- 1Department Animal Ecology I and BayCEER, University Bayreuth, Bayreuth, Germany
- 2Coastal Research and Management Group, Leibniz-Institute for Baltic Sea Research Warnemuende (IOW), Rostock, Germany
- 3RSS Remote Sensing Solutions GmbH, Munich, Germany
- 4Section 1.4 Remote Sensing and Geoinformatics, Helmholtz Centre Potsdam – GFZ German Research Centre for Geosciences, Potsdam, Germany
- 5Aquatic Systems Biology Unit, School of Life Sciences Weihenstephan, Technical University of Munich, Freising, Germany
Due to high spatiotemporal variability of aquatic systems, relationships between microplastic sources and sinks are highly complex and transportation pathways yet to be understood. Field data acquisitions are a necessary component for monitoring of microplastic contamination but alone cannot capture such complex relationships. Remote sensing is a key technology for environmental monitoring through which extrapolation of spatially limited field data to larger areas can be obtained. In this field study we tested whether microplastic distribution follows the same transport pattern as water constituents depictable from satellite images, namely chlorophyll-a, suspended particulate matter, and colored dissolved organic matter, and discuss their applicability as proxies. As rivers are a major source for marine microplastic contamination, we sampled three example river systems: the lower courses and river mouths of the Trave and Elbe estuary in Germany and the Po delta in Italy. For a full quantitative analysis of microplastics (>300 μm), ATR- and FPA-based μFT-IR spectroscopy and NIR imaging spectroscopy were utilized. Comparing water constituents with in-situ data using regression analysis, neither a relationship for the Elbe estuary nor for the Po delta was found. Only for the Trave river, a positive relationship between microplastics and water constituents was present. Differences in hydrodynamic conditions and spatiotemporal dynamics of water constituents and microplastic emissions among the river systems are possible explanations for the contrary results. Based on our results no conclusions on other river systems and likewise different seasons can be drawn. For remote sensing algorithms of water constituents to be used as microplastic proxy an adaption for each system as well as for different seasons would thus be necessary. The lower detection limit of 300 μm for microplastics could also have influenced relationships as microplastic abundance exponentially increases with decreasing size class. Further studies with improved sampling methods are necessary to assess our proposed method.
Introduction
Pollution of aquatic ecosystems with microplastic (MP) has recently gained particular attention due to its ability to accumulate in food webs and its potential threat to human health (Sharma and Chatterjee, 2017; Wang et al., 2019). To combat MP pollution in marine systems, MP (synthetic polymer particles <5 mm) litter has been included in several local and global directives for the protection of the marine environment (Directive of the European parliament, 2008; UN Resolution, 2020). Further, member states are required to adapt operational monitoring programs to assess sources, transport, and accumulation of MP within marine systems. As most plastic products are produced and consumed on land, rivers are a major transport pathway for MP litter to the oceans (van Wijnen et al., 2019). But to date, only limited data on MP abundances in estuaries and adjacent coastal areas exist (Rezania et al., 2018). Reported concentrations are highly variable, from less than one to over a thousand particles per cubic meter (Rezania et al., 2018) and are often accompanied by high standard deviations. Especially estuaries, as transition zone between fresh- and saltwater, are highly dynamic systems influenced by both local and global meteorological and hydrodynamical factors (e.g., local surface wind and waves, tidal influences, basin specific currents, regional precipitation). Consequently, in-situ sampling of MP within estuaries and adjacent coastal areas requires sufficient replication to cover the high spatiotemporal variability. Nevertheless, current field data collection of MP samples from estuaries and adjacent coastal areas are associated with high-cost ship expeditions. Further, the use of state-of-the-art sample processing and analytical methodologies for MP identification in environmental samples remains very time consuming (Enders et al., 2020). Thus, existing data of MP abundance at the water surface exhibits high uncertainties and are both spatially and temporally limited.
Remote sensing offers a promising monitoring tool to obtain data on large spatial and temporal scales, and thus could provide valuable data for improved microplastic monitoring approaches. One drawback is that the direct detection of MP is not possible due to its size and concentrations within the water surface layer which are too low to sufficiently influence the water surface reflectance signal. An indirect approach to investigate plastic litter transport was first conducted by Pichel et al. (2007) studying the sub-tropical convergence zone (STCZ) within the North Pacific. The STCZ accumulates floating materials due to its convergent current patterns, including plastic litter (Lebreton et al., 2012). Pichel et al. (2007) used chlorophyll-a (Chl-A) concentration as well as sea surface temperature (SST) derived from satellite images to identify the location of the STCZ. With this approach they were able to extrapolate single in-situ measurements of Chl-A and SST to a larger area. Moreover, a spatial correlation could be detected for Chl-A and SST with macroplastic litter (Pichel et al., 2007) but MP litter was not investigated. Additionally, a further spatial relationship of Chl-A and MP seems to be possible as it was shown that several species of algae attach to floating polymer particles (Masó et al., 2003) possibly due to the formation of extracellular polymeric substances enhancing aggregate formation (Xiao and Zheng, 2016). Besides Chl-A, further water constituents that can be derived from satellite images are suspended particulate matter (SPM) and colored dissolved organic matter (CDOM). As SPM comprises the same size range as MP and exhibits the same diversity of characteristics, i.e., consisting of a heterogenous mixture of particles of various sizes, densities and shapes, a spatial relationship due to similar transport pathways seems reasonable. A spatial correlation between CDOM (consisting of both decaying material of terrestrial origin and/or in-situ biological activity in the respective area) and MPs is further assumed likely as terrestrial inputs are thought to dominate the CDOM source in coastal areas (Stedmon and Nelson, 2014; Harvey et al., 2015) similar to MP litter inputs. Assuming that algae (Chl-A), SPM, CDOM and MPs are passive drifters which are mixed and transported by the same physical mechanisms (i.e., wind, waves, and currents) we tested the hypotheses that with (i) increasing Chl-A, (ii) SPM, and (iii) CDOM concentration, the concentration of MP increases. Moreover, an alternative indirect monitoring approach for floating MP through mapping proxies (the water constituents) measurable with remote sensing methods is proposed.
Materials and Methods
Study Area
Sampling was conducted at three different terminal river systems (Trave, Elbe, and Po) entering different seas (North Sea, Baltic Sea, Northern Adriatic Sea), which were chosen as to cover a broad range of expected MP abundances (Figure 1).
