- 1School of Natural and Environmental Sciences, Newcastle University, Newcastle upon Tyne, United Kingdom
- 2Sustainable Environment Research Centre, University of South Wales, Pontypridd, United Kingdom
- 3School of Engineering, Newcastle University, Newcastle upon Tyne, United Kingdom
The upstream protection of the biomass present in biological treatment processes is a vital challenge as the consequences of failure could include exposure of water users to hazardous chemicals in addition to loss of treatment performance. Online detection of toxic compounds in wastewater could enable processes to be monitored in real-time and promote pro-active responses to pollution incidents. Recently, Microbial Fuel Cells (MFCs) which generate electricity from organic matter oxidation have shown potential as sensors for online detection of toxicity. In this study, the detection of a model toxicant (4-nitrophenol) was investigated using a multi-stage MFC-based toxicity sensor. MFCs were operated with synthetic wastewater to maintain realistic conditions while enabling organic carbon levels to be controlled. A positive correlation was observed between the 4-NP concentrations and the current drop area showing that the response was proportional to the toxicity level. In addition, the sensor anodic biofilm exhibited resilience to acute toxic events with recovery of 75% of the initial current following a toxic event comprising 500 mg/L 4-NP after 4 h. However, repetitive toxicity events could lead to the selection of resistant bacteria able to degrade the toxic compounds. In this study, a maximal 4-NP degradation rate of 36 mg/h was observed. This limitation could be overcome by re-calibration after a determined number of toxic events. An additional feature of the multi-stage configuration of the sensor is that a drop in output caused by the presence of a toxic compound could be distinguished from a drop in output caused by a decrease in BOD. The microbial community on the sensor anode was characterized by 16S rRNA gene sequencing and shown to comprise an anaerobic community of fermentative bacteria capable of producing volatile fatty acids and hydrogen that were consumed by electrogenic Geobacter spp (2.76 to 21.39% of the anode community) that generated the electrical signal in the sensor. The multi-stage MFC biosensor could provide an early warning system capable of alerting process operators to the presence and level of toxicity in influent wastewater.
1. Introduction
The upstream protection of the biomass present in biological treatment processes is a vital challenge as the consequences of failure could include exposure of water users to hazardous chemicals in addition to loss of treatment performance (Quevauviller et al., 2007). Online detection of toxic compounds in wastewater could enable processes to be monitored in real-time and promote pro-active responses to pollution incidents. Existing methods for toxicity detection often involve offline incubation of samples with known species of micro-organisms (e.g., MicrotoxⓇ; Johnson, 2005) which are not representative of the microbial diversity involved in biological treatment process. It has been shown that a higher biodiversity tends to increase the stability of ecosystem functions following disturbance (Saikaly and Oerther, 2011). Online toxicity analyzers predominantly measure indirect proxies for the treatment microbial communities [e.g., oxygen uptake rate (OUR) or methane production] and it can take several hours before results are generated. This is too slow to allow operators to respond to a shock loading (Kong et al., 1996; Xiao et al., 2015). Recently, Microbial fuel cells (MFCs) which generate electricity from organic matter oxidation by microorganisms have shown potential as online toxicity sensors (Abrevaya et al., 2015; Xiao et al., 2015; Yu et al., 2017; Chouler et al., 2018; Adekunle et al., 2019). The organic matter present in wastewater is oxidized by an electrogenic biofilm grown on the anode of an electrical cell. Current inhibition occurs when a substance (e.g., toxicant) enters the anode chamber inhibiting the bacteria present in the biofilm. The goal of this study was to explore the potential of using multi-stage MFCs as a biosensor for enhanced, online detection of toxic compounds in wastewater.
Studies investigating the use of MFC as biosensors have often focused on the detection of biochemical oxidation demand (BOD)/organic load and the BOD5 test which is the standard method used by the wastewater industry for quantifying biodegradable organic carbon pollution. It has been established that a positive correlation can be obtained between the concentration of BOD and the electrical current generated by an MFC (Chang et al., 2004; Kim et al., 2006a; Di Lorenzo et al., 2009a,b; Liu et al., 2011; Abrevaya et al., 2015; Sun et al., 2015). This correlation was observed with diverse substrates from simple non-fermentable compounds such as acetate, fermentable sugars such as glucose (Di Lorenzo et al., 2009b), more complex synthetic sewage (Kim et al., 2006a) and real wastewaters (Kim et al., 2003, 2007; Liu et al., 2011). In comparison, relatively few papers have employed MFC devices as toxicity sensors (Stein et al., 2010; Shen et al., 2013; Di Lorenzo et al., 2014; Jiang et al., 2015; Xu et al., 2016). Some substances including heavy metals inhibit current generation and have demonstrated promising results (Kim et al., 2007; Patil et al., 2010; Liu et al., 2014; Yu et al., 2017; Adekunle et al., 2019). Conversely, fewer studies of toxic organic compounds have been reported. Patil et al. (2010) observed no response of electroactive biofilms to antibiotics (sulfamethaxozole and sulfadiazin) and disinfectants (chloramine B at low concentrations). Wu et al. (2014) observed a toxic effect of antibiotics (tobramycin) but only at high concentrations (100 mg/L), far exceeding levels observed in wastewater treatment plants. However, inhibition of current generation was reported for pesticides such as aldicarb (King et al., 2014) or organophosphorus compounds (Kim et al., 2007), and for PCBs (Polychlorobiphenyls) (Kim et al., 2007). It is apparent therefore that MFCs respond to many toxic substances, but the magnitude of the response depends on the concentration and inherent toxicity of any particular compound. Importantly, using the MFC-based toxicity sensors reported to date there, are difficulties in distinguishing a drop in sensor output caused by a drop in BOD levels from a drop in sensor output caused by the presence of a toxic compound (Kim et al., 2006b; Jiang et al., 2016; Tan et al., 2018). There is therefore a clear need for the development of a sensor capable of distinguishing between BOD and toxicity detection. Ideally, a combined BOD–toxicity sensor would be able to operate online, providing real-time estimation of the BOD and detection of the presence of toxicant. Recently, Spurr (2017); Spurr et al. (2018) reported the use of a multi-stage MFC configuration which could extend the range of BOD detection compared with single stage sensors, and simultaneously detect the presence of a toxicant. This monitoring technique has two principles of measurement; the generation of current by BOD and the loss of current due to toxic inhibition and by using this configuration a decrease in current can be elucidated from the ordered response in current (Spurr et al., 2020). Wu et al. (2019) have subsequently used a multi-stage MFC configuration but only for detection of toxicity of chromium (VI) using a pure culture of Exiguobacterium aestuarii. A BOD-toxicity sensor could be used in wastewater treatment plants which periodically receive toxic compounds in their influent streams if mechanisms involved in the detection (biological and electrochemical degradation, mass transport and biofilm formation) are better understood.
In this study, the detection of toxic organic compounds was investigated using a multi-stage MFC-based toxicity sensor. MFCs were operated with synthetic wastewater to maintain realistic conditions, and control for the effect of variable BOD concentrations 4-nitrophenol (4-NP) was used as a model toxic compound. Mono-nitrophenols are used as precursors in the manufacture of various industrial chemicals (including pharmaceuticals, dyes and pesticides; US PHS, 1992) and are regulated by the United States Environmental Protection Agency (Agency for Toxic Substances and Disease Registry (ATSDR), 1992). Among mono-nitrophenol, 4-NP is considered as the most toxic and there is an extensive literature on 4-NP toxicity (Gerike and Fischer, 1979; Nyholm et al., 1984; Ingerslev and Nyholm, 2000; Toräng and Nyholm, 2005). 4-NP is also used as a model compound for biodegradation tests of pollutants as recommended by the Organization for Economic Co-operation and Development (OECD, 2001). To evaluate the impact of 4-NP on MFC output, the extent of decrease in current was recorded following exposure to different concentrations of 4-NP. The residence time distribution was observed and a mass balance was calculated to understand if processes such as degradation or adsorption could affect toxicity detection. The chemical composition of the MFC feed, including total organic carbon, acetate and sulfate concentration, was followed as synthetic wastewater medium passed through the MFC stages. Finally, the microbial communities present in the electroactive biofilms of each individual cell in the multi-stage MFC were characterized by 16S rRNA gene sequencing.
2. Materials and Methods
2.1. MFC Reactor Operation
The tubular Microbial Fuel Cells (MFCs) with a helical anodic design (Figure 1) (Kim et al., 2012; Michie et al., 2014) used in this study were manufactured by the University of South Wales. Duplicate, four stage reactors were used in this study. Each reactor was composed of four single chamber MFCs 29.38 (±0.92) ml (70 mm long and 25 mm diameter). The anodes were of a helical design (area ≈ 96 cm2) (Kim et al., 2012; Michie et al., 2014) and were made from carbon felt (CV080/20301/1000, PRF Composite materials). The spacing between helices was 1 cm (4 helices in each MFC) and the width of the anode was 3 mm. The air cathodes (area ≈ 79 cm2) were carbon cloth loaded with 0.5 mg/cm2 Platinum (EC4019, Fuel Cell Earth). A cation exchange membrane (CMI-7000, Membrane International Inc., USA) was inserted between the anode compartment and the cathode to avoid oxygen diffusion into the anodic compartment. Each MFC was connected in parallel to an external resistor of 100 ohms and the voltage from each MFC was recorded using a data logger (DataTaker DT85).
