- 1Global Lands, The Nature Conservancy, Fort Collins, CO, United States
- 2The Woods Hole Research Center, Falmouth, MA, United States
- 3Global Lands, The Nature Conservancy, Arlington, VA, United States
- 4Conservation International, Arlington, VA, United States
Meeting climate mitigation and sustainable development goals requires rapid increases in both renewable energy development and carbon storage in ecosystems. If sited with the sole goal of maximizing production, renewable energy may negatively impact biodiversity and carbon storage. Here, we evaluated the potential unintended environmental consequences of this type of business-as-usual development scenario. We spatially allocated land-based, utility-scaled wind and solar energy needed to achieve emission reduction goals from nationally determined contributions under the Paris Climate Agreement. Siting was conducted at 1-km resolution and followed a scenario where on-shore wind, concentrated solar power, and photovoltaic solar renewable energy developments were located where wind and solar resources were highest. Once sited, we evaluated the potential losses of natural lands, Key Biodiversity Areas (KBAs), threatened and endangered species, and carbon emissions. Over 11 million ha of natural lands can be lost, releasing almost 415 million tons of carbon storage, which equals 8.6% of overall Paris Agreement emission reduction goals. Globally we found that over 3.1 million ha of KBAs and ranges of 1,574 threatened and endanger species could be impacted, with the highest number of impacted species in the tropical countries of Indonesia (282), Malaysia (273), and Thailand (253). Avoiding land-based emissions through improved renewable energy siting can reduce these losses, potentially saving $47.5–$155.9 billion USD based on social carbon costs. Consideration of these impacts will help reduce investor risk to facilitate a timely transition to a low-carbon economy.
Introduction
Humanity is facing two major existential challenges (Ripple et al., 2017): increasing demand for natural resources for a human population that may exceed 9 billion by 2050 (Crist et al., 2017), and global warming that increases the frequency of environmental disasters and causes economic damages (Burke et al., 2018). In response to these challenges, countries signed the Paris Climate Agreement (PCA) to keep global warming to <2°C below pre-industrial levels, through their “Nationally Determined Contributions” (NDCs) that specify greenhouse gas (GHG) emission reduction targets (United Nations, 2015a). Concurrently, countries committed to a new agenda for Sustainable Development (United Nations, 2015b) that aims to implement 17 Sustainable Development Goals (SDGs) by 2030, including efforts to advance affordable and clean energy access and to protect natural lands and biodiversity (Fuso-Nerini et al., 2017). Implementing PCA and SDG goals presents a complex challenge that requires policymakers to think systematically about the potential unintended negative environmental consequences (Fuso-Nerini et al., 2017; Smith et al., 2019).
Renewable energy requires significant land areas, in some cases several fold greater than conventional energy sources (Kiesecker and Naugle, 2017). Historically, renewable energy development has occurred in areas with the highest resource potential (Kiesecker et al., 2011; Oakleaf et al., 2019) and has impacted natural lands (e.g., wind development in the U.S. Great Plains, Fargione et al., 2012). Clearing natural land for energy development removes and fragments habitat resulting in species declines, and removes aboveground carbon storage resulting in increased emissions (e.g., Diffendorfer and Compton, 2014; Allred et al., 2015; Moreira, 2019). If renewable energy proceeds in this manner it could counteract its expected benefits. The potential for land use conflicts is high, given that a 9-fold increase in renewable energy generation (9,017 TWh) is needed to meet PCA goals (Figure 1C, Baruch-Mordo et al., 2019). Others have assessed impacts associated with renewable energy development for individual countries (McDonald et al., 2009; Arent et al., 2014) or globally for biodiversity impacts (Santangeli et al., 2016). Yet to date, no global assessments have evaluated how the projected increase in renewable energy under PCA goals will affect both biodiversity and terrestrial carbon storage, or grounded such analyses in country level policy commitments. To address this gap, we present a spatially explicit forecast of wind and solar energy expansion under the PCA, and explore the biodiversity and carbon storage consequences of maximizing exploitation of solar and wind energy resources to meet PCA goals. We used estimates of energy production needed to meet PCA emission reduction targets for 109 countries (representing 83% of global terrestrial lands, and 92% of global GHG emissions) whose NDC emissions goals could be attributed to electricity and heat generation from fossil fuels (World Bank, 2017; Baruch-Mordo et al., 2019). We calculated the area needed to meet NDC energy targets within each country and estimated the natural land that would be cleared if development focuses solely on maximizing resource potential. We then spatially assessed impacts of land clearing on biodiversity (km2 loss of Key Biodiversity Areas and number of threatened and endangered species potentially impacted) and carbon storage (tons of stored carbon expressed also as emission loss). Because emissions from land clearing can create a carbon deficit in meeting PCA goals, and because it is essential to meet peak carbon emissions in the next 2–3 years (Figueres et al., 2017), we calculated the number of years required to repay such losses if renewables are sited without considering land-based carbon values.
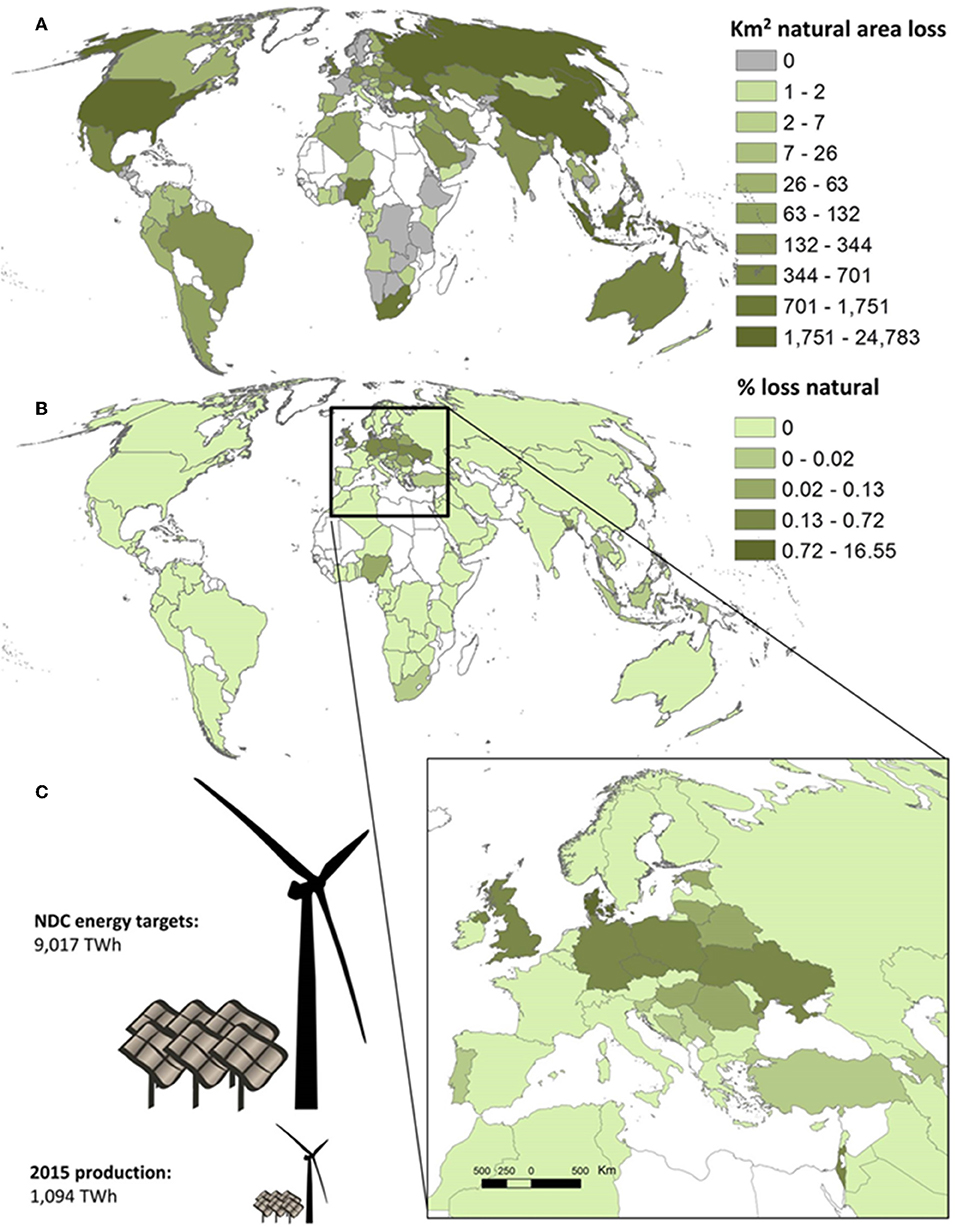
Figure 1. Per country losses of natural areas under a development scenario in which wind and solar renewable energy is sited where resources are the highest. Results are shown for (A) the total km2 of natural lands losses by 10% quantile breaks, and (B) the % that the lost km2 represent out of total natural area in the country. Only countries with NDC energy targets (n = 109) were included in the analyses (colored countries), and gray color represent countries for which the siting scenario resulted in no loss of natural areas. (C) Wind turbine and solar panel inserts are drawn to a relative scale to represent the amount of wind and solar renewable energy that were produced in 2015 (International Energy Agency Statistics website https://www.iea.org/classicstats/statisticssearch/) and the amount that will be required for the NDC energy targets.
