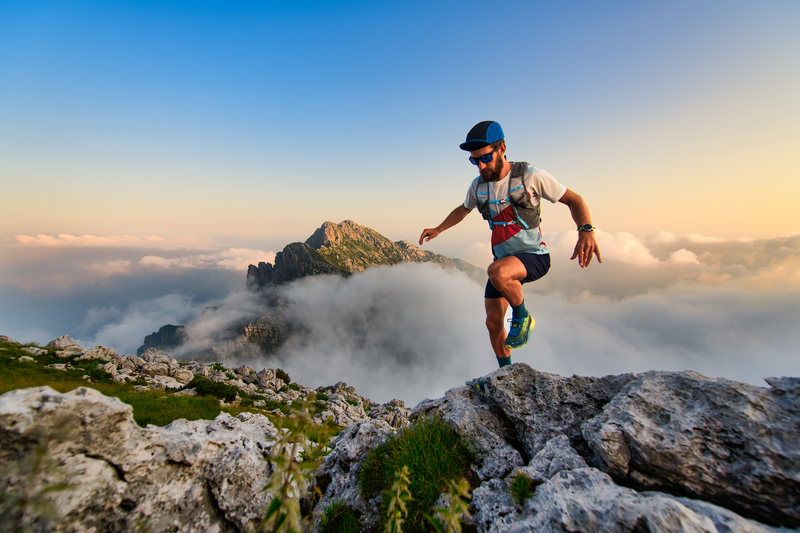
95% of researchers rate our articles as excellent or good
Learn more about the work of our research integrity team to safeguard the quality of each article we publish.
Find out more
ORIGINAL RESEARCH article
Front. Environ. Sci. , 17 May 2019
Sec. Soil Processes
Volume 7 - 2019 | https://doi.org/10.3389/fenvs.2019.00058
This article is part of the Research Topic Assessment and Modeling of Soil Functions or Soil-Based Ecosystem Services: Theory and Applications to Practical Problems View all 12 articles
Agricultural soils provide society with several functions, one of which is primary productivity. This function is defined as the capacity of a soil to supply nutrients and water and to produce plant biomass for human use, providing food, feed, fiber, and fuel. For farmers, the productivity function delivers an economic basis and is a prerequisite for agricultural sustainability. Our study was designed to develop an agricultural primary productivity decision support model. To obtain a highly accurate decision support model that helps farmers and advisors to assess and manage the provision of the primary productivity soil function on their agricultural fields, we addressed the following specific objectives: (i) to construct a qualitative decision support model to assess the primary productivity soil function at the agricultural field level; (ii) to carry out verification, calibration, and sensitivity analysis of this model; and (iii) to validate the model based on empirical data. The result is a hierarchical qualitative model consisting of 25 input attributes describing soil properties, environmental conditions, cropping specifications, and management practices on each respective field. An extensive dataset from France containing data from 399 sites was used to calibrate and validate the model. The large amount of data enabled data mining to support model calibration. The accuracy of the decision support model prior to calibration supported by data mining was ~40%. The data mining approach improved the accuracy to 77%. The proposed methodology of combining decision modeling and data mining proved to be an important step forward. This iterative approach yielded an accurate, reliable, and useful decision support model for the assessment of the primary productivity soil function at the field level. This can assist farmers and advisors in selecting the most appropriate crop management practices. Embedding this decision support model in a set of complementary models for four adjacent soil functions, as endeavored in the H2020 LANDMARK project, will help take the integrated sustainability of arable cropping systems to a new level.
Soils play a unique role for agriculture and provide numerous functions to society, among them primary productivity (Schulte et al., 2014). The primary productivity function is the capacity of a soil to supply nutrients and water and to produce plant biomass for human use, providing food, feed, fiber, and fuel within natural or managed ecosystem boundaries. This function is the economic foundation for farmers and all connected sectors and is thereby directly linked to societal demands (Tóth et al., 2013; Schulte et al., 2014). The United Nations predict that, by 2050, global agricultural production must grow by 60% to feed the increasing world population (WWAP, 2015). At the same time, however, an estimated one quarter of all agricultural soils are degraded: their future potential for biomass production has decreased and will continue to decline without intervention (Conijn et al., 2013). Moreover, crops grown in short rotations or monoculture face yield declines compared to crops grown in more diverse crop rotations. This is most likely due to biotic factors, including increased plant pathogens, or abiotic factors, including agricultural management practices, both of which can reduce nutrient availability (Bennett et al., 2012; Mazzilli et al., 2016; Weiner, 2017). Soils that are not managed sustainably may lose their productivity function over the longer term (Mueller et al., 2010). More importantly, the function of agricultural soils goes beyond primary productivity to include water regulation and purification, carbon sequestration and climate regulation, provision of habitat, and soil biodiversity, as well as nutrient cycling (Mueller et al., 2010; Schulte et al., 2014; Techen and Helming, 2017). Societal demands for different soil functions pose further challenges because they involve different spatial and temporal scales (Valujeva et al., 2016), and different stakeholders have diverse demands (O'Sullivan et al., 2015). Farmers play a key role in managing agricultural soil resources, but it remains difficult to find simple tools to help them manage primary productivity, let alone simultaneously manage multiple soil functions. Therefore, sustainably managing agricultural soil resources continues to be a challenge.
Considering that primary productivity is a priority in the agricultural sector, several methods and models have been used to evaluate the productivity function of soils (e.g., Tóth et al., 2013). Mueller et al. (2010) reviewed such approaches with the aim of finding a universal strategy that could be used globally at various scales. The authors concluded that there was no common global method to assess productivity at the field level and recommended that evaluations like Muencheberg Soil Quality Rating (Mueller et al., 2007, 2012) and the Canadian Land Suitability Rating System (Bock et al., 2018) would be good basis for developing one. The target was scalability across different regions and scales in addition to integrability into existing or forthcoming evaluation frameworks (Mueller et al., 2010). Tóth et al. (2013) provided a European assessment of productivity based on available data for grasslands, croplands, and forests, showing general trends in productivity across Europe. That type of assessment, however, lacks accuracy when the need is to assess primary productivity at the field scale for farmers. Several models including DAISY (Abrahamsen and Hansen, 2000), DNDC (Gilhespy et al., 2014), EPIC (Balkovič et al., 2013), and STICS (Brisson et al., 1998) all delve deeper into the different aspects of productivity, alongside other factors such as water and nutrient dynamics. Although several detailed options are available, many evaluation tools and methods remain in the research sector and are not used in cooperation with the end-users, i.e., to advise farmers on the optimal management of their agricultural fields or to incorporate farmers' and advisors' knowledge into the evaluation tools (Rose et al., 2016). Mechanistic models—STICS (Brisson et al., 1998), CENTURY (Parton and Rasmussen, 1994), and DayCent (Parton et al., 1998)—often require many variables (Trajanov et al., 2015) that farmers rarely address. Recently, Thoumazeau et al. (2019) presented a tool consisting of a set of 12 in-field indicators to measure soil functions. That tool, however, omits measures for primary productivity and fails to take into account various management practices. Therefore, there is a demand for approaches with qualitative decision modeling in which the current or desired management practices of farmers or farm advisors can be incorporated into assessments and advice regarding production and other soil management-related targets. This would enable the main decision concept, i.e., primary productivity in the present case, to be broken down into smaller, less complex subconcept. Expert knowledge would be considered at all levels of the model (Mouron et al., 2013; Craheix et al., 2016; Bohanec et al., 2017a) and be reflected in the final outputs.
