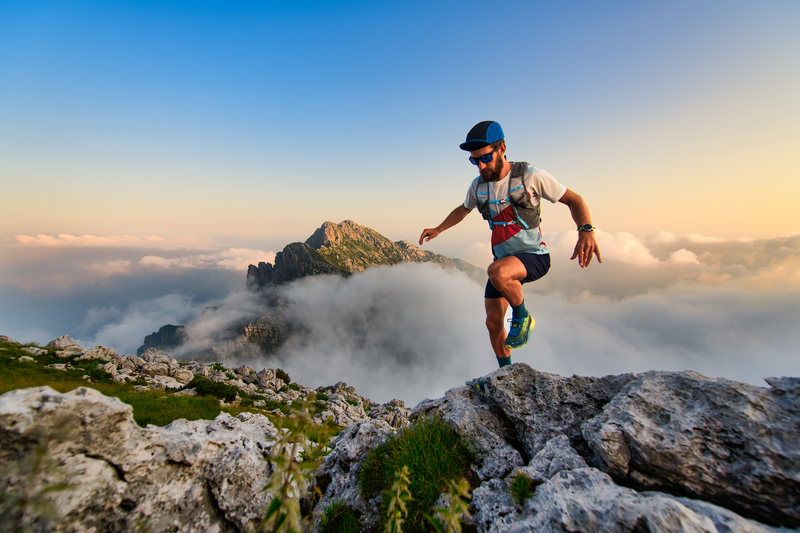
94% of researchers rate our articles as excellent or good
Learn more about the work of our research integrity team to safeguard the quality of each article we publish.
Find out more
ORIGINAL RESEARCH article
Front. Environ. Sci. , 05 November 2019
Sec. Interdisciplinary Climate Studies
Volume 7 - 2019 | https://doi.org/10.3389/fenvs.2019.00150
This article is part of the Research Topic Use of Earth Observations for Actionable Decision Making in the Developing World View all 17 articles
Land cover maps are a critical component to make informed policy, development, planning, and resource management decisions. However, technical, capacity, and institutional challenges inhibit the creation of consistent and relevant land cover maps for use in developing regions. Many developing regions lack coordinated capacity, infrastructure, and technologies to produce a robust land cover monitoring system that meets land management needs. Local capacity may be replaced by external consultants or methods which lack long-term sustainability. In this study, we characterize and respond to the key land cover mapping gaps and challenges encountered in the Lower Mekong (LMR) and Hindu Kush-Himalaya (HKH) region through a needs assessment exercise and a collaborative system design. Needs were assessed using multiple approaches, including focus groups, user engagement workshops, and online surveys. Efforts to understand existing limitations and stakeholder needs resulted in a co-developed and modular land cover monitoring system which utilizes state-of-the-art cloud computing and machine learning which leverages freely available Earth observations. This approach meets the needs of diverse actors and is a model for transnational cooperation.
Accurate and timely land cover maps play a critical role in a variety of sectors in the developing world including food security, land use planning, hydrology modeling, and natural resource management planning. Countries like Cambodia and Vietnam suffer from substantial rice crop yield losses and understanding the spatial distribution of such variable yields are critical for agricultural planning for food security (Pandey et al., 2007; Saah et al., 2019). National development plans use land cover as a basis for understanding changes in a country's natural capital, that in turn forms the basis for budget priorities and allocations (Tucker et al., 1985; Bounoua et al., 2002; Foley et al., 2005; Jung et al., 2006; Running, 2008). Land cover maps also underpin hydrology models that are used by governments to inform flood risk and preparedness in order to build resilience to climate change (Ge et al., 2007; Hibbard et al., 2010; Imaoka et al., 2010; Gong et al., 2013; Tolentino et al., 2016). Foresters use land cover maps to develop sustainable harvest management plans, integrate biodiversity conservation, and engage in climate finance initiatives such as Reducing Emissions from Deforestation and forest degradation and the role of conservation, sustainable management of forests and enhancement of forest carbon stocks in Developing countries (REDD+) or Nationally Appropriate Mitigation Action (NAMA) (Buchanan et al., 2008; Hall et al., 2011; Potapov et al., 2019). However, many developing regions lack the coordinated capacity to produce timely, accurate, and temporally comparable geospatial data products sufficient to meet their management needs (Jha and Chowdary, 2007).
The Lower Mekong (LMR) and Hindu Kush-Himalaya (HKH) regions are both experiencing an acceleration in the rate of land cover change that is impacting the long-term sustainability of ecosystem services including food, water, and energy. Local decision makers are using infrequently updated national maps with no ability to monitor in a timely or integrated fashion. Furthermore, existing classification systems do not always meet the user group's needs, data products are often not widely shared between agencies and institutions, and accuracy assessment is often lacking. The users and developers of these maps are typically from different organizations, with different priorities and technical understandings. These differences pose a variety of challenges that often create roadblocks to the effective use of appropriate land cover data for policy formulation, planning, management, and other decision contexts. As a result, global land cover products are frequently used as the best available alternative when appropriate and timely maps are not available at the regional, national, or sub-national levels. The global products have their limitations in that they have been created using different sensors and different techniques and vary in spatial resolution and classification typology, and contain inconsistencies on global (Hansen and Reed, 2000; McCallum et al., 2006; Herold et al., 2008; Hansen et al., 2013) and regional (Fritz et al., 2010; Perera et al., 2010; Leinenkugel et al., 2013, 2014) scales. These inconsistencies hinder more widespread and effective use of land cover data to valuably contribute to policy formulation, planning, management, and other processes where more effective, transparent, and defensible decisions are known to lead to better real-world outcomes.
To understand and respond to the key gaps and challenges encountered in regional, national, and subnational land cover mapping efforts, we compiled existing information, undertook proactive and targeted needs assessment activities, and compiled other information relevant to this topic from a variety of ongoing activities and communications. This paper summarizes the details and findings of those efforts and proposes a framework for addressing the identified challenges. The purpose of this paper is to review the key challenges in land cover mapping and monitoring in the Lower Mekong and HKH region and to outline design principles for the co-development of a Regional Land Cover Monitoring System.
