- 1Joint Center for Earth System Technology, University of Maryland Baltimore County, Baltimore, MD, United States
- 2Ocean Ecology Laboratory, NASA Goddard Space Flight Center, Greenbelt, MD, United States
- 3Department of Physics, University of Maryland Baltimore County, Baltimore, MD, United States
- 4Jet Propulsion Laboratory, California Institute of Technology, Pasadena, CA, United States
- 5NASA Goddard Institute for Space Studies, Columbia University, New York, NY, United States
- 6Earth Science Group, SRON Netherlands Institute for Space Research, Utrecht, Netherlands
- 7Laboratoire d'Optique Atmosphérique, Université de Lille, Villeneuve d'Ascq, France
- 8NASA Goddard Space Flight Center, Science Application International Corp., Greenbelt, MD, United States
- 9School of Marine Sciences, University of Maine, Orono, ME, United States
- 10NASA Goddard Institute for Space Studies, New York, NY, United States
- 11Laboratory for Atmospheric and Space Physics, University of Colorado, Boulder, CO, United States
- 12Departments of Marine Science and Geography, University of Connecticut, Groton, CT, United States
- 13Scripps Institution of Oceanography, University of California, San Diego, La Jolla, CA, United States
- 14Naval Research Laboratory, Washington, DC, United States
- 15Science Systems and Applications, Inc., Lanham, MD, United States
- 16Laboratory for Climate and Radiation, NASA Goddard Space Flight Center, Greenbelt, MD, United States
- 17Chemistry and Dynamics Branch, NASA Langley Research Center, Hampton, VA, United States
The Plankton, Aerosol, Clouds, ocean Ecosystem (PACE) mission presents new opportunities and new challenges in applying observations of two complementary multi-angle polarimeters for the space-based retrieval of global aerosol properties. Aerosol remote sensing from multi-angle radiometric-only observations enables aerosol characterization to a greater degree than single-view radiometers, as demonstrated by nearly two decades of heritage instruments. Adding polarimetry to the multi-angle observations allows for the retrieval of aerosol optical depth, Angstrom exponent, parameters of size distribution, measures of aerosol absorption, complex refractive index and degree of non-sphericity of the particles, as demonstrated by two independent retrieval algorithms applied to the heritage POLarization and Directionality of the Earth's Reflectance (POLDER) instrument. The reason why this detailed particle characterization is possible is because a multi-angle polarimeter measurement contains twice the number of Degrees of Freedom of Signal (DFS) compared to an observation from a single-view radiometer. The challenges of making use of this information content involve separating surface signal from atmospheric signal, especially when the surface is optically complex and especially in the ultraviolet portion of the spectrum where we show the necessity of polarization in making that separation. The path forward is likely to involve joint retrievals that will simultaneously retrieve aerosol and surface properties, although advances will be required in radiative transfer modeling and in representing optically complex constituents in those models. Another challenge is in having the processing capability that can keep pace with the output of these instruments in an operational environment. Yet, preliminary algorithms applied to airborne multi-angle polarimeter observations offer encouraging results that demonstrate the advantages of these instruments to retrieve aerosol layer height, particle single scattering albedo, size distribution and spectral optical depth, and also show the necessity of polarization measurements, not just multi-angle radiometric measurements, to achieve these results.
Introduction
NASA's Plankton, Aerosols, Clouds, ocean Ecosystems (PACE) mission offers unique and new opportunities for characterizing aerosol from space. Part of the challenge of PACE will be to advance and combine our current infrastructure and algorithms to take advantage of PACE's new and unique observations from a combination of synergistic satellite instruments.
PACE will carry three instruments. The primary instrument is the Ocean Color Instrument (OCI). This is a radiometer that spans the spectral range, hyperspectrally, from near 350 to 885 nm, measuring at 5 nm spectral resolution. OCI also includes seven discrete bands in the shortwave infrared (SWIR) centered at 940, 1,038, 1,250, 1,378, 1,615, 2,130, and 2,260 nm. In addition to OCI, PACE is expected to carry two multi-wavelength, multi-angle imaging polarimeters (MAPs). One of these is Spectro-Polarimeter for Planetary Exploration-1 (SPEXone: Hasekamp et al., 2019), an instrument contributed by the Netherlands. The other is Hyper Angle Rainbow Polarimeter-2 (HARP2), an instrument contributed by the University of Maryland Baltimore County (UMBC). SPEXone uses the spectral modulation technique (Snik et al., 2009) to achieve a highly accurate hyperspectral polarization from 385 to 770 nm. Spectral resolution for scalar radiometric intensity is 5 nm in the continuum and 2 nm in the Oxygen-A band, and for polarization 15–45 nm (polarimetric spectral resolution increases with wavelength). SPEXone's swath width is 100 km, with 5 × 5 km spatial resolution sampled at 2.5 × 2.5 km pixel resolution, and it views each Earth target at five different angles. HARP2 uses a Philips prism beam splitting technique with stripe filters to achieve accurate polarization in four discrete wavelengths (440, 550, 670, and 870 nm), but across a broad swath of 1,550 km. HARP2 is hyperangular, viewing each nominally 2.5 km pixel at 10–60 different angles. Thus, the two polarimeters together offer synergistic opportunities, compensating for wide vs. narrow swaths, and hyperangle vs. hyperspectral capabilities. Table 1 shows the characteristics of each MAP, including the differences in polarimetric accuracy.
In this paper, we present an overview of the opportunities and challenges of using a multi-angle polarimeter (MAP) for aerosol characterization in a PACE context. We will briefly review our heritage operational space-based multi-angle radiometers and polarimeters, and how they have contributed to our current understanding of aerosol characterization. We will present an analysis of information content for aerosol characterization from a MAP, as compared to a radiometer like OCI, and then discuss specific issues of making a MAP retrieval of aerosol. Throughout this paper we will refer to a generic MAP that is neither SPEXone nor HARP2 but can be applied to either sensor or to others that might participate in other missions. Because the emphasis of PACE, as a mission, has been focused on ocean biology, we will address the specific challenges of retrieving aerosol characteristics over oceans, although observations from the PACE MAPs will be used to characterize aerosol over land, as well. Despite the focus in the over ocean retrievals of aerosols here, this paper does not directly address atmospheric correction. Instead, Frouin et al. (2019) explores atmospheric correction or the retrieval of normalized water-leaving radiance in the PACE era. The at-launch aerosol retrieval algorithms to be applied to the individual PACE MAPs have not been formulated in detail at this time, and therefore, this paper cannot provide specifics on the eventual operational algorithms or exact final PACE aerosol products.
One of the challenges of PACE will be the attempt to extract additional information from the ultraviolet (UV) wavelengths. The use of UV observations by the OCI radiometer for aerosol retrievals is addressed in this issue in a separate paper (Remer et al., 2019), but here we present a summary of the results of a theoretical sensitivity study (Zhai et al., 2017b) that highlights the importance of polarimetry to constrain the uncertainty introduced by the UV (Kahn et al., 2016). Note that we discuss these results from the perspective of aerosol characterization. Frouin et al. (2019) addresses the challenges of the UV specifically for atmospheric correction, whereas Chowdhary et al. (2019) touches upon ocean color contributions to spaceborne UV observations.
The inclusion of MAPs in the mission has elevated PACE capabilities for global aerosol characterization to new levels. While the focus of this paper is aerosol characterization, a MAP, with its enhanced information content, provides the opportunity for simultaneous retrievals of aerosols and surface properties. Success of a simultaneous retrieval depends on the availability of radiative transfer models that are sufficiently accurate to make use of the full information content measured by a MAP. Such radiative transfer models need to couple ocean and atmosphere and need to represent all processes and constituents appropriately. An extensive exploration of the current state of ocean and atmospheric radiative transfer modeling is presented in Chowdhary et al. (2019) and will only be briefly discussed here in the context of aerosol characterization.
Heritage Multi-Angle and Polarimeter Aerosol Characterization
Cloud Masking and Other Scene Selection Processes
Heritage quantitative aerosol remote sensing algorithms, especially those applied to single-view radiometers like the MODerate resolution Imaging Spectroradiometer (MODIS) and the Ozone Monitoring Instrument (OMI), needed to constrain their retrievals by making assumptions regarding the scene observed by the instrument. A scene with clouds or certain surface types such as snow/ice that does not conform to algorithm assumptions will be identified and then excluded from retrieval. Included in that screening for single-view radiometers would be sun glint over water. Recent algorithms for these single-view heritage instruments have begun to retrieve aerosol above clouds (Waquet et al., 2009b; Torres et al., 2012; Jethva et al., 2013, 2014, 2016; Meyer and Platnick, 2015; Sayer et al., 2016), but these algorithms still require identification of the cloud scene in order to choose the correct algorithmic path. Single-view polarimetry will not add much value for cloud identification, as polarimetry is not sensitive to water droplet clouds, except by accident when the single-view coincides with the rainbow peak.
The situation has been less restrictive for heritage multiangle radiometers and polarimeters. Multiangular satellite observations, such as from Multi-angle Imaging SpectroRadiometer (MISR) instrument (Diner et al., 1998) provide additional information for scene selection that cannot be discerned from single-view radiometers. For example, MISR uses multi-angular smoothness and spatial correlation tests to assist in cloud screening as part of the aerosol retrieval algorithm (Martonchik et al., 2002). Multi-angle instruments also have the advantage that some of the view angles always avoid glint so that every scene can be viewed at some non-glint geometry (see e.g., Harmel and Chami, 2013). An example using MISR to fill in glint-induced gaps in MODIS-Terra aerosol optical depth (AOD) product over the ocean is shown in Figure 1. This increases the availability of retrieved aerosol characteristics over oceans. The information contained in multi-angle polarimetry has also been shown to be sufficient to perform masking by simply assessing the goodness of fit in the retrieval process, thereby avoiding deterministic thresholds inherent to single-view techniques, which may inadvertently over- or under-screen the data (Stap et al., 2015). The PACE MAPs have the capabilities to make use of new techniques that avoid masking, and to have the capabilities to mask when needed. Advanced algorithms developed for these instruments should address scene selection with the same rigor as they apply to characterizing the aerosol once scene selection has been accomplished.
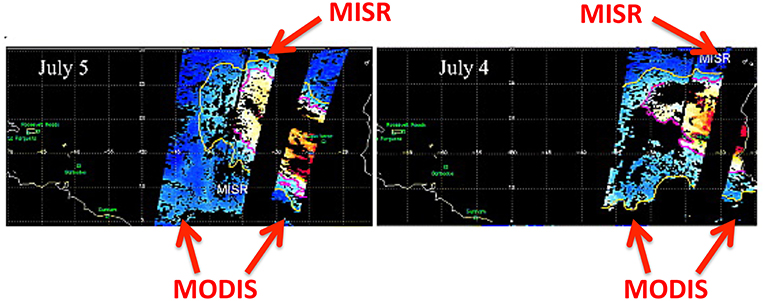
Figure 1. Complementary MISR and MODIS coverage over dust plumes transported over the Atlantic. The narrower MISR swath partly fills in areas that would otherwise be lost to sun glint masking in the broader MODIS swath. MODIS AOD data (right and left of the MISR swath) is plotted together with the narrower MISR swath for two successive days in this dust plume event. AOD data in the middle of the MODIS swath is missing because of glint masking. MISR can retrieve AOD despite glint because only 2–3 out of nine MISR cameras view the scene in glint while other cameras point at the same scene, but at non-glint angles. These can be used for retrievals. Adapted with permission from Kalashnikova and Kahn (2008), John Wiley & Sons.
A comprehensive study of cloud detection by PACE that includes discussion of both OCI and MAP capabilities and limitations can be found in Platnick et al. (2018).
Heritage Aerosol Retrievals From Multi-Spectral Multi-Angular Radiance Measurements
The concept of using satellite multi-angle measurements to derive aerosol properties over land and ocean has a long history (Martonchik and Diner, 1992; Wang and Gordon, 1994). The first satellite sensor providing opportunity to test the concept was the dual angle Along Track Scanning Radiometer 2 (ATSR-2) that surveyed each pixel twice, once in the forward direction at 52–55° view angle and then near nadir at 0–22° view angle (Flowerdew and Haigh, 1995). Aerosol retrievals were devised to make use of the dual-look information to separate aerosol signal from surface reflectance and bi-reflection directional function (BRDF) over land (Flowerdew and Haigh, 1996). Soon after an algorithm making use of both multi-angle and spectral information was applied to ATSR-2 data and validated (Veefkind et al., 1998). Since then a series of algorithms have been applied to the multi-angle observations of ATSR-2 and its successors (e.g., Holzer-Popp et al., 2002; Kokhanovsky et al., 2009; Kolmonen et al., 2016; de Leeuw et al., 2018).
While the European multi-angle aerosol retrieval effort has focused on ATSR-2 and its successors, NASA's primary heritage multi-angle instrument is MISR. MISR has been on orbit, observing Earth and retrieving aerosol characteristics since soon after Terra launch in 2000. Besides retrieving AOD, MISR's multi-angle capability has been used for additional aerosol characterization. Previous MISR studies demonstrate the ability of multi-angular radiances to distinguish dust from other airborne particles (e.g., Kalashnikova and Kahn, 2006; Kalashnikova et al., 2013) over the ocean surfaces, and show the value of such observations for separating aerosol and surface scattering over non-black ocean waters (where the red and near infrared radiance emanating from the ocean is not zero, Limbacher and Kahn, 2017). As a demonstration of multi-angular capabilities, Limbacher and Kahn (2017) introduced a coupled, self-consistent retrieval of aerosols over open oceans with Chlorophyll-a concentration [Chla] < 1.5 mg/m3, with a primary goal to correct MISR ocean retrievals of AOD at low aerosol loadings. Because MISR-based ocean reflectance is derived self-consistently with aerosol amount and type in a physical retrieval, it can potentially provide a more robust atmospheric correction for collocated MODIS-Terra ocean retrievals.
Currently, the only plans to continue multi-angle radiometry without polarization after MISR are with the European Sea and Land Surface Temperature Radiometer (SLSTR) instrument, a follow-on of the Advanced Along Track Scanning Radiometer (AATSR), on the European Space Agency (ESA) Sentinel-3 mission, which was launched in 2016. These instruments measure the same scene at two view angles (Grey et al., 2006), which is less advantageous than a 9-camera MISR-like observation. Future multi-angle sensors will combine multi-angle capability with polarimetry. However, there are paths forward for multi-angle radiometry beyond MISR and AATSR that would provide additional capability for aerosol characterization that do not require polarimetry. One such configuration would be for a multi-angle instrument with hyperspectral capabilities. Such a configuration could resolve the Oxygen A-band signal through different slant paths from multi-angle views and enhance the ability to derive the heights of cloud (Ferlay et al., 2010) and aerosol (Davis and Kalashnikova, 2019) layers. There are no current plans for such an instrument featuring radiometry alone. Instead, all multi-angle instruments in the queue are combined with polarimetric capability, although SPEXone on PACE will combine polarimetry with multi-angle hyperspectral views out to 770 nm, and may provide the information described by Davis and Kalashnikova (2019) on coarser resolution. Currently, SPEXone, HARP2, NASA's Multi-Angle Imager for Aerosols (MAIA), and the European Multi-viewing, Multi-channel, Multi-polarization Imager (3MI) are the only planned instruments with multi-angle capability that could continue the NASA MISR aerosol record. These are all multi-angle polarimeters (MAPs) that exceed MISR's capabilities by adding information for aerosol characterization associated with polarimetric measurements and enhanced spectral coverage, though MAIA will not provide continuous, near-global coverage, and instead will observe a globally distributed set of discrete target areas (Diner et al., 2018).
