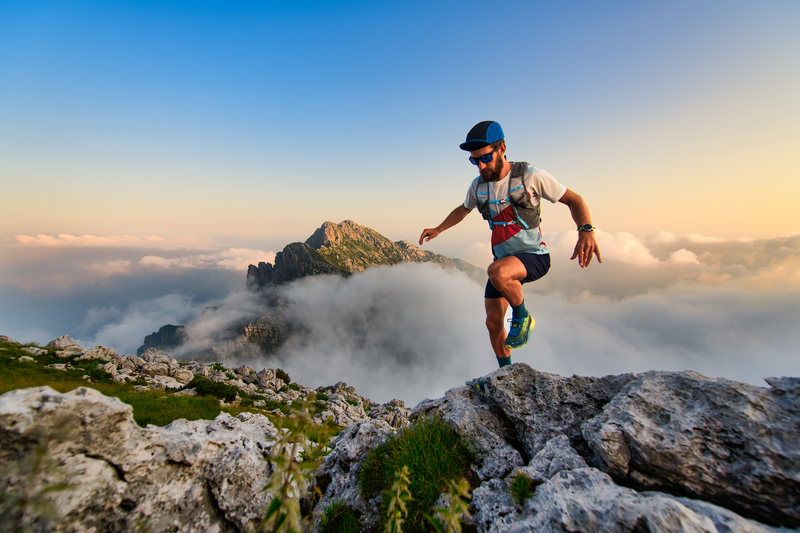
95% of researchers rate our articles as excellent or good
Learn more about the work of our research integrity team to safeguard the quality of each article we publish.
Find out more
REVIEW article
Front. Environ. Sci. , 27 August 2019
Sec. Science and Environmental Communication
Volume 7 - 2019 | https://doi.org/10.3389/fenvs.2019.00123
This article is part of the Research Topic Use of Earth Observations for Actionable Decision Making in the Developing World View all 17 articles
In this review paper, the authors identify priority areas, and opportunities for electric utilities in developing and emerging economies to incorporate Earth observation (EO) data into rural electrification planning, renewable energy resource assessment, distributed generation, grid operation and reliability, and disaster risk reduction and recovery efforts. Using a methodological framework, the authors conducted a comprehensive literature review of primary and gray literature. This paper reviews the many existing applications for EO data, such as the use of nighttime lights imagery for estimations of rural electrification, EO-derived normalized difference vegetation index (NDVI) products for vegetation monitoring for overhead transmission line management, solar radiance data for renewable energy project planning, and nowcasting for extreme weather events and other disaster monitoring. These and other applications can enhance energy security through improved governance of and access to modern and reliable electricity, renewable energy management, and disaster risk assessment in developing nations, paving the way for more sustainable social and economic development. Real-world examples of EO data use by utilities in developing and emerging economies, as well as barriers and opportunities for EO technology transfer, are discussed. Recommendations for stakeholder engagement, future EO training opportunities, and human capacity building are also presented.
The 2030 Agenda for Sustainable Development, adopted by the United Nations (UN) Member States in 2015, provides a shared blueprint of action centered around the 17 Sustainable Development Goals (SDGs), and an urgent call for action by all countries—developed and developing—to act in a global partnership to promote peace and prosperity. As one of the SDGs, UN SDG 71, “Ensuring access to affordable, reliable, sustainable, and modern energy for all” and electrification is a priority in many developing regions. Globally, about 1.1 billion people are without electricity, mostly in rural and remote locations in developing countries (International Energy Agency, 2017a). Lack of access to reliable, modern electricity is a significant obstacle to social and economic development, quality of life, and public safety, particularly within developing countries (International Energy Agency, 2014; Cook et al., 2015)2. SDG 7 plays a central role in supporting all other SDGs through direct and indirect contributions to environmental sustainability, human development, and sustainable economic growth.
Satellite-based Earth Observation (EO) data offer opportunities to advance understanding of both natural and human-induced global changes from which forecast models, information products and other tools could improve decision- and policy-making for various societal benefit areas (National Research Council, 2007; National Academies of Sciences, Engineering and Medicine, 2015; National Academies of Sciences, 2018). This is particularly important when addressing issues that are regional to global in scale or require resources that may necessitate transboundary cooperation. There are several distinct roles EO can offer when applied across atmospheric, marine, and terrestrial systems to assist with environmental governance, which could also potentially be applied to other societal benefit areas, such as the energy sector. The roles EO can offer center around the supply of information (including identifying data needed to address an issue, monitoring status of the issue or data trends over time, and assessing or evaluating success of applied solutions); assisting in early warning and/or disaster response; and documenting compliance with specific requirements or prohibitions (Young and Onoda, 2017). EO data also reduce the manual effort required to conduct feasibility assessments and increase accuracy. The combination of EOs and geospatial data with demographic and other sector-specific data offers an opportunity to provide the derived information needed by stakeholders to analyze and model potential resource related supply and demand scenarios, evaluate impacts across sectors and geographical regions, create maps and other visualizations to relay information, and otherwise assist end-users in making informed decisions that will contribute toward achieving the SDGs. Combined EO and demographic or socio-economic information can also provide a more nuanced analysis of environmental risks or management issues, allowing for improved governance of natural resources, energy resources, and populations. Applying this framework of EO applications to the energy sector offers a wide range of opportunities for facilitating developing countries' quest to improve access to reliable electricity and resilience infrastructure, incorporate renewable energy into the electric sector, and improve energy governance.
Public acceptance of new or unfamiliar technologies and infrastructure investments and siting is a crucial component in effectively addressing evolving energy needs and promoting good environmental governance (Haggett, 2009a; Devine-Wright, 2010). While regulatory frameworks vary drastically, in many countries the potential environmental, social, economic, and health impacts of large-scale projects, such as renewable energy installations, require a formal impact assessment process. For example, EO information can support environmental impact assessments (EIA) by providing historical, current, and projected environmental or human settlement-related information, contributing where knowledge and data gaps may otherwise occur and otherwise supporting consensus building between developers and community members (Maclean et al., 2014). Governing bodies must employ a constructive approach to obtaining public buy-in, particularly in renewable energy projects where community opposition to the unfamiliar and the Not-In-My-Backyard (“NIMBY”) phenomenon often plagues operational equipment siting (Larson and Krannich, 2016). Public engagement mechanisms take many forms and at a minimum should allow the public some degree of influence over the decision-making process. For example, granting local actors the authority over organization activities, such as coordination of meetings or creation of informational leaflets, enables a sense of ownership of the process and the project, thus increasing the level of trust (Areizaga et al., 2012). These and other approaches contribute to public acceptance of new approaches to energy management. The availability of EO information can support public engagement processes, such as in the siting of new renewable energy development projects (Hindmarsh and Matthews, 2008). Similarly, EO tools can also serve to provide stakeholders such as potential project developers or funders, grid operators, or national or private scale transmission entities, with renewable energy studies that take into account site-specific, seasonal variability forecasts for improved financial analyses (Kosmopoulos et al., 2018). Governance and public engagement can also be improved with greater understanding of human and physical environments or geography through EOs of appropriate resolution (Butler et al., 2011). EO tools can help to inform the public on potential impacts and opportunities of renewable energy installations by allowing visualizations to graphically represent relevant data or forecasts contributing to better understand potential impacts, community benefits, or justification for a new project. In Morocco, for example, EO information was used to identify settlements, and the boundaries of communities when assessing the development of large-scale solar installations (Hanger et al., 2016).
Additionally, the opportunity to engage end users of data products, such as those tools and information products incorporating EO, can influence future satellite mission design and ensure that investments in future EO capabilities are applications-oriented and serve both the scientific and user community. The need to foster closer relationships between the science and end user community, as well as incorporate socioeconomic factors in the planning and implementation of EO missions, is well documented (National Research Council, 2007; Brown et al., 2013; National Academies of Sciences, Engineering and Medicine, 2015).
This article provides an overview of the existing gaps and challenges to the use of EO data, current state of research, and examples of how electricity providers are incorporating EO data into rural electrification planning, renewable energy resource assessment, grid management, and disaster risk reduction and recovery efforts throughout the developing world. While there are myriad opportunities for improved utilization of EO by developing countries to meet their energy needs and goals, challenges to the successful use of EO remain and require careful consideration (Lahoz and Schneider, 2014). This review, based on a methodological literature search, is not meant to be exhaustive or encompassing of all applications, tools, research areas, or case studies, but instead sets a necessary foundation or baseline for understanding and addressing common barriers for EO uptake for the energy sector in the developing world. Toward that end, the article concludes with recommendations on how to maximize current applications and reduce barriers, particularly through stakeholder engagement, future tailored EO needs, and human capacity building.
A literature search that followed a methodological framework was conducted using targeted vocabulary terms to query Google Scholar and Google Search. Google Scholar allows simultaneous searching of a large number of unique publication types, including peer-reviewed journal articles, theses and dissertations, books, abstracts, and technical reports from academic publishers, professional societies, online repositories, universities, and other web sites. Google Scholar was selected over other scholarly databases because of its breadth of publication types. In an exploratory scan of literature, the authors found that journal articles were primarily focused on highly technical research or one-off projects, whereas non-traditional documents provided a better representation of information about the existing use of EO in real-world energy management applications. A perceived limitation to using Google Scholar is that publication quality is lower compared with other scholarly databases. However, a recent comparison of Google Scholar, Scopus, and Web of Science concluded that Google Scholar found “nearly all” Scopus and Web of Science citations and that “most citations found only by [Google Scholar] were from non-journal sources” (Martín-Martín et al., 2018). Another potential limitation to using Google Scholar is that results are ordered largely by citation count, which may not be as robust as other scholarly databases that rank by relevance. To mitigate this limitation, the authors used focused search strings to limit results to those most relevant and performed a scan of all document titles returned to assess potential relevancy. Titles deemed potentially relevant were marked for further review.