Machine learning is increasingly being used in order to utilize agricultural data to make evidence-based decisions. This includes important attributes that can be used to optimize predictions, such as on primary productivity. Machine learning has now been utilized (i) to predict single soil attributes or study what governs them (Hobley et al., 2015; Hobley and Wilson, 2016; Chang et al., 2017; Bondi et al., 2018), (ii) for continental or even global soil property predictions (Henderson et al., 2005; Hashimoto et al., 2017; Hengl et al., 2017), and (iii) to classify soils in digital soil mapping (McBratney et al., 2003; Heung et al., 2016). Trajanov et al. (2018) successfully used data mining to generate predictive models that identify the key factors governing primary productivity (r > 0.80). The increasing amount of earth observation data has also been applied to agricultural decision-making (Liakos et al., 2018). Such data have been used to guide water and fertilizer management for cropping systems (Vuolo et al., 2016) and, on a more regional level, to map crop rotations over time (Vuolo et al., 2018). Such data can also serve as a basis for more comprehensive qualitative decision support models that help develop simple tools to guide agricultural practices (Debeljak et al., in review1). Such tools can then be used together or separately by end-users including researchers, farmers, advisors, and regional agricultural governance personnel. This co-creation of a final decision support tool would support greater acceptance by farmers and advisors because it would be easier to use and more relevant to the end-users. This could be further enhanced through peer recommendations by farmers, who have already been testing the decision support tool. Finally, it would help develop a tool that is fit for use by advisory services (Kerselaers et al., 2015; Rose et al., 2016).
Our study was designed to develop a decision support model for agricultural primary productivity. This work was done in close cooperation with the development of decision support models for four other soil functions within the H2020 LANDMARK project (Debeljak et al., in review1; Delgado et al., submitted2, Van den Broek et al., in review3; Van Leeuwen et al., in review4). To obtain a highly accurate model that helps farmers and advisors assess and manage the primary productivity of their agricultural fields, we addressed the following specific objectives: (i) to construct a qualitative decision support model to assess the primary productivity at the agricultural field level; (ii) to carry out verification, calibration, and sensitivity analysis of the model; and (iii) to validate the model with independent empirical data. The goal is to develop a generic model for primary productivity that can be applied across different environmental zones (after conducting the required standard modeling procedures to operationalize it to the respective location and scale).
The primary productivity decision support model was built using Multi-Criteria Decision Analyses (MCDA), in particular DEX (Decision Expert) integrative methodology (Bohanec and Rajkovic, 1990; Bohanec et al., 2013; Bohanec, 2014, 2017b) for qualitative decision modeling. The principles of this methodology follow intuitive human decision-making, where the main decision problem (concept, in our case, being primary productivity) is broken down into smaller, less complex subproblems (subconcepts, in our case, being soil, environment, crop, and management).
This breakdown is represented in the form of a hierarchy, where the main concept (primary productivity) is at the top of the hierarchy and is related to lower-level attributes on which it depends. The attributes at the lowest level of the hierarchy are the basic attributes: the soil, environment, crop, and management parameters. The intermediate attributes represent aggregations of the lower-level attributes. Their values (suitable, neutral, unsuitable) are obtained using decision rules. Decision rules (further referred to as integration rules) are a tabular representation (integration table) of a mapping from lower-level attributes to higher-level ones. The qualitative modeling approach of the DEX methodology helps formalize the input values into discrete (finite) scales. Our case unifies the scales along all basic attributes in a set of three categorical values: “Low,” “Medium,” and “High.” Exceptions are attributes that play binary roles, represented with value scales consisting of two values: “Yes” and “No.”
A standard modeling procedure was applied to obtain a reliable decision support model. It consists first of verification, sensitivity analysis, and calibration in an iterative way, followed by validation (Jorgensen and Fath, 2011). Verification is a test of the internal operational logic and behavior of the model. Domain experts (soil scientists) helped design the theoretical scenarios used to experimentally compare the model results with the expected outcomes.
The goal of the sensitivity analysis was to reduce model complexity by distinguishing between those input attributes whose values have a significant impact on model behavior, and those attributes whose values have low or no impact. After which, redundant input attributes were eliminated. This was done based on weights, which are commonly used in decision analysis to estimate the importance of attributes. The weights define the contribution of a corresponding attribute to the final evaluation of the alternative. Because the attributes had different value scales (some attributes have more values than the other), the weights had to be normalized. This adjusted all scales to the same unit interval. We used global normalized weights, which considered the structure of the entire model and the relative importance of its part. The weight of the top-most attribute in the model was 100%, whereas the weight of the basic or intermediate attributes could be 0%.
Calibration was conducted as an attempt to find the best agreement between the computed and observed data by varying the selected parameters. Calibration is usually performed on selected sets of parameters, and the model outputs are compared with the measured values of the modeled variable. The parameter set that gives the best agreement between model output and measured values is chosen. Calibration was performed by modifying the integration rules. We determined the selection of integration rules whose variation could significantly improve model performance by data mining that helps find and understand new patterns and knowledge from data based on methods from statistical modeling or machine learning. We utilized machine learning methods to supervise learning, in particular methods for learning decision trees, i.e., classification trees (Breiman et al., 1984). Classification trees (in a predictive task) predict the value of a dependent/target attribute (in our case primary productivity) from the values of independent attributes (soil, environment, crop, and management parameters). The model's structure is hierarchical. Its nodes test (compare) the values of an attribute against a splitting criterion (given as constants). The edges branching off the nodes contain the outcomes of the test. The model's terminal nodes, termed leaves, contain the predictions. To predict the class of the target attribute of a new example, it is traversed down the tree. When it reaches a leaf, the class value in this leaf determines the class value of the given example (Witten et al., 2011). We selected classification trees as a proper model because of their interpretability and comprehensibility, as well as their stepwise approach in solving non-linear classification problems.
The decision support model for primary productivity was finally validated using a representative dataset from France containing 399 sites from Atlantic Central and Mediterranean North environmental zones across France (Metzger et al., 2005). This objective test showed how well the model output performs and fits the real data. The decision support model was validated by directly comparing the estimated values with those provided in the empirical data. The direct comparison was facilitated by discretizing the values of the dependent variable. The discretization was done similarly as for the other variables. However, the added weight of the validation step and the demand for an accurate validation process required defining accurate thresholds that reflected the statistical and expert distribution of the measured values. The thresholds of the dependent variable that expressed the primary productivity were defined in the context of a selected crop based on the differences in yields between different crops. The model validation was set up as a set of rules and defined as follows: an estimation of the primary productivity soil function was considered accurate if the estimated value or estimated most probable value (based on estimated probability distribution) was equal to the appropriate discrete value of the primary productivity of a selected site in the empirical dataset. Otherwise, the estimation was considered to be incorrect. The ratio between correct estimations and total estimations is taken as an accuracy measure for model performance.