Methods
Technical Potential Maps
We used technical potential maps estimates derived by Baruch-Mordo et al. (2019), where for TWh needed to offset electricity and heat generation from fossil fuel into renewable energy in order to meet the GHG emission reductions established by the NDCs (see details in Supplementary Text). Each 1 km resolution pixel represented the maximum TWh potential across on-shore wind, concentrated solar power (CSP), and utility-scaled and rooftop photovoltaic (PV) renewable energy sectors. We focused on wind and solar sectors because they have already penetrated the market and are projected to consist of 80% of global capacity growth in the next 5 years (International Energy Agency, 2017). Potential was derived based on suitability maps accounting for resources available at the focal site (e.g., wind speed at 80 m height, direct normal irradiance (DNI) for CSP), biophysical constraints (e.g., elevation, slope, minimum patch size) and land-use constraints (e.g., protected areas, areas with high multi-use competition). Specifically, wind was located at wind speeds ≥5 m/s and where a contiguous suitable patch size >15 km2 to account for total wind farm size, and was excluded from areas with elevation >2,000 m, slope >30%, or with urban landcover type; CSP was located where DNI ≥ 2100 kWh·m−2·y−1 and where a contiguous patch size ≥2 km2, and along with utility-scale PV (which had no GHI, or patch size restrictions) was excluded from areas with slope >5%, where landcover type was classified as urban, or where amount of agricultural lands in the vicinity (3 × 3 cell neighborhood) was >20%; see full descriptions of sector-specific derivation of technical potential in Supplementary text of Baruch-Mordo et al. (2019).
Suitability maps were multiplied by a constant sector-specific power density (TWh/km2) derived from empirical and review studies (see SI in Baruch-Mordo et al., 2019), a spatially-explicit sector-specific capacity factor map which represents the actual over rated power output, and a 8,760 h/y conversion factor. While we acknowledge that a given 1 km2 cell can accommodated more than one sector, we wished to remain conservative in our estimates of technical potential; to avoid overestimating, only the maximum TWh across all sectors was attributed to the final map in which >34 million km2 had technical potential >0 with a median across all suitable 1 km cells of 0.0505 TWh (range: <0.0001–0.0832 TWh).
The technical potential maps included sector-specific biophysical constraints (e.g., resource value, slope, minimum patch size, etc.), excluded areas unavailable for development (e.g., protected areas, areas with high multi-use competition, etc.), and avoided double counting by selecting one renewable energy sector for each 1 km2 cell based on the max TWh potential across all sectors (see Baruch-Mordo et al., 2019; SI-section 1 for details).
PCA Energy Targets
We used PCA energy targets derived by Baruch-Mordo et al. (2019) for which the % reduction from reference emissions (or emission intensities) was translated to total MtCO2 reductions, or 17.7 GtCO2 across 151 countries (see Supplementary Data 2 in Baruch-Mordo et al., 2019; Figure 3 insert). The total emission reductions were multiplied by the fraction of CO2 emissions attributed to electricity and heat production, which can be more easily converted to renewable energy compared, for example, to emissions from transportation. Using the emissions reductions attributed to electricity and heat production (6.0 GtCO2 for the 109 countries included in this study; Figure 3 insert), the remaining emission reduction targets were multiplied by the inverse IPCC life cycle emission factors (lce; kWh/gCO2eq); lce provide sector-specific emissions per unit of energy produced while accounting for the total emissions associated with the life of a power plant, e.g., component production, plant construction, and operations (Krey et al., 2014). We converted lce to TWh/MtCO2 and multiplied it by the proportion of emissions attributed to fossil fuels (coal, oil, and gas), which we extracted using 2014 International Energy Agency' production statistics. Summing across fossil fuel sectors, the resulting TWh represented the renewable energy targets to reduce emissions that are attributed to fossil fuel electricity and heat generation, or 34% of the total Paris Climate Agreement emission reduction commitments.
Renewable Energy Siting
Given the focus on terrestrial impacts, we excluded allocation to marine-based renewable energy sectors. We also excluded hydropower because of its high potential to negatively impact freshwater systems and geothermal power because of its low global potential (Gibson et al., 2017). Using lifecycle conversion factors, the derivation of NDC energy targets was specific to the energy portfolio of each country using most recent International Energy Agency energy production data (see details in Baruch-Mordo et al., 2019 section Biodiversity Losses). To spatially allocate renewable energy, we used global renewable energy technical potential maps at a 1-km resolution, for land-based, utility-scale wind, concentrated solar power (CSP), and photovoltaic (PV), and for urban rooftop PV (Baruch-Mordo et al., 2019). We focused on wind and solar sectors because they have already penetrated the market and are projected to consist of 80% of global capacity growth in the next 5 years (International Energy Agency, 2017). Given the focus on terrestrial impacts, we excluded allocation to marine-based renewable energy sectors. We also excluded hydropower because of its high potential to negatively impact freshwater systems and geothermal power because of its low global potential (Gibson et al., 2017).
The technical potential maps included sector-specific biophysical constraints (e.g., resource value, slope, minimum patch size, etc.), excluded areas unavailable for development (e.g., protected areas, areas with high multi-use competition, etc.), and avoided double counting by selecting one renewable energy sector for each 1 km2 cell based on the max TWh potential across all sectors (see Baruch-Mordo et al., 2019 SI-section 1 for details). Assuming development would seek to maximize production, we allocated cells to meet PCA energy targets (hereafter also impacted cells) by (1) ranking cells within each country from highest to lowest based on their max TWh technical potential, and (2) starting from the top ranked cell, allocating renewable energy development by the ranked order until the total energy potential matched or just surpassed the country's PCA energy target. If at the stopping point, more than one cell had similar TWh potential and rank, we randomly allocated the last cell(s) to meet targets. All spatial analyses were conducted in program R v.3.4.3 (R Core Team, 2017).