The Google Scholar review was supplemented by a query of Google Search to identify gray literature, which are non-peer reviewed materials produced by government, business, and industry in a variety of formats including white papers, presentations, newsletters, and other non-traditional materials where “publishing is not the primary activity of the producing body” (Schöpfel, 2010). Gray literature was an important component of this review because of the authors' desire to highlight the practical applications of EO use outside of a research context. To ensure coverage of all major aspects of space-based remote sensing for energy applications, hand searches of individual websites of select electricity providers and consortiums were also conducted.
Search terms related to the use of EO data for energy management were used to query online databases and resources. Broad keywords used for the search are summarized in a table included as Supplementary Material. The keywords were identified in a preliminary review of recent government reports and similar documents to identify priority areas within the electricity sector. Search terms were augmented as appropriate to include plurals and variants, root terms, alternative spellings, and synonyms. Terms were combined as necessary using Boolean AND/OR/NOT logic to achieve a manageable number of search results. Due to the extensive body of research available, the search focused on resources published from 2010 to the present. Using this search strategy, 9,856 potential titles related to EO data for energy management were identified. To further narrow the focus, the titles were initially scanned for relevancy. A total of 2,763 documents were down selected for abstract/summary review. If an abstract or executive summary suggested that a document was relevant or did not make relevance clear, the document was prioritized for full text review. Of the 2,763 abstracts/summaries reviewed, 384 documents were prioritized for full text review according to the following categories and criteria3:
• Key Studies (High Relevance): Documents that described in detail the specific uses of satellite imagery or data products related to priority areas or documents that described sector needs directly related to priority areas. A total of 261 documents were identified as “Key Studies” based on abstract/summary review. An in-depth review of full text was performed for these documents.
• Relevant Studies (Intermediate Relevance): Documents that broadly described general uses of satellite imagery or data products directly related to priority areas or that were highly technical in nature. A total of 55 documents were identified as “Relevant Studies” based on abstract/summary review. An expedited review of full text was performed for these documents. Upon expedited review, documents deemed high relevance were recategorized as “Key Studies” and subject to an in-depth review.
• Supporting Studies (Low Relevance): Documents that broadly described uses of satellite imagery or data products tangentially related to priority areas. A total of 68 documents were identified as “Supporting Studies” based on abstract/summary review. An expedited review of full text was performed for these documents. Upon expedited review, documents deemed high relevance were recategorized as “Key Studies” and subject to an in-depth review.
• Not Relevant: Documents not meeting the criteria for any of the preceding categories. A total of 2,379 abstracts/summaries reviewed were considered “Not Relevant” for the purposes of this project and were excluded from full text review.
The bibliography sections of particularly useful or key articles/reports were also further examined for additional relevant references. These secondary references were then obtained and reviewed for relevance according to the above criteria. An exhaustive review of all available literature, however, was not conducted. The final results of the literature search included peer-reviewed literature as well as gray literature, such as industry/consortia reports, workshop/conference reports, and key research reports from federal government agencies and others.
Results are presented in this section according to four key themes identified through the literature review: rural electrification, renewable energy, grid management, and disaster risk reduction, and response. For each theme, gaps and challenges are discussed, information regarding the current state of research is presented, and examples of the real-world use of EO data in developing countries are provided.
While significant progress toward UN SDG 7.1 “Ensuring access to affordable, reliable, sustainable and modern energy for all” has been achieved in certain regions of the world, a significant proportion of the global population, particularly in rural and remote locations, still lack this critical resource. For example, sub-Saharan African countries are among the least electrified in the world4. In Zambia, approximately 72% of a population of 17 million lacks access to electricity, with rates of inaccessibility as high as 95% in rural areas (Cader et al., 2018). Electrification rates in urban vs. rural areas may vary drastically even within a single country. For example, the electrification rate in the urban areas of Cambodia is 97% while only about 50% of Cambodia's rural population has access to modern electricity (International Energy Agency, 2017b). Cambodia plans to extend the national grid and build hydropower plants to improve the access of both urban and rural populations to electricity (International Energy Agency, 2017b).
In Indonesia and Myanmar, it is estimated that about 45 million people in total were without access to electricity in 2016 (International Energy Agency, 2017b). Improving access in these countries is a high governmental priority. Myanmar has developed a National Electrification Plan that includes expanding the centralized grid and financing DG/microgrid solutions. Similarly, electrification plans for Indonesia include extension of the existing grid, as well as microgrids that rely largely on renewable energy, such as hydro and solar.
The World Bank reported in 2014 that more than 300 million people in India are impacted by frequent outages or a complete lack of power due to insufficient infrastructure5. In 2015, the Government of India's Ministry of Power launched the “Deen Dayal Upadhyaya Gram Jyoti Yojana” scheme (DDUGJY) to reform the power sector, which claimed in April 2018 to have electrified all villages in India6. However, the Indian government considers a village “electrified” if it has basic electrical infrastructure, public places are electrified, and 10% of its households have electricity7. Given this interpretation, there appears to be ample opportunity to expand access to reliable electrification to most households in India.
Spatial information for energy access planning traditionally involves labor-intensive field studies and surveys to collect and analyze data. Freely available EO data have facilitated the development of spatial maps for developing countries, providing critical information for addressing the problem of electricity access. In areas where ground-based data are sparse, EO data can provide critical insight into environmental or energy-related parameters. Further, tools that combine satellite-based EO data with in-situ ground-based data can provide a valuable resource for understanding conditions for siting new projects or expanding grid infrastructure.
The application of EO resources offers the potential for electricity providers in developing nations to identify human settlement areas that may still be lacking the infrastructure and access to electricity and monitoring progress in electrification of remote, rural areas. While EO data cannot completely replace ground-based information, it provides an actionable, objective resource for identifying unelectrified areas, ideal siting locations for grid expansion, and areas suitable for self-sustaining microgrids to help in meeting electrification goals.
There are many barriers to electrification in developing countries, including inadequate policy or legal mechanisms, market drivers, governance structures, physical infrastructure, human capital, and data access or technological gaps (Urmee et al., 2009; Ahlborg and Hammar, 2014; Chauhan and Saini, 2015). In some cases, even land acquisition for project siting can be complicated due to protected land areas. Data on renewable resources is required but many developing countries are data poor in terms of ground-based data systems that monitor and map renewable resources. Often, data for electrification planning are difficult or costly to access because on-the-ground resources are required. EO data, particularly open access data, can be used to overcome the logistical and financial challenges of data acquisition in particular. The use of nighttime lights imagery is a well-established method for identifying unelectrified areas and the extent of rural settlements, although ground-based population data are often needed to supplement imagery-based population assessments to ensure accuracy (Cader et al., 2018). EO-based land use data have been used for the siting of grid infrastructure, although, without the use of data analytics, this method requires manual effort to assess aerial imagery for optimal locations. Further, internet access is required to obtain EO datasets, which can be a challenge in regions where accessibility is limited.
To assess rural access to electricity in regions where census data may be lacking, data are needed to determine population density in relation to existing utility assets. Current research in this area is largely focused on the development of machine learning models that analyze high-quality EO data to reduce manual efforts involving field surveys and human interpretation of aerial images. Researchers have demonstrated the use of data analytics with EO data products to assess population density and village attributes. Settlement identification and the evaluation of density distribution using open access data from satellites such as Landsat, the Advanced Spaceborne Thermal Emission and Reflection Radiometer (ASTER), Visible Infrared Imaging Radiometer Suite (VIIRS), and others have been validated by researchers as useful methods that can supplement survey data in regions where census data are incomplete or inaccurate. EO-derived data such as nighttime brightness, tree cover change, and thermal emission and reflection can be combined and modeled to predict likely population clusters (Stevens et al., 2015; Longwei and Dengsheng, 2016; Robinson et al., 2017; Engstrom et al., 2019). Researchers are also developing machine learning models with EO data to assess electric infrastructure assets, such as locations of power plants and overhead transmission lines, and to estimate asset reliability. Researchers at Duke University's Energy Data Analytics Lab recently developed a machine learning method that automates the evaluation of satellite imagery to determine electrification rates8. These and other models have demonstrated the ability to predict the current availability of electricity in remote areas and the quality of existing infrastructure using EO data from Landsat 8, Sentinel 1, VIIRS, Google Maps, and others (Varshney et al., 2015; Mann et al., 2016; Oshri et al., 2018). EO data and machine learning together will facilitate improved prediction and analytic capabilities for efficient grid planning and monitoring of electrification progress. The US National Aeronautics and Space Administration (NASA) introduced its Black Marble product in 2012 to replace the DMSP-OLS nighttime lights dataset. Black Marble provides images of Earth at night, taken from NASA's Suomi satellite using VIIRS, which offers a resolution significantly better than the previous system used to acquire nighttime lights data. Black Marble can be used to classify different types of population centers, such as urban, rural, suburban, villages, towns, etc. (Altaweel, 2018).