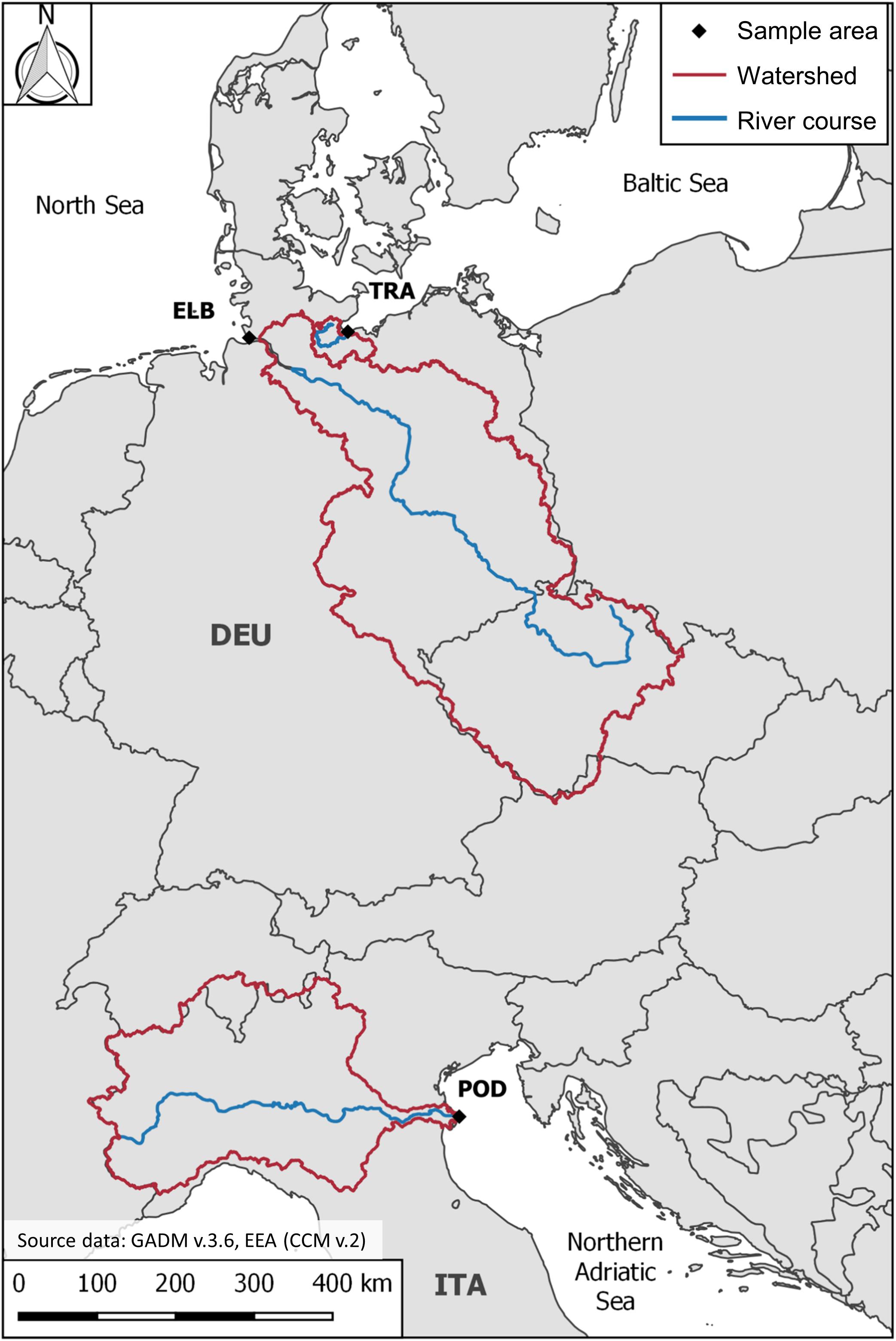
Figure 1. Study areas of the Trave (TRA) and the Elbe estuary (ELB) in Germany (DEU) and the Po delta (POD) in Italy (ITA), course of the rivers and their respective watersheds.
The Trave river in northeastern Germany has a length of 113 km, a watershed of 1,804 km2 (LM-MV and LM-SH, 2011) and exhibits an average annual discharge of 7.37 m3/s into the western Baltic Sea. Samples were taken from 22.05.2014 to 25.05.2014 at the brackish river mouth, a riverine shallow water firth with an average depth of 5.5 meter. Sampling was conducted along a distance of approximately 5 km with river width varying from approximately 22 to 535 m (see Supplementary Information 4). Water exchange with the Baltic Sea is limited through a narrow connection at Lübeck Bay with salinity ranges from 0.2 to 17.3 PSU. Oscillations of the sea level (<20 cm) is a result of wind-induced water movements as well as the effect of buildup of water reaching the coast. The Trave drainage area is highly influenced by agriculture, and tourism is an additional important economic factor. The harbor of Lübeck, located ∼18 km inland of the river mouth, serves as a shipyard location and a nationally important transfer point for passenger ferries and commercial goods.
The Elbe river in northwestern Germany is one of the largest rivers of Central Europe with a length of 1,100 km, an average yearly discharge of 844 m3/s into the North Sea and a watershed of 148,000 km2 which is home to 25 million people. Samples were taken from 19.08.2015 to 21.08.2015 within transitional waters of the tidally influenced river mouth (widths between ∼0.2 and 21 km) and adjacent coastal waters spanning a total length of ∼32 km (see Supplementary Information 5). The Elbe estuary is a partly- to well-mixed mesotidal estuary with a tidal amplitude ranging between 2 to 4 m. Between a single tide, an average distance of 20 km is traveled between capsize points. As a hyper-synchronous estuary, the tidal amplitude increases to a maximum between the coast toward the city of Hamburg and thereafter decreases. The Elbe estuary morphology and hydrology leads to a so called “maximum turbidity zone” (MTZ) where concentrations of suspended matter reach their highest value. Inputs from upstream are supplemented with additional inputs from adjacent areas such as dike-free river branches and wastewater discharges. The investigated area is significantly influenced by ship traffic, as the harbor of Hamburg is the third largest container port in Europe.
The Po river in Italy has a watershed of 74,000 km2 (Artioli et al., 2005) and a length of around 652 km (Lanzoni et al., 2015). Although exhibiting a smaller watershed as compared to the Elbe river, it contains a much higher population density representing almost half of the Italian population (∼30 million people). It is the longest Italian river and has an average annual discharge of 1,500 m3/s (Falcieri et al., 2014) flowing into the Northern Adriatic Sea (Figure 1). Given these factors, the Po river is thought to be the second most important marine litter source to the Adriatic basin (Liubartseva et al., 2016). Potential sources for MP include run-off and wastewater from intensively practiced agriculture, several industrial parks as well as large cities. The terminal river splits into seven active branches before entering the sea (Supplementary Information 6). As deltaic system, it exhibits a net sediment transport from the river to the delta. The Northern Adriatic Basin is influenced by tidal fluctuation of around 30 cm but under specific conditions can reach up to 140 cm (Maicu et al., 2018). Sampling was conducted from 10.06.2016 to 23.06.2016 at the terminal river branches with varying width from ∼63 to 795 m and extending ∼7.8 km offshore (see Supplementary Information 6).
Sampling
At each sampling location, water surface samples for MP and water constituents (Chl-A, SPM, CDOM), were simultaneously taken as described in detail by Atwood et al. (2019). Microplastic samples were concurrently collected from the top 15 cm of the water surface alongside motorboats and sailing ships using a mini-manta trawl equipped with a flowmeter (30 × 15 cm opening, 2 m long net with a mesh size of 300 μm). Water samples were always taken with flow direction of the river and in zigzag pattern perpendicular to the flow direction except for the Elbe estuary, where this maneuver was not possible due to intense ship traffic. Samples were stored in glass jars until processing in the laboratory. In total, 13 locations were sampled at the Trave river and 20 locations each at the Elbe estuary and the Po delta (see Supplementary Information 4, 5, and 6) as to cover a wide range of water constituents and MP concentrations. To assure similar conditions over the length of one trawl, water clarity was measured with a secchi disk at the beginning and the end of each trawl. For water constituents, 2 L water samples were concurrently collected with a glass flask from the water surface (top 40 cm) and kept both dark and cold until further processing. Later that same day, samples were separated and filtered on GF/F glass microfiber filters (0.7 μm pore size) for Chl-A following the JGOFS protocol (Knap et al., 1996) or pre-weighted cellulose acetate filters (0.45 μm pore size) for SPM following the protocol of Lindell et al. (1999).