Reactors were inoculated with primary settled wastewater from a Northumbrian Water Ltd treatment works and fed a modified OECD synthetic sewage medium (50:50 v/v) (OECD, 2001). The system was initially enriched in re-circulation mode (flow rate : 11.5 ml/min). After one week MFCs were fed in continuous mode with the same modified OECD synthetic sewage medium. The OECD synthetic sewage medium was composed of 0.640 g/L peptone, 0.440 g/L meat extract, 0.120 g/L urea, 7 mg/L NaCl, 2 mg/L MgSO4, 4 mg/L CaCl2, and 25 mM of phosphate buffer pH = 7.2 (2.68 g/L K2HPO4 and 1.31 g/L KH2PO4). The conductivity was 2.19 mS/cm. The medium was sparged with N2 before utilization. In continuous mode the flow rate was 2.31 ml/min and was controlled by a peristaltic pump. The temperature was controlled at 30 ± 2°C by placing the tubular reactors on a heating platform inside a polystyrene enclosure. The maximal power output of the MFCs was measured from polarization curves determined by linear voltammetry utilizing a two electrode arrangement with a potentiostat. Linear voltammetry was performed from the open circuit potential to 0 V using a scan rate of 1 mV/s The power was calculated by multiplying the current by the voltage.
2.2. Tests of Acute Toxicity Events
Toxicant-amended synthetic sewage was prepared by addition of an appropriate amount of 4-nitrophenol (4-NP) from a 3 g/L stock solution, to synthetic sewage to obtain final concentrations ranging from 75–500 mg/L. The toxic medium was then sparged with N2 and samples were taken to verify the 4-NP concentrations. The tests of acute toxicity events were undertaken only if the current was stable at least 24 h before. The percentage variation of the current 24 h before the test was 4.7 ± 2.1%, and only 2.6 ± 1.0% 2 h before the test. The acute toxicity events were simulated by switching the peristaltic pump inlet feed using a three-way valve installed upstream of the pump (Figure S1). Samples were taken at the outlet of MFC4 (Figure 1). The toxicant amended medium was pumped for 55 min (127 ml total volume; 1 HRT of the system) before reconnecting to the synthetic medium containing no 4-NP. In order to compare the response of the MFC to the presence of the toxic 4-NP, every distinct measurement of the current Itox(t), recorded every minute over the 55 min duration of the acute toxicity event, was normalized Inorm(t) by the average of the stable current Imean 1 h before the toxicity event (Equation 1).
2.3. Residence Time Distribution
In order to follow changes in the residence time distribution (RTD) of 4-NP in the linked MFCs, 4-NP concentrations Cout were measured as a function of time during acute toxicity event experiments. The samples were filtered (pore size: 0.2 μm), then 4-NP was quantified by visible light spectrophotometry at a wavelength of 320 nm (detection range: 0.5–30 mg/L) after acidification with HCl (pH < 3). Output 4-NP concentrations Cout(in mg/ml) were normalized by the injected quantity of 4-NP NNP(in mg) (Equation 2). NNPis equal to the product of the initial concentration Cin(in mg/L), the flow rate υ (2.31 ml/min) and the duration of 4-NP injection T (55 min).
The percentage of NNP detected at the output was determined by the integral of E(t). The quantification of 4-NP lost MNP(in mg) was calculated by the (Equation 3):
A solution of sodium chloride of 5 g/L (conductivity = 8.91 mS/cm) was used as tracer to determine the hydraulic characteristics of the MFC reactors before inoculation and operation. The NaCl tracer was injected continuously for the same time T and at the same flow rate υ as detailed above.
2.4. Chemical Analysis
In order to track carbon consumption through the MFC stages, total organic and inorganic carbon (TOC and IC), chemical oxidation demand (COD) and volatile fatty acid (VFA) concentrations were measured (Figure 2). Sulfate, nitrate and nitrite concentrations were also measured in order to follow electron acceptors which may compete with the electrode in the MFC reactors. Samples (10 mL) were taken from the MFCs and filtered (pore size: 0.45 mm). These were taken before the synthetic wastewater entered the MFC reactor and after each MFC stage. TOC and IC were determined using a TOC 5050A Total Organic Carbon analyzer. COD was measured using a photometric COD cell test (Merck). An ICS-1000 ion chromatography system fitted with a 9 × 250 mm Dionex™ IonPac™ ICE-AS1 column was used to measure VFA concentrations (eluent: 1 mM of octanesulfonic acid, flow rate: 0.8 ml/min). An ICS-1000 ion chromatography system fitted with a 3 × 150 mm Dionex™ IonPac™ AS14A column was used to measure anion concentrations (eluent: 8 mM sodium carbonate and 1mM sodium bicarbonate, flow rate: 0.5 ml/min).
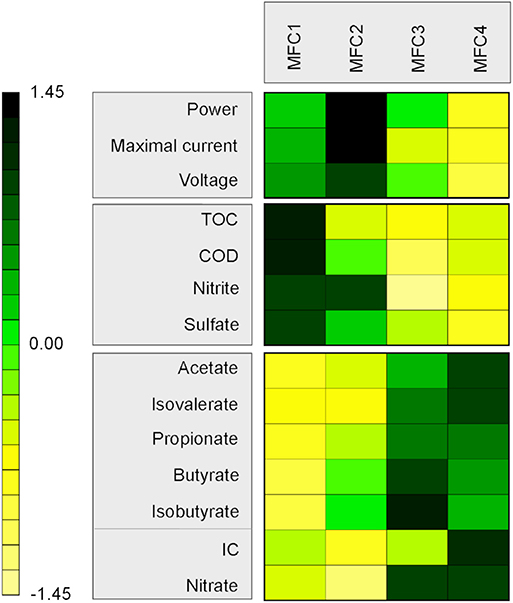
Figure 2. Heatmap of chemical variables as a function of MFC stages. Variables were centered and scaled. The higher values are in green and the lower values in yellow. TOC and IC variables are respectively the total organic carbon and the inorganic carbon concentration. COD variable is the chemical oxygen demand concentration.
2.5. Determining the Fate of 4-NP and Its Transformation Products
2.5.1. Chemical Polymerization of 4-Aminophenol (4-AP)
Under anaerobic and aerobic conditions, 200 mg/L of 4-aminophenol (4-AP), a product of the reduction of 4-NP, was added to OECD synthetic sewage to determine if the polymerization of 4-AP was possible under conditions within the MFC reactor. Following incubation (24 h) a precipate was seen to form in OECD synthetic sewage containing 4-AP. The solution was centrifuged, the supernatant was removed and the precipitate was washed three times with deionized water. The precipitate was then dried 2 days at 40°C and weighed. The solubility of the precipitate was tested in different solvents (ethanol, acetone, dimethylsulfoxyde (DMSO), HCl (pH = 1) and NaOH (pH = 10). Finally, precipitate samples were analyzed by Fourier transform infrared spectroscopy (FT-IR) and UV-visible spectrophotometry.
2.5.2. Biodegradation Tests
In order to identify the mechanisms involved in 4-NP degradation through the reactors, degradation tests were undertaken in MFC reactors and microcosms with no electrodes. 250 ml single chamber MFCs were used in batch conditions using the same electrode and membrane materials as in the tubular reactors. The anode and cathode were connected via an external resistor of 100 Ω and the voltage of MFCs was recorded using a data logger (DataTaker DT85). Microcosms experiments were conducted in 100 ml serum vials sealed with PTFE-lined butyl rubber stoppers an aluminum crimp top. The same synthetic medium used to feed the tubular reactors was used for all batch MFCs and microcosms.
Six MFCs and six microcosms containing modified OECD synthetic sewage medium were inoculated (50:50 v/v) with primary settled wastewater from a Northumbrian Water Ltd treatment works. After incubation for 10 days, when the voltage stabilized, 50 mg/L 4-NP was added to two MFCs (MFC-4NP) and two microcosms (M-4NP) in order to adapt the microbial population to the presence of 4-NP. As controls, two further MFCs (MFC-no4NP) and two microcosms (M-no4-NP) were maintained in the same initial conditions without 4-NP addition. Finally, two MFCs (MFC-St) and two microcosms (M-St) were sterilized immediately after inoculation to test for abiotic, chemical or electrochemical transformation of 4-NP. After four weeks of operation/incubation, 4-NP (300 mg/L) was added to the 6 MFCs and 6 microcosms. The solution was sampled after 90 min and after one day. 4-NP was measured by spectrophotometry at a wavelength of 320 nm. In order to determine if a polymer of 4-AP was present in the samples, the solution was centrifuged, the supernatant was removed and the precipitate was washed three times with water and dried. The solubility of the precipitate was tested in different solvents as described above. The precipitate was also analyzed by FT-IR and UV-visible spectrophotometry.
2.6. Microbial Community Analysis
2.6.1. DNA Extraction and qPCR of 16S rRNA Gene
At the end of the study, the tubular MFC reactors were disassembled and the anodes were sampled from each individual MFC (two replicate samples of anode of 1.0 × 0.3 cm). Because of biomass growth in the anode chamber flow channel (Figure 3), biomass sludge from within the reactor was also sampled (three replicates of 250 mg). DNA was extracted using a FastDNA™ Spin Kit for soil (MP Biomedicals) and quantified using a Qubit™ fluorometer (Invitrogen).
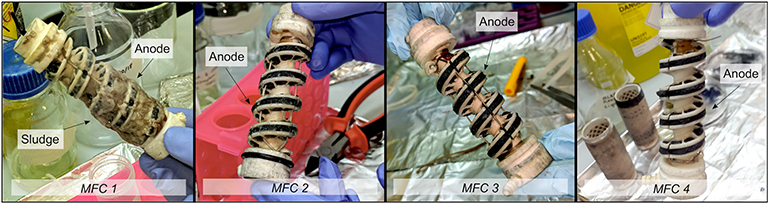
Figure 3. Biomass present through the reactors observed after 115 days operation with synthetic wastewater. An abundant quantity of sludge was found in the anodic compartment of MFC1. Only residues of sludge were present in MFC2. No sludge was observed in MFC3 and MFC4.