There are a limited number of current applications or real world uses of EO data in developing countries. Satellite imagery known as “nighttime lights” has been used to assess the expansion of electrification and electricity consumption over time and space. The National Oceanic and Atmospheric Administration (NOAA) maintains archived Defense Meteorological Satellite Program Operational Linescan System (DMSP-OLS) nighttime lights data from 1992 to 2013; however, the ongoing value of the DMSP-OLS dataset is limited because the data are no longer current. Nonetheless, researchers at the University of Michigan and The World Bank used this dataset to develop a map of the nighttime brightness of India's 600,000 villages as an indicator of electrification9. The project, known as India Lights, has been used extensively to assess the value of EO data for rural electrification planning. An analysis of the India Lights platform concluded that EO data are “surprisingly accurate” for assessing rural electrification, less so when the power supply is unreliable, but GIS maps and processing tools can help to improve assessments of brightness. On its own, nighttime lights may not be adequate but can be complemented with field information to identify specific locations for electricity expansion or microgrid installations (Dugoua et al., 2018).
EO data can also inform grid infrastructure siting. The Power Grid Company of Bangladesh Limited (PGCBL) used high-resolution satellite images in the development of a transmission line to expand the distribution system and increase access to electricity. The EO data were used in conjunction with maps and consultation with local stakeholders to assess geographic land features and alternative routes as options for the transmission line. EO data were crucial in identifying an optimal route that minimized impacts to existing infrastructure, settlements, and the environment. Specifically, satellite imagery was used to assess fish habitats along the proposed transmission route, urban areas, connecting roadways, river crossings, and other areas of importance (Asian Development Bank, 2017).
Microgrids, a variety of distributed generation (DG) that can operate autonomously, are widely used in developing countries to provide access to electricity in rural areas where connection to the central grid is not feasible. Powerhive East Africa Ltd., a U.S.-based microgrid solutions provider that is licensed to generate, distribute, and sell electricity in Kenya, uses a proprietary tool called SWARM for microgrid site selection10. The SWARM tool uses EO data to “identify potential customers and create preliminary microgrid designs for viable sites”11. In particular, the tool identifies the extent of the existing electric grid by incorporating nighttime lights imagery derived from NASA's Black Marble product, which is a cloud-free, higher resolution replacement for DMSP-OLS nighttime lights imagery (Román et al., 2019). As noted earlier, EO data can be complemented by ground-based data to assess population clusters and unelectrified areas.
Renewable energy is typically used in developing and emerging economies to power DG or microgrid systems in areas where connection to the centralized grid is impractical due to lack of infrastructure or other barriers. Often, these systems are part of a utility's overall solution to improving access to electricity. There are excellent renewable energy opportunities in many developing regions; however, policy, capacity, or financial barriers may prevent large-scale deployment of renewable energy technologies. Conversely, renewables-based small-scale microgrid systems have seen rapid expansion in developing nations and are a crucial component to providing electricity access to rural populations (Stiles and Murove, 2018) and making further progress toward SDG 7.1.
The primary renewable technologies used in developing regions are solar photovoltaics (PV), small wind turbines, small-scale hydroelectric power, and biomass combustion devices (Stiles and Murove, 2018). Growth and resource potential are strong, particularly for solar energy. In 2016, developing countries added about 34 GW of solar capacity (BloombergNEF, 2018). In sub-Saharan Africa, solar energy potential is tremendous, particularly for microgrid solutions. Studies suggest that there is a “theoretical annual electricity generation potential of 660,000 TWh for Solar PV in Africa” (Quansah et al., 2016). Significant solar resources are also noted in Mexico, regions of Central and South America, Southeast Asia, India, and the Middle East12.
In addition to the potential presented with solar energy, the World Energy Council estimates that global wind capacity could grow “from 435 GW in 2015 to 977 GW in 2030” (World Energy Council, 2016), with China, India, and Brazil leading installed capacity and continued sector growth among developing economies (Global Wind Energy Council, 2017). There is also substantial wind energy potential in Chile, Argentina, Kenya and other parts of sub-Saharan Africa, the Middle East, Vietnam, and the Philippines13. The greatest potential for utilization of wind energy is in remote villages where connection to a centralized grid is not feasible, and many of these countries have administrative goals for wind energy projects, such as the Philippines with a target of 2.3 GW of capacity by 2030 (International Energy Agency, 2017b) and Kenya with a goal to add 0.4 GW of capacity by 2020 (International Energy Agency, 2014).
Hydroelectric power is “the leading renewable source for electricity generation globally” (World Energy Council, 2016) with China, Brazil, and India as the fastest growing countries for this sector in 2017 (International Hydropower Association, 2018). Although, Brazil has recently removed several hydropower projects in favor of decentralized energy, other South American countries are seeing large growth in the hydroelectric power sector with plans for future plants in Peru, Columbia, and Argentina. Planned and installed hydroelectric projects also dominate the renewable energy sector in sub-Saharan Africa, where there exists significant untapped potential for hydroelectric power development (Stiles and Murove, 2018). While the potential growth of the hydroelectric power industry offers substantial opportunity for new use of EO data-derived products in this sector, so does the potential decline of water resources in certain regions due to environmental changes such as climate variability and change; therefore, electricity providers must adopt resilience planning for water shortages and manage risk accordingly if pursuing this energy source.
Geothermal energy is feasible in countries located in the “Ring of Fire,” such as Mexico, Indonesia, the Philippines, as well as other regions worldwide. Turkey and various Pacific coastal countries in Central and South America have seen strong growth in the geothermal sector largely due to supportive governmental policies (Stiles and Murove, 2018). Ethiopia and Kenya in Africa's Rift Valley also provide strong potential for geothermal power. Moreover, Costa Rica, El Salvador, and the Philippines derive more than 10 percent of their electricity from geothermal sources (Van Nguyen et al., 2015).
EO data can play an important role in assessing the feasibility of renewable energy systems and prospecting for resources. Solar energy resource assessment, management of PV installations, wind power estimation and wind farm siting, and environmental impact assessment are long established uses of EO data. Similarly, EO-derived thermal infrared data, surface wavelength measurements, and gravity anomalies have been used for more than a decade in the geothermal prospecting of large land areas. EO data are also commonly used to assess water quantity and availability for hydroelectric project planning and monitoring, determination of ideal dam locations and reservoir size, and environmental impact from rerouting or damming water.
For successful renewable energy projects, both government, and community expectations must be met. Participatory governance for consensus building among stakeholders should include participation mechanisms throughout the life of a project, from the early proposal stage to post-project. Public engagement plays a “crucial role” in the social acceptance of renewable energy technologies, and may involve collaborative decision-making, community shareholding, and public education and consultation (Langer et al., 2017). Such approaches provide the community some level of control, which facilitates trust between stakeholders, provided that the participation process is fair and just. However, research suggests that mandating engagement between developers and the public in the siting process is difficult, and without incentives, developers may not be motivated to address community concerns (Haggett, 2009b; Bell et al., 2013; Christidis et al., 2017). Furthermore, public concern over “not being heard” is a theme repeated in the literature (Walker et al., 2010; Natarajan et al., 2018). Thus, consideration must be given to all stakeholders to ensure effective collaboration and trust. The U.S. Department of Energy (DOE) maintains a toolkit for public involvement in renewable energy and infrastructure14 and among its key strategies include identifying issues of concerns and communicating clear and concise messages. Using EO data in maps or visualizations can offer the public clear, unbiased information on environmental parameters such as land use, land change, and available resources.
Trust is a critical factor in community adoption of renewable energy and associated data for energy assessment, such as EO. Level of knowledge and experience pertaining to renewable energy varies widely between policymakers, researchers, developers, and the public. Trust between stakeholders is largely contingent on accurate and timely communication, which is an “essential component of [the] public engagement process” (Pellizzone et al., 2015). Accurate information about proposed projects should be tailored to the community and disseminated using appropriate methods (e.g., use of leaflets instead of social media in regions where the internet is inaccessible; McNeish et al., 2019). Knowledge is a surmountable barrier to behavioral change provided that the public is confident in the information source and the information is relatable. For example, Liu et al. (2019) found that in many countries around the world, trust in policymakers or decision-makers has supported the successful development of renewable energy projects, including in China and the Netherlands. A similar study of renewable energy development in China found that the public was more open to project development when accurate and reliable data were presented, including maps and analyses (Guo et al., 2015). Encouraging learning through public participation is an effective tactic to changing public opposition of renewable energy (Parkins et al., 2018).
Challenges that have inhibited development of renewable energy-powered DG and microgrids in developing countries include difficulty accessing data, lack of tools for energy resource monitoring, and lack of accurate energy forecasts (Rinaldo et al., 2017). Lack of technical expertise can be a barrier to appropriately accessing and applying EO data products to renewable resource planning. To bridge this knowledge gap, there are a multitude of web-based viewers and mapping tools available to the public online. For example, the Global Solar Atlas provides yearly averaged solar resource and photovoltaic (PV) power potential data at a resolution of about 1 km in a user-friendly and intuitive color-coded map with optional data download15. Additionally, there remains an opportunity to provide capacity building efforts among electric utility providers or other key stakeholders to increase awareness of relevant tools and the benefits of utilizing EO data in this sector, and train technical staff in the proper access and application of EO data to meet specific needs they are facing.
