J. Hazard. Mater. 374, 219–227. doi: 10.1016/j.jhazmat.2019.04.039
Yokota, K., Waterfield, H., Hastings, C., Davidson, E., Kwietniewski, E., and Wells, B. (2017). Finding the missing piece of the aquatic plastic pollution puzzle: interaction between primary producers and microplastics. Limnol. Oceanogr. Lett. 2, 91–104. doi: 10.1002/lol2.10040
Zhang, C., Chen, X., Wang, J., and Tan, L. (2017). Toxic effects of microplastic on marine microalgae Skeletonema costatum: interactions between microplastic and algae. Environ. Pollut. 220, 1282–1288. doi: 10.1016/j.envpol.2016.11.005
Keywords: metaanalysis, algal growth inhibition, hazard assessment, nanoplastics, microplastics, particulate matter, suspended solids
Citation: Reichelt S and Gorokhova E (2020) Micro- and Nanoplastic Exposure Effects in Microalgae: A Meta-Analysis of Standard Growth Inhibition Tests. Front. Environ. Sci. 8:131. doi: 10.3389/fenvs.2020.00131
Received: 12 May 2020; Accepted: 16 July 2020;
Published: 21 August 2020.
Edited by:
Juliana Assunção Ivar Do Sul, Leibniz Institute for Baltic Sea Research (LG), GermanyReviewed by:
Robson G. Santos, Federal University of Alagoas, BrazilCopyright © 2020 Reichelt and Gorokhova. This is an open-access article distributed under the terms of the Creative Commons Attribution License (CC BY). The use, distribution or reproduction in other forums is permitted, provided the original author(s) and the copyright owner(s) are credited and that the original publication in this journal is cited, in accordance with accepted academic practice. No use, distribution or reproduction is permitted which does not comply with these terms.
*Correspondence: Sophia Reichelt, U29waGlhLlJlaWNoZWx0QGFjZXMuc3Uuc2U=
Disclaimer: All claims expressed in this article are solely those of the authors and do not necessarily represent those of their affiliated organizations, or those of the publisher, the editors and the reviewers. Any product that may be evaluated in this article or claim that may be made by its manufacturer is not guaranteed or endorsed by the publisher.
Research integrity at Frontiers
Learn more about the work of our research integrity team to safeguard the quality of each article we publish.