Heritage Multi-Angular and Polarimetric Observations
The POLarization and Directionality of the Earth's Reflectance (POLDER) (Deschamps et al., 1994) instrument, was a MAP that enjoyed three deployments into space. The first two were relatively short due to catastrophic failures in its spacecraft (Advanced Earth Observation Satellite—ADEOS), not in the sensor itself. The third POLDER, aboard the Polarization and Anisotropy of Reflectances for Atmospheric Sciences coupled with Observations from a Lidar space craft (PARASOL) (Tanré et al., 2011), produced a 9-year data record. POLDER could potentially retrieve AOD and ocean reflectance simultaneously, and, to some extent, could characterize absorbing aerosol amount. POLDER's first-generation aerosol retrieval was the very first attempt to use polarization measurements. The standard aerosol algorithm was originally very conservative in its output, limiting itself to producing only MODIS-like aerosol products (AOD and size) and using simplified radiative transfer assumptions (Deuzé et al., 2000).
In addition to the original operational stream, a more comprehensive, although computationally expensive, retrieval algorithm (Dubovik et al., 2011, 2014) has been applied to POLDER measurements, This is the Generalized Retrieval for Aerosol and Surface Properties (GRASP) that produces an expanded product line that includes additional quantitative aerosol properties (size, complex refractive index, fraction of non-sphericity, and scale height). This algorithm uses a multi-pixel approach that assumes limited temporal variability of surface reflectance (over land) or limited spatial variability of aerosol characteristics (over both land and ocean). Progress has been made in speeding up processing, and at present, the whole archive of 9 years (2004–2013) POLDER-3/PARASOL data and 18 months of POLDER-1,2/ADEOS-1,2 have been processed (Dubovik et al., 2019). Figure 2 illustrates the retrieval results for single scattering albedo. These results are preliminary in that complex situations have not yet been properly addressed, and thus, snow/ice, heterogeneous scenes, and clouds are likely introducing errors in the plotted values. Even so, the GRASP/POLDER products are showing, over all expected global patterns, good agreement with the Aerosol Robotics Network (AERONET) inversions and have recently been used for the quantification of emissions of biomass burning and mineral dust aerosols over Africa (Chen et al., 2018).
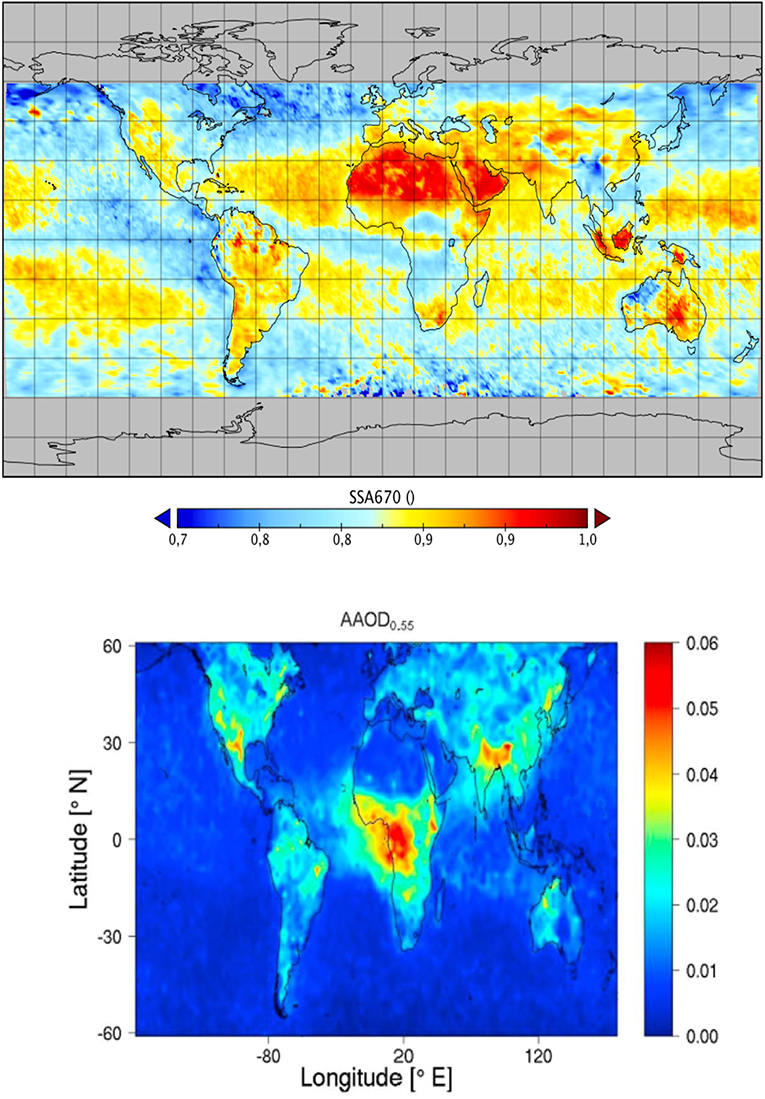
Figure 2. Annual mean aerosol absorption properties for 2006 derived from PODER-3/PARASOL observations using the GRASP algorithm reporting single scattering albedo (SSA) at 670 nm (Top) and using the SRON retrieval algorithm (Hasekamp et al., 2011; Fu and Hasekamp, 2018) reporting absorbing aerosol optical depth (AAOD) at 550 nm (Bottom). Adapted with permission from Lacagnina et al. (2017), John Wiley & Sons. Note that SSA is an intrinsic aerosol property while AAOD is dependent also on the aerosol loading. Direct comparisons of the two maps are not possible. However, very high AAOD should roughly correspond to low SSA, and vice-versa. Also, please note that the GRASP results are still preliminary and may be affected by cloud, snow/ice, surface heterogeneity, and other contamination issues.
A separate POLDER algorithm development effort is also underway at SRON, the Netherlands Institute for Space Research (Hasekamp et al., 2011; Fu and Hasekamp, 2018), which like GRASP is computationally expensive but more fully exploits the information content inherent in a POLDER observation than did the first generation algorithm (Tanré et al., 2011). For example, the SRON algorithm produces aerosol size, single scattering albedo (SSA) or absorbing AOD (AAOD), complex refractive index, and degree of particle non-sphericity. An example of the algorithm to characterize global aerosol particle absorption (AAOD) is shown in Figure 2 (Lacagnina et al., 2017). Both the GRASP and SRON retrievals have been validated against direct sun measurements and compared with inverted sky radiances from AERONET, and both report a significant ability to quantify column aerosol particle properties. The advances represented by GRASP and SRON to more fully exploit the multi-angle and polarimetric capabilities of POLDER and increase its aerosol characterization ability are already offering new insight in a variety of long-standing global aerosol questions. These include quantifying of emissions of biomass burning and mineral dust aerosols over Africa (Chen et al., 2018), evaluating global model aerosol absorption (Lacagnina et al., 2015), computing the direct radiative effect of aerosols (Lacagnina et al., 2017), and aerosol typing (Russell et al., 2014).
Multi-Angular Polarimetric Information Content for Aerosol Retrieval Over Ocean
There is strong consensus within the aerosol community that a multi-angle polarimeter is part of the essential next step in improving our understanding of the global aerosol system, reducing uncertainty in estimates of aerosol forcing of climate, improving knowledge of aerosol-cloud processes, advancing space-based air quality monitoring and forecasting, and documenting important aerosol events. The POLDER missions provided the first taste of multi-angle polarimetry. As technology advances to include hyperspectral capability, possibly broader wavelength ranges and higher polarimetric accuracy, the expectation is that aerosol characterization will also advance. As noted above, multi-angle radiometry is an important step up from the single-look instruments, and polarimetry allows even better characterization of particle properties from space.
Tanré et al. (1996) quantified the information content or degrees of freedom inherent in a 6-wavelength, single look, unpolarized satellite observation of aerosol over Case 1 ocean waters using principal component analysis. Their conclusion is that these six wavelengths resulted in only 2–3 degrees of freedom, expressed as the aerosol loading, the weighting between modes and if one mode was dominant, then also the effective radius of the dominant mode. The six wavelengths spanned the MODIS spectrum (0.55–2.13 μm). OCI, extending to hyperspectral and into the UV, should add information content to the MODIS-like measurements, and the possibility of using this information to retrieve absorption and aerosol layer height was explored in a companion paper (Remer et al., 2019). However, the most significant increase in information content (often expressed as Degrees of Freedom for Signal, DFS) can be made with multi-angle polarimetry, thus offering the potential for more complete, and accurate, aerosol characterization (Hasekamp and Landgraf, 2005; Hasekamp, 2010; Knobelspiesse et al., 2012).
Figure 3 shows the results of a study that compares the information contained in an observation by an example multi-angle polarimeter, the European 3MI instrument (Manolis et al., 2017; Fougnie et al., 2018) to that of OCI. For this study, we assumed 3MI has a polarimetric accuracy (expressed in terms of Degree of Linear Polarization—DoLP) of 1%. This study was performed for a scene parameterized from the “maritime” aerosol model in Smirnov et al. (2003) and a simple Case-I ocean reflectance model based on Chlorophyll-a and wind speed, as described in Table 2. Note that the (isotropic) wind speed parameterizes both the ocean surface reflected sunglint (Cox and Munk, 1954), and the spectra and quantity of sea foam (Koepke, 1984; Frouin et al., 1996). It follows the Bayesian information content methods described in Rodgers (2000) and implemented for aerosol and ocean scenes as in Knobelspiesse et al. (2012). This type of analysis can also be used to determine the expected measurement uncertainty of other parameters not included as free parameters (italicized in Table 2), so long as they uniquely express the derived parameter. We used this property to derive Single Scattering Albedo for the combined aerosol state, which is a function determined largely by the imaginary refractive index, but also depends on other properties (real refractive index, size distribution and fine mode fraction). See section 2.1 in Knobelspiesse et al. (2012) for more details.
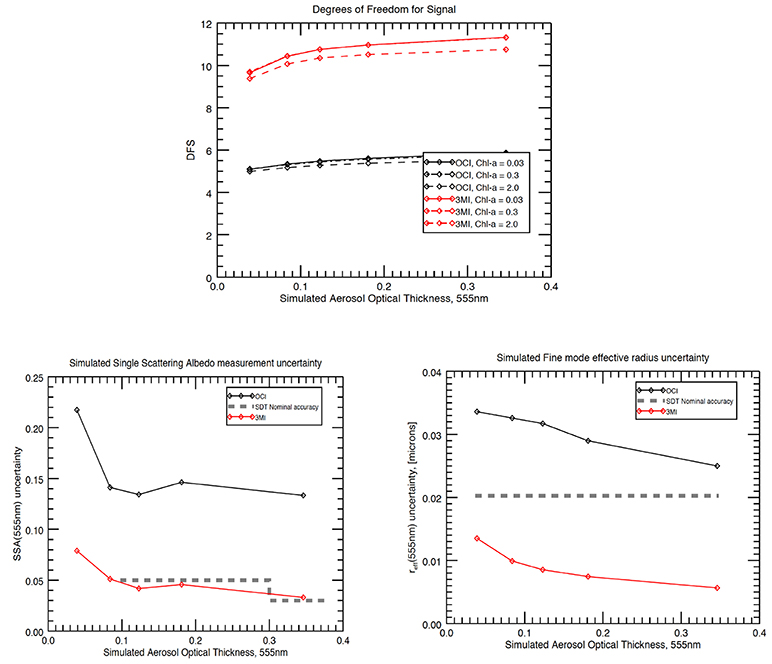
Figure 3. (Top) Calculated degrees of freedom (information content) from OCI (black) and a multi-angle polarimeter, the European 3MI instrument (red), as function of calculated Aerosol Optical Thickness (AOT). The different lines represent different chlorophyll concentrations in the simulated ocean. Note that 3MI is used as a generic example of a MAP, and is not expected to fly on PACE. (Bottom) Calculated uncertainties in simultaneous retrievals of single scattering albedo (bottom left) and fine mode effective radius (bottom right) for the two instruments, following the same color scheme. The dashed line indicates the uncertainty requirements of these parameters, defined by the PACE Science Definition Team report (NASA, 2012).
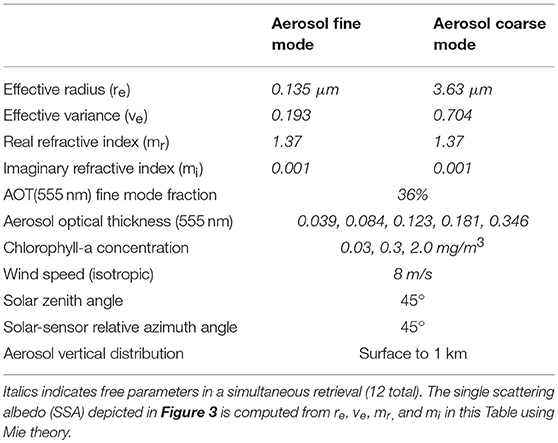
Table 2. Scene parameterization for results in Figure 3.
The top plot in Figure 3 shows the DFS for each instrument for aerosol retrieval over ocean, given 12 free parameters describing the aerosol and ocean state (as in Table 2; the maximum DFS value is 12). Since the aerosol loading in a scene determines how well microphysical properties can be retrieved, this analysis has been repeated for five different AOT's, represented as the abscissa in each plot. This analysis is an expression of measurement uncertainty in terms of desired retrieval parameters and is therefore independent of the retrieval algorithm method. Like any simulation, this represents a parameterization that may not span the entire range of geophysical reality. However, these simplifications are consistent for all tested measurement systems, so this is a powerful means to compare in a relative sense, and to some extent covers differences for varying geometries. Furthermore, Knobelspiesse et al. (2012) has shown that calculated uncertainties are insensitive to the simulated value of aerosol intensive properties (size distribution and complex refractive index) so this analysis is representative of most scenes, as long as the extensive properties (AOT, Chl-a) are assessed at various values. This is why we chose to represent our results in terms of varying AOT, and for different magnitudes of [Chl-a]. These results show that the 3MI DFS is much higher than that of OCI alone. This means that 3MI can independently determine most of the aerosol and ocean parameters in the simulation, while OCI would need to “add” information via the use of assumptions of particle properties based on empirical information. The ability to determine SSA (bottom left) and fine size mode effective radius (bottom right) are shown as predicted uncertainties. The gray dashed lines indicate the MAP uncertainty requirements for these parameters from the PACE Science Definition Team report (NASA, 2012). Thus, 3MI with an assumed polarimetric accuracy of 1% would meet those requirements for simple scenes such as this, while OCI alone would not be able to do so. The PACE MAPs are expected to have even better polarimetric accuracy and should perform as well, or better, even in more complex situations than the simple case presented in Table 2 and shown in Figure 3.