Challenges such as resource monitoring and accuracy of energy forecasts may be mitigated through the application of EO data to assess renewable energy resources for siting and ongoing monitoring efforts. Although EO data have been used extensively for solar resource mapping, one pitfall is the spatial and temporal resolution for solar forecasts, which is much higher with ground-based images. Also, satellite instruments may have difficulty detecting cloud shadow, which can indicate sudden changes in solar irradiance, a possible issue for larger scale PV operations (Wan et al., 2015). EO-derived wind data are generally viewed as having good temporal and spatial coverage for wind resource planning, although care must be taken when selecting an appropriate dataset, as some wind data may be captured at a height suboptimal for comparison with wind turbines. For example, the NOAA High Density Infrared Cloud Drift Winds product16 records winds at an approximate height between 3,000 and 13,700 m above ground, whereas today's wind turbines have a hub height that rarely exceeds 125 m above ground (Lantz et al., 2017). Research supports this concern and has suggested that “wind profiles from lidar and masts” are more appropriate for estimating wind resource potential than EO data in estimating hub height winds (Hasager et al., 2010).
In general, geothermal energy has higher installation costs than solar and wind installations. As a result, geothermal energy projects in developing countries typically rely on government incentives, which can be a roadblock for many countries. Freely available EO data can address some of the outstanding financial challenges associated with geothermal exploration and plant siting by providing a more accessible data source compared with ground-based exploration.
Like other areas of research using EO data for energy solutions, emerging research largely involves the use of machine learning models to mechanize the analysis of satellite images. For example, Duke University Energy Data Analytics Lab researchers recently developed machine learning models that automate the identification of DG solar and wind resources using open access EO and other data17. Most other research in this field is primarily focused on the automation and validation of solar nowcasting and forecasting using machine learning methods based on satellite images to accurately predict fluctuating meteorological conditions and PV output (Eissa et al., 2013; Jang et al., 2016; Catalina et al., 2019; Cornejo-Bueno et al., 2019).
EO data are commonly used to assess water quantity and availability for hydroelectric project planning and monitoring, determine ideal dam locations and reservoir size, and assess environmental impacts from rerouting or damming water for hydroelectric projects. Electric utilities in India and Colombia have demonstrated the practical use of EO data for hydroelectric power projects, and India's Central Electricity Authority best practices for hydroelectric project development promote the use of satellite imagery for efficient interpretation of land use and geology for environmental impact of projects (Central Electricity Authority, 2002). For the Miel I hydroelectric power plant in Norcasia, Colombia, Isagen Productive Energy monitors and tracks landscape restoration efforts as part of the post-construction process. Satellite imagery and aerial photographs are used to assess changes in vegetation cover to understand the adaptation of hydrobiological communities to the reconstructed habitat. Isagen maintains a comprehensive environmental management plan for the dam and reservoir, and EO data are part of the restoration plan to address the effects of plant construction (Isagen Productive Energy, 2015).
EO-derived thermal infrared data, surface wavelength measurements, and gravity anomalies have been used for more than a decade in the identification of likely geothermal hotspots and exploration of geothermal energy potential of large land areas. To target ideal locations for geothermal drilling and well siting, the Kenya Electricity Generating Company, also known as KenGen, uses a GIS system to map potential hotspots identified using a combination of EO data, seismic maps, surface geology maps, and other data inputs (Wekesa and Gichini, 2016). KenGen also operates hydroelectric and wind power plants as part of its electric generation portfolio. To facilitate effective management of its resources, KenGen recently proposed the development of an “Integrated Spatial Resource Management” GIS system that would incorporate EO data products and other data sources, such as aerial photography, for resource and asset management (KenGen, 2018). EO data provide a more cost-effective data source for renewable energy exploration and plant siting compared with ground-based exploration.
The success of grid management, i.e., grid infrastructure operation and reliability, is often dependent on environmental factors. It is estimated that about 90 percent of load forecasting errors are weather-related, and the matching of supply and demand necessitates accurate data, as inaccuracies can instigate brownouts that impact other sectors, such as manufacturing and health care (Global Science and Technology Inc, 2016). Unreliable electricity plagues many developing countries, and frequent and long outages negatively impact socio-economic growth. Freely available EO data provide an opportunity for decision makers in developing countries to improve their knowledgebase. EO-derived data on current weather conditions and historical climate trends can be used in energy forecasting models and for infrastructure planning to improve grid reliability. Outage magnitude can be assessed and monitored with nighttime lights EO data. Inadequate line maintenance is also a cause of outages. Overhead transmission lines require routine vegetation management to mitigate the potential for falling limbs. Many global EO-derived products that measure vegetation are freely accessible, such as Terra/Aqua MODIS Normalized Difference Vegetation Indices (NDVIs) that are available at variable temporal and spatial resolutions18. These and other EO data products offer electric utilities an opportunity to enhance grid operation and reliability.
One challenge to using EO data for grid management includes the need for technical expertise to access and use EO data products. To mitigate this challenge, NASA, NOAA, and other organizations have developed freely available land use, nighttime lights, vegetation index products, and other useful datasets that are available in simple web-based map viewers. One example is the Visible Infrared Imaging Radiometer Suite (VIIRS) Day/Night Band (DNB) Nighttime Lights interactive map, which provides global, nightly imagery at a moderate spatial resolution19. However, it has been suggested that nighttime lights may be of limited value in areas where power is intermittent, depending on the temporal resolution of the EO data product (Dugoua et al., 2018).
Another potential barrier to adoption of EO data products by utilities is unfamiliarity that could lead to uncertainty or hesitation in using the data for decision making. Most electricity providers will need some level of training in the appropriate use and interpretation of EO data, even in its most simple form as a map viewer, which could potentially be another roadblock to use. Depending on the structure of the electricity sector in any given country or region, training may need to occur across administrative jurisdictions (National Research Council, 2003).
The most notable current trend in grid management is using artificial intelligence (AI) to manage grid operations and improve grid resilience. EO data are being integrated into AI systems along with information on the electrical distribution system and utility operations to build a body of knowledge that, with the proper algorithms, allows the grid to operate autonomously or alerts operators to vulnerabilities. Such systems could identify fluctuations in power supply and automatically reconfigure loads and resources (Kosowatz, 2018).
Many recent feasibility studies have been focused on demonstrating the effectiveness of high-resolution EO data for monitoring transmission lines for vegetation that might disrupt distribution. Satellite images can be used in transmission line management, for example, to determine whether the average height of vegetation beneath a power line exceeds a specific threshold and should be trimmed (Häme et al., 2016). EO data could potentially replace the more costly methods of using observation helicopters and unmanned aerial vehicles for visual inspection of overhead lines. Furthermore, emerging research shows the applicability of machine learning algorithms using EO-derived NDVI data to effectively identify transmission lines at highest risk of being affected by vegetation (Klein et al., 2018).
The Central American Electrical Interconnection System, known as SIEPAC, is an interconnection of the power grids of six Central American nations: Panama, Costa Rica, Honduras, Nicaragua, El Salvador, and Guatemala. SIEPAC regularly employs EO data for environmental impact and geotechnical studies for electric infrastructure development. For example, SIEPAC used Landsat images to understand features of geologic importance for transmission line siting in Nicaragua. The satellite images were used to identify complex volcanic features, areas of lava flow, and permanently flooded areas of flat relief. These aspects were used in part to assess the geological risk of constructing a transmission line to expand access to reliable electricity in the region (SIEPAC, 2004).
Falling trees account for most overhead transmission line failures, and downed power lines can spark fires. Energisa, a Brazilian power company, uses EO data to track vegetation conditions to identify trees that need pruning near transmission lines. Satellite imagery is coupled with an algorithm and digital photogrammetry, packaged as an “intelligent tool,” which can estimate the presence and height of trees near overhead lines, enabling effective and efficient vegetation management with minimal to no field effort required (Energisa, 2018).
Extreme weather events and other natural disasters that could impact the energy sector in developing countries may include hurricanes, flooding, volcanic eruptions, earthquakes, tsunamis, and landslides. The IPCC finds that weather- and climate-related disasters have resulted in increased economic loss and inequity exists among local adaptive capacity for disaster risk management, with developed countries often better equipped for disaster response (IPCC, 2012). The Sendai Framework for Disaster Risk Reduction targets to “substantially reduce disaster damage to critical infrastructure and disruption of basic services, among them health and educational facilities, including through developing their resilience by 203020.” EO data can be integrated into tools to improve forecasting, risk modeling, and recovery related to these extreme weather and natural disaster events and assist electric utility providers with resilience planning efforts to minimize impact to critical infrastructure and societal services.
Nighttime lights imagery is commonly used in the days immediately after a natural disaster to determine the extent of an electric outage. This information is crucial to identify neighborhoods in the greatest need of assistance. Precipitation, temperature, and other freely available remotely sensed weather data can lower costs and increase the accuracy of forecasting tools for natural disasters or extreme events. Similarly, high-resolution, near-real time daytime satellite imagery can be used to identify building damage, debris, and other obstacles that may impede rescue and recovery efforts after a disaster. Other research involves measurement of vegetation loss; monitoring of landslides; the application of machine learning and big data to improve tropical cyclone/wind forecasts and assess the likelihood of flooding as well as other types of disasters (Yu et al., 2018); and the use of differential interferometry from radar satellite data to monitor surface deformation in evaluating landslide and flooding hazards to electrical transmission lines (Li et al., 2018).