Bacterial abundamce in the samples was determined by qPCR of 16S rRNA genes using Ssoadvanced™ Universal SYBRⓇGreen Supermix (Bio-Rad) as reaction mix. The primers used were those used to quantify bacteria by Callbeck et al. (2013). A standard curve was constructed using DNA standards ranging from 103 and 109 16S rRNA gene copies/μ L (R2 = 0.993, Efficiency = 84.8%). The qPCR thermal cycling protocol comprised 98.0°C for 3 min, then 35 cycles of 95.0°C for 15 s, 60.5°C for 30 s, 72.0°C for 1 min, and a final step at 72.0°C for 3 min. A melt curve was performed from 65.0°C to 95°C (0.5°C/5s) to identify the presence of non-specific amplification products.
2.6.2. 16S rRNA Gene Sequencing
The V4 region of 16S rRNA genes PCT-amplified from DNA extracts was sequenced to characterize the microbial community present on the MFC reactor anodes and the sludge. The sequencing was conducted using a MiSeq sequencer (Illumina) and the V2 chemistry (read length: 2 × 250 bp). Sequence data were analyzed using RStudio with the package “dada2.” The primer sequences were removed. Read 1 and 2 were trimmed respectively at 240 and 190 bp and the sequences with a maximal expected error greater than 2 were removed. After denoising, the sequences were merged (nmismatch = 0, final length = 213.0 ± 0.5bp). 7.5% of the sequences were discarded after the merging step. Amplicon single variants (ASV) were annotated with reference to the rdp database with a minimal bootstrap confidence of 0.8. Following this procedure 95.2% of sequences could be assigned at the Genus level.
Because Geobacter is an electroactive bacteria often present in anodic biofilms and relatively abundant in our samples, the absolute abundance was calculated from the 16S rRNA gene copie values multiplied by the relative abundance of Geobacter. The result was divided by the number of 16S rRNA gene copies of Geobacter (2.5 copies from the ribosomal RNA operon copy number database; Stoddard et al., 2014) in order to convert from 16S rRNA gene copies/μL to cells/μL.
2.7. Statistical Analysis
All calculations and statistics were performed with RStudio (RStudio Team, 2018; R Core Team, 2019). Normalization of residence time distribution and calculations of 4-NP loss were performed using the “pracma” package (Borchers, 2019). Bar charts of the relative abundance of microbial taxa were produced using the package “phyloseq” (McMurdie and Holmes, 2013). Non-metric multi-dimensional scaling (NMDS) analysis from a genus-level contingency table was conducted using the package “vegan” (Oksanen et al., 2019). MANOVA and ANOVA tests were performed using the package “stats” (R Core Team, 2019).
3. Results
3.1. Chemical Conditions Across MFC Reactors
3.1.1. Substrates Concentration
TOC, COD and VFAs were measured at different positions in the tubular reactor to determine substrate utilization through the MFC stages (Figure 2, Table S1). The synthetic wastewater contained 634.3 mg/L of TOC (COD = 1036.0 mg/L). The TOC concentrations decreased partially across the MFC stages, and concentrations in the first, second, third and fourth stage respectively, were 581.8, 494.2, 494.4, and 460.7 mg/L. Even if MFC3 is higher than MFC2, the difference is small and not significant. It is probably due to some disturbances of the flow during the sampling. The same decreasing pattern was observed for the COD concentrations from 1044.0 in MFC1 to 900.0 mg/L in MFC4. An increase of soluble inorganic carbon concentrations was observed across the stages (from 56.88 in MFC1 to 67.76 mg/L in MFC4), showing that some of the organic carbon was completely mineralized. Several VFAs were produced across the MFC stages whereas only acetate was detected in the initial medium at 8.7 mg/L (Table S1). Acetate and propionate were the main VFAs detected with concentrations of 82.6 and 45.3 mg/L respectively in MFC1 to 178.5 and 102.8 mg/L in MFC4. Butyrate, isobutyrate and isovaleric were also detected but in smaller concentrations (<30 mg/L).
Sulfate, nitrite and nitrate were also measured across the MFC stages. Sulfate was abundant in the synthetic wastewater (26.3 mg/L) and decreased to 8.4 mg/L after MFC1 and 4.8 mg/L after MFC4. Nitrite and nitrate were present at lower concentration in the synthetic wastewater, respectively 3.3 and 0.3 mg/L, and remained low after MFC4 (respectively 4.8 and 0.7 mg/L).
3.1.2. Microbial Biomass
The microbial concentration present on the anode of the tubular MFCs was dependent of the difference in the substrate concentration (Figure S2). 1.45 ± 0.83 × 109 16S rRNA gene copies/cm2 were present on the anodic biofilm of MFC1 and only 5.36 ± 1.14 × 108 16S rRNA gene copies/cm2 in MFC4. A considerable amount of biomass sludge, not associated directly with the anode was present in MFC1 of both duplicate reactors (Figure 3). 7.760 ± 0.610 g of wet weight (0.682 ± 0.006 g of dried mass) was recovered from MFC1. Residues of sludge were observed in MFC2 but no sludge was observed in MFC3 and 4 from both replicate tubular reactors. In the MFC1 sludge, the mean microbial abundance was 8.51 ± 5.42 × 109 16S rRNA gene copies/g wet weight of sludge.
3.1.3. Electricity Production
The variations of organic carbon levels influenced the electricity production in different stages of the MFC reactors (Table S2). The maximal power was higher in the first stages than the later stages. Maximal power ranged from 270.4 mW/m2 in MFC1 to 64.6 mW/m2 in MFC4 of reactor I, and from 435.0 mW/m2 in MFC2 to 224.17 mW/m2 in MFC4 in reactor II. The difference in performance between reactors was due to the difference of maximal current density production. The operating voltage under non-toxic conditions, measured before the measurement of maximal power, was similar between reactors and was also higher for the first stages than the later stages ranging from 252.87 mV for MFC1 to 95.90 mV for MFC4 in reactor I, and from 267.92 for MFC2 to 90.47 mV in MFC4 in reactor II.
3.2. Toxicity Detection
The response of MFCs to acute toxicant exposure was assessed with 4-NP (Figure 4). Four concentrations were tested over a 55 min period each (Figure S1).
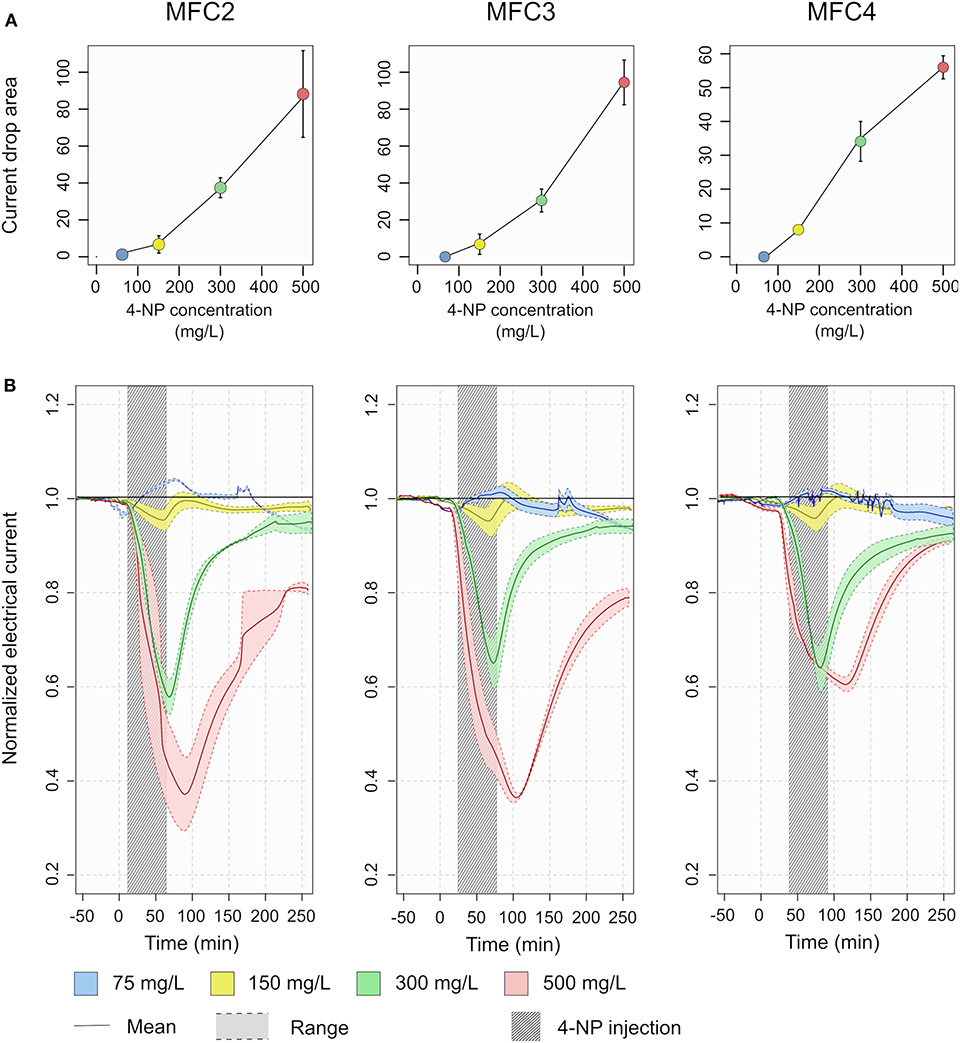
Figure 4. Toxicity detection in MFC2, 3 and 4. (A) The current drop area, measured as normalized current (Inorm) between –50 and 250 min were calculated for each acute toxicity test. (B) The normalized electrical current measured as a function of time during acute toxicity tests for different concentrations of 4-NP. The lines represent the mean of the reactors and the shadded area around the colored lines represent the range. The dark gray shaded rectangle represents the period where the 4-NP was present in the influent medium.