Water Constituent Analysis
Chlorophyll pigments were extracted from the filters using 90% acetone [following JGOFS protocol (Knap et al., 1996)]. Extracted chlorophylls from the Trave river were analyzed with a fluorometer (Turner Designs, TD-700) with an excitation wavelength of 340–500 nm and an emission wavelength >665 nm. For the Elbe estuary and the Po delta, chlorophylls were extracted using 96% ethanol and extracts analyzed with a fluorometer (JASCO, FP-8600) at an excitation wavelength of 435 nm and an emission wavelength of 670 nm. The fluorometer was calibrated using a photometer (JASCO, V-670) and a Chl-A standard (Sigma-Aldrich, C6144-1MG). After each first measurement, samples were acidified with HCl and again measured to allow the subtraction of phaeopigments in order to obtain Chl-A concentration following the JGOFS protocol (Knap et al., 1996). Although extraction protocols slightly differed, obtained results do not influence the general results, as regression analysis with MPs were performed separately for each river system.
Samples for SPM analysis were dried for 2 h at 60–80°C and cooled in a desiccator for 10–15 min before weighted on an analytical balance (Sartorius, R 200 D).
Absorbance analysis of CDOM was conducted using the filtrate of the SPM samples (material <0.45 μm). Duplicate samples were stored in glass flasks covered with aluminum foil and stored at a temperature of 7°C until spectrophotometric analysis. Measurements were taken with a UV-VIS-NIR spectroradiometer (PerkinElmer, LAMBDA 950) using 5 cm glass cuvettes. Absorbance measurements were performed at the wavelength positions 400, 412, 420, and 440 nm.
Microplastic Sample Processing
Each sample was first fractionated into two size classes: 5000–500 and 500–300 μm. All potential MP particles >500 μm were visually pre-sorted under a stereomicroscope (Leica, M50) using a counting chamber for zooplankton. For a more efficient visual pre-sorting, samples with a high organic content were additionally treated with a wet peroxide oxidation (Masura et al., 2015). Potential MPs were photographed (Olympus, DP 26), and stored in Eppendorf tubes for analysis with Attenuated Total Reflectance (ATR)-Fourier transform-infrared (FT-IR) spectroscopy (Löder et al., 2015).
The smaller size fraction (500–300 μm) of all samples was treated with an enzymatic purification protocol (Löder et al., 2017) and afterward with wet peroxide oxidation (Masura et al., 2015) to remove organic matter, which would interfere with subsequent spectroscopic analysis. As remaining particle numbers were still very high, subsamples were filtered onto 25 mm aluminum oxide filters (Whatman, Anodisc 25). Detailed information on the subsamples can be found in the Supplementary Information (see Supplementary Information 1). Subsamples were analyzed with a Focal Plane Array (FPA) based micro FT-IR (μFT-IR) spectroscope (Bruker, Tensor 27 FT-IR spectrometer equipped with a Hyperion 3000 FT-IR microscope with a 15× Cassegrain objective and a 64 × 64 FPA detector) according to Löder et al. (2015). In order to conduct a full quantitative analysis, the remainder of each sample was filtered onto glass fiber filters (Macherey-Nagel, MN 85/90 BF) and analyzed with a newly developed hyperspectral imaging methodology (Schmidt et al., 2018) using a near-infrared (NIR) imaging spectrometer (Norsk Elektro Optics AS, HySpex SWIR-320m-e).
To account for potential airborne contamination of the samples with small microplastics (500–300 μm), negative controls with filtered water were processed together with the microplastic samples for each set of samples from a river. In total, six negative controls were measured with the HySpex imaging spectrometer on which no MP particles were found. Mostly fibers were found (0.7 to 11.6 fibers on average for the three investigated data sets) within a total of 15 negative controls analyzed with FPA based μFT-IR spectroscopy (Supplementary Information 3). Only for the Po delta was one polypropylene particle found in one out of six negative controls corresponding to 0.17 particles on average. This amount was subtracted from measured polypropylene abundances in each sample within the Po delta data set. Synthetic fibers were generally not considered within this study as synthetic fibers normally exhibit diameters between 6 and 175 μm (Cole et al., 2015; Dris et al., 2016, 2018) which could not be sampled quantitatively with the utilized manta net. As no fibers or particles >500 μm were observed within the negative controls, it is assumed that airborne contamination of the larger size class can be neglected. Finally, obtained MP particle numbers from all three methods were added for each sample and standardized according to the filtered water volume to MP particles per cubic meter.
Statistical Analysis
All statistical analyses were performed using the software R v.3.5.2. Linear regression analyses were conducted to test the unidirectional null hypothesis that MP abundance will not increase with increasing water constituent abundance. To meet model assumptions for parametric linear regression analysis, the Trave data were square root transformed and for the Po delta data a log transformation was applied. Correlation analyses among all investigated water constituents were conducted to check for collinearity. Relationships were accepted as significant at p < 0.05, and all results are shown as average ± one standard deviation (SD).
To further investigate potential patterns within the datasets, principal component analyses (PCA) were applied. The packages “FactoMineR” (v.1.34) and “factoextra” (v.1.0.7) were used for PCA and biplots for visualization of the data. First the predictor variables were scaled to have a SD of one and a mean of zero. Two missing values within the Po delta dataset were excluded from the analysis, although imputing the two data points by predictive mean matching produced similar results. The function “prcomp” was used to calculate principal components (PCs) via singular value decomposition on the original data matrix.
To investigate potential spatial relationships between MP and water constituent abundance, subgroups according to sampling location were defined. For the Trave, the categories included river, wastewater treatment plant (WWTP), and coastal samples. For the Elbe data, a distinction between coastal and river samples was made, whereas the categories river, mouth (representing the seven distal branches of the delta), and coastal samples were defined for the Po delta data.
Results
The three chosen river systems exhibited the assumed wide range of MP concentrations, ranging from 1 to 78 particles/m3 at the Po delta, followed by the Elbe estuary with 0–11 particles/m3 to only a few particles at the Trave river (0–1 particles/m3; Figure 2, specific values in table Supplementary Information 1). For the Trave river generally higher MP concentrations were observed within the river than the coastal area (Supplementary Information 4) whereas for the Elbe estuary MP concentrations showed an increasing trend toward the coast (Supplementary Information 5). At the Po delta elevated MP concentrations were observed within the river branches, as well as the outer river plume (Supplementary Information 6). Linear regression analysis revealed a significant relationship between the abundance of MP and all three investigated water constituents for the Trave river, whereas Chl-A explaining the largest portion of the variance (Figure 2). Nevertheless, this result has to be interpreted with caution as MP abundances were generally low within this river system (Figure 2, Supplementary Information 1). PCA was conducted and results displayed within biplots to reveal further patterns within the data sets (Figure 3).