One challenge in using EO data to assess impacts to energy infrastructure and access after a natural disaster is that disaster response occurs across a wide array of sectors, with many moving parts, each uniquely important. In many countries, responding agencies may work in silos, which could result in a lack of information sharing or inefficient data gathering. These administrative difficulties could impede effective use of EO data across a multitude of sectors, including the power sector (Anderson et al., 2017). It may also be difficult to determine the best dataset or product resource in a time of crisis. Routine disaster monitoring may be useful in that, in the event of a disaster, systems would already be in place to inform decision making, communication methods could be established, and hazard mitigation plans would be developed and ready to implement at the first warning sign.
Other challenges include the availability of tools to handle large amounts of EO data and combine them with data from other sources, such as maps or measurement studies (Van Westen, 2000). EO data can also be also limited by an absence of global coverage and by dependence on weather and daylight conditions (Washaya et al., 2018).
Recent research is largely focused on implementing EO data for disaster warning systems. For example, the Institute of Hydrology, Meteorology and Environmental Studies of the Republic of Colombia (IDEAM) embarked on a project to strengthen disaster risk management in Colombia. The organization demonstrated the value of incorporating seasonal precipitation forecasts derived from GOES16 satellite data into a warning system for climate-related disasters and disruptions to energy generation (IDEAM, 2018). Researchers are also focused on using EO data to assess post-disaster damage. Research spans the rapid assessment of building damage to inform emergency responders of areas most severely impacted (Ramlal et al., 2018) to the evaluation of vegetation loss to inform vegetation protection strategies (Long et al., 2016; Hu and Smith, 2018).
Other similar research ties the use of EO data to flood management, tsunami monitoring and forecasting, landslide and volcanic activity monitoring, and general disaster management. Concepts covered span such aspects as applications of EO data, accuracy of EO-derived data processing, integrated management platforms, and case studies (Alfieri et al., 2018; Novellino et al., 2018; Schwarz et al., 2018; Wang and Xie, 2018; Korup et al., 2019).
Emerging research involves applying machine learning to natural disaster assessment and susceptibility. Projects include NASA's Deep Learning-based Hurricane Intensity Estimator, which uses machine learning methodology to estimate wind speeds by monitoring EO data from NASA satellites in near-real time, decreasing the time required to update the forecasting model21. Other research is largely focused on flood analysis, such as applying machine learning informed by land use maps derived from satellite images to estimate flood probability for a region of interest (Mojaddadi et al., 2017) and using a machine learning approach to analyze satellite images to classify and predict whether roads in a flooded region are passable (Said et al., 2018), among other projects.
Brazil's Energisa uses satellite imagery to assess weather conditions that contribute to grid interruptions and infrastructure damage after weather events have occurred. In the case of an event where strong winds overturned five distribution towers near Canarana, Energisa used images from GOES 16 to analyze the temperature and cloud conditions, along with wind intensity data from other sources, on the day of the event. Energisa is using these data to predict future events and to assess the need to reinforce certain assets to withstand extreme weather events (Energisa, 2017).
Empresa de Transmisión Eléctrica S.A. (ETESA), Panama's state transmission company, uses satellite imagery obtained from NOAA's geostationary satellite GOES-R in near-real time to “contribute to more accurate and reliable weather forecasts of severe weather perspectives.” EO-derived climatic parameters, such as air and soil temperature, relative humidity, barometric pressure, wind speed and direction, and solar radiation have expanded automation of Panama's National Hydrometeorological Network and modernized measurement and data processing systems. With the acquisition of receiving stations to obtain meteorological data from GOES-R, ETESA is better prepared for severe storms and climate-related events that may affect electrical transmission and distribution (Jimenez, 2016).
A review of recent literature and relevant websites summarized current or ongoing research areas as well as identified key opportunities for electricity providers to incorporate EO data into rural electrification planning, renewable energy assessment, grid management, and disaster risk reduction and recovery efforts. As discussed in this article, EO data have the potential to be useful in several ways for improving governance of and access to reliable, affordable, resilient, and sustainable energy. More specifically, the use of “nighttime lights” imagery for estimations of rural electrification, EO-derived NDVIs for vegetation monitoring for overhead transmission line management, solar radiance data for renewable energy project planning, and weather event nowcasting for natural disaster monitoring have all demonstrated value added for the energy sector in developing countries. Broadly, these and other applications of EO data can improve access to electricity, renewable energy management, and disaster risk management. This review paper provides an important resource in understanding the broad applications and future possibilities for improved energy access and management in the developing world.
This article intends to provide a global overview and baseline of gaps, challenges, and opportunities for using EO data in the energy sector in developing countries, as well as providing a sample of specific examples of real-world application already occurring in developing nations. However, it is important to note that such gaps, challenges, and opportunities may vary between and within regions and countries, and that our focus was specifically on developing countries, while developed countries, particularly in Europe, North America, and parts of Asia, may have additional examples of real-world application of EO data for energy access, renewable energy management, and disaster management. Due to the nature of the literature review and the keywords utilized, no particular region or country was the subject of in-depth search or analysis. Therefore, this review provides a high-level overview in developing countries only and may not include all relevant current applications in the energy sector worldwide. Further research into country specific needs in the developing world is needed to uncover additional, nuanced barriers to uptake of EO data in the energy sector given particular local context.
Nonetheless, the results of this review outline several important challenges, gaps, and opportunities for the continued and expanded utilization of EO data for the energy sector in developing countries. For example, though myriad EO information is available for free online, internet access can be unreliable or unavailable altogether, especially in remote areas. Some models require automated access to data continuously so that they can run with the most recent data outputs. Without consistent access to the internet, obtaining and/or applying EO data, models, tools, or other resources will remain difficult. To mitigate this risk, EO data could be accessed using infrastructure in urban areas where accessibility may be higher. Data could also be offered via data CDs or external hard drives where access is limited. Or, electricity providers could partner with other organizations, stakeholders, or value-added providers to access information or EO data in alternative arrangements. However, open data sharing is uncommon in several regions of the world, potentially making these types of arrangements complicated. For example, in Kenya, a qualitative study found that although study participants recognized the importance of sharing data, additional trust was needed between researchers before open data sharing policies would be followed (Jao et al., 2015). A study in Vietnam showed that while many Western countries share consensus around policy, infrastructure, and best practices in data sharing, these practices are less often found in areas where institutions or universities face funding limitations (e.g., increasing a feeling of competition between potential collaborators) or a lack of technical, governance, or practical solutions for data sharing (Merson et al., 2015). Pursuing a culture of transparency and data sharing, alongside concrete policies, best practices, and other drivers, is important within the research and applied management or decision-making communities.
Even with the ability to access EO data online, analysts or other electricity provider staff may lack general awareness of relevant EO resources, not to mention how to appropriately apply such resources to their planning and decision-making efforts. NASA, NOAA, and other organizations have developed freely available land use, “nighttime lights,” vegetation index products, and other useful datasets that are available in simple web-based map viewers or data interfaces that are meant to be easily accessible for end users. However, without capacity building efforts, through technical trainings, demonstrations or provision of case studies, understanding where and how to obtain EO resources–and how to appropriately apply them—will remain a barrier. This can lead to uncertainty or hesitation in using EO data for improved decision making and management. Understanding data or model uncertainty or other technical limitations is especially important, particularly with regard to climate information and projections, and training should accompany initial application of related datasets. Training on topics could be as preliminary as data management using statistical or geospatial software to advanced statistical or AI techniques.
If existing, such training is not always available in the language, time zone, or context needed for a developing country. Researchers and data providers should ensure that trainings or capacity building is available and adapted for audiences around the world. Trainings should take place over extended periods of time so that time-constrained staff are able to accommodate the additional workload. Focusing on several staff increases the likelihood of technical capabilities remaining despite turnover. Documentation of procedures including analyses, modeling, or other activities should be detailed and maintained over time.
Limitations in staffing is a common but serious practical human capacity challenge. Many electricity providers (as well as government ministries working in environmental planning or energy) are short-staffed and face technical expertise gaps. If management is aware of the benefit of using EO for energy management, resources could be committed over time to increase analysis and planning utilizing EO resources. In addition, collaboration between the private sector and government or research bodies, who may have resources or technical expertise, could benefit electricity providers. While consultants are often able to provide these services, relying on third party expertise is expensive and unsustainable.
Instead, further expanding knowledge of EO data applications through case studies, training, and technical assistance specific to electricity providers can facilitate access to reliable electricity in developing countries and lead to increased use of renewable energy and microgrids, and natural disaster risk reduction. There are resources available for training, such as NASA's Applied Remote Sensing Training (ARSET) program22 which provides online trainings (guided and self-instructed) on several EO applications. NOAA's Climate Resilience Toolkit (CRT)23 includes case studies and other resources on EO applications across many sectors. Other organizations such as IRENA or the International Energy Agency (IEA) similarly offer resources for EO application in the energy management sector. While several key resources for energy management using EO exist (Eckman and Stackhouse, 2012), additional work is needed to determine the use of those resources in developing countries. Further, additional attention should be paid to if applications are specifically tailored and adapted for electric utilities or electricity providers in the developing world.