The process of cooperatively developing a consistent, flexible Regional Land Cover Monitoring System (RLCMS) across multiple nations in SE and South Asia will allow the creation of a unified pool of land cover data and land cover mapping/monitoring architecture that can be shared across agencies and countries. Such an RLCMS will also allow for consistent change analysis to be performed going into the future, and potentially over the entire historical satellite record. Such a system would represent a landmark improvement in the availability of remote sensing-based data products for use in planning and governance.
SERVIR is a unique partnership between the U.S. Agency for International Development (USAID) and the U.S. National Aeronautics and Space Agency (NASA) focused on bringing space-based technologies to environmental decision makers in developing regions. SERVIR is represented in different parts of the world through regional hubs. The program is harnessing space-based remote sensing technology and open data to help address development challenges related to climate resilience. As a development program aimed at promoting use and development of regional geospatial data, SERVIR works in partnership with leading regional organizations to help countries in the hub regions use information provided by Earth observing satellites and geospatial technologies to manage climate risks and improve disaster preparedness. The program services are driven by a needs assessment of the partnering agencies. We selected the SERVIR hubs within the LMR and HKH regions to identify what challenges exist in creating and using land cover data products due to its multidimensional diversity that includes political differences, vegetation gradients, and a variety of needs for and uses of land cover (Figure 1). The Mekong hub was initiated in 2014, and the work during the first year was focused on conducting a needs assessment with organizations working in the partnering countries. The SERVIR HKH hub was established prior to the Mekong hub, and while their outreach methods varied slightly they also conduct needs assessments with their country stakeholders. Land cover/use mapping and monitoring challenges facing our stakeholders were compiled during the first year user needs assessment phase in the Mekong and the on-going needs assessment work in the HKH, and provides the basis for this manuscript.
Figure 1. Study area map highlighting South-Southeast Asia with the Hindu Kush-Himalaya (HKH) and the Lower Mekong region (LMR) and countries of focus.
The LMR covers a 1.9 million km2 area, has a population of 240 million people, and includes five continental Southeast Asian countries: Cambodia, Laos, Myanmar, Thailand, and Vietnam (Figure 1). The LMR is largely tropical, with subtropical extensions in Laos, Myanmar, and Vietnam, and minor areas of temperate vegetation in Northern Myanmar (Peel et al., 2007). The region falls within the Indo-Burma biodiversity hotspot and over three-quarters of the population depends directly or indirectly on agriculture (Kityuttachai et al., 2016) and other economic activities including tourism, forestry, fishing, manufacturing, and energy production (Costenbader et al., 2015). Between 1973 and 2009, LMR lost almost a third of its forest cover with national level reductions amounting to 43% in Vietnam and Thailand, 24% in Lao PDR and Myanmar, and 22% in Cambodia (Watch, 2015). The main proximate drivers of land cover change in the past decade included expansion of agriculture and plantation estates, development of infrastructure and settlements, extraction of minerals and gas, dam and water infrastructure development, logging, and forest fires (Curtis et al., 2018). Underlying drivers of land cover change included population and economic growth, often intensified by weak governance (Stibig et al., 2013; Costenbader et al., 2015; Poortinga et al., 2019).
The HKH region extends 3,500 km over all or part of eight countries from Afghanistan in the west to Myanmar in the east (Figure 1). The eight countries include Afghanistan, Bangladesh, Bhutan, China, India, Myanmar, Nepal and Pakistan. The region has the highest number of mountain peaks in the world, including Mt. Everest. The predominant land cover of the region is grassland (54%), followed by agriculture (26%), and forest (14%) (Singh et al., 2011). It is the source of ten large Asian river systems and provides water, ecosystem services, and the basis for livelihoods to a population of around 210 million people. These river basins provide water to 1.3 billion people, a fifth of the world's population. It supports a rich variety of gene pools, species, ecosystems, and endemic species of global importance; making up four of the global biodiversity hotspots—Himalayan, Indo-Burma, Mountains of South-West China and Mountains of Central Asia (Myers et al., 2000).
Despite the emphasis on conservation, with 39% of land under protected areas (Chettri et al., 2008), the HKH region is facing significant deforestation (Ives and Messerli, 2003; Pandit et al., 2007). The dependency on fuel wood and timber from forests, population pressure for conversion of forest to agriculture and agriculture to built up area, shifting cultivation, and forest fire are among the many anthropogenic and natural causes of land cover change. The lack of historical land cover maps using a comparable and consistent methodology and classification scheme makes it difficult to analyze land cover changes within countries while inconsistency in typologies among different countries makes it difficult to develop regionally consistent land cover maps for regional analysis, studies and models.
There is a significant and growing body of evidence that meaningful participation of stakeholders in the identification of problems and the formulation of corresponding solutions has numerous benefits–especially in the context of complex, transdisciplinary challenges (Reed, 2008). To understand stakeholder needs in the HKH and LMR and focus their program strategies accordingly, each hub conducted both a general geospatial user needs assessment and a follow up workshop focused specifically on challenges regarding land cover mapping and monitoring.
The Mekong hub completed the general needs assessment phase most recently, from late 2014 to mid-2015. Data was collected through (1) a literature review of relevant reports and articles, (2) in person consultations with regional stakeholders (in part during regional workshops and focus groups), and (3) an online questionnaire. The stakeholder outreach campaign completed by the HKH hub was similar. The specific aims of the assessment were to identify geospatial data and technology needs in the following thematic areas:
• Key areas where geospatial information is considered important for decision making such as land governance and management, water governance and management, climate change adaptation, disaster risk assessment etc.
• Capacity building requirements such as basic GIS skills, managing complex server structures etc.