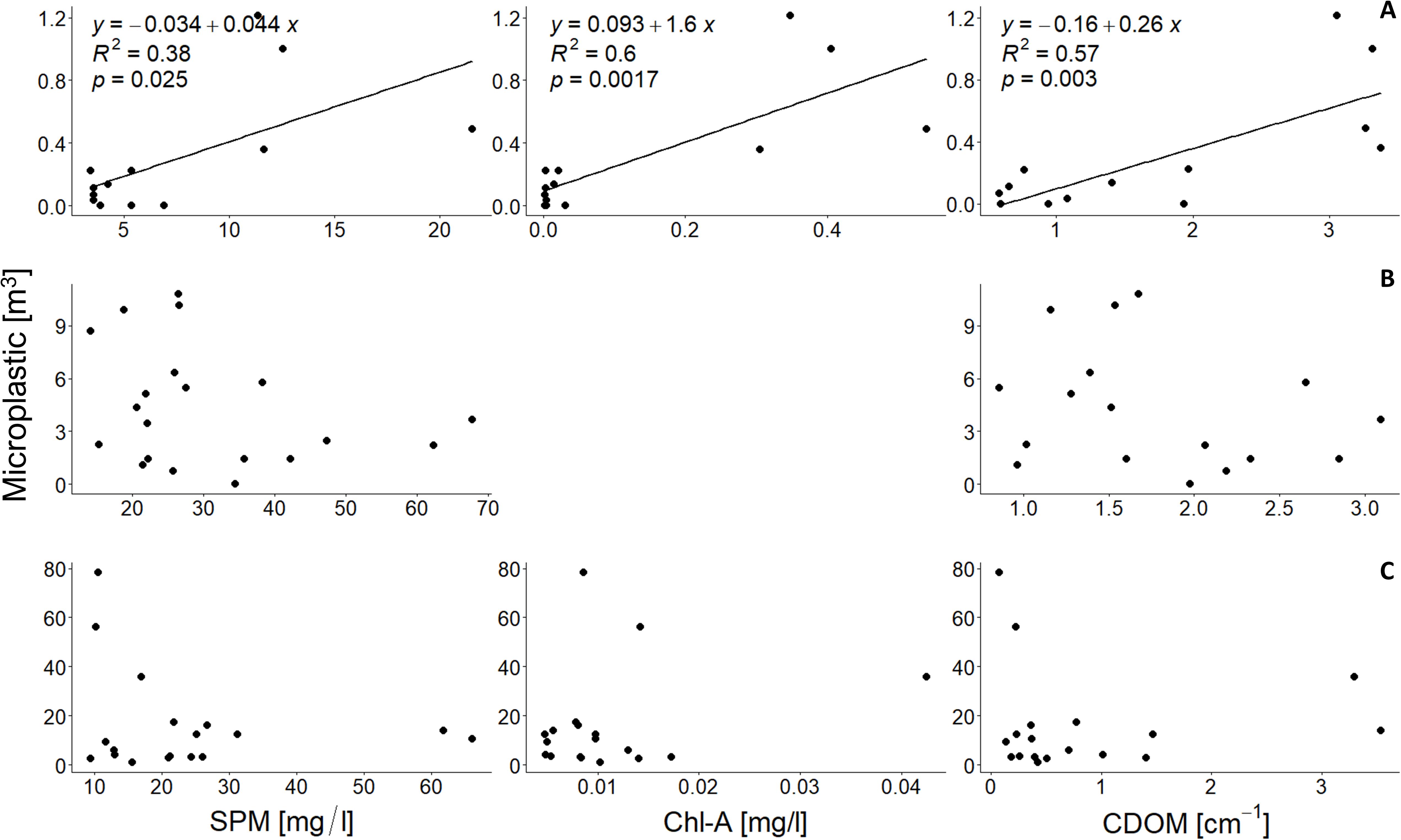
Figure 2. Relationship between measured water constituents and microplastic (0.3–5 mm) at the Trave river (A), the Elbe estuary (B), and the Po delta (C). Linear regression analysis revealed a significant positive relationship among the abundance of all three water constituents and microplastic abundance for the Trave river (A). Chl-A, chlorophyll-a, SPM, suspended particulate matter, CDOM, colored dissolved organic matter.
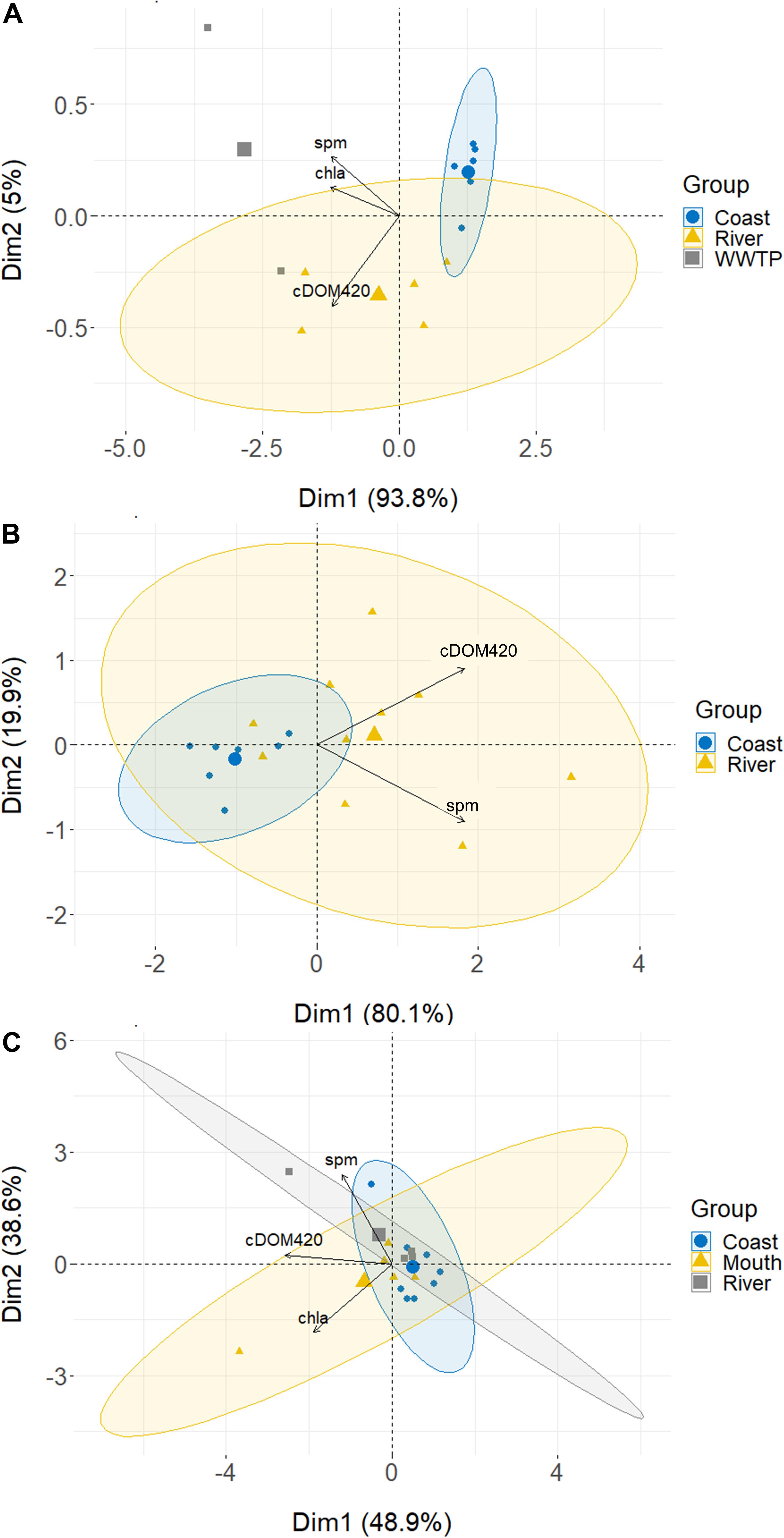
Figure 3. Biplots of the Trave river (A), the Elbe estuary (B), and the Po delta (C) showing the scores of individual samples grouped by different symbols and colors. Loadings of the predictor variables chlorophyll-a (chla), suspended particulate matter (spm), and colored dissolved organic matter (cDOM420) on the first two principal components (Dim1 and Dim2) are represented as vectors. Larger symbols depict the center of the confidence ellipse (95% confidence level) drawn around the groups.
