- 1Argonne National Laboratory, Lemont, IL, United States
- 2Earth Systems Research Center, University of New Hampshire, Durham, NH, United States
- 3Geophysical Institute, University of Alaska, Fairbanks, AK, United States
- 4Pakistan Water and Power Development Authority, Lahore, Pakistan
Water stored in the form of snow and glaciers in the High Mountain Asia (HMA) region regulates the water supply, and resultant water-based economies, that support the livelihoods of millions of people. Trends in the seasonal and long-term melting of snow and glaciers, governed by initial ice reserves, meteorological factors and geographic features, vary across sub-basins in the HMA region. We examined the economic impacts of climate-led changes in river flow in two drainage basins, one each from the Karakoram and Central Himalaya region. We used an integrated assessment framework to estimate the changes in economic value of the hydropower generation from hydropower plants on rivers fed by snow and glacier melt in the two sub-basins. The framework, developed under a NASA High Mountain Asia project, coupled biophysical models (a suite of climate models, snow/glacier-hydrology, and hydropower model) with economic analysis. We compared the differences in estimated river flow over historic and future time using the water balance model in sixteen scenarios (eight climate models and two emissions scenarios) for rivers upstream of hydropower plants in each sub-basin. Using the hydropower model we developed, we estimated the changes in hydropower generation at the Naltar IV hydropower plant, with an 18 MW capacity, located in Hunza, Karakoram, and the Trishuli hydropower plant, with a 19.6 MW capacity, in Trishuli, Central Himalaya. When compared to their baselines, the estimated impact of climate change and temporal variability were higher for the Naltar plant than for the Trishuli plant. Our sensitivity analysis shows that hydropower plants with water storage facilities help reduce the impact of changes, but the estimated impacts are higher for the higher capacity plants. This study provides an example of the differential impacts of climate change on hydropower plants located in rivers fed by varying amounts of snow and glacier melt at different decades in this century. This type of integrated assessment of climate change impact will support the scientific understanding of hydrologic flow and its impacts on a hydropower economy under various climate scenarios, as well as generate information about water resource management in a changing climate.
Introduction
Water stored in the form of snow and glaciers in the High Mountain Asia (HMA) region regulates the water supply, and resultant water-based economies, that support the livelihoods of millions of people (Viviroli et al., 2011; Lutz et al., 2014; Biemans et al., 2019; Immerzeel et al., 2019; Pritchard, 2019). Climate-mediated changes, such as retreating glaciers, variability in precipitation, snow cover extent, and melting, have changed the availability of water downstream seasonally and over the century. The hydrologic cycles of the sub-basins in the HMA are impacted differently by climate drivers and the geographic and socioeconomic diversity across the study footprint results in a range of impacts across the sub-basins.
The hydrology of the HMA region is influenced by the quantity of snowfall and rainfall, the characteristics of the glaciers, the atmospheric temperature in the melt season, and the share of snow and glacier melt in river flows. The extent of westerly and monsoon influence varies with the location of a given sub-basin in the region, but the melting of snow and glaciers differ from sub-basin to sub-basin depending upon such factors as the quantity, location and orientation of snowpack, solar radiation, rainfall, etc. Governments in this region have been encouraging the development of relatively low-cost electricity production from hydropower plants to meet the increasing demand for electricity in the region. Information about projected changes in river flow, seasonally and over the century, is important for infrastructure development and planning downstream, including critical hydropower and irrigation infrastructure.
Climatic drivers and their influences on the hydrology in the Karakoram are different from those in the Central Himalaya region. Figure 1 shows the locations of the two regions in HMA. Fowler and Archer (2006) and Khattak et al. (2011) observed trends of increasing precipitation as well as rising winter mean and maximum temperature (including at high elevations) in the Karakoram region. In Central Himalaya, an increasing temperature trend was observed, but no significant trend in precipitation (Shrestha et al., 1999; Liu and Chen, 2000; Shrestha et al., 2000; Lutz, 2016). In the HMA region, the snow cover peaks in winter, but in the Karakoram region of the Upper Indus basin the maximum snow cover occurs in the spring, and a negative winter snow cover trend was observed (Immerzeel et al., 2009). In the Karakoram region, summer precipitation is increasing, but glacier and snowmelt contributions to river flow during the summer season are decreasing. Glaciers cover 12,500 km2 of the Karakoram distributed across four sub-basins (Bajracharya et al., 2014). Kraaijenbrink et al. (2017) estimated that by 2099, 64 ± 7% of the ice mass stored in HMA glaciers will remain with a global temperature increase of 1.5°C. Glacier mass loss is greater for Himalaya (−0.32 m w.e. yr–1) than for the Karakoram (ranges from 0.18 to 0.11 m w.e. yr–1) (Lutz, 2016; Brun et al., 2017; Shean et al., 2020). Glaciers in the Karakoram exhibit characteristics of both the “summer accumulation” due to the summer monsoon and the “winter accumulation” due to the winter westerly disturbances (Kapnick et al., 2014; Bashir et al., 2017). The stable or positive rate of glacier mass change in the Karakoram has been associated with trends of increased snow accumulation and attributed to stronger winter westerly disturbances (Forsythe et al., 2017; Smith and Bookhagen, 2018). As a result, the rate of glacier mass change in the Karakoram is neutral or positive. The seasonal changes in river flow pose a threat to downstream economic activities, such as hydropower generation and crop irrigation in the region, because the water demand for hydropower generation and crop irrigation is highest in the spring season.
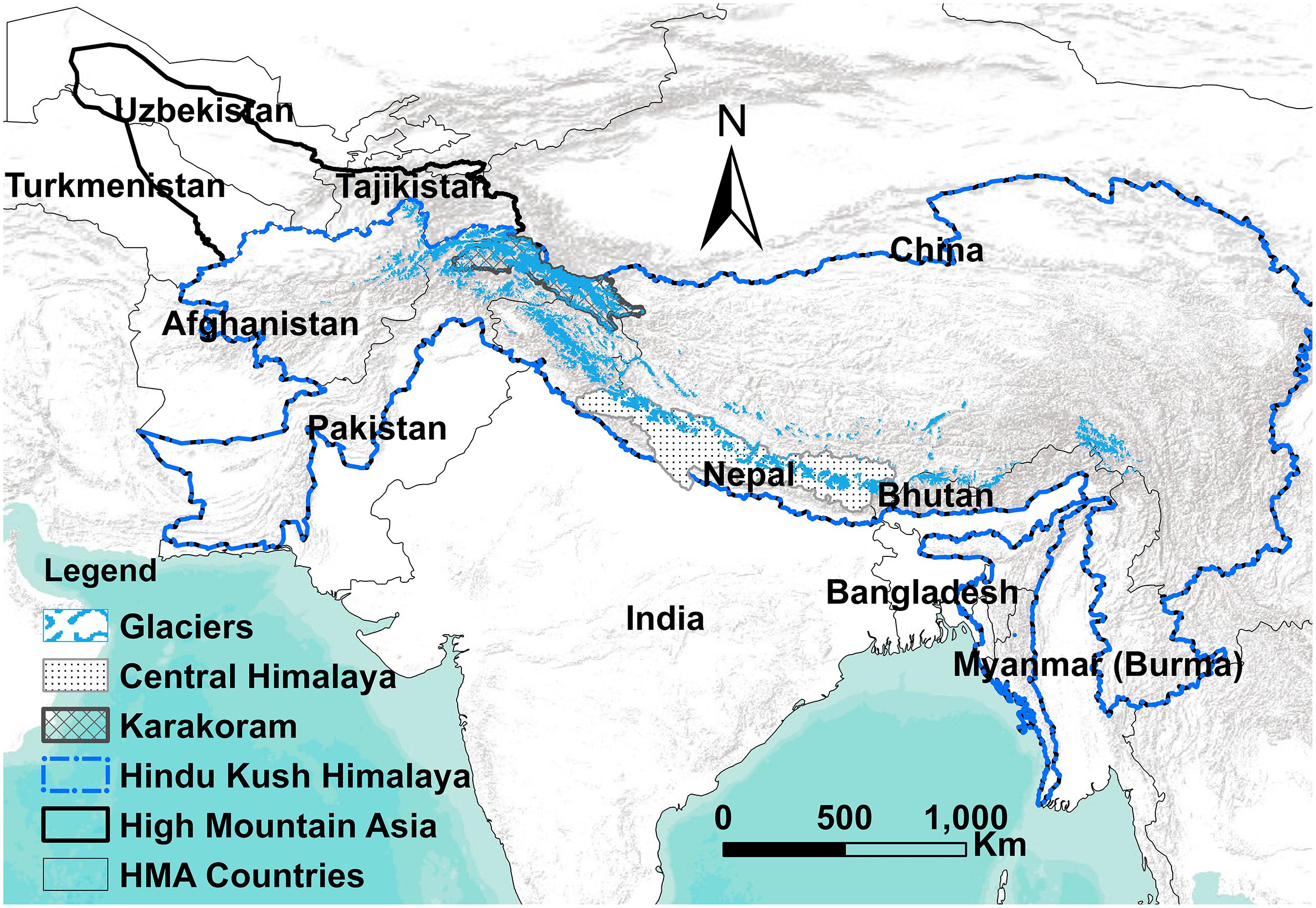
Figure 1. High mountain Asia, Hindu-Kush-Himalaya, Karakoram and Central Himalaya regions. Karakoram region is the northernmost Pakistan, covered by the glaciers and borders Tajikistan in the North.
Nepal and Pakistan are investing in hydropower development to meet the growing demand for electricity. Pakistan’s energy security is considered to be at risk due to the large share of imported fossil fuel in its energy mix (Rehman et al., 2019). Primarily due to supply problems, in 2013 Pakistan typically shed load (cut electricity supply to consumers) up to 8 h per day in urban areas and 16–18 h in rural areas (Khokhar et al., 2015). To achieve energy security, stability, and reliability goals, the government of Pakistan has promoted renewable development in the energy mix (Kessides, 2013). In 2015, 438 MW of hydropower projects were added to the national grid, raising the share of hydropower electricity to 30.4% of the total electricity production in Pakistan (Ministry of Finance, 2013; Ahmed and Suphachalasai, 2014). By 2030, with the completion of 24 hydropower projects, Pakistan is expected to have a total capacity of 42 GW (Water Power Development Authority Report [WAPDA], 2013). The Gilgit-Baltistan (GB) region of the Karakoram is estimated to have a hydropower potential of 19,696 MW (Abbasi et al., 2017). Currently, 126 hydropower plants are operating in the region with an installed capacity of 132 MW. According to the WAPDA GB government, in 2016 the region faced a capacity shortfall of 52 MW in the summer and 173 MW in the winter. WAPDA is working on mega-hydropower projects with a total capacity of 18,720 MW, and 27 of the hydropower projects with a total capacity of 248 MW are in Gilgit-Baltistan region (Abbasi et al., 2017).
In Nepal, where the current electrical generating capacity is less than 1000 MW, the Department of Electricity Development (DED) granted generation licenses to hydropower companies that are expected to develop hydropower capacity of 5,465 MW (Nepal Electricity Authority [NEA], 2015). According to Nepal Electricity Authority [NEA] (2015), the DED has also approved survey licenses for an additional 266 new hydropower plants (6000 MW capacity). It is therefore important to understand how projected changes in climate will affect river flows and the resultant electricity generation. Hydropower developers are deeply concerned about making investments based on limited information about historic hydrologic flow and no data on projected flow under a changing climate.
Earlier studies have focused on climate change impacts on glaciers in larger drainage basins such as the Indus, Ganga, and Brahmaputra basins; however, water resource management-decisions take place at a smaller sub-basin scale. Glaciers melt is estimated to contribute 26% and 3% of total runoff in Indus basin and Ganga Basin respectively (Immerzeel and Bierkens, 2012). A few studies, including Alford and Armstrong (2010), Pradhananga et al. (2014), and Tahir et al. (2015) have looked at hydrological impacts on smaller sub-basins. Tahir et al. (2011) found that (a) a 1% increase in snow cover area in Hunza under constant temperature will increase summer discharge by 0.7%, (b) an increase in 1°C mean temperature (keeping precipitation and snow cover constant) is expected to increase summer discharge by 33%, and (c) a 20% increase in snow cover in combination with a 2–4°C rise in mean temperature is expected to increase future stream flows by 100%.
In the high-altitude Langtang River tributary in the Trishuli basin, glaciers contribute more than 50% of the total annual streamflow (Racoviteanu et al., 2013; Brown et al., 2014). The projected change in river flow in Representative Concentration Pathways (RCP) 4.5 exhibited an increase in flow until 2050 and a slight decrease from 2050 to 2099, while RCP 8.5 projected an increase from 0.03 to 11.10 m3s–1(Mishra et al., 2018; Kayastha and Shrestha, 2019). However, only a few studies, like Mishra et al. (2018), have investigated the potential impacts of climate-led changes in river flow on hydropower generation. Hydropower generation in run-of-the-river hydropower plants (typical in the HMA region) are impacted by low flow during certain seasons including late spring and early summer seasons. Coupled with the high demand and resultant high price (and value) of electricity in those seasons, the impact of low seasonal flow quickly adds up. This work is the first of its kind that compares the impacts of changes in hydrology on hydropower generation in two basins in the HMA region, one located in the Karakoram and the other in Himalaya.
In order to quantify the size and extent of the impacts of river flow changes on hydropower generation in two different regions of HMA, we generated information on hydrologic flow under various climate change scenarios and estimated the economic costs/benefits of climate change, focusing on hydropower value streams. Specifically, we used sixteen general circulation models (GCM)/RCP combinations to explore a range of possible futures in two sub-basins in the Karakoram and Central Himalaya regions. We estimated the hydrologic flow under these climate scenarios using the University of New Hampshire Water Balance Model (WBM). We quantified the impacts on seasonal river flow and translated that into cumulative economic output of the rivers, with a focus on hydroelectricity energy production. An integrated assessment approach (IAA) developed by Mishra et al. (2018) under a NASA High Mountain Asia project was used for the comprehensive assessment and comparison.