Biodiversity Losses
Once we allocated cells to meet PCA energy targets, we quantified the amount of overlap between impacted cells and natural lands to estimate potential natural lands loss. We defined natural lands following Oakleaf et al. (2015) as any 1 km2 cell without nighttime lights, croplands, or roads. We then summed the amount of natural lands lost and the percentage they represent from the remaining natural lands for each country (Figure S1). We acknowledge that by focusing on potential biodiversity (and carbon) impacts only on natural lands, we likely underestimate the losses of biodiversity (and carbon) associated with converted lands (e.g., croplands, urban areas) (e.g., Johnson et al., 2014; Baccini et al., 2017). However, these assets can be temporary (e.g., carbon stored in croplands) or relatively lower in value (e.g., biodiversity in urban areas), hence we chose a more conservative approach to estimating losses.
In addition to the loss to natural lands, we quantified the potential loss of Key Biodiversity Areas (KBA) (Eken et al., 2004; Langhammer et al., 2007) that are intended to support the strategic expansion of protected-area networks to achieve the Convention on Biological Diversity's “Aichi Biodiversity Targets” (Butchart et al., 2012). Impacts to these areas could undermine a countries' ability to maintain effective protection of biodiversity. We used KBA spatial polygon data (UNEP-WCMC and IUCN, 2017) and restricted our analyses only to terrestrial KBAs with confirmed status (n = 14,755). For each country we calculated the total ha of KBAs that would be converted as the median % area impacted across all KBA that were impacted within the country.
In addition to habitat loss, we calculated the number of threatened and endangered species that might be affected if development were sited to maximize production. For the 109 countries included in the analyses, we queried a list of species classified as vulnerable, near-threatened, endangered, and critically endangered using rredlist v. 0.4.1.9420 package in R (Chamberlain, 2019). We then obtained digital distribution maps for mammals, birds, reptiles, and amphibians from the IUCN Red List Data (International Union for Conservation of Nature, 2019) and examined their overlay of impacted cells using point-in-polygon procedures, where we evaluated the overlap between the centroid of each impacted cell and the polygon distribution of each species. If at least one impacted cell overlapped with the species distribution map we considered the species as being potentially impacted, and to ensure impacted species were counted only once per country we tallied across country and taxa the number of impacted threatened and endangered species' ranges.
Carbon Stock and Emission Losses
While we considered losses to natural areas and KBAs to occur within all areas of the cells selected for development, we considered only the direct development footprint, i.e., the amount of land cleared due to wind turbines or solar power plant placement, when calculating carbon losses. If the sited development was wind, which has a more dispersed configuration (Denholm and Margolis, 2008), we adjusted cell conversion to 10% (see Supplementary Text for derivation of estimate). If the sited development was utility-scale solar, which has a highly clustered configuration and a median plant size of 0.9 km2 for PV and 1.6 km2 for CSP (based on data from Ong et al., 2013), we assumed the entire cell to be converted. We assumed no natural land loss for rooftop PV, as it was always located on urban area rooftops.
We estimated the total metric ton (t) of carbon (C) loss using a global aboveground vegetation biomass map updated from Baccini et al. (2012), which we resampled from 15 arcsecond to 1 km resolution and converted from tC/ha to tC/km2. For natural lands impacted by our renewable energy footprint, we summed the amount of tC loss, where for cells in which wind power plants were sited, we adjusted carbon loss to 10% of the cell's tC (see details in Supplementary Text). We then calculated MtCO2 loss by converting tC to MtC, and multiplying the result by a factor of 3.67 CO2/C (United States Environmental Protection Agency, 2016). We summarized for each country the total tC and MtCO2 emissions losses resulting from the footprint needed to meet PCA energy targets.
We also calculated the years (yri) it would take to compensate for carbon losses using:
where emi is the emission losses in MtCO2/km2 for impacted cell i, Δlces is the average difference between the inverse IPCC life cycle emission factors (MWh/MtCO2; Schlömer et al., 2014) of fossil fuel sectors and the renewable energy sector s that was sited in focal cell i (details below), pds is the sector-specific power density in MW/km2, cfs is the sector-specific unitless capacity factor, or the observed output over rated capacity, and 8,760 is a conversion factor from hours to years. Because each renewable energy sector s has three differences—one for each of the three fossil fuel sectors of oil, coal, and gas—we calculated for each renewable sector s its average difference in lces (Δlces) across the three fossil fuel sectors (calculations in Supplementary Text, where we also detail conversion of the reported gCO2/kWh to MtCO2/MWh for Δlces). For each of the countries' impacted cells, we summarized the median value of years to recover emissions loss (Figure S2).
Results and Discussion
To achieve the 2°C target of the Paris Climate Accord, fossil fuels need to be phased out and replaced by low-carbon sources of energy. This transition will require the nearly complete decarbonization of the energy sector by 2050, and an accelerated shift toward electricity as a final energy carrier (Jacobson et al., 2017), while increasing terrestrial carbon storage (Griscom et al., 2019). Shifting energy production from fossil fuels to renewable energy will require a larger spatial footprint to support production resulting in land-use tradeoffs (Smil, 2015; Tallis et al., 2018). Our results suggest that development that is sited based solely on maximizing renewable energy production has the potential to convert >11 million ha of natural lands, with over 73% of that loss occurring in the top 10 GHG emitting countries (Table 1, Figure 1). For some countries, this loss represents a large component of their remaining natural lands (e.g., Denmark 16.6%, Czech Republic 8.8%, Germany 8.85%, UK 8.2%; Figure 1, Table S1). Over 3.1 million ha could result in habitat loss or fragmentation in KBAs intended to support the strategic expansion of protected-area networks. The median KBA area impacted across all countries was 2.5%; Israel (54.6%) had more than half of its KBAs' area reduced while Spain (25.4%) more than a quarter (Table S1). Failure to recognize these potential impacts could ultimately slow adherence to the PCA commitments and jeopardize the financial investments needed to fuel the renewable energy transition.
The increase in renewable energy production to meet PCA commitments may be compatible with biodiversity and other conservation goals when properly sited. However, we found that the ranges of 1,574 threatened and endangered species could be impacted if renewable energy is sited without consideration of current land cover (Table S1, Figure 2). All threatened and endangered species terrestrial vertebrates groups could experience some impacts ranging from a low of 158 amphibian species to a high of 782 bird species (Table 1). Not surprising, the highest numbers of species ranges that intersect potential renewable energy development are in the tropical countries of Indonesia (282), Malaysia (273), and Thailand (253) (Table S1). Impacts can be reduced by constraining new renewable energy to already cleared or degraded lands which would reduce the potential conversion of habitats important for biodiversity. A recent analysis suggests >6 million km2 of already converted lands are suitable for renewable energy development and can produce 17 times the PCA energy targets (Baruch-Mordo et al., 2019). Therefore, there is ample opportunity to meet the PCA energy targets in areas already disturbed by humans, which generally have lower value for biodiversity (Keith et al., 2013). These converted areas also represent lower quality habitats for biodiversity (Gibson et al., 2011) and for long-term carbon storage (Pütz et al., 2014; Houghton and Nassikas, 2017). Yet, we acknowledge that converted or degraded lands, such as rangelands, can harbor biodiversity and carbon storage value, and therefore additional spatial planning of renewables siting should also occur within human-dominated lands.