Challenges in Exploiting MAP Information Content Over Ocean
Full exploitation of MAP information content faces several challenges. The relative newness of such algorithms, and the complexity of simultaneous retrieval of multiple parameters, is a barrier to full operational exploitation. This complexity can lead to computationally expensive algorithms that are difficult to implement on a global scale. Radiative transfer calculations at the heart of retrieval algorithms must be confirmed as being appropriate for the measurement uncertainty of a MAP instrument, and these measurement uncertainty models need to be developed, validated, and incorporated into a retrieval. Furthermore, validation of aerosol optical properties retrieved from these algorithms can be challenging for some parameters. Fortunately, the PACE mission can benefit from the history of such development for POLDER, the Aerosol Cloud Ecosystems (ACE) mission study, and the variety of MAP prototypes that have been deployed on aircraft for research field campaigns, and other instruments (Dubovik et al., 2019).
The difficulty of aerosol retrieval algorithm development is illustrated with the data processing experience of the POLDER mission, which in the original operational algorithm over the ocean reported a limited parameter set (Aerosol Optical Thickness and the Ångström exponent) and relied on only two of the three polarimetric channels (670 and 865 nm) (Deuzé et al., 1999, 2000). Many algorithms have been developed since that time, and some of them (such as GRASP) have specifically tackled the issue of computational expense. A complete review of such algorithms can be found in Kokhanovsky et al. (2015), and descriptions of more recent algorithms include those by Gao et al. (2018) and Stamnes et al. (2018). In addition to modern advancements of computational power since the era of original POLDER algorithms, some have investigated the exploitation of alternate methods to converge to a retrieval solution efficiently, such as utilizing fast Neural Networks (Di Noia et al., 2017). In some ways, the algorithms required for proper exploitation of MAP information content may require different paradigms than those that were appropriate for the era of single view angle multi-spectral instruments.
Measurement uncertainty, and the estimated uncertainty of retrieved aerosol products, is of particular importance to MAPs. This is because the retrieval algorithms mentioned above must weigh the relative importance of the hundreds or thousands of individual observations (at various geometries, spectral channels, and polarimetric states) that comprise a “measurement” of a scene. Polarimetric observations of DoLP are several factors more accurate than the corresponding radiometric observations and have an entirely different relationship with regards to systematic and random errors. The relative importance of these errors and the correlations between errors of different observations can have an impact on the uncertainty of the retrieved geophysical products (Knobelspiesse et al., 2012). Measurement uncertainty has been characterized for some airborne polarimetric instruments (Diner et al., 2010; Harten et al., 2018; Stamnes et al., 2018), but coordinated validation of these uncertainty models is difficult and limited (Knobelspiesse et al., 2019).
Validation of aerosol products beyond Aerosol Optical Thickness and Ångström exponent also presents a challenge. While AERONET (Holben et al., 1998) represents the standard to which most aerosol satellite products over land are compared, and its extension, the Maritime Aerosol Network (MAN, Smirnov et al., 2009), can be used over the oceans, both are limited in the validation capability for other aerosol optical properties. AERONET does not measure aerosol optical properties directly, and AERONET aerosol optical property retrieval quality is highest for AOD > 0.4 (Dubovik et al., 2000); MAN instruments do not have sky scanning capabilities, and cannot be used for aerosol retrievals. While other measurements besides these networks do exist, they are limited by the same fundamental information constraints. In situ observations, on the other hand, must contend with the difficulties of accounting for temporal and spatial scale, and have their own measurement uncertainties. Aerosol chemical properties from commonly used in-situ sensors are measured with 15–30% accuracy, for example, size-resolved aerosol black carbon (BC) mass from Soot Photometer (SP2) has 30% relative uncertainty, while bulk aerosol chemical composition from Aerosol Mass Spectrometer (AMS) has 17–19% relative uncertainty (Ryerson et al., 2013; Kalashnikova et al., 2018).
The Coupled Atmospheric-Oceanic System
Retrieving aerosol properties over ocean requires separating atmosphere and ocean signals. This is a significant challenge given that the atmospheric and ocean systems are coupled physically, chemically, biologically—and most pressing for PACE, radiatively. The radiative coupling of the atmosphere and ocean, and the consequences for PACE remote sensing is explored in depth in Chowdhary et al. (2019). Here, we briefly discuss approaches that are used to manage this coupling in the retrieval of aerosol characteristics from MAP observations.
One approach to isolate the atmospheric signal for aerosol characterization is to focus on observations that are minimally affected by ocean scattering contributions. This can be done spectrally, by focusing on the near infrared (NIR) part of the spectrum where absorption by pure sea water becomes very large (Smith and Baker, 1981), and geographically by focusing on Case 1 (open ocean) waters where scattering by the ocean in the NIR part becomes negligible (Morel and Maritorena, 2001). The former choice further avoids scattering contributions by phytoplankton and their by-products that vary with biomass and can become large in the visible (VIS)/UV (see again Morel and Maritorena, 2001), while the latter choice avoids complex ocean scenes, such as coasts and estuaries, where scattering contributions vary e.g., suspended sediments and can become large even in the NIR (Li et al., 2003). These choices have been necessary in heritage algorithms for inversions of spaceborne single-view radiance observations to either retrieve aerosols properties (Tanré et al., 1997), or to simply account for aerosol scattering in atmospheric correction (Gordon, 1997). However, as we move into the PACE era, the expectation is to expand aerosol property inversions spectrally into the shorter wavelengths (UV, blue and green) and geographically into the near-shore environment. In addition, for ocean color retrievals from PACE there will be a need to expand atmospheric correction spectrally into the UV. This will require a new way of approaching the atmospheric-ocean system.
The process for more sophisticated aerosol retrieval over ocean is to make use of a MAP's large information content to retrieve aerosol and ocean properties simultaneously. Joint retrieval of aerosol and hydrosol properties requires a sophisticated and computationally efficient inverse model to disentangle their contributions to top-of-atmosphere (TOA) radiometry and polarimetry. Currently, several approaches are developed for the open ocean applications. For example, Chowdhary et al. (2002, 2012) used a simple, 1-parameter bio-optical model (i.e., varying only with [Chla]) for Case 1 waters to characterize water reflectance and retrieved aerosol properties using least squares fitting of the Research Scanning Polarimeter (RSP: an airborne multi-angle polarimeter: Cairns et al., 1999) data. Xu et al. (2016) developed an optimization approach for joint aerosol and water-leaving radiance retrieval with the constraints of multi-pixel measurements and a simplified bio-optical model for open ocean, and tested the retrieval with data from the Airborne Multiangle SpectroPolarimetric Imager (AirMSPI: see Diner et al., 2013). Hasekamp et al. (2011) have developed aerosol retrieval algorithms over ocean using PARASOL data for a radiatively coupled atmosphere-ocean system using PARASOL data, also with the simple case 1 water bio-optical model of Chowdhary et al. (2012).
The problem with using a simple 1-parameter bio-optical model for case 1 waters is that it is inappropriate for coastal and inland waters, or even case 1 waters that vary from the ideal (e.g., Mobley et al., 2004). When the inherent optical properties (IOP) do not tightly co-vary with [Chla], more sophisticated bio-optical models (i.e., that include more free parameters such as marine particulate scattering and Colored Dissolved Organic Material -CDOM), absorption coefficients; Werdell et al., 2013) are needed to simultaneously retrieve aerosol and ocean properties. Stamnes et al. (2018) and Gao et al. (2018) have taken the first steps toward including more free parameters in the joint retrieval of aerosol and hydrosol properties from MAP data. However, additional research is needed to quantify polarimetric properties of plankton and suspended mineral particles for such waters, such that these more sophisticated bio-optical models can take fullest advantage of MAP data for joint aerosol-ocean retrievals over complex (coastal) waters.
Aerosol retrieval algorithms that use only the polarized reflectance measured by a MAP in the VIS offer an alternative, albeit partial solution, for open ocean observations. They rely less on the goodness of bio-optical models but are also less sensitive to some aerosol properties, such as SSA and AOD. These types of algorithms are based on our understanding of the absolute and relative magnitudes of intensity and polarized contributions to the TOA radiance, especially in parsing the contributions from ocean and atmosphere. We have acquired this information from various sources. Chowdhary et al. (2006) have studied the magnitude and angular variation of the total and polarized water-leaving reflectances, based on bio-optical models of case 1 waters, in which inherent optical properties (IOPs) are parameterized in terms of [Chla]. The wavelength range was from 400 to 600 nm and for [Chla] spanning 0.03–3.0 mg/m3. Chami (2007) has studied the importance of the top-of-atmosphere polarized reflectance for both open ocean and coastal waters. The wavelength range was from 443 to 870 nm. Chami (2007) suggested that the polarized signal may be preferred for aerosol retrieval because the top-of-atmosphere polarized signal is insensitive to variations in open ocean water properties. This has been verified by PARASOL measurements (Harmel and Chami, 2008). However, these conclusions do not hold for modern-age polarimetric observations with higher accuracy than PARASOL (Mishchenko et al., 2007) in the short-wave visible part of the spectrum (Chowdhary et al., 2012) or for waters dominated by algal blooms or sediments (Chami, 2007; Loisel et al., 2008). Because extending this type of analysis spectrally and for more complex waters will be necessary to make full use of PACE capabilities, we summarize a specific study of this type in section Characterizing Signal in the UV and Over Complex Waters.
Meanwhile, we note that a joint retrieval, that includes total reflectance measurements contains its own challenges for retrieving accurate ocean parameters (including normalized ocean leaving radiance) because an overwhelming portion of the signal measured by the MAP originates in the atmosphere not from the ocean. Small errors in forward modeling will translate into a large, errors in retrieved ocean parameters. These small errors could be introduced from cloud contamination, trace gas, or adjacency effects, or from inaccurate assumptions of aerosol optical properties, atmospheric profiles, horizontal homogeneity or ocean salinity. Even basic radiative transfer assumptions, such as plane parallel assumptions, truncating scattering diffraction peaks, using limited number of streams and Fourier terms, ignoring inelastic scattering processes, or using inaccurate solar spectra can affect the robustness of the joint retrieval, especially the retrieval of the ocean parameters.
Although the retrieved aerosol parameters are less susceptible to these small errors than the ocean parameters, these errors in the forward model can also affect the aerosol retrieval accuracy or the number of aerosol parameters that can be retrieved with confidence. Case I waters will always be easier than complex waters, and the stronger the aerosol loading, the more reliable the aerosol retrieval. At low aerosol loading over coastal regions, even a MAP may not return much more additional aerosol information beyond what we have come to expect from heritage instruments. However, we expect progress over the next few years that will address these sources of error in forward models and provide more sophisticated bio-optical models and better representation of polarimetric properties of hydrosols. Thus, in the PACE era, we expect to see attempts of simultaneous retrievals of aerosol and ocean properties, and better realization of the information content measured by the PACE MAPs.
Characterizing Signal in the UV and Over Complex Waters
The PACE MAPs will cover the spectrum from 385 to 870 nm, which will surpass the wavelength range studied in the above-mentioned literature (Chowdhary et al., 2006; Chami, 2007; Harmel and Chami, 2008). In order to gain a better understanding of the water-leaving contribution to the TOA radiances in the UV and the deep blue part of the spectrum, Zhai et al. (2017b) performed a sensitivity study for a set of wavelengths from 340 to 865 nm. This study uses a vector radiative transfer model for coupled atmosphere and ocean systems based on the successive order of scattering method (Zhai et al., 2009, 2010). All inelastic scattering processes in ocean waters are accounted for in this study, which include Raman scattering, fluorescence from CDOM and chlorophyll (Zhai et al., 2015, 2017a). In order to be realistically representative, two water types are employed: one is dominated by phytoplankton particles (hereafter referred as PDW), and the other is dominated by non-algae particles (hereafter referred as NDW). In this way, this study fills in several holes that earlier studies missed.
PDW consists of three components: pure sea water with absorption and scattering coefficients based on (Pope and Fry, 1997; Zhang and Hu, 2009), phytoplankton particles with IOPs parameterized in terms of [Chla] (Voss, 1992; Bricaud et al., 1998, 2010), and CDOM with absorption coefficients increasing exponentially as wavelength decrease (Morel and Maritorena, 2001). Specifically, the absorption coefficient of phytoplankton particles in the UV is from the BIogeochemistry and Optics SOuth Pacific Experiment (BIOSOPE) (Bricaud et al., 2010), which is combined with the visible data (Bricaud et al., 1998) to form a complete dataset. The scattering of light by CDOM is neglected. The scattering phase function of phytoplankton particles is characterized by the Fournier-Forand (FF) phase function, whose shape is dominated by the backscattering fraction (Fournier and Forand, 1994; Mobley et al., 2002). The scattering matrix of phytoplankton particles is based on the measurement reported by Voss and Fry (1984). In addition to the three components of PWD, NWD contains non-algae particles with IOPs characterized by the non-algae particle concentration [NAP]. Similar to CDOM, the absorption coefficient of NAP increases exponentially as wavelength decreases, though the spectral slope is different from that of CDOM (Babin et al., 2003). The scattering coefficient and scattering matrix for NAP are calculated using Mie theory assuming spherical particles. The particle size distribution (PSD) used for this calculation is a Junge size distribution with a slope of 4, and the refractive index for the calculation is assumed to be 1.2 relative to water. Readers are referred to Zhai et al. (2017b) for more details of the bio-optical model used in this work.
We studied both the reflectances at the TOA and just above the ocean surface. The TOA total reflectance is defined as: ρt = πLt/Ed, where Lt is the total radiance exiting the whole atmosphere and ocean system, and Ed is the downwelling irradiance at the TOA. The water leaving signal is , where is the TOA radiance for the system with the same atmosphere and ocean surface but without ocean water. The following wavelengths are simulated: 340, 354, 388, 416, 442, 452, 470, 510, 551, 670, 765, and 865 nm. These span the spectral range from UV to NIR, in which water leaving radiance is significant. Figure 4 shows that the total reflectance ρt decreases with increasing wavelength due to the decrease of molecular and aerosol scattering as wavelength increases. The water signal in the total reflectance is noticeable, but subtle, between 400 and 600 nm for phytoplankton (quantified by chlorophyll and designated by PDW) and non-algal particle concentrations (NDW), with the signal from NDW being more prominent.
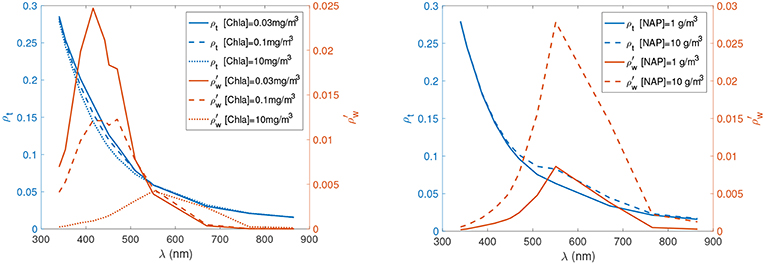
Figure 4. TOA Reflectance at nadir as a function of wavelength. The total reflectance is shown in blue by the left y axis and the water leaving signal is shown in maroon by the right y axis. The differences between heavy, solid and dashed lines are due to differences in chlorophyll amounts (left) or non-algal particle concentrations (right). The solar zenith angle is 45 degrees and the aerosol optical depth at 550 nm is 0.15. Reprinted with permission from Zhai et al. (2017a), The Optical Society (OSA).