Study Area
For this study we compared the westerly dominated snow-and-glacier-fed Hunza sub-basin of the Karakoram region to the monsoon-dominated snow-and-glacier-fed Trishuli sub-basin of the Central Himalayan region (Figure 2). The Hunza sub-basin with a surface area of 13,733 km2 is located in the upper Indus River, with altitudes ranging from 1,415 m to 7,809 m a.s.l. (Figure 2). Sixty-four percent of the sub-basin is at or above an altitude of 4,300 m, 25% of the area is between 3,301 and 4,300 m, and the rest is below 3,300 m (Supplementary Figure S1). The estimated glacier-covered area in the Hunza is 3,840 km2 (RGI Consortium, 2017), which is the second highest area under ice and snow among the eight sub-basins of the upper Indus (Bajracharya and Shrestha, 2011; Baig et al., 2016). In the Trishuli sub-basin, only 603 km2 out of 5,757 km2 total area is glacier-covered. More information on the sub-basins is shown in Supplementary Table S1. The elevation ranges from 411 m to 7,381 m a.s.l., and half of the area has an elevation range between 4,301 m and 7,900 m (Figure 2). In both sub-basins, several small hydropower plants are operational and large hydropower plants are under construction. The impacts of climate change on river flow in Hunza and Trishuli were assessed using comparative methods that measure the relative difference of river channel flow and hydropower production in Naltar IV and Trishuli hydropower plants using WBM output that is driven by climate model projections.
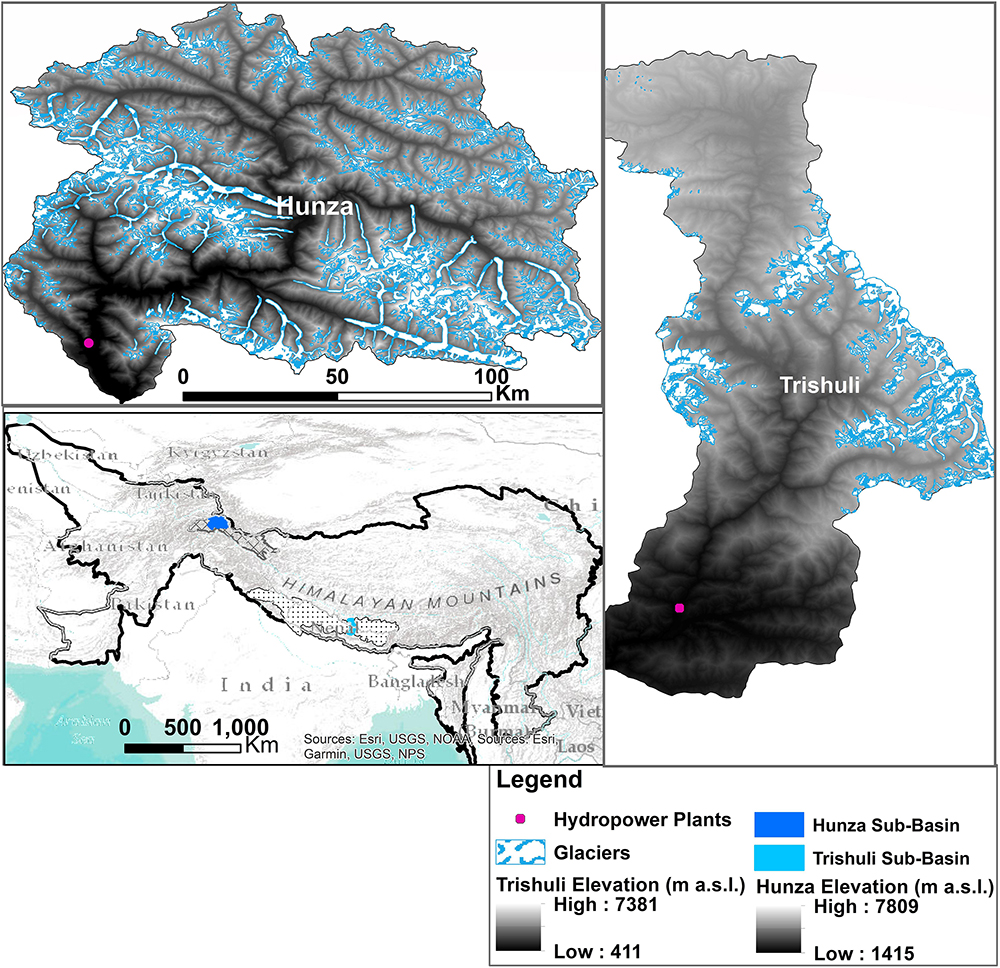
Figure 2. Study areas in HMA region: Hunza sub-basin in Karakoram and Trishuli sub-basin in Central Himalaya showing elevation characteristics and locations of glaciers and hydropower plants (Power plants < 10 MW are not included in the figure).
In order to provide an example of the difference in the impacts of climate change from sub-basin to sub-basin, we selected two hydropower plants of similar generation capacity located in snow and glacier fed rivers in the two HMA sub-basins. Trishuli is a “pure” run-of-river hydropower plant. As Trishuli is a run-of-river plant without a dam for water storage, the flow of water that is diverted into the power plant is at all times equal to the water discharge (i.e., outflow). The timing of the electricity generated from the hydropower is therefore primarily a function of river water inflows and the attributes of the hydropower facility. Naltar IV has a small reservoir that holds enough water to generate electricity at full capacity for approximately 2 h. To a limited extent, the plant can shape hourly water releases during the day such that production is highest during peak load hours. Characteristics of the two power plants are provided in Table 1.
Data and Methods
Based on the IAA developed by Mishra et al. (2018), we coupled the principles of climate science, cryosphere sciences, hydrology, and hydropower engineering with economic analysis to analyze and compare the economic impacts of climate change in the two sub-basins, as illustrated in Figure 3.
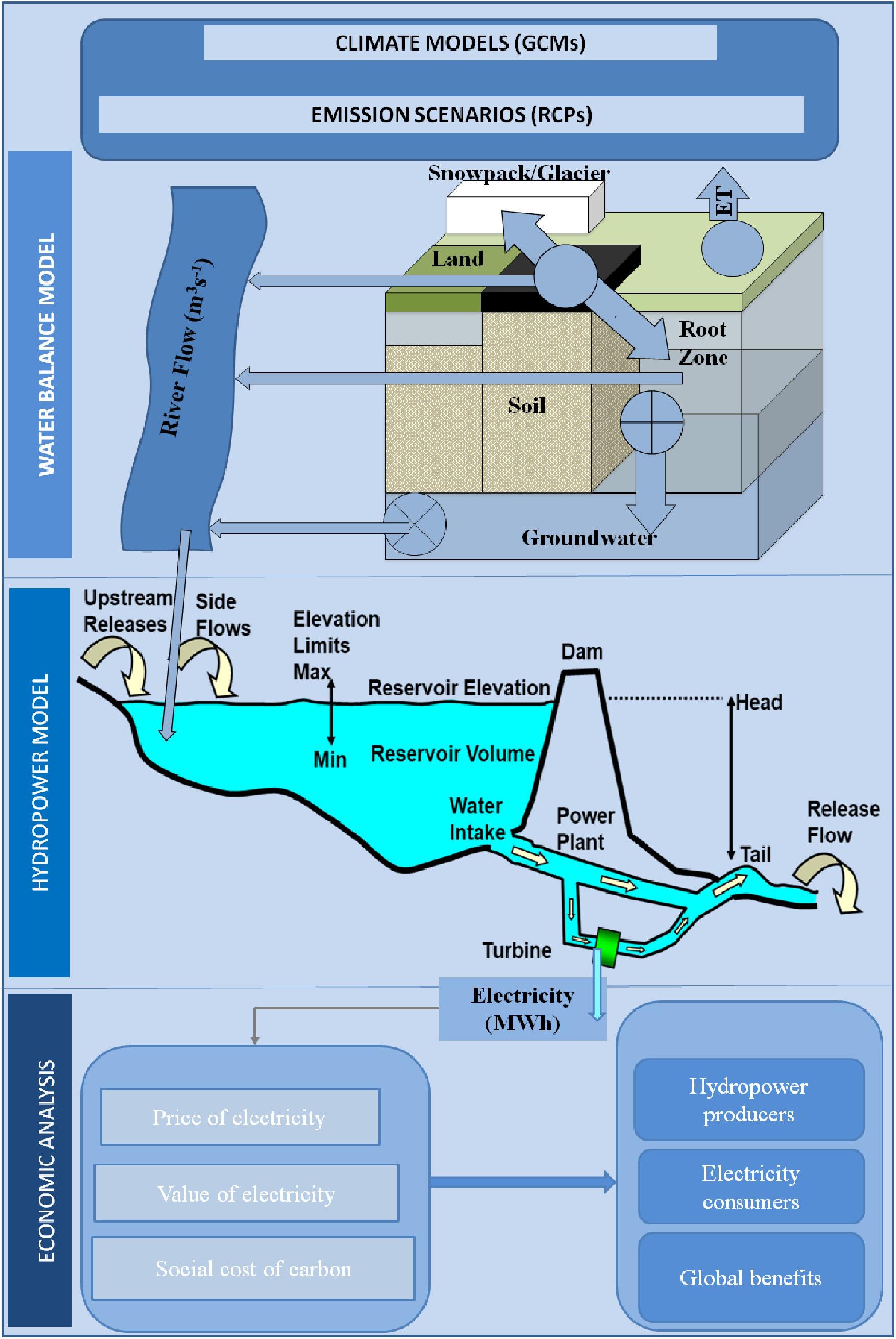
Figure 3. Major components of the coupled water balance model, hydropower systems model and economic analysis.
Water Balance Model (WBM)
The University of New Hampshire WBM is a process-based, spatially distributed, gridded model incorporating the major elements of the hydrological cycle. Full model details can be found in Grogan (2016). WBM simulates both the vertical water exchange between the atmosphere and land surface and horizontal water transport, through both land surface runoff and via the river network. Both natural and human processes, such as evapotranspiration, snowpack development and melt, glacier melt, river flow, and river impoundments from dams and diversions, are included in the model. Snowpack is based on a sub-grid approach using elevation bands (Lammers et al., 1997; Hartman et al., 1999) of 200 m to account for the strong vertical temperature gradients in this region. The model was run for all GCM in all historical and RCP combinations, at daily time steps, at a spatial granularity of 0.8 × 0.8 km grid cells for the entirety of the Hunza and Trishuli basins. Daily river flow was output from the model, and these fields were sampled at the hydropower stations locations.
WBM Configuration
Two separate spatial domains for the Nepal and Karakoram regions were used to run WBM. Both domains were subsets of a digital river network representing surface flow direction clipped from the HydroSHEDS 30 arc-second (approximately 0.8 km at these latitudes) resolution dataset for Asia (Lehner and Grill, 2013) downloaded from the USGS HydroSHEDS web site (HydroSHEDS, 2019). This network resolution allowed for a sufficient allocation of land features to represent all relevant hydrological processes in the catchment area of the studied sites listed in Table 1. Flow impediments, such as managed reservoirs, have been included (Nepal domain only) from the Global Reservoir and Dam database (GRanD) (Lehner et al., 2011), but no water diversions for irrigation or other human demands (such as domestic, industrial, livestock) have been accounted for, due to limited agricultural hydro-infrastructure development in these high mountain regions. Because climate input variables from the CMIP5 collection (see below) are limited, evapotranspiration was simulated using the Hamon method (Hamon, 1961). We use Hamon because of its simplicity and because it has the smallest bias over a range of climate zones (Federer et al., 1996). Deep groundwater aquifers have not been included in the WBM model setup for this study because information and input data layers for these regions are not available.
The elevation grid for the WBM run-time climate downscaling is based on 30 m resolution ASTER DEM v.2 dataset (Tachikawa et al., 2011). A temperature lapse rate of 6.4°C km–1 (Rennick, 1977) is applied at a sub-grid cell resolution to represent elevation gradients within the high-resolution grid cell. This sub-grid cell elevation correction is essential for representing snow accumulation and melt in high elevation gradient regions All other land cover, soil properties, and basic input layers required to run WBM were taken from Global 30 and 6 arc-minute resolution datasets used in previous global hydrological studies (Vörösmarty et al., 2000; Lammers et al., 2001; Wisser et al., 2010; Liu et al., 2017).
Climate Drivers
Monthly temperature and precipitation are used to drive WBM simulations. The ERA-Interim climate reanalysis dataset (Dee et al., 2011) is used for historical simulations, and CMIP5 GCMs are used for future simulations (Table 2). The spatial resolution of both ERA-Interim and CMIP5 climate drivers are too coarse for this study’s domain, due to the steep elevation gradients. To address this issue, two spatial downscaling steps were applied to increase the resolution. First, a bi-linear interpolation was applied to both the temperature and precipitation fields, resulting in a higher-resolution grid scale matching WBM’s river network resolution. Second, due to the high elevation gradients in the study domain, an elevation-based correction was applied to temperature at the high-resolution grid scale. A lapse rate of 6.4°C km–1 (Rennick, 1977) was applied based on the elevation difference between the high-resolution river network and the lower-resolution geopotential layer of the climate driver, resulting in elevation-based temperature variation at sub-climate driver resolution. In addition to downscaling, we also applied the delta-change bias correction method to the GCM climate drivers for future simulations by applying, on a pixel level, the monthly climatology difference between the ERA-Interim and corresponding GCM fields. While WBM represents monthly river flows, it makes use of sub-monthly variability in precipitation. For precipitation, the delta change was applied to a constant daily variability over each month, using year 2001 values, which represents the fewest precipitation extremes (Fekete et al., 2004). Temporal downscaling of temperature does not have a significant effect on the monthly model output used for all analyses in this paper, so a constant monthly temperature was used by WBM to project future water flow rates. Spatial variability, through snowpack formation and melt, is important, and this is discussed in section “Water Balance Model (WBM).”
Integrated Glacier Runoff
WBM used output data from the Python Glacier Evolution Model (PyGEM) developed at the University of Alaska at Fairbanks (Rounce et al., 2020; in press). PyGEM estimated the mass balance of every glacier using 10 m elevation bins and a monthly time step. The model was forced with air temperature and precipitation data from the same climate drivers as WBM (Table 2), and computed glacier melt using a degree-day model, accumulation based on a temperature threshold, and refreezing based on mean annual air temperature. Glacier retreat/advance was modeled using mass redistribution curves from Huss and Hock (2015). The model provided glacier runoff, glacier volume, glacier area and other point data variables in the study basins for each glacier in the RGI Version 6.0 database (RGI Consortium, 2017). PyGEM output was rasterized for the study area using the same spatial grid as the river network. Given the monthly temporal resolution of the PyGEM output, no daily temporal downscaling was applied. For each 1 × 1 km grid cell in WBM, glacier outflow was summed and provided as an input to the river system. Precipitation from the GCMs proportional to the glacier area was removed from WBM input precipitation fields to avoid double counting precipitation over the glaciers, because the glacier runoff produced by PyGEM accounts for precipitation, snow accumulation and melt, glacier melt, and refreezing. Hence, all PyGEM outputs (including the water entering the glaciated area as precipitation) are passed to WBM as an input (Rounce et al., 2020, in press).