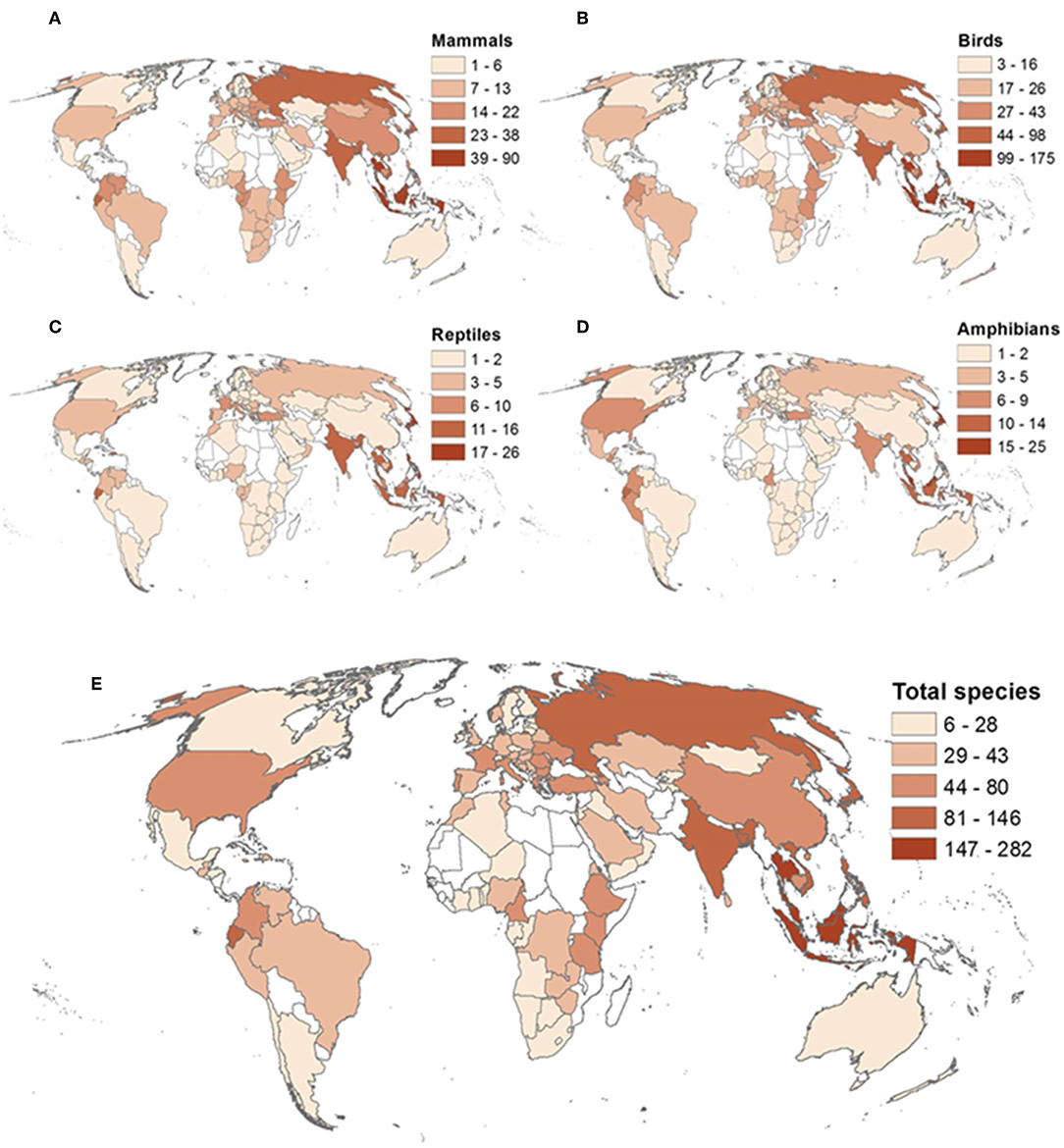
Figure 2. Per country potential losses of threatened and endangered species under a development scenario in which wind and solar renewable energy is sited where resources are the highest. Results represent the number of IUCN ranges that overlapped cells selected for renewable energy siting and are shown for (A) mammals, (B) birds, (C) reptiles, (D) amphibians, and (E) total across the four taxa. Only countries with NDC energy targets (n = 109) were included in the analyses (colored countries) and all maps were classified into five categories using natural breaks.
The push to develop renewable energy is driven by the reality that modern energy systems represent the largest portion of global emissions and in turn represent a critical pathway needed to reduce CO2 emissions (Davis et al., 2010). However, the climate change mitigation benefits from renewable energy will be reduced if development cannot avoid impacts to remaining habitat and subsequent CO2 emissions (Kiesecker and Naugle, 2017). Soils and plant biomass are the two largest biologically active stores of terrestrial carbon, together containing ~2.7 times more carbon than the atmosphere (Schlesinger, 1997). Converting native habitats for energy development releases CO2 as a result of vegetation clearing and the decomposition of organic carbon stored in plant biomass and soils, thus creating a carbon deficit from land conversion (Fargione et al., 2008). Our results indicate that the land-use footprint of maximizing renewable energy production could result in ~415 million tons of additional natural land carbon stock loss (Table 1) or ~1.5 GtCO2 emissions loss, which represents ~8.6% of the total global NDC goal (Figure 3, inset). As expected, tropical countries, e.g., Indonesia and Malaysia, may lose less km2 of natural lands relative to other countries, but those losses translate into the large amounts of MtCO2 (Figure 3). For five countries (Belarus, Gabon, Indonesia, Malaysia, and Republic of Congo), emissions from poorly sited renewable energy development could exceed their total NDC emission reduction target (Table S1). Over time, renewable energy that converts land can repay this carbon deficit. Our results indicate that the median time to recover carbon loss from renewable energy is a relatively short timeframe, but this varies spatially and in places could be over 20 years (Figure 4). For the majority of countries, carbon deficits were small but for 22 countries, including the top 10 emitter Japan (median 11.47 years), 12 of the EU28 countries (ranging from 1.2 years for Germany to 12.3 years for Czech Republic), and six tropical countries (ranging from 1.3 years in Jamaica to 4.2 years in the Republic of Congo), it would require ≥1 year (median of impacted cells) for renewable energy to be in operation to make up for the carbon loss from land clearing (Figure 4, Table S1). Development that proceeds in this manner will need to make up losses through additional actions. Given the short time frame between the establishment of the NDC targets and implementation of the actions needed to reduce emissions, delaying the timing of peak emissions also shortens the time for the economic transformations that are necessary for the shift to renewable energy (Figueres et al., 2017).