The dataset used in this study is composed of attributes underlying a soil's capacity to produce plant biomass for human use within agricultural ecosystem boundaries, i.e., primary productivity. These attributes included soil properties (S), environmental aspects (E), crop (C), and management options (M) (Table 1), partly based on van Leeuwen et al. (2017). Soil and management data were collected within the French Soil Monitoring Network (RMQS) that was established to provide a national framework for observing changes in soil quality across France (Arrouays et al., 2011). This dataset covered a broad spectrum of climatic, soil, and agricultural conditions at all 399 sites. It consisted of a total of 2,200 soil samples extracted from the nodes of a 16-km grid that covered the French Metropolitan Territory. We extracted data from the topsoil samples (0–30 cm) from Atlantic Central and Mediterranean North environmental zones (Metzger et al., 2005) that were sampled as described previously by Martin et al. (2009). For environmental attributes, climatic data were obtained by interpolating observational data using the SAFRAN model (Quintana-Seguí et al., 2008). The RMQS site-specific data were linked to the climatic data by finding for each RMQS site the closest node within the 12 × 12 km2 climatic grid and then averaging for the 1990–2016 period. Altitude and slope information were derived from a digital elevation model (USGS, 2004). The crop attributes and management practices from the last 5 years, including the studied year at the sites where the soil was sampled, were collected by an agricultural survey with the farmers. Due to differences in management information from one site to another, the percentage of legumes and catch crops in the rotation was calculated over maximum 5 years or less, depending on the amount of available information. Three crops were used to validate the primary productivity model: winter wheat, rapeseed, and sunflower. This allowed the RMQS survey to cover 44% of sites on arable land.
Table 1. Primary productivity attributes that underwent discretization with corresponding units and scale values.
To build, calibrate, and validate the primary productivity decision support model, we pre-processed the original data. The main focus was on handling missing values and data cleansing (removing identifiers and correlated attributes).
Building and validating the DEX models requires the data to have qualitative values from a discrete scale of values (Table 1). All data were therefore discretized into values from a set of discrete values, using thresholds defined by domain experts (Figure 1). For certain attributes (e.g., soil organic matter, clay content, ground water table depth, and precipitation), different thresholds were defined for different environmental zones. The primary productivity in the soil monitoring data was expressed as a quantity (kg ha−1) and was also discretized into the values corresponding to the scale of “Low,” “Medium,” and “High” values, meaning low, medium, and high capacity of the primary productivity soil function. In order to define the scales, the observed crop yield of the soil sampling site of the year was compared with the statistics on the agricultural yields supplied by the French Ministry of Agriculture. The quantiles (10, 25, 50, 90%) on the population of the yearly departmental statistics were calculated in order to estimate how the observed yield at the soil sampling site rated with regard to the national distribution. The quantiles yielded a score between 0 and 20 for a year yield at the site as follows: 20 points if the yield was >90%, 15 points if the yield was between the median and 90%, and so forth. For the soil sampling sites where yields were measured for many years, we averaged notes over the years available. Then, the values were discretized to an average score as follows: Low = 0–10, Medium = 10–15, and High = 15–20. Thus, the more the observed yield is situated in the superior quantiles, the more positively the function was estimated.
Figure 1. The discretization of the dataset into values corresponding to “Low,” “Medium,” “High,” “Yes,” “No,” and “Stockless”.
The next step in the data pre-processing was handling missing values during the validation process. The DEX methodology (Bohanec and Rajkovic, 1990; Bohanec et al., 2013) supports missing values and handles them considering all possible values of the attribute that has missing values. This yields a set of values and their probabilities (rather than a single value) assigned to the main attribute—the primary productivity. Hence, the missing values were not removed from the dataset but assigned with a required sign understandable for DEX.
For the data mining analyses, the same original dataset was used. The values of the attributes were not discretized, except for the values of the primary productivity attribute, which were assessed by an independent expert, and took values from the scale “Low,” “Medium,” and “High” as described above.
The developed decision support model for primary productivity is structured in a hierarchical way to take into consideration soil (S), environment (E), crop (C), and management (M) attributes (Figure 2). It comprises 4 levels and has 25 basic attributes. The top of the hierarchy represents the capacity of the primary productivity function; the intermediate levels represent attributes that integrate lower level attributes down to the basic input attributes. These S × E × C × M interactions determine whether the capacity of a soil to produce biomass is “Low,” “Medium,” or “High.” The soil attributes consist of physical (e.g., clay content and bulk density) and chemical (e.g., macro-elements including phosphorus, potassium, and magnesium) attributes as well as attributes known to influence the biological activity of soils (soil organic matter, C/N ratio, soil pH). Environment is divided into attributes connected to orography (slope degree, altitude) and climate (temperature, precipitation). The crop consists of stocking rate as well as attributes linked to crop rotation (i.e., share of legumes, catch crops, cover crops, and green manure in the rotation, as well as the number of crops in rotation). Management attributes cover irrigation, pest management, and fertilization. Each attribute in the decision support model can have one out of three (or two) values (e.g., “High,” “Medium,” “Low,” or “yes,” “no”). Subsequently, values of a similar nature are assigned to the overarching process of each possible combination of two or three underlying attributes, until the ultimate function primary productivity (at the top) is reached.
Figure 2. The decision support model for primary productivity that is built up from basic attributes (gray boxes on right) via aggregated attributes (e.g., biological activity and soil) to the ultimate soil function—primary productivity.
Figure 3 shows the variability of importance of each attribute to the output (primary productivity). The first level in the hierarchy between the aggregated attributes soil, environment, crop, and management shows that these aggregated attributes each contribute 22, 30, 20, and 28%, respectively, to the overall primary productivity. This reflects similar distribution of importance (expressed as global normalized weights in Figure 3). This means that the inner variability of these structures contributes equally to the variability of the outcome. Nonetheless, examining the lower level of the hierarchy reveals that the water inflow (“Precipitation” and “Irrigation”), as well as orography (“Slope degree”) and fertilization (“Mineral nitrogen fertilization” and “Organic nitrogen fertilization”) greatly influence the variability of the primary productivity. In contrast, the least important individual attributes involve the structure of the soil properties, whereby physical properties dominate somewhat over chemical and biological ones.
Figure 3. Importance of attributes in the primary productivity model. Importance is expressed in percentage representing the contribution (ratio) of attribute's variability in outcome's variability. Hence, subconcepts (attributes at first level in the hierarchical structure) soil, environment, crop, and management contribute 22, 30, 20, and 28%, respectively, to the primary productivity value.
Once the structure of the decision model was built, we followed a standard modeling procedure to obtain a reliable decision support model ready to be used by agricultural advisors and farmers by iteratively applying verification, sensitivity analysis, and calibration. This was followed by model validation. The first model outputs showed need for further model structure modification that was done according to the knowledge and experience of the involved domain experts. Once the structure of the model was verified, sensitivity analysis was conducted. This procedure led to further structural changes and simplifications. The sensitivity analysis showed that we had to eliminate a small part describing micro-elements (not shown in the final model in Figure 2), because the global normalized weights of all three basic attributes (Fe, Mn, and Cu) were 0% and the global weight of their aggregated attribute (micro-elements) was only 1%. This reduced model complexity was verified, and the integration rules were modified accordingly.
The last step in the procedure was model calibration. To determine which integration rules were to be modified in order to calibrate the model to the French study area, we generated a data mining model in a form of classification tree to predict the capacity of the primary productivity soil function from the set of input attributes to the decision support model. The classification tree was generated using the French data described in the section Description of the Dataset and is presented in Figure 4. The accuracy of the data mining model was 77.7%, which was sufficiently reliable to calibrate the decision support model. The structure of this classification tree indicates that the most important initial attribute for the primary productivity at a field scale in our French dataset was the cation exchange capacity (CEC). Other important parameters were altitude and the available phosphorus (P) level in the soil. The integration tables incorporating these basic attributes were modified according to the attribute importance as they appeared in the classification tree. Accordingly, the integration rules originally defined by domain experts were modified and improved by the results of data mining modeling (see Appendix 1 for details on changes in integration rules).