River Network and Topography
Digital river networks were used to establish horizontal connectivity of the land surface within each drainage basin. Elevation for the snow bands was derived from the 30 m ASTER GDEM v.2 dataset (Tachikawa et al., 2011). Soil properties required to simulate runoff generation in WBM were from the Harmonized World Soil Database v1.2 (Fischer et al., 2008). Data used for calibration and validation included hydrologic flow observation obtained from power plant and stream gauges located at Naltar and Trishuli river basins, from WAPDA, Pakistan and Department of Hydrology and Meteorology Nepal respectively.
The WBM model output provides local runoff, streamflow (river discharge), and primary water source components (fractions) in surface flows and storage. The latter allows tracking of the glacier melt, snowmelt, and rainwater components downstream from the originating runoff grid cells. The component tracking is critical in understanding the streamflow changes over the century in the regions with substantial presence of cryospheric processes, e.g., glacier and snow dynamics.
Hydropower Systems Model (HSM)
To gain a better understanding of the potential impacts of future climates on hydropower operations in the Trishuli and Hunza sub-basins, the hydropower systems model (HSM) was used to estimate daily energy production, in megawatt hours (MWh), between January 1, 2020, and December 31, 2099, inclusive. More specifically, we modeled and analyzed the Trishuli hydropower plant located in the Trishuli sub-basin and the Naltar-IV hydropower plant in the Naltar sub-basin of the Hunza basin.
Because the characteristics of these two hydropower plants differ, and the available data describing the plants and their historical operations are dissimilar, the HSM was customized for each application. Both models take into account power plant capacity and generator unit availability, the efficiency of converting flowing water to electricity and other plant-specific characteristics presented in Table 1. The WBM model water discharge results are a key HSM driver/predictor of daily hydropower production for both power plants. In addition, at both Trishuli and Naltar we modeled water-diversion structures and the routing of water through power plant turbines and flows that circumvent the power plant (non-power water flows). Using historical power plant inflows and generation data, the water-to-power conversion factor used for this study was set to 0.32 MW per cubic-meter per second (MW cms–1). We computed the maximum turbine flow rate using this power conversion factor and the capacity of the plant. Trishuli inflows estimated by WBM that are in excess of this maximum turbine flow rate are assumed to be non-power releases (water diverted around plant). Daily generation levels were simulated for each plant for 16 GCM/RCP combinations.
For computational efficiency, we used a simulation model that projects daily unit-level generation levels under a large number of unit on/off states, in which a randomly drawn “off” state represents a unit outage. On and off states were estimated for each day of the 2020–2099 time period using a random number generator. Various random trials were modeled, all of which had similar results. For consistency among RCP 4.5 and RCP 8.5 HSM runs, all model runs used the same set of random draws. The selected outage set was nearly identical to the target outage.
The representation of Naltar in the HSM is based primarily on information supplied by the WAPDA and turbine efficiency surface for Pelton turbines.1 Modeled power generation at Naltar uses WBM daily simulated water discharges and an adjusted power conversion efficiency surface. Because the hydraulic head is much higher at Naltar than Trishuli, Naltar turbines typically generate more than 10 times the amount of electricity per volume of turbine water release than the Trishuli hydropower plant. Unlike at the Trishuli complex, the water diversion structure that is located upstream of Naltar is capable of redirecting virtually all of the Naltar River to the channel that connects the river to the reservoir.
The HSM also accounts for plant outages and idle hours.2 These include outages for scheduled maintenance that occur during the months of February, June, September, and December. Based on historical outage levels, HSM assumed that each unit would be available 90% of the time for Trishuli. Historically, each unit is on average “idle” approximately 90 min per day.
Economic Analysis Model
The economic impacts of climate change are estimated as the change in welfare or the welfare-equivalent income loss (Tol, 2018). While no estimate of the economic impact of climate change is perfect (Pindyck, 2013; Tol, 2018), we attempted to use a method consistently across the two geographic domains to compare and contrast the climate change impacts on hydropower plants located in the HMA regions. We estimated the changes in economic values for Naltar under RCP 4.5 and RCP 8.5 scenarios and compared the changes in economic values with those for Trishuli.
The change in welfare associated with hydropower generation is the sum of the change in the benefits to producers and consumers (residential, commercial and industrial) of the electricity produced at the plant. The hydropower owner/manager’s objective is represented as the following profit maximization function:
where π is the profit from electricity generation, Pe is the price of electricity, Qeis the quantity of electricity generated (which is a function of the amount of water available qwtattimet, water to power conversion factor μ, operational efficiency factor σ, head of reservoir, Ht at time t) (Hirsch et al., 2014). The cost Cis the total cost of electricity production. Cost is mostly independent of the actual operation, because employees will be paid regardless of hourly input, and water is a common property and a free resource. Therefore, cost is eliminated from profit maximization.
Both state-owned and private power producers have power purchase agreements (PPAs) with the central regulatory authority (Nepal Electricity Authority [NEA] and Water and Power development authority Report [WAPDA], 2013) respectively. A provision is made in the PPAs to allow a price difference during the dry months (December to March) and wet months (April to November) of the year (for Nepal, for example, current prices are 7.4 ȼ per KWh in dry months and 4.2 ȼ in wet months). A change in water flow in February or March will have a higher impact on the producers than an equal change in flow in wetter months.
The change in profits for each producer due to changes in water availability is estimated by applying the chain rule to the (Equation 1):
where is the change in quantity of electricity generation as a result of change in water availability.
Using equation three below, we estimated the total value of the impact of climate-led change in water availability for the hydropower producers as the marginal change in profit resulting from a change in seasonal water availability.
The total value of the impact on electricity consumers is estimated as the change in total benefit from electricity usage, or consumers’ surplus. In Nepal, 95% of the total consumers of electricity are domestic (Nepal Electricity Authority [NEA], 2012). Rural households use electricity for lighting, watching television, and in some cases for cooking and refrigeration. Farmers with larger farms and more capital use electricity for farming activities as well. However, large farms in rural mountain regions are few and cannot be considered representative. Due to the lack of factories and industries in these rural regions, households act as the production units that use electricity and the consumption units that consume the intermediary or final outputs.
The benefits of electricity consumption to the rural population include increased working hours due to lighting provided by electrical appliances, improved information through television programs, educational benefits due to increased study time for students and improved school facilities, improved health due to reduced indoor pollution, and reduced postharvest crop loss with the availability of refrigeration facilities. The population also benefits from higher quality healthcare facilities due to refrigeration and other facilities that are possible only with a continuous supply of electricity.
For this paper, without established collaboration, it was difficult to collect all the data required to run an econometric model to estimate the consumers’ surplus of electricity. The price of electricity in Nepal and Pakistan is regulated by a central authority. In Nepal, the Electricity Tariff Fixation Committee fixes the price of electricity. The National Electric Power Regulatory Authority (NEPRA) of Pakistan fixes the price in Pakistan. Prices have been changed only two times in the past twenty years in Nepal, although demand is projected to grow at an annual rate of 8.34% and is expected to exceed 17,400 GWh by 2027 — about four times the current demand of 4,430 GWh (Nepal Electricity Authority [NEA], 2012). At the same time, electricity supply increased from less than 1,000 to 5,000 MW within a decade, and additional 5,000-MW-capacity power plants are likely to contribute energy production to the power grid in the next decade. While the marginal increase in electricity demand attributed to climate change is likely to further increase under the high-emission scenario, projecting the demand, price, and consumer surplus under various climate scenarios is an exercise that falls outside the scope of this study. Because our objective is to compare climate change impacts using a consistent method, we estimated the consumer surplus per unit of electricity consumption using a benefit transfer method. The World Bank Report (2008) developed a method and analyzed the benefits of rural electrification from a large number of projects implemented in developing countries from across the world, including Nepal and Pakistan. The estimated benefit of electricity to provide lighting and television services ranged from $0.20 to $0.60 per kWh in developing countries.
In addition, we estimated the benefits to people globally from carbon dioxide emissions displaced by the hydropower plants. That is, electricity from these hydropower plants displaces the energy supply from other sources, such as fossil fuel and biomass, so electricity translates into displacement of carbon dioxide emissions. The avoided social cost of carbon provides global benefits in addition to the local benefits discussed earlier. Carbon prices estimated by the High-Level Commission on Carbon Prices (Stiglitz and Stern, 2017) were used for the benefit transfer. The report recommends using a low and high estimate of carbon price of US$40–$80 per ton CO2 by 2020 and increasing to $50–$100, $110–$130, and $130–$160 by 2030, 2040, and 2050 respectively. We used the recommended price per ton CO2 for 2020 to derive the value of carbon dioxide displacement per KWh and used that for our further analysis.
We used the benefits of electricity estimated by the World Bank Report (2008) adjusted to 2017 USD using consumer price index (CPI) data (World Bank) for Nepal and Pakistan. The values used to estimate the benefits from electricity are $0.8 to $2.5 per kWh for Nepal and $0.4 to $1.2 per kWh for Pakistan. The change in value associated with increased electricity demand and prices, corresponding to changes in temperature, economic prosperity, mechanization, urbanization, and industrialization, is not incorporated in this study due to unavailability of data.
We estimated and compared the change in electricity value for the months that were identified as the most affected by climate change, as well as for the annual and decadal periods, to compare the long-term impacts of the two hydropower plants in the two countries. We derived a set of discounting factors required to estimate discounted present value. Based on the GDP growth rates for the past ten years (2008 to 2017) of the two countries, the average discount rates used were 9.1% for Nepal and 7.5% for Pakistan. Because projects with environmental benefits, such as benefits from carbon dioxide emissions reduction, should use a lower discount rate, we also estimated the change in value for each basin using a discount rate of 3% for the benefits associated with displaced CO2 emission. We then compared the impacts of climate change on the economic value of electricity generated at the Naltar and Trishuli hydropower plants.
Results
Hydrologic Flow Estimation and Future Estimates
We estimated historic river flow using the WBM model, validated the results, and projected flows to the end of the century for 16 GCM/RCP scenarios. The observed monthly discharge from 2000 to 2010 for the Trishuli hydropower plant site (Department of Hydrology and Meteorology, Nepal) was used to validate WBM results. A Nash-Sutcliffe coefficient (NSC; Nash and Sutcliffe, 1970) of 0.78 between the observed and modeled streamflow indicates a very good match (Figure 4).
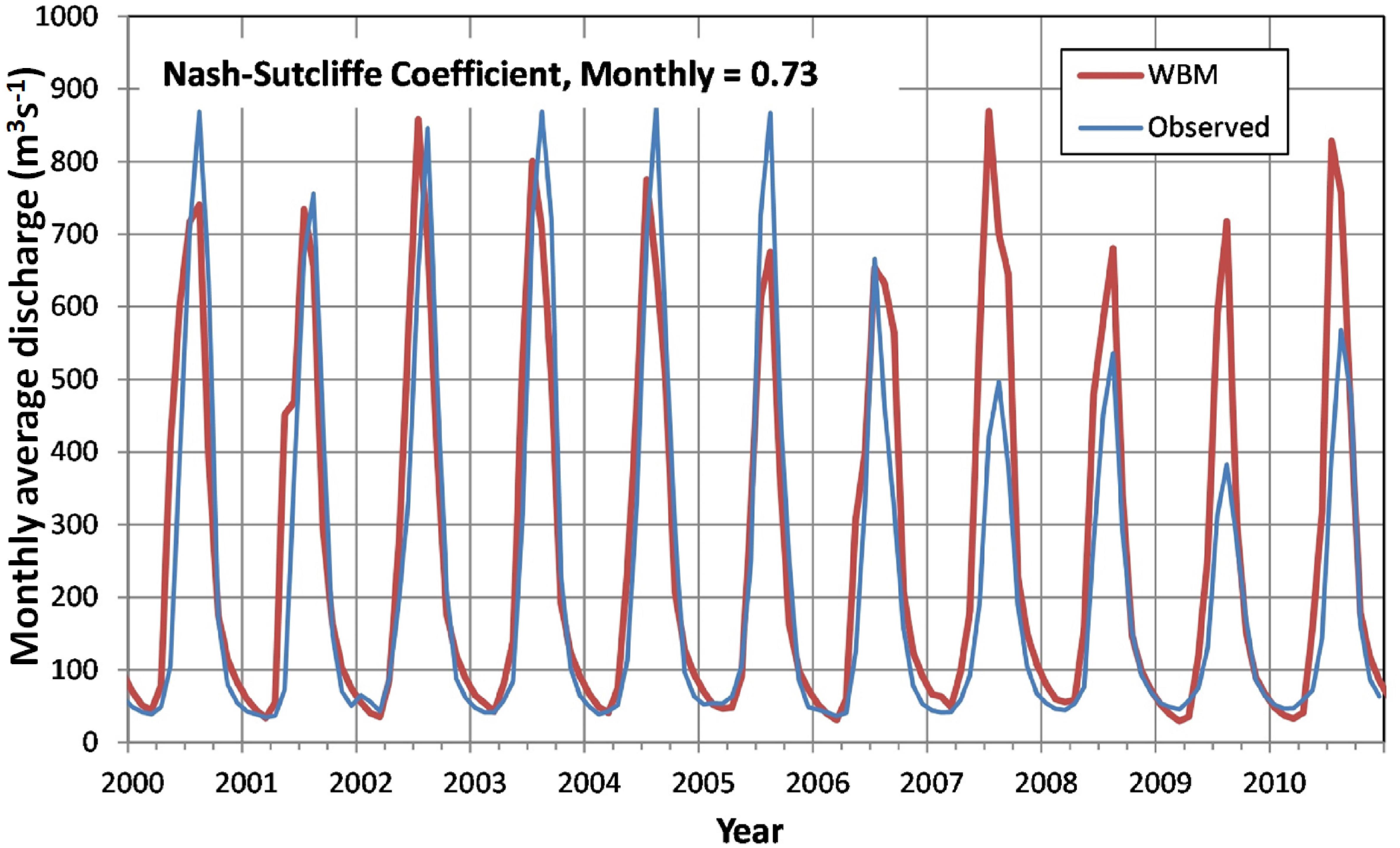
Figure 4. Comparison of observed river discharge to water balance model values at the Trishuli site.