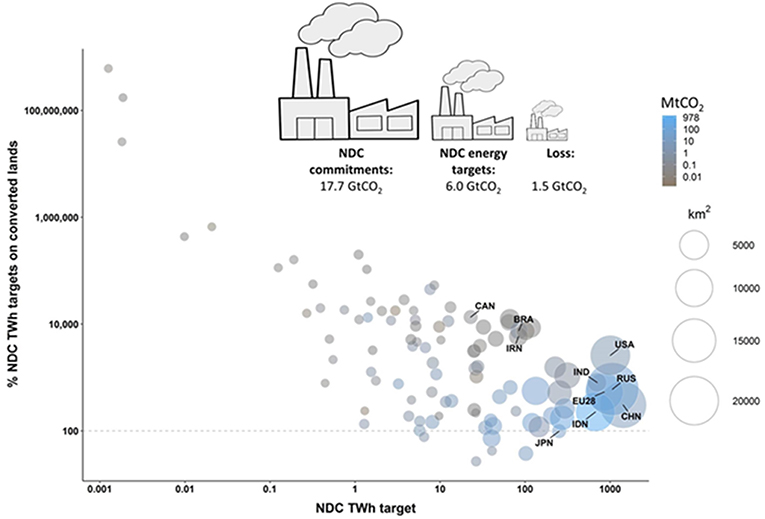
Figure 3. Per country km2 of natural lands losses and the resulting greenhouse gas emission losses (MtCO2) from carbon storage removals under a renewable energy siting scenario in which developments are placed where wind and solar resources are the highest. NDC TWh targets (x-axis and middle square insert) are the estimated renewable energy offsets that account for the proportion of NDC emissions reductions related to electricity and heat generation from fossil fuels. Percent NDC TWh targets on converted lands (y-axis) represent the percent ratio of TWh available from wind and solar energy on converted lands to NDC TWh targets (Baruch-Mordo et al., 2019), where the dotted line at 100% represents a 1:1 ratio. Bubble size represents the km2 loss of natural lands, and gray (low) to blue (high) color gradation represents the MtCO2 loss from above ground carbon stock removal that result from clearing of natural lands for renewable energy development. Data are based on Table S1, and only countries for which natural lands loss was >0 km2 are plotted (n = 96; omitting BEL, BEN, CHE, CYP, ETH, LUX, MKD, MLT, MUS, NOR, SGP, SWE, TGO, ZMB). The top 10 emitter countries are labeled, and EU28 countries are plotted collectively and individually. Power plant image inserts represent the global summaries for quantifiable NDC emission reduction commitments (100% for n = 151 countries based on Baruch-Mordo et al., 2019), emissions accounted for with the NDC energy targets (34% for n = 109 countries), and total MtCO2 losses under the unconstrained siting scenario (8%).
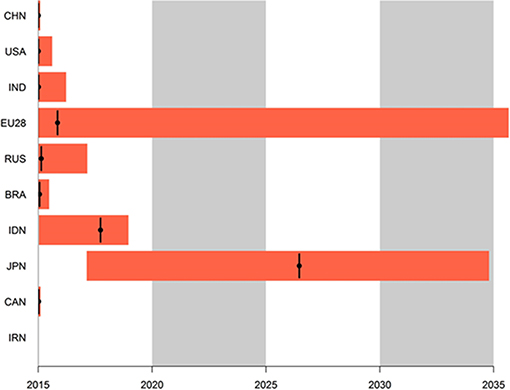
Figure 4. Years to recover carbon loss for the top 10 greenhouse gas emitter countries plotted on a timeline with base year of the Paris Climate Agreement signing (2015). Losses are calculated when wind and solar renewable energy development are sited where the wind and solar resources are the highest (i.e., impacted cells). Black dotted line represent the median years to recover across all impacted natural land cells, while bars' lows and highs, respectively, represent the minimum and maximum years to recover carbon loss across all impacted cells.
Failure to safeguard global climate could result in devastating impacts: extreme weather events such as flooding and deadly storms, the spread of disease, sea level rise, increased food insecurity, and other disasters (Challinor et al., 2017). These impacts can cost businesses, governments, and taxpayers hundreds of billions of dollars through rising health care costs, destruction of property, and increased food prices. The social cost of carbon (SCC) is a measure of the economic harm from those impacts, expressed as the dollar value of the total damages from emitting one ton of carbon dioxide into the atmosphere (Nordhaus, 2017). Our results suggest that siting renewable energy on natural lands could potentially result in the loss of ~1.5 GtCO2 of GHG, which could currently cost $47.5 billion USD, and as much as $155.9 billion USD by 2050 (based on base parameter SSC estimates in 2010 international USD; Table 1 in Nordhaus 2017). Avoiding land based emissions during siting could reduce SCC costs and diminish investor risk.
Our renewable energy scenario forecasts a significant amount of impact yet we recognize that environmental impacts by infrastructure development can be avoided or reduced through careful decisions about location and full implementation of mitigation measures (McKenney and Kiesecker, 2010). But to date, these approaches advance with “band aid” actions to environmental destruction and reactive and insufficient impact mitigation (Kiesecker et al., 2010; Clare et al., 2011). Ultimately impact mitigation will occur thru the lens of country level environmental licensing polices which are highly variable (Villarroya et al., 2014). Furthermore, some countries have environmental policies that may facilitate impacts from renewable energy. For example, in India wind or solar power development is not required to undergo an environmental impact assessment (EIA) process (i.e., Mohan, 2017). Renewable development is categorized as “green” by state control boards which mean these projects, irrespective of their size, capacity or location, do not require EIA assessments. Highlighting countries where unrestricted development could have significant impacts could serve as a mechanism to promote stronger policy development.
While providing a starting point for renewable energy siting our scenarios are not intended to prescribe exactly where renewable energy infrastructure will be located, but instead to highlight that there are many options to site wind and solar development in a manner that will avoid land-use conflicts (Baruch-Mordo et al., 2019). Our analyses omit several important considerations that should be included in more detail before siting. For example, site-specific characteristics that can't be captured with global data or landowner preferences may limit the ability to develop specific parcels of converted land. Care should be given to proactively incorporate land ownership, in particular land claims of marginalized populations such as indigenous groups (Heiner et al., 2019).
Given the global extent of our analysis, we undertook a somewhat simplified approach to estimating renewable energy impacts on natural land, and our assessment has several sources of uncertainty in quantifying impacts associated with PCA renewable energy targets (see Baruch-Mordo et al., 2019 for a detailed discussion). First we may have under-estimated impacts to areas important for biodiversity given that our binary definition of converted and unconverted lands may exclude areas with human activities (e.g., livestock grazing, logging, recreation) that still support biodiversity and carbon storage. We may have also underestimated the number of species impacted, because on average only 39% of the species listed with red list status had spatial data available, thus, our analyses excludes some taxa, in particular plants and invertebrates. We also recognize that a 1 km2 impact on a threatened or endangered species range may represent a very small portion of the species' range in some cases (e.g., Polar bear, Ursus maritimus), but in others, it may exceed a species' known range (e.g., Kandyan dwarf toad, Adenomus kandianus), or be located in a critical area (e.g., breeding areas). Given we should avoid any negative impact to any threatened or endangered species, we proceeded with the overlap analyses recognizing these results represent only the number of species that can be potentially impacted, and that additional analyses are warranted to estimate the extent of such impacts. In addition by using a scenario that focuses solely on decarbonizing energy generation from electricity and heat sources (Baruch-Mordo et al., 2019), we accounted for only about a third of the quantifiable NDC emission reduction targets, potentially underestimating energy needs and in turn the footprint needed to meet those goals. Conversely, the development scenario we adapted (Baruch-Mordo et al., 2019) excluded concurrent development (e.g., siting both wind and solar in a given cell), potentially increasing the land base needed and perhaps increasing the estimated potential conversion of natural lands and biodiversity and carbon losses. Lastly given the global surplus in wind and solar energy, it is conceivable that countries that have limited resources or have limited flexibility in how they meet their renewable goals, could import electricity from countries where there is a surplus of low conflict renewable energy. Many countries have a significant surplus of renewable energy potential on converted lands where additional development would not likely cause significant loss of natural lands (Baruch-Mordo et al., 2019); energy development and cross-boundary transmissions may alleviate some impacts to biodiversity or carbon assets, if feasible given transmission and political constraints.