There are also several opportunities for the research community to address these barriers and contribute to the uptake of EO data for utilities in the developing world. Data providers could provide or compile case studies on how their datasets have been utilized by other users. Case studies of existing applications could highlight leaders who have demonstrated EO data applications. Data providers can seek to make their datasets available in intuitive, easily accessible formats, providing ample documentation, and guidance on possible applications. User communities have noted in previous studies that datasets should be tailored to user requirements in easily accessible formats that reflect the software used for analysis (Lautenbacher, 2006).
Communities of practice or other data end user networks offer opportunities for knowledge exchange and other mechanisms for data users to share information and learn from each other. South-South information exchange, collaboration, and communication also allow for sharing of common ideas, needs, challenges, and innovative solutions within and between developing countries. The United Nations (UN) has advocated for South-South exchange in tackling issues such as climate change, inequality, and energy access24. In fact, several UN partnerships exist based on several themes relevant to sustainable development; data applications for energy access and management should be added as a key topic of interest25. The UN Framework Convention of Climate Change (UNFCCC) has similarly called for South-South cooperation and “triangular cooperation” on technologies for adaptation to climate change on topics such as technology transfer and capacity building26.
Nonetheless, through broad multi-directional dialogue exchange to understand end user needs and opportunities from the earth observation research community, refinement of existing tools and resources, or the development of new tools may be able to continue to progress efforts to improve electrification, renewable energy management and disaster management in the energy sector among developing nations. There may be the opportunity to leverage existing venues and networks that bring the EO research community together, such as the Group on Earth Observations (GEO) or internationally attended scientific meetings such as the American Geophysical Union (AGU) or American Meteorological Society (AMS). Participation in these organizations can allow for the development of innovative solutions and set new research strategies for future efforts. Government science agencies as well as other private data providers could have a role in facilitating those linkages. For example, the SERVIR program27, jointly funded by NASA and the US Agency for International Development (USAID), offers a unique opportunity for funded research teams to work together with data users to apply EO datasets to development-related questions including governance of resources and communities. Similar efforts could encourage a focus on energy management, providing scientific expertise to practical applications brought forward by practitioners, decision-makers, or other stakeholders in developing countries. Increased attention, in the form of funding, research, technology transfer, and dialogue, should be paid to energy applications given the central role that energy access plays in so many SDGs as an enabler of sustainable development28.
AL conducted the literature review and was the primary author of the manuscript. AT, NS, and TM assisted in writing. AT submitted the manuscript. All provided review and revision.
This work was funded through NASA Grant No. 80NSSC18K0325.
All authors are employed by Battelle Memorial Institute, a non-profit research institute. All authors declare that the research was conducted in the absence of any commercial or financial relationships that could be construed as a potential conflict of interest.
The authors acknowledge the support of funding from NASA in making this research possible, as well as the contributions of Dr. Richard Eckman and Dr. Paul Stackhouse. The authors also acknowledge the support of Dr. Craig Zamuda of the US Department of Energy.
The Supplementary Material for this article can be found online at: https://www.frontiersin.org/articles/10.3389/fenvs.2019.00123/full#supplementary-material
1. ^https://sustainabledevelopment.un.org/sdg7
2. ^https://sustainabledevelopment.un.org/?page=view&nr=2749&type=13&menu=1634
3. ^The complete list of references deemed relevant are included in Supplementary Material.
4. ^https://data.worldbank.org/indicator/EG.ELC.ACCS.ZS
5. ^http://www.worldbank.org/en/news/feature/2014/06/24/switching-on-power-sector-reform-in-india
6. ^https://twitter.com/narendramodi/status/990455176581517312
7. ^http://www.ddugjy.gov.in/portal/definition_electrified_village.jsp
8. ^https://bassconnections.duke.edu/project-teams/energy-data-analytics-lab-electricity-access-developing-countries-aerial-imagery-2017
9. ^http://india.nightlights.io
10. ^http://www.powerhive.com/our-technology/
11. ^Ibid.
12. ^https://solargis.com/maps-and-gis-data/download
13. ^https://www.globalwindatlas.info/
14. ^https://openei.org/wiki/RAPID/Best_Practices/Public_Involvement_in_Renewable_Energy_and_Infrastructure_Project_Development
15. ^https://globalsolaratlas.info/
16. ^https://www.ospo.noaa.gov/Products/atmosphere/wind.html
17. ^https://ssri.duke.edu/news/gauging-renewable-energy-generation-using-satellite-imagery
18. ^https://modis.gsfc.nasa.gov/data/dataprod/mod13.php
19. ^https://maps.ngdc.noaa.gov/viewers/VIIRS_DNB_nighttime_imagery/index.html
20. ^https://www.unisdr.org/we/coordinate/sendai-framework
21. ^http://hurricane.dsig.net/
22. ^https://arset.gsfc.nasa.gov/
23. ^https://toolkit.climate.gov/
24. ^https://www.un.org/press/en/2019/dev3388.doc.htm
25. ^http://www.expo.unsouthsouth.org/resources/gssd-expo-solutions/
26. ^http://unfccc.int/ttclear/misc_/StaticFiles/gnwoerk_static/tn_meetings/18e2ee898379443c85397bd1b3d210a4/ee99ac~7846a44132b3bd6c3dca058c5a.pdf
27. ^https://www.nasa.gov/mission_pages/servir/index.html
28. ^https://sustainabledevelopment.un.org/?page=view&nr=2749&type=13&menu=1634
Ahlborg, H., and Hammar, L. (2014). Drivers and barriers to rural electrification in Tanzania and Mozambique–Grid-extension, off-grid, and renewable energy technologies. Renew. Energy 61, 117–124. doi: 10.1016/j.renene.2012.09.057
Alfieri, L., Cohen, S., Galantowicz, J., Schumann, G. J.-P., Trigg, M. A., Zsoter, E., et al. (2018). A global network for operational flood risk reduction. Environ. Sci. Policy 84, 149–158. doi: 10.1016/j.envsci.2018.03.014
Anderson, K., Ryan, B., Sonntag, W., Kavvada, A., and Friedl, L. (2017). Earth observation in service of the 2030 Agenda for Sustainable Development. Geo Spatial Inform. Sci. 2, 77–96. doi: 10.1080/10095020.2017.1333230
Areizaga, J., Sano, M., Medina, R., and Juanes, J. (2012). Improving public engagement in ICZM: a practical approach. J. Environ. Manage. 109, 123–135. doi: 10.1016/j.jenvman.2012.05.006
Asian Development Bank (2017) Bangladesh: Bangladesh Power System Enhancement and Efficiency Improvement Project: Initial Environmental Examination. Manila: ADB.
Bell, D., Gray, T., Haggett, C., and Swaffield, J. (2013). Re-visiting the ‘Social Gap': public opinion and relations of power in the local politics of wind energy. Env. Polit. 22, 115–135 doi: 10.1080/09644016.2013.755793
BloombergNEF (2018). Climatescope: Emerging Markets Outlook 2018. Energy Transition in the World's Fastest Growing Economies. London: BloombergNEF.
Brown, M. E., Escobar, V. M., Aschbacher, J., Milagro-Pérez, M. P., Doorn, B., Macauley, M. K., et al. (2013). Policy for robust space-based earth science, technology and applications. Space Policy 29, 76–82. doi: 10.1016/j.spacepol.2012.11.007
Butler, C., Parkhill, K., and Pidgeon, N. (2011). “From the material to the imagined: public engagement with low carbon technologies in a nuclear community,” in Renewable Energy and the Public: From NIMBY to Participation, ed P. Devine-Wright (London; Washington, DC: Earthscan, 301–316.
Cader, C., Radu, A., Bertheau, P., and Blechinger, P. (2018). “Remote sensing techniques for village identification: improved electrification planning for Zambia,” in Africa-EU Renewable Energy Research and Innovation Symposium 2018, eds M. Mpholo, D. Steuerwald, and T. Kukeera (Cham: Springer), 91–96. doi: 10.1007/978-3-319-93438-9_7
Catalina, A., Torres-Barrán, A., Alaíz, C. M., and Dorronsoro, J. R. (2019). Machine learning nowcasting of PV energy using satellite data. Neural Proc. Lett. 1–19. doi: 10.1007/s11063-018-09969-1
Central Electricity Authority (2002). Best Practices on Survey and Investigations of Hydro Electric Projects. New Delhi: Central Electricity Authority.
Chauhan, A., and Saini, R. P. (2015). Renewable energy based off-grid rural electrification in Uttarakhand state of India: technology options, modelling method, barriers and recommendations. Renew. Sustain. Energy Rev. 51, 662–681. doi: 10.1016/j.rser.2015.06.043
Christidis, T., Lewis, G., and Bigelow, P. (2017). Understanding support and opposition to wind turbine development in Ontario, Canada and assessing possible steps for future development. Renew. Energy 112, 93–103. doi: 10.1016/j.renene.2017.05.005
Cook, N., Campbell, R. J., Brown, P., and Ratner, M. (2015). Powering Africa: Challenges of and U.S. Aid for Electrification in Africa. Washington, DC: Congressional Research Service.
