- USDA-ARS Crops Pathology and Genetics Research Unit, University of California, Davis, Davis, CA, United States
Anaerobic soil disinfestation (ASD) is an organic amendment-based management practice for controlling soil-borne plant pathogens. Pathogen suppression appears to be carbon source-dependent and mediated by bacteria that proliferate and produce volatile organic compounds, as well as physico-chemical changes (i.e., elevated temperature, lowered redox potential and pH, release of metal ions) in soil. ASD is under study for adoption in tree crops as a replacement for chemical-fumigation, but its widespread use is limited by incomplete understanding of its suppression mechanisms and high economic costs. The carbon substrate is one component of the ASD process that can be optimized to enhance effectiveness and affordability. While rice bran is currently the standard carbon source used for ASD, we identified three alternative substrates (molasses, mustard seed meal, and tomato pomace) that are similar in efficacy to rice bran at generating and sustaining soil anoxia and reducing populations of introduced plant pathogens. Here, we used replicated ASD field trials to determine if rice bran and the alternative carbon substrates would elicit similar soil bacterial communities (characterized via amplicon sequencing of the 16S rRNA gene v4 region) and to assess if any observed community shifts were consistent across repeated trials. We found significant, but minimal differences in community composition between ASD carbon treatments (F4, 30 = 2.80, P < 0.001, R2 = 0.22) and trials (F1, 30 = 5.24, P < 0.001, R2 = 0.10). In both trials, the abundances of Bacteroidales, Clostridiales, Selenomonadales, and Enterobacteriales increased significantly (>5 log2 fold change) in all ASD treatments compared to untreated controls. A group of shared core genera belonging to the Clostridiales and Selenomonadales were identified in both trials and constituted 22.6 and 21.5% of the communities. Bacterial taxa that were most responsive to ASD treatments had the genomic potential for denitrification, nitrogen fixation, and fermentation reactions that produce organic acids (such as acetate and butyrate) known to inhibit in vitro growth of plant pathogens based on predicted metagenomes. Together, these results indicate that reproducible and effective implementation of ASD is achievable with alternative carbon substrates to rice bran.
Introduction
Pre-plant soil fumigation using chemicals, such as methyl bromide, methyl iodide, 1,3-dichloropropene, and chloropicrin has been an important tool for the management of soil-borne plant pathogens (Duniway, 2002; Hanson et al., 2013). The application of chemical fumigants, however, is increasingly restricted due to negative environmental impacts, regulatory constraints, and human health concerns (Carpenter et al., 2001; Ajwa et al., 2013). The near complete phase-out of methyl bromide (https://www.epa.gov/ods-phaseout/methyl-bromide) combined with the concerns listed above for all soil fumigants has prompted development of alternative strategies to manage soil-borne plant pathogens (Martin, 2003; Schneider et al., 2003). Anaerobic soil disinfestation (ASD) is one such alternative gaining considerable attention (Blok et al., 2000; Momma et al., 2013). For example, ASD is used commercially as a pre-plant soil treatment for strawberry production and rice cultivation (Momma et al., 2013; Shennan et al., 2014, 2018) and is under active development for use in many cropping systems including various vegetables (Butler et al., 2014), apples (Hewavitharana et al., 2014; Hewavitharana and Mazzola, 2016), walnuts (Strauss and Kluepfel, 2015; Strauss et al., 2017), and almonds (Browne et al., 2018). Widespread implementation of ASD is currently limited by incomplete knowledge of its mode of action and the need for optimization across a variety of crops and soil types (Strauss and Kluepfel, 2015; Shrestha et al., 2016).
ASD is an organic amendment-based control for plant pathogens and weeds. Unlike conventional organic amendments used to increase soil organic matter or improve other soil properties (i.e., water retention, nutrient pools, etc.) over time, many carbon substrates used to stimulate ASD are expected to be readily consumed by microbes and not persist long-term in the soil. ASD consists of generating anaerobic conditions in soil through incorporation of a carbon source, irrigation to field capacity, and covering the soil with an impermeable plastic tarp to prevent gas exchange with the atmosphere (Blok et al., 2000; Momma et al., 2013). Together, these manipulations result in physico-chemical and biological shifts in soils that effectively reduce populations of many plant pathogens. ASD is generally effective at controlling plant pathogens, but does exhibit some variability depending on the pathogen targeted and carbon source used (Strauss and Kluepfel, 2015; Shrestha et al., 2016). Plant pathogens suppressed by ASD include, but are not limited to Agrobacterium tumefaciens, Fusarium oxysporum, Ralstonia solanacearum, Rhizoctonia solani, Pythium spp., Verticillium dahliae, and parasitic nematodes (Momma et al., 2006; Messiha et al., 2007; Hewavitharana et al., 2014; Strauss et al., 2017; Browne et al., 2018; Shennan et al., 2018).
The mechanisms involved in ASD-mediated pathogen suppression are not fully understood. However, numerous reports indicate that shifts in the microbial communities, evolution of volatile organic compounds, lowered pH, and reduced oxygen levels contribute to pathogen mortality (Momma et al., 2006, 2011; Momma, 2008; Hewavitharana et al., 2014). Soils subjected to ASD typically become hypoxic to anoxic within 3 days as microbes consume available oxygen. Subsequent anaerobic conditions can persist for 5–7 weeks under the gas impermeable tarp. During this time, bacterial taxa affiliated with known anaerobes and fermenters (e.g., Acidobacteria, Bacteroidetes, Firmicutes, etc.) proliferate and produce volatile fatty acids and other organic compounds (e.g., acetic acid, butyrate, etc.), which are lethal or suppressive to plant pathogens (Momma et al., 2006, 2011; Momma, 2008; Hewavitharana et al., 2014; Hewavitharana and Mazzola, 2016; Strauss et al., 2017). Other properties of ASD treated soils, such as lowered pH, release of metal ions (e.g., Mn2+ and Fe2+), and elevated temperature under the tarp also contribute to pathogen control (Momma et al., 2006, 2011; Butler et al., 2014).
Key determinants of ASD effectiveness include the carbon source used and application method and rate (Strauss and Kluepfel, 2015; Shrestha et al., 2016; Browne et al., 2018). Adjustment of these parameters offers opportunities to tailor ASD for particular crops, soil types, or localities. Ideally, carbon sources used for ASD should be broad-spectrum with respect to pathogen control, easily applicable, readily available, and affordable. Carbon inputs examined for ASD in commercial crop production include ethanol, molasses, composted poultry litter, and rice bran (Momma et al., 2013; Butler et al., 2014; Shennan et al., 2014, 2018). Rice bran applied at 20 t ha−1 is the standard carbon source and rate used for ASD in strawberry production in California (Shennan et al., 2014, 2018). Other carbon sources (e.g., cruciferous biomass, grasses, seed meals, wheat bran, etc.) have been found to be effective in the suppression of plant pathogens (Strauss and Kluepfel, 2015; Shrestha et al., 2016). In addition, common agricultural by-products, such as tomato pomace, red grape pomace, nut hulls and shells, etc. are also being explored for use in ASD applications. Agricultural by-products are particularly promising carbon substrates for examining and optimizing ASD efficacy. Many of them are locally available at low costs, which would increase the likelihood that ASD is adopted as a management practice for pathogen control by growers.
In preliminary ASD trials, we identified three agricultural by-products—molasses, mustard seed meal, and tomato pomace—which were similar in efficacy to rice bran at generating anaerobic conditions and reducing populations of introduced plant pathogens. These substrates contain readily labile carbon sources (i.e., sucrose, fructose, glucose, etc.), but also differ in their overall chemical composition (Saunders, 1985; Del Valle et al., 2006; Teclu et al., 2009; Rahman et al., 2018). Molasses can be characterized as the most labile substrate as its primary component is sucrose (Teclu et al., 2009), whereas rice bran, mustard seed meal, and tomato pomace contain large fractions of less labile forms of carbon (i.e., cellulose, hemicellulose, lignin, pectin, fatty acids, etc.) (Saunders, 1985; Del Valle et al., 2006; Rahman et al., 2018). We expect to observe large shifts in soil bacterial communities due to the imposition of anoxia and the input of readily available organic carbon (Pett-Ridge and Firestone, 2005; Cleveland et al., 2007). The various ASD carbon substrates may also stimulate the growth of particular bacterial groups due to differences in the degradability of their carbon components (Goldfarb et al., 2011). Hence, our aims were to (1) determine the effects of ASD implemented with different carbon substrates on bacterial community composition and (2) assess if any observed shifts in bacterial communities were consistent across repeated field trials.
Materials And Methods
Study Design
Two separate ASD field trials were conducted at the University of California, Kearney Agricultural Research Center located in Parlier, CA, USA. Soils at the location are Hanford series sandy loam texture with a pH of 7.3, total organic matter content of 1% (organic carbon content < 0.5%; Strauss et al., 2017), electrical conductivity of 0.8 dS m−1, and exchangeable potassium 5.6 meq 100 g−1. Typical amounts of extractable -P, -N, and -N in the soil are 0.3, 5.7, and 1.7 mg L−1, respectively (Albu et al., unpublished). Both trials were held in conventionally managed field sites. Prior to the establishment of the trials, large vegetative debris was removed and the ground leveled at both locations. Trial 1 was conducted from 20 September to 18 October 2016 and Trial 2 occurred from 24 October to 28 November 2016. Treatments in both trials consisted of no treatment controls (NTC), and ASD initiated with rice bran (RB; Pajaro Valley Gold 1.0, Farm Fuel, Inc., Freedom, CA, USA), mustard seed meal (MSM; Pescadero Gold 1.0, Farm Fuel, Inc., Freedom, CA, USA), molasses derived from sugar beets (MOL; Farm Fuel, Inc., Watsonville, CA, USA), tomato pomace (TP; Culinary Farms, Woodland, CA, USA), and tomato pomace and molasses (MTP) in combination. NTC plots did not receive a carbon amendment and were not covered with plastic tarps. In Trial 2, four additional control plots with irrigation and tarping, but no carbon substrate (NCC) were established. All plots (n = 4 per treatment) were 9.3 m2 and arranged in a completely randomized design, with a distance of 1.5 m between all plots.
Solid carbon substrates for ASD (RB, MSM, and TP) were ground into a powder and applied at 20.2 t ha−1 and incorporated into the soil using a rotary cultivator to a depth of 15 cm. The liquid carbon source, molasses, was first diluted 1:1 with water and then applied through the drip irrigation system directly onto the soil surface (MOL) or at a depth of 46 cm (MTP). Water was applied via three evenly spaced irrigation drip tape lines (emitters spaced 30.5 cm apart) per plot. After carbon source incorporation and positioning of irrigation lines, the soil was covered with transparent totally impermeable film (TIF, VAPORSAFE, Raven Engineered Films, Sioux Falls, SD, USA). A total of 12 acre-inches of water was applied to all ASD (and NCC in Trial 2) plots during the course of the trials across four separate time points. NTC plots were only irrigated at the onset of the trial with 3 acre-inches of water.
Soil Sampling
Soil for DNA extraction was collected prior to carbon source amendment (Pre-ASD) and at the end of each trial (4 weeks for Trial 1 and 5 weeks for Trial 2) from each plot. The top 2 cm of soil was removed prior to obtaining soil cores. Soil cores were collected using a 2.5 cm diameter soil corer at a depth of 15.2 cm from two random locations within each plot. In Trial 2, only one core per plot was retrieved from pre- and post-ASD NCC treatments. The cores were frozen immediately on dry ice for transport to the University of California at Davis and stored at −80°C until DNA extraction.
DNA Extraction, 16S rRNA Gene Sequencing, and Bioinformatic Processing
DNA was extracted from one soil core per plot for Pre-ASD soil (n = 24 for Trial 1, n = 28 for Trial 2) and all soil cores for post-ASD treatments (n = 48 for Trial 1; n = 52 for Trial 2). Briefly, the PowerLyzer PowerSoil DNA Isolation Kit (Mo Bio Laboratories, Inc., Carlsbad, CA, USA) was used to purify DNA from ~0.4 g of soil following the manufacturer's protocol. DNA integrity and purity was checked via gel electrophoresis, absorbance, and PCR amplification. DNA quantity was determined via fluorescence using the Qubit™ dsDNA High Sensitivity assay (Invitrogen, Carlsbad, CA, USA). DNA (~5 ng uL−1) was sent to the Michigan State University Research Technology Support Facility Genomics Core for sequencing of the16S rRNA gene v4 region using primer set 515F/806R (Caporaso et al., 2011), which targets Bacteria and Archaea as described in Kozich et al. (2013).
Raw sequences were processed into amplicon sequence variants (ASVs) using dada2 (version 1.4.0) implemented in R (version 3.4.1) on the USDA high performance computing cluster Ceres (Callahan et al., 2016; Team, 2017). ASVs were classified against the Silva v128 rRNA database (Quast et al., 2013) using the naïve Bayesian classifier with a cutoff of 0.80 (Wang et al., 2007). Libraries generated from duplicate cores were merged to generate composite communities for each treatment plot, resulting in 104 samples (48 for Trial 1 and 56 for Trial 2) with library sizes of 24,641–322,868 sequences (10,180,563 in total) and 19,996 ASVs. Raw sequence files are available in the NCBI Short Read Archive (BioProject PRJNA411854).
Libraries were then filtered to retain ASVs with a minimum abundance of 10 in at least four samples using phyloseq (version 1.23.1; McMurdie and Holmes, 2013). Filtering retained 89.7% of sequences and yielded libraries ranging in size from 21,750 to 304,811 sequences (9,131,043 in total) and containing 3,626 ASVs. SINA (version 1.2.11) was used to align the ASV sequences to a Silva v128 reference alignment spanning only the v4 region of the 16S rRNA gene (Pruesse et al., 2012). The ASV sequence alignment was used to infer a phylogenetic tree using FastTreeMP (version 2.1.8) and the GTR model (Price et al., 2010). Finally, predicted metagenomes for NTC and ASD carbon treatments (RB, MSM, MOL, TP, and MTP) were generated from the ASV sequences and abundance table using the online tool Piphillin (Iwai et al., 2016). The inferred metagenomes were based on the BioCyc database (version 21; Caspi et al., 2016) and a 97% identity cutoff to assign ASV sequences to a genome.
Statistical Analysis
All statistical analyses were performed using R v3.4.1 (Team, 2017). The R package ggplot2 (version 2.2.1) (Wickham, 2016) was used to generate graphs unless otherwise specified. The R package phyloseq was used to collapse ASVs by taxonomic rank, subsample libraries, and calculate alpha diversity measures (number of observed ASVs and the Simpson Evenness Index) (McMurdie and Holmes, 2013). The significance of differences in alpha diversity measures between treatments within each trial was assessed via analysis of variance (ANOVA) followed by Tukey's HSD for pairwise comparisons or Kruskal Wallis tests followed by Dunn tests for pairwise comparisons.
We used several approaches to test for significant differences in community composition between trials and treatments. First, we constructed an abundance-weighted phylogenetic dissimilarity matrix using PDcalc (version 0.3.1.9; Nipperess et al., 2010; Nipperess and Wilson, 2017) and visualized this matrix via non-metric multidimensional scaling (NMDS; dimensions = 2) implemented with the metaMDS function of vegan (version 2.4.6; Oksanen et al., 2016). The phylogenetic distance matrix then was used to determine if group variances were equal using the betadisper and permutest functions of vegan with each trial analyzed separately and the inclusion of only Pre-ASD, NTC, and ASD communities. Permutational multivariate analysis of variance (PERMANOVA) was used to determine if there were significant differences in community composition between trials and treatments for ASD communities. The adonis function in vegan was used to perform a two-factor PERMANOVA using a phylogenetic distance matrix of ASD soils with trial and ASD carbon substrate as categorical variables. Lastly, Multi-Response Permutation Procedure (MRPP) with 999 permutations was used to determine the values and significance of within and between group compositional dissimilarities based on the phylogenetic distances for ASD communities; these distances were graphed as boxplots for visualization.
A negative Binomial Wald test implemented with DESeq2 (Love et al., 2014) was used to determine if there was a significant change in taxa abundances at the order level between NTC, NCC, and ASD soils. Each trial was analyzed separately using non-subsampled libraries. Taxa were considered significantly differentially abundant between NTC soils and NCC or the ASD carbon treatments (RB, MSM, MOL, TP, and MTP) if they exhibited a log2 fold change (log2FC) >2 and a false discovery rate (FDR) <0.05. The results were graphed as a bar plot.
The core microbiome in ASD soils was determined using COREMIC (Rodrigues et al., 2018), which converts an abundance table to a presence/absence matrix and then uses one-tailed Fisher's Exact tests with Benjamini–Hochberg corrected P-values to determine if taxa are present at significantly higher proportions in a group of interest (ASD soils) vs. an out-group (NTC soils). We analyzed each trial separately and considered genera to be core responders to ASD carbon input if they were present in at least 90% of ASD soils with a FDR <0.05. We generated a heat map of the relative abundances of genera in each ASD treatment and NTC soils to visualize results.
To analyze the predicted metagenomes of NTC and ASD communities obtained using Piphillin, we filtered the feature table to retain reactions present with counts of 100 in at least four samples using phyloseq (McMurdie and Holmes, 2013) and calculated the magnitude and significance of log2FC of reactions in ASD communities compared to NTC using DESeq2 (Love et al., 2014). We then uploaded the log2FC and FDR values for all features to the MetaCyc Omics Dashboard (https://metacyc.org/dashboard/dashboard-intro.shtml; Caspi et al. (2016) and used enrichment analysis to identify subsystems with a high degree of statistically significant reaction abundance changes in the ASD communities based on an enrichment score or -log10(P-value) (Grossmann et al., 2007). We also determined the correlation between distance matrices based on phylogenetic diversity and reaction composition of NTC and ASD communities using a Mantel's test implemented in vegan with 999 permutations (Oksanen et al., 2016).
Results
Comparison of Community Taxonomic and Phylogenetic Diversity Between and Within Trials and Treatments
We estimated alpha diversity in Pre-ASD, NTC, NCC, and ASD communities (Table 1). In both trials, there were significant overall differences between treatments in ASV richness and community evenness. In Trial 1, RB and MTP communities were significantly less diverse than NTC communities, which had the highest number of ASVs. Otherwise, there were no significant pairwise differences in ASV richness in Trial 1. Community evenness in Trial 1 was significantly lower in ASD soils than in NTC communities. In Trial 2, alpha diversity measures were more variable within treatments. MOL communities had the highest number of ASVs, but were only significantly different from the treatments with the lowest number of ASVs (RB and MSM). MSM communities in Trial 2 also had particularly low evenness and differed significantly from NCC communities, but were not significantly dissimilar from the other ASD carbon treatments.
We used NMDS of a phylogenetic distance matrix of Pre-ASD, NTC, NCC, and ASD communities that integrates phylogenetic relationships and the abundances of ASVs to visualize shifts in community composition (Figure 1). The NMDS plot showed considerable overlap between soil communities in both trials. Pre-ASD, NTC, and NCC communities were positioned on the left side of NMDS1 with NCC and NTC communities clustering separately from Pre-ASD soils. ASD communities were mostly scattered on the right side of NMDS1 with no clear clustering by ASD carbon substrate. Testing for homogeneity of dispersions for communities grouped by treatment within each trial (i.e., Pre-ASD, NTC, RB, MSM, MOL, TP, and MTP) confirmed ASD carbon input to soils resulted in communities with similar variances that tended to be higher than Pre-ASD and NTC treatments (Table 2; Trial 1—F6, 41 = 3.28, P < 0.01; Trial 2—F6, 41 = 4.15, P < 0.005). Based on the NMDS plot and homogeneity of variance testing, we focused only on ASD soils to determine if there were significant differences in community composition between trials and treatments. To do so, we implemented a two-factor PERMANOVA using a phylogenetic distance matrix with trial and ASD carbon substrate as grouping variables. There were significant differences in community composition between trials (F1, 30 = 5.24, P < 0.001) and ASD carbon treatments (F4, 30 = 2.80, P < 0.001), but no significant interaction effect (F4, 30 = 1.09, P = 0.353). Although significant effects were detected, the variance explained by trial and ASD carbon substrate was low in both cases with R2 values of 0.10 and 0.22 for trial and ASD carbon treatment, respectively.
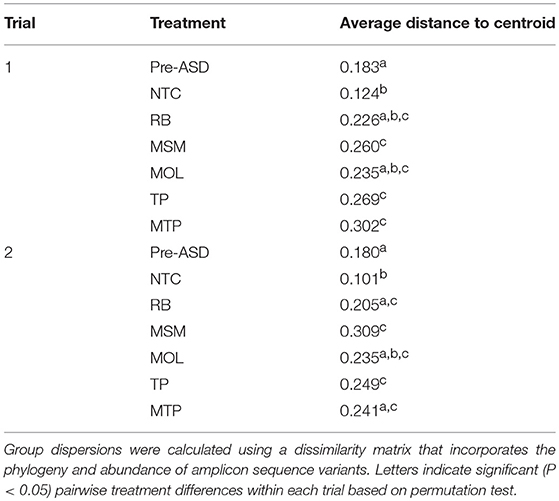
Table 2. Dispersions of communities grouped by treatment (NTC, no treatment control; RB, rice bran; MSM, mustard seed meal; MOL, molasses; TP, tomato pomace; MTP, molasses and tomato pomace) within each trial.
We then used MRPP to examine within and between treatment compositional dissimilarities for ASD communities in each trial separately. MRPP results for Trial 1 were not significant (observed and expected δ of 0.426 and 0.449, respectively and P = 0.105) and plots of the within and between phylogenetic distances clearly show the overall similarity of these distances for ASD communities (Figure 2A). MRPP for Trial 2 ASD communities showed a significant, but once again small effect of carbon input on community composition. The chance corrected within-group agreement for ASD carbon treatments in this trial was 0.118 and the within group distances (observed δ of 0.409) were significantly smaller (P = 0.002) than between group distances (expected δ of 0.464). The significant effect of ASD carbon input on communities in Trial 2 appeared to be driven by the addition of RB and MOL, which tended to have slightly smaller within group phylogenetic distances than the other ASD carbon substrates (Figure 2B).
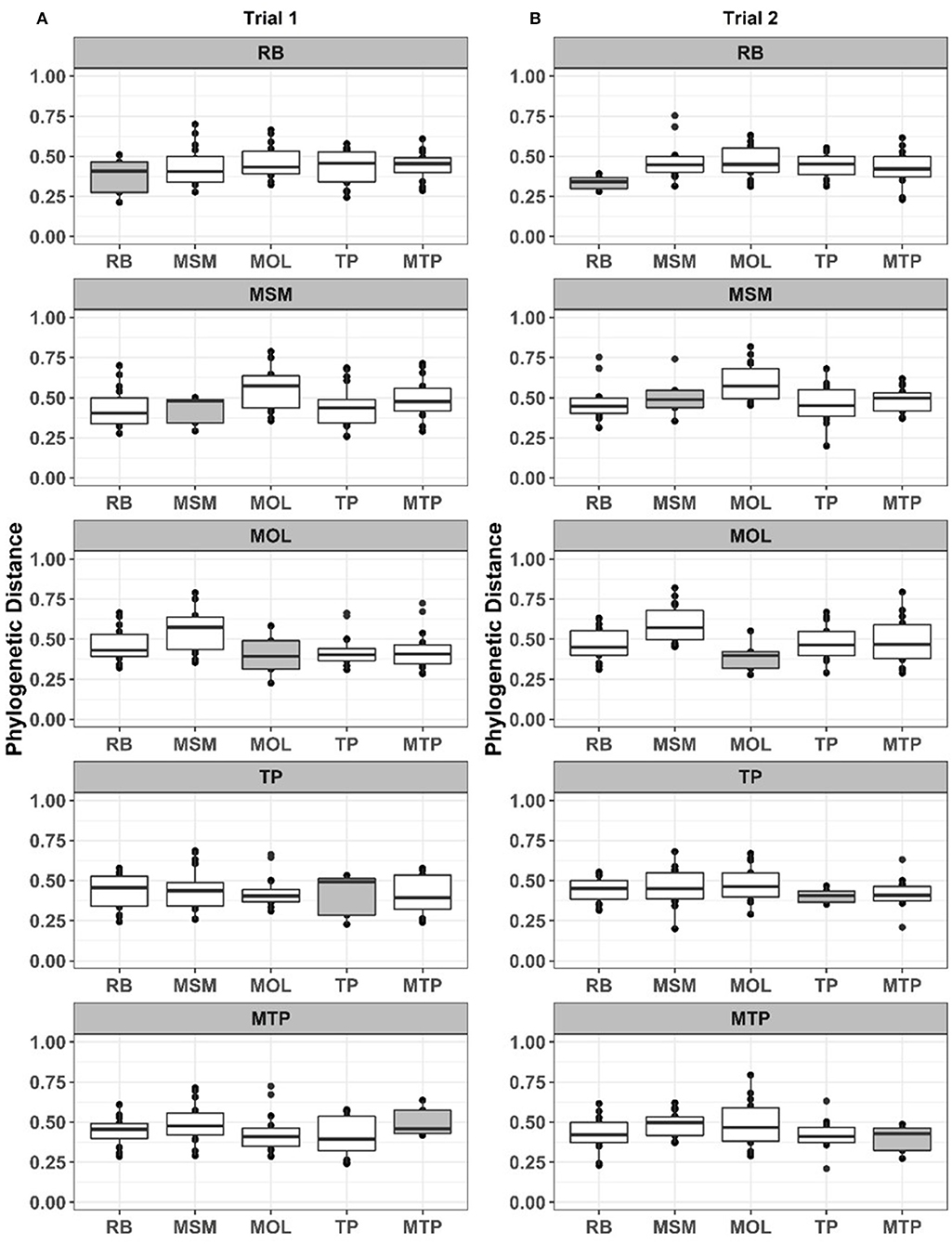
Figure 2. Phylogenetic distances between and within treatments (ASD communities only) in Trial 1 (A) and Trial 2 (B). In each panel, the shaded boxes show the distribution of within treatment distances and the open boxes show the distribution of between treatment distances.
Taxonomic Shifts in Response to ASD
Pre-ASD, NTC, NCC, and ASD communities differed in the relative abundances of major taxa, which included 12 phyla present at abundances of 1% or more (Figure 3). Overall, the communities were composed largely of Bacteria (>98%). Archaea in soils were mostly affiliated with the Soil Crenarchaeotic Group of the Thaumarchaeota. Proteobacteria were a large proportion of all communities (20.3–47.0%), while Firmicutes were equally or more abundant than Proteobacteria in ASD soils (25.4–60.4%). Acidobacteria, Chloroflexi, Cyanobacteria, Gemmatimonadetes, Planctomycetes, and Verrucomicrobia had higher abundances in Pre-ASD, NTC, and NCC soils than in ASD soils. Bacteroidetes were more abundant in ASD, NTC, and NCC soils compared to Pre-ASD soils. Conversely, Actinobacteria were present at higher proportions in Pre-ASD soils.
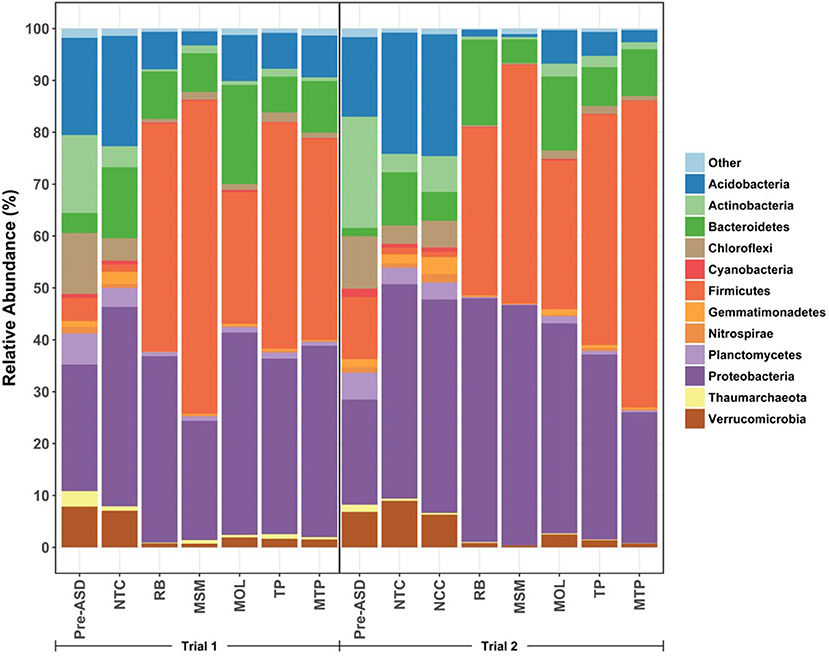
Figure 3. Relative proportion plot of phyla (>1% relative abundance) in Trial 1 (left) and Trial 2 (right). Values are the average of treatment replicates.
DESeq2 was used to detect significant differences in taxa abundances at the order level between NTC, NCC, and ASD treatments at the end of each trial (Table S1). In both trials, more taxa tended to significantly increase rather than decrease in abundance in the ASD treatments. In Trial 1, 25–42 (60 in total) of 151 orders were present at significantly different abundances in ASD treatments compared to NTC with RB communities containing the highest number of responsive orders. In Trial 2, the number of differentially abundant orders for ASD treatments ranged from 19 to 46 (58 in total) of 158 orders and MSM soils contained the highest number of responsive orders. Sixty-two orders were differentially abundant in at least one treatment across both trials. Most taxa abundances in NCC and NTC communities in Trial 2 were similar with only seven of 158 orders detected as significantly different.
The log2 fold changes in abundance of the top 20 most prevalent orders in NTC, NCC, and ASD communities are shown in Figure 4. Taxa abundances of Subgroup_6, Caulobacterales, and Rhizobiales did not change significantly due to treatment in either trial. However, a number of orders exhibited notable increases in abundance in response to ASD carbon input and these shifts were largely mirrored between trials. Taxa belonging to the strictly and facultative anaerobic orders Bacteroidales, Clostridiales, Selenomonadales, and Enterobacteriales increased by more than 5 log2FC in both trials. Members of the Bacillales also increased by more than 2 log2FC in both trials, but to a lesser extent than the other responsive Firmicutes. Rhodocyclales abundances also increased substantially in both trials, with the exception of the MOL treatment in Trial 2. Likewise, Pseudomonadales abundances increased by at least 5 log2FC in Trial 1, but only in RB and MTP soils in Trial 2. Other orders exhibited much more variable responses to ASD carbon input. Acidobacteriales and Myxococcales abundances increased by 2–6 log2FC in Trial 1 in at least three ASD treatments, but their abundances in Trial 2 did not change significantly. Taxa that exhibited this type of response in Trial 2 were members of the Desulfuromonadales and Sphingobacteriales. The abundances of Rhodospirillales and Burkholderiales generally did not change substantially in either trial, except in RB (both trials) and MSM (Trial 2) soils. RB input into soil generally yielded more taxa with significant log2FC decreases than the other ASD carbon substrate (Table S1) and a number of these taxa belong to the Blastocatellales, Sphingomonadales, and Chthoniobacterales.
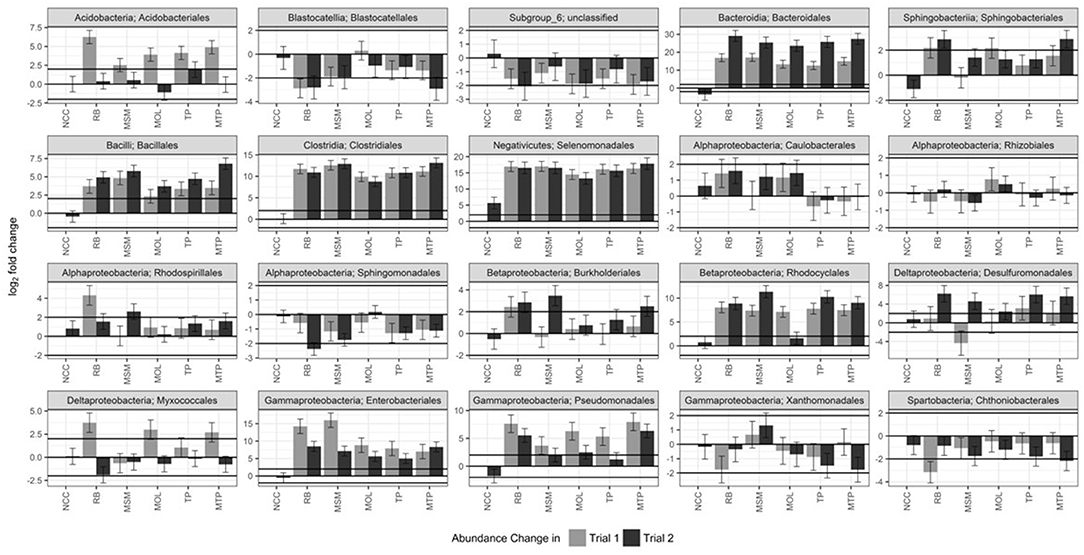
Figure 4. Differential abundance of the top 20 most abundant orders in ASD and NCC communities compared to NTC soils in both trials. DESeq2 was used to calculate log2 fold changes and test for significance (FDR < 0.05). Error bars are the standard error of treatment replicates. Black lines demarcate a log2FC of 2. Generally, abundance changes that exceeded 2 log2FC were significant. Please note, the log2FC axes for taxa are scaled independently.
We identified core responders to ASD carbon input at the genus level in each trial using the program COREMIC (Rodrigues et al., 2018) and graphed the relative abundances of these genera in NTC and ASD communities in Figure 5. In trials 1 and 2, respectively, 24 and 22 genera were identified as core taxa in ASD soils with 15 of these genera shared between the two trials. The core genera represented 39.9–55.2% (average of 51.4%) of the ASD communities in Trial 1 and 21.6–57.6 (average of 41.9%) of the communities in Trial 2. Most of the core genera in ASD soils were not detected in NTC soils or were present at abundances between 0.01 and 0.05%. The shared core genera between trials were all members of the Clostridiales or Selenomonadales and were on average 22.6% (13.6–33.5%) and 21.5% (10.1–30.6%) of the ASD communities in Trials 1 and 2, respectively.
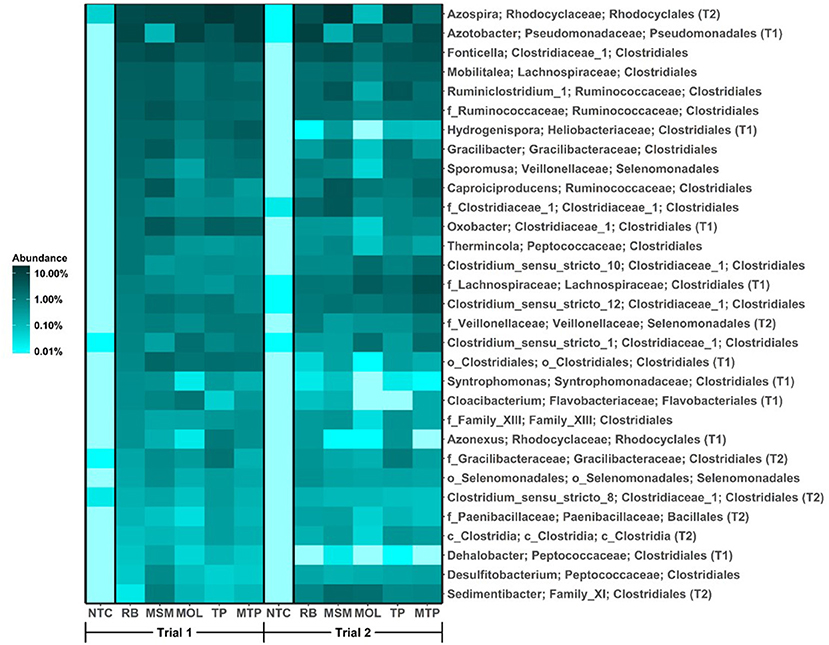
Figure 5. Heat map of the relative abundance of core genera in ASD soils in both trials. Core genera unique to each trial are designated by trial number. Genera identified as core members were present at significantly higher proportions in ASD soils (FDR < 0.05).
Changes in Metabolic Pathway Abundances Based on Predicted Metagenomes
We used the Piphillin algorithm (Iwai et al., 2016) and BioCyc database (Caspi et al., 2016) to generate inferred metagenomes of NTC and ASD communities based on a 97% identity cutoff for assignment of ASV sequences to known genomes. At this cutoff, 17.8% of the ASVs were assigned to genomes by the Piphillin algorithm. This is not a large proportion of the total community to use in the prediction of metagenomes, but there was a high and significant correlation (Mantel's r = 0.69; P < 0.001) between the distance matrices based on phylogenetic/taxonomic and predicted metagenomic compositions of NTC and ASD soils. Therefore, we believe the predicted metagenomes were adequate to capture potential metabolic changes in the ASD communities compared to NTC.
Enrichment analysis, which indicates the degree to which subsystems have a substantial number of reactions or pathways with significant abundance changes, showed the importance of fermentation, anaerobic respiration, and hydrogen (H2) production in ASD soils (Figure 6). Fermentation pathways had large enrichment scores for all ASD carbon treatments in Trial 1 with –log10(P-values) between 11 and 24; these scores were lower in Trial 2 with values between 2.45 and 15.7. MSM communities had the largest enrichment scores for fermentation pathways in both trials, while MOL, TP, and MTP enrichment scores were similar within each trial. Fermentation reactions with significant log2 fold changes had reactants and products that included acetate, acetyl-CoA, acetoacetate, acetoin, acetone, butanol, butyrate, dihydroxyacetone, formate, glycerol, methylaspartate, pyruvate, sorbitol, succinate, etc. (Table S2). Pathways involved in anaerobic respiration and H2 production had lower enrichment scores (0.25–4.63) than fermentation pathways. For some treatments, the subsystems did not have a large number of reactions with significant changes in abundances between NTC and ASD communities (i.e., TP in Trial 1 and MSM and MOL in Trial 2 for anaerobic respiration; Figure 6). Nonetheless, specific anaerobic respiratory reactions that were enriched in ASD communities involved denitrification and sulfur cycling (i.e., sulfite reduction and dimethyl sulfoxide reduction; Table S2). For example, the pathway for nitrous oxide reduction to N2 was enriched by 2.45–4.1 log2FC in all ASD communities. In addition to energy metabolism pathways, the predicted metagenomes of most ASD communities also had higher abundances of reactions for N2 fixation, assimilatory nitrate reduction [NAD(P)H- and ferredoxin-dependent pathways], ferric iron reduction, and quorum sensing (Figure 7). The reaction abundances for these pathways in the predicted metagenomes from all Trial 1 ASD soils increased by >2 log2FC, with quorum sensing in RB and MSM communities increasing by ~6 and 7 log2FC. In Trial 2, only the abundance of pathways for quorum sensing increased by >5 log2FC in all treatments. MOL communities in Trial 2 did not show an abundance increase for N2 fixation, assimilatory nitrate reduction, and ferric iron reduction.
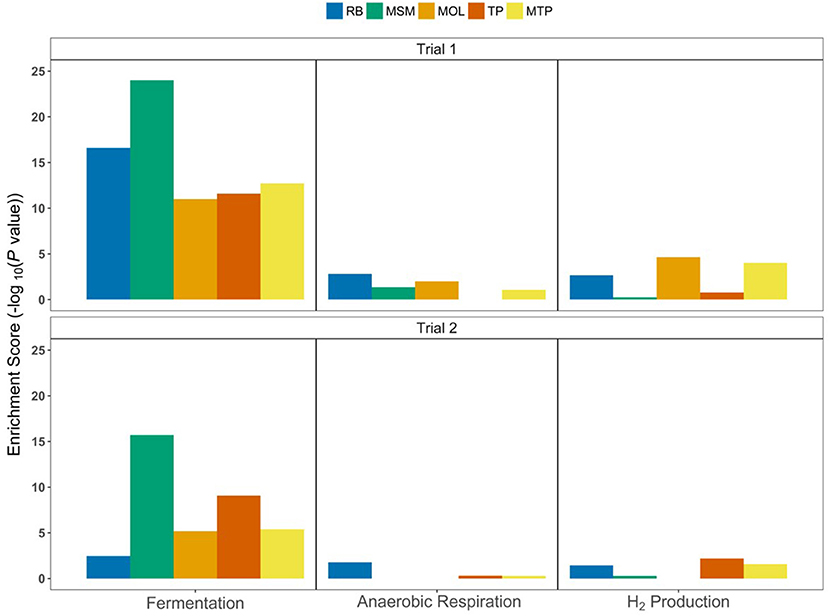
Figure 6. Enrichment scores for energy metabolism pathways in ASD communities based on predicted metagenomes. The –log10(P-value) scores were determined using the values and significance of log2 fold changes (calculated using DESeq2) in predicted pathway abundances in ASD communities compared to NTC.
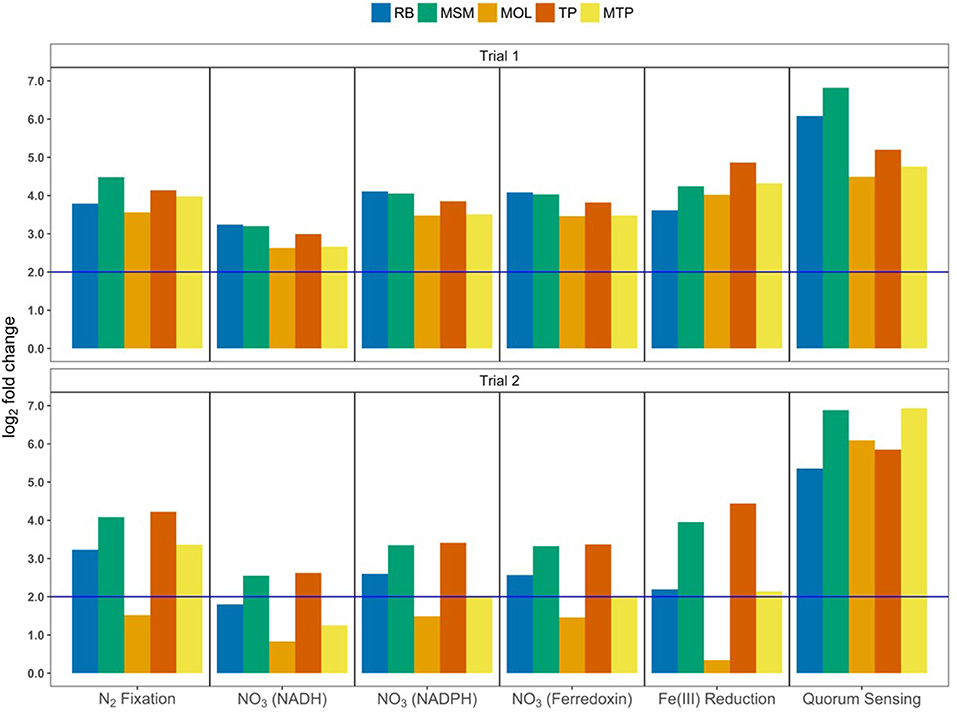
Figure 7. Differential abundance of pathways involved in nitrogen assimilation, ferric iron reduction, and quorum sensing in ASD communities compared to NTC based on predicted metagenomes. DESeq2 was used to calculate log2FC and values above 2 (blue line) were significant (FDR < 0.05).
Discussion
Widespread adoption of ASD for control of soil-borne plant pathogens requires an understanding of its efficacy in different settings (e.g., time of year implemented, cropping systems, and soil types), as well as identification of optimal carbon sources and application rates (Strauss and Kluepfel, 2015; Shrestha et al., 2016; Browne et al., 2018). Selection of carbon substrates for ASD is particularly important because anaerobic soil conditions alone are not sufficient to reduce populations of plant pathogens (Blok et al., 2000). Moreover, the effectiveness of ASD depends on both physicochemical and biological shifts in soils (Strauss and Kluepfel, 2015; Shrestha et al., 2016), which may relate to carbon substrates used to induce ASD (Hewavitharana et al., 2014; Hewavitharana and Mazzola, 2016). In this study, we examined microbial community shifts in repeated ASD field trials using different carbon sources: rice bran, mustard seed meal, molasses, and tomato pomace. We previously showed the latter three carbon sources performed similarly to rice bran, the standard ASD substrate, with respect to generation of anaerobic soil conditions and reduction of A. tumefaciens populations in greenhouse soil mesocosms (Albu et al., unpublished data). The greenhouse results were confirmed in the replicated field trials (Albu et al., unpublished data), as soil redox potential (a proxy for anoxia), temperature, and moisture were similar among all treatments at a depth of 30.5 cm over the course of both trials. The addition of ASD carbon substrates also significantly reduced populations of A. tumefaciens and P. ultimum at 15 cm in Trial 1. Pathogen control at the same depth in Trial 2 was more variable. A question that remained was whether these ASD carbon inputs (RB, MSM, MOL, TP, and MTP) would result in similar soil microbial communities in the field. The ASD carbon substrates are a potential source of microbes, but we assume any microbial populations in the carbon sources are at low abundances and would likely be outcompeted by resident soil microbes. Hence, we attribute changes in bacterial communities in response to ASD carbon amendment to shifts in the soil microbes.
The carbon inputs we tested significantly shifted soil bacterial community composition toward taxa known to be diazotrophic and anaerobic (Jones et al., 2008; Luque-Almagro et al., 2011; Dos Santos et al., 2012; Oren, 2014; Anantharaman et al., 2018), as well as microbial taxa with the ability to differentially respond to labile organic matter addition to soil (Fierer et al., 2007; Goldfarb et al., 2011). However, ASD did not result in communities with highly clustered and distinct compositions as a function of carbon substrate (Figure 1). ASD carbon treatments minimally affected community composition (i.e., no within or between group differences in phylogenetic distance in Trial 1 and 11.8% of distances explained by ASD carbon substrate in Trial 2; Figure 2). Additionally, soils that received ASD carbon substrates tended to have higher group variances than both non-treated soils (NTC) and soils that were tarped and irrigated but did not receive carbon input (NCC) in Trial 2 (Figure 1 and Table 2). These results indicate soil communities that assembled in response to ASD carbon input, especially of the solid carbon substrates, in both trials were heterogeneous, but composed of taxa with similar physiological capabilities. Indeed, the most highly responsive taxa to the carbon substrate addition and the shared core members of ASD soil communities across both trials were members of the Firmicutes (Figures 3–5). These taxa were at low abundances in Pre-ASD soils (Figure 3). This is commonly observed in soils for Bacilli and Clostridia (von Mering et al., 2007), which were likely in a state of dormancy prior to ASD (Galperin, 2013). In this study, the conditions induced by ASD, regardless of carbon source, may have functioned as a habitat filter, allowing the proliferation of closely related taxa with shared physiological adaptations (Horner-Devine and Bohannan, 2006). The differential ability of cells to awaken from dormancy also may have played a role in the local heterogeneity of ASD communities (Lennon and Jones, 2011). It is also possible that bacterial communities were more strongly differentiated by ASD treatments immediately after carbon input and induction of anaerobic conditions. Hence, future studies to track community shifts over time are needed to fully understand the responses of soil bacteria to ASD as a function of carbon substrate (i.e., identifying immediate vs. late responders) and the potential roles of habitat filtering and cells awakening from dormant states in shaping community composition.
Because we conducted replicated field trials, we also were able to address a question related to ASD efficacy: Were the taxonomic shifts in response to ASD carbon input consistent between trials? We detected a significant difference in community composition of ASD soils between trials based on the phylogenetic distances between ASVs, but this effect was small (i.e., 10% of explained variance based on PERMANOVA). Furthermore, assessment of the congruence between trials in compositional shifts at higher taxonomic levels (i.e., order and genus) indicate the existence of a core group of taxa that were responsive to ASD. The direction and magnitude of the log2 fold changes of bacterial orders in ASD soils relative to NTC were largely similar between trials, especially for these highly responsive groups: Bacillales, Bacteroidales, Clostridiales, Enterobacteriales, Rhodocyclales, Pseudomonadales, and Selenomonadales (Figure 4). The COREMIC analysis further supports this assertion, as the shared core genera between trials (all members of the Firmicutes) constituted ~22% of the community in ASD soils (Figure 5).
Several of the highly responsive taxa to ASD carbon input exhibit some degree of ecological coherence or shared physiological strategies that differentiate them from other taxonomic groups (Philippot et al., 2010). It is not surprising that members of the Firmicutes and Bacteroidetes (specifically the Bacteroidales) were among the microbes that thrived in anoxic soils amended with organic carbon, given the traits of these groups. Clostridiales have been shown to increase in abundance in after the addition of recalcitrant forms of organic carbon to soil (Goldfarb et al., 2011) and Bacteroidetes tend to respond to high carbon availability in soil (Fierer et al., 2007). Members of the Betaproteobacteria, including the order Rhodocyclales that increased in abundance in both trials, are among the first initial responders to labile carbon addition to soil (Fierer et al., 2007; Goldfarb et al., 2011). The Rhodocyclales response was largely due to ASVs classified as Azospira and to a lesser extent Azoarcus and Azonexus. These genera are often plant-associated and capable of growth on organic acids, use of nitrate as a terminal electron acceptor, and N2 fixation (Oren, 2014). Another diazotroph, Azotobacter, largely accounted for the increased abundance of Pseudomonadales in ASD soils. This bacterium is often included in biofertilizers for its capacity to fix N2 and produce exopolysaccharides (Gauri et al., 2012). On average, ~16.2% (5.4–24.7%) of the ASD communities were putative diazotrophs, indicating that N2 fixation was likely an important physiological trait of the responsive taxa in this soil system.
Even among the Acidobacteria, which declined in proportion at the phylum level in both trials (Figure 3), the abundance changes at lower taxonomic ranks of this group was consistent with previous studies of environmental constraints on their distribution. For example, Blastocatellia (Subgroup_4)- and Subgroup_6-affiliated ASVs tended to decrease in abundance, although not by more than 2 log2FC in ASD soils compared to NTC (Figure 4). This is in accord with a positive relationship between these groups and soil pH (Jones et al., 2009). ASD-treated soils are generally acidic (Momma et al., 2006, 2011) and we have found that addition of RB and TP to soil collected from a similar location as the trial sites in this study lowers the pH from 7.4 to 5.8 and 5.6, respectively. The order Acidobacteriales in Trial 1 increased by >2 log2FC in ASD soils, but not in Trial 2. This was largely due to the presence of an ASV in Trial 1 related to a heterotrophic acidobacterium, Candidatus Koribacter, with the genomic potential to tolerate wet/dry cycles in soil and use carbon substrates that range from simple carbohydrates to complex hemicellulose, cellulose, and chitin (Ward et al., 2009).
Numerous studies across different soil types and with various carbon substrates show ASD alters microbial communities in ways similar to those reported in this study (Messiha et al., 2007; Mowlick et al., 2012, 2013; Hewavitharana et al., 2014; Hewavitharana and Mazzola, 2016; Strauss et al., 2017). Our study and that of Strauss et al. (2017), which found ASD implemented with rice bran for 6 weeks was effective at controlling populations of A. tumefaciens and P. ultimum, were conducted ~2–3 years apart at the same agricultural experimental station on soil of similar texture and organic carbon content. Both studies found decreased abundances of Acidobacteria (phylum), Actinobacteria, Chloroflexi, Gemmatimonadetes, Nitrospirae, Planctomycetes, Alphaproteobacteria, and Verrucomicrobia in ASD soils amended with rice bran compared to no treatment. Likewise, both studies observed a substantially higher abundance of Firmicutes, especially Clostridia, in ASD-treated soils. Nonetheless, there were some slight differences between results from the studies, which may be due to the use of different databases and approaches for sequence processing or other factors (i.e., previous plantings on soil). Strauss et al. (2017) found similar proportions (~16%) of Bacilli in ASD and non-treated soils, while we found this group was 1.25% of the community in non-treated soils and ~5% of RB amended soils. Bacteroidetes and the classes Betaproteobacteria and Acidobacteria were more abundant in rice bran amended soils in the Strauss et al. (2017) study, while particular orders from these classes (i.e., Bacteroidales, Acidobacteriales in Trial 1, and Rhodocyclales) were more abundant in rice bran soils in this study. Taken together, both studies indicate that implementation of ASD at least with rice bran results in largely reproducible community shifts and ability to suppress or kill introduced plant pathogens.
We generated predicted metagenomes of ASD and NTC communities to explore potential metabolic pathways of responsive taxa, especially in relation to other studies that have started to elucidate the mechanisms by which ASD suppresses targeted plant pathogens. We detected a number of fermentation reactions that produce organic acids as significantly enriched in the predicted metagenomes of ASD communities relative to NTC (Figure 6; Table S2). For example, the enzymes phosphotransbutyrylase and butyrate kinase produce butyrate from butyryl-CoA through the intermediate butyryl-phosphate in Clostridium acetobutylicum (Louis et al., 2004). In the predicted metagenomes of ASD communities (all treatments) in both trials, reactions for these enzymes were 2.1–3.8 log2FC (phosphotransbutyrylase) and 4.4–6.3 log2FC (butyrate kinase) more abundant than in NTC soils (Table S2). We also found the metabolic potential for the production of butanol, a precursor for long-chain alcohols, was more abundant in ASD communities than NTC by 4.8–6.5 log2FC (Table S2), which may be due to the presence of Clostridium species (Richter et al., 2016). Fermentation products, such as organic acids (e.g., acetate, butyrate, etc.), accumulate in ASD-treated soils and can decrease the viability of the plant pathogens F. oxysporum and R. solanacearum when directly amended into soil at concentrations similar to those achieved during ASD (Momma et al., 2006). The profile of other volatiles produced during ASD is also carbon source-dependent (i.e., ethanol, grass residues, and MSM) and includes compounds, such as allyl isothiocyanate (derived from MSM), dimethyl trisulfide, 2-ethyl-1-hexanol, hexanol, non-anal, and decanal (Hewavitharana et al., 2014). Similar to organic acids, these compounds also reduce in vitro growth of F. oxysporum, P. ultimum, R. solani, and the parasitic nematode Pratylenchus penetrans (Hewavitharana et al., 2014). This preliminary linking of taxonomic shifts with potential metabolic changes points to the utility of metagenomic sequencing for understanding the role of specific microbial groups in pathogen suppression in ASD soils.
The predicted metagenomes also were useful in the identification of potential metabolic pathways in soil that may be important biogeochemically under conditions generated by ASD. In this study, ASD communities were enriched in taxa with predicted pathways for N2 fixation, assimilatory nitrate reduction, denitrification, and sulfur cycling (Figures 6, 7; Table S2). The increased prevalence of these putative metabolic pathways in ASD soils may be unique to this study or a more general feature of communities that assemble in response to ASD. All of these pathways are phylogenetically widespread among bacteria anaerobic (Jones et al., 2008; Luque-Almagro et al., 2011; Dos Santos et al., 2012; Oren, 2014; Anantharaman et al., 2018), thus it would not be surprising that taxa capable of denitrification or anaerobic reduction of sulfur compounds (i.e., sulfate, sulfite, and DMSO) thrive in ASD-treated soils. The assertion that denitrification occurs in ASD-treated soils is bolstered by a recent study that found ASD implemented with composted poultry litter or molasses resulted in higher nitrous oxide emissions post-puncturing of gas impermeable tarps for planting (Di Gioia et al., 2017). This study and our findings suggest investigations on the potential of ASD to contribute to greenhouse gas emissions are needed, especially as the adoption of ASD as a pre-plant treatment for a wider variety of crops increases. Additionally, one of the core shared microbes between trials, Desulfitobacterium (Figure 5), can grow on humic acids in soil and may even form syntrophic partnerships with sulfate-reducing bacteria (Villemur et al., 2006). Taxa capable of N2 fixation (i.e., Azospira and Azotobacter) were among the most abundant microbes in the ASD communities. We did not measure total or inorganic nitrogen pools in ASD-treated soils, hence we do not know how addition of carbon substrates (which contain nitrogen as well as carbon) changed nitrogen availability over the course trial. However, the increase in diazotrophs suggests the soil in this study may have been nitrogen-limited or ASD generated conditions that were favorable to the growth of these organisms (i.e., anoxia and in situ N2 production). The possibility that diazotrophs in ASD soils were using N2 produced via denitrification is supported by the predicted metagenomes of ASD communities, which were enriched in reactions for denitrification from dissimilatory nitrate reduction to generation of N2 by >2 log2FC for most ASD treatments (Table S2). If this stimulation of N2-fixers in ASD soil is reproducible, then it raises possibility that ASD, as a pre-plant soil treatment, may be another method of enhancing nitrogen availability in soil, as has been shown for other soil nutrients, especially phosphorus and potassium (Di Gioia et al., 2017).
Conclusion
In repeated field trials, we have shown that ASD induced with different agricultural by-products resulted in soil communities largely composed of taxa with the genomic potential for anaerobic respiration, including denitrification, N2 fixation, and ability to produce a wide variety of compounds inhibitory to the growth of plant pathogens. We also found the phylogenetic/taxonomic composition of communities in soils amended with the ASD carbon substrates were not highly distinguishable. The addition of ASD carbon substrates and resultant soil conditions generally reduced populations of A. tumefaciens and P. ultimum. Our results indicate that reproducible and effective implementation of ASD is achievable using alternative carbon substrates to rice bran, which all support development of pathogen suppressive microbial communities.
Author Contributions
SA, AM, and DK designed the study. AP-P, SA, AM, and DK conducted field work. AP-P performed lab work, bioinformatics, statistical analyses, and wrote the first draft of the manuscript. All authors edited the manuscript and approved submission.
Funding
This research was funded by the USDA CRIS Project Integrated Disease Management Strategies for Woody Perennial Species (2032-22000-016-00D).
Conflict of Interest Statement
The authors declare that the research was conducted in the absence of any commercial or financial relationships that could be construed as a potential conflict of interest.
Acknowledgments
We kindly thank Nhu Do, Janine Klein, and Dara Russell for field and laboratory assistance and the University of California Kearney Agricultural Research and Extension Center for field use, support, and management. We greatly appreciate the donation of tomato pomace by Culinary Farms, Woodland, CA, USA. We also thank Enid Gonzalez-Orta and Kerri Steenwerth for critical review of this manuscript.
Supplementary Material
The Supplementary Material for this article can be found online at: https://www.frontiersin.org/articles/10.3389/fenvs.2018.00160/full#supplementary-material
References
Ajwa, H., Stanghellini, M. S., Gao, S., Sullivan, D. A., Khan, A., Ntow, W., et al. (2013). Fumigant emission reductions with TIF warrant regulatory changes. Calif. Agric. 67, 147–152. doi: 10.3733/ca.v067n03p147
Anantharaman, K., Hausmann, B., Jungbluth, S. P., Kantor, R. S., Lavy, A., Warren, L. A., et al. (2018). Expanded diversity of microbial groups that shape the dissimilatory sulfur cycle. ISME J. 12, 1715–1728. doi: 10.1038/s41396-018-0078-0
Blok, W. J., Lamers, J. G., Termorshuizen, A. J., and Bollen, G. J. (2000). Control of soilborne plant pathogens by incorporating fresh organic amendments followed by tarping. Phytopathology 90, 253–259. doi: 10.1094/phyto.2000.90.3.253
Browne, G., Ott, N., Poret-Peterson, A., Gouran, H., and Lampinen, B. (2018). Efficacy of anaerobic soil disinfestation for control of Prunus replant disease. Plant Dis. 102, 209–219. doi: 10.1094/pdis-09-16-1392-re
Butler, D. M., Kokalis-Burelle, N., Albano, J. P., McCollum, T. G., Muramoto, J., Shennan, C., et al. (2014). Anaerobic soil disinfestation (ASD) combined with soil solarization as a methyl bromide alternative: vegetable crop performance and soil nutrient dynamics. Plant Soil 378, 365–381. doi: 10.1007/s11104-014-2030-z
Callahan, B. J., McMurdie, P. J., Rosen, M. J., Han, A. W., Johnson, A. J. A., and Holmes, S. P. (2016). DADA2: high-resolution sample inference from Illumina amplicon data. Nat. Methods 13, 581–583. doi: 10.1038/nmeth.3869
Caporaso, J. G., Lauber, C. L., Walters, W. A., Berg-Lyons, D., Lozupone, C. A., Turnbaugh, P. J., et al. (2011). Global patterns of 16S rRNA diversity at a depth of millions of sequences per sample. Proc. Natl. Acad. Sci. U.S.A. 108(Supplement 1x), 4516–4522. doi: 10.1073/pnas.1000080107
Carpenter, J., Lynch, L., and Trout, T. (2001). Township limits on 1,3-D will impact adjustment to methyl bromide phase-out. Calif. Agric. 55, 12–18. doi: 10.3733/ca.v055n03p12
Caspi, R., Billington, R., Ferrer, L., Foerster, H., Fulcher, C. A., Keseler, I. M., et al. (2016). The MetaCyc database of metabolic pathways and enzymes and the BioCyc collection of pathway/genome databases. Nucleic Acids Res. 44, D471–D480. doi: 10.1093/nar/gkv1164
Cleveland, C. C., Nemergut, D. R., Schmidt, S. K., and Townsend, A. R. (2007). Increases in soil respiration following labile carbon additions linked to rapid shifts in soil microbial community composition. Biogeochemistry 82, 229–240. doi: 10.1007/s10533-006-9065-z
Del Valle, M., Camara, M., and Torija, M. E. (2006). Chemical characterization of tomato pomace. J. Sci. Food Agric. 86, 1232–1236. doi: 10.1002/jsfa.2474
Di Gioia, F., Ozores-Hampton, M., Zhao, X., Thomas, J., Wilson, P., Li, Z. O., et al. (2017). Anaerobic soil disinfestation impact on soil nutrients dynamics and nitrous oxide emissions in fresh-market tomato. Agric. Ecosyst. Environ. 240, 194–205. doi: 10.1016/j.agee.2017.02.025
Dos Santos, P. C., Fang, Z., Mason, S. W., Setubal, J. C., and Dixon, R. (2012). Distribution of nitrogen fixation and nitrogenase-like sequences amongst microbial genomes. BMC Genomics 13:162. doi: 10.1186/1471-2164-13-162
Duniway, J. M. (2002). Status of chemical alternatives to methyl bromide for pre-plant fumigation of soil. Phytopathology 92, 1337–1343. doi: 10.1094/PHYTO.2002.92.12.1337
Fierer, N., Bradford, M. A., and Jackson, R. B. (2007). Toward an ecological classification of soil bacteria. Ecology 88, 1354–1364. doi: 10.1890/05-1839
Galperin, M. Y. (2013). Genome diversity of spore-forming Firmicutes. Microbiol. Spectr. 1:TBS-0015-2012. doi: 10.1128/microbiolspectrum.TBS-0015-2012
Gauri, S. S., Mandal, S. M., and Pati, B. R. (2012). Impact of azotobacter exopolysaccharides on sustainable agriculture. Appl. Microbiol. Biotechnol. 95, 331–338. doi: 10.1007/s00253-012-4159-0
Goldfarb, K. C., Karaoz, U., Hanson, C. A., Santee, C. A., Bradford, M. A., Treseder, K. K., et al. (2011). Differential growth responses of soil bacterial taxa to carbon substrates of varying chemical recalcitrance. Front. Microbiol. 2:94. doi: 10.3389/fmicb.2011.00094
Grossmann, S., Bauer, S., Robinson, P. N., and Vingron, M. (2007). Improved detection of overrepresentation of gene-ontology annotations with parentchild analysis. Bioinformatics 23, 3024–3031. doi: 10.1093/bioinformatics/btm440
Hanson, B. D., Gao, S. D., Gerik, J., Qin, R. J., Cabrera, J. A., Jhala, A. J., et al. (2013). Preplant 1,3-D treatments test well for perennial crop nurseries, but challenges remain. Calif. Agric. 67, 181–189. doi: 10.3733/ca.E.v067n03p181
Hewavitharana, S. S., and Mazzola, M. (2016). Carbon source-dependent effects of anaerobic soil disinfestation on soil microbiome and suppression of Rhizoctonia solani AG-5 and Pratylenchus penetrans. Phytopathology 106, 1015–1028. doi: 10.1094/phyto-12-15-0329-r
Hewavitharana, S. S., Ruddell, D., and Mazzola, M. (2014). Carbon source-dependent antifungal and nematicidal volatiles derived during anaerobic soil disinfestation. Eur. J. Plant Pathol. 140, 39–52. doi: 10.1007/s10658-014-0442-5
Horner-Devine, M. C., and Bohannan, B. J. M. (2006). Phylogenetic clustering and overdispersion in bacterial communities. Ecology 87, S100–S108. doi: 10.1890/0012-9658(2006)87[100:Pcaoib]2.0.Co;2
Iwai, S., Weinmaier, T., Schmidt, B. L., Albertson, D. G., Poloso, N. J., Dabbagh, K., et al. (2016). Piphillin: improved prediction of metagenomic content by direct inference from human microbiomes. PLoS ONE 11:e0166104. doi: 10.1371/journal.pone.0166104
Jones, C. M., Stres, B., Rosenquist, M., and Hallin, S. (2008). Phylogenetic analysis of nitrite, nitric oxide, and nitrous oxide respiratory enzymes reveal a complex evolutionary history for denitrification. Mol. Biol. Evol. 25, 1955–1966. doi: 10.1093/molbev/msn146
Jones, R. T., Robeson, M. S., Lauber, C. L., Hamady, M., Knight, R., and Fierer, N. (2009). A comprehensive survey of soil acidobacterial diversity using pyrosequencing and clone library analyses. ISME J. 3, 442–453. doi: 10.1038/ismej.2008.127
Kozich, J. J., Westcott, S. L., Baxter, N. T., Highlander, S. K., and Schloss, P. D. (2013). Development of a dual-index sequencing strategy and curation pipeline for analyzing amplicon sequence data on the MiSeq Illumina sequencing platform. Appl. Environ. Microbiol. 79, 5112–5120. doi: 10.1128/aem.01043-13
Lennon, J. T., and Jones, S. E. (2011). Microbial seed banks: the ecological and evolutionary implications of dormancy. Nat. Rev. Microbiol. 9, 119–130. doi: 10.1038/nrmicro2504
Louis, P., Duncan, S. H., McCrae, S. I., Millar, J., Jackson, M. S., and Flint, H. J. (2004). Restricted distribution of the butyrate kinase pathway among butyrate-producing bacteria from the human colon. J. Bacteriol. 186, 2099–2106. doi: 10.1128/jb.186.7.2099-2106.2004
Love, M. I., Huber, W., and Anders, S. (2014). Moderated estimation of fold change and dispersion for RNA-seq data with DESeq2. Genome Biol. 15:550. doi: 10.1186/s13059-014-0550-8
Luque-Almagro, V. M., Gates, A. J., Moreno-Vivian, C., Ferguson, S. J., Richardson, D. J., and Roldan, M. D. (2011). Bacterial nitrate assimilation: gene distribution and regulation. Biochem. Soc. Trans. 39, 1838–1843. doi: 10.1042/bst20110688
Martin, F. N. (2003). Development of alternative strategies for management of soilborne pathogens currently controlled with methyl bromide. Annu. Rev. Phytopathol. 41, 325–350. doi: 10.1146/annurev.phyto.41.052002.095514
McMurdie, P. J., and Holmes, S. (2013). phyloseq: an R package for reproducible interactive analysis and graphics of microbiome census data. PLoS ONE 8:e61217. doi: 10.1371/journal.pone.0061217
Messiha, N. A. S., van Diepeningen, A. D., Wenneker, M., van Beuningen, A. R., Janse, J. D., Coenen, T. G. C., et al. (2007). Biological soil disinfestation (BSD), a new control method for potato brown rot, caused by Ralstonia solanacearum race 3 biovar 2. Eur. J. Plant Pathol. 117, 403–415. doi: 10.1007/s10658-007-9109-9
Momma, N. (2008). Biological soil disinfestation (BSD) of soilborne pathogens and its possible mechanisms. Jpn. Agric. Res. Q. 42, 7–12. doi: 10.6090/jarq.42.7
Momma, N., Kobara, Y., and Momma, M. (2011). Fe2+ and Mn2+, potential agents to induce suppression of Fusarium oxysporum for biological soil disinfestation. J. Gen. Plant Pathol. 77, 331–335. doi: 10.1007/s10327-011-0336-8
Momma, N., Kobara, Y., Uematsu, S., Kita, N., and Shinmura, A. (2013). Development of biological soil disinfestations in Japan. Appl. Microbiol. Biotechnol. 97, 3801–3809. doi: 10.1007/s00253-013-4826-9
Momma, N., Yamamoto, K., Simandi, P., and Shishido, M. (2006). Role of organic acids in the mechanisms of biological soil disinfestation (BSD). J. Gen. Plant Pathol. 72, 247–