For the water signal, (maroon curves in Figure 4) with PDW, there is an inflection wavelength between 510 and 550 nm where does not change for different [Chla] values. When the wavelength is shorter than this inflection wavelength, is larger for smaller [Chla] values. This trend is reversed when wavelength is longer than the inflection wavelength. In contrast to PDW, the NDW water signal does not have an inflection wavelength. The NDW water signal is larger for larger [NAP] across the whole spectral interval 400–600 nm, and the wavelength of the maximum remains almost constant for a wide range of [NAP]. For both PDW and NDW, the values are smaller in both UV and NIR than in the visible bands. The small-to-negligible values in the NIR are common for open (and many coastal) waters, and form the basis for heritage atmospheric correction schemes using NIR bands to constrain aerosol for retrievals in the VIS (Gordon and Wang, 1994). However, note that for NWD, that reflectance at 865 nm becomes about 0.001 for [NAP] = 10 g/m3, which is small but not negligible in terms of an aerosol retrieval or atmospheric correction. Furthermore, the small values in the UV for PDW can become very large (and even superceed the values in the VIS) if the amount of CDOM becomes very low (Chowdhary et al., 2019). Such low CDOM values can be found in extreme oligotrophic waters, such as the South Pacific (Morel et al., 2007). The small values at 340 nm for NDW, the water-type of which is more characteristic for coastal (eutropic) environments, is around the same level as in the NIR.
Figure 5 shows the relative contribution of water leaving signal at the TOA for PDW and NDW at nadir, respectively. For PDW, the maximum fraction decreases from 16 to 8%, and the wavelength corresponding to the maximum fraction shifts from 470 to 670 nm, as [Chla] increases from 0.03 to 10 mg/m3, respectively. Note that, for [Chla] = 0.03 mg/m3, the wavelength of maximum occurrence shifts into the UV if CDOM becomes very low (Chowdhary et al., 2019). At both sides of the maximum peak, the fraction decreases as wavelength decreases toward the UV or increases toward NIR. The fraction is around or <2% at wavelengths shorter than 354 nm or longer than 760 nm. For NDW, the maximum relative contribution occurs around 550 nm, and this peak does not shift spectrally as [NAP] values change. However, the relative contribution does increase in magnitude from around 15–35%, when [NAP] increases from 1 to 10 g/m3. As wavelength approaches the UV, the fraction decreases at a faster rate than that of PDW due to the light absorption in that part of the spectrum by the NAP constituents in the water. In the NIR, the water-leaving fraction is still significant for NDW, which is around 8% or less at nadir.
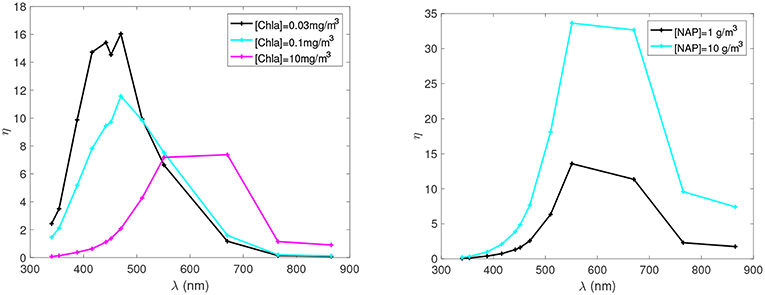
Figure 5. Water-leaving relative percentage contribution in the TOA reflectance for the same case as in Figure 4. Reprinted with permission from Zhai et al. (2017a), The Optical Society (OSA).
Figure 5 also illustrates the reasons why traditional algorithms, needing to separate atmospheric and oceanic signals in the radiometric measurements have made use of the NIR. See section The Coupled Atmospheric-Oceanic System. In this wavelength range, η is much lower than in the visible, allowing characterization of the atmospheric constituents including aerosol without much contribution from the ocean. From Figure 5, we can also anticipate similar advantages for aerosol characterization by using the UV wavelengths. Without a MAP, the recommendation is to make use of careful spectral range selection to separate ocean and atmospheric signals. However, there remains some sensitivity to [Chla] and [NAP] in both the UV and in the NIR radiometric quantities, which can be reduced by working with polarized measurements.
Figure 6 show the relative percentage contribution of the polarized water leaving signal to the TOA polarized radiance field for the same case shown in Figure 4. The spectral variation of the polarized water-leaving fraction is due to the different bio-optical properties of the PDW and NDW waters, as the atmosphere properties are kept the same for these cases. The spectral behavior is similar to that of the total reflectance, but the magnitude of relative contribution is much less. Note the different scales on the y-axes of Figure 6 vs. Figure 5. The wavelength of the PDW maximum fraction strongly depends on [Chla], but the wavelength of the NDW maximum fraction is less sensitive to [NAP]. The PDW maximum fraction is larger for smaller [Chla]; while the NDW maximum fraction is larger for larger [NAP]. The PDW maximum fraction increases only from 2 to 5.5% for [Chla] decreasing from 10 to 0.03 mg/m3; while the NDW maximum fraction increases only from 6 to 16% for [NAP] increasing from 1 to 10 g/m3. Note that these increases will become larger if the amount of CDOM in the ocean becomes less (see Chowdhary et al., 2012, which was computed for smaller amounts of CDOM). Nevertheless, the satellite-measured polarized signal will be much less affected by variability in oceanic constituents than the total radiometric signal. The sensitivity study shown in Figures 4–6 did not include the viewing angular variation of water leaving contributions to the TOA signals, which was previously studied in Chowdhary et al. (2006) for open ocean waters.
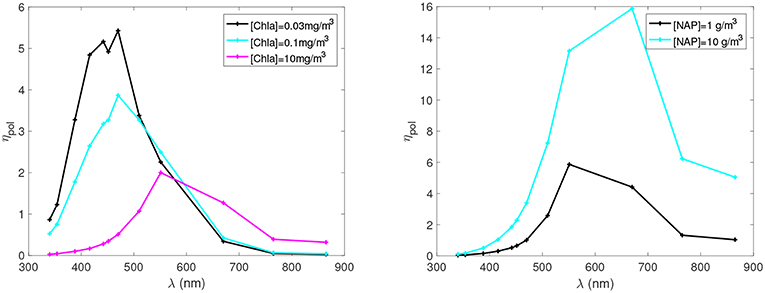
Figure 6. Polarized water-leaving relative percentage contribution in the TOA polarized reflectance for the same case as Figure 4. Reprinted with permission from Zhai et al. (2017a), The Optical Society (OSA).
These results are important for designing algorithms for PACE MAPs, either for retrieving aerosol or for atmospheric correction. In terms of retrieving aerosol, these results show an interplay in the UV and shortwave blue range between the effects of chlorophyll and non-algal particle concentrations. Adding non-algal particles will increase the absorption in that range, and offset the scattering expected from PDW. This oceanic complexity in this spectral range adds to the already complex atmospheric situation in the shortwave in which there is interplay between the spectral aerosol absorption, aerosol particle size, and height of the aerosol layer. Furthermore, there are aerosols (specifically: brown carbon particulates) that exhibit the same spectral increase in absorption toward the UV as CDOM in the ocean, which complicates separating the atmospheric and oceanic contribution to TOA radiance in the UV (Chowdhary et al., 2019). Together these oceanic and atmospheric properties will introduce significant uncertainty in making use of the shortwave observations in these wavelengths (Kahn et al., 2016).
An encouraging outcome from these results is that the relative and absolute magnitudes of the different contributions from PDW, and non-algal particles show that the polarized water signal contributes <1/3 the signal to TOA measurements than does the total water signal, across all wavelengths and for both PDW and non-algal water constituents. This suggests aerosol-retrieval algorithms based on polarized measurements or joint atmospheric-oceanic retrievals that exploit both polarized and total TOA measurements from the UV to the NIR should find adequate constraints on the ocean signal to better characterize aerosol properties than non-polarized sensors. In addition, we note that this sensitivity study was conducted at only one geometry and does not illustrate the added capability of combining polarimetry with multi-angle views. However, this theoretical study only touches upon the complexities exhibited by total and polarized spectral water reflectances. In practice, the uncertainties introduced by these complexities may hinder some of the advantages suggested by Figure 6 for optimized joint atmosphere-ocean retrievals.
Multi-Angle Polarimeter Aerosol Retrievals Over Ocean and Land
Multiangular polarimetric observations of the coupled atmosphere-ocean system offer unique opportunities to separate and retrieve variations in the oceanic and atmospheric properties that otherwise lead to similar spectral signatures in TOA total radiance. As noted above, given that some aerosols show a strong spectral increase in absorption coefficient in the near-UV (e.g., Koven and Fung, 2006; Bergstrom et al., 2007; Russell et al., 2010) that also occurs for oceanic non-algal particles and CDOM (e.g., Jerlov, 1976; Bricaud and Stramski, 1990; Nelson and Siegel, 2013; Wei et al., 2016), their impact on spaceborne measurements of intensity will be difficult to distinguish or might even cancel each other out. On the other hand, variations in absorbing aerosols and non-algal particles will likely lead to different changes in angular features of TOA polarized light that can be distinguished even if these changes occur simultaneously (Chowdhary et al., 2019).
A multi-angle polarimeter inversion is different from the types of retrievals applied to radiometers for the past 15 years. Heritage aerosol retrievals must use a priori assumptions about the aerosol and surface properties plus a pre-calculated Look Up Table, and are often limited to only retrieving aerosol loading and some information about aerosol type and height for general aerosol conditions. In contrast, the simultaneous inversion of multiple wavelengths, angles and polarization states, with a minimum of constraints, can retrieve aerosol optical thickness, particle shape, size distribution and complex refractive index, while simultaneously constraining parameters of the surface beneath, even if a full simultaneous retrieval of the surface characteristics is not made (Mishchenko et al., 2007; Waquet et al., 2009a; Dubovik et al., 2011; Knobelspiesse et al., 2012; Wu et al., 2015). Some of this potential is illustrated in Figure 3. Multi-angle radiance and polarimetric observation improves upon radiometry alone in reducing the number of a priori assumptions required for aerosol retrieval and allows a more physical characterization of the scene.
An early example of the power of multi-angle polarimetry is the use of ocean glint as a bright backdrop from which to accurately retrieve aerosol absorption. The success of this retrieval depends on first constraining the aerosol scattering properties, including the real part of the refractive index. This can only be done with multi-angle looks at the same aerosol, with at least one view in glint and one not in glint, and to achieve the required accuracy in the scattering properties retrieval, only polarimetry provides sufficient information (Kaufman et al., 2002; Ottaviani et al., 2013).
Other examples, based on analyses of airborne MAP data, have shown that not only can a multi-angle polarimeter retrieve a comprehensive set of aerosol properties in cloud-free scenes over open ocean waters (Chowdhary et al., 2001, 2002, 2005; Xu et al., 2016; Stamnes et al., 2018), they can do the same for coastal waters (Gao et al., 2018, 2019). Moreover, MAP can be used to characterize aerosol properties above clouds and below thin cirrus clouds, at least in some situations, because aerosols and clouds have different polarimetric signatures (Knobelspiesse et al., 2011; Waquet et al., 2013; Peers et al., 2015; Xu et al., 2018). In principle, aerosol retrievals within broken cloud fields may also be possible (Hasekamp, 2010), although three dimensional (3D) effects may seriously hamper the accuracy (Davis et al., 2013; Stap et al., 2016). To overcome this limitation, substantial development of 3D multi-angle/multi-pixel algorithms will be required (Martin et al., 2014; Levis et al., 2015; Martin and Hasekamp, 2018). The ability of a MAP to retrieve in traditionally cloud-masked scenes increases the availability of the aerosol characterization and provides a more accurate global picture of aerosol forcing. We expect improvement from MAPs in this respect despite new algorithms applied to heritage radiometers or to the PACE OCI that are allowing for aerosol retrievals above clouds (Jethva et al., 2013, 2014, 2016; Meyer and Platnick, 2015; Sayer et al., 2016; Remer et al., 2019).
While we have focused this study mostly on retrieving aerosol characteristics over ocean, a MAP represents opportunity to step up from heritage capabilities in retrieving aerosol properties over land as well (Waquet et al., 2009b; Dubovik et al., 2011; Wu et al., 2015; Xu F. et al., 2017; among others). As an example, Figure 7 shows a comparison of retrieved aerosol optical depth (AOD), single scattering albedo (SSA), and size distributions with collocated AERONET Level 1.5 aerosol products. The MAP measurements resulting in these retrievals are from AirMSPI, which has 8 aerosol bands in the UV to near infrared, 7 of which are used for aerosol retrieval (Diner et al., 2013). The AirMSPI retrievals demonstrate a reasonable agreement except for the coarse particle size mode, likely due to the lack of shortwave infrared bands on AirMSPI. Overall, AirMSPI AOD validation with 27 cases of available field observations shows a spectrally mean R2 of 0.95, linear regression mean slope of 0.97, and mean intercept of 0.01, reflecting high retrieval quality (Xu F. et al., 2017).
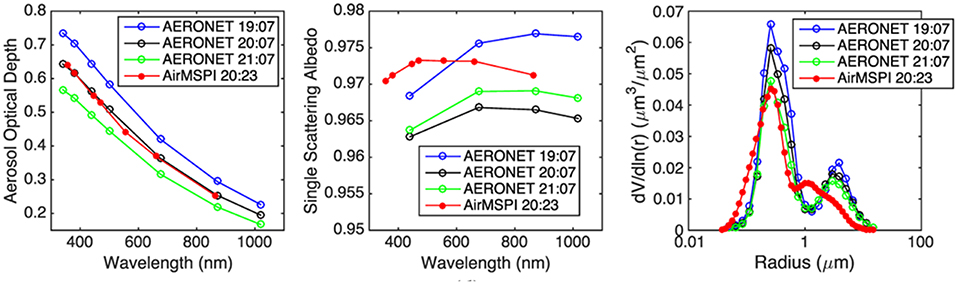
Figure 7. AirMSPI-retrieved aerosol optical depth (AOD), single scattering albedo (SSA), and volume-weighted aerosol size distribution collocated with the Fresno AERONET site on January 6, 2012, compared to the AERONET-derived values. Different times of observation noted. Adapted with permission from Xu F. et al. (2017), John Wiley & Sons.
There are some limitations to using polarimetry for aerosol characterization. All aerosol retrieval procedures using polarimetry require measurements over specific ranges of scattering angles, depending on the retrieval approach. The ranges and values of scattering angles that would be measured by a polar orbiting instrument will vary with solar and viewing geometries. Thus, the availability and quality of derived products will vary with location and time, especially for the outer portions of an instrument's swath. However, as demonstrated in Figure 8, polarimetry yields still substantial improved capability over radiometry even for less favorable or reduced scattering angles.