The last step in developing the decision support model was its validation. This was performed before and after calibrating the decision support model, which was supported by the classification model from data mining that was based on the empirical data from the same sites that were used for validation. The performance of the final decision support model, combining expert knowledge and machine learning, was expressed by its accuracy in correctly estimating the level of production compared to the local domain experts' evaluation (Figure 5). The local domain experts based their evaluation on the yield data they had access to. These comparisons revealed that primary productivity was more often underestimated by the domain experts compared to the outcomes of our decision support model. Since the outcome was defined by the discrete scale of “Low,” “Medium,” and “High,” we examined model performance for each value separately, as well as its overall performance (Table 2). Calibration improved model performance to 83%, thus achieving overall accuracy of 77% compared to 42% before the calibration step. The primary productivity model performed best for the category of “High,” followed by “Medium” and “Low” (97, 71, and 63%, respectively).
Figure 5. Comparison between the estimated primary productivity as discretization of data by the domain experts (A, left) as low, medium, or high and the outcomes of the primary productivity decision support model (B, right) as low, medium, high, or combinations thereof.
Primary productivity is critical for the profitability and sustainability of agricultural systems; this makes it of pivotal importance that farmers plan for long-term maintenance of crop yields. The environment accounted for 30% of the important attributes underlying primary productivity in our decision support model (Figure 3). Other authors have also shown that orography (altitude and slope degree) and climate (precipitation and temperature) are among the main environmental factors that influence primary productivity (e.g., Mueller et al., 2010; Tóth et al., 2013). Primary productivity is often limited by climatic parameters such as drought, wetness, length of growing season, and irradiance (Fischer et al., 2002).
Management accounted for nearly 30% of a soil's primary productivity (Figure 3). The aim of management is to improve soil physical, chemical, and biological quality in order to overcome yield-limiting (e.g., soil moisture) and yield-reducing (e.g., pests) factors. In order to confirm a positive or negative effect of a management practice on primary productivity, long-term experiments can function as living laboratories (Johnston and Poulton, 2018; Sandén et al., 2018). Zavattaro et al. (2015) observed slight yield reductions following application of organic amendments, including farmyard manure and incorporation of crop residues, most likely due to N immobilization. The same authors also showed that, beyond management, the interplay between climate, soil type, and duration of management plays a role. Trajanov et al. (2018) showed that the crop grown and the compost amendment applied had major effects on primary productivity: higher yields were achieved when sufficient mineral or a combination of compost and mineral fertilization was applied compared to the application of compost amendments alone. Note, however, that independent from the chosen management practices, farm management options always have a site-specific component and should therefore ideally be tailored to as many local conditions (“supply”) and requirements (“demands”) as possible. Thus, practices showing benefits on one farm do not automatically result in similar benefits on a different farm. Accordingly, our decision support model often provides two or even three possible outcomes for a given location, as seen in Figure 5B. To decide which option should be selected, site-specific requirements need to be considered in the final decision-making process, as well as in the decision support tool to be developed (Stavi et al., 2016).
In assessing whether a field has suitable soil for primary productivity, our model further considers soil chemical and physical attributes as well as the attributes affecting biological activity. Soil properties accounted for about 20% of the total capacity to produce crops (Figure 3). CEC indicates the capacity of a soil to store nutrients and water—key aspects for supporting primary productivity. In our French dataset, a CEC (cobalt–hexamine method) up to 34 cmol kg−1 was shown to be optimal for primary productivity. This corresponds to rather high values when compared to national data (mean CEC 14 cmol kg−1, 90 percentile 30 cmol kg−1; Arrouays et al., 2011). According to Figure 4, estimated primary productivity was high when plant-available phosphorus contents were between 46 and 135 mg kg−1. Plant-available phosphorus contents are known to affect primary productivity (Sheil et al., 2016; Buczko et al., 2018; Trajanov et al., 2018). Furthermore, the classification tree confirms findings from Spiegel et al. (2001), who reported that very high yielding crops grown on soils with low plant available phosphorus concentrations are more likely to result in lower yields. Other factors known to limit the productivity function include shallow soils, stoniness, hardpan, anaerobic conditions, salinity, sodicity, acidity, nutrient depletion, and contamination (Mueller et al., 2010). Unfavorable soil structure can also negatively affect crop yields, for example, due to greater leaching losses (Kavdir and Smucker, 2005). Whether or not increased soil organic matter concentrations improve crop yields is still a subject of debate (e.g., Hijbeek et al., 2017), but it has been shown to greatly improve the soil biota (e.g., D'Hose et al., 2018).
The remaining 20% of our primary productivity model was affected by crop attributes (Figure 3). Zavattaro et al. (2015) observed that crop rotation and cover crops, in particular, had positive effects on crop yields, which is supported by our decision support model as well as by a recent study that recommended crop rotation as a promising management practice (Barão et al., 2019). Zavattaro et al. (2015) also observed that in more than 80% of the examined cases, the yield of a crop grown in a rotation practice was larger than that of a monoculture. According to their study, crop rotation worked well on sandy and loamy soils in western Europe, whereas clayey soils were less favorable for that system. Cover/catch crops had positive effects on the yields of the main crops in 60% of the cases, and it was of minor importance which cover/catch crop was grown (leguminous vs. non-leguminous) (Zavattaro et al., 2015). The positive effects of crop rotation and catch crops on primary productivity were confirmed by Sandén et al. (2018), who analyzed a total of 251 European long-term experiments. They reported an increase in yields of about 5% and 4% when crop rotation and catch crops were applied, respectively. Trajanov et al. (2018) also observed that the preceding crop had a large influence on crop yields in an Austrian long-term experiment: cereal yields were significantly lower when sugar beet or winter wheat (vs. soybean and spring wheat) preceded the crops.
Expert knowledge is a central element in developing decision support models (Uusitalo et al., 2015), and modelers therefore heavily rely on such expertise and competence. Nonetheless, several issues arise when solely relying on expert knowledge (Wieland and Mirschel, 2017). The first challenge is acquiring expert knowledge, representing it in a formalized way and making it accessible for further use in decision modeling (Shaw and Woodward, 1990). Other common challenges are that such knowledge may be biased and that there may be a discrepancy between the expert's innate cognitive abilities and the complexity of the reasoning tasks required for certain scientific problems (Tversky and Kahneman, 1974). In developing our model, we worked with a wide group of experts to come up with the first ideas for the model and also incorporated experts who were very familiar with the data used to calibrate and validate the model. This approach helped minimize these challenges and tapped into varied knowledge. A further bias may arise from the data itself (Figure 1). In the present case, the French dataset focused on crops (e.g., winter wheat) that are usually grown in intensively managed and productive locations with suitable soil conditions, and only few are grown in less favorable conditions (Figure 5).
Acquisition of expert knowledge can be a hurdle: reliable experts may be unavailable or may offer opposing opinions (Shaw and Woodward, 1990). Those authors identified an even bigger challenge: the inability to verify the different opinions of the selected experts. This can partly be solved by weighing the different responses, as by Rutgers et al. (2012). Machine learning is an alternative way of obtaining domain knowledge from empirical data (Trajanov et al., 2015, 2018; Idé, 2016; Bondi et al., 2018). Machine learning algorithms for rule and tree induction are a useful framework for extracting knowledge from data and representing it in a format that can be directly used in constructing decision support models. In our case, we combined expert knowledge with data mining, which was proven successful with another dataset (Trajanov et al., 2018).