Part way through the experimental period an electrical disconnect between the anode of MFC1 (reactor II) and its current collector wire meant that no voltage could be recorded reliably from this cell. In addition to this, there was considerable biomass sludge occupying both MFC1 cells in the replicate reactors (sec: microbial biomass), thus data from this stage were disregarded for toxicity detection experiments (but are presented in Figure S3).
No response above the margin of error was detectable in output from the MFC reactors with 4-NP at a concentration of 75 mg/L (Figure 4). For the higher concentrations, decreases in current density were observed in each of MFC2, 3 and 4 (Figure 4). From a concentration of 300 mg/L 4-NP, a significant reduction in the normalized current density was observed from 100% to a minimum of 59 ± 5, 66 ± 7, and 65 ± 7% respectively for MFC2, 3 and 4. At a concentration of 500 mg/L 4-NP, the current density decreased to 37 ± 16, 37 ± 2, 61 ± 3% respectively for MFC2, 3 and 4. Even though the maximum response in MFC4 was lower than in MFC2 and 3 for this higher concentration, the current drop areas from MFC2, 3 and 4 were positively correlated with 4-NP concentrations (p-value <10−5, R2 = 0.88) indicating that the MFC response can be used for, at least semi-quantitative detection of toxicity.
When the non-toxic synthetic wastewater feed to the MFC reactors was restored, the current density recovery following exposure to the toxic 4-NP. After 4 h, more than 90% of the output was recovered for all the MFCs for concentrations of 75, 150, and 300 mg/L of 4-NP. Even following exposure to 500 mg/L 4-NP, the current density recovered to more than 75% of its pre-exposure level, showing a good resilience of the biofilms on the MFC anode to the acute exposure to toxic 4-NP.
3.3. Residence Time and Mass Balance of 4-NP
Figure 5A describes the residence time distribution of 4-NP in the reactors. In all acute toxicity tests, the quantity of 4-NP was not completely recovered in the effluent. Only 16.56% was recovered in the acute toxicity test with 150 mg/L 4-NP. The percentage recovered increased to 28.93% with 300 mg/L 4-NP and 47.89% with 500 mg/L 4-NP. It was observed that the concentration in the outlet was much lower than the initial concentration. The concentrations of 4-NP was 43.2± 4.9, 28.5 ± 1.8, 76.4 ± 2.1, and 228.5 ± 8.1 mg/L respectively for the initial concentrations of 75, 150, 300 and 500 mg/L (equating to 4.0, 15.4, 28.4, and 33.1 mg lost in total respectively in each test). The 4-nitrophenol degradation rates are presented on the Figure 5B. In addition, a brown precipitate was observed in the outlet samples after being stored overnight at 4°C. The total recovered mass of the brown precipitate was 16.03 ± 0.54 following the 500 mg/l test, in the same range as the 4-NP lost.
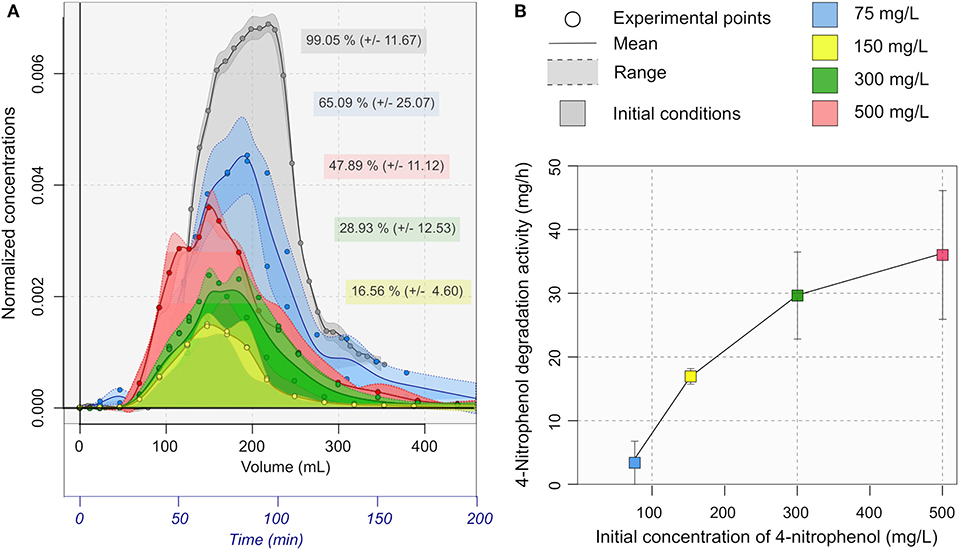
Figure 5. (A) Residence time distribution of 4-NP. The normalized concentrations were expressed as a function of the Time and the cumulative volume in the outlet. The area represents the percentage of 4-NP quantity recovered in the outlet. (B) 4-NP degradation rate in mg/h calculated from the residence time distribution.
3.4. The Fate of 4-Nitrophenol in the Sensor
3.4.1. Biodegradation of 4-Nitrophenol
In order to elucidate the mechanisms involved in the 4-NP transformation in the MFC-based toxicity sensor, acute toxicity event tests were performed in batch incubations. 300 mg/L of 4-NP was injected in two sterile MFCs (MFC-St) and two sterile microcosms (M-St) after 27 days operation/incubation under non-toxic conditions. After 90 min, no decrease of 4-NP concentrations was observed (MFC-St = 309.1 mg/L, M-St = 306.2 mg/L) (Figure 6), indicating no chemical or electrochemical process was involved in 4-NP degradation.
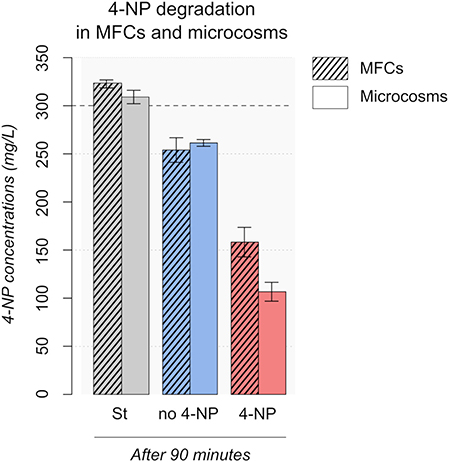
Figure 6. 4-NP degradation in MFCs and microcosms. 300 mg/L 4-NP dashed horizontal line on graph, was added after 25 days of operation. The 4-NP concentrations were measured after 90 min. Footnote: St, Sterile; no 4-NP, no previous exposure to 4-NP; 4-NP, enriched with 50 mg/l 4-NP after 10 days.
The same experiment was conducted with two MFCs acclimated (MFC-4NP) with 50 mg/L 4-NP and two MFCs (MFC-no-4NP) with non-toxic medium. The electricity production before the acute toxicity test is shown in Figure 7. No 4-NP was added to MFC-4NP during the first 10 days to favor the development of an electrogenic biofilm on the anode. After 10 days the current density (MFC-4NP = 28.7 μA/cm2 and MFC-no-4NP = 29.4 μA/cm2) and the maximal power (MFC-4NP = 34.0 ± 8.4 mW/m2 and MFC-no-4NP = 35.0 ± 2.0 mW/m2) were similar in both conditions. 50 mg/L 4-NP was then added to MFC-4NP by replacing 50% of the medium with medium containing 4-NP. Following incubation for 25 days the current density and the maximal power decreased to 15.6 μA/cm2 and 25.0 ± 3.7 mW/m2, whereas the current density (25.0 μA/cm2) and the maximal power (31.7 ± 0.4 mW/m) stayed stable in MFC-no-4NP. During the acclimation period, the color of the medium changed from yellow to clear (Figure S4) indicating degradation of 4-NP. After a total of 26 days, an acute toxicity test was performed by adding 300 mg/L of 4-NP. After 90 min, the 4-NP concentration was respectively 158.2 and 254.0 mg/L in MFC-4NP and MFC-no-4NP. These results show that the 4-NP was degraded by the bacterial community and the degradation activity was higher when the bacterial community was already adapted to 4-NP even at low concentrations. A brown precipitate was observed in the samples a few hours after sampling, suggesting that the 4-NP was transformed to 4-AP which can be polymerized in presence of oxygen.
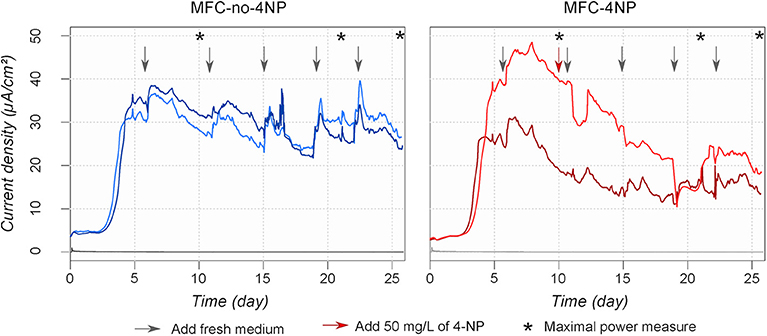
Figure 7. Current density produced by MFCs amended or not in 4-NP. After 10 days 50 mg/L of 4-NP was added only to MFC-4NP (red arrow). The arrows represent the addition of fresh synthetic wastewater amended (red) or not (black) with 50 mg/L of 4-NP. The asterisks show when maximal power density measurements were made.