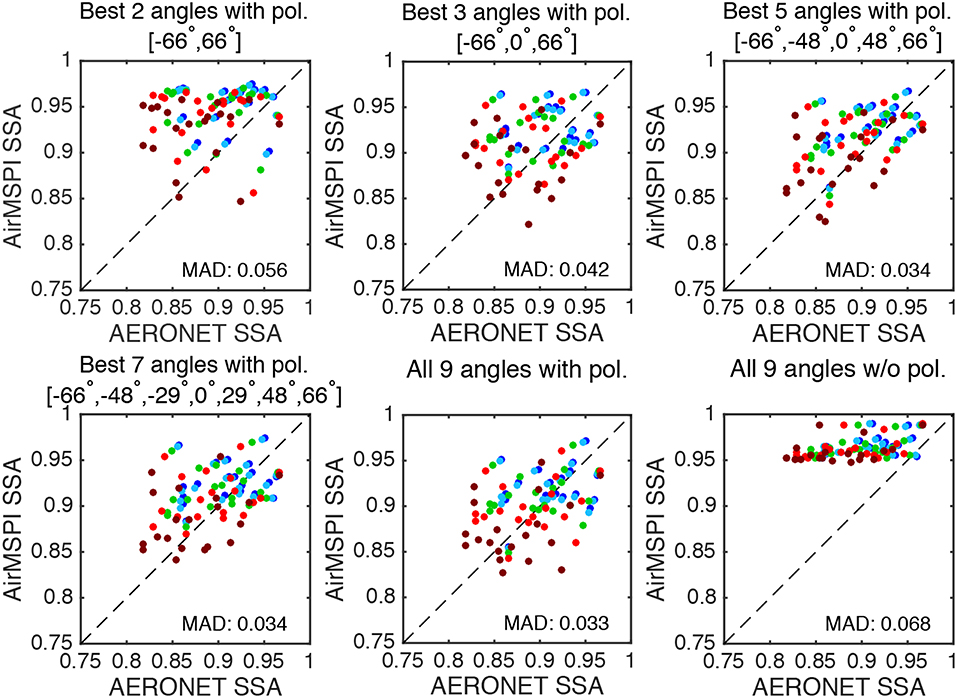
Figure 8. Scatter plots of AirMSPI-retrieved SSA against collocated AERONET values using a different number of viewing angles and polarization features. The results of SSA are plotted in different colors for five wavelengths from visible to near-infrared: dark blue (445 nm), light blue (470 nm), green (555 nm), red (660 nm), and brown (865 nm). The mean absolute difference (MAD) between the two retrievals is listed in the bottom right of each subplot. “Best angles” are identified as the subset of AirMSPI viewing angles that gives the best agreement with AERONET derived values. Reprinted with permission from Xu F. et al. (2017), John Wiley & Sons.
In addition to characterizing aerosol microphysical and optical properties, MAP measurements at 410 nm also provide information on aerosol layer height. The reason is that, depending on its altitude, an elevated aerosol layer shields the strong polarization signal from Rayleigh scattering by air molecules. The potential of aerosol layer height retrievals from MAP measurements has been demonstrated by Wu et al. (2016) using airborne measurements from the Research Scanning Polarimeter (RSP: Cairns et al., 1999). They found good agreement between the aerosol layer height retrieved from RSP and measurements from the Cloud Physics Lidar (CPL: McGill et al., 2002) flying on the same platform. The left panel of Figure 9 shows the comparison between aerosol layer heights derived from RSP and CPL measurements, and the right panel shows the comparison if only radiance (intensity) measurements of RSP are used. Clearly, without the polarization measurements the information on aerosol height is virtually absent. Wu et al. (2016) also showed that the availability of 410 nm polarization measurements and a high polarimetric accuracy are the most important factors determining the capability to retrieve aerosol layer height. These results do not invalidate the possibility that other methods without polarization, such as Oxygen A-band retrievals, may also deliver similar information on aerosol layer height (Xu X. G. et al., 2017; Davis and Kalashnikova, 2019). Nevertheless, one advantage of using polarimetry to retrieve aerosol height is that it is uses observations in the blue-UV, where aerosol optical depths are larger than in the Oxygen A-band, which may allow height retrievals in situations with less aerosol loading.
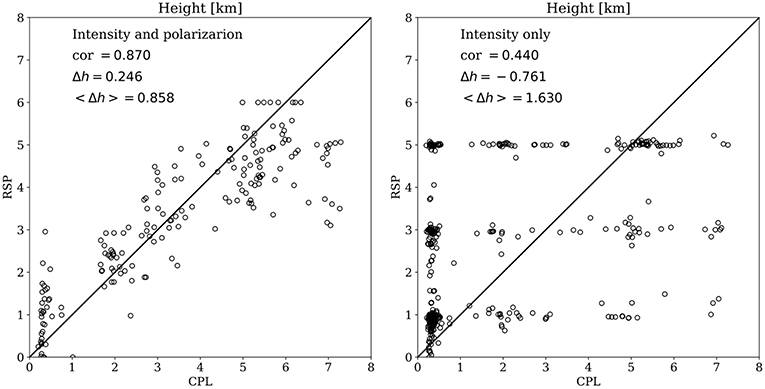
Figure 9. Retrieved aerosol layer height from the Research Scanning Polarimeter (RSP) vs. the same derived from the Cloud Physics Lidar (CPL) using both radiance and polarization measurements (left), and using only radiance measurements (right). Measurements were obtained during the Polarimeter Definition Experiment (PODEX) and the Studies of Emissions, Atmospheric Composition, Clouds and Climate Coupling by Regional Surveys (SEAC4RS) measurement campaigns. Adapted with permission from Wu et al. (2016), John Wiley & Sons.
The caveats to relying on multi-angle polarimetry for characterizing aerosol from the PACE mission include the details of the limitations of an individual instrument's swath, spectral coverage, spatial resolution and polarimetric accuracy. By flying two complementary polarimeters on PACE, namely SPEXone and HARP2, the mission somewhat compensates individual instrument limitations. At the very least, the instrument teams intend to use their collocation on the PACE observatory to cross calibrate radiometric and polarimetric measurements. Furthermore, comparison of instrument-specific retrievals of aerosol properties, made by each sensor along the SPEXone narrow swath, will aid in evaluating individual sensor performance and algorithms. Actual retrieval applied to the joint simultaneous measurements of both PACE MAPs is challenging. Despite having two MAPs on the same platform, PACE will fly with no polarimetric measurements at wavelengths longer than 850 nm, which may affect accuracy of aerosol coarse mode characterization and overall abilities over land surfaces. Algorithms used to fully exploit the advantages of polarimetry for aerosol retrievals are complex, requiring effort to develop and maintain. Moreover, existing approaches are currently computationally costly, although we expect computational power to increase substantially by the time the PACE mission launches. Furthermore, development of more computationally efficient retrieval algorithms is also underway (Dubovik et al., 2014; Di Noia et al., 2017).
In summary, despite the caveats, the potential PACE mission benefits of multi-angle polarization in characterizing aerosol are substantial, proven and documented.
Synergy Between OCI and MAP for Aerosol Retrievals
The primary PACE instrument is the Ocean Color Instrument (OCI), a 5-nm spectral resolution single-view radiometric sensor, that will share the satellite with the two secondary contributed MAPs. Collocated data from OCI and the multi-angle polarized measurements will provide a rich dataset for Earth system exploration, however, new retrieval techniques still need to be developed to take advantage of the large information content of such datasets.
OCI's capabilities could aid aerosol retrievals from the MAPs in the realm of radiometric calibration, gaseous correction and constraints on aerosol height and coarse mode particles. OCI's well-calibrated 5-nm measurements allow for better radiometric calibration of both PACE MAPs if the MAPs are designed to match OCI's measurements. For example, to make optimal use of the high radiometric accuracy of OCI, SPEXone has chosen two of its viewing angles at +20° and −20° to overlap with OCI views. Note that OCI is a single view instrument but will not point nadir. In order to avoid ocean sun glint, OCI will perform a tilt maneuver every orbit at the sub-solar point so that it will point 20° forward in the northern hemisphere and 20° aft in the southern hemisphere. Also, OCI, with its 5-nm resolution, will quantify and subsequently correct for absorbing gases and retrieve information on aerosol height across HARP2's wide swath. OCI provides more bands in the chlorophyll absorption peak, which is useful in validating and/or better constraining a rudimentary ocean reflectance model for constraining ocean reflectance for aerosol retrievals, again across the broad swath. SPEXone also observes at 5 nm resolution, but only in its much narrower swath. Also, OCI provides information on aerosol absorption further into the UV than the MAPs allowing for better constraint on the direct radiative effect of aerosols. Lacagnina et al. (2017) have used a similar synergy between POLDER and OMI.
However, most of the synergy between PACE instruments flows in the other direction with the MAPs aiding OCI in separating the aerosol signal from the ocean signal, and vice-versa. Conversely, MAP's information content as compared with OCI will allow for much better characterization of aerosol properties. This will help to constrain OCI aerosol characterization and atmospheric correction (Frouin et al., 2019). Additionally, because large amounts of sediments and CDOM in coastal waters can masquerade as absorbing aerosols in the satellite-measured signal in the UV/shortwave visible range, algorithms applied to OCI alone will find it difficult to isolate the atmospheric signal to make use of the aerosol signal there, or to isolate the ocean signal to derive water-leaving radiance. This is when making use of MAP observations can help separate and characterize the aerosol scattering contributions for aerosol retrievals.
Another synergy between OCI and the MAPs is the quantification of the direct radiative effect of aerosols above clouds by calculating the difference between the total outgoing irradiance and the outgoing irradiance for an atmosphere with no aerosol and only the cloud (de Graaf et al., 2014). Here, the total outgoing irradiance can be estimated from the measured spectral radiance. Currently, this is being done from single viewing angle radiance measurements, but this estimate can be significantly improved using multi-angle measurements. To best estimate the outgoing irradiance combined measurements of cloud and aerosol properties are needed, and it will take the combination of both PACE MAPs with the PACE OCI to deliver the required characterization.
Discussion
The PACE mission will be an opportunity to expand characterization of global aerosols with the Ocean Color Instrument (OCI), a single-view broad spectrum, wide swath, radiometer and two complementary multi-angle polarimeters (MAPs). For aerosols, OCI primarily has the capability to continue the heritage retrieval algorithms associated with heritage sensors such as MODIS and OMI. OCI also has the potential to enhance aerosol characterization by means of its broad spectrum and fine spectral resolution through the oxygen A band (Davis and Kalashnikova, 2019; Remer et al., 2019). However, any OCI aerosol retrieval algorithm will require a priori assumptions because its single view radiometric measurements are limited in information content.
Multi-Angle Polarimeters will dramatically increase the information content of the PACE mission to produce a more complete characterization of aerosol parameters not possible today. The challenge in making use of the full information content of a MAP is in the separation of satellite-measured signal into atmospheric and oceanic components. One approach to retrieving aerosol properties from satellite is to avoid the ocean signal as much as possible by using wavelengths that are assumed to be dark in water leaving reflectance and avoiding situations that do not meet this assumption. This will often regulate the aerosol retrieval to Case I waters, far from estuaries and coastlines, sometimes where the most societally relevant aerosol occurs.
The alternative to this limiting set of assumptions is to approach the atmospheric-oceanic decoupling with a joint retrieval that returns both aerosol and ocean color properties together. The requisite radiative transfer models that can accurately (i.e., consistent with current and future MAP accuracies) and realistically (e.g., including inelastic underwater light scattering, scattering by complex inhomogeneous particles, and light propagation in horizontally inhomogeneous media) simulate radiative transfer of polarized light through atmosphere-ocean systems are now becoming feasible (Chowdhary et al., 2019). Such joint retrievals are possible (e.g., Chowdhary et al., 2012; Stamnes et al., 2018; Gao et al., 2019), but come with challenges. One of the requirements is that oceanic bio-optical models that were used in past polarimetric retrievals need to catch up (i.e., incorporate more inherent optical parameters, e.g., parameterization of the oceanic Mueller matrix in terms of oceanic constituents) with those used for modern-age radiometric ocean color retrievals. There is also a need to better characterize the scattering matrices of suspended hydrosols. Both of these requirements are currently in the process of being addressed (Chowdhary et al., 2019). We need to continue our research on these issues in the next few years, in order to be prepared for the PACE mission and the simultaneous inversions that such a mission will support.
While the expectations are high for the PACE MAPs' aerosol characterization capabilities, this enthusiasm does not negate the need to continue and expand upon aerosol retrievals from radiometry. In particular, the PACE OCI with its fine spectral resolution measurements and broad spectral range offers an alternative and complementary path forward for aerosol characterization during the PACE era (Remer et al., 2019). Single-view radiometry for aerosol retrieval enjoys a long heritage, and while algorithms applied to radiometers require greater reliance on assumptions to constrain the retrieval, those assumptions are based in physical understanding and experience acquired over decades. As research continues to meet the challenges to obtain joint atmospheric-oceanic retrievals from the PACE MAPs, we must not neglect preparation for OCI-centered aerosol characterization.
Sensitivity studies have been conducted using coupled radiative transfer modeling during the tenure of this PACE science team. These studies model the contribution of water-leaving radiance to the TOA signal, across the spectrum, for both total radiance and polarimetric radiance for a variety of water constituents. The results indicate that polarized radiance is much less affected by variability of chlorophyll-containing and non-algal constituents in the water than is total radiance (intensity). Thus, when attempting retrievals of aerosol properties over water with unconstrained water-leaving radiances, using the polarized signal instead of intensity should introduce much less uncertainty.
To prepare for PACE, a test bed of multi-angle polarimeter data is needed to verify the sensitivity studies and to prepare and test algorithms. There are now several airborne MAPs flying and making measurements that could be used as test cases. Recently, four different airborne MAPs flew on the NASA ER-2 as part of the Aerosol Characterization from Polarimeter and Lidar (ACEPOL) campaign. These data span the specific capabilities expected of any MAP that would fly on the PACE mission, and therefore provide adequate test data for algorithm development. This is in addition to new algorithms being developed and applied to POLDER/PARASOL observations The effort should be placed in continuing to fly these airborne instruments over scenes of particular interest to PACE, including aerosol events and clouds, over land and ocean, and to collocate these scenes with ancillary data that can be used to verify proto-algorithm retrievals. Also, effort should be directed toward processing the collected data and making it quickly available for algorithm development, as well as to use POLDER/PARASOL data as an opportunity to explore MAP information content with actual space borne measurements.
Retrievals applied to real observations show that MAP-retrieved aerosol parameters match ground truth to accuracies within expectations, and also demonstrate the relative importance of polarization to multi-angle capabilities. We expect a PACE MAP to improve accuracy of the global aerosol parameters that we now retrieve, expand the type of parameters that we can retrieve, and extend retrievals to situations previously unattainable from heritage aerosol satellite missions. In summary, the potential PACE mission benefits of multi-angle polarization in characterizing aerosol are substantial, proven, and documented.