One task is to overcome these biases in expert knowledge and to satisfy the need to rely on scientific evidence and high-quality data when developing complex decision support models. This is promoted by the interplay between machine learning and decision support (Chlingaryan et al., 2018), as underlined by our decision support model. Machine learning models can provide accurate predictions (such as the capacity of the primary productivity soil function) by considering empirical data (Cherkassky and Mulier, 2007; Trajanov et al., 2018). Reliable predictions are invaluable, but in many cases, decisions must be made about the best course of action (e.g., what management practice to choose in order to increase the capacity of the primary productivity soil function). This can be achieved by feeding the predictions generated by machine learning models into a decision support model, which then evaluates alternative actions and recommends the optimal decision (Tulabandhula and Rudin, 2014). Our model aims to serve as a generic model for primary productivity that can be used across different environmental zones alongside models for four other soil functions. This requires appropriate calibration, including application of data mining.
An ideal decision support model will enable farmers to optimize long-term primary productivity while simultaneously accounting for management effects on other important soil functions. Improved knowledge on the effects of other soil functions on primary productivity and vice versa can help farmers make decisions on how to more holistically and sustainably manage their soils. Giving due attention to modeling scale (local, regional, national, European) is important when using decision support models: it is not trivial to upscale and/or downscale soil functions and management practices across different spatial scales (Schulte et al., 2015; Valujeva et al., 2016). Note also that not all attributes that influence primary productivity are equally relevant or have the same level of influence at every scale. While the initial development of our primary productivity model was supported by a study that focused solely on long-term experimental data in Austria (Trajanov et al., 2018), those authors suggested that a more comprehensive dataset on a larger spatial scale could more comprehensively identify the important attributes influencing primary productivity. Taking France as a case study provided us with a harmonized dataset for this purpose. Our decision support model for primary productivity will underpin the Soil Navigator decision support tool developed within the LANDMARK Horizon 2020 project. The latter is designed to integrate the simultaneous assessment of five soil functions: primary productivity, nutrient cycling, climate regulation, water regulation and purification, and biodiversity (Debeljak et al., in review1). The Soil Navigator is based on the concept of Functional Land Management (Schulte et al., 2014, 2015), which aims to manage soils such that the supply and demand of soil functions is balanced across a landscape. The strategy is to optimize different soil functions spatially, identifying where they have the best opportunities to thrive and where they are needed to fulfill societal demands. Engaging farmers to consider the effects of management on different soil functions requires (i) helping them to identify and understand the various influencing soil (S), environment (E), crop (C), and management (M) attributes affecting their field, and (ii) supporting them and their advisors with appropriate decision support tools. When adopting management practices, farmers will consider a range of other factors including performance, usability, relevance, cost-effectiveness, and compatibility with compliance demands (Rose et al., 2016). Furthermore, including farmers and advisors in the co-design of decision support tools has been shown to improve targeting toward user needs and ease of use as well as to provide additional benefits to end-users (Allen et al., 2017; Oliver et al., 2017). Previous research investigating farmers' knowledge on soil functions across Europe and their demands for a decision support tool showed that not all farmers want the same kind of advice (Bampa et al., 2019). That study, in agreement with Mills et al. (2018), concluded that farmer's motivations need to be taken into account to increase environmental benefits through management of agricultural landscapes. Bampa et al. (2019) observed that farmers were generally highly interested in practical solutions and in access to high-quality information in conjunction with one-on-one personal communication with soil scientists, agronomists, and advisors. Nonetheless, farmers' needs concerning mobile apps for agricultural advice and other decision support tools differed greatly between countries and even between scales (local, regional, and national) within a country (Bampa et al., 2019). These findings support a call for interactive dialogue between different stakeholders and direct involvement of farmers and advisors in the design of decision support tools. This is the most promising route to enhance and build understanding between research and practice adopters (Ingram et al., 2016).
Our study generated a primary productivity decision model using expert knowledge and data mining that can be used by farmers and advisors at the field level. We carried out improved standard modeling procedures to obtain a reliable decision support model by applying verification, sensitivity analysis, and calibration in an iterative manner. We then validated the primary productivity model with an extensive French empirical dataset in order to increase its usability. The proposed methodology of combining decision modeling and data mining proved to be complementary and clearly improved model performance. This approach yielded an accurate, reliable, and useful decision support model to assess the primary productivity soil function at the field level. It can also be used to improve future management practices and to maintain the primary productivity function of soils. Importantly, this model will underpin the LANDMARK H2020 project Soil Navigator, together with four other soil function models.
This article resulted from cooperation within the primary productivity task group in the LANDMARK H2020 project. TS, AT, HS, VK, and MD were mainly responsible for the development of the model. TS and HS as domain experts, AT with data mining, VK with model validation, and MD as the main responsible modeler throughout the study. NS and CP were responsible for the French dataset and acted as additional domain experts during the model development. CH was mainly responsible for future prospects. TS did most of the writing, with major inputs from AT, VK, NS, and MD. All authors contributed to the manuscript revision and read and approved the submitted version.
The authors declare that the research was conducted in the absence of any commercial or financial relationships that could be construed as a potential conflict of interest.
This study was conducted as part of the LANDMARK (LAND Management: Assessment, Research, Knowledge Base) project. LANDMARK has received funding from the European Union's Horizon 2020 research and innovation programme under grant agreement no. 635201. RMQS soil sampling and physicochemical analyses were supported by the GIS Sol, which is a scientific interest group on soils involving the French Ministry for Ecology and Sustainable Development and the Ministry of Agriculture, the French National Forest Inventory (IFN), ADEME (Agence de l'environnement et de la maîtrise de l'énergie, which is a French government agency concerned with environmental protection and energy management), IRD (Institut de recherche pour le développement, which is a French public research organization dedicated to southern countries), and INRA (Institut national de la recherche agronomique, which is a French public research organization dedicated to agriculture s.l). Claudy Jolivet is thanked for his strong involvement in the RMQS monitoring network. Michael Schwarz (AGES, Austria) is acknowledged for creating Figure 5. The authors wish to thank Michiel Rutgers [The National Institute for Public Health and the Environment (RIVM), Netherlands]; David Wall (Teagasc, Ireland); Erika Micheli, Adan Csorda, and Tamas Szegi (Szent István University, Hungary); and Rachel Creamer and Francesca Bampa (Wageningen University, Netherlands) for proofreading the manuscript and providing valuable comments. Michael Stachowitsch and Mariana Debernardini are acknowledged for English proofreading.
The Supplementary Material for this article can be found online at: https://www.frontiersin.org/articles/10.3389/fenvs.2019.00058/full#supplementary-material
1. ^Debeljak et al. A field-scale decision support system for assessment and management of soil functions. In review in Frontiers in Environmental Science, this issue.
2. ^Delgado et al. Farming systems targeted to water regulation and purification in agricultural soils. Submitted to Frontiers in Environmental Science, this issue.
3. ^Van de Broek et al. Assessing the climate regulation potential of agricultural soils using a decision support tool adapted to stakeholders' needs and possibilities. In review in Frontiers in Environmental Science, this issue.
4. ^Van Leeuwen et al. Modelling of soil functions for assessing soil quality: Soil biodiversity and habitat provisioning. In review in Frontiers in Environmental Science, this issue.