• Availability of geospatial data such as Landsat remote sensing products, land cover maps, flood forecast maps, etc.
• Data sharing practices and protocols such as sharing of data from public agencies with the public, sharing of data between agencies, standards for metadata and data quality to facilitate sharing, etc.
• Access to geospatial tools and applications including decision support tools, online information portals, custom desktop applications, etc.
A snowball sample strategy was used, which entails building up the initial sample size via enrolments of relevant stakeholders made by participants during the course of the study (Biernacki and Waldorf, 1981). This resulted in a list of country and regional stakeholders produced from government agencies, academic and research institutions, non-governmental organizations (NGOs) and other civil society organizations, multi- and bilateral aid agencies, United Nations agencies and similar extra-national governance and support institutions, private sector entities, and individual citizens. The sample is not a probabilistic sample, however there was good representation from the targeted organizations by type (e.g., government, civil society) and by country. Further details are provided in Appendix A.
Stakeholder consultations were implemented in two formats and included the participation of 199 people from 128 organizations. Consultation trips to Cambodia, Vietnam, Myanmar, Thailand, and the Lao PDR were conducted between December 2014 and May 2015. These trips included roundtable discussions and direct meetings.
An invitation to complete an online questionnaire was circulated via e-mail to more than 300 potential respondents thought to have valuable perspectives on the above topics. The respondents were queried regarding their professional role, institutional affiliation, and GIS and remote sensing background; perspectives on geospatial data needs and gaps, data sharing challenges, technology issues, capacity needs and gaps, and decision support tools and applications. A special effort was made to reach stakeholders who could not attend the live stakeholder consultations by means of the questionnaire.
Relevant published scientific reports, articles, and papers were used to complement the primary data collected through in-person consultations and the online questionnaire. The full list of documents reviewed is included in the Appendix A.
A series of four land cover mapping workshops were first conducted in the Mekong region from 2016 to 2017. The first workshop focused on user needs and objectives and outline a system design to address these challenges. The findings presented in this manuscript summarize the findings from this event. There were also three subsequent workshops: two production workshops and a product launch. Eighty stakeholders from the LMR contributed perspectives and ideas during these four regional land cover mapping workshops. Participants were involved in everything from conceptualization to classifications and algorithm development for emergent challenges from discussions.
The same approach of conducting at least four workshops began with regional partners in the HKH in 2018, with some slight adjustments. The initial needs assessment was a regional workshop that included participants from Afghanistan, Bangladesh, Nepal, and Myanmar. During the event the participants discussed and agreed on the conceptual framework and approach for typology and algorithm at regional scale. However, the two subsequent production workshops were organized as separate events with each mapping agency, as opposed to a series of integrated regional production workshops. These country level workshops are being conducted with larger groups of stakeholders to address the needs and approach for customizing the framework to need the country specific needs. This approach allowed for a greater number of participants from each agency, targeted the data generation focus in on one geospatial region, and helped garner agency ownership of the national product. At the culmination of the national workshops, the land cover products will be integrated into a regional data set.
Separate manuscripts are being prepared to describe the resulting land cover product and co-designed system architecture that resulted from these production workshops (Poortinga et al., 2019; Potapov et al., 2019; Saah et al., 2019, in press). The current manuscript summarizes only the results regarding challenges and design principles from the geospatial needs assessment campaign and first land cover focused workshop.
The results are presented in two sections. First is a description of the major land cover mapping challenges facing government and civil society organizations in the LMR and HKH regions. These results summarize the findings from the geospatial needs assessment, workshop activities, and experiences with local land cover mapping efforts from multiple agencies, countries, and programs. Second, we present the co-designed solution that was developed during the land cover monitoring workshop aimed at designing solution(s) to address these challenges. The goal was to design a system which can be implemented in a long-term sustainable fashion.
Participants of the regional geospatial needs assessment identified two major types of challenges. The first was an insufficient commitment to land cover mapping efforts, and the second was the challenge accessing geospatial infrastructure. The first major challenge highlights the integration issues associated with people, finance, and priorities. These issues are common among many organizational structures but have specific implications when trying to develop land cover mapping products in the developing world.
An online questionnaire was sent to 300 individuals within the LMR region with 55 responses, and 199 contributed to in-person meetings and discussion during the 2015 kick-off phase of the LMR SERVIR hub. Of the online survey respondents, 81% were familiar with GIS and internet mapping tools, while 60% reported familiarity with remote sensing. Similarly, 72% of respondents indicated that their organization acquired GIS data at least annually, while 54.5% indicated they acquired remotely sensed data at least annually. The majority (52 out of 53) of respondents indicated that the top priority application of geospatial data was for management purposes, including disaster reduction, early warning systems, and flood monitoring and management. Responses to gaps in data sharing and capacity building are depicted in Figure 2 (see also Tables 1–5 in Appendix B). These results identify an understanding of and a need for geospatial data and training within the LMR region.
Figure 2. Information on stakeholder responses and representation in the needs assessment process from the online questionnaire distributed in the Mekong region.
This online survey was not completed by individuals that attended in person consultation events, nor was it delivered in the HKH region. However, participants that attended the regional geospatial needs assessment workshops, focus groups, or the land cover monitoring workshop identified similar challenges.
Stakeholder involvement fostered participation which proved to be critical toward building a spirit of collaboration and trust. Based on the overall data gathered, the following salient challenges and gaps were identified which led to the co-development of a viable strategy to address key issues in land cover mapping.
Key challenges found from the assessment are outlined as follows. In general, participants pointed out insufficient commitment to the provision of resources, building of capacity, and setting up of unrealistically tight timelines for work for geospatial applications. Within these identified gaps, lack of coordination was noted to lead to disconnected workflows which reinforced many of the other stated challenges. The interrelated shortages were found to be lack of data access, computing power, availability of technical capacity and proper procedures, as well as modeling and use of consistent land cover maps.