Cornejo-Bueno, L., Casanova-Mateo, C., Sanz-Justo, J., and Salcedo-Sanz, S. (2019). Machine learning regressors for solar radiation estimation from satellite data. Solar Energy 183, 768–775. doi: 10.1016/j.solener.2019.03.079
Devine-Wright, P. (2010). Public engagement with large-scale renewable energy technologies: breaking the cycle of NIMBYism. Wiley Int. Rev. Clim. Change 2, 19–26. doi: 10.1002/wcc.89
Dugoua, E., Kennedy, R., and Urpelainen, J. (2018). Satellite data for the social sciences: measuring rural electrification with night-time lights. Int. J. Remote Sens., 39, 2690–2701. doi: 10.1080/01431161.2017.1420936
Eckman, R. S., and Stackhouse, P. W. Jr. (2012). CEOS contributions to informing energy management and policy decision making using space-based Earth observations. Appl. Energy 90, 206–210. doi: 10.1016/j.apenergy.2011.03.001
Eissa, Y., Marpu, P. R., Gherboudj, I., Ghedira, H., Ouarda, T. B. M. J., and Chiesa, M. (2013). Artificial neural network based model for retrieval of the direct normal, diffuse horizontal and global horizontal irradiances using SEVIRI images. Solar Energy 89, 1–16. doi: 10.1016/j.solener.2012.12.008
Energisa (2018). Satellite Imagery and Pruning Management: A Revolutionary Approach. Tulsa: Distributech/Clarion Energy.
Engstrom, R., Newhouse, D., and Soundararajan, V. (2019). Estimating Small Area Population Density Using Survey Data and Satellite Imagery: An Application to Sri Lanka. Washington, DC: The World Bank. doi: 10.1596/1813-9450-8776
Global Science and Technology Inc (2016). NCEI Climate Products and Services Market Analysis: Power Sector Engagement. Oxford: Acclimatise.
Guo, Y., Ru, P., Su, J., and Anadon, L. D. (2015). Not in my backyard, but not far away from me: Local acceptance of wind power in China. Energy 82, 722–733. doi: 10.1016/j.energy.2015.01.082
Haggett, C. (2009a). “Chapter 2: Planning and persuasion: public engagement in renewable energy decision-making,” in Renewable Energy and the Public: from NIMBY to Participation, ed P. Devine-Wright (London: Earthscan).
Haggett, C. (2009b). “Public engagement in planning for renewable energy,” in Planning for Climate Change: Strategies for Mitigation and Adaptation for Spatial Planners, eds S. Davoudi, J. Crawford, and A. Mehmood (London: Taylor and Francis, 297–307.
Häme, L., Norppa, J., Salovaara, P., and Pylvänäinen, J. (2016). Power line monitoring using optical satellite data. CIRED Workshop, Helsinki, Paper 0383. Available online at: www.semanticscholar.org
Hanger, S., Komendantova, N., Schinke, B., Zejli, D., Ihlal, A., and Patt, A. (2016). Community acceptance of large-scale solar energy installations in developing countries: Evidence from Morocco. Energy Res. Social Sci. 14, 80–89. doi: 10.1016/j.erss.2016.01.010
Hasager, C. B., Badger, M., Mouche, A., Astrup, P., Stoffelen, A., and Karagali, I. (2010). “Offshore wind resource estimation using satellite images: what are the challenges?” in Geophysical Research Abstracts, eds H. Charlotte Bay, B. Merete, M. Alexis, A. Poul, S. Ad, and K. Ioanna (Munich: EGU), 2010–4650.
Hindmarsh, R., and Matthews, C. (2008). Deliberative speak at the turbine face: community engagement, wind farms, and renewable energy transitions, in Australia. J. Environ. Policy Planning 10, 217–232. doi: 10.1080/15239080802242662
Hu, T., and Smith, R. B. (2018). The impact of hurricane maria on the vegetation of dominica and puerto rico using multispectral remote sensing. Remote Sens. 10, 827–847. doi: 10.3390/rs10060827
IDEAM (2018). Strengthening Hydrometeorological Monitoring. Early Warning Systems and Climate Services in Colombia: The IDEAM, a Successful Case in Latin America. Bogotá: IDEAM.
International Energy Agency (2014). Africa Energy Outlook: A Focus on Energy Prospects in Sub-Saharan Africa. Paris: OECD/IEA. doi: 10.1787/weo-2014-en
International Energy Agency (2017a). Energy Access Outlook 2017: From Poverty to Prosperity. Paris: OECD/IEA.
International Hydropower Association (2018). Hydropower Status Report: Sector Trends and Insights. London: IHA.
IPCC (2012) “Summary for policymakers,” in Managing the Risks of Extreme Events Disasters to Advance Climate Change Adaptation, eds C. B. Field, V. Barros, T. F. Stocker, D. Qin, D. J. Dokken, K. L. Ebi, et al. (Cambridge; New York, NY: A Special Report of Working Groups, I. II of the Intergovernmental Panel on Climate Change; Cambridge University Press, 1–19.
Isagen Productive Energy (2015). Environmental Management Plan: Miel I Hydroelectric Power Plant. Medellin: Isagen.
Jang, H. S., Bae, K. Y., Park, H., and Sung, D. K. (2016). Solar power prediction based on satellite images and support vector machine. IEEE Trans. Sustain. Energy, 7, 1255–1263. doi: 10.1109/TSTE.2016.2535466
Jao, I., Kombe, F., Mqalukore, S., Bull, S., Parker, M., Kamuya, D., et al. (2015). Research Stakeholders' views on benefits and challenges for public health research data sharing in kenya: the importance of trust and social relations. PLoS ONE 10:e0135545. doi: 10.1371/journal.pone.0135545
KenGen (2018). Request for Proposals (RFP) for Development of an Integrated Spatial Resources Management for KenGen. Nariobi: KenGen.
Klein, L., Wamburu, J. M., and Hamann, H. (2018). A Deep Learning Framework for Vegetation Management for Electrical Utilities Using Multispectral High-Resolution Satellite Imagery. Washington, DC: American Geophysical Union, EP51E−1873.
Korup, O., Seidemann, J., and Mohr, C. H. (2019). Increased landslide activity on forested hillslopes following two recent volcanic eruptions in Chile. Nat. Geosci. 12, 284–289. doi: 10.1038/s41561-019-0315-9
Kosmopoulos, P. G., Kazadzis, S., El-Askary, H., Taylor, M., Gkikas, A., Prestakis, E., et al. (2018). Earth-observation-based estimation and forecasting of particulate matter impact on solar energy in Egypt. Remote Sens. 12, 1870–1893. doi: 10.3390/rs10121870
Lahoz, W. A., and Schneider, P. (2014). Data assimilation: making sense of Earth Observation. Front. Environ. Sci. 28, 1–28. doi: 10.3389/fenvs.2014.00016
Langer, K., Decker, T., and Menrad, K. (2017). Public participation in wind energy projects located in Germany: which form of participation is the key to acceptance? Renew. Energy 112, 63–73. doi: 10.1016/j.renene.2017.05.021
Lantz, E., Roberts, O., and Dykes, K. (2017). Trends, Opportunities, and Challenges for Tall Wind Turbine and Tower Technologies, Presented at the American Wind Energy Association Wind Power 2017 Conference, Anaheim, California. Golden, CO: NREL.
Larson, E. C., and Krannich, R. S. (2016). “A Great Idea, Just Not Near Me!” understanding public attitudes about renewable energy facilities. Soc. Nat. Resources 29, 1436–1451. doi: 10.1080/08941920.2016.1150536
Lautenbacher, C. C. (2006). The global earth observation system of systems: Science serving society. Space Policy 22, 8–11. doi: 10.1016/j.spacepol.2005.12.004
Li, G., Tan, Q., Xie, C., et al. (2018). The transmission channel tower identification and landslide disaster monitoring based on INSAR, Intl. Arch. Photogrammetry Remote Sens. Spat. Info Sci. 42, 807–813. doi: 10.5194/isprs-archives-XLII-3-807-2018
Liu, L., Bouman, T., Perlaviciute, G., and Steg, L. (2019). Effects of trust and public participation on acceptability of renewable energy projects in the Netherlands and China. Energy Res. Soc. Sci. 53, 137–144. doi: 10.1016/j.erss.2019.03.006
Long, J., Giri, C., Primavera, J., and Trivedi, M. (2016). Damage and recovery assessment of the Philippines' mangroves following Super Typhoon Haiyan. Mar. Pollut. Bull. 109, 734–743. doi: 10.1016/j.marpolbul.2016.06.080
Longwei, L., and Dengsheng, L. (2016). Mapping population density distribution at multiple scales in Zhejiang Province using Landsat Thematic Mapper and census data. Int. J. Remote Sens. 37, 4243–4260. doi: 10.1080/01431161.2016.1212422
Maclean, I. M. D., Inger, R., Benson, D., Booth, C. G., Embling, C. B., Grecian, W. J., et al. (2014). Resolving issues with environmental impact assessment of marine renewable energy installations. Front. Mar. Sci. 75, 1–5. doi: 10.3389/fmars.2014.00075
Mann, M. L., Melass, E. K., and Malik, A. (2016). Using VIIRS Day/Night band to measure electricity supply reliability: preliminary results from Maharashtra, India. Remote Sens. 8, 711–723. doi: 10.3390/rs8090711
Martín-Martín, A., Orduna-Malea, E., Thelwall, M., and Delgado López-Cózar, E. (2018). Google Scholar, Web of Science, and Scopus: a systematic comparison of citations in 252 subject categories. J. Informetr. 12, 1160–1177. doi: 10.1016/j.joi.2018.09.002
McNeish, R., Rigg, K. K., Tran, Q., and Hodges, S. (2019). Community-based behavioral health interventions: developing strong community partnerships. Eval. Program Plann. 73, 111–115. doi: 10.1016/j.evalprogplan.2018.12.005
Merson, L., Phong, T. V., Nhan, L. N. T., Dung, N. T., Ngan, T. T. D., Kinh, V. N., et al. (2015). Trust, respect, and reciprocity: informing culturally appropriate data-sharing practice in Vietnam. J. Empirical Res. Hum. Res. Ethics 10, 251–263. doi: 10.1177/1556264615592387
Mojaddadi, H., Pradhan, B., Nampak, H., Ahmad, N., and bin Ghazali, A. H. (2017). Ensemble machine-learning-based geospatial approach for flood risk assessment using multi-sensor remote-sensing data and GIS. Geomat. Nat. Hazards Risk 8, 1080–1102. doi: 10.1080/19475705.2017.1294113
Natarajan, L., Rydin, Y., Lock, S. J., and Lee, M. (2018). Navigating the participatory processes of renewable energy infrastructure regulation: a ‘local participant perspective' on the NSIPs regime in England and Wales. Energy Policy 114, 201–210. doi: 10.1016/j.enpol.2017.12.006
National Academies of Sciences Engineering and Medicine. (2015). Continuity of NASA Earth Observations from Space: A Value Framework. Washington, DC: The National Academies Press.