Abrahamsen, P., and Hansen, S. (2000). Daisy: an open soil-crop-atmosphere system model. Environ. Modell. Softw. 15, 313–330. doi: 10.1016/S1364-8152(00)00003-7
Allen, W., Cruz, J., and Warburton, B. (2017). How decision support systems can benefit from a theory of change approach. Environ. Manag. 59, 956–965. doi: 10.1007/s00267-017-0839-y
Arrouays, D., Saby, N. P. A., Thioulouse, J., Jolivet, C., Boulonne, L., and Ratié, C. (2011). Large trends in French topsoil characteristics are revealed by spatially constrained multivariate analysis. Geoderma 161, 107–114. doi: 10.1016/j.geoderma.2010.12.002
Balkovič, J., van der Velde, M., Schmid, E., Skalský, R., Khabarov, N., Obersteiner, M., et al. (2013). Pan-European crop modelling with EPIC: implementation, up-scaling and regional crop yield validation. Agric. Syst. 120, 61–75. doi: 10.1016/j.agsy.2013.05.008
Bampa, F., O'Sullivan, L., Madena, K., Sandén, T., Spiegel, H., Henriksen, C. B., et al. (2019). Harvesting European knowledge on soil functions and land management using multi-criteria decision analysis. Soil Use Manage 35, 6–20. doi: 10.1111/sum.12506
Barão, L., Alaoui, A., Ferreira, C., Basch, G., Schwilch, G., Geissen, V., et al. (2019). Assessment of promising agricultural management practices. Sci. Total Environ. 649, 610–619. doi: 10.1016/j.scitotenv.2018.08.257
Bennett, A. J., Bending, G. D., Chandler, D., Hilton, S., and Mills, P. (2012). Meeting the demand for crop production: the challenge of yield decline in crops grown in short rotations. Biol. Rev. 87, 52–71. doi: 10.1111/j.1469-185X.2011.00184.x
Bock, M., Gasser, P.-Y., Pettapiece, W. W., Brierley, A. J., Bootsma, A., Schut, P., et al. (2018). The land suitability rating system is a spatial planning tool to assess crop suitability in Canada. Front. Environ. Sci. 6:77. doi: 10.3389/fenvs.2018.00077
Bohanec, M. (2014). “DEXi: Program for Multi-Attribute Decision Making, User's Manual, Version 4.01,” in IJS Report DP-11739 (Ljubljana: Jožef Stefan Institute).
Bohanec, M. (2017b). “Multi-criteria DEX models: an overview and analyses,” in The 14th International Symposium on Operational Research in Slovenia, eds L. Z. Stirn, M. K. Borštar, J. Žerovnik, and S. Drobne (Ljubljana: Slovenian Society Informatika - Section for Operational Research), 155–60.
Bohanec, M., Boshkoska, B. M., Prins, T. W., and Kok, E. J. (2017a). SIGMO: a decision support system for identification of genetically modified food or feed products. Food Control 71, 168–177. doi: 10.1016/j.foodcont.2016.06.032
Bohanec, M., and Rajkovic, V. (1990). DEX: an expert system shell for decision support. Sistemica 1, 145–157.
Bohanec, M., Žnidaršič, M., Rajkovič, V., Bratko, I., and Zupan, B. (2013). DEX methodology: three decades of qualitative multi-attribute modeling. Informatica 37, 49–54. Available online at: http://www.informatica.si/index.php/informatica/article/view/433
Bondi, G., Creamer, R., Ferrari, A., Fenton, O., and Wall, D. (2018). Using machine learning to predict soil bulk density on the basis of visual parameters: tools for in-field and post-field evaluation. Geoderma 318, 137–147. doi: 10.1016/j.geoderma.2017.11.035
Breiman, L., Friedman, J., Stone, C. J., and Olshen, R. A. (1984). Classification and Regression Trees. Taylor and Francis. Monterey: Wadsworth & Brooks.
Brisson, N., Mary, B., Ripoche, D., Jeuffroy, M. H., Ruget, F., Nicoullaud, B., et al. (1998). STICS: a generic model for the simulation of crops and their water and nitrogen balances. I. Theory and parameterization applied to wheat and corn. Agronomie 18, 311–346.
Buczko, U., van Laak, M., Eichler-Löbermann, B., Gans, W., Merbach, I., Panten, K., et al. (2018). Re-evaluation of the yield response to phosphorus fertilization based on meta-analyses of long-term field experiments. Ambio 47, 50–61. doi: 10.1007/s13280-017-0971-1
Chang, H.-X., Haudenshield, J. S., Bowen, C. R., and Hartman, G. L. (2017). Metagenome-wide association study and machine learning prediction of bulk soil microbiome and crop productivity. Front. Microbiol. 8:519. doi: 10.3389/fmicb.2017.00519
Cherkassky, V., and Mulier, F. M. (2007). Learning From Data: Concepts, Theory and Methods, 2nd Edn. New Jersey, JC: Wiley - IEEE Press.
Chlingaryan, A., Sukkarieh, S., and Whelan, B. (2018). Machine learning approaches for crop yield prediction and nitrogen status estimation in precision agriculture: a review. Comput. Electron. Agric. 151, 61–69. doi: 10.1016/j.compag.2018.05.012
Conijn, J. G., Bai, Z. G., Bindraban, P. S., and Rutgers, B. (2013). Global Changes of Net Primary Productivity, Affected by Climate and Abrupt Land Use Changes Since 1981. Towards mapping global soil degradation. Wageningen: ISRIC - World Soil Information.
Craheix, D., Angevin, F., Doré, T., and de Tourdonnet, S. (2016). Using a multicriteria assessment model to evaluate the sustainability of conservation agriculture at the cropping system level in France. Eur. J. Agron. 76, 75–86. doi: 10.1016/j.eja.2016.02.002
D'Hose, T., Molendijk, L., Van Vooren, L., van den Berg, W., Hoek, H., Runia, W., et al. (2018). Responses of soil biota to non-inversion tillage and organic amendments: an analysis on European multiyear field experiments. Pedobiologia 66, 18–28. doi: 10.1016/j.pedobi.2017.12.003
Fischer, G., Velthuizen, H. T., Shah, M. M., and Nachtergaele, F. O. (2002). Global Agro-Ecological Assessment for Agriculture in the 21st Century: Methodology and Results. Laxenburg: IIASA.
Gilhespy, S. L., Anthony, S., Cardenas, L., Chadwick, D., del Prado, A., Li, C., et al. (2014). First 20 years of DNDC (DeNitrification DeComposition): model evolution. Ecol. Modell. 292, 51–62. doi: 10.1016/j.ecolmodel.2014.09.004
Hashimoto, S., Nanko, K., Tupek, B., and Lehtonen, A. (2017). Data-mining analysis of the global distribution of soil carbon in observational databases and Earth system models. Geosci. Modell. Dev. 10, 1321–1337. doi: 10.5194/gmd-10-1321-2017
Henderson, B. L., Bui, E. N., Moran, C. J., and Simon, D. A. P. (2005). Australia-wide predictions of soil properties using decision trees. Geoderma 124, 383–398. doi: 10.1016/j.geoderma.2004.06.007
Hengl, T., Mendes de Jesus, J., Heuvelink, G. B., Ruiperez Gonzalez, M., Kilibarda, M., Blagotić, A., et al. (2017). SoilGrids250m: global gridded soil information based on machine learning. PLoS ONE 12:e0169748. doi: 10.1371/journal.pone.0169748
Heung, B., Ho, H. C., Zhang, J., Knudby, A., Bulmer, C. E., and Schmidt, M. G. (2016). An overview and comparison of machine-learning techniques for classification purposes in digital soil mapping. Geoderma 265, 62–77. doi: 10.1016/j.geoderma.2015.11.014
Hijbeek, R., van Ittersum, M. K., ten Berge, H. F. M., Gort, G., Spiegel, H., and Whitmore, A. P. (2017). Do organic inputs matter—a meta-analysis of additional yield effects for arable crops in Europe. Plant Soil 411, 293–303. doi: 10.1007/s11104-016-3031-x
Hobley, E., Wilson, B., Wilkie, A., Gray, J., and Koen, T. (2015). Drivers of soil organic carbon storage and vertical distribution in Eastern Australia. Plant Soil 390, 111–127. doi: 10.1007/s11104-015-2380-1
Hobley, E. U., and Wilson, B. (2016). The depth distribution of organic carbon in the soils of eastern Australia. Ecosphere 7:e01214. doi: 10.1002/ecs2.1214
Idé, T. (2016). “Formalizing expert knowledge through machine learning,” in Global Perspectives on Service Science: Japan, eds S. K.J. C. KwanSpohrer and Y. Sawatani (New York, NY: Springer New York), 157–175.