With respect to data challenges, lack of consistent (spatially and temporally), pre-processed satellite data was expressed as a common obstacle which oftentimes led to production of outdated maps. This also meant stakeholders were not able to meet user requirements or provided inconsistent products with respect to user needs. Another byproduct of these practices is the production of multiple maps for different agency needs which were not based on the same underlying data. Participants also cited political/bureaucratic issues which are often entangled with scientific procedures and decisions–often these limitations are undocumented or unacknowledged (e.g., reference data collection and compilation, mapping procedures, etc.). One example that was referred to frequently in the discussions was the resistance to using the updated Mekong River Commission (MRC) land cover maps, because they had not been produced under explicitly approved procedures. Further analysis of the stakeholder inputs also revealed a lack of proper understanding of error structures in the map making process and final map products. This finding can be attributed to a lack of proper communication of geospatial science and related technologies in the region.
Results from the needs assessment in HKH show that multiple agencies are involved in land cover mapping, and have inconsistencies related to the classification schema, underlying satellite datasets used, and interpretation methods. The inconsistencies across spatial and temporal dimensions create problems when comparing land cover products and change analyses. The topographic variability in the HKH region also poses a challenge for generating land cover interpretation and collection of field data for training and validation. Restrictions on data sharing vary between countries. While multiple agencies within the same nation are independently producing their own land cover maps, generating redundant data sets with conflicting statistics. The typologies used are also varied across different countries, which makes it difficult to harmonize the datasets across the region.
A crippling limitation was the provisioning of and access to resources. Resource limitations included financial as well as human resources. Well funded organizations often could not find the right personnel, others reported that adequately staffed organizations had a hard time retaining qualified team members. Land cover mapping often requires diverse teams that are technically skilled and understand departmental needs. We found that within the represented organizations, these individuals were often over-tasked and under-resourced. Also local land cover mapping programs are often partially resourced for a specific project or program related to a country's donor activities with no core funding for sustainable support of land cover monitoring programs.
Local land cover mapping professionals had a hard time remaining abreast of new data, emerging technologies, and the latest science. Many organizations had standard operating procedures developed by donor countries which relied on accessing commercial data, which have not been updated to take advantage of new data streams and information products. This has often left departments using dated data products that introduce time lags. Geospatial professionals often lacked access to emerging new technologies due to budgetary constraints, licensing issues, or barriers in language. Professional geospatial growth was dependent on participation in donor-related activities focused on free and open source solutions. The same was also true for accessing data.
A key outcome of the stakeholder engagement process that aims to address the challenges stated above was the decision of stakeholder to collaborate on the co-development of a regional land cover monitoring system (RLCMS). This system can serve as a vehicle for a shared process of development and capacity building. Ultimately it serves as an example of a working land cover monitoring system based on best practices, and leverages state of the art technologies.
The first component of the system is the representation of a land cover scheme, or typology. The design of the typology is guided by the following list of 10 principles, which were established from the literature review (e.g., Running et al., 1994; Triepke et al., 2008; Lillesand et al., 2014) and group discussions:
1 Stakeholder engagement: Stakeholders identify their needs as a basis for the typology
2 Objective driven: A typology facilitates stakeholder objectives
3 Simple: A typology is no more complex than necessary to address stakeholder objectives
4 Exhaustive: Each location on the map is represented in the typology
5 Integrity among classes: Classes are mutually exclusive and have explicit class boundaries
6 Consistent: The typology is consistent from one area of the map to another, and from one generation of land cover mapping to another to support trends monitoring
7 Clear definitions: Map classes reflect measurable, diagnostic, biophysical features
8 Differentiates land use from land cover: The typology separates land use and land cover themes
9 Mappable: The typology is technologically and operationally feasible, for given budget and time constraints
10 Considers existing land cover schemes: Uses components of existing typologies whenever possible to maximize compatibility, shareability, and the use of available mapping technology, data, and applications.
Stakeholders further proposed that any land cover monitoring system appropriate for regional or national use meets (at minimum) the following design criteria:
1 Flexibility
• The system accommodates land cover typologies that vary by country by implementing a process to create and assemble biophysical land cover layers, referred to as primitives. These are continuous layers of biophysical attributes, such as fractional forest cover and forest height.
• Land cover primitives can be swapped for the most state-of-the-science product available at any time.
2 Consistency
• Every country uses the same primitives and the same assembly system, but can customize an assembly logic to create maps that accommodate regionally varying land cover semantics and objectives.
3 Based on remotely sensed data
• The system is data-driven with access to publicly available big Earth observing geodatasets, provided by cloud computing platforms.
4 Explicit quantification of uncertainty
• Monte-Carlo methods will be used in conjunction with independent validation data sets to incorporate uncertainty at the primitive level to provide pixel-based estimates of land cover uncertainty of the final map product.
5 Capacity building
• The collaborative nature of the system facilitates information and technology exchange.
• Free and broadly accessible, open tools are used wherever possible.
On the basis of these principles, the basic structure of a RLCMS typology and architecture was co-developed and a collaborative development process was completed through a series of four workshops and production segments from 2016 to 2017 in the Mekong. The HKH region began this process in 2018. During the first regional production workshop partners defined their end user objectives, a uniform land cover typology, data requirements, and laid out the collaborative design and production process (Steps 1 and 2 in Figure 3). The proposed land cover monitoring system production process includes six elements: typology development, land cover algorithms, reference data, accuracy assessment, assemblage of land cover algorithms, and production/metadata. At the conclusion of the first workshop, Mekong participants formed six co-development teams, each focusing on one of six key processes envisioned as central to the example system. However, the approach in the HKH was organized by as separate production events with each mapping agency.
Figure 3. Operational framework for a representative Regional Land Cover Monitoring System for the LMR. The arrows represent the direction of the process, while the dotted lines represent the iterative phases of the development of the system.