The same experiment was repeated in simple microcosms with no electrodes under the same conditions (M-4NP and M-no-4NP) in order to determine if the biodegradation activity was due to the electrogenic anodic community or to planktonic bacteria present in the medium. The same results were observed as in the MFCs. After 90 min, the 4-NP concentration decreased to 201.5 and 274.1 mg/L respectively in M-4NP and M-no-4NP and a brown precipitate was observed in the samples a few hours after sampling. So, the 4-NP degradation activity is not attributed specifically to the anodic community in the MFC but to bacteria in the medium. If repetitive acute toxicity event tests were performed (or in a real-world setting multiple events occurred), bacteria able to degrade 4-NP to 4-AP could be selected increasing the 4-NP degradation activity and reducing the sensitivity of toxicity detection.
3.4.2. Chemical Polymerization of 4-Aminophenol
4-Aminophenol (4-AP) has been reported as a major product of 4-NP transformation in anaerobic environments (Gurevich et al., 1993) and polymerization of 4-AP is also known to occur (Thenmozhi et al., 2011, 2014). We therefore hypothesized that the 4-NP loss was due to its reduction to 4-AP and/or its direct or indirect polymerization. Polymerization of 4-NP and 4-AP was tested in anaerobic and aerobic conditions. A brown precipitate was observed only under aerobic conditions with 4-AP but not with 4-NP.
In order to determine if the 4-AP-derived polymer produced under oxic conditions was similar to the precipitate observed during the acute toxicity tests, the solubility of both precipitates in acetone, ethanol, DMSO, HCl, and NaOH was tested. Both precipitates were very soluble in DMSO forming a dark brown solution, and in NaOH (pH = 10) giving a dark purple color. Both were insoluble in HCl (pH = 1) and ethanol, and poorly soluble in acetone.
For the sake of characterizing the polymer, Fourier transformation infrared spectra (FT-IR) of 4-NP, 4-AP and the unknown precipitate were measured (Figure 8). Phenolic compounds are known to have absorbance peaks between 3,200 and 3,600 cm−1 due to the alcohol functional group. Several peaks were observed in 4-AP and 4-NP FT-IR spectra but not in spectra of the precipitate. Amines have an IR absorbance 2,800 cm−1. Two peaks can be observed only in spectra of 4-AP and the precipitate around 2,800 cm−1.
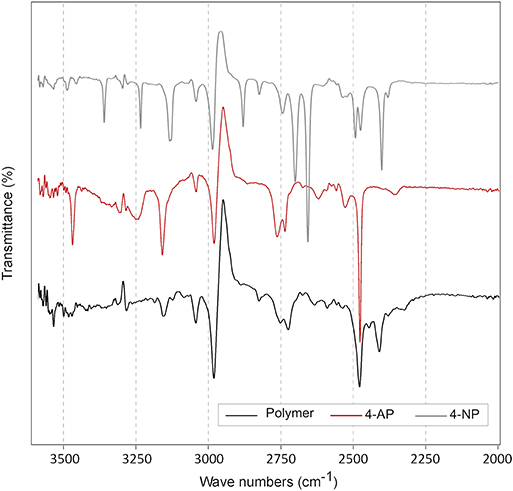
Figure 8. Fourier transform infrared spectra (FT-IR) of 4-AP, 4-NP and the unknown brown precipitate labeled “polymer” in the figure legend. The three compounds were solubilized in DMSO. The transmittance between 2,000 and 3,500 cm−1 were presented in this figure. No differences were observed at lower wave number.
3.5. Microbial Community
Differences between microbial communities from the samples were evaluated by non-metric multidimensional scaling (NMDS) of Bray-Curtis dissimilarity values (Figure 9). Samples were separated into five clusters across one axis. These represented sludge, MFC1, 2, 3, and 4 (MANOVA test: p-value = 0.001, R = 0.75). Methanogenic Archae were relatively more abundant in the sludge (12.32 ± 3.88%) than on the anodes (6.60 ± 1.84%) and were mainly represented by Methanobacteriaceae (5.76 ± 0.42%, main genus: Methanobrevibacter) and Methanomassiliicoccaceae (7.46 ± 1.55%, genus: Methanomassiliicoccus) (Figure 10, Figures S5, S6). Bacteria from the class Clostridia which are likely to be fermenters (Wiegel et al., 2006) were more prevalent in the sludge (12.85 ± 0.86%) than in MFC4 (6.67 ± 1.34%) and comprised a large number of taxa. The major families were Ruminococcaceae (4.56 ± 0.40%), Clostridiales incertae sedis (2.35 ± 1.24%) and Clostridiaceae (2.06 ± 0.46%). Bacteroidia were also relatively more abundant in the sludge (15.29 ± 0.90%) than in MFC4 (4.93 ± 0.51%). The main families present were Porphyromonadaceae (9.48 ± 0.78%, main genus: Parabacteroides and Dysgonomonas) and Bacteroidaceae (4.93 ± 0.47%, main genus: Bacteroides). The last main family significantly more abundant in the sludge than in MFC4 was a family of sulfate-reducing bacteria Desulfovibrionaceae (5.75 ± 0.43%, main genus:Desulfovibrio) (Kuever, 2014).
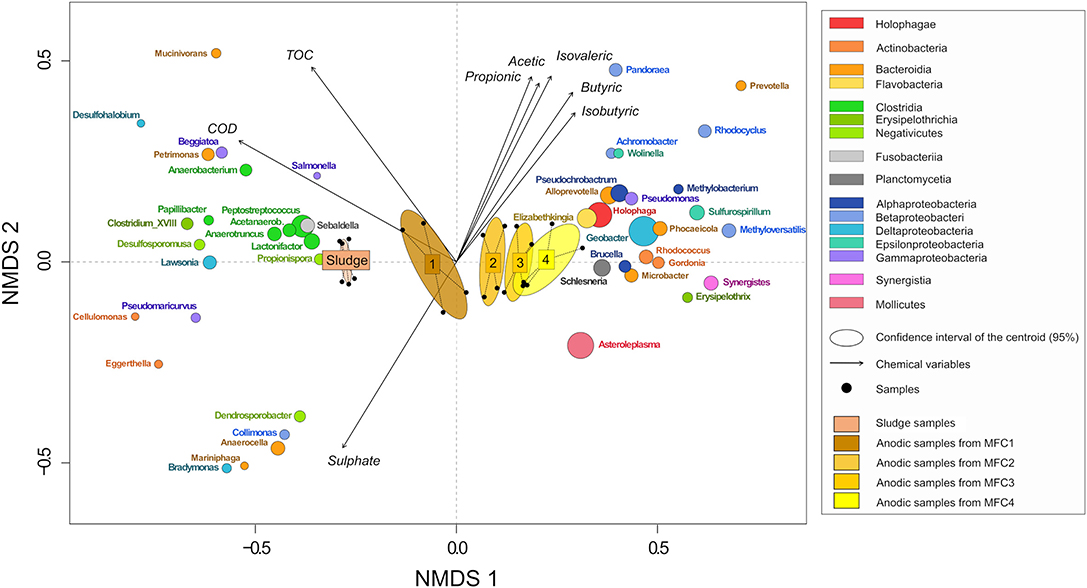
Figure 9. NMDS plot of samples and taxa from bray-curtis dissimilarity matrix. The size of the ellipses for each genus is proportional to its relative abundance in all the samples. Genus with a short vector length or a low relative abundance were removed. Only the more significant environmental variables were kept (stress = 0.035).
At the other extreme, Deltaproteobacteria was relatively the most abundant class in MFC4 (24.49 ± 4.34%) and mainly comprised electroactive bacteria from the genus Geobacter (Koch and Harnisch, 2016) (21.39 ± 4.69% against 0.04 ± 0.01% in sludge and only 2.76 ± 0.85% in MFC1). The absolute abundance of Geobacter spp. was calculated from 16S rRNA gene qPCR data in order to assess if the electrical performances between MFCs could be explained by the abundance of Geobacter spp.. The abundance was respectively 3.25 ± 1.72 × 106, 1.11 ± 0.86 × 107, 8.64 ± 1.80 × 106, and 8.85 ± 0.64 × 106 cells/μL in MFC1, 2, 3, and 4. Geobacter spp. abundance was slightly higher in MFC4 than MFC1 and was not positively correlated to electrical performances. The other main classes more prevalent in MFC4 were Acidobacteria, Holophagae (genus: Holophaga) and Mollicutes (genus: Asteroleplasma).
Some taxa were relatively abundant and were present everywhere without significant differences between different stages of the sensor. These included Synergistiaceae (15.72 ± 2.50%, main genus: Cloacibacillus), Flavobacteriaceae (8.95 ± 2.08%, main genus: Spongimonas), Enterobacteriaceae (3.35 ± 1.24%, main genus: Proteus, Providencia, Morganella). The family Veillonellaceae (main genus: Anaerospora, Anaeromusa) was more prevalent on the anode (12.65 ± 1.27%) than in the sludge (6.72 ± 0.62%).