Despite the good match to the NSC metric, seasonal differences between observed and modeled discharge were observed. Summertime high flows deviated from observations by 10–35%, but it is more important for hydro-power generation capacity limitations that the winter and early spring low flows match well with the observation. The deviations of high flows are controlled by the quality of the input climate driver (ERA-Interim), which has a coarse spatial resolution and a limited set of variables (Table 2). Also, it is known that gauging high flows at a river reach is often difficult to calibrate, which may lead to significant observation errors (Shiklomanov et al., 2006).
Analysis of WBM Streamflow for 21st Century Projections
The results show different projected seasonal variability of discharge in the Karakoram and Central Himalaya (Nepal) study sites. In addition to seasonal change, we present variability and trends of projected annual, decadal, mid-century and end of century hydrological regimes in these two sub-basins (see Supplementary Tables S2, S3 for Mann-Kendall test and Sen’s slope analysis results). In most GCM/RCP combinations, the summer discharge initially tends to increase by about 10% at the mid-century for both site, and then experience a sharper decline — by about 50% for Karakoram site — toward the end of the 21st century (Figure 5).
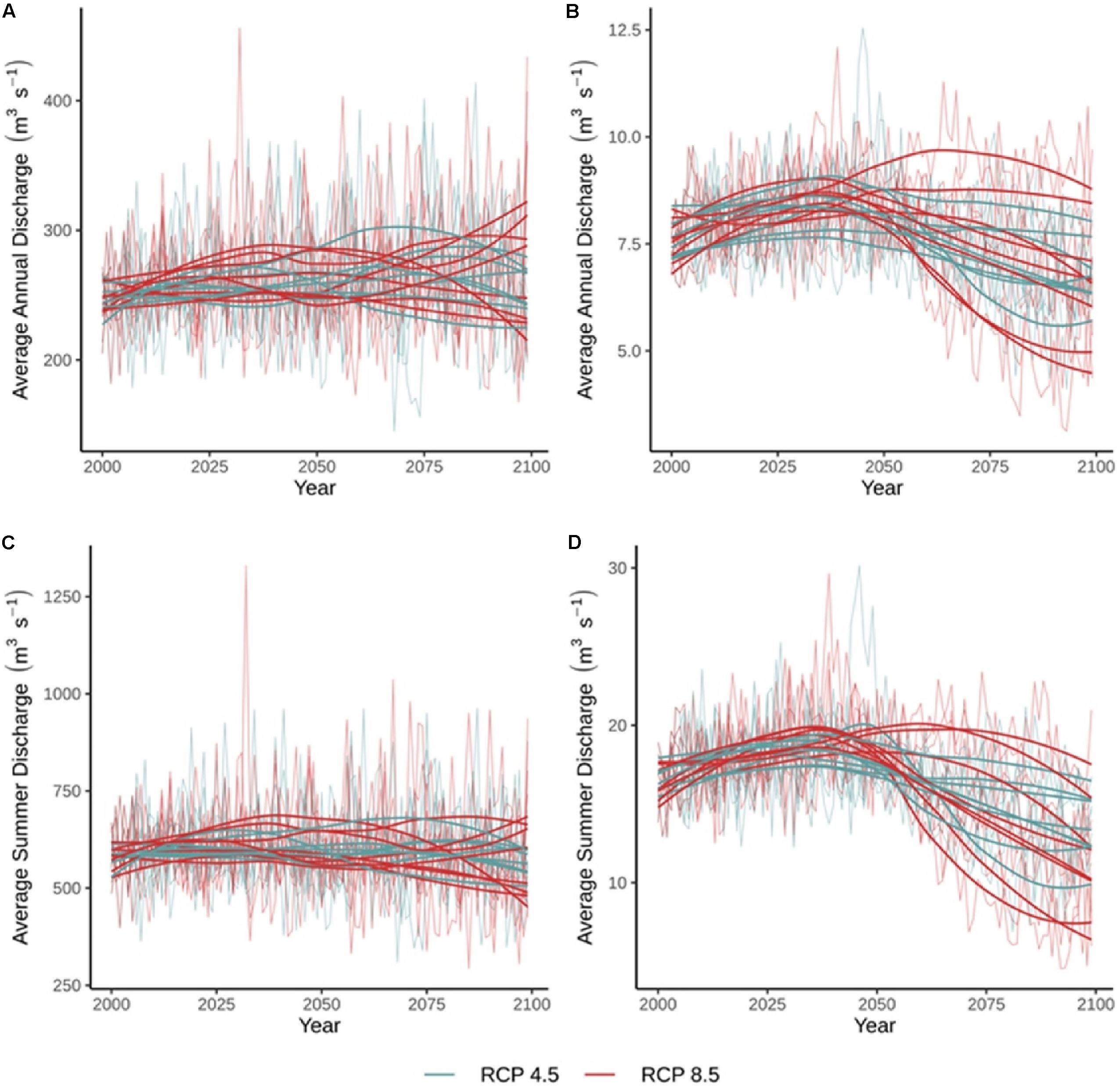
Figure 5. Simulated river discharge for each GCM/RCP are as thin lines, and a non-parametric loess smoothing is shown as thick lines for (A) average annual river discharge at Trishuli, (B) average annual river discharge at Naltar, (C) average summer (JJA) discharge at Trishuli, and (D) average summer (JJA) discharge at Naltar. For all panels, RCP 4.5 is shown in blue and RCP 8.5 is shown in red.
Annual changes are primarily driven by changes in spring and early summer flows (April to June for the Karakoram, and March to May for Nepal). These increase significantly (over 90.4 and 32.6% respectively for the Karakoram and Nepal sites) by the middle of the 21st century (Tables 3, 4), due to temperature-enhanced snow and glacier melt, until the time of peak-water (Supplementary Figure S5), which occurs in 2050–2080 in Karakoram and 2035–2050 in Nepal. Toward the end of the century, the model predicts a moderate decline in streamflow (3.6% in the Karakoram and 5.3% in Nepal) during the summer and fall seasons (July to August for the Karakoram, and June to September for Nepal), compared to contemporary hydrology represented by WBM simulations for ERA-Interim (2000–2017) climate drivers (Tables 3, 4).
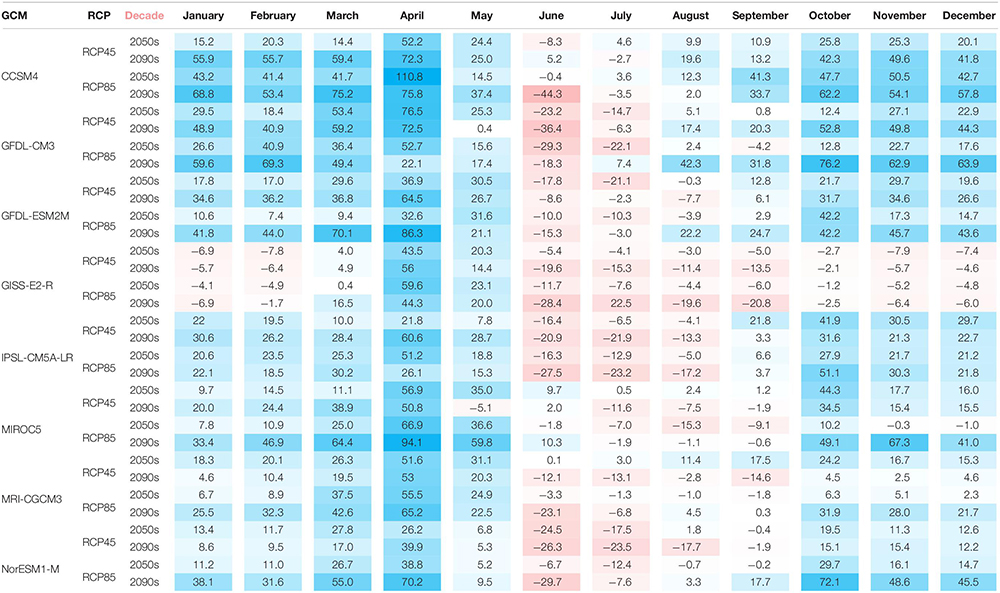
Table 3. Trishuli monthly streamflow changes by CMIP5 climate as compared to contemporary flows by ERA-Interim, in %.
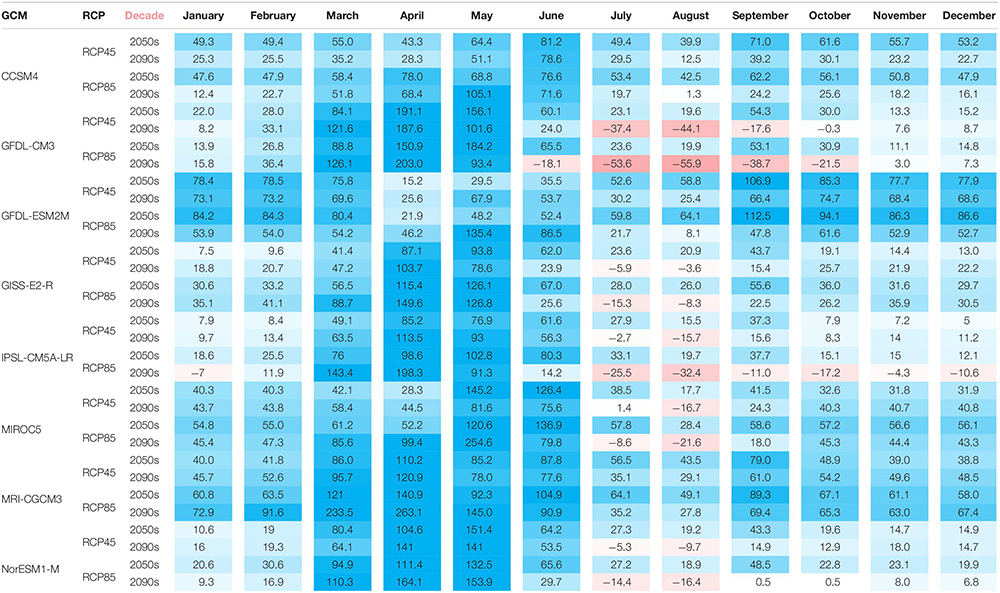
Table 4. Naltar monthly streamflow changes by CMIP5 climate as compared to contemporary flows by ERA-Interim, in %.
We found that decline in the end-of-century average annual flow was three times larger for Naltar (35% drop) than for Trishuli (10% drop). While no significant differences were observed between river flow trend lines between the RCP 4.5 and RCP 8.5 scenarios, the latter has higher intra-annual variability and divergence over time.
In addition to the above-described seasonal low volumes, the timing of glacier runoff and snowmelt changes greatly thereby affecting the seasonality and inter-annual variability of river flow at both study sites. The onset of the snow and glacier melt season shifts from May to March-April for both sites. However, winter flows in the Nepal region increase by 20–40% due to sporadic snow melt events caused by warm weather waves. That is not predicted for the Karakoram winter flows.
The decline of glaciers and snow storage upstream of the hydropower plants is quite evident (Supplementary Figures S3, S4). Our analysis shows the steepest glacier mass loss to occur at mid-century, around 2035–2050 in Nepal and 2050–2070 in the Karakoram. The rate of glacier mass loss (Supplementary Figure S2) can be directly translated to an additional discharge contribution. This comparison allows us to conclude that the rate of core mass loss yields about 5% and 10% additional high-flow seasonal discharge for the Trishuli and Naltar sites, respectively.
In addition to the shifts in snow accumulation, melt seasons and glacier runoff evolution, changes in precipitation patterns and volumes will influence river flows over the 21st century. A moderate to strong decline in annual precipitation is projected toward the end of the century in most of the GCM/RCP combinations at both Naltar and Trishuli. This directly impacts runoff and discharge volumes, along with the seasonality shifts due to changes in snow accumulation, melting seasons and the intensity of glacier runoff in the summer. However, some GCMs, e.g., GFDL-CM3, project an increased precipitation that leads to increased monsoon runoff in Trishuli. In addition, the projected late start of snow accumulation, coupled with increased monsoon rainfall, translates to much higher (about 25%) late summer and fall river flow in Trishuli (Supplementary Figure S5).
Details of the inter-annual changes in discharge during the 21st century for both regions are illustrated in Figure 6. Estimated changes in spring (March to June) river flow were found to be the largest compared to other seasons. Discharge almost doubles in Trishuli, while at Naltar the estimated increase was almost an order of magnitude higher than observed flows (Tables 3, 4, most GCMs with RCP 8.5). The source of water during the spring months was observed to be dominated by significantly higher volumes of snowmelt in RCP 8.5 as compared to RCP 4.5 (Figure 7). Summer (June to August) seasonal streamflow trends exhibited changes over the century (Figure 6). In Naltar, we observed an increase in flows from 15 to 19 m3 s–1 in 2060 and by the end of the century flow decreased to 4–18 m3 s–1. We also observed an increase in variability from 7 m3 s–1 in 2060 to 16 m3 s–1 by the end of the century corresponding to about 25 and 70% of the mean discharge. During the mid-summer months, lower river flow was seen in RCP 8.5 (Figure 6). From the standpoint of water availability for hydropower the most important flows are the hydrograph changes during the winter when streamflow values fall below plant capacity (see section “Hydroelectricity generation model results” below). Our study indicates a significant increase of winter flows from 2018 to 2100 for both sites, specifically by 25.0 and 46.4% for Trishuli and Naltar sites respectively (Figure 7).