National Academies of Sciences Engineering and Medicine. (2018). Thriving on Our Changing Planet: A Decadal Strategy for Earth Observation from Space. Washington, DC: The National Academies Press.
National Research Council (2003). Using Remote Sensing in State and Local Government: Information for Management and Decision Making. Washington, DC: NAP.
National Research Council (2007). Earth Science and Applications from Space: National Imperatives for the Next Decade and Beyond. Washington, DC: NAP.
Novellino, A., Jordan, C., Ager, G., Bateson, L., Fleming, C., and Confuorto, P. (2018). “Remote sensing for natural or man-made disasters and environmental changes” in Geological Disaster Monitoring Based on Sensor Networks, eds. T. Durrani, W. Wang, and S. Forbes (Singapore: Springer), 23–31. doi: 10.1007/978-981-13-0992-2_3
Oshri, B., Hu, A., Adelson, P., Chen, X., Dupas, P., Weinstein, J., et al. (2018). “Infrastructure Quality Assessment in Africa using Satellite Imagery and Deep Learning” in Proceedings of the 24th ACM SIGKDD International Conference on Knowledge Discovery and Data Mining (New York, NY: ACM), 616–625. doi: 10.1145/3219819.3219924
Parkins, J. R., Rollins, C., Anders, S., and Comeau, L. (2018). Predicting intention to adopt solar technology in Canada: The role of knowledge, public engagement, and visibility. Energy Policy 114, 114–122. doi: 10.1016/j.enpol.2017.11.050
Pellizzone, A., Allansdottir, A., De Franco, R., Muttoni, G., and Manzella, A. (2015). Exploring public engagement with geothermal energy in southern Italy: a case study. Energy Policy 85, 1–11. doi: 10.1016/j.enpol.2015.05.002
Quansah, D. A., Adaramola, M. S., and Mensah, L. D. (2016). Solar Photovoltaics in Sub-Saharan Africa – Addressing Barriers, Unlocking Potential. Energy Procedia 106, 97–110. doi: 10.1016/j.egypro.2016.12.108
Ramlal, B., Davis, D., and De Bellot, K. (2018). A rapid post-hurricane building damage assessment methodology using satellite imagery. West Indian J. Eng. 41, 74–83.
Rinaldo, R., Coppola, D., Walawalkar, R., and Thacker, H. (2017). Integrated Applications for Microgrids in Developing Economies: Webinar. Paris: ESA.
Robinson, C., Hohman, F., and Dilkina, B. (2017). “A deep learning approach for population estimation from satellite imagery” in Proceedings of the 1st ACM SIGSPATIAL Workshop on Geospatial Humanities (New York, NY: ACM), 47–54. doi: 10.1145/3149858.3149863
Román, M., Wang, R., Shrestha, R., Yao, T., and Kalb, V. (2019). Black Marble User Guide Version 1.0. Washington, DC: NASA.
Said, N., Pogorelov, K., Ahmad, K., Riegler, M., Ahmad, N., Ostroukhova, O., et al. (2018). “Deep learning approaches for flood classification and flood aftermath detection” in MediaEval 18 (Sophia Antipolis).
Schöpfel, J. (2010). “Towards a Prague Definition of Grey Literature” in Twelfth International Conference on Grey Literature: Transparency in Grey Literature (Prague: Národní technická knihovna).
Schwarz, B., Pestre, G., Tellman, B., Sullivan, J., Kuhn, C., Mahtta, R., et al. (2018). “Mapping floods and assessing flood vulnerability for disaster decision-making: a case study remote sensing application in senegal” in Advanced Remote Sensing Technology for Tsunami Modelling and Forecasting, eds P. P. Mathieu and C. Aubrecht (Boca Raton: CRC Press), 293–300. doi: 10.1007/978-3-319-65633-5_16
SIEPAC (2004). Geotechnical Study and Classification of Soils in the Line of Transmission. SIEPAC Final Review, Revision 01: Tomo V – Nicaragua. San José: SIEPAC.
Stevens, F. R., Gaughan, A. E., Linard, C., and Tatem, A. J. (2015). Disaggregating census data for population mapping using random forests with remotely-sensed and ancillary data. PLoS ONE 10:e0107042. doi: 10.1371/journal.pone.0107042
Stiles, G., and Murove, C. (2018). SADC Renewable Energy and Energy Efficiency Status Report. Paris: REN21.
Urmee, T., Harries, D., and Schlapfer, A. (2009). Issues related to rural electrification using renewable energy in developing countries of Asia and Pacific. Renew. Energy 34, 354–357. doi: 10.1016/j.renene.2008.05.004
Van Nguyen, M., Arason, S., Gissurarson, M., and Pálsson, P. G. (2015). Uses of Geothermal Energy in Food and Agriculture: Opportunities for Developing Countries. Rome: FAO.
Van Westen, C. J. (2000). Remote sensing for natural disaster management. Int. Arch. Photogrammet. Remote Sens. 33, 1609–1617.
Varshney, K. R., Chen, G. H., Abelson, B., Nowocin, K., Sakhrani, V., Xu, L., et al. (2015). Targeting villages for rural development using satellite image analysis. Big Data 3, 41–53. doi: 10.1089/big.2014.0061
Walker, G., Cass, N., Burningham, K., and Barnett, J. (2010). Renewable energy and sociotechnical change: imagined subjectivities of ‘the public' and their implications. Environ. Plann. A 42, 931–947. doi: 10.1068/a41400
Wan, C., Zhao, J., Song, Y., Xu, Z., Lin, J., et al. (2015). Photovoltaic and solar power forecasting for smart grid energy management. CSEE J. Power Energy Syst. 1, 38–46. doi: 10.17775/CSEEJPES.2015.00046
Wang, X., and Xie, H. (2018). A review on applications of remote sensing and Geographic Information Systems (GIS) in water resources and flood risk management. Water 10, 608–619. doi: 10.3390/w10050608
Washaya, P., Balz, T., and Mohamadi, B. (2018). Coherence change-detection with sentinel-1 for natural and anthropogenic disaster monitoring in urban areas. Remote Sens. 10:1026. doi: 10.3390/rs10071026
Wekesa, F., and Gichini, B. (2016). The Use of GIS in Geothermal Resource Management—A Case Study of Olkaria Geothermal Project. Nairobi: KenGen.
Young, O. R., and Onoda, M. (2017). “Chapter 1.4 Taxonomy of Roles,” in Satellite Earth Observations and Their Impact on Society and Policy, eds M. Onoda and O. Young (Singapore: Springer, 13–17.
Keywords: earth observation, rural electrification, renewable energy, electric grid, disaster risk
Citation: Leibrand A, Sadoff N, Maslak T and Thomas A (2019) Using Earth Observations to Help Developing Countries Improve Access to Reliable, Sustainable, and Modern Energy. Front. Environ. Sci. 7:123. doi: 10.3389/fenvs.2019.00123
Received: 02 May 2019; Accepted: 10 August 2019;
Published: 27 August 2019.
Edited by:
Ashutosh S. Limaye, National Aeronautics and Space Administration (NASA), United StatesReviewed by:
Laurence Delina, Boston University, United StatesCopyright © 2019 Leibrand, Sadoff, Maslak and Thomas. This is an open-access article distributed under the terms of the Creative Commons Attribution License (CC BY). The use, distribution or reproduction in other forums is permitted, provided the original author(s) and the copyright owner(s) are credited and that the original publication in this journal is cited, in accordance with accepted academic practice. No use, distribution or reproduction is permitted which does not comply with these terms.
*Correspondence: Natasha Sadoff, c2Fkb2ZmbkBiYXR0ZWxsZS5vcmc=
Disclaimer: All claims expressed in this article are solely those of the authors and do not necessarily represent those of their affiliated organizations, or those of the publisher, the editors and the reviewers. Any product that may be evaluated in this article or claim that may be made by its manufacturer is not guaranteed or endorsed by the publisher.
Research integrity at Frontiers
Learn more about the work of our research integrity team to safeguard the quality of each article we publish.