Conclusions
While our analysis likely presents a “worst case scenario” of renewable energy impacts, the flexibility to site development with proactive planning (i.e., Kiesecker et al., 2011; Fargione et al., 2012) on lands with lower conservation values. We can ensure that goals to rapidly expand renewable energy can occur without creating conflicts that might jeopardize investment. Steering renewable development to already converted or disturbed lands represents the first step in a series of hierarchical filters that could reduce future impacts (Kiesecker et al., 2011). Harnessing the power of landscape planning will allow stakeholders to simultaneously examine impacts to lands important for climate mitigation and biodiversity conservation while identifying renewable energy “go zones,” thus ultimately minimizing investment risks (Kiesecker and Naugle, 2017). We see two major applications for the results of our analysis to guide future research. First, our assessment will help decision makers see the extent and nature of future potential impacts resulting from renewable energy creating the impetus for planning needed to advance renewable energy. Land-based renewable energy (i.e., wind and solar) requires a considerable amount of space, as its electricity output per unit of area is low when compared with fossil-fuel-powered generation (McDonald et al., 2009). This can lead to the challenge known as “energy sprawl” (Kiesecker and Naugle, 2017). As our analysis has demonstrated if these energy installations are not sited carefully, they can negatively impact wildlife, habitats, and critical ecosystem services—and even generate GHG emissions that reduce their climate benefits. The challenge from a planning perspective is to design landscapes that embrace growing energy demand while minimizing repercussions to nature and people. Society however remains largely uninformed about the consequences of energy sprawl and the urgent need for planning (Kiesecker and Naugle, 2017). Second, our analysis can be used to guide a spatial prioritization of countries to identify places where conservation assets are most at risk from renewable energy infrastructure expansion and where more detail assessments that balance multiple objectives are needed. Planning of this nature will need to involve partners and data that don't typically get integrated—bringing together environmental and power grid experts. Given this challenge it will be necessary to carefully identify where this planning will be most useful. Obviously top emitting countries will have the greatest need for renewable energy and in turn the greatest potential for land use conflicts. However, top emitting countries vary greatly in terms of potential impacts to natural lands, carbon emissions and at risk species and our analysis may be useful in ensuring policy goals focus on the currencies most at risk i.e., avoiding carbon loss in Indonesia (Table 1).
Failure to recognize potential impacts could ultimately slow adherence to the PCA commitments and jeopardize the financial investments needed to fuel the renewable energy transition. Energy projects are generally considered high risk by investors, and the main finance challenge is how to de-risk them politically, socially, and environmentally. An ~$28 trillion USD financing gap for energy infrastructure exists through 2030 (Bielenberg et al., 2016), which can only be closed with access to the capital held by large institutional investors such as pension, insurance, and sovereign wealth funds (Bhattacharya et al., 2015). To attract these larger investors, a proactive identification of renewable energy “no regret” areas can promote pools of low-risk projects attractive to affordable capital (Kiesecker and Naugle, 2017). Our results can guide policymakers to think systematically about where energy-conservation tradeoffs are likely, and how to reduce the associated impacts to biodiversity and carbon storage. We argue for policies and financial incentives that will promote development on converted lands, serving as a mechanism that can de-risk investment in renewable energy to facilitate its proliferation.
Data Availability Statement
Additional Supporting Information may be found in the online version of this article at the publisher's web site. Data are available from www.nature.org/paris2practice or by request from corresponding authors.
Author Contributions
JK conceived the study. SB-M and JK designed the study. SB-M collected and analyzed the data, and produced figures and tables. CK, JO, AB, and BG assisted in data collection and provided input on the analysis approach. JK and SB-M interpreted results and wrote the paper with contributions from CK, JO, and BG.
Funding
We thank Enterprise Foundation, MacArthur Foundation, The Nature Conservancy, Roy Vagelos, and Anne Ray Charitable Trust for funding support.
Conflict of Interest
The authors declare that the research was conducted in the absence of any commercial or financial relationships that could be construed as a potential conflict of interest.
Supplementary Material
The Supplementary Material for this article can be found online at: https://www.frontiersin.org/articles/10.3389/fenvs.2019.00151/full#supplementary-material
Supplementary text includes: Procedures to calculate wind development footprint; and Differences in IPCC life cycle emission factors.
Figure S1. Example calculation of median percent area potential loss of the three KBAs in Singapore. Each KBA's area loss (dark brown) was calculated based on the overlap between KBA (green) and the impacted cells under an unrestricted renewable energy development in which development is sited where wind and solar resources are the highest (gray). For the country of Singapore we reported the median loss across all impacted KBAs as the median of 13.61, 14.28, and 26.98%, which was 14.28%.
Figure S2. Example calculation of years to recover carbon loss in Japan under a development scenario in which wind and solar renewable energy is sited (resulting in impacted cells) where resources are the highest. (a) areas of overlap (darker green) between impacted cells (gray) and natural areas (lighter green), (b) tons of carbon loss for each pixel of overlap areas which were multiplied by the insert equation to obtain, (c) emissions loss for each pixel which were multiplied by the insert equation to obtain, (d) the number of years it would take to recover the carbon loss. Δlces is the average difference between the inverse IPCC life cycle emission factors (MWh/MtCO2; Schlömer et al., 2014) of fossil fuel sectors and the renewable energy sector s that was sited in focal cell i, pds is the sector-specific power density in MW/km2, cfs is the sector-specific unit-less capacity factor, or the observed output over rated capacity, and 8,760 is a conversion factor from hours to years.
Table S1. Country-specific biodiversity and carbon losses.
References
Allred, B. W., Smith, W. K., Twidwell, D., Haggerty, J. H., Running, S. W., Naugle, D. E., et al. (2015). Ecosystem services lost to oil and gas in North America. Science 348, 401–402. doi: 10.1126/science.aaa4785
Arent, D., Pless, J., Mai, T., Wiser, R., Hand, M., Baldwin, S., et al. (2014). Implications of high renewable electricity penetration in the US for water use, greenhouse gas emissions, land-use, and materials supply. Appl. Energy 123, 368–377. doi: 10.1016/j.apenergy.2013.12.022
Baccini, A., Goetz, S. J., Walker, W. S., Laporte, N. T., Sun, M., Sulla-Menashe, D., et al. (2012). Estimated carbon dioxide emissions from tropical deforestation improved by carbon-density maps. Nat. Clim. Change 2, 182–185. doi: 10.1038/nclimate1354
Baccini, A., Walker, W., Carvalho, L., Farina, M., Sulla-Menashe, D., and Houghton, R. A. (2017). Tropical forests are a net carbon source based on aboveground measurements of gain and loss. Science 358, 230–234. doi: 10.1126/science.aam5962
Baruch-Mordo, S., Kiesecker, J., Kennedy, C. M., Oakleaf, J. R., and Opperman, J. J. (2019). From Paris to practice: sustainable implementation of renewable energy goals. Environ. Res. Lett. 14:024013. doi: 10.1088/1748-9326/aaf6e0
Bhattacharya, A., Oppenheim, J., and Stern, N. (2015). “Driving sustainable development through better infrastructure: key elements of a transformation program,” in Brookings Global Economy and Development Working Paper no. 91 (Washington, DC).