In the Mekong, the development of each of the six land cover monitoring system components would be completed and refined during three follow up workshops. During the second regional workshop, prototype algorithms for generating land cover primitives were drafted along with documentation of the tools, procedures and reference data needed to successfully execute the algorithms (steps 3 and 4 in Figure 3). Participants worked on three objectives during the third workshop, including (1) the development of a flexible, customizable assembly protocol to combine thematic primitives maps into a final land cover classification with the users' specific target land cover classes, (2) specifying accuracy assessment procedures and (3) documenting end user needs to ensure the system responds (steps 5 and 6 in Figure 3). The final workshop culminated in the presentation of preliminary land cover maps and a road map to support end users customize the products to meet their specific needs. The HKH production phase also followed this three step workshop approach. However, their focus was on testing, refining, and, as necessary, customizing design principles and algorithms developed by the Mekong team to meet the needs of a new set of stakeholders. These production phases were co-implemented with the Mekong SERVIR hub to ensure that lessons learned were transferred between the two hubs to continue the joint improvement of the system.
The system is implemented in Google Earth Engine, a freely available cloud computing infrastructure (Gorelick et al., 2017). For the first iteration, most primitives were built using a random forest classifier built primarily with data from the Landsat archive (Saah et al., in press). The Landsat program made the full archive of moderate resolution data with a long temporal range freely available (Wulder et al., 2012; Roy et al., 2014). However, because the system is modular other classification approaches are available and being tested, such as neural networks. Additionally, depending on the temporal requirements of the primitive layers, some are created from a data fusion between Landsat, MODIS (Justice et al., 1998), Sentinel-1 and Sentinel-2 (Berger et al., 2012), or climate hazards infrared precipitation (CHIRPS) (Funk et al., 2015) data sets, to name a few. For example, maps of rubber and palm oil plantations were developed using Sentinel-1 and Sentinel-2 data (Poortinga et al., 2019). The assembly logic includes a user-specified decision tree. The final land cover map product is created through a series of Monte Carlo iterations of the assembly logic and primitive error rates. Error rates are generated for both the primitive and land cover products using independent, probabilistic reference data sets. For the full details of the system architecture and co-designed products, please refer to Poortinga et al. (2019), Saah et al. (2019), Saah et al. (in press), and Potapov et al. (2019); other manuscripts are forthcoming. The Mekong data is available at https://rlcms-servir.adpc.net/en/. The launch date of the HKH data products is scheduled for 2020.
Land cover monitoring is a key activity which helps ensure health and sustainability of ecosystems. Earth observations and geospatial technology are playing an increasingly important role in this regard (Poortinga et al., 2017; Simons et al., 2017). In this paper we have outlined a SERVIR-facilitated stakeholder engaged approach to identify needs and key challenges faced in the countries of the Lower Mekong and Hindu Kush Himalayan regions. There is a shared view among stakeholders in the region working on land cover mapping that there are significant gaps in the capacity, technical tools and systems, and data needed to undertake effective land cover mapping. This includes lack of commitment of resources and support by relevant authorities and disconnected workflows due to limited access to data, computing power, and availability of technical capacity. Similar challenges were echoed during the stakeholder workshops in the HKH region. Many projects have been conducted in HKH countries to develop land cover maps for specific target years. In most cases these maps were generated using different data sources and typologies that makes it difficult to understand the changes. Also the topographic and terrain heterogeneity in the HKH region put additional challenges for accurate land cover mapping and monitoring.
We found this approach useful as it fostered collaboration and trust, leading to the co-development of a RLCMS, which aims to address all of the issues raised, and provide greatly improved access to, and quality of land cover data products. However, data sharing challenges and perceptions about data uncertainty remain formidable obstacles to analysis and acceptance of spatial data products. The former is due to existing data sharing policies and the latter reflects the widely held view that error and/or uncertainty is a flaw rather than an inherent feature of both scientific measurement and modeling. Uncertainty tended to be viewed as a negative to be minimized by people on both sides of the decision making process, to the point that it may be under-represented or simply left out of how results are presented to policy makers. However, presenting uncertainty hand-in-hand with the costs of making poor decisions can be vital in using scientific data to inform decision making (Reckhow, 1994). Fischhoff and Davis (2014) presented a useful outline for how to productively think about and present uncertainty in a decision-making context: careful identification of the core question to be answered, assessment of the degree and nature of uncertainty around the methods used to answer the question, and conveying that uncertainty in a clear, precise way that addresses any gaps that might exist in the mental models of the world used by decision makers compared to scientists or analysts. Ultimately, this boils down to understanding the important role of uncertainty as a natural part of research and decision-making that depends on everything from the precise question asked, whether one method was chosen over another, the real-world conditions in which the work takes place, and the appropriateness of a given data product to answer the question being asked. This process can be facilitated through the development and application of spatial data standards, with a standardized representation and level of acceptable error that can be applied across many data products. This could be expressed in terms of standards for completeness of coverage, confidence intervals and root mean square error in position, or through other criteria.
Respondents in both the online questionnaire and in-person discussions mentioned problems with data accessibility and sharing. Data producers are concerned about data security and ownership leading data providers to be possessive or defensive of the use of their data products, keeping them within their departments and minimizing availability to other work groups or agencies. While surprisingly common at many levels of government around the world, this behavior is antithetical to effective resource management, and greatly impedes the ability of groups to work together effectively for large scale projects, such as MRV for compliance with international programs like REDD+. These views and behaviors toward data sharing ultimately lead to inconsistent data across regions inhibiting progress toward environmental monitoring and policy. Moving toward an open, non-proprietary model of cooperation and data sharing is essential in establishing resilient, high functioning institutional arrangements necessary for sustainable development and addressing climate change challenges (GFOI, 2016). Many of these gaps can be addressed through the application of well established prevailing best practices such as, (1) data standardization, (2) metadata collections, (3) common data formats, and (4) open and transparent methods (i.e., free and open source code). Promoting these best practices facilitates interoperability, data exchange among different institutions, and reduction of barriers to access data.