PCA of the Trave data revealed that the first PC, explaining 93.8% of the variance within the data, is almost equally represented by all three water constituents: Chl-A (35%), SPM (34%), and CDOM (32%) (Figure 3A). The second PC (explaining 5% of variance) instead is mostly represented by CDOM (65%) and SPM (28%). Microplastic abundances in coastal areas are better represented by CDOM with only a small angle between the main axis of the ellipse and the CDOM vector (Figure 3A).
Considering PCA results of the Elbe estuary a similar pattern can be seen with coastal samples better represented by CDOM (Figure 3B). The two predictor variables SPM and CDOM are equally represented (each 50%) by both PCs (Figure 3B).
Contrarily, none of the investigated water constituents could predict MP abundance for either the Elbe estuary (SPM: F = 1.72, p = 0.21 and CDOM: F = 1.25, p = 0.28) or the Po delta (Chl-A: F = 1.14, p = 0.30, SPM: F = 1.71, p = 0.21, and CDOM: F < 0.001, p = 0.99). For the Elbe estuary, the lacking relationship was further indicated by a second field data acquisition in June. Here, one of the highest MP values (4.44 MP particles/m3) correlated with one of the highest SPM concentration (323.87 g/l) but the very highest MP value (4.60 MP particles/m3) was sampled on a spot with a low SPM concentration (20.78 g/l) (Supplementary Information 1).
Furthermore, all water constituents showed a positive correlation among each other for both German river systems, whereas for the Po delta none of the investigated predictor variables correlated (Supplementary Information 2). Conducting PCA for the Po delta, the first PC is best represented by CDOM (57%) and Chl-A (31%), but only explaining around 49% of the variance (Figure 3C). The second PC is mostly represented by SPM (62%) and Chl-A (37%), explaining around 37% of the variance (Figure 3C). No clear differences in MP abundances between river mouth, river, and coastal samples can be seen (Figure 3C).
Discussion
A clear relationship among water constituents and MP for all three investigated river systems could not be identified. The positive relationships between the three proxy water constituents and MP abundances found for the Trave river needs to be verified in future studies, as the results are based on very low MP abundances within the obtained water samples (Supplementary Information 1). As indicated by the biplots, depending on the estuary type, spatial relationships between water constituents and MPs are further spatially limited. Thus, potential relationships need to be evaluated for various river systems separately and additionally for the river course and the coastal zone.
One factor influencing possible relationships between water constituents and MPs are the presence and location of their emission sources. In the Trave river, higher concentrations of MPs were found within the river, specifically close to the WWTP (Supplementary Information 4) and gradually decreasing toward the Baltic Sea. For the Baltic Sea, it is assumed that land-based litter inputs are dominating (Haseler et al., 2018) and thus the lower MP water surface concentrations at the coastal area may be a dilution effect. For the Elbe estuary, results show a tendency of higher abundance of MPs within the adjacent coastal area compared to the inner parts of the river (Supplementary Information 5). The increasing MP concentrations at the Elbe estuary toward the coastal area may be a consequence of additional inputs of sea-based sources. Both the study system of the Elbe estuary and the Po delta are highly influenced by fishing and aquaculture related litter inputs (Simeoni and Corbau, 2009; Kammann et al., 2018). Nevertheless, for the Po delta with its diverse terminal branches no pattern in MP abundance could be detected (Figure 3C and Supplementary Information 6). The protruding form of the Po delta plays an important role for dispersion of suspended material, as MP and water constituents emitted by the branches exhibit different hydrodynamical and meteorological conditions. The hydrodynamical conditions spanning from a mixed mesotidal estuary (Elbe) to nearly stratified systems (Trave, Po) generally influence spatial patterns of suspended materials and thus, further may explain observed differences.
For example, SPM showed no spatial relationship with MPs at the Elbe estuary. Nevertheless, a study on larger litter collected with bottom trawls and at the shore within an estuary in South America, reported that larger litter was accumulating in the MTZ (Acha et al., 2003). In our study, we focused on water surface MP concentrations at the Elbe estuary which also exhibits an MTZ. Even though our sampling did not cover the MTZ in August, during another sampling in June samples were taken closer to Hamburg city (Supplementary Information 5) and the MTZ was covered according to salinity measurements (Supplementary Information 1; located between 0.1 and 4 PSU; Herman and Heip, 1999). Here, a correlation analysis revealed a relationship between SPM and MPs (Spearman’s rank correlation, S = 154, p = 0.01, rho = 0.66). The positive relationship of SPM and MPs at the Trave, explaining 38% of the variance, could be due to dominating riverine inputs for both. The missing relationship at the Po delta on the opposite could be the result of differing sources of SPM and MPs among the several distal branches at the investigated site. Suspended particulate matter dynamics are coupled to meteorological conditions and will vary on small temporal scales. For example, higher SPM inputs occur during high precipitation events due to increased terrestrial runoff as well as mobilization of riverbed sediments. Likewise, high MP export was already associated with high flow events in rivers (Hurley et al., 2018). Considering CDOM as proxy water constituent for MPs, only at the Trave river was a positive relationship found, whereas PCA showed that CDOM explained most of the variance in coastal samples (Figure 3A). A similar pattern was found for the Elbe estuary (Figure 3B) even though a relationship could not be verified by regression analysis (Figure 2B). The absence of a relationship for the other two investigated sites can be explained by differing DOM dynamics among systems. For example, Berto et al. (2010) has shown an influence on DOM dynamics on a monthly and sub-monthly time scale at the Po delta. Processes leading to higher CDOM concentrations in spring and summer vary with higher inputs due to melt-water run-off from rivers in spring (Ferrari and Dowell, 1998; Stedmon and Nelson, 2014). Moreover, higher photo-bleaching in summer can alter the optical properties of surface waters and lead to decreased CDOM absorption and fluorescence (Vodacek et al., 1997). Both processes influence potential spatial as well as temporal relationships, in particular on a seasonal scale, of CDOM and MPs.
Even though a positive relationship between water constituents and MP abundance was found for the Trave river, the results need further validation since results are based on generally low MP abundances (Supplementary Information 1) as well as only one sampling campaign. Following linear regression, most variance was explained by Chl-A (62%). Contrarily, a correlation between MPs and Chl-A could not be detected for the Po delta. Although the slightly different extraction protocols used should not have influenced the observed results as samples were treated equally within a dataset, this source of potential error cannot be definitely excluded. Even though standard protocols for the analysis of chlorophyll exist, they vary in their results. A relationship would be further influenced by seasonal patterns, as well as the ability of some algae to influence their vertical position in the water column to counteract sinking out from the euphotic zone as well as resource acquisition (Raven and Doblin, 2014).