Author Contributions
This work represents part of the overall consensus statement agreed to by the first NASA PACE Science Team and includes contributions from the Principal Investigators of the Atmospheric Correction subgroup of that Science Team, along with their associates involved with the aerosol characterization focus of that subgroup. All authors of this paper were part of the discussions of that subgroup and Science Team from 2014 to 2018. In addition, LR was Deputy Lead of the Science Team and Leader of the subgroup. She organized this particular paper and consolidated the input from all authors. KK made significant contributions to the discussion and writing of all sections, but was primarily responsible for the information content study and the section that discusses the challenges in realizing the value of that content. P-WZ was responsible for the analysis and writing of the section that explored the sensitivity of using the UV part of the spectrum for aerosol retrieval over complex water. FX did the analysis and provided the figures that show AirMSPI retrievals validated against AERONET. He was supported in that effort by OK, who also took responsibility for the multi-angle radiometer-only section. JC provided much of the material in the section that discusses the issues with separating aerosol signal from ocean signal. OH and OD provided most of the material for the section on heritage retrievals using POLDER. OH and LW gave us the aerosol height retrieval information and figures. ZA, EB, BC, OC, AD, HD, DD, BF, RF, B-CG, AI, RL, JM, AO, and OT were active participants during the years of discussion that resulted in this paper, and each has made contributions, edited, or commented on this manuscript.
Funding
This work was supported by NASA Grants under the solicitation NNH13ZDA001N-PACEST. In addition, LR, RL, and OT acknowledge funding from NASA grant NNX15AD15G. OK and AD contribution was supported by the PACE science team grant, under Paula Bontempi. P-WZ acknowledges funding from NASA grant 80NSSC18K0345. OC acknowledges funding from NASA grant NNX15AC78G.
Conflict of Interest Statement
AI was employed by Science Application International Inc.
The remaining authors declare that the research was conducted in the absence of any commercial or financial relationships that could be construed as a potential conflict of interest.
The handling editor is currently co-organizing a Research Topic with one of the authors OD, and confirms the absence of any other collaboration.
Acknowledgments
Portions of this work were performed by OK, AD and DD at the Jet Propulsion Laboratory, California Institute of Technology, under a contract with the National Aeronautics and Space Administration.
Abbreviations
3D, Three dimensional; 3MI, Multi-viewing, Multi-channel, Multi-polarization Imager; AAOD, Absorbing Aerosol Optical Depth; AATSR, Advanced Along Track Scanning Radiometer; ACE, Aerosol Cloud Ecosystems; ACEPOL, Aerosol Characterization from Polarimeter and Lidar; ADEOS, Advanced Earth Observation Satellite; AERONET, Aerosol Robotics Network; AirHARP, Airborne version of Hyper Angle Rainbow Polarimeter; AirMSPI, Airborne Multiangle SpectroPolarimetric Imager; AirSPEX, Airborne version of Spectro-Polarimeter for Planetary Exploration; AMS, Aerosol Mass Spectrometer; AOD, Aerosol Optical Depth; AOT, Aerosol Optical Thickness; ATSR-2, Along Track Scanning Radiometer 2; BC, Black Carbon; BIOSOPE, BIogeochemistry and Optics SOuth Pacific Experiment; BRDF, Bi-Reflection Directional Function; CDOM, Colored Dissolved Organic Material; Chla, Chlorophyll-a; CPL, Cloud Physics Lidar; DFS, Degrees of Freedom for Signal; DoLP, Degree of Linear Polarization; ER-2, Earth Research-2; ESA, European Space Agency; FF, Fournier-Forand; GRASP, Generalized Retrieval for Aerosol and Surface Properties; HARP2, Hyper Angle Rainbow Polarimeter-2; IOP, Inherent Optical Properties; MAIA, Multi-Angle Imager for Aerosols; MAN, Maritime Aerosol Network; MAP, Multi-Angle imaging Polarimeters; MISR, Multi-angle Imaging SpectroRadiometer; MODIS, MODerate resolution Imaging Spectroradiometer; NAP, Non-Algae Particle concentration; NASA, National Aeronautics Space Administration; NDW, Non-algae Dominated Water; NIR, Near InfraRed; OCI, Ocean Color Instrument; OMI, Ozone Monitoring Instrument; PACE, Plankton, Aerosols, Clouds, ocean Ecosystems; PARASOL, Polarization and Anisotropy of Reflectances for Atmospheric Sciences coupled with Observations from a Lidar; PDW, Phytoplankton Dominated Water; PODEX, Polarimeter Definition Experiment; POLDER, POLarization and Directionality of the Earth's Reflectance; PSD, Particle Size Distribution; RSP, Research Scanning Polarimeter; SEAC4RS, Studies of Emissions, Atmospheric Composition, Clouds and Climate Coupling by Regional Surveys; SLSTR, Sea and Land Surface Temperature Radiometer; SP2, Soot Photometer; SPEXone, Spectro-Polarimeter for Planetary Exploration-1; SRON, Netherlands Institute for Space Research; SSA, Single Scattering Albedo; TOA, Top Of Atmosphere; UV, Ultraviolet; VIS, Visible.
References
Babin, M., Stramski, D., Ferrari, G. M., Claustre, H., Bricaud, A., Obolensky, G., et al. (2003). Variations in the light absorption coefficients of phytoplankton, nonalgal particles, and dissolved organic matter in coastal waters around Europe. J. Geophys. Res. 108:3211. doi: 10.1029/2001JC000882
Bergstrom, R. W., Pilewskie, P., Russell, P. B., Redemann, J., Bond, T. C., Quinn, P., et al. (2007). Spectral absorption properties of atmospheric aerosols. Atmos. Chem. Phys. 7, 5937–5943. doi: 10.5194/acp-7-5937-2007
Bricaud, A., Babin, M., Claustre, H., Ras, J., and Tie‘che, F. (2010). Light absorption properties and absorption budget of Southeast Pacific waters. J. Geophys. Res. 115:C08009. doi: 10.1029/2009JC005517
Bricaud, A., Morel, A., Babin, M., Allali, K., and Claustre, H. (1998). Variations of light absorption by suspended particles with chlorophyll a concentration in oceanic (case 1) waters: analysis and implications for bio-optical models. J. Geophys. Res. 103, 31033–31044. doi: 10.1029/98JC02712
Bricaud, A., and Stramski, D. (1990). Spectral absorption coefficients of living phytoplankton and nonalgal biogenous matter: a comparison between the Peru upwelling area and the Sargasso Sea. Limnol. Oceanogr. 35, 562–582. doi: 10.4319/lo.1990.35.3.0562
Cairns, B., Travis, L. D., and Russel, E. E. (1999). The Research Scanning Polarimeter: Calibration and ground-based measurements. Proc. SPIE 3754, 186–196. doi: 10.1117/12.366329
Chami, M. (2007). Importance of the polarization in the retrieval of oceanic constituents from the remote sensing reflectance. J. Geophys. Res. 112:C05026. doi: 10.1029/2006JC003843
Chen, C., Dubovik, O., Henze, D. K., Lapyonak, T., Chin, M., Ducos, F., et al. (2018). Retrieval of desert dust and carbonaceous aerosol emissions over Africa from POLDER/PARASOL products generated by the GRASP algorithm. Atmos. Chem. Phys. 18, 12551–12580. doi: 10.5194/acp-18-12551-2018
Chowdhary, J., Cairns, B., Mishchenko, M., and Travis, L. (2001). Retrieval of aerosol properties over the ocean using multispectral and multiangle photopolarimetric measurements from the Research Scanning Polarimeter. Geophys. Res. Lett. 28, 243–246. doi: 10.1029/2000GL011783
Chowdhary, J., Cairns, B., Mishchenko, M. I., Hobbs, P. V., Cota, G. F., Redemann, J., et al. (2005). Retrieval of aerosol scattering and absorption properties from photopolarimetric observations over the ocean during the CLAMS experiment. J. Atmos. Sci. 62, 1093–1117. doi: 10.1175/JAS3389.1
Chowdhary, J., Cairns, B., and Travis, L. D. (2002). Case studies of aerosol retrievals over the ocean from multiangle, multispectral photopolarimetric remote sensing data. J. Atmos. Sci. 59, 383–397. doi: 10.1175/1520-0469(2002)059%3C0383:CSOARO%3E2.0.CO;2
Chowdhary, J., Cairns, B., and Travis, L. D. (2006). Contribution of water-leaving radiances to multiangle, multispectral polarimetric observations over the open ocean: bio-optical model results for case 1 waters. Appl. Opt. 45, 5542–5567. doi: 10.1364/AO.45.005542
Chowdhary, J., Cairns, B., Waquet, F., Knobelspiesse, K., Ottaviani, M., Redemann, J., et al. (2012). Sensitivity of multiangle, multispectral polarimetric remote sensing over open oceans to water-leaving radiance: analyses of RSP data acquired during he MILAGRO campaign. Remote Sens. Environ. 118, 284–308. doi: 10.1016/j.rse.2011.11.003
Chowdhary, J., Zhai, P., Boss, E., Dierssen, H., Frouin, R., Ibrahim, A., et al. (2019). Radiative transfer in atmosphere-ocean systems. Front. Earth Sci. 7:100. doi: 10.3389/feart.2019.00100
Cox, C., and Munk, W. (1954). Measurement of the roughness of the sea surface from photographs of the sun's glitter. Opt. Soc. Amer. 44, 838–850. doi: 10.1364/JOSA.44.000838
Davis, A. B., Garay, M. J., Xu, F., Qu, Z., and Emde, C. (2013). “3D radiative transfer effects in multi-angle/multi-spectral radio-polarimetric signals from a mixture of clouds and aerosols viewed by a non-imaging sensor,” in S.P.I.E. Proceedings, Vol. 8873, Polarization Science and Remote Sensing VI, eds J. A. Shaw and D. A. LeMaster (San Diego, CA). doi: 10.1117/12.2023733
Davis, A. B., and Kalashnikova, O. V. (2019). “Aerosol layer height over water via O2 A-band observations from space: a tutorial,” in Springer Series in Light Scattering, Vol. 3: Radiative Transfer and Light Scattering, ed A. A. Kokhanovsky (Heidelberg: Springer), 133–166. doi: 10.1007/978-3-030-03445-0_4
de Graaf, M., Bellouin, N., Tilstra, L. G., Haywood, J., and Stammes, P. (2014). Aerosol direct radiative effect of smoke over clouds over the southeast Atlantic Ocean from 2006 to 2009. Geophys. Res. Lett. 41, 7723–7730. doi: 10.1002/2014GL061103
de Leeuw, G., Sogacheva, L., Rodriguez, E., Kourtidis, K., Aristeidis, K., Georgoulias, G., et al. (2018). Two decades of satellite observations of AOD over mainland China using ATSR-2, AATSR and MODIS/Terra: data set evaluation and large-scale patterns. Atmos. Chem. Phys. 18:1573. doi: 10.5194/acp-18-1573-2018
Deschamps, P. Y., Breon, F. M., Leroy, M., Podaire, A., Bricaud, A., Buriez, J. C., et al. (1994). The POLDER mission: instrument characteristics and scientific objectives. IEEE Trans. Geosci. Rem. Sens. 32, 598–615. doi: 10.1109/36.297978
Deuzé, J. L., Goloub, P., Herman, M., Marchand, A., Perry, G., Susana, S., et al. (2000). Estimate of the aerosol properties over the ocean with POLDER. J. Geophys. Res. 105, 15329–15346. doi: 10.1029/2000JD900148
Deuzé, J. L., Herman, M., Goloub, P., Tanr,é, D., and Marchand, A. (1999). Characterization of aerosols over ocean from POLDER/ADEOS-1. Geophys. Res. Lett. 26, 1421–1424. doi: 10.1029/1999GL900168
Di Noia, A., Hasekamp, O. P., Wu, L., van Diedenhoven, B., Cairns, B., and Yorks, J. E. (2017). Combined neural network/Phillips-Tikhonov approach to aerosol retrievals over land from the NASA Research Scanning Polarimeter. Atmos. Meas. Tech. 10, 4235–4252. doi: 10.5194/amt-10-4235-2017
Diner, D. J., Beckert, J. C., Reilly, T. H., Bruegge, C. J., Conel, J. E., Kahn, R. A., et al. (1998). Multi-angle Imaging SpectroRadiometer (MISR) instrument description and experiment overview. IEEE Trans. Geosci. Remote Sens. 36, 1072–1087. doi: 10.1109/36.700992
Diner, D. J., Boland, S. W., Brauer, M., Bruegge, C., Burke, K. A., Chipman, R., et al. (2018). Advances in multiangle satellite remote sensing of speciated airborne particulate matter and association with adverse health effects: from MISR to MAIA. J. Appl. Remote Sens. 12:042603. doi: 10.1117/1.JRS.12.042603
Diner, D. J., Davis, A., Hancock, B., Geier, S., Rheingans, B., Jovanovic, V., et al. (2010). First results from a dual photoelastic-modulator-based polarimetric camera. Appl. Opt. 49, 2929–2946. doi: 10.1364/AO.49.002929
Diner, D. J., Xu, F., Garay, M. J., Martonchik, J. V., Rheingans, B. E., Geier, S., et al. (2013). The Airborne Multiangle SpectroPolarimetric Imager (AirMSPI): a new tool for aerosol and cloud remote sensing. Atmos. Meas. Tech. 6, 2007–2025. doi: 10.5194/amt-6-2007-2013
Dubovik, O., Herman, M., Holdak, A., Lapyonok, T., Tanr,é, D., Deuz,é, J. L., et al. (2011). Statistically optimized inversion algorithm for enhanced retrieval of aerosol properties from spectral multi-angle polarimetric satellite observations. Atmos. Meas. Tech. 4, 975–1018. doi: 10.5194/amt-4-975-2011
Dubovik, O., Lapyonok, T., Litvinov, P., Herman, M., Fuertes, D., Ducos, F., et al. (2014). “GRASP: a versatile algorithm for characterizing the atmosphere,” in SPIE: Newsroom (Bellingham, WA). doi: 10.1117/2.1201408.005558
Dubovik, O., Li, Z., Mishchenko, M. I., Tanré, D., Karol, Y., and Yin, D. (2019). Polarimetric remote sensing of atmospheric aerosols: Instruments, methodologies, results, and perspectives. J. Quant. Spectrosc. Radiat. Trans. 224, 474–511. doi: 10.1016/j.jqsrt.2018.11.024
Dubovik, O., Smirnov, A., Holben, B. N., King, M. D., Kaufman, Y. J., Eck, T. F., et al. (2000). Accuracy assessments of aerosol optical properties retrieved from Aerosol Robotic Network (AERONET) Sun and sky radiance measurements. J. Geophys. Res. 105, 9791–9806. doi: 10.1029/2000JD900040
Ferlay, N., Thieuleux, F., Cornet, C., Davis, A. B., Dubuisson, P., Ducos, F., et al. (2010). Toward new inferences about cloud structures from multidirectional measurements in the oxygen A band: middle-of-cloud pressure and cloud geometrical thickness from POLDER-3/PARASOL. J. Appl. Meteorol. Climatol. 49, 2492–2507. doi: 10.1175/2010JAMC2550.1
Flowerdew, R. J., and Haigh, J. D. (1995). An approximation to improve accuracy in the derivation of surface reflectances from multi-look satellite radiometers. Geophys. Res. Lett. 22, 1693–1696. doi: 10.1029/95GL01662
Flowerdew, R. J., and Haigh, J. D. (1996). Retrieval of aerosol optical thickness over land using the ATSR-2 Dual-Look Satellite Radiometer. Geophys. Res. Lett. 23, 351–354. doi: 10.1029/96GL00153
Fougnie, B., Marbach, T., Lacan, A., Lang, R., Schlüssel, P., Poli, G., et al. (2018). The multi-viewing multi-channel multi-polarisation imager–overview of the 3MI polarimetric mission for aerosol and cloud characterization. J. Quant. Spectrosc. Radiat. Trans. 219, 23–32. doi: 10.1016/j.jqsrt.2018.07.008
Fournier, G. R., and Forand, J. L. (1994). “Analytic phase function for ocean water,” in Ocean Optics XII, Vol. 2258 (Bergen: International Society for Optics and Photonics), 194–202. doi: 10.1117/12.190063
Frouin, R., Schwindling, M., and Deschamps, P. Y. (1996). Spectral reflectance of sea foam in the visible and near-infrared: in situ measurements and remote sensing implications. J. Geophys. Res. C6, 14361–14371. doi: 10.1029/96JC00629
Frouin, R. J., Franz, B. A., Ibrahim, A., Knobelspiesse, K., Ahmad, Z., Cairns, B., et al. (2019). Atmospheric correction of satellite ocean-color imagery during the PACE era. Front. Earth Sci. doi: 10.3389/feart.2019.00145
Fu, G., and Hasekamp, O. (2018). Retrieval of aerosol microphysical and optical properties over land using a multimode approach. Atmos. Meas. Tech. 11, 6627–6650. doi: 10.5194/amt-11-6627-2018
Gao, M., Zhai, P., Franz, B., Hu, Y., Knobelspiesse, K., Werdell, P. J., et al. (2018). Retrieval of aerosol properties and water-leaving reflectance from multi-angular polarimetric measurements over coastal waters. Opt. Express 26, 8968–8989. doi: 10.1364/OE.26.008968
Gao, M., Zhai, P., Franz, B., Hu, Y., Knobelspiesse, K., Werdell, P. J., et al. (2019). Inversion of multi-angular polarimetric measurements over open and coastal ocean waters: a joint retrieval algorithm for aerosol and water leaving radiance properties. Atmos. Meas. Tech. Discuss. doi: 10.5194/amt-2019-67. [Epub ahead of print].