4. Discussion
4.1. Biodegradation of 4-NP
Anaerobic and aerobic biodegradation of nitrophenols has been reported in several studies (Gorontzy et al., 1993; Gurevich et al., 1993; Haderlein and Schwarzenbach, 1995; Spain, 1995; Karim and Gupta, 2001; She et al., 2005). Under anaerobic conditions, it was reported that 4-NP was reduced in 4-AP via fortuitous reactions (Spain, 1995). The effect and fate of 4-NP was tested in single chamber MFC operated in batch mode and in a flow-through multi-stage MFC sensor and in microcosms identical to the single chamber MFCs except they were not connected to an electrical circuit. These studies demonstrated that 4-NP was degraded under both anoxic and oxic conditions. In the batch MFCs and microcosms, the presence of live bacteria was necessary for 4-NP degradation. Similar degradation rates were observed in MFCs and microcosms showing that 4-NP degradation was not directly linked to electrogenic activity of the anodic biofilm. The microbial community present in sludge obtained from the 1st stage MFCs was mainly composed of methanogens (12.32 ± 3.88%) fermenters from the class Clostridia (12.85 ± 0.86%) (Wiegel et al., 2006) and sulfate-reducing bacteria Desulfovibrio (5.75 ± 0.43%) (Kuever, 2014). Microorganisms from these taxa have been previously reported to degrade 4-NP (Gorontzy et al., 1993). It was shown that the reduction of 4-NP can be catalyzed by different types of methanogenic archaea, 4 species of Desulfovibrio and one species of Clostridium. In addition, it was shown that H2S (produced by sulfate-reducing bacteria such as Desulfovibrio sp.) can reduce aromatic nitro-substituted compounds (Haderlein and Schwarzenbach, 1995). Under anoxic conditions, it has been reported that 4-NP can be reduced to 4-aminophenol (4-AP) via biotic reactions (Spain, 1995). In the study of Gurevich et al. (1993), ~60% of 4-NP was converted to 4-AP in 1 h, by a microbial community from sheep rumen. In our study, conversion of 4-NP (measured in effluent samples) during 55 min acute toxicity event tests was similar at 50.54 ± 7.62% of 500 mg/L 4-NP transformed.
In our study, a brown precipitate was observed in samples from acute 4-NP toxicity tests exposed to air after only several minutes exposure to oxic conditions. We hypothesize that this was due to oxidative polymerization of 4-AP. The diversity of compounds present in the synthetic sewage which is a complex medium (peptone, meat extract and urea) and the abundance of sulfate (26.3 ± 0.7 mg/L in the initial medium) could also favor this reaction. Furthermore, it has been shown that 4-AP can polymerize in presence of oxidizing agents such as hydrogen peroxide or ammonium persulfate (APS) (Duan et al., 2014; Thenmozhi et al., 2014). Thenmozhi et al. (2014) observed the chemical synthesis of 4-AP in acidic conditions and Duan et al. (2014) used horseradish peroxidase to catalyze 4-AP polymerization at pH 7 in the presence of hydrogen peroxide. In our study, the brown precipitate was observed only several minutes after sampling and in aerobic conditions and the oxygen could be the oxidative agent necessary to the reaction.
In order to determine if bacteria degrading 4-NP are selected during repeated exposure of the sensor biofilm to acute toxicity events, MFC-4NP were fed medium containing 50 mg/L of 4-NP. MFC-4NP showed higher levels of 4-NP degradation compared to MFC-no-4NP which had not experienced prior exposure to 4-NP. 47.26 ± 7.25% of the 4-NP was degraded in 90 min in MFC-4NP and only 15.35 ± 6.03% in MFC-no-4NP. Even if the toxicity events are acute, repeated toxicity events could lead to the selection of bacteria resistant to the toxic compounds and changes in the calibration of the biosensors. This limitation can be overcome by re-calibration of the sensor after a specified number of toxicity events, however it might result in reduced sensitivity of the sensor.
4.2. Toxicity Detection
While the sensor can reliably detect the presence of a toxic material in the sensor influent, and a reliable calibration of the sensor response to 4-NP at different concentrations was obtained with the sensor configuration tested here, quantification of a particular toxicant would be impractical in real-world conditions. This is in part because the sensor response is not selective and different toxicants at the same concentration may lead to a different response in the sensor, moreover if other toxicants are present then the response will be a function of the different toxicities and concentration of the co-occurring toxicants and the contribution of each to the sensor response, would not be possible to deconvolute. In some respects this is a strength of the sensor as it measures toxicity independent of the particular toxic material present and the sensor would be capable of providing an early warning system alerting process control operators about the toxicity level of an influent. The quantification of current loss could be correlated to a level of toxicity rather than a precise quantity of a specific pollutant. This level or threshold of toxicity can be due to the intrinsic property of the toxic compounds, their concentrations or a cumulative effect of multiple toxicants. Yi et al. showed that the inhibition effect of a toxicant mixture can be comparable to the addition of the inhibition effect from each isolated toxic compound (Yi et al., 2019). In this study, increasing levels of toxicity have been simulated by the increase of 4-NP concentrations. A positive proportional correlation was observed between the 4-NP concentrations and the current drop area showing that the response is proportional to the toxicity level.
The detection of 4-NP was possible for 4-NP concentrations superior or equal to 150 mg/L which is similar to the 4-NP concentration detected by the toxicity respirometry test (EC50 ~ 150 mg/L) (Ricco et al., 2004). In addition, several studies have shown that toxicity test by respirometry are better to characterize the toxicity of effluent in wastewater treatment plant than MicrotoxⓇ test (Gutiérrez et al., 2002; Ricco et al., 2004; Polo et al., 2011). It is due to the fact that MicrotoxⓇ test used a pure culture leading to an overestimation of the toxicity. For example, Ricco et al. observed a EC50 value of only 8.76 mg/L for 4-NP using the MicrotoxⓇ test (Ricco et al., 2004). In opposite, the respirometry test use a mix culture of activated sludge which is more closed to the conditions present in wastewater. MFC as biosensor could offer the same characteristic than respirometry test but with a more direct method of measure.
The response time was fast and seems dependent only of the RTD. In the recovery part, even if the 4-NP concentrations decreased and become under the detection threshold of the spectrophotometer the current need more time to recover. More the toxicity level was high and less the biofilm was resilient. However, biofilms have shown good resilience to the acute toxic event. After 4 h, more than 90% was recovered for a concentration of 75, 150 and 300 mg/L of 4-NP. Even for the toxic event at 500 mg/L, more than 75% of the current density was recovered.
4.3. Specificity of a Multi-Stage MFC as Toxicity Biosensor
In this study, a multi-stage MFC was used as reactor for the detection of acute toxic events. The multi-stage MFCs offer the advantage of measuring BOD concentration over a large range (Spurr et al., 2018) exhibiting a positive correlation between current generation and BOD while also allowing the presence of toxic compounds to be detected due to loss of current. The large range of BOD concentrations was possible due to the multi-stage array able to use unconsumed substrate in downstream MFCs (as previously reported by Spurr et al., 2018). The hydraulic connection between MFCs leads to a gradient of chemical conditions through the MFC stages which modifies the electrochemical performance of each MFC and so could modify the detection of toxic compound. In this study, TOC concentration decreased along the reactors leading to a decrease of current generation and bacterial density of the anodic biofilms (Figure S2). The composition of the organic carbon was also modified. Acetate and propionate concentrations increased along the reactor leading to stronger selection of Geobacter spp. and a lower proportion of fermenters such as Clostridium spp. in MFC4. It is often reported that MFCs with a higher relative abundance of Geobacter spp. have higher current generation (Sun et al., 2011; Paitier et al., 2017). However, in our study, a minor difference in abundance of Geobacter spp. was observed between stages. Thus in the sensor the current generation was dependent only on the TOC concentrations modulated by the presence of toxins.
By contrast with substrates for MFCs, poorly degradable toxic compounds should maintain at a stable concentration along the MFC stages in the sensor leading to a similar inhibition response at each stage. This pattern of signal loss is a distinctive feature distinguishing the presence of a toxic compound from a decrease of BOD. The detection of 4-NP was observed from MFC2 to MFC4. The signal was similar in each of the MFC stages in the sensor except when the sensor was exposed to the highest 4-NP concentration used. At 500 mg/L, the loss of current was more extensive in MFC2 and 3 than MFC4. It could be due to the biodegradation of 4-NP and so a decrease of 4-NP concentrations along the reactor. It results that three conditions should be considered for the application of a multi-stage MFC BOD/toxicity biosensor. A decrease of BOD would lead to a progressive decrease of the current from the first stage to the last one. The presence of a poorly degradable toxic compound would lead to a similar inhibition response at each stage. Finally, a readily degradable toxic compound such as 4-NP would lead to the highest decrease of current in the first stage, following by a progressive increase of the current in the next stages until to come back to the initial current. A multi-stage MFC biosensor could give online information about the presence of a toxicant but also about the biodegradability of this toxicant which is critical in the analysis of the potential risk of discharging a toxicant in the biological reactor of a wastewater treatment plant.
4.4. Conclusion
In this study, different levels of toxicity in a synthetic wastewater were simulated by increasing 4-NP concentrations. A positive correlation was observed between the 4-NP concentrations and the MFC current drop area showing that the response is proportional to the toxicity level. In addition, the anodic biofilms exhibited good resilience to acute toxic event with a recovery of at least 75% of the current density for a toxic event at 500 mg/L after 4 h. However, repeated exposure to a particular toxic compound could lead to the selection of bacteria resistant to the compound and change the sensitivity/calibration of the biosensor. In this study, a maximal 4-NP degradation activity of 36 mg/h was observed. These difficulties could be overcome by re-calibration after a predetermined amount of toxicant exposure. The multi-stage MFC biosensor could provide an early warning system capable of alerting process control operators about the level of toxicity in influents entering a wastewater treatment plant allowing early intervention to prevent inhibition and reduced treatment performance.
Data Availability Statement
Data and metadata supporting this publication is available at: http://dx.doi.org/10.25405/data.ncl.11676423.