Ingram, J., Mills, J., Dibari, C., Ferrise, R., Ghaley, B. B., Hansen, J. G., et al. (2016). Communicating soil carbon science to farmers: incorporating credibility, salience and legitimacy. J. Rural Stud. 48, 115–128. doi: 10.1016/j.jrurstud.2016.10.005
Johnston, A. E., and Poulton, P. R. (2018). The importance of long-term experiments in agriculture: their management to ensure continued crop production and soil fertility; the Rothamsted experience. Eur. J. Soil Sci. 69, 113–125. doi: 10.1111/ejss.12521
Jorgensen, S. E., and Fath, P. D. (2011). Fundamentals of Ecological Modelling: Applications in Environmental Management and Research. Amsterdam: Elsevier.
Kavdir, Y., and Smucker, A. J. M. (2005). Soil aggregate sequestration of cover crop root and shoot-derived nitrogen. Plant Soil 272, 263–276. doi: 10.1007/s11104-004-5294-x
Kerselaers, E., Rogge, E., Lauwers, L., and Van Huylenbroeck, G. (2015). Decision support for prioritising of land to be preserved for agriculture: can participatory tool development help? Comput. Electron. Agric. 110, 208–220. doi: 10.1016/j.compag.2014.10.022
Liakos, K. G., Busato, P., Moshou, D., Pearson, S., and Bochtis, D. (2018). Machine learning in agriculture: a review. Sensors 18:E2674. doi: 10.3390/s18082674
Martin, M. P., Lo Seen, D., Boulonne, L., Jolivet, C., Nair, K. M., Bourgeon, G., et al. (2009). Optimizing pedotransfer functions for estimating soil bulk density using boosted regression trees. Soil Sci. Soc. Am. J. 73, 485–493. doi: 10.2136/sssaj2007.0241
Mazzilli, S. R., Ernst, O. R., de Mello, V. P., and Pérez, C. A. (2016). Yield losses on wheat crops associated to the previous winter crop: impact of agronomic practices based on on-farm analysis. Eur. J. Agron. 75, 99–104. doi: 10.1016/j.eja.2016.01.007
McBratney, A. B., Mendonça Santos, M. L., and Minasny, B. (2003). On digital soil mapping. Geoderma 117, 3–52. doi: 10.1016/S0016-7061(03)00223-4
Metzger, M. J., Bunce, R. G. H., Jongman, R. H. G., Mücher, C. A., and Watkins, J. W. (2005). A climatic stratification of the environment of Europe. Glob. Ecol. Biogeogr. 14, 549–563. doi: 10.1111/j.1466-822X.2005.00190.x
Mills, J., Gaskell, P., Ingram, J., and Chaplin, S. (2018). Understanding farmers' motivations for providing unsubsidised environmental benefits. Land Use Policy 76, 697–707. doi: 10.1016/j.landusepol.2018.02.053
Mouron, P., Aubert, U., Heijne, B., Naef, A., Strassemeyer, J., Hayer, F., et al. (2013). “A multi-attribute decision method for assessing the overall sustainability of crop protection strategies: a case study based on Apple production in Europe,” in Methods and Procedures for Building Sustainable Farming Systems: Application in the European Context, eds A. A. Marta-Costa and E. L. D. G. Soares da Silva (Dordrecht: Springer Netherlands), 123–37.
Mueller, L., Schindler, U., Behrendt, A., Eulenstein, F., and Dannowski, R. (2007). The Muencheberg Soil Quality Ranking (SQR): Field Manual for Detecting and Assessing Properties and Limitations of Soils for Cropping and Grazing. Muencheberg: Leibniz-Zentrum fuer Agrarlandschaftsforschung (ZALF).
Mueller, L., Schindler, U., Mirschel, W., Shepherd, T. G., Ball, B. C., Helming, K., et al. (2010). Assessing the productivity function of soils. A review. Agron. Sust. Dev. 30, 601–614. doi: 10.1051/agro/2009057
Mueller, L., Schindler, U., Shepherd, T. G., Ball, B. C., Smolentseva, E., Hu, C., et al. (2012). A framework for assessing agricultural soil quality on a global scale. Arch. Agron. Soil Sci. 58, S76–S82. doi: 10.1080/03650340.2012.692877
Oliver, D. M., Bartie, P. J., Heathwaite, A. L., Pschetz, L., and Quilliam, R. S. (2017). Design of a decision support tool for visualising E. coli risk on agricultural land using a stakeholder-driven approach. Land Use Policy 66, 227–234. doi: 10.1016/j.landusepol.2017.05.005
O'Sullivan, L., Creamer, R. E., Fealy, R., Lanigan, G., Simo, I., Fenton, O., et al. (2015). Functional Land Management for managing soil functions: a case-study of the trade-off between primary productivity and carbon storage in response to the intervention of drainage systems in Ireland. Land Use Policy 47, 42–54. doi: 10.1016/j.landusepol.2015.03.007
Parton, W. J., Hartman, M., Ojima, D., and Schimel, D. (1998). DAYCENT and its land surface submodel: description and testing. Glob. Planet. Change 19, 35–48. doi: 10.1016/S0921-8181(98)00040-X
Parton, W. J., and Rasmussen, P. E. (1994). Long-term effects of crop management in wheat-fallow: II. CENTURY model simulations. Soil Sci. Soc. Am. J. 58, 530–536. doi: 10.2136/sssaj1994.03615995005800020040x
Quintana-Seguí, P., Moigne, P. L., Durand, Y., Martin, E., Habets, F., Baillon, M., et al. (2008). Analysis of near-surface atmospheric variables: validation of the SAFRAN analysis over France. J. Appl. Meteorol. Clim. 47, 92–107. doi: 10.1175/2007jamc1636.1
Rose, D. C., Sutherland, W. J., Parker, C., Lobley, M., Winter, M., Morris, C., et al. (2016). Decision support tools for agriculture: towards effective design and delivery. Agric. Syst. 149, 165–174. doi: 10.1016/j.agsy.2016.09.009
Rutgers, M., van Wijnen, H. J., Schouten, A. J., Mulder, C., Kuiten, A. M., Brussaard, L., et al. (2012). A method to assess ecosystem services developed from soil attributes with stakeholders and data of four arable farms. Sci. Total Environ. 415, 39–48. doi: 10.1016/j.scitotenv.2011.04.041
Sandén, T., Spiegel, H., Stüger, H.-P., Schlatter, N., Haslmayr, H.-P., Zavattaro, L., et al. (2018). European long-term field experiments: knowledge gained about alternative management practices. Soil Use Manage 34, 167–176. doi: 10.1111/sum.12421
Schulte, R. P. O., Bampa, F., Bardy, M., Coyle, C., Creamer, R. E., Fealy, R., et al. (2015). Making the most of our land: managing soil functions from local to continental scale. Front. Environ. Sci. 3:81. doi: 10.3389/fenvs.2015.00081
Schulte, R. P. O., Creamer, R. E., Donnellan, T., Farrelly, N., Fealy, R., O'Donoghue, C., et al. (2014). Functional land management: a framework for managing soil-based ecosystem services for the sustainable intensification of agriculture. Environ. Sci. Policy 38, 45–58. doi: 10.1016/j.envsci.2013.10.002
Shaw, M. L. G., and Woodward, J. B. (1990). Modeling expert knowledge. Knowl. Acquisit. 2, 179–206. doi: 10.1016/S1042-8143(05)80015-9