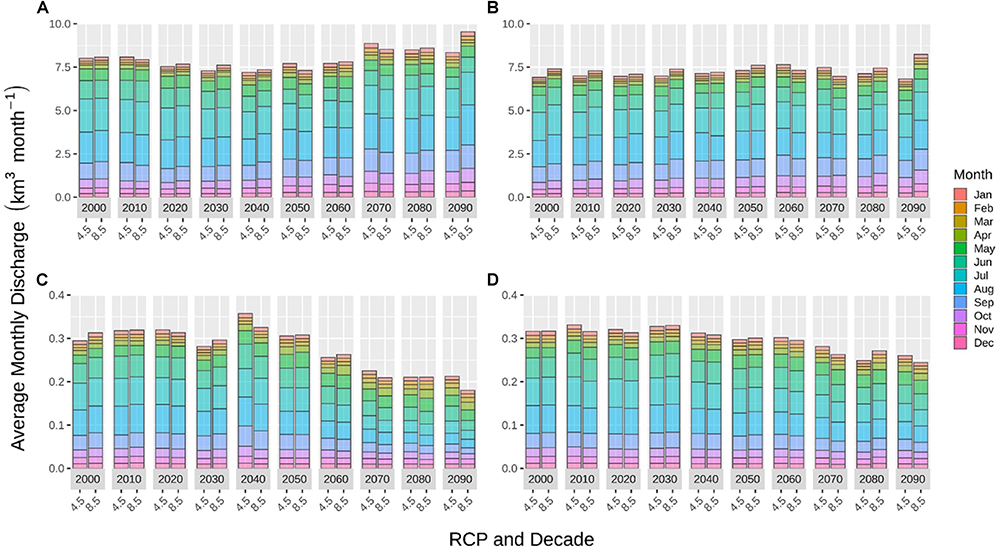
Figure 6. Decadal discharge volume by month for two GCMs (GFDL-CM3 and NorESM1-M) and two RCPs (RCP 4.5 and RCP 8.5) from year 2000 to 2099. The upper panel bar graphs (A) and (B) represent Trishuli site under climate models GFDL-CM3 and NorESM1-M respectively and the lower panel (C,D) represent Naltar site under GFDL-CM3 and NorESM1-M respectively.
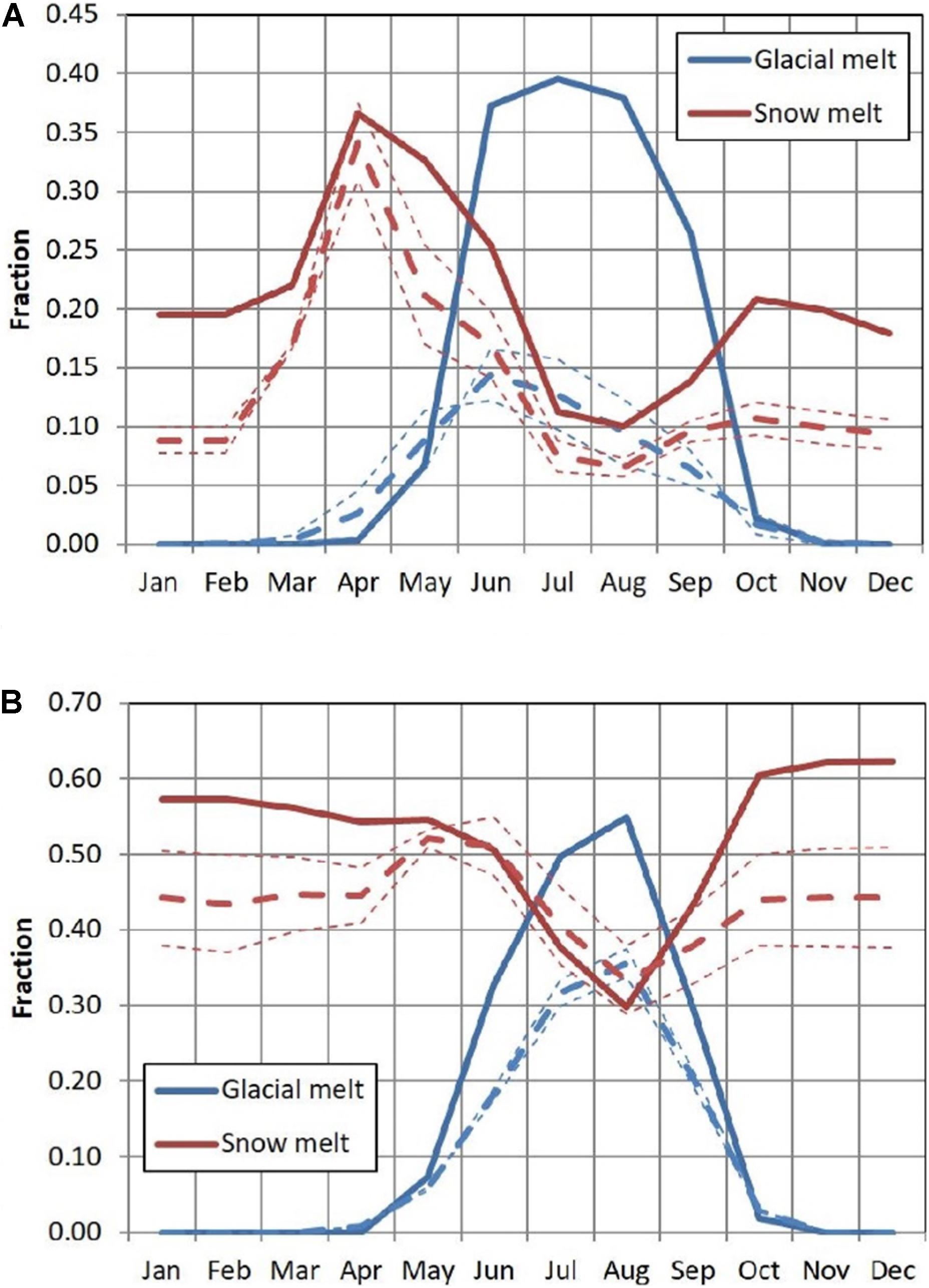
Figure 7. Average monthly snow and glacial melt fractions in discharge for Trishuli (A) and Naltar (B) sites. Solid line is for historical (2000–2017) and dashed line is for average combined future climates (2018–2100). Thick dashed line is all GCM/RCP combinations, top thin dashed line for all RCP 8.5 and bottom thin dashed line for RCP 4.5 scenarios. Where the sum of snow melt and glacier melt fractions is less than one, the remainder is composed of rain water.
Source water analysis, which identifies the rain, glacier melt, and snow melt discharge fractions, is presented in Figure 7. At Trushuli, snow melt accounts for 30% of river discharge from April through June, then declines to 10–12% for the rest of the year, with the lowest contribution (∼9%) occurring in August through September. Under both RCP scenarios, the pattern of snow melt contribution is similar at Trishuli, but the decline from 30% to 10% occurs more rapidly, and the lowest fraction is 5%. Glacier melt at Trishuli is out of phase with snow melt, providing water later in the season. Glacier melt contributes 0% to flows from November through April, but then increases rapidly from 0 to 30% between April and July, and remains around 30% through August, declining back to 0% by November. Under all RCP scenarios, glacier contributions decline significantly, with maximum contributions in July at 18–21%. At Naltar, snowmelt contributes more to flow than at Trishuli through most of the year; snow melt fractions are 50–58% from October through June historically, and decline to ∼32% in the late summer months. This large contribution is due to the dominant presence of snowmelt in groundwater storage, which returns to the surface flows through baseflow. This pattern continues in the future under both RCPs, though with a reduced amplitude. The maximum contribution is reduced to 40–52%, while the minimum contribution is increased to 40–45%. The glacier melt fraction for the Naltar site shows a much shorter summer melt season than Trishuli, with a greater maximum contribution (50%). As in Trishuli, glacier melt contributions at Naltar are projected to decrease during the summer melt season, declining from 50% to ∼25% in July and August.
Comparison of contemporary and future component fractions indicate (a) an increase of glacier runoff at mid-century followed by sharp decline, (b) the snowmelt component declines in all simulations, and (c) there was an increase of total precipitation under some climate models (Figure 7). The recession of glaciers and snow storage in the catchment area above the study hydropower plant locations is observed in Supplementary Figure S2. Peak glacier melt (peak-water) occurs at mid 21st century, caused by accelerated glacier mass loss due to climate change, and is followed by a sharp decline as glacier volume becomes depleted (Supplementary Figure S3). Snow melt, as a primary component of streamflow especially at the Naltar site (Supplementary Figure S4B), also reflects the impact of climate change. Unlike glacier waters, the snow component does not have a peak and gradually declines toward the end of the century (Figure 7).
Hydroelectricity Generation Model Results
Electricity Generation
We compared the observed monthly electricity generation levels with the HSM computed hydropower generation using the WBM streamflow rates for the 2000 through 2010 historical period. The HSM results were similar to historical average generation at both Naltar and Trishuli (Figure 8). At Trishuli, the modeled generation is slightly lower than observed levels from March through June and is higher during the late winter and early spring. For Naltar, the modeled generation levels are noticeably lower than the historical average from February through June. During the summer high flow months, both Trishuli and Naltar generation levels are largely unaffected by WBM underestimates, because during these periods water flow rates are above the maximum turbine rate a vast majority of the time (see Supplementary Figure S6 for more details).
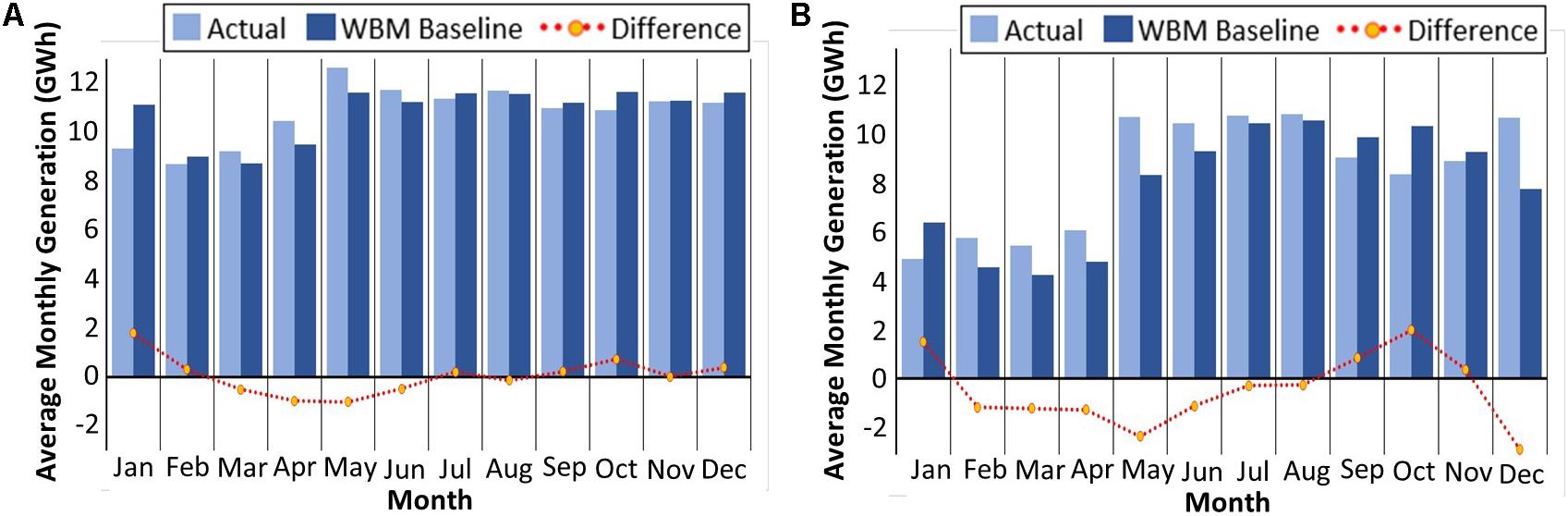
Figure 8. Comparison of historical average monthly generation and hydropower model simulation results for Trishuli (A) and Naltar (B).
Projection of Generation
Projections are based on (a) simulations that used WBM streamflow driven by all 16 GCM/RCP model combinations and (b) potential alternative hydropower plant development in the two basins. Figure 9 shows projected hydropower production, assuming that no changes will be made to Trishuli and Naltar and future hydropower resource development above the plants will be pure ROR resources. The estimated changes in annual electricity generation over the 80 years study period (trend lines) over all climate models range from a 6.2% increase to a 4.7% decrease for Trishuli (Figure 9A) and from a 7.5 increase to an 8.4% decrease for Naltar (Figure 9B). The average change in generation over all climate models is expected to be less than 2 percent (Figures 9C,D). Annual variability in electricity generation was found to be about ± 7% in both RCP 4.5 and RCP 8.5 for Trishuli, while a maximum deviation of about ± 25% is projected for Naltar (Supplementary Figure S7 shows more details).
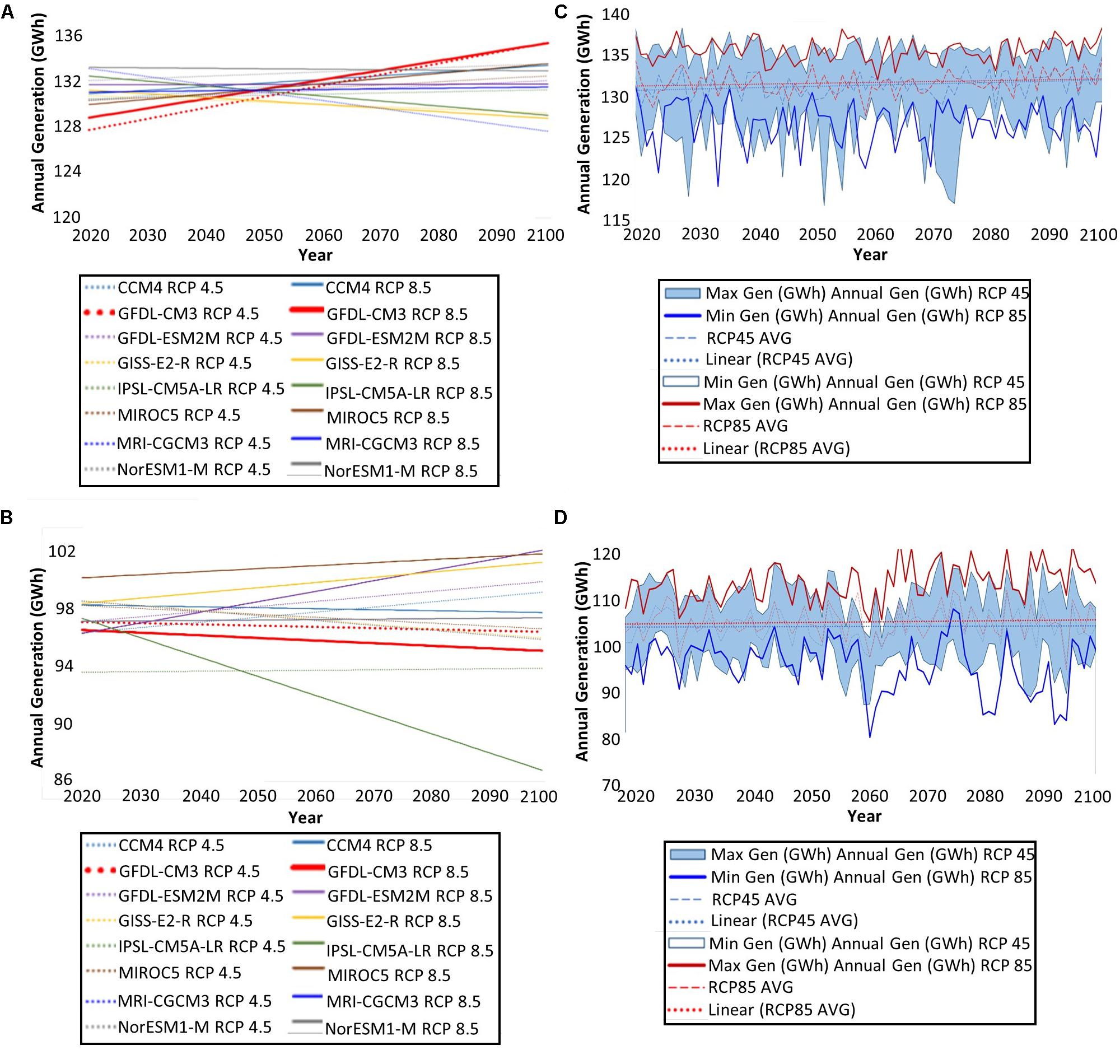
Figure 9. Trends and ranges of annual hydropower production at Trishuli (A,C) and Naltar (B,D) under RCP 4.5 and RCP 8.5 scenarios based on GCM models.