Remote sensing of water constituents in coastal systems is difficult due to the overlapping spectral signal from different optical components. The Trave provided the clearest CDOM signal of all three river systems, in that the SPM concentration was relatively low and the CDOM concentration relatively high. The Po delta was found to have the lowest CDOM concentrations and some of the highest SPM concentrations of all three study systems. Even though the least amount of variance was explained by SPM within the Trave data, from an in-situ monitoring point of view, SPM fulfills the requirements for an operational monitoring in being cost- and labor-effective, as well as easy to assess. A relationship between MP concentrations and sediment grain size as well as organic matter deposition has been proposed in previous studies (Vianello et al., 2013; Maes et al., 2017; Haave et al., 2019). Haave et al. (2019) further reported that particles <100 μm made up >95% of the MP concentration, whereas particles >500 μm showed different spatial distribution patterns. This points toward the importance of MP particles <300 μm not covered by our MP sampling method. Thus, it would be interesting in future studies to test the relationship of SPM and MPs with recently developed pumping systems which are able to extract MPs down to 1 μm (Lenz and Labrenz, 2018). In conjunction with the development of faster identification methods of MPs, higher sample replication can be achieved, resulting in more precise model results.
Further research in the field is desirable as satellite imagery has the potential to complement spatial and temporal limited point measurements and thus improve our current understanding of sources, transport, and accumulation of MPs within river courses and adjacent coastal areas.
In this study, we analyzed a spatial relationship (Figure 4) between in-situ MPs (Figure 4, component 1) and in-situ water constituents (Figure 4, component 2). Further steps are necessary to transfer remotely sensed water constituents into MP abundance to produce MP distribution maps along rivers and coasts which is described in detail by Atwood et al. (2019) and shown in Figure 4 (components 3, 4, 6, and 7). Near-range spectral measurements (Figure 4, component 3) taken concurrent to sample collection would be needed to build regionally calibrated remote sensing spectral reflectance water parameter algorithms (Figure 4, component 6) for different satellite platforms (Figure 4, component 4) as specified in Atwood et al. (2019). Through the adaption of the remote sensing imagery of water constituents (Figure 4, component 7) with the calibrated algorithms, maps of the water constituents could then be used as proxy for MP. Existing single point measurements could thereby be extrapolated to large spatial scales to produce maps of MP distribution along rivers and coastal areas (Figure 4, component 8).
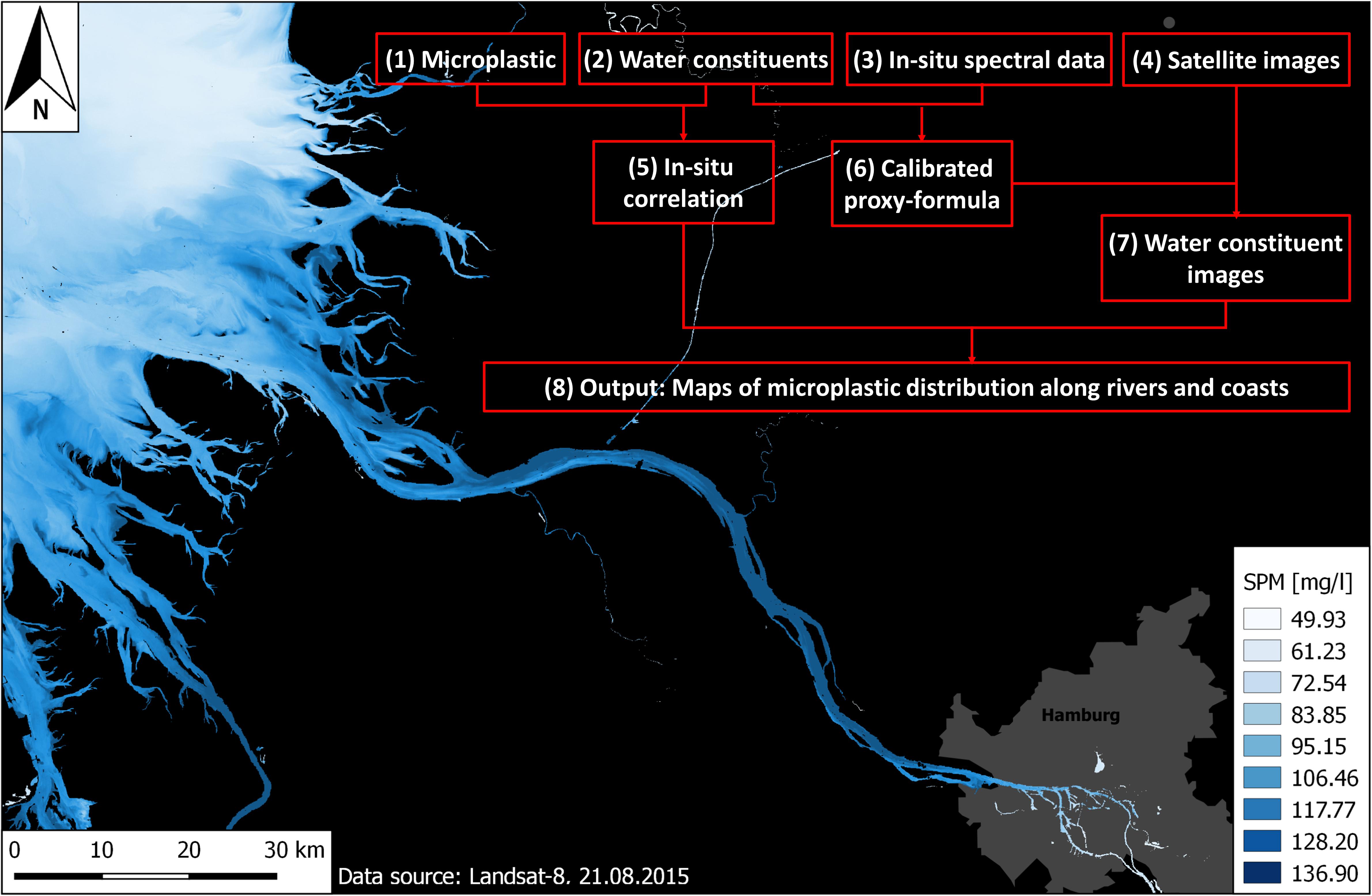
Figure 4. Satellite image of suspended particulate matter (SPM) at the Elbe esturay. The diagram illustrates the proposed framework to track microplastic distribution via proxy water constituent maps (software: QGIS 2.14.8).
Data Availability Statement
All datasets generated for this study are included in the article/Supplementary Material.
Author Contributions
MB, JF, FS, and CL initiated the study. All authors designed the study. SP, EA, MB, and HI conducted the field sampling. SP prepared the microplastic samples with the help of four technichal assistants, conducted the microplastic particle identification with FT-IR spectroscopy, and wrote the first draft of the manuscript. MB conducted the NIR microplastic particle identification as well as did the analysis of CDOM. SP and HI did Chl-A and SPM analysis. EA processed the remote sensing data. SP analyzed the data. SP, EA, MB, HI, JF, and CL discussed and interpreted the data. SP and EA created the figures and tables. All authors were involved in revision and rewriting of the manuscript.
Funding
This publication was funded by the German Research Foundation (DFG) and the University of Bayreuth in the funding programme Open Access Publishing. This study was partly funded by the German Federal Ministry for Economic Affairs and Energy (Bundesministerium für Wirtschaft und Energie, or BMWi) via the DLR Space Administration under the grant numbers 50EE1301 and 50EE1269.