Gordon, H. R. (1997). Atmospheric correction of ocean color imagery in the Earth observing era. J. Geophys. Res. 102, 17081–17106. doi: 10.1029/96JD02443
Gordon, H. R., and Wang, M. (1994). Retrieval of water-leaving radiance and aerosol optical thickness over the oceans with SeaWiFS: a preliminary algorithm. Appl. Opt. 33, 443–452. doi: 10.1364/AO.33.000443
Grey, W. M., North, P. R., Los, S. O., and Mitchell, R. M. (2006). Aerosol optical depth and land surface reflectance from multiangle AATSR measurements: global validation and intersensor comparisons. IEEE Trans. Geosci. Remote Sens. 44, 2184–2197. doi: 10.1109/TGRS.2006.872079
Harmel, T., and Chami, M. (2008). Invariance of polarized reflectance measured at the top of atmosphere by PARASOL satellite instrument in the visible range with marine constituents in open ocean waters. Opt. Express 16, 6064−6080. doi: 10.1364/OE.16.006064
Harmel, T., and Chami, M. (2013). Estimation of the sunglint radiance field from optical satellite imagery over open ocean: multidirectional approach and polarization aspects. J. Geophys. Res. 118, 76–90. doi: 10.1029/2012JC008221
Harten, G. V., Diner, D. J., Daugherty, B. J., Rheingans, B. E., Bull, M. A., Seidel, F. C., et al. (2018). Calibration and validation of Airborne Multiangle SpectroPolarimetric Imager (AirMSPI) polarization measurements. Appl. Opt. 57, 4499–4513. doi: 10.1364/AO.57.004499
Hasekamp, O. P. (2010). Capability of multi-viewing-angle photo-polarimetric measurements for the simultaneous retrieval of aerosol and cloud properties. Atmos. Meas. Tech. 3, 839–851. doi: 10.5194/amt-3-839-2010
Hasekamp, O. P., Fu, G., Rusli, S. P., Wu, L., Di Noia, A., and de Brugh, J., et al. (2019). Aerosol measurements by SPEXone on the NASA PACE mission: expected retrieval capabilities. J. Quant. Spectrosc. Radiat. Transf. 227, 170–184. doi: 10.1016/j.jqsrt.2019.02.006
Hasekamp, O. P., and Landgraf, J. (2005). Retrieval of aerosol properties over the ocean from multispectral single-viewing-angle measurements of intensity and polarization: retrieval approach, information content, and sensitivity study. J. Geophys. Res. 110:D20207. doi: 10.1029/2005JD006212
Hasekamp, O. P., Litvinov, P., and Butz, A. (2011). Aerosol properties over the ocean from PARASOL multiangle photopolarimetric measurements. J. Geophys. Res. 116:D14204. doi: 10.1029/2010JD015469
Holben, B. N., Eck, T. F., Slutsker, I., Tanre, D., Buis, J. P., Setzer, A., et al. (1998). AERONET—A federated instrument network and data archive for aerosol characterization. Remote Sens. Environ. 66, 1–16. doi: 10.1016/S0034-4257(98)00031-5
Holzer-Popp, T., Schroedter, M., and Gesell, G. (2002). Retrieving aerosol optical depth and type in the boundary layer over land and ocean from simultaneous GOME spectrometer and ATSR-2 radiometer measurements, 1, Method description. J. Geophys. Res. 107, AAC16-1–AAC16-17. doi: 10.1029/2001JD002013
Jethva, H., Torres, O., Remer, L., and Bhartia, P. (2013). A color ratio method for simultaneous retrieval of aerosol and cloud optical thickness of above-cloud absorbing aerosols from passive sensors: application to MODIS measurements. IEEE Trans. Geosci. Remote Sens. 51, 3862–3870. doi: 10.1109/TGRS.2012.2230008
Jethva, H., Torres, O., Remer, L., Redemann, J., Livingston, J., Dunagan, S., et al. (2016). Validating MODIS above-cloud aerosol optical depth retrieved from “color ratio” algorithm using direct measurements made by NASA's airborne AATS and 4STAR sensors. Atmos. Meas. Tech. 9, 5053–5062. doi: 10.5194/amt-9-5053-2016
Jethva, H., Torres, O., Waquet, F., Chand, D., and Hu, Y. (2014). How do A-train sensors intercompare in the retrieval of above-cloud aerosol optical depth? A case study-based assessment. Geophys. Res. Lett. 41, 186–192. doi: 10.1002/2013GL058405
Kahn, R. A., Sayer, A. M., Ahmad, Z., and Franz, B. A. (2016). The sensitivity of SEAWIFS ocean color retrievals to aerosol amount and type. J. Atmos. Ocean. Technol. 33, 1185–1209. doi: 10.1175/JTECH-D-15-0121.1
Kalashnikova, O. V., Garay, M. J., Bates, K. H., Kenseth, C. M., Kong, W., Cappa, C. D., et al. (2018). Photo-polarimetric sensitivity to black carbon content of wildfire smoke: results from the 2016 IMPACT-PM field campaign. J. Geophys. Res. 123, 5376–5396. doi: 10.1029/2017JD028032
Kalashnikova, O. V., Garay, M. J., Martonchik, J. V., and Diner, D. J. (2013). MISR Dark Water aerosol retrievals: operational algorithm sensitivity to particle non-sphericity. Atmos. Meas. Tech. 6, 2131–2154. doi: 10.5194/amt-6-2131-2013
Kalashnikova, O. V., and Kahn, R. (2006). Ability of multiangle remote sensing observations to identify and distinguish mineral dust types: 2. Sensitivity over dark water. J. Geophys. Res. 111:D11207. doi: 10.1029/2005JD006756
Kalashnikova, O. V., and Kahn, R. A. (2008). Mineral dust plume evolution over the Atlantic from combined MISR/MODIS aerosol retrievals. J. Geophys. Res. 113:D24204. doi: 10.1029/2008JD010083
Kaufman, Y. J., Martins, J. V., Remer, L. A., Schoeberl, M. R., and Yamasoe, M. A. (2002). Satellite retrieval of aerosol absorption over the oceans using sunglint. Geophys. Res. Lett. 29:1928. doi: 10.1029/2002GL015403
Knobelspiesse, K., Cairns, B., Mishchenko, M., Chowdhary, J., Tsigaridis, K., van Diedenhoven, B., et al. (2012). Analysis of fine-mode aerosol retrieval capabilities by different passive remote sensing instrument designs. Opt. Express 20, 21457–21484. doi: 10.1364/OE.20.021457
Knobelspiesse, K., Cairns, B., Redemann, J., Bergstrom, R. W., and Stohl, A. (2011). Simultaneous retrieval of aerosol and cloud properties during the MILAGRO field campaign. Atmos. Chem. Phys. 11, 6245–6263. doi: 10.5194/acp-11-6245-2011
Knobelspiesse, K., Tan, Q., Bruegge, C., Cairns, B., Chowdhary, J., van Diedenhoven, B., et al. (2019). Intercomparison of airborne multi-angle polarimeter observations from the Polarimeter Definition Experiment (PODEX). Appl. Opt. 58, 650–669. doi: 10.1364/AO.58.000650
Koepke, P. (1984). Effective reflectance of oceanic whitecaps. Appl. Opt. 23,1816–1824. doi: 10.1364/AO.23.001816
Kokhanovsky, A. A., Curier, R. L., De Leeuw, G., Grey, W. M. F., Lee, K.-H., Bennouna, Y., et al. (2009). The inter-comparison of AATSR dual-view aerosol optical thickness retrievals with results from various algorithms and instruments. Int. J. Remote Sens. 30, 4525–4537. doi: 10.1080/01431160802578012
Kokhanovsky, A. A., Davis, A. B., Cairns, B., Dubovik, O., Hasekamp, O., Sano, I., et al. (2015). Space-based remote sensing of atmospheric aerosols: the multi-angle spectro-polarimetric frontier. Earth Sci. Rev. 145, 85–116. doi: 10.1016/j.earscirev.2015.01.012
Kolmonen, P., Sogacheva, L., Virtanen, T. H., de Leeuw, G., and Kulmala, M. (2016). The ADV/ASV AATSR aerosol retrieval algorithm: current status and presentation of a full-mission AOD dataset. Int. J. Digital Earth 9, 545–561. doi: 10.1080/17538947.2015.1111450
Koven, C. D., and Fung, I. (2006). Inferring dust composition from wavelength-dependent absorption in Aerosol Robotic Network (AERONET) data. J. Geophys. Res. 111:D14205 doi: 10.1029/2005JD006678
Lacagnina, C., Hasekamp, O. P., Bian, H., Curci, G., Myhre, G., Van Noije, T., et al. (2015). Aerosol single-scattering albedo over the global oceans: comparing PARASOL retrievals with AERONET, OMI, and AeroCom models estimates. J. Geophys. Res. Atmos. 120, 9814–9836. doi: 10.1002/2015JD023501
Lacagnina, C., Hasekamp, O. P., and Torres, O. (2017). Direct radiative effect of aerosols based on PARASOL and OMI satellite observations. J. Geophys. Res. Atmos. 122, 2366–2388. doi: 10.1002/2016JD025706
Levis, A., Aides, A., Schechner, Y. Y., and Davis, A. B. (2015). “Airborne three-dimensional cloud tomography,” in Proceedings of the IEEE International Conference on Computer Vision (Santiago Chile), 3379–3387. doi: 10.1109/ICCV.2015.386
Li, R.-R., Kaufman, Y. J., Gao, B.-C., and Davis, C. O. (2003). Remote sensing of suspended sediments and shallow coastal waters. IEEE Trans. Geosci. Remote Sens. 41, 559–566. doi: 10.1109/TGRS.2003.810227
Limbacher, J. A., and Kahn, R. A. (2017). Updated MISR dark water research aerosol retrieval algorithm – Part 1: coupled 1.1 km ocean surface chlorophyll a retrievals with empirical calibration corrections. Atmos. Meas. Tech.10, 1539–1555. doi: 10.5194/amt-10-1539-2017
Loisel, H., Duforet, L., Dessailly, D., Chami, M., and Dubuisson, P. (2008). Investigation of the variations in the water leaving polarized reflectance from the POLDER satellite data over two biogeochemical contrasted oceanic areas. Opt. Express 16, 12905–12918. doi: 10.1364/OE.16.012905
Manolis, I., Bézy, J.-L., Meynart, R., Porciani, M., Loiselet, M., Mason, G., et al. (2017). “The 3MI instrument on the Metop second generation,” in Proceedings of SPIE 10563, International Conference on Space Optics — ICSO 2014 (Tenerife).