Author Contributions
IH, MS, and EY contributed conception of the study. MS, IH, and AG contributed design of the study. GP and HB contributed conception and production of multi-stage MFCs used in the study. AG performed the experimental work and the statistical analysis and wrote the first draft of the manuscript. AG, MS, and IH wrote sections of the manuscript. All authors contributed to manuscript revision, read and approved the submitted version.
Funding
Our research on bioelectrochemical systems for toxicity sensing applications has been supported by the NERC (Follow on Fund, NE/R009473/1; Resource Recovery from Waste Programme NE/L01422X/1) and EPSRC (SUPERGEN Biological Fuel Cells Consortium; EP/H019480/1).
Conflict of Interest
The authors declare that the research was conducted in the absence of any commercial or financial relationships that could be construed as a potential conflict of interest.
Supplementary Material
The Supplementary Material for this article can be found online at: https://www.frontiersin.org/articles/10.3389/fenvs.2020.00005/full#supplementary-material
References
Agency for Toxic Substances and Disease Registry (ATSDR). (1992). Toxicological Profile for Nitrophenols: 2-Nitrophenol and 4-Nitrophenol. Atlanta, GA: Department of Health and Human Services, Public Health Service.
Abrevaya, X. C., Sacco, N. J., Bonetto, M. C., Hilding-Ohlsson, A., and Cortón, E. (2015). Analytical applications of microbial fuel cells. Part II: Toxicity, microbial activity and quantification, single analyte detection and other uses. Biosensors Bioelectron. 63, 591–601. doi: 10.1016/j.bios.2014.04.053
Adekunle, A., Raghavan, V., and Tartakovsky, B. (2019a). On-line monitoring of heavy metals-related toxicity with a microbial fuel cell biosensor. Biosensors Bioelectron. 132, 382–390. doi: 10.1016/j.bios.2019.03.011
Callbeck, C. M., Sherry, A., Hubert, C. R. J., Gray, N. D., Voordouw, G., and Head, I. M. (2013). Improving PCR efficiency for accurate quantification of 16s rRNA genes. J. Microbiol. Methods 93, 148–152. doi: 10.1016/j.mimet.2013.03.010
Chang, I. S., Jang, J. K., Gil, G. C., Kim, M., Kim, H. J., Cho, B. W., et al. (2004). Continuous determination of biochemical oxygen demand using microbial fuel cell type biosensor. Biosensors Bioelectron. 19, 607–613. doi: 10.1016/S0956-5663(03)00272-0
Chouler, J., Cruz-Izquierdo, Á., Rengaraj, S., Scott, J. L., and Di Lorenzo, M. (2018). A screen-printed paper microbial fuel cell biosensor for detection of toxic compounds in water. Biosensors Bioelectron. 102, 49–56. doi: 10.1016/j.bios.2017.11.018
Di Lorenzo, M., Curtis, T. P., Head, I. M., and Scott, K. (2009a). A single-chamber microbial fuel cell as a biosensor for wastewaters. Water Res. 43, 3145–3154. doi: 10.1016/j.watres.2009.01.005
Di Lorenzo, M., Curtis, T. P., Head, I. M., Velasquez-Orta, S. B., and Scott, K. (2009b). A single chamber packed bed microbial fuel cell biosensor for measuring organic content of wastewater. Water Sci. Techn. 60, 2879–2887. doi: 10.2166/wst.2009.699
Di Lorenzo, M., Thomson, A. R., Schneider, K., Cameron, P. J., and Ieropoulos, I. (2014). A small-scale air-cathode microbial fuel cell for on-line monitoring of water quality. Biosensors Bioelectron. 62, 182–188. doi: 10.1016/j.bios.2014.06.050
Duan, H., Zheng, K., Zhang, L., and Cui, Y. (2014). Synthesis of poly (4-aminophenol) by horseradish peroxidase and the evaluation of its adsorptivity for silver ions. J. Appl. Polym. Sci. 131. doi: 10.1002/app.40367
Gerike, P., and Fischer, W. (1979). A correlation study of biodegradability determinations with various chemicals in various tests. Ecotoxicol. Environ. Safety 3, 159–173.
Gorontzy, T., Küver, J., and Blotevogel, K.-H. (1993). Microbial transformation of nitroaromatic compounds under anaerobic conditions. Microbiology 139, 1331–1336.
Gurevich, P., Oren, A., Sarig, S., and Henis, Y. (1993). Reduction of aromatic nitro compounds in anaerobic ecosystems. Water Sci. Techn. 27, 89–96.
Gutiérrez, M., Etxebarria, J., and De Las Fuentes, L. (2002). Evaluation of wastewater toxicity: comparative study between microtox® and activated sludge oxygen uptake inhibition. Water Res. 36, 919–924. doi: 10.1016/S0043-1354(01)00299-8
Haderlein, S. B., and Schwarzenbach, R. P. (1995). “Environmental processes influencing the rate of abiotic reduction of nitroaromatic compounds in the subsurface,” in Biodegradation of Nitroaromatic Compounds, Environmental Science Research, ed J. C. Spainpages (Boston, MA: Springer), 199–225.
Ingerslev, F., and Nyholm, N. (2000). Shake-flask test for determination of biodegradation rates of 14c-labeled chemicals at low concentrations in surface water systems. Ecotoxicol. Environ. Safety 45, 274–283. doi: 10.1006/eesa.1999.1877
Jiang, Y., Liang, P., Liu, P., Bian, Y., Miao, B., Sun, X., et al. (2016). Enhancing signal output and avoiding bod/toxicity combined shock interference by operating a microbial fuel cell sensor with an optimized background concentration of organic matter. Int. J. Mol. Sci. 17:1392. doi: 10.3390/ijms17091392
Jiang, Y., Liang, P., Zhang, C., Bian, Y., Yang, X., Huang, X., et al. (2015). Enhancing the response of microbial fuel cell based toxicity sensors to cu (ii) with the applying of flow-through electrodes and controlled anode potentials. Bioresource Techn. 190, 367–372. doi: 10.1016/j.biortech.2015.04.127
Johnson, B. T. (2005). “Microtox® acute toxicity test,” in Small-scale Freshwater Toxicity Investigations: Toxicity Test Methods, eds C. Blaise, and J.-F. Férard (Dordrecht: Springer Netherlands), 69–105.
Karim, K., and Gupta, S. K. (2001). Biotransformation of nitrophenols in upflow anaerobic sludge blanket reactors. Bioresource Techn. 80, 179–186. doi: 10.1016/S0960-8524(01)00092-X
Kim, B. H., Chang, I. S., Cheol Gil, G., Park, H. S., and Kim, H. J. (2003). Novel BOD (biological oxygen demand) sensor using mediator-less microbial fuel cell. Biotechnol. Lett. 25, 541–545. doi: 10.1023/A:1022891231369
Kim, B. H., Chang, I. S., and Moon, H. (2006a). Microbial fuel cell-type biochemical oxygen demand sensor. Studies 3. 10, 1–12.
Kim, J. R., Boghani, H. C., Amini, N., Aguey-Zinsou, K.-F., Michie, I., Dinsdale, R. M., et al. (2012). Porous anodes with helical flow pathways in bioelectrochemical systems: The effects of fluid dynamics and operating regimes. J. Power Sources 213, 382–390. doi: 10.1016/j.jpowsour.2012.03.040
Kim, M., Hyun, M. S., Gadd, G. M., and Kim, H. J. (2007). A novel biomonitoring system using microbial fuel cells. J. Environ. Monitor. 9, 1323–1328. doi: 10.1039/b713114c
Kim, M., Park, H. S., Jin, G. J., Cho, W. H., Lee, D. K., Hyun, M. S., et al. (2006b). “A novel combined biomonitoring system for bod measurement and toxicity detection using microbial fuel cells,” in SENSORS, 2006 IEEE (IEEE), 1247–1248.
King, S. T., Sylvander, M., Kheperu, M., Racz, L., and Harper, W. F. (2014). Detecting recalcitrant organic chemicals in water with microbial fuel cells and artificial neural networks. Sci. Total Environ. 497–498, 527–533. doi: 10.1016/j.scitotenv.2014.07.108
Koch, C., and Harnisch, F. (2016). Is there a specific ecological niche for electroactive microorganisms? ChemElectroChem 3, 1282–1295. doi: 10.1002/celc.201600079
Kong, Z., Vaerewijck, M., and Verstraete, W. (1996). On-line stBOD measurement and toxicity control of wastewaters with a respirographic biosensor. Environ. Techn. 17, 399–406. doi: 10.1080/09593331708616399
Kuever, J. (2014). “The family desulfovibrionaceae,” in The Prokaryotes: Deltaproteobacteria and Epsilonproteobacteria eds E. Rosenberg, E. F. DeLong, S. Lory, E. Stackebrandt, and F. Thompson (Berlin; Heidelberg: Springer Berlin Heidelberg), 107–133.
Liu, B., Lei, Y., and Li, B. (2014). A batch-mode cube microbial fuel cell based “shock” biosensor for wastewater quality monitoring. Biosensors Bioelectron. 62, 308–314. doi: 10.1016/j.bios.2014.06.051
Liu, Z., Liu, J., Zhang, S., Xing, X.-H., and Su, Z. (2011). Microbial fuel cell based biosensor for in situ monitoring of anaerobic digestion process. Bioresource Techn. 102, 10221–10229. doi: 10.1016/j.biortech.2011.08.053
McMurdie, P. J., and Holmes, S. (2013). phyloseq: an r package for reproducible interactive analysis and graphics of microbiome census data. PLoS ONE 8:e61217. doi: 10.1371/journal.pone.0061217
Michie, I. S., Kim, J. R., Dinsdale, R. M., Guwy, A. J., and Premier, G. C. (2014). The influence of anodic helical design on fluid flow and bioelectrochemical performance. Bioresource Techn. 165, 13–20. doi: 10.1016/j.biortech.2014.03.069
Nyholm, N., Lindgaard-Jørgensen, P., and Hansen, N. (1984). Biodegradation of 4-nitrophenol in standardized aquatic degradation tests. Ecotoxicol. Environ. Safety 8, 451–470.