Changes in the timing of snow and glacier melt as well as precipitation dictate the impact of climate change on power production. Our results show that the projected increase in summertime river flow through mid-century does not impact hydropower generation at Trishuli, although some impact was noted at Naltar. This occurs because the additional water is routed around the power plants (i.e., non-power flows) eight months of the year for Trishuli and five months of the year for Naltar. However, during lower flow periods, when power generation is below the capacity of the plant, the projected changes in inflows in various climate futures impact generation levels.
The difference between solid and dashed lines of the same color in Figure 9 indicates the difference in hydropower production between the RCP 4.5 and RCP 8.5 scenarios. The largest differences in generation between RCP 4.5 and RCP 8.5 scenarios were in February, March, and April for Trishuli, and February, March, April, October, November, and December for Naltar. Although the gaps are relatively small, the differences were larger at Naltar (see Supplementary Figures S8, S9 for more details).
The characteristics of hydropower and associated upstream water storage have an impact on power production. Based on the current characteristics of Naltar and Trishuli, the impacts are expected to be small, because both plants underutilize available water resources as measured by the large amounts of non-power water flows. Therefore, we analyzed a set of scenarios with alternative hydropower characteristics and/or water storage at Trishuli to investigate how water and power resource characteristics impact model outcomes. The alternative scenarios analyzed include (a) only water storage, (b) higher capacity, and (c) both water storage and higher capacity (Figure 10). The intent of these purely hypothetical scenarios is to demonstrate the potential impacts on new hydropower plants with higher capacities as well as the potential for mitigation of climate change impacts through water storage. It is not intended to suggest that any changes should be made to Trishuli.
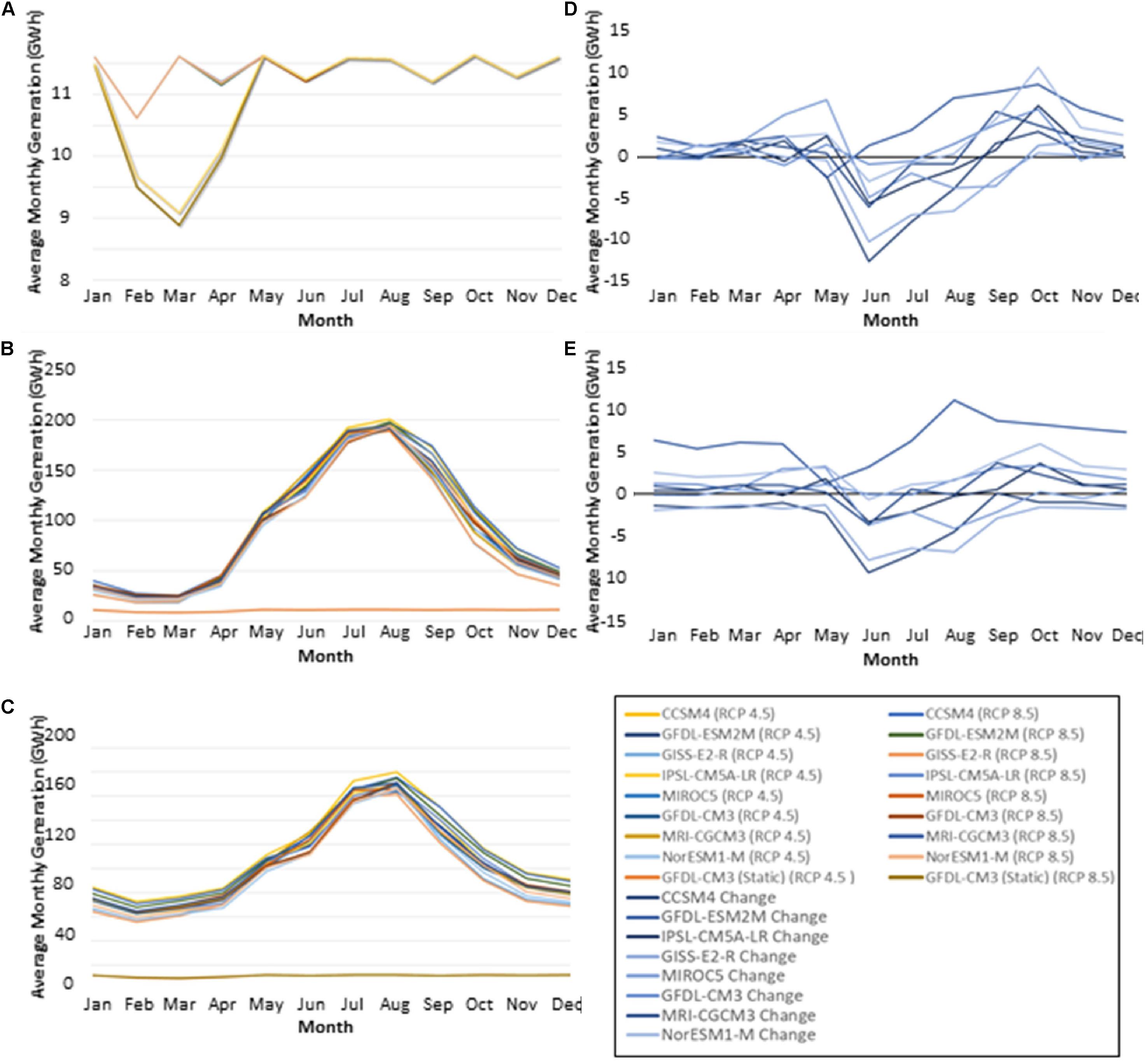
Figure 10. Average 80-year monthly generation in alternative scenarios: (A) water storage (top left); (B) higher capacity (middle left) and (C) higher capacity with water storage (lower left). Average monthly generation increase in a warmer climate by alternative scenario: (D) higher capacity (middle right) and (E) higher capacity with water storage development (lower right).
In the water storage scenario, water resource managers can regulate monthly water flow volumes to maximize power production. All climate models predict nearly the same pattern under all 16 runs of GCM/RCPs futures when water storage resources are available. In other words, Trishuli generation changes due to a warmer climate are zero. This occurs because water storage and water management allows the hydropower plants to be fully utilized at maximum output at all times. Therefore, the average annual generation levels are estimated to increase by approximately 15% over the previous model runs.
The second alternative scenario replaces the current plant with one that has a total capacity of 350 MW and assumes that an increase in head will double the water-to-power conversion efficiency. Under this scenario, all eight GCMs indicate year-round impacts of a warmer climate on power generation (Figure 10D). The largest impacts are expected to occur during the summer and autumn, with minimal impact in February through April; that is, the opposite of the existing system. We also noted that modeled generation levels were higher compared to smaller capacity power plants with storage only.
The third alternative scenario combines a larger plant and water storage capabilities. By reshaping the monthly water released and dampening daily flow volatility, the average annual generation of the larger power plant increased by more than 10% over the previous scenario. The reshaping of monthly flows at Trishuli reduces non-power flow releases, allowing for an increased utilization of water resources for power production. As compared to the previous scenarios, the addition of storage significantly increases generation levels during both winter and early spring and increases the sensitivity of the plant to climate change. Also, note that climate change impacts are more evenly distributed throughout the year when storage resources are developed.
Comparison of Changes in Economic Value of Electricity Supplied by Hydropower Plants in the Karakoram and Himalaya
We estimated and compared the changes in the multimodal median values of the impacts on Naltar and Trishuli hydropower plants under RCP 4.5 and RCP 8.5 as compared to baseline conditions for the pre-peak-water decade, peak-water decade, and post-peak-water decade. Because the available longitudinal data was insufficient (<10 years for Naltar) to scientifically project a business as usual condition, we used historical monthly data as a proxy for the baseline condition in order to maintain consistency between the Trishuli and Naltar. We found differences in the estimated economic impacts on Naltar and Trishuli. The peak- water period is 2035–2050 for Trishuli and 2051–2065 for Naltar. We estimated the average value of the economic impacts for the peak-water periods of 2046–2055 for Trishuli and 2051–2060 for Naltar. For both the Naltar and Trishuli, we did not find significant differences between the estimated annual revenues for the hydropower owners under either RCP 4.5 or RCP 8.5 scenarios as compared to baseline conditions for the pre-peak-water decade.
During the peak-water period, the estimated additional annual benefits for both Naltar and Trishuli hydropower plants under both climate scenarios are higher than the baseline. For the Naltar, the estimated additional annual profits ranges from US $140,000 – 378,000 million under RCP 4.5 and US $228,000 – 618,000 under RCP 8.5. Similarly for Trishuli power plant owners, the additional estimated average annual profit ranges from US $ 33,000 – 139,000 and US $52,000 – 222,000 respectively under RCP 4.5 and RCP 8.5 scenarios.
We analyzed the changes in economic benefits for the hydro-electricity consumers and the global value of displaced CO2 emission from hydroelectricity generation. The estimated annual societal value of Trishuli for the peak-water decade ranges from $3.8 – $4.2 million and $4.7 – $5 million higher in RCP 4.5 and RCP 8.5 scenarios, respectively. The values for Naltar in RCP 4.5 and RCP 8.5 range from $2.6 – 6.3 million and $3.2 – 8.4 million respectively. Similarly, the estimated value of displaced CO2 emission at Trishuli ranges respectively from $65,000 – 70,000 and from $78,000 – 85,000 higher under RCP 4.5 and RCP 8.5. For Naltar, the annual average values range from $117,000 – 253,000 and $158,000 – 354,000 for RCP 4.5 and RCP 8.5 respectively.
Similarly, the societal benefit of the electricity generated by Naltar during the peak-water period ranges from $2.1 – 6.3 million and $ 3.4 – 10.3 million higher in RCP 4.5 and RCP 8.5 scenarios respectively as compared to the baseline. The estimated value of CO2 displacement averaged over the peak-water decade ranges from $ 97,000 – 196,000 under RCP 4.5 and $160,000 – 319,000 under RCP 8.5 higher than the baseline. In Trishuli however, the estimated average annual values of the societal benefit for the peak-melt decade ranges from $1.01 – 3.06 million and $1.6 – 4.8 million and the value of CO2 displacement ranges from $ 22,000 – 46,000 and $ 36,000–73,000.
While the estimated annual values over the decades lay out a picture for long-term expected changes, observed annual variation and seasonal variation in the estimated values could of import from short term operational perspectives as well as from the perspectives of mitigating the inter-annual variation through various interventions in hydro power plants.
In Trishuli, while the loss for the months of February, March and April in the peak-water decade is lower than the pre- peak-water decade, the Trishuli power companies will keep incurring losses. In the Naltar however, the average estimated value for the months of January through April are higher than the baseline under both the RCP 4.5 and RCP 8.5. While profits in January under both RCP 4.5 and RCP 8.5 generation higher than the baseline, a high inter-annual variation in revenues for the plant owners in pre-peak-water period under RCP 4.5 and RCP 8.5 was observed for February as compared to baseline. Increased profits under RCP 8.5 in the peak-water period for April is double than that in RCP 4.5. In contrast, expected average profit for the month of May in peak-water period, is negative in both the RCPs as compared to the baseline.
The future economic and financial value of existing run-of-river hydropower plants such as Trishuli and Naltar are subject to a several uncertainties. One uncertainty is that future water inflow rates are subject to large forecast errors as driven by weather and climatic events. As shown in Figure 4, historical inflows at the Trishuli Site (blue line) display large variations between the winter (low inflows) and summer (high inflows). In addition, peak summertime flow rates varied significantly from year to year from over 850 m3/sec to less than 400 m3/sec; that is, by a factor of more than 2. This summertime volatility is projected to continue in the future under both climate projections (see Figure 5). On the other hand, wintertime minimum inflow rates are fairly stable at Trishuli with base flow rates that, in absolute terms, change relatively little from year to year Figure 4.
Under status quo operations in which water storage and hydropower resources do not change at Trishuli and Naltar, this high summertime inflow volatility does not impact power production because the lowest historical summertime flow rates far exceed the turbine flow rate maximums; that is power production is always at the physical maximum level. The impact of summertime inflow volatility on power system uncertainty is, therefore, inconsequential. In addition to inflow uncertainty, another uncertainty that impacts power production is the availability status of hydropower generating units. This uncertainty was modeled as random independent events during which time it was assumed that specific generating unit(s) were unavailable for power production. Figure 9 shows the band of future power system generation output and therefore one simple measure of uncertainty under both climate projections (RCP 4.5 and RCP 8.5).
In addition to generation uncertainty the value of hydropower is also dependent on time-dependent incremental values of grid firm capacity and energy production that vary by geographical locations (e.g., grid buses). Hydropower has power grid value because it displaces generation that is, under most but not all situation, costly to produce. That is, for example, hydropower production may reduce the generation requirements for other resources that typically burn expensive fuel (e.g., natural gas). These marginal values of energy production are a function of production supply curves and load, both of which evolve over time. These grid incremental value uncertainties were beyond the scope of this paper and were not quantified in this study. We were limited by the extremely limited data that we collected using all possible means, to complete all the analysis.