Conflict of Interest
EA, JF, and FS were employed by the company RSS Remote Sensing Solutions GmbH.
The remaining authors declare that the research was conducted in the absence of any commercial or financial relationships that could be construed as a potential conflict of interest.
Acknowledgments
The authors would like to thank the crew of the sailing ships “Bildungsschiff Niederelbe e.V.” and “Aldebaran Marine Research and Broadcast” as well as our boat captains in Italy for flexible ship times and help during field data acquisitions. For storage and shipping of Chl-A samples, we would like to thank the Thünen Institut of Fisheries Ecology in Cuxhaven. For help with the analysis of the Chl-A samples, we thank Dr. Ben Gilfedder and Silke Hammer from the Limnological Station/chair of hydrology of the University of Bayreuth and Prof. Stibor of the Department Biology II/Aquatic Ecology of the Ludwig-Maximilians University Munich. For measurements of the SPM samples, thanks are extended to Prof. Dettner of the Department Animal Ecology II of the University of Bayreuth. Ursula Wilczek, Heghnar Martirosyan, Marion Preiß, and Lena Löschel are very heartfully thanked for their help in the lab with the microplastic samples. Finally, we would like to thank the reviewers for their valuable comments which substantially improved the manuscript.
Supplementary Material
The Supplementary Material for this article can be found online at: https://www.frontiersin.org/articles/10.3389/fenvs.2020.00092/full#supplementary-material
References
Acha, E. M., Mianzan, H. W., Iribarne, O., Gagliardini, D., Lasta, C., and Daleo, P. (2003). The role of the Río de la Plata bottom salinity front in accumulating debris. Mar. Pollut. Bull. 46, 197–202. doi: 10.1016/s0025-326x(02)00356-9
Artioli, Y., Bendoricchio, G., and Palmeri, L. (2005). Defining and modelling the coastal zone affected by the Po river (Italy). Ecol. Model. 184, 55–68. doi: 10.1016/j.ecolmodel.2004.11.008
Atwood, E. C., Falcieri, F. M., Piehl, S., Bochow, M., Matthies, M., Franke, J., et al. (2019). Coastal accumulation of microplastic particles emitted from the Po River, Northern Italy: comparing remote sensing and hydrodynamic modelling with in situ sample collections. Mar. Pollut. Bull. 138, 561–574. doi: 10.1016/j.marpolbul.2018.11.045
Berto, D., Giani, M., Savelli, F., Centanni, E., Ferrari, C. R., and Pavoni, B. (2010). Winter to spring variations of chromophoric dissolved organic matter in a temperate estuary (Po River, northern Adriatic Sea). Mar. Environ. Res. 70, 73–81. doi: 10.1016/j.marenvres.2010.03.005
Cole, M., Webb, H., Lindeque, P. K., Fileman, E. S., Halsband, C., and Galloway, T. S. (2015). Isolation of microplastics in biota-rich seawater samples and marine organisms. Sci. Rep. 4:4528. doi: 10.1038/srep04528
Directive of the European parliament. (2008). Establishing a framework for Community action in the field of marine environmental policy (Marine Strategy Framework Directive). Official J. Eur. Union L164, 19–40.
Dris, R., Gasperi, J., Rocher, V., and Tassin, B. (2018). Synthetic and non-synthetic anthropogenic fibers in a river under the impact of Paris Megacity: sampling methodological aspects and flux estimations. Sci. Total Environ. 618, 157–164. doi: 10.1016/j.scitotenv.2017.11.009
Dris, R., Gasperi, J., Saad, M., Mirande, C., and Tassin, B. (2016). Synthetic fibers in atmospheric fallout: a source of microplastics in the environment? Mar. Pollut. Bull. 104, 290–293. doi: 10.1016/j.marpolbul.2016.01.006
Enders, K., Lenz, R., Ivar do Sul, J. A., Tagg, A. S., and Labrenz, M. (2020). When every particle matters: a QuEChERS approach to extract microplastics from environmental samples. MethodsX 7:100784. doi: 10.1016/j.mex.2020.100784
Falcieri, F. M., Benetazzo, A., Sclavo, M., Russo, A., and Carniel, S. (2014). Po River plume pattern variability investigated from model data. Cont. Shelf Res. 87, 84–95. doi: 10.1016/j.csr.2013.11.001
Ferrari, G. M., and Dowell, M. D. (1998). CDOM absorption characteristics with relation to fluorescence and salinity in coastal areas of the southern Baltic Sea. Estuar. Coast. Shelf Sci. 47, 91–105. doi: 10.1006/ecss.1997.0309
Haave, M., Lorenz, C., Primpke, S., and Gerdts, G. (2019). Different stories told by small and large microplastics in sediment - first report of microplastic concentrations in an urban recipient in Norway. Mar. Pollut. Bull. 141, 501–513. doi: 10.1016/j.marpolbul.2019.02.015
Harvey, E. T., Kratzer, S., and Andersson, A. (2015). Relationships between colored dissolved organic matter and dissolved organic carbon in different coastal gradients of the Baltic Sea. Ambio 44, 392–401. doi: 10.1007/s13280-015-0658-4
Haseler, M., Schernewski, G., Balciunas, A., and Sabaliauskaite, V. (2018). Monitoring methods for large micro- and meso-litter and applications at Baltic beaches. J. Coast. Conserv. 22, 27–50. doi: 10.1007/s11852-017-0497-5
Herman, P. M. J., and Heip, C. H. R. (1999). Biogeochemistry of the MAximum TURbidity Zone of Estuaries (MATURE): some conclusions. J. Mar. Syst. 22, 89–104. doi: 10.1016/S0924-7963(99)00034-2
Hurley, R., Woodward, J., and Rothwell, J. (2018). Microplastic contamination of river beds significantly reduced by catchment-wide flooding. Nat. Geosci. 11, 251–257. doi: 10.1038/s41561-018-0080-1
Kammann, U., Aust, M.-O., Bahl, H., and Lang, T. (2018). Marine litter at the seafloor – Abundance and composition in the North Sea and the Baltic Sea. Mar. Pollut. Bull. 127, 774–780. doi: 10.1016/j.marpolbul.2017.09.051
Knap, A., Michaels, A., Close, A., Ducklow, H., and Dickson, A. (eds) (1996). Protocols for the Joint Global Ocean Flux Study (JGOFS) Core Measurements. JGOFS Report Nr. 19, vi+170 pp. Reprint of the IOC Manuals and Guides No. 29. Paris: UNESCO, 1994.
Lanzoni, S., Luchi, R., and Bolla Pittaluga, M. (2015). Modeling the morphodynamic equilibrium of an intermediate reach of the Po River (Italy). Adv. Water Resour. 81, 95–102. doi: 10.1016/j.advwatres.2014.11.004
Lebreton, L. C.-M., Greer, S. D., and Borrero, J. C. (2012). Numerical modelling of floating debris in the world’s oceans. Mar. Pollut. Bull. 64, 653–661. doi: 10.1016/j.marpolbul.2011.10.027
Lenz, R., and Labrenz, M. (2018). Small microplastic sampling in water: development of an encapsulated filtration device. Water 10:1055. doi: 10.3390/w10081055
Lindell, T., Pierson, D., and Premazzi, G. (1999). Manual for Monitoring European Lakes using Remote Sensing Techniques. Italy: Joint Research Centre.