OECD (2001). Test No. 303: Simulation Test? Aerobic Sewage Treatment? A: Activated Sludge Units; B: Biofilms, OECD Guidelines for the Testing of Chemicals, Section 3. Paris: OECD Publishing. doi: 10.1787/9789264070424-en
Oksanen, J., Blanchet, F. G., Friendly, M., Kindt, R., Legendre, P., McGlinn, D., et al. (2019). vegan: Community Ecology Package. R package version 2.5-5.
Paitier, A., Godain, A., Lyon, D., Haddour, N., Vogel, T. M., and Monier, J.-M. (2017). Microbial fuel cell anodic microbial population dynamics during mfc start-up. Biosensors Bioelectron. 92, 357–363. doi: 10.1016/j.bios.2016.10.096
Patil, S., Harnisch, F., and Schröder, U. (2010). Toxicity response of electroactive microbial biofilms-a decisive feature for potential biosensor and power source applications. Chemphyschem. 11, 2834–2837. doi: 10.1002/cphc.201000218
Polo, A., Tobajas, M., Sanchis, S., Mohedano, A., and Rodríguez, J. (2011). Comparison of experimental methods for determination of toxicity and biodegradability of xenobiotic compounds. Biodegradation 22, 751–761. doi: 10.1007/s10532-010-9448-7
Quevauviller, P., Thomas, O., and Beken, A. V. D. (2007). Wastewater Quality Monitoring and Treatment. John Wiley & Sons. doi: 10.1002/9780470058725
R Core Team (2019). R: A Language and Environment for Statistical Computing. Vienna: R Foundation for Statistical Computing.
Ricco, G., Tomei, M. C., Ramadori, R., and Laera, G. (2004). Toxicity assessment of common xenobiotic compounds on municipal activated sludge: comparison between respirometry and microtox®. Water Res. 38, 2103–2110. doi: 10.1016/j.watres.2004.01.020
Saikaly, P. E., and Oerther, D. B. (2011). Diversity of dominant bacterial taxa in activated sludge promotes functional resistance following toxic shock loading. Microb. Ecol. 61, 557–567. doi: 10.1007/s00248-010-9783-6
She, Z., Gao, M., Jin, C., Chen, Y., and Yu, J. (2005). Toxicity and biodegradation of 2,4-dinitrophenol and 3-nitrophenol in anaerobic systems. Process Biochem. 40, 3017–3024. doi: 10.1016/j.procbio.2005.02.007
Shen, Y., Wang, M., Ng, H., and Chang, I. (2013). Effect of shear rate on the response of microbial fuel cell toxicity sensor to cu(ii). Bioresour. Technol. 136, 707–710. doi: 10.1016/j.biortech.2013.02.069
Spurr, M. W., Yu, E. H., Scott, K., and Head, I. M. (2018). Extending the dynamic range of biochemical oxygen demand sensing with multi-stage microbial fuel cells. Environm. Sci. Water Res. Techn. 4, 2029–2040. doi: 10.1039/C8EW00497H
Spurr, M. W. A. (2017). Microbial fuel cell-based biosensors for estimation of biochemical oxygen demand and detection of toxicity. Ph.D. thesis, Newcastle University.
Spurr, M. W. A., Yu, E. H., Scott, K., and Head, I. M. (2020). A microbial fuel cell sensor for unambiguous measurement of organic loading and definitive identification of toxic influents. Environ. Sci.: Water Res. Technol. doi: 10.1039/C9EW00849G. [Epub ahead of print].
Stein, N. E., Hamelers, H. V., and Buisman, C. N. (2010). Stabilizing the baseline current of a microbial fuel cell-based biosensor through overpotential control under non-toxic conditions. Bioelectrochemistry 78, 87–91. doi: 10.1016/j.bioelechem.2009.09.009
Stoddard, S. F., Smith, B. J., Hein, R., Roller, B. R., and Schmidt, T. M. (2014). rrnDB: improved tools for interpreting rRNA gene abundance in bacteria and archaea and a new foundation for future development. Nucleic Acids Res. 43, D593–D598. doi: 10.1093/nar/gku1201
Sun, J.-Z., Peter Kingori, G., Si, R.-W., Zhai, D.-D., Liao, Z.-H., Sun, D.-Z., et al. (2015). Microbial fuel cell-based biosensors for environmental monitoring: a review. Water Sci. Techn. 71, 801–809. doi: 10.2166/wst.2015.035
Sun, Y., Wei, J., Liang, P., and Huang, X. (2011). Electricity generation and microbial community changes in microbial fuel cells packed with different anodic materials. Bioresource Techn. 102, 10886–10891. doi: 10.1016/j.biortech.2011.09.038
Tan, Y. C., Kharkwal, S., Chew, K. K. W., Alwi, R., Mak, S. F. W., and Ng, H. Y. (2018). Enhancing the robustness of microbial fuel cell sensor for continuous copper (ii) detection against organic strength fluctuations by acetate and glucose addition. Bioresource Techn. 259, 357–364. doi: 10.1016/j.biortech.2018.03.068
Thenmozhi, G., Arockiasamy, P., and Santhi, R. J. (2014). Isomers of poly aminophenol: chemical synthesis, characterization, and its corrosion protection aspect on mild steel in 1 m hcl. Int. J. Electrochem., 2014:961617. doi: 10.1155/2014/961617
Thenmozhi, G., JayaKumar, D., Gopalswamy, M., and Raj, R. (2011). Synthesis, characterisation and biological applications of conducting poly (p- amino phenol) and its nano compound. Der Pharma Chemica 3, 116–126. Available online at: https://www.derpharmachemica.com/archive/dpc-volume-3-issue-4-year-2011.html
Toräng, L., and Nyholm, N. (2005). Biodegradation rates in adapted surface water can be assessed following a preadaptation period with semi-continuous operation. Chemosphere 61, 1–10. doi: 10.1016/j.chemosphere.2005.03.040
US PHS. (1992). Toxicological profile for nitrophenols: 2-nitrophenol, 4-nitrophenol. Tech. Rep. Agency for Toxic Substances and Disease Registry, US Public Health Service.
Wiegel, J., Tanner, R., and Rainey, F. A. (2006). “An introduction to the family clostridiaceae,” in The Prokaryotes: Volume 4: Bacteria: Firmicutes, Cyanobacteria, eds M. Dworkin, S. Falkow, E. Rosenberg, K.-H. Schleifer, and E. Stackebrandt (New York, NY: Springer US), 654–678.
Wu, L.-C., Wang, G.-H., Tsai, T.-H., Lo, S.-Y., Cheng, C.-Y., and Chung, Y.-C. (2019). Three-stage single-chambered microbial fuel cell biosensor inoculated with exiguobacterium aestuarii yc211 for continuous chromium (vi) measurement. Sensors 19:1418. doi: 10.3390/s19061418
Wu, W., Lesnik, K. L., Xu, S., Wang, L., and Liu, H. (2014). Impact of tobramycin on the performance of microbial fuel cell. Microbial Cell Factor. 13:91. doi: 10.1186/s12934-014-0091-6
Xiao, Y., Araujo, C. D., Sze, C. C., and Stuckey, D. C. (2015). Toxicity measurement in biological wastewater treatment processes: A review. J. Hazard. Mater. 286, 15–29. doi: 10.1016/j.jhazmat.2014.12.033
Xu, Z., Liu, Y., Williams, I., Li, Y., Qian, F., Zhang, H., et al. (2016). Disposable self-support paper-based multi-anode microbial fuel cell (pmmfc) integrated with power management system (pms) as the real time “shock” biosensor for wastewater. Biosensors Bioelect. 85, 232–239. doi: 10.1016/j.bios.2016.05.018
Yi, Y., Xie, B., Zhao, T., Li, Z., Stom, D., and Liu, H. (2019). Effect of external resistance on the sensitivity of microbial fuel cell biosensor for detection of different types of pollutants. Bioelectrochemistry 125, 71–78. doi: 10.1016/j.bioelechem.2018.09.003
Keywords: microbial fuel cells (MFCs), biosensors, multi-stage, toxicity, wastewater, nitrophenol, aminophenol, bioelectrochemistry
Citation: Godain A, Spurr MWA, Boghani HC, Premier GC, Yu EH and Head IM (2020) Detection of 4-Nitrophenol, a Model Toxic Compound, Using Multi-Stage Microbial Fuel Cells. Front. Environ. Sci. 8:5. doi: 10.3389/fenvs.2020.00005
Received: 16 October 2019; Accepted: 09 January 2020;
Published: 31 January 2020.
Edited by:
Alberto Tiraferri, Politecnico di Torino, ItalyReviewed by:
Pierangela Cristiani, Ricerca Sul Sistema Energetico, ItalyXin Wang, Nankai University, China
Copyright © 2020 Godain, Spurr, Boghani, Premier, Yu and Head. This is an open-access article distributed under the terms of the Creative Commons Attribution License (CC BY). The use, distribution or reproduction in other forums is permitted, provided the original author(s) and the copyright owner(s) are credited and that the original publication in this journal is cited, in accordance with accepted academic practice. No use, distribution or reproduction is permitted which does not comply with these terms.
*Correspondence: Alexiane Godain, YWdvZGFpbkBnbWFpbC5jb20=