Bielenberg, A., Kerlin, M., Oppenheim, J., and Roberts, M. (2016). Financing Change: How to Mobilize Private-Sector Financing for Sustainable Infrastructure. McKinsey Center for Business and Environment. Available online at: https://newclimateeconomy.report/workingpapers/workingpaper/financing-change-how-to-mobilize-private-sector-financing-for-sustainable-infrastructure/ (accessed July 7, 2019).
Burke, M., Davis, M., and Diffenbaugh, N. S. (2018). Large potential reduction in economic damages under UN mitigation targets. Nature 557, 549–553. doi: 10.1038/s41586-018-0071-9
Butchart, S. H. M., Scharlemann, J. P., Evans, M., Quader, S., Aricò, S., Arinaitwe, J., et al. (2012). Protecting important sites for biodiversity contributes to meeting global conservation targets. PLoS ONE 7:e32529. doi: 10.1371/journal.pone.0032529
Challinor, A. J., Adger, W. N., and Benton, T. G. (2017). Climate risks across borders and scales. Nat. Clim. Change 7, 621–623. doi: 10.1038/nclimate3380
Chamberlain, S. (2019). rredlist: ‘IUCN' Red List Client. R Package Version 0.4.1.9420. Available online at: https://github.com/ropensci/rredlist
Clare, S., Krogman, N., Foote, L., and Lemphers, N. (2011). Where is the avoidance in the implementation of wetland law and policy? Wetl. Ecol. Manage. 19, 165–182. doi: 10.1007/s11273-011-9209-3
Crist, E., Mora, C., and Engelman, R. (2017). The interaction of human population, food production, and biodiversity protection. Science 80, 260–264. doi: 10.1126/science.aal2011
Davis, S. J., Caldeira, K., and Matthews, H. J. (2010). Future CO2 emissions and climate change from existing energy infrastructure. Science 329, 1330–1333. doi: 10.1126/science.1188566
Denholm, P., and Margolis, R. M. (2008). Land-use requirements and the per-capita solar footprint for photovoltaic generation in the United States. Energy Policy 36, 3531–3543. doi: 10.1016/j.enpol.2008.05.035
Diffendorfer, J. E., and Compton, R. W. (2014). Land cover and topography affect the land transformation caused by wind facilities. PLoS ONE 9:e88914. doi: 10.1371/journal.pone.0088914
Eken, G., Bennun, L., Brooks, T. M., Darwall, W., Fishpool, L. D. C., Foster, M., et al. (2004). Key biodiversity areas as site conservation targets. Bioscience 54, 1110–1118. doi: 10.1641/0006-3568(2004)054[1110:KBAASC]2.0.CO;2
Fargione, J., Hill, J., Tilman, D., Polasky, S., and Hawthorne, P. (2008). Land clearing and the biofuel carbon debt. Science 319, 1235–1238. doi: 10.1126/science.1152747
Fargione, J., Kiesecker, J., Slaats, M. J., and Olimb, S. (2012). Wind and wildlife in the northern great plains: identifying low-impact areas for wind development. PLoS ONE 7:e41468. doi: 10.1371/journal.pone.0041468
Figueres, C., Schellnhuber, H. J., Whiteman, G., Rockström, J., Hobley, A., and Rahmstorf, S. (2017). Three years to safeguard our climate. Nature 546, 593–595. doi: 10.1038/546593a
Fuso-Nerini, F., Tomei, J., To, L. S., Bisaga, I., Parikh, P., Black, M., et al. (2017). Mapping synergies and trade-offs between energy and the sustainable development goals. Nat. Energy 3, 10–15. doi: 10.1038/s41560-017-0036-5
Gibson, L., Lee, T. M., Koh, L. P., Brook, B. W., Gardner, T. A., Barlow, J., et al. (2011). Primary forests are irreplaceable for sustaining tropical biodiversity. Nature 478, 378–381 doi: 10.1038/nature10425
Gibson, L., Wilman, E. N., and Laurance, F. (2017). How green is “green” energy neTrends Ecol. Evol. 32, 922–935. doi: 10.1016/j.tree.2017.09.007
Griscom, B. W., Lomax, G., Kroeger, T., Fargione, J. E., Adams, J., Almond, L., et al. (2019). We need both natural and energy solutions to stabilize our climate. Glob. Change Biol. 25, 1889–1890. doi: 10.1111/gcb.14612
Heiner, M., Hinchley, D., Fitzsimons, J., Weisenberger, F., Bergmann, W., McMahon, T., et al. (2019). Moving from reactive to proactive development planning to conserve Indigenous community and biodiversity values. Environ. Impact Assess. Rev. 74, 1–13. doi: 10.1016/j.eiar.2018.09.002
Houghton, R. A., and Nassikas, A. A. (2017). Global and regional fluxes of carbon from land use and land cover change 1850–2015. Glob. Biogeochem. Cycles 31, 456–472, doi: 10.1002/2016GB005546
International Energy Agency (2017). Renewables 2017 Analysis and Forecasts to 2022. International Energy Agency Market Report Series. Available online at: https://www.iea.org/Textbase/npsum/renew2017MRSsum.pdf (accessed November 14, 2017).
International Union for Conservation of Nature (IUCN) (2019). The IUCN Red List of Threatened Species. Version 6.1. Available online at: http://www.iucnredlist.org (accessed March 6, 2019).
Jacobson, M. Z., Delucchi, M. A., Bauer, Z. A. F., Goodman, S. C., Chapman, W. E., Cameron, M. A., et al. (2017). 100% clean and renewable wind, water, and sunlight all-sector energy roadmaps for 139 countries of the world. Joule 1, 108–121. doi: 10.1016/j.joule.2017.07.005
Johnson, J. A., Runge, C. F., Senauer, B., Foley, J., and Polasky, S. (2014). Global agriculture and carbon trade-offs. Proc. Natl. Acad. Sci. U.S.A. 111, 12342–12347. doi: 10.1073/pnas.1412835111
Keith, D. A., Rodríguez, J. P., Rodríguez-Clark, K. M., Nicholson, E., Aapala, K., Alonso, A., et al. (2013). Scientific foundations for an IUCN red list of ecosystems. PLoS ONE 8:e62111. doi: 10.1371/journal.pone.0062111
Kiesecker, J. M., Copeland, H., Pocewicz, A., and McKenney, B. (2010). Development by design: blending landscape-level planning with the mitigation hierarchy. Front. Ecol. Environ. 8, 261–266. doi: 10.1890/090005
Kiesecker, J. M., Evans, J. S., Fargione, J., Doherty, K., Foresman, K. R., Kunz, T. H., et al. (2011). Win-win for wind and wildlife: a vision to facilitate sustainable development. PLoS ONE 6:e17566. doi: 10.1371/journal.pone.0017566
Kiesecker, J. M., and Naugle, D. E. (2017) . Energy Sprawl Solutions: Balancing Global Development Conservation. Washington DC: Island Press.
Krey, V., Masera, O., Balnford, G., Bruckner, T., Cooke, R., Fisher-Vanden, K., et al. (2014). “Annex II: metrics & methodology,” in Climate Change 2014: Mitigation of Climate Change. Contribution of Working Group III to the Fifth Assessment Report of the Intergovernmental Panel on Climate Change (Cambridge, UK).