Liubartseva, S., Coppini, G., Lecci, R., and Creti, S. (2016). Regional approach to modeling the transport of floating plastic debris in the Adriatic Sea. Mar. Pollut. Bull. 103, 115–127. doi: 10.1016/j.marpolbul.2015.12.031
LM-MV and LM-SH. (2011). Vorläufige Bewertung des Hochwasserrisikos (gem. Art. 4) und Bestimmung der Gebiete mit potenziell signifikantem Hochwasserrisiko (gem. Art. 5). Schleswig-Holstein: Ministerium für Energiewende, Umwelt, Landwirtschaft, Natur und Digitalisierung. Available online at: https://www.schleswig-holstein.de/DE/Fachinhalte/H/hochwasserschutz/Downloads/Bewertung_SchleiTrave.pdf?__blob=publication File&v=1
Löder, M. G. J., Imhof, H. K., Ladehoff, M., Löschel, L. A., Lorenz, C., Mintenig, S., et al. (2017). Enzymatic purification of microplastics in environmental samples. Environ. Sci. Technol. 51, 14283–14292. doi: 10.1021/acs.est.7b03055
Löder, M. G. J., Kuczera, M., Mintenig, S., Lorenz, C., and Gerdts, G. (2015). Focal plane array detector-based micro-Fourier-transform infrared imaging for the analysis of microplastics in environmental samples. Environ. Chem. 12:563. doi: 10.1071/EN14205
Maes, T., Van der Meulen, M. D., Devriese, L. I., Leslie, H. A., Huvet, A., Frère, L., et al. (2017). Microplastics baseline surveys at the water surface and in sediments of the north-east atlantic. Front. Mar. Sci. 4:135. doi: 10.3389/fmars.2017.00135
Maicu, F., De Pascalis, F., and Ferrarin, C. (2018). Hydrodynamics of the po river-delta-sea system. J. Geophys. Res. 123, 6349–6372. doi: 10.1029/2017JC013601
Masó, M., Garcés, E., Pagès, F., and Camp, J. (2003). Drifting plastic debris as a potential vector for dispersing Harmful Algal Bloom (HAB) species. Sci. Mar. 67, 107–111. doi: 10.3989/scimar.2003.67n1107
Masura, J., Baker, J., Foster, G., and Arthur, C. (2015). “Laboratory Methods for the Analysis of Microplastics in the marine environment: recommendations for quantifying synthetic particles in waters and sediments,” in Proceedings of the Technical Memorandum NOS-OR&R-48 (Silver Spring, MD: NOAA Marine Debris Program, NOAA Marine Debris Division).
Pichel, W. G., Churnside, J. H., Veenstra, T. S., Foley, D. G., Friedman, K. S., Brainard, R. E., et al. (2007). Marine debris collects within the north pacific subtropical convergence zone. Mar. Pollut. Bull. 54, 1207–1211. doi: 10.1016/j.marpolbul.2007.04.010
Raven, J. A., and Doblin, M. A. (2014). Active water transport in unicellular algae: where, why, and how. J. Exp. Bot. 65, 6279–6292. doi: 10.1093/jxb/eru360
Rezania, S., Park, J., Md Din, M. F., Mat Taib, S., Talaiekhozani, A., Kumar Yadav, K., et al. (2018). Microplastics pollution in different aquatic environments and biota: a review of recent studies. Mar. Pollut. Bull. 133, 191–208. doi: 10.1016/j.marpolbul.2018.05.022
Schmidt, L. K., Bochow, M., Imhof, H. K., and Oswald, S. E. (2018). Multi-temporal surveys for microplastic particles enabled by a novel and fast application of SWIR imaging spectroscopy – Study of an urban watercourse traversing the city of Berlin. Germany. Environ. Pollut. 239, 579–589. doi: 10.1016/j.envpol.2018.03.097
Sharma, S., and Chatterjee, S. (2017). Microplastic pollution, a threat to marine ecosystem and human health: a short review. Environ. Sci. Pollut. Res. 24, 21530–21547. doi: 10.1007/s11356-017-9910-8
Simeoni, U., and Corbau, C. (2009). A review of the Delta Po evolution (Italy) related to climatic changes and human impacts. Geomorphology 107, 64–71. doi: 10.1016/j.geomorph.2008.11.004
Stedmon, C. A., and Nelson, N. B. (2014). The Optical Properties of DOM in the Ocean. Second Edi. Amsterdam: Elsevier Inc, doi: 10.1016/B978-0-12-405940-5.00010-8
UN Resolution (2020). Transforming our World: the 2030 Agenda for Sustainable Development. New York, NY: United Nations.
van Wijnen, J., Ragas, A. M. J., and Kroeze, C. (2019). Modelling global river export of microplastics to the marine environment: sources and future trends. Sci. Total Environ. 673, 392–401. doi: 10.1016/j.scitotenv.2019.04.078
Vianello, A., Boldrin, A., Guerriero, P., Moschino, V., Rella, R., Sturaro, A., et al. (2013). Microplastic particles in sediments of Lagoon of Venice, Italy: first observations on occurrence, spatial patterns and identification. Estuar. Coast. Shelf Sci. 130, 54–61. doi: 10.1016/j.ecss.2013.03.022
Vodacek, A., Blough, N. V., DeGrandpre, M. D., Peltzer, E. T., and Nelson, R. K. (1997). Seasonal variation of CDOM and DOC in the Middle Atlantic Bight: terrestrial inputs and photooxidation. Limnol. Oceanogr. 42, 674–686. doi: 10.4319/lo.1997.42.4.0674
Wang, W., Gao, H., Jin, S., Li, R., and Na, G. (2019). The ecotoxicological effects of microplastics on aquatic food web, from primary producer to human: a review. Ecotoxicol. Environ. Saf. 173, 110–117. doi: 10.1016/j.ecoenv.2019.01.113
Keywords: microplastic, spectroscopy, remote sensing, HySpex, SPM, chlorophyll, CDOM
Citation: Piehl S, Atwood EC, Bochow M, Imhof HK, Franke J, Siegert F and Laforsch C (2020) Can Water Constituents Be Used as Proxy to Map Microplastic Dispersal Within Transitional and Coastal Waters? Front. Environ. Sci. 8:92. doi: 10.3389/fenvs.2020.00092
Received: 12 March 2020; Accepted: 03 June 2020;
Published: 26 June 2020.
Edited by:
André Ricardo Araújo Lima, Center for Marine and Environmental Sciences (MARE), PortugalReviewed by:
Erik Van Sebille, Utrecht University, NetherlandsTomoya Kataoka, Tokyo University of Science, Japan
Jesse Patrick Harrison, CSC – IT Center for Science (Finland), Finland
Copyright © 2020 Piehl, Atwood, Bochow, Imhof, Franke, Siegert and Laforsch. This is an open-access article distributed under the terms of the Creative Commons Attribution License (CC BY). The use, distribution or reproduction in other forums is permitted, provided the original author(s) and the copyright owner(s) are credited and that the original publication in this journal is cited, in accordance with accepted academic practice. No use, distribution or reproduction is permitted which does not comply with these terms.
*Correspondence: Christian Laforsch, Y2hyaXN0aWFuLmxhZm9yc2NoQHVuaS1iYXlyZXV0aC5kZQ==