Sheil, T. S., Wall, D. P., Culleton, N., Murphy, J., Grant, J., and Lalor, S. T. J. (2016). Long-term effects of phosphorus fertilizer on soil test phosphorus, phosphorus uptake and yield of perennial ryegrass. J. Agric. Sci. 154, 1068–1081. doi: 10.1017/S0021859615001100
Spiegel, H., Lindenthal, T., Mazorek, M., Ploner, A., Freyer, B., and Köchl, A. (2001). Results of three long-term P-field experiments in Austria: 1. Report: Effects of different types and quantities of P-fertiliser on yields and PCAL/DL-contents in soils. Die Bodenkultur 52, 3–17. Available online at: https://diebodenkultur.boku.ac.at/volltexte/band-52/heft-1/spiegel.pdf
Stavi, I., Bel, G., and Zaady, E. (2016). Soil functions and ecosystem services in conventional, conservation, and integrated agricultural systems. A review. Agron. Sust. Dev. 36:32. doi: 10.1007/s13593-016-0368-8
Techen, A.-K., and Helming, K. (2017). Pressures on soil functions from soil management in Germany. A foresight review. Agron. Sust. Dev. 37:64. doi: 10.1007/s13593-017-0473-3
Thoumazeau, A., Bessou, C., Renevier, M.-S., Trap, J., Marichal, R., Mareschal, L., et al. (2019). Biofunctool®: a new framework to assess the impact of land management on soil quality. Part A: concept and validation of the set of indicators. Ecol. Indic. 97, 100–110. doi: 10.1016/j.ecolind.2018.09.023
Tóth, G., Gardi, C., Bódis, K., Ivits, É., Aksoy, E., Jones, A., et al. (2013). Continental-scale assessment of provisioning soil functions in Europe. Ecol. Process 2, 1–18. doi: 10.1186/2192-1709-2-32
Trajanov, A., Kuzmanovski, V., Leprince, F., Real, B., Dutertre, A., Maillet-Mezeray, J., et al. (2015). Estimating drainage periods for agricultural fields from measured data: data-mining methodology and a case study (La JailliÈRe, France). Irrig. Drain 64, 703–716. doi: 10.1002/ird.1933
Trajanov, A., Spiegel, H., Debeljak, M., and Sandén, T. (2018). Using data mining techniques to model primary productivity from international long-term ecological research (ILTER) agricultural experiments in Austria. Reg. Environ. Change, 19, 325–337. doi: 10.1007/s10113-018-1361-3
Tulabandhula, T., and Rudin, C. (2014). On combining machine learning with decision making. Mach. Learn. 97, 33–64. doi: 10.1007/s10994-014-5459-7
Tversky, A., and Kahneman, D. (1974). Judgment under uncertainty: heuristics and biases. Science 185, 1124–1131. doi: 10.1126/science.185.4157.1124
USGS (2004). Shuttle Radar Topography Mission, 1 Arc Second Scene SRTM_u03_n008e004, Unfilled Unfinished 2.0. Available online at: http://glcf.umd.edu/data/srtm/
Uusitalo, L., Lehikoinen, A., Helle, I., and Myrberg, K. (2015). An overview of methods to evaluate uncertainty of deterministic models in decision support. Environ. Modell. Softw. 63, 24–31. doi: 10.1016/j.envsoft.2014.09.017
Valujeva, K., O'Sullivan, L., Gutzler, C., Fealy, R., and Schulte, R. P. O. (2016). The challenge of managing soil functions at multiple scales: an optimisation study of the synergistic and antagonistic trade-offs between soil functions in Ireland. Land Use Policy 58, 335–347. doi: 10.1016/j.landusepol.2016.07.028
van Leeuwen, J. P., Saby, N. P. A., Jones, A., Louwagie, G., Micheli, E., Rutgers, M., et al. (2017). Gap assessment in current soil monitoring networks across Europe for measuring soil functions. Environ. Res. Lett. 12:124007. doi: 10.1088/1748-9326/aa9c5c
Vuolo, F., Neuwirth, M., Immitzer, M., Atzberger, C., and Ng, W.-T. (2018). How much does multi-temporal Sentinel-2 data improve crop type classification? Int. J. Appl. Earth Obs. Geoinf. 72, 122–130. doi: 10.1016/j.jag.2018.06.007
Vuolo, F., Pipitone, C., Zappa, L., Wenng, H., Immitzer, M., Weiss, M., et al. (2016). Data service platform for sentinel-2 surface reflectance and value-added products: system use and examples. Remote Sens. 8:938. doi: 10.3390/rs8110938
Weiner, J. (2017). Applying plant ecological knowledge to increase agricultural sustainability. J. Ecol. 105, 865–870. doi: 10.1111/1365-2745.12792
Wieland, R., and Mirschel, W. (2017). Combining expert knowledge with machine learning on the basis of fuzzy training. Ecol. Inform. 38, 26–30. doi: 10.1016/j.ecoinf.2017.01.001
Witten, I. H., Frank, E., and Hall, M. A. (2011). Data Mining: Practical Machine Learning Tools and Techniques, 3rd Edn. Burlington, VT: Morgan Kaufmann.
WWAP (2015). The United Nations World Water Development Report 2015: Water for a Sustainable World. Paris: UNESCO.
Keywords: decision support model, data mining, expert knowledge, yield, soil functions, agricultural decision-making
Citation: Sandén T, Trajanov A, Spiegel H, Kuzmanovski V, Saby NPA, Picaud C, Henriksen CB and Debeljak M (2019) Development of an Agricultural Primary Productivity Decision Support Model: A Case Study in France. Front. Environ. Sci. 7:58. doi: 10.3389/fenvs.2019.00058
Received: 07 December 2018; Accepted: 15 April 2019;
Published: 17 May 2019.
Edited by:
Philippe C. Baveye, AgroParisTech Institut des Sciences et Industries du Vivant et de L'Environnement, FranceReviewed by:
Zamir Libohova, United States Department of Agriculture, United StatesCopyright © 2019 Sandén, Trajanov, Spiegel, Kuzmanovski, Saby, Picaud, Henriksen and Debeljak. This is an open-access article distributed under the terms of the Creative Commons Attribution License (CC BY). The use, distribution or reproduction in other forums is permitted, provided the original author(s) and the copyright owner(s) are credited and that the original publication in this journal is cited, in accordance with accepted academic practice. No use, distribution or reproduction is permitted which does not comply with these terms.
*Correspondence: Taru Sandén, dGFydS5zYW5kZW5AYWdlcy5hdA==
Disclaimer: All claims expressed in this article are solely those of the authors and do not necessarily represent those of their affiliated organizations, or those of the publisher, the editors and the reviewers. Any product that may be evaluated in this article or claim that may be made by its manufacturer is not guaranteed or endorsed by the publisher.
Research integrity at Frontiers
Learn more about the work of our research integrity team to safeguard the quality of each article we publish.