Langhammer, P. F., Bakarr, M. I., Bennun, L. A., Brooks, T. M., Clay, R. P., Darwall, W., et al. (2007). Identification and Gap Analysis of Key Biodiversity Areas: Targets for Comprehensive Protected Area Systems. Gland, Switzerland: IUCN (Best Practice Protected Area Guidelines Series 15).
McDonald, R. I., Fargione, J., Kiesecker, J., Miller, W. M., and Powell, J. (2009). Energy sprawl or energy efficiency: climate policy impacts on natural habitat for the United States of America. PLoS ONE 4:e6802. doi: 10.1371/journal.pone.0006802
McKenney, B. A., and Kiesecker, J. M. (2010). Policy development for biodiversity offsets: a review of offset frameworks. Environ. Manage. 45, 165–176. doi: 10.1007/s00267-009-9396-3
Mohan, A. (2017). Whose land is it anyway? Energy futures & land use in India. Energy Policy 110, 257–262 doi: 10.1016/j.enpol.2017.08.025
Moreira, F. (2019). Love me, love me not: perceptions on the links between the energy sector and biodiversity conservation. Energy Res. Soc. Sci. 51, 134–137. doi: 10.1016/j.erss.2019.01.002
Nordhaus, W. D. (2017). Revisiting the social cost of carbon. Proc. Natl. Acad. Sci. U.S.A. 114, 1518–1523. doi: 10.1073/pnas.1609244114
Oakleaf, J. R., Kennedy, C. M., Baruch-Mordo, S., Gerber, J. S., West, P. C., Johnson, J. A., et al. (2019). Mapping global development potential for renewable energy, fossil fuels, mining and agriculture sectors. Sci. Data 6:101. doi: 10.1038/s41597-019-0084-8
Oakleaf, J. R., Kennedy, C. M., Baruch-Mordo, S., West, P. C., Gerber, J. S., Jarvis, L., et al. (2015). A world at risk: aggregating development trends to forecast global habitat conversion. PLoS ONE, 10:e0138334. doi: 10.1371/journal.pone.0138334
Ong, S., Campbell, C., Denholm, P., Margolis, R., and Heath, G. (2013). Land-Use Requirements for Solar Power Plants in the United States. Nrel/Tp-6a20-56290. Golden, CO.
Pütz, S., Groeneveld, J., Henle, K., Knogge, C., Martensen, A. C., Metz, M., et al. (2014). Long-term carbon loss in fragmented Neotropical forests. Nat. Commun. 5:5037. doi: 10.1038/ncomms6037
R Core Team (2017). R: A Language and Environment for Statistical Computing v.3.4.3. Vienna, Austria: R Foundation for Statistical Computing. Available online at: https://www.R-project.org/
Ripple, W. J., Wolf, C., Galetti, M., Newsome, T. M., Alamgir, M., Crist, E., et al. (2017). World scientists' warning to humanity: a second notice. Bioscience 67, 1026–1028. doi: 10.1093/biosci/bix125
Santangeli, A., Toivonen, T., Pouzols, F., Pogson, M., Hastings, A., Smith, P., et al. (2016). Global change synergies and trade-offs between renewable energy and biodiversity. GCB Bioenergy 8, 941–951, doi: 10.1111/gcbb.12299
Schlesinger, W. H. (1997). Biogeochemistry: An Analysis of Global Change. San Diego, CA: Academic Press.
Schlömer, S, Bruckner, T., Fulton, L., Hertwich, E., McKinnon, A., Perczyk, D, et al. (2014) “Annex III: Technology-specific cost performance parameters,” in Climate Change 2014: Mitigation of Climate Change. Contribution of Working Group III to the Fifth Assessment Report of the Intergovernmental Panel on Climate Change, eds O. Edenhofer, R. Pichs-Madruga, Y. Sokona, E. Farahani, S. Kadner, K. Seyboth, A. Adler, I. Baum, S. Brunner, P. Eickemeier, B. Kriemann, J. Savolainen, S. Schlömer, C. von Stechow, T. Zwickel, and J. C. Minx (Cambridge, UK; New York, NY: Cambridge University Press).
Smil, V. (2015). Power Density: A Key to Understanding Energy Sources and Uses. Cambridge, MA: The MIT Press. doi: 10.7551/mitpress/10046.001.0001
Smith, P., Adams, J., Beerling, D. J., Beringer, T., Calvin, K. V., Fuss, S., et al. (2019). “Impacts of Land-Based Greenhouse Gas Removal Options on Ecosystem Services and the United Nations Sustainable Development Goals,” in Annual Review of Environment and Resources (Sheffield, UK: The University of Sheffield). doi: 10.1146/annurev-environ-101718-033129
Tallis, H. M., Hawthorne, P. L., Polasky, S., Reid, J., Beck, M. W., Brauman, K., et al. (2018). An attainable global vision for conservation and human well-being. Front. Ecol. Environ. 16, 563–570. doi: 10.1002/fee.1965
UNEP-WCMC IUCN (2017). Protected Planet: The World Database on Protected Areas (WDPA). Cambridge: UNEP-WCMC and IUCN. Available online at: www.protectedplanet.net
United Nations (2015b). Sustainable Development Goals. Available online at: https://sustainabledevelopment.un.org/content/documents/21252030%20Agenda%20for%20Sustainable%20Development%20web.pdf
United States Environmental Protection Agency (2016). Greenhouse Gasses Equivalencies Calculator - Calculations and References. Available online at: https://www.epa.gov/energy/greenhouse-gases-equivalencies-calculator-calculations-and-references (accessed September 7, 2016).
Villarroya, A., Barros, A. C., and Kiesecker, J. (2014). Policy development for environmental licensing and biodiversity offsets in Latin America. PLoS ONE 9:e107144. doi: 10.1371/journal.pone.0107144
World Bank (2017). CO2 emissions from electricity and heat production, total (% of total fuel combustion) [WWW Document]. Available online at: https://data.worldbank.org/indicator/EN.CO2.ETOT.ZS (accessed February 27, 2017).
Keywords: renewable energy, Paris Climate Agreement, nationally determined contributions, energy development impacts, sustainable development, energy sprawl
Citation: Kiesecker J, Baruch-Mordo S, Kennedy CM, Oakleaf JR, Baccini A and Griscom BW (2019) Hitting the Target but Missing the Mark: Unintended Environmental Consequences of the Paris Climate Agreement. Front. Environ. Sci. 7:151. doi: 10.3389/fenvs.2019.00151
Received: 24 June 2019; Accepted: 18 September 2019;
Published: 09 October 2019.
Edited by:
Ioan Cristian Ioja, University of Bucharest, RomaniaReviewed by:
Elzbieta Antczak, University of Łódz, PolandThomas Knoke, Technical University of Munich, Germany
Copyright © 2019 Kiesecker, Baruch-Mordo, Kennedy, Oakleaf, Baccini and Griscom. This is an open-access article distributed under the terms of the Creative Commons Attribution License (CC BY). The use, distribution or reproduction in other forums is permitted, provided the original author(s) and the copyright owner(s) are credited and that the original publication in this journal is cited, in accordance with accepted academic practice. No use, distribution or reproduction is permitted which does not comply with these terms.
*Correspondence: Joseph Kiesecker, jkiesecker@tnc.org; Sharon Baruch-Mordo, sbaruch-mordo@tnc.org; Bronson W. Griscom, bgriscom@conservation.org
†These authors have contributed equally to this work