Discussion and Conclusion
Interactions within and among physical processes and economic activities, such as those that occur during global climate change, are both complex and uncertain (Abbasi et al., 2017). Our study customized an integrated assessment-modeling framework to explore and learn about the relative impacts of projected changes in river flow and its constituents — snow and glacier melt and rainfall — in two basins located in different sub-regions of HMA. Because each individual tool in the framework is subject to modeling errors and uncertainty about the future, we used the framework to gain high-level insights into relative impact trends and magnitudes in the two basins.
The WBM simulations of future river discharge showed an increase in river flow at Trishuli and reduced flows at Naltar. Separating the flow into the primary sources of rain, snow and glacier meltwater shows decreased contribution of both glacier melt and snowmelt to river flow, with glacier meltwater reduced by 40 and 60% for the Trishuli and Naltar sites respectively. A strong seasonal shift in river flow is consistent across most GCMs and RCPs. There is a significantly higher variability across the models toward the end of the century, which is expected given the uncertainties in running these GCMs so far into the future. An earlier spring melt and later accumulation of the snowpack reduce the length of the winter low-flow period, and this is more pronounced for Naltar. We also observed a reduced summer river flow in the model output, although the time of year is different for each location due to lower contributions of glacier meltwater. At Trishuli, the reduction in summer flows occurs primarily in May to June, while at Naltar the lower summer flows are mostly concentrated in July and August. Lutz (2016) found projected river flow in the Hunza decreased during the melt season under both RCP 4.5 and RCP 8.5. The fall and spring shifts in melt move more of the snowmelt away from the summer period, causing overall reduced river flows during this high-flow period. Tahir et al. (2015) found that despite stable or increasing snow cover trends, the river flow trend is decreasing in Hunza. The shortening of the snowmelt period from April through June only is very distinct to the Nepal watershed. The overall expected effect is a longer high-flow season, but with lower streamflow intensity (discharge).
The results from our glacier and hydrology modeling components, showing increasing glacier melt driving increasing river discharge from glaciers and a subsequent decline in river flow, are a characteristic of “peak water” (Kraaijenbrink et al., 2017; Huss and Hock, 2018). These changes are strongest in the RCP 8.5 simulations. Immerzeel and Bierkens (2012) found the dominant factors for changing water supply in the Indus and Ganges basins to be uncertainty in precipitation, population growth, and in the Indus only, groundwater depletion. Lutz et al. (2014) focused on the upstream basins in HMA and showed the greatest future contribution to runoff was glacier melt in the Indus and rainfall in the Ganges. In both cases the metrics were based on large areas where the results used basin wide (Immerzeel and Bierkens, 2012) and aggregated HMA headwaters (Lutz et al., 2014) that do not necessarily indicate the dominant factors at play in the small, headwater basins explored in this research.
The impact of changes in annual inflows does not translate to significant changes in annual power production at small power plants (<20 MW) at Trishuli and Naltar. However, the timing of water inflows during the year and projected changes in flow during low-flow periods in a changed climate are important. In both RCP 4.5 and RCP 8.5 scenarios, during most of the year most of the river water bypasses the Trishuli and Naltar hydropower plants because river flow rates exceed the maximum rate of turbine-water-flow. Power plants therefore mostly operate at the maximum physical limit and are unresponsive to climate change under the current system configuration. However, with an upcoming large storage type hydropower four km upstream from the power plant may influence the power plant especially in drier months. While GCM/RCP results agree that changes are expected to be small at both Trishuli and Naltar, we cannot conclude that a changed climate will have minimal impacts on power systems in HMA.
We found that climate change impacts differ significantly by location, water resource diversity, power plant attributes, electricity demand and sectors, and the attributes of the electricity users. For example, Naltar is projected to have larger impacts than at Trishuli. We also found that the water bypass period is expected to be about twice as long for Trishuli than for Naltar. The estimated changes in economic values under both emission scenarios, compared to their respective baseline scenarios, vary more for Naltar than for Trishuli. We disaggregated the economic impacts in terms of private benefit for the power plant owners, societal benefits for the electricity consumers and benefits of displaced carbon dioxide emission to understand the impacts on various stakeholders. Our estimates show that while the impacts during the peak-water periods are similar for the two power plants, the impacts prior to and after peak-water decades are different. Hydropower plants in both regions are expected to benefit from increased flow during the peak-water period. In Trishuli, the benefits are not significantly different prior to or after peak-water period. However, in Naltar, the estimates show a high variability in potential changes in benefits in the post peak-water period.
In the dry months, when the monthly electricity generation is at the lowest, Trishuli was found to gain from increased melt during dry months. While in Naltar, all dry months are projected to have increased profit except for May in peak-water duration. The loss is further exacerbated for Naltar for the months of June after peak-water duration.
We also estimated the value of carbon dioxide emissions displacement from increased electricity generation during the peak-water duration. This is to showcase the potential carbon credits associated with hydropower plants that could be used to mitigate climate change impacts and generate discussions on mitigation infrastructure financed by carbon credit funds. For e.g., water storage infrastructure upstream of hydropower plants funded through carbon credit could dampen the impacts of inter-annual variability in flow, as well as embankment infrastructure could potentially reduce the impact in case of glacial lake outburst and flow events.
The future economic and financial value of existing run-of-river hydropower plants such as Trishuli and Naltar are subject to several uncertainties. One uncertainty is that future water inflow rates are subject to large forecast errors as driven by weather and climatic events. We tried to address that by analyzing the values for the multimodal minimum, median, and maximum of the projected riverflow for RCP 4.5 and RCP 8.5. Historical inflows at the Trishuli Site display large variations between the winter (low inflows) and summer (high inflows). In addition, peak summertime flow rates varied significantly from year to year. This summertime volatility is projected to continue in the future under both climate projections.
At Trishuli and Naltar, this high summertime inflow volatility is not expected to impact power production because the lowest historical summertime flow rates far exceed the turbine flow rate maximums; that is power production is always at the physical maximum level. The impact of summertime inflow volatility on power system uncertainty is, therefore, inconsequential. On the other hand, wintertime minimum inflow rates are stable at Trishuli with base flow rates that, in absolute terms, change relatively little from year to year. In addition to inflow uncertainty, another uncertainty that impacts power production is the availability status of hydropower generating units. This uncertainty was modeled as random independent events for which it was assumed that specific generating unit(s) were unavailable for power production.
The interactions between climate variables, the source water composition (contribution of glaciers, snow, rain, base flow) of river flow, and the projected changes in hydrologic regime vary geographically from sub-basin to sub-basin and temporally (seasonal, annual, as well as before and after peak-water). The impact of such changes of hydrologic regime on downstream hydropower plants also varies from sub-basin to sub-basin. Our sensitivity analysis shows that power production in storage-type power plants of similar capacity in Trishuli would increase by 15%, and the climate change impacts are negligible. However, higher-capacity power plants in Trishuli were found to be more sensitive to climate change led changes in river flow in our analysis. Because of the rapidly evolving power grids in the region, it is important to understand the dynamics and interactions among existing and new hydropower plant designs and characteristics, water storage development, the overall power grid and projected changes in climate.
The HMA nations, including India, Nepal, and Pakistan, are investing in hydropower development to meet the growing demand for electricity and achieve energy security, stability, and reliability goals. In Pakistan, a combined hydropower capacity of 42 GW is expected to be operating by 2030, and Nepal granted generation and survey licenses to develop hydropower capacity of 5.5 and 6 GW, respectively. In Trishuli’s main river, 770 MW capacity hydropower plants are expected to start operation in near future. A sub-basin-level analysis for the HMA region will help the government and the private sector make informed decisions and assess financial risks based on site-specific analyses that encompass the power grid and hydrological dynamics, using an ensemble of projected flows driven by a set of projected downscaled climatic variables.
Data Availability Statement
The authors confirm that some access restrictions apply to the data such as hydrologic flow and electricity generation that were used in the models. Some of the data were purchased and others availed by respective government offices (for research purposes only). The request to access this data should be directed to the corresponding author. Data products such as graphs and tables are available in the Supplementary Material.
Author Contributions
SM designed the study. AP, DG, and RL estimated river flows in the two sub-basins. TV and MC estimated electricity generation from hydropower plants. SM conducted the economic analysis. DR provided simulated glacier runoff estimates for High Mountain Asia. SM coordinated with all the co authors including SA to integrate all the components into the manuscript.
Funding
This work was enabled by the funds of the National Aeronautical and Space Administration, Earth Science Division’s High Mountain Asia program Grant Number NNH15ZDA001NHMA for the scientists from all three organizations under separate grants. Argonne National Laboratory’s work was supported under interagency agreement, through U.S. Department of Energy contract DE-AC02-06CH11357. The University of New Hampshire effort was supported by NASA Grant Number NNX17AB28G. The University of Alaska, Fairbanks effort was supported by NASA Grant Number NNX17AB27G.
Conflict of Interest
The authors declare that the research was conducted in the absence of any commercial or financial relationships that could be construed as a potential conflict of interest.
Acknowledgments
We are grateful to the Department of Hydrology and Meteorology, Nepal, Nepal Electricity Authority, and Water and Power Development Authority, Pakistan for providing us the data on hydrology, meteorology, and electricity generation required for our analysis.
Supplementary Material
The Supplementary Material for this article can be found online at: https://www.frontiersin.org/articles/10.3389/fenvs.2020.00026/full#supplementary-material
FIGURE S1 | Elevation histograms for the Trishuli, Nepal and Naltar, Karakoram study sites.
FIGURE S2 | Glacier melt contribution in Trishuli (A) and Naltar (B).
FIGURE S3 | Trend lines of glacier water fractions in discharge for (A) Trishuli, Nepal, and (B) Naltar, Karakoram sites. The mid-century increase is caused by additional contribution of core glacier mass loss due to changed climate.
FIGURE S4 | Trendlines of snow melt fractions in discharge for (A) Trishuli, Nepal, and (B) Naltar, Karakoram site.
FIGURE S5 | Average inter-annual changes of discharge for 2010/2050/2090 decades by select GCM/RCPs projections for Nepal (A,C, top row) and Karakoram (B,D, bottom row) sites.
FIGURE S6 | Average monthly increase in monthly hydropower production in Trishuli and Naltar.
FIGURE S7 | Annual hydropower production under RCP 4.5 and RCP 8.5 based on climate model.
FIGURE S8 | Average monthly hydropower production during the 80-year study period under RCP 4.5 and RCP 8.5 based on eight climate models for the static resource development future Trishuli (upper), Naltar (lower).
FIGURE S9 | Impacts of a warmer climate on average monthly hydropower production in Trishuli.
TABLE S1 | Key characteristics of Hunza and Trishuli sub-basins.
TABLE S2 | Mann-Kendall and Sen’s Slope results for the Trishuli basin.
TABLE S3 | Mann-Kendall and Sen’s Slope results for the Naltar basin.
Footnotes
- ^ The efficiency surface is a function of both hydraulic head and water flow rate. According to information provided by APDA, each Naltar turbine has a maximum turbine efficiency of 85%, and at full turbine release the water-to-power conversion factor is approximately 3.53 MW cms–1. Given these two data points, the generic Pelton efficiency curve was adjusted such that the two WAPDA data points are on the efficiency surface. Using identical efficiency curves for each turbine, a power equation related efficiency, head, and water flows to electricity production. Using the power equation, the optimal unit-commitment and dispatch (i.e., output) of each unit was determined at power plant inflow levels ranging from the minimum turbine flow rate of 0.17 cms, to the maximum turbine flow rate of 5.12 cms.
- ^ Idle hours are represented in the model as the times when a unit is capable of producing power but it is not operating.
References
Abbasi, S. S., Ahmad, B., Ali, M., Anwar, M. Z., Dahri, Z. H., Habib, N., et al. (2017). The Indus Basin: A Glacier-Fed Lifeline for Pakistan. Kathmandu: HI-AWARE.
Ahmed, M., and Suphachalasai, S. (2014). Assessing the Costs of Climate Change and Adaptation in South Asia. Available at: http://www.adb.org/sites/default/files/pub/2014/assessing-costs-climate-change-and-adaptation-south-asia.pdf (accessed February 23, 2015).
Alford, D., and Armstrong, R. (2010). The role of glaciers in stream flow from the Nepal Himalaya. Cryosphere Discuss. 4, 469–494. doi: 10.5194/tcd-4-469-2010
Baig, S. U., Khan, H., and Din, A. (2016). Spatio-temporal analysis of glacial ice area distribution of Hunza River Basin, Karakoram region of Pakistan. Hydrologic Processes 32, 1491–1501. doi: 10.1002/hyp.11508
Bajracharya, S., Maharjan, S., Shrestha, F., Bajracharya, O., and Baidya, S. (2014). Glacier Status in Nepal and Decadal Change from 1980 to 2010 Based on Landsat Data. Geneva: World Meteorological Organization.
Bajracharya, S. R., and Shrestha, B. (eds) (2011). The Status of Glaciers in the Hindu Kush-Himalayan Region. Kathmandu: ICIMOD.
Bashir, F., Zeng, X., Gupta, H., and Hazenberg, P. (2017). A hydrometeorological perspective on the karakoram anomaly using unique valley-based synoptic weather observations. Geophys. Res. Lett. 44, 470–410. doi: 10.1002/2017GL075284
Biemans, H., Siderius, C., Lutz, A. F., Nepal, S., Ahmad, B., Hassan, T., et al. (2019). Importance of snow and glacier meltwater for agriculture on the Indo-Gangetic Plain. Nat. Sustain. 2, 594–601. doi: 10.1038/s41893-019-0305-3
Brown, M. E., Racoviteanu, A. E., Tarboton, D. G., Gupta, A. Sen, Nigro, J., Policelli, F., et al. (2014). An integrated modeling system for estimating glacier and snow melt driven streamflow from remote sensing and earth system data products in the Himalayas. J. Hydrol. 519, 1859–1869. doi: 10.1016/j.jhydrol.2014.09.050
Brun, F., Berthier, E., Wagnon, P., Kääb, A., and Treichler, D. (2017). A spatially resolved estimate of high mountain asia glacier mass balances from 2000 to 2016. Nat. Geosci. 10, 668–673. doi: 10.1038/ngeo2999
Dee, D. P., Uppala, S. M., Simmons, A. J., and Vitart, F. (2011). The ERA-Interim reanalysis: configuration and performance of the data assimilation system. Q. J. R. Meteorol. Soc. 137, 553–597.