Fortunately, in many cases the cost and technical expertise needed to apply these technologies and practices to address gaps is generally decreasing. The availability of high-quality remotely sensed and GIS data has steadily increased with processing packages and large public data repositories such as that hosted by Google Earth Engine (GEE) now freely available to academic, NGO, and public sectors (Gorelick et al., 2017; Markert et al., 2018; Poortinga et al., 2018). Many new tools, sites, and organizations have emerged to increase the power and ease of web-mapping and web-based map and GIS services, while reducing the cost and other barriers to entry. Similarly, high quality technical training for use with tools like GEE, QGIS, Python, R, SEPAL, OpenFORIS, and other free or low cost spatial analytical tools is now increasingly available. In addition to these tools that can be used for self-directed learning and improvement, many organizations (including the authors of this paper) are working to produce workshops that develop new tools and help build institutional capacity.
In summary, our stakeholder based approach can potentially be applied in other contexts to foster collaborative work toward addressing similar issues and building partnerships and networks including institutions, agencies and experts across countries with shared goals. In our case, this development process was fostered by actively engaging regional end user groups in discussion, consultation and capacity building to ensure that the RLCMS was effectively embedded in policy formulation and implementation at all levels regionally, while also acting as a means of extending regional cooperation in land management.
The datasets generated for this study are available on request to the corresponding author.
All authors listed have made a substantial, direct and intellectual contribution to the work, and approved it for publication.
KT, PC, AP, and RB are employed by spatial informatics group, LLC. NC is employed by Google. MP, IH, and PM are employed by RedCastle Resources.
The remaining authors declare that the research was conducted in the absence of any commercial or financial relationships that could be construed as a potential conflict of interest.
We thank the participating agencies, civil society organizations, and university partners for sharing the key challenges they face in creating and using geospatial data to inform decision making and for scoping out design criteria to guide the collaborative development of a shared regional land cover monitoring system. Support for this work was provided through the joint US Agency for International Development (USAID) and National Aeronautics and Space Administration (NASA) initiative SERVIR and the USAID Regional Development Mission for Asia.
The Supplementary Material for this article can be found online at: https://www.frontiersin.org/articles/10.3389/fenvs.2019.00150/full#supplementary-material
Berger, M., Moreno, J., Johannessen, J. A., Levelt, P. F., and Hanssen, R. F. (2012). Esa's sentinel missions in support of earth system science. Remote Sens. Environ. 120, 84–90. doi: 10.1016/j.rse.2011.07.023
Biernacki, P., and Waldorf, D. (1981). Snowball sampling: problems and techniques of chain referral sampling. Sociol. Methods Res. 10, 141–163. doi: 10.1177/004912418101000205
Bounoua, L., DeFries, R., Collatz, G. J., Sellers, P., and Khan, H. (2002). Effects of land cover conversion on surface climate. Clim. Change 52, 29–64. doi: 10.1023/A:1013051420309
Buchanan, G. M., Butchart, S. H., Dutson, G., Pilgrim, J. D., Steininger, M. K., Bishop, K. D., et al. (2008). Using remote sensing to inform conservation status assessment: estimates of recent deforestation rates on new britain and the impacts upon endemic birds. Biol. Conservat. 141, 56–66. doi: 10.1016/j.biocon.2007.08.023
Chettri, N., Shakya, B., Thapa, R., and Sharma, E. (2008). Status of a protected area system in the hindu kush-himalayas: an analysis of pa coverage. Int. J. Biodivers. Sci. Manage. 4, 164–178. doi: 10.3843/Biodiv.4.3:4
Costenbader, J., Broadhead, J., Yasmi, Y., and Durst, P. B. (2015). Drivers Affecting Forest Change in the Greater Mekong Subregion (GMS): An Overview. Rome: FAO.
Curtis, P. G., Slay, C. M., Harris, N. L., Tyukavina, A., and Hansen, M. C. (2018). Classifying drivers of global forest loss. Science 361, 1108–1111. doi: 10.1126/science.aau3445
Fischhoff, B., and Davis, A. L. (2014). Communicating scientific uncertainty. Proc. Natl. Acad. Sci. U.S.A. 111(Suppl. 4):13664–13671. doi: 10.1073/pnas.1317504111
Foley, J. A., DeFries, R., Asner, G. P., Barford, C., Bonan, G., Carpenter, S. R., et al. (2005). Global consequences of land use. Science 309, 570–574. doi: 10.1126/science.1111772
Fritz, S., See, L., and Rembold, F. (2010). Comparison of global and regional land cover maps with statistical information for the agricultural domain in Africa. Int. J. Remote Sens. 31, 2237–2256. doi: 10.1080/01431160902946598
Funk, C., Peterson, P., Landsfeld, M., Pedreros, D., Verdin, J., Shukla, S., et al. (2015). The climate hazards infrared precipitation with stations—a new environmental record for monitoring extremes. Sci. Data 2:150066. doi: 10.1038/sdata.2015.66
Ge, J., Qi, J., Lofgren, B., Moore, N., Torbick, N., and Olson, J. (2007). Impacts of land use/cover classification accuracy on regional climate simulations. J. Geophys. Res. 112, D05107, 1–12. doi: 10.1029/2006JD007404
GFOI (2016). Integration of Remote-Sensing and Ground-Based Observations for Estimation of Emissions and Removals of Greenhouse Gases in Forests: Methods and Guidance from the Global Forest Observations Initiative, Edition 2.0. Rome: Food and Agriculture Organization, 1–224.