Martin, W., Cairns, B., and Bal, G. (2014). Adjoint methods for adjusting three-dimensional atmosphere and surface properties to fit multi-angle/multi-pixel polarimetric measurements. J. Quant. Spectrosc. Radiat. Trans. 144, 68–85. doi: 10.1016/j.jqsrt.2014.03.030
Martin, W. G., and Hasekamp, O. P. (2018). A demonstration of adjoint methods for multi-dimensional remote sensing of the atmosphere and surface. J. Quant. Spectrosc. Radiat. Trans. 204, 215–231. doi: 10.1016/j.jqsrt.2017.09.031
Martonchik, J. V., and Diner, D. J. (1992). Retrieval of Aerosol optical properties from multi-angle satellite imagery. IEEE Trans. Geosci. Remote Sens. 30, 223–230. doi: 10.1109/36.134073
Martonchik, J. V., Diner, D. J., Crean, K. A., and Bull, M. A. (2002). Regional aerosol retrieval results from MISR. IEEE Trans. Geosci. Remote Sens. 40, 1520–1531. doi: 10.1109/TGRS.2002.801142
McGill, M., Hlavka, D., Hart, W., Scoutt, V. S., Spinhirne, J., and Schmid, B. (2002). Cloud physics lidar: instrument description and initial measurement results. Appl. Opt. 41, 3725–3734. doi: 10.1364/AO.41.003725
Meyer, K. G., and Platnick, S. E. (2015). Simultaneously inferring above-cloud absorbing aerosol optical thickness and underlying liquid phase cloud optical and microphysical properties using MODIS. J. Geophys. Res. Atmos. 120, 5524–5547. doi: 10.1002/2015JD023128
Mishchenko, M. I., Cairns, B., Kopp, G., Schueler, C. F., Fafaul, B. A., Hansen, J. E., et al. (2007). Accurate monitoring of terrestrial aerosols and total solar irradiance: introducing the Glory mission. Bull. Amer. Meteorol. Soc. 88, 677–691. doi: 10.1175/BAMS-88-5-677
Mobley, C. D., Stramski, D., Bissett, W. P., and Boss, E. (2004). Optical modeling of ocean waters: is the case 1 - case 2 classification still useful? Oceanography 17, 60–67. doi: 10.5670/oceanog.2004.48
Mobley, C. D., Sundman, L. K., and Boss, E. (2002). Phase function effects on oceanic light fields. Appl. Opt. 41, 1035–1050. doi: 10.1364/AO.41.001035
Morel, A., Gentili, B., Claustre, H., Babin, M., Bricaud, A., Ras, J., et al. (2007). Optical properties of the “clearest” natural waters. Limnol. Oceanogr. 52, 217–229. doi: 10.4319/lo.2007.52.1.0217
Morel, M., and Maritorena, S. (2001). Bio-optical properties of oceanic waters: a reappraisal. J. Geophys. Res. 106, 7163–7180. doi: 10.1029/2000JC000319
NASA (2012). PACE Science Definition Team Report. Available online at: https://pace.oceansciences.org/docs/pace_sdt_report_final.pdf
Nelson, N. B., and Siegel, D. A. (2013). The global distribution and dynamics of chromophoric dissolved organic matter. Annu. Rev. Mar. Sci. 5, 447–476. doi: 10.1146/annurev-marine-120710-100751
Ottaviani, M., Knobelspiesse, K., Cairns, B., and Mishchenko, M. (2013). Information content of aerosol retrievals in the sunglint region. Geophys. Res. Lett. 40, 631–634. doi: 10.1002/grl.50148
Peers, F., Waquet, F., Cornet, C., Dubuisson, P., Ducos, F., Goloub, P., et al. (2015). Absorption of aerosols above clouds from POLDER/PARASOL measurements and estimation of their direct radiative effect. Atmos. Chem. Phys. 15, 4179–4196. doi: 10.5194/acp-15-4179-2015
Platnick, S., Coddington, O., Ackerman, S. A., Frey, R., Heidinger, A., Walter, A., et al. (2018). Cloud Retrievals in the PACE Mission: PACE Science Team Consensus Document. PACE Technical Report Series, Volume 4, NASA/TM-2018-219027. Available online at: https://pace.oceansciences.org/docs/TM2018219027Vol.4.pdf
Pope, R. M., and Fry, E. S. (1997). Absorption spectrum (380–700 nm) of pure water. II Integrating measurements. Appl. Opt. 36, 8710–8723. doi: 10.1364/AO.36.008710
Remer, L. A., Davis, A. B., Mattoo, S., Levy, R. C., Kalashnikova, O. V., Chowdhary, J., et al. (2019). Retrieving aerosol characteristics from the PACE mission, Part 1: Ocean Color Instrument. Front. Earth Sci. doi: 10.3389/feart.2019.00152
Rodgers, C. D. (2000). Inverse Methods for Atmospheric Sounding: Theory and Practice, Vol. 2. Singapore: World Scientific. doi: 10.1142/9789812813718
Russell, P. B., Bergstrom, R. W., Shinozuka, Y., Clarke, A. D., DeCarlo, P. F., Jimenez, J. L., et al. (2010). Absorption Ångstrom Exponent in AERONET and related data as an indicator of aerosol composition. Atmos. Chem. Phys. 10, 1155–1169. doi: 10.5194/acp-10-1155-2010
Russell, P. B., Kacenelenbogen, M., Livingston, J. M., Hasekamp, O. P., Burton, S. P., Schuster, G. L., et al. (2014). A multiparameter aerosol classification method and its application to retrievals from spaceborne polarimetry. J. Geophys. Res. 119, 9838–9863. doi: 10.1002/2013JD021411
Ryerson, T. B., Andrews, A. E., Angevine, W. M., Bates, T. S., Brock, C. A., Cairns, B., et al. (2013). The 2010 California research at the nexus of air quality and climate change (CalNex) field study. J. Geophys. Res. 118, 5830–5866. doi: 10.1002/jgrd.50331
Sayer, A. M., Hsu, N. C., Bettenhausen, C., Lee, J., Redemann, J., Schmid, B., et al. (2016). Extending “Deep Blue” aerosol retrieval coverage to cases of absorbing aerosols above clouds: sensitivity analysis and first case studies. J. Geophys. Res. 121, 4830–4854. doi: 10.1002/2015JD024729
Smirnov, A., Holben, B. N., Dubovik, O., Frouin, R., Eck, T. F., and Slutsker, I. (2003). Maritime component in aerosol optical models derived from Aerosol Robotic Network data. J. Geophys. Res. 108, AAC14-1–AAC14-11. doi: 10.1029/2002JD002701
Smirnov, A., Holben, B. N., Slutsker, I., Giles, D. M., McClain, C. R., Eck, T. F., et al. (2009). Maritime aerosol network as a component of aerosol robotic network. J. Geophys. Res. 114:D06204. doi: 10.1029/2008JD011257
Smith, R. C., and Baker, K. S. (1981). Optical properties of the clearest natural waters (200-800 nm). Appl. Opt. 20, 177–184. doi: 10.1364/AO.20.000177
Snik, F., Karalidi, T., and Keller, C. (2009). Spectral modulation for full linear polarimetry. Appl. Opt. 48, 1337–1346. doi: 10.1364/AO.48.001337
Stamnes, S., Hostetler, C., Ferrare, R., Burton, S., Liu, X., Wasilewski, A., et al. (2018). Simultaneous polarimeter retrievals of microsphysical aerosol and ocean color parameters with comparison to high spectral resolution lidar atmosphere and ocean products: the MAPP algorithm. Appl. Opt. 57, 2394–2413. doi: 10.1364/AO.57.002394
Stap, F. A., Hasekamp, O. P., Emde, C., and Roeckmann, T. (2016). Influence of 3D effects on 1D aerosol retrievals in synthetic, partially clouded scenes. J. Quant. Spectrosc. Radiat. Trans. 170, 54–68. doi: 10.1016/j.jqsrt.2015.10.008
Stap, F. A., Hasekamp, O. P., and Röckmann, T. (2015). Sensitivity of PARASOL multi-angle photopolarimetric aerosol retrievals to cloud contamination. Atmos. Meas. Tech. 8, 1287–1301. doi: 10.5194/amt-8-1287-2015
Tanré, D., Bréon, F. M., Deuzé, J. L., Dubovik, O., Ducos, F., François, P., et al. (2011). Remote sensing of aerosols by using polarized, directional and spectral measurements within the A-Train: the PARASOL mission. Atmos. Meas. Tech. 4, 1383–1395. doi: 10.5194/amt-4-1383-2011
Tanré, D., Herman, M., and Kaufman, Y. J. (1996). Information on aerosol size distribution contained in solar reflected spectral radiances. J. Geophys. Res. 101, 19043–19060. doi: 10.1029/96JD00333
Tanré, D., Kaufman, Y. J., Herman, M., and Mattoo, S. (1997). Remote sensing of aerosol properties over oceans using the MODIS/EOS spectral radiances. J. Geophys. Res. 102, 16971–16988. doi: 10.1029/96JD03437
Torres, O., Jethva, H., and Bhartia, P. K. (2012). Retrieval of aerosol optical depth above clouds from OMI observations: sensitivity analysis and case studies. J. Atmos. Sci. 69, 1037–1053. doi: 10.1175/JAS-D-11-0130.1
Veefkind, J. P., de Leeuw, G., and Durkee, P. A. (1998). Retrieval of aerosol optical depth over land using two-angle view satellite radiometry during TARFOX. Geophys. Res. Lett. 25, 3135–3138. doi: 10.1029/98GL02264
Voss, K. J. (1992). A spectral model of the beam attenuation coefficient in the ocean and coastal areas. Limnol. Oceanogr. 37, 501–509. doi: 10.4319/lo.1992.37.3.0501
Voss, K. J., and Fry, E. S. (1984). Measurement of the Mueller matrix for ocean water. Appl. Opt. 23, 4427–4439. doi: 10.1364/AO.23.004427
Wang, M., and Gordon, H. R. (1994). Estimating aerosol optical properties over the oceans with the multiangle imaging sprectroradiometer: some preliminary studies. Appl. Opt. 33, 4042–4057. doi: 10.1364/AO.33.004042
Waquet, F., Cairns, B., Knobelspiesse, K., Chowdhary, J., Travis, L. D., Schmid, B., et al. (2009a). Polarimetric remote sensing of aerosols over land. J. Geophys. Res. 114:D01206. doi: 10.1029/2008JD010619
Waquet, F., Peers, F., Ducos, F., Goloub, P., Platnick, S., Riedi, J., et al. (2013). Global analysis of aerosol properties above clouds. Geophys. Res. Lett. 40, 5809–5814. doi: 10.1002/2013GL057482
Waquet, F., Riedi, J., Labonnote, L. C., Goloub, P., Cairns, B., and Tanre, J. L.' (2009b). Aerosol remote sensing over clouds using A-Train observations. J. Atmos. Sci. 66, 2468–2480. doi: 10.1175/2009JAS3026.1
Wei, J., Lee, Z., Ondrusek, M., Mannino, A., Tzortziou, M., and Armstrong, R. (2016). Spectral slopes of the absorption coefficient of colored dissolved and detrital material inverted from UV-visible remote sensing reflectance. J. Geophys. Res. Oceans 121, 1953–1969. doi: 10.1002/2015JC011415
Werdell, P. J., Franz, B. A., Bailey, S. W., Feldman, G. C., Boss, E., Brando, V. E., et al. (2013). Generalized ocean color inversion model for retrieving marine inherent optical properties. Appl. Opt. 52, 2019–2037. doi: 10.1364/AO.52.002019
Wu, L., Hasekamp, O., van Diedenhoven, B., and Cairns, B. (2015). Aerosol retrieval from multiangle, multispectral photopolarimetric measurements: importance of spectral range and angular resolution. Atmos. Meas. Tech. 8, 2625–2638. doi: 10.5194/amt-8-2625-2015
Wu, L., Hasekamp, O., van Diedenhoven, B., Cairns, B., Yorks, J. E., and Chowdhary, J. (2016). Passive remote sensing of aerosol layer height using near-UV multi-angle polarization measurements. Geophys. Res. Lett. 43, 8783–8790. doi: 10.1002/2016GL069848
Xu, F., Dubovik, O., Zhai, P.-W., Diner, D. J., Kalashnikova, O. V., Seidel, F. C., et al. (2016). Joint retrieval of aerosol and water-leaving radiance from multispectral, multiangular and polarimetric measurements over ocean. Atmos. Meas. Tech. 9, 2877–2907. doi: 10.5194/amt-9-2877-2016
Xu, F., van Harten, G., Diner, D. J., Davis, A. B., Seidel, F., Rheingans, B., et al. (2018). Coupled retrieval of liquid water cloud and aerosol above cloud properties using the Airborne Multiangle SpectroPolarimetric Imager (AirMSPI). J. Geophys. Res. Atmos. 123, 3175–3204. doi: 10.1002/2017JD027926
Xu, F., van Harten, G., Diner, D. J., Kalashnikova, O. V., Seidel, F. C., Bruegge, C. J., et al. (2017). Coupled retrieval of aerosol properties and land surface reflection using the Airborne Multiangle SpectroPolarimetric Imager. J. Geophys. Res. Atmos. 122, 7004–7026. doi: 10.1002/2017JD026776
Xu, X. G., Wang, J., Wang, Y., Zeng, J., Torres, O., Yang, Y. K., et al. (2017). Passive remote sensing of altitude and optical depth of dust plumes using the oxygen A and B bands: first results from EPIC/DSCOVR at Lagrange-1 point. Geophys. Res. Lett. 44, 7544–7554. doi: 10.1002/2017GL073939
Zhai, P., Hu, Y., Trepte, C. R., and Lucker, P. L. (2009). A vector radiative transfer model for coupled atmosphere and ocean systems based on successive order of scattering method. Opt. Express 17, 2057–2079. doi: 10.1364/OE.17.002057
Zhai, P.-W., Hu, Y., Chowdhary, J., Trepte, C. R., Lucker, P. L., and Josset, D. B. (2010). A vector radiative transfer model for coupled atmosphere and ocean systems with a rough interface. J. Quant. Spectrosc. Radiat. Trans. 111, 1025–1040. doi: 10.1016/j.jqsrt.2009.12.005
Zhai, P.-W., Hu, Y., Winker, D. M., Franz, B., and Boss, E. (2015). Contribution of Raman scattering to polarized radiation field in ocean waters. Opt. Express 23, 23582–23596. doi: 10.1364/OE.23.023582
Zhai, P.-W., Hu, Y., Winker, D. M., Franz, B., Werdell, J., and Boss, E. (2017a). A vector radiative transfer model for coupled atmosphere and ocean systems including inelastic sources in ocean waters. Opt. Express 25, A223–A239. doi: 10.1364/OE.25.00A223
Zhai, P. W., Knobelspiesse, K., Ibrahim, A., Franz, B. A., Hu, Y., Gao, M., et al. (2017b). Water-leaving contribution to polarized radiation field over ocean. Opt. Express 25, A689–A708. doi: 10.1364/OE.25.00A689
Keywords: aerosol, multi-angle, polarimeter, PACE, remote sensing, multi-wavelength
Citation: Remer LA, Knobelspiesse K, Zhai P-W, Xu F, Kalashnikova OV, Chowdhary J, Hasekamp O, Dubovik O, Wu L, Ahmad Z, Boss E, Cairns B, Coddington O, Davis AB, Dierssen HM, Diner DJ, Franz B, Frouin R, Gao B-C, Ibrahim A, Levy RC, Martins JV, Omar AH and Torres O (2019) Retrieving Aerosol Characteristics From the PACE Mission, Part 2: Multi-Angle and Polarimetry. Front. Environ. Sci. 7:94. doi: 10.3389/fenvs.2019.00094
Received: 30 November 2018; Accepted: 03 June 2019;
Published: 23 July 2019.
Edited by:
David Antoine, Curtin University, AustraliaReviewed by:
Fischer Juergen, Freie Universität Berlin, GermanyBertrand Fougnie, European Organisation for the Exploitation of Meteorological Satellites, Germany
Copyright © 2019 Remer, Knobelspiesse, Zhai, Xu, Kalashnikova, Chowdhary, Hasekamp, Dubovik, Wu, Ahmad, Boss, Cairns, Coddington, Davis, Dierssen, Diner, Franz, Frouin, Gao, Ibrahim, Levy, Martins, Omar and Torres. This is an open-access article distributed under the terms of the Creative Commons Attribution License (CC BY). The use, distribution or reproduction in other forums is permitted, provided the original author(s) and the copyright owner(s) are credited and that the original publication in this journal is cited, in accordance with accepted academic practice. No use, distribution or reproduction is permitted which does not comply with these terms.
*Correspondence: Lorraine A. Remer, cmVtZXJAdW1iYy5lZHU=