Federer, C. A., Vörösmarty, C., and Fekete, B. (1996). Intercomparison of methods for calculating potential evaporation in regional and global water balance models. Water Resour. Res. 32, 2315–2321. doi: 10.1029/96wr00801
Fekete, B. M., Vorosmarty, C. J., Roads, J. O., and Willmott, C. J. (2004). Uncertainties in precipitation and their impacts on runoff estimates. J. Clim. 17, 294–304. doi: 10.1175/1520-0442(2004)017<0294:uipati>2.0.co;2
Fischer, G., Nachtergaele, F., Prieler, S., van Velthuizen, H. T., Verelst, L., and Wiberg, D. (2008). Global Agro-ecological Zones Assessment for Agriculture (GAEZ 2008). IIASA, Laxenburg, Austria and FAO, Rome, Italy.
Forsythe, N., Fowler, H. J., Li, X. F., Blenkinsop, S., and Pritchard, D. (2017). Karakoram temperature and glacial melt driven by regional atmospheric circulation variability. Nat. Clim. Chang. 7, 664–670. doi: 10.1038/NCLIMATE3361
Fowler, H. J., and Archer, D. R. (2006). Conflicting signals of climate change in the Upper Indus basin. J. Clim. 19, 4276–4293. doi: 10.1175/jcli3860.1
Grogan, D. S. (2016). Global and Regional Assessments of Unsustainable Groundwater Use in Irrigated Agriculture. New Hampshire: University of New Hampshire.
Hartman, M. D., Baron, J. S., Lammers, R. B., Cline, D. W., Band, L. E., Liston, G. E., et al. (1999). Simulations of snow distribution and hydrology in a mountain basin. Water Resour. Res. 35, 1587–1603. doi: 10.1029/1998wr900096
Hirsch, P. E., Schillinger, S., Weigt, H., and Burkhardt-Holm, P. (2014). A hydro-economic model for water level fluctuations: combining limnology with economics for sustainable development of hydropower. PLoS One 9:e114889. doi: 10.1371/journal.pone.0114889
Huss, M., and Hock, R. (2015). A new model for global glacier change and sea-level rise. Front. Earth Sci. 3:54. doi: 10.3389/feart.2015.00054
Huss, R., and Hock, R. (2018). Global-scale hydrological response to future glacier mass loss. Nat. Clim. Chang. 8, 135–140. doi: 10.1038/s41558-017-0049-x
Immerzeel, W., and Bierkens, M. (2012). Asia’s water balance. Nat. Geosci. 5, 841–842. doi: 10.1038/ngeo1643
Immerzeel, W. W., Droogers, P., de Jong, S. M., and Bierkens, M. F. P. (2009). Large-scale monitoring of snow cover and runoff simulation in Himalayan river basins using remote sensing. Remote Sens. Environ. 113, 40–49. doi: 10.1016/j.rse.2008.08.010
Immerzeel, W. W., Lutz, A. F., Andrade, M., Bahl, A., Biemans, H., Bolch, T., et al. (2019). Importance and vulnerability of the world’s water towers. Nature 577, 364–369. doi: 10.1038/s41586-019-1822-y
Kapnick, S. B., Delworth, T. L., Ashfaq, M., and Malyshev, S. (2014). Snowfall less sensitive to warming in Karakoram than in Himalayas due to a unique seasonal cycle. Nat. Geosci. 7, 834–840. doi: 10.1038/ngeo2269
Kayastha, R. B., and Shrestha, A. (2019). “Snow and ice melt contribution in the daily discharge of langtang and modi rivers, Nepal,” in Environmental Change in the Himalayan Region: Twelve Case Studies, eds A. Saikia, and P. Thapa, (Cham: Springer), 1–21. doi: 10.1007/978-3-030-03362-0-1
Kessides, I. N. (2013). Chaos in power: pakistan’s electricity crisis. Energy Policy 55, 271–285. doi: 10.1016/j.enpol.2012.12.005
Khattak, M. S., Babel, M. S., and Sharif, M. (2011). Hydro-meteorological trends in the upper Indus River basin in Pakistan. Clim. Res. 46, 103–119. doi: 10.3354/cr00957
Khokhar, S., Min, X., and Chu, Q. (2015). Electricity crisis and energy efficiency to poultry production in Pakistan. World Poult. Sci. J. 71, 539–546. doi: 10.1017/S0043933915002123
Kraaijenbrink, P., Bierkens, M., Lutz, A., and Immerzeel, W. W. (2017). Impact of a global temperature rise of 1.5 degrees Celsius on Asia’s glaciers. Nature 549, 257–260. doi: 10.1038/nature23878
Lammers, R. B., Band, L. E., and Tague, C. L. (1997). Scaling Behaviour of Watershed Processes. Scaling-up. Cambridge: Cambridge University Press.
Lammers, R. B., Shiklomanov, A. I., Vorosmarty, C. J., Fekete, B. M., and Peterson, B. J. (2001). Assessment of contemporary arctic river runoff based on observational discharge records. J. Geophys. Res. 106, 3321–3334. doi: 10.1029/2000jd900444
Lehner, B., and Grill, G. (2013). Global river hydrography and network routing: baseline data and new approaches to study the world’s large river systems. Hydrol. Process. 27, 2171–2186. doi: 10.1002/hyp.9740
Lehner, B., Liermann, C. R., Revenga, C., and Wisser, D. (2011). High-resolution mapping of the world’s reservoirs and dams for sustainable river-flow management. Front. Ecol. Environ. 9, 494–502. doi: 10.1890/100125
Liu, J., Hertel, T. W., Lammers, R. B., Prusevich, A., Baldos, U. L. C., Grogan, D. S., et al. (2017). Achieving sustainable irrigation water withdrawals: global impacts on food security and land use. Environ. Res. Lett. 12:104009. doi: 10.1088/1748-9326/aa88db
Liu, X., and Chen, B. (2000). Climatic warming in the tibetan plateau during recent decades. Int. J. Climato. 20, 1729–1742. doi: 10.1371/journal.pone.0088178
Lutz, A. F. (2016). Impact of Climate Change on the Hydrology of High Mountain Asia. Ph.D. thesis, Universiteit Utrecht, Utrecht.
Lutz, A. F. F., Immerzeel, W. W., Shrestha, A. B., and Bierkens, M. F. P. (2014). Consistent increase in High Asia’s runoff due to increasing glacier melt and precipitation. Nat. Clim. Chang. 4, 587–592. doi: 10.1038/nclimate2237
McLeod, A. I. (2011). Kendall: Kendall Rank Correlation and Mann-Kendall Trend Test. R Package Version 2.2.
Ministry of Finance (2013). Pakistan Economic Servey 2012-2013 Chapter-14 Energy, 187–188. New Delhi: Ministry of Finance.
Mishra, S. K., Hayse, J., Veselka, T., Yan, E., Kayastha, R. B., LaGory, K., et al. (2018). An integrated assessment approach for estimating the economic impacts of climate change on River systems: an application to hydropower and fisheries in a Himalayan River, Trishuli. Environ. Sci. Policy 87, 102–111. doi: 10.1016/j.envsci.2018.05.006
Nash, J. E., and Sutcliffe, J. V. (1970). River flow forecasting through conceptual models part I - A discussion of principles. J. Hydrol. 10, 282–290. doi: 10.1016/0022-1694(70)90255-6
Nepal Electricity Authority [NEA], (2012). Annual Report 2011-2012. Nepal Electricity Authority. Kathmandu: Electricity Authority [NEA].
Nepal Electricity Authority [NEA], (2015). Data Collection From Personal Communication. Kathmandu: Electricity Authority [NEA].
Pindyck, R. S. (2013). Climate change policy: what do the models tell us? J. Econ. Liter. 51, 860–872. doi: 10.1257/jel.51.3.860
Pohlert, T. (2018). Trend: Non-Parametric Trend Tests and Change-Point Detection. R Package version 1.1.1.
Pradhananga, N. S., Kayastha, R. B., Bhattarai, B. C., Adhikari, T. R., Pradhan, S. C., Devkota, L. P., et al. (2014). Estimation of discharge from Langtang River basin, Rasuwa, Nepal, using a glacio-hydrological model. Ann. Glaciol. 55, 223–230. doi: 10.3189/2014AoG66A123
Pritchard, H. D. (2019). Asia’s shrinking glaciers protect large populations from drought stress. Nature 569, 649–654. doi: 10.1033/s41586-019-1240
Racoviteanu, A. E., Armstrong, R., and Williams, M. W. (2013). Evaluation of an ice ablation model to estimate the contribution of melting glacier ice to annual discharge in the Nepal Himalaya. Water Resour. Res. 49, 5117–5133. doi: 10.1002/wrcr.20370
Rehman, A. S., Cai, Y., Hussain, N., and Das, G. (2019). Energy-environment-economy nexus in pakistan : lessons from a PAK-TIMES Model. Energy Policy 126, 200–211. doi: 10.1016/j.enpol.2018.10.031
Rennick, M. A. (1977). Parameterization of tropospheric lapse rates in terms of surface-temperature. J. Atmos. Sci. 34, 854–862. doi: 10.1175/1520-0469(1977)034<0854:tpotlr>2.0.co;2
RGI Consortium, (2017). Randolph Glacier Inventory – A Dataset of Global Glacier Outlines: Version 6.0. Technical Report, Global Land Ice Measurements from Space. Colorado: Digital Media.
Rounce, D., Hock, R., Shean, D., and Khurana, T. (2020). Quantifying glacier mass change in High Mountain Asia through 2100 using the open-source python glacier evolution model (PyGEM). Front. Earth Sci. 7:331. doi: 10.3389/feart.2019.00331
Shean, D., Montesano, P., Rounce, D., Bhushan, S., Arendt, A., and Osmanoglu, B. (2020). A systematic, regional assessment of High-mountain Asia mass balance. Front. Earth Sci. 7:363. doi: 10.3389/feart.2019.00363
Shiklomanov, A. I., Yakovleva, T. I., Lammers, R. B., Karasev, I. P., Vorosmarty, C. J., and Linder, E. (2006). Cold region river discharge uncertainty - estimates from large Russian rivers. J. Hydrol. 326, 231–256. doi: 10.1016/j.jhydrol.2005.10.037
Shrestha, A. B., Wake, C. P., Dibb, J. E., and Mayewski, P. A. (2000). Precipitation fluctuations in the Himalaya and its vicinity: an analysis based on temperature records from Nepal. Int. J. Clim. 20, 317–327. doi: 10.1002/(sici)1097-0088(20000315)20:3<317::aid-joc476>3.0.co;2-g
Shrestha, A. B., Wake, C. P., Mayewski, P. A., and Dibb, J. E. (1999). Maximum temperature trends in the Himalaya and its vicinity: an analysis based on temperature records from Nepal for the period 1971–94. J. Clim. 12, 2775–2786. doi: 10.1175/1520-0442(1999)012<2775:mttith>2.0.co;2
Smith, T., and Bookhagen, B. (2018). Changes in seasonal snow water equivalent distribution in high mountain Asia (1987 to 2009). Sci. Adv. 4:e1701550. doi: 10.1126/sciadv.1701550
Stiglitz, J., and Stern, N. (2017). Report of the High-Level Commission on Carbon Prices. Washington D.C: World Bank.
Tachikawa, T., Kaku, M., Iwasaki, A., Gesch, D. B., Oimoen, M. J., Zhang, Z., et al. (2011). “ASTER global digital elevation model version 2 - summary of validation results,” in Paper Presentation at the 39th ASTER Science Team Meeting, Tokyo.
Tahir, A. A., Chevallier, P., Arnaud, Y., Ashraf, M., and Bhatti, M. T. (2015). Snow cover trend and hydrological characteristics of the Astore River basin (Western Himalayas) and its comparison to the Hunza basin (Karakoram region). Sci. Total Environ. 505, 748–761. doi: 10.1016/j.scitotenv.2014.10.065
Tahir, A. A., Chevallier, P., Arnaud, Y., Neppel, L., and Ahmad, B. (2011). Modeling snowmelt-runoff under climate scenarios in the Hunza River basin, Karakoram Range, Northern Pakistan. J. Hydrol. 409, 104–117. doi: 10.1016/j.jhydrol.2011.08.035
van Buuren, S., and Groothuis-Oudshoorn, K. (2011). Mice: multivariate imputation by chained. Equations in R. J. Statist. Softw. 45, 1–67.
Viviroli, D., Archer, D. R., Buytaert, W., Fowler, H. J., Greenwood, G. B., Hamlet, A. F., et al. (2011). Climate change and mountain water resources: overview and recommendations for research, management and policy. Hydrol. Earth Syst. Sci. 15, 471–504.
Vörösmarty, C. J., Green, P., Salisbury, J., and Lammers, R. B. (2000). Global water resources: vulnerability from climate change and population growth. Science 289, 284–288. doi: 10.1126/science.289.5477.284
Water and Power development authority Report [WAPDA], (2013). Unpublished Report Pakistan Water and Power Development Authority. Lahore: WAPDA.
Wisser, D., Fekete, B. M., Vorosmarty, C. J., and Schumann, A. H. (2010). Reconstructing 20th century global hydrography: a contribution to the Global Terrestrial Network- Hydrology (GTN-H). Hydrol. Earth Syst. Sci. 14, 1–24. doi: 10.5194/hess-14-1-2010
Keywords: remote sensing, hydrology, glaciers, climate change, hydropower, economic analysis, Karakoram anomaly and Himalaya
Citation: Mishra SK, Veselka TD, Prusevich AA, Grogan DS, Lammers RB, Rounce DR, Ali SH and Christian MH (2020) Differential Impact of Climate Change on the Hydropower Economics of Two River Basins in High Mountain Asia. Front. Environ. Sci. 8:26. doi: 10.3389/fenvs.2020.00026
Received: 19 April 2019; Accepted: 25 February 2020;
Published: 13 March 2020.
Edited by:
Tomas Halenka, Charles University, CzechiaReviewed by:
Xander Wang, University of Prince Edward Island, CanadaCameron Scott Watson, University of Leeds, United Kingdom
Copyright © 2020 Mishra, Veselka, Prusevich, Grogan, Lammers, Rounce, Ali and Christian. This is an open-access article distributed under the terms of the Creative Commons Attribution License (CC BY). The use, distribution or reproduction in other forums is permitted, provided the original author(s) and the copyright owner(s) are credited and that the original publication in this journal is cited, in accordance with accepted academic practice. No use, distribution or reproduction is permitted which does not comply with these terms.
*Correspondence: Shruti K. Mishra, bWlzaHJhQGFubC5nb3Y=