Gong, P., Wang, J., Yu, L., Zhao, Y., Zhao, Y., Liang, L., et al. (2013). Finer resolution observation and monitoring of global land cover: first mapping results with landsat tm and etm+ data. Int. J. Remote Sens. 34, 2607–2654. doi: 10.1080/01431161.2012.748992
Gorelick, N., Hancher, M., Dixon, M., Ilyushchenko, S., Thau, D., and Moore, R. (2017). Google earth engine: planetary-scale geospatial analysis for everyone. Remote Sens. Environ. 202, 18–27. doi: 10.1016/j.rse.2017.06.031
Hall, D., Hirsch, P., and Li, T. M. (2011). Introduction to Powers of Exclusion: Land Dilemmas in Southeast Asia. Singapore: National University of Singapore Press; Honolulu, HI: University of Hawaii Press.
Hansen, M. C., Potapov, P. V., Moore, R., Hancher, M., Turubanova, S. A., Tyukavina, A., et al. (2013). High-resolution global maps of 21st-century forest cover change. Science 342, 850–853. doi: 10.1126/science.1244693
Hansen, M. C., and Reed, B. (2000). A comparison of the igbp discover and university of maryland 1 km global land cover products. Int. J. Remote Sens. 21, 1365–1373. doi: 10.1080/014311600210218
Herold, M., Mayaux, P., Woodcock, C., Baccini, A., and Schmullius, C. (2008). Some challenges in global land cover mapping: an assessment of agreement and accuracy in existing 1 km datasets. Remote Sens. Environ. 112, 2538–2556. doi: 10.1016/j.rse.2007.11.013
Hibbard, K., Janetos, A., van Vuuren, D. P., Pongratz, J., Rose, S. K., Betts, R., et al. (2010). Research priorities in land use and land-cover change for the earth system and integrated assessment modelling. Int. J. Climatol. 30, 2118–2128. doi: 10.1002/joc.2150
Imaoka, K., Kachi, M., Fujii, H., Murakami, H., Hori, M., Ono, A., et al. (2010). Global change observation mission (gcom) for monitoring carbon, water cycles, and climate change. Proc. IEEE 98, 717–734. doi: 10.1109/JPROC.2009.2036869
Ives, J. D., and Messerli, B. (2003). The Himalayan Dilemma: Reconciling Development and Conservation. London; New York, NY: Routledge.
Jha, M. K., and Chowdary, V. (2007). Challenges of using remote sensing and gis in developing nations. Hydrogeol. J. 15, 197–200. doi: 10.1007/s10040-006-0117-1
Jung, M., Henkel, K., Herold, M., and Churkina, G. (2006). Exploiting synergies of global land cover products for carbon cycle modeling. Remote Sens. Environ. 101, 534–553. doi: 10.1016/j.rse.2006.01.020
Justice, C. O., Vermote, E., Townshend, J. R., Defries, R., Roy, D. P., Hall, D. K., et al. (1998). The moderate resolution imaging spectroradiometer (modis): land remote sensing for global change research. IEEE Trans. Geosci. Remote Sens. 36, 1228–1249. doi: 10.1109/36.701075
Kityuttachai, K., Heng, S., and Sou, V. (2016). “Land cover map of the lower mekong basin,” in Technical Paper (Vientiane).
Leinenkugel, P., Kuenzer, C., Oppelt, N., and Dech, S. (2013). Characterisation of land surface phenology and land cover based on moderate resolution satellite data in cloud prone areas—a novel product for the mekong basin. Remote Sens. Environ. 136, 180–198. doi: 10.1016/j.rse.2013.05.004
Leinenkugel, P., Oppelt, N., and Kuenzer, C. (2014). A new land cover map for the mekong: Southeast Asia's largest transboundary river basin. Pacif. Geograph. 41, 10–14.
Lillesand, T., Kiefer, R. W., and Chipman, J. (2014). Remote Sensing and Image Interpretation. Hoboken, NJ: John Wiley & Sons.
Markert, K., Schmidt, C., Griffin, R., Flores, A., Poortinga, A., Saah, D., et al. (2018). Historical and operational monitoring of surface sediments in the lower mekong basin using landsat and google earth engine cloud computing. Remote Sens. 10:909. doi: 10.3390/rs10060909
McCallum, I., Obersteiner, M., Nilsson, S., and Shvidenko, A. (2006). A spatial comparison of four satellite derived 1 km global land cover datasets. Int. J. Appl. Earth Observat. Geoinformat. 8, 246–255. doi: 10.1016/j.jag.2005.12.002
Myers, N., Mittermeier, R. A., Mittermeier, C. G., Da Fonseca, G. A., and Kent, J. (2000). Biodiversity hotspots for conservation priorities. Nature 403:853. doi: 10.1038/35002501
Pandey, S., Bhandari, H. S., and Hardy, B. (2007). “Economic costs of drought and rice farmers' coping mechanisms: a cross-country comparative analysis,” in International Rice Research Institute (Los Baños).
Pandit, M., Sodhi, N. S., Koh, L. P., Bhaskar, A., and Brook, B. W. (2007). Unreported yet massive deforestation driving loss of endemic biodiversity in Indian Himalaya. Biodiver. Conservat. 16, 153–163. doi: 10.1007/s10531-006-9038-5
Peel, M., Finlayson, B., and McMahon, T. (2007). Updated world map of the koppen-geiger climate classification. Hydrol. Earth Syst. Sci. 11, 1633–1644.
Perera, K., Herath, S., Apan, A., and Samarakoon, L. (2010). Mapping mekong land cover at 250m resolution without in situ observations. Asian J. Geoinformat. 10, 31–41.
Poortinga, A., Bastiaanssen, W., Simons, G., Saah, D., Senay, G., Fenn, M., et al. (2017). A self-calibrating runoff and streamflow remote sensing model for ungauged basins using open-access earth observation data. Remote Sens. 9:86. doi: 10.3390/rs9010086
Poortinga, A., Clinton, N., Saah, D., Cutter, P., Chishtie, F., Markert, K., et al. (2018). An operational before-after-control-impact (BACI) designed platform for vegetation monitoring at planetary scale. Remote Sens. 10:760. doi: 10.3390/rs10050760
Poortinga, A., Tenneson, K., Shapiro, A., Nquyen, Q., San Aung, K., Chishtie, F., et al. (2019). Mapping plantations in myanmar by fusing landsat-8, sentinel-2 and sentinel-1 data along with systematic error quantification. Remote Sens. 11:831. doi: 10.3390/rs11070831
Potapov, P., Tyukavina, A., Turubanova, S., Talero, Y., Hernandez-Serna, A., Hansen, M., et al. (2019). Annual continuous fields of woody vegetation structure in the lower mekong region from 2000-2017 landsat time-series. Remote Sens. Environ. 232:111278. doi: 10.1016/j.rse.2019.111278
Reckhow, K. H. (1994). Importance of scientific uncertainty in decision making. Environ. Manage. 18, 161–166. doi: 10.1007/BF02393758
Reed, M. S. (2008). Stakeholder participation for environmental management: a literature review. Biol. Conservat. 141, 2417–2431. doi: 10.1016/j.biocon.2008.07.014
Roy, D. P., Wulder, M., Loveland, T. R., Woodcock, C., Allen, R., Anderson, M., et al. (2014). Landsat-8: science and product vision for terrestrial global change research. Remote Sens. Environ. 145, 154–172. doi: 10.1016/j.rse.2014.02.001
Running, S. W. (2008). Ecosystem disturbance, carbon, and climate. Science 321, 652–653. doi: 10.1126/science.1159607
Running, S. W., Loveland, T. R., and Pierce, L. L. (1994). A vegetation classification logic-based on remote-sensing for use in global biogeochemical models. Ambio 23, 77–81.
Saah, D., Johnson, G., Ashmall, B., Tondapu, G., Tenneson, K., Patterson, M., et al. (2019). Collect earth: an online tool for systematic reference data collection in land cover and use applications. Environ. Model. Softw 118, 166–171. doi: 10.1016/j.envsoft.2019.05.004
Saah, D., Tenneson, K., Poortinga, A., Nquyen, Q., and Chishtie, F. (in press). Primitives as building blocks for constructing land cover maps. Int. J. Appl. Earth Observat. Geoinformat.
Simons, G., Poortinga, A., Bastiaanssen, W. G., Saah, D., Troy, D., Hunink, J., et al. (2017). On Spatially Distributed Hydrological Ecosystem Services: Bridging the Quantitative Information Gap Using Remote Sensing and Hydrological Models. FutureWater.
Singh, S. P., Bassignana-Khadka, I., Singh Karky, B., and Sharma, E. (2011). Climate Change in the Hindu Kush-Himalayas: The State of Current Knowledge. Technical report, International Centre for Integrated Mountain Development (ICIMOD).
Stibig, H., Achard, F., Carboni, S., Raši, R., and Miettinen, J. (2013). Change in tropical forest cover of Southeast Asia from 1990 to 2010. Biogeosci. Discuss 10, 12625–12653. doi: 10.5194/bgd-10-12625-2013
Tolentino, P. L., Poortinga, A., Kanamaru, H., Keesstra, S., Maroulis, J., David, C. P. C., et al. (2016). Projected impact of climate change on hydrological regimes in the philippines. PLoS ONE 11:e0163941. doi: 10.1371/journal.pone.0163941
Triepke, F. J., Brewer, C. K., Leavell, D. M., and Novak, S. J. (2008). Mapping forest alliances and associations using fuzzy systems and nearest neighbor classifiers. Remote Sens. Environ. 112, 1037–1050. doi: 10.1016/j.rse.2007.07.014
Tucker, C. J., Townshend, J. R., and Goff, T. E. (1985). African land-cover classification using satellite data. Science 227, 369–375. doi: 10.1126/science.227.4685.369
Watch, G. F. (2015). Global Forest Watch. Washington, DC: World Resources Institute. Available online at: http://www.globalforestwatch.org (accessed June 2015).
Keywords: land cover/land use, GIS, remote sensing, Lower Mekong region, Hindu Kush region
Citation: Saah D, Tenneson K, Matin M, Uddin K, Cutter P, Poortinga A, Nguyen QH, Patterson M, Johnson G, Markert K, Flores A, Anderson E, Weigel A, Ellenberg WL, Bhargava R, Aekakkararungroj A, Bhandari B, Khanal N, Housman IW, Potapov P, Tyukavina A, Maus P, Ganz D, Clinton N and Chishtie F (2019) Land Cover Mapping in Data Scarce Environments: Challenges and Opportunities. Front. Environ. Sci. 7:150. doi: 10.3389/fenvs.2019.00150
Received: 02 June 2019; Accepted: 17 September 2019;
Published: 05 November 2019.
Edited by:
Niall Patrick Hanan, New Mexico State University, United StatesReviewed by:
Francis Kwabena Dwomoh, University of Oklahoma, United StatesCopyright © 2019 Saah, Tenneson, Matin, Uddin, Cutter, Poortinga, Nguyen, Patterson, Johnson, Markert, Flores, Anderson, Weigel, Ellenberg, Bhargava, Aekakkararungroj, Bhandari, Khanal, Housman, Potapov, Tyukavina, Maus, Ganz, Clinton and Chishtie. This is an open-access article distributed under the terms of the Creative Commons Attribution License (CC BY). The use, distribution or reproduction in other forums is permitted, provided the original author(s) and the copyright owner(s) are credited and that the original publication in this journal is cited, in accordance with accepted academic practice. No use, distribution or reproduction is permitted which does not comply with these terms.
*Correspondence: Farrukh Chishtie, ZmFycnVraC5jaGlzaHRpZUBhZHBjLm5ldA==
Disclaimer: All claims expressed in this article are solely those of the authors and do not necessarily represent those of their affiliated organizations, or those of the publisher, the editors and the reviewers. Any product that may be evaluated in this article or claim that may be made by its manufacturer is not guaranteed or endorsed by the publisher.
Research integrity at Frontiers
Learn more about the work of our research integrity team to safeguard the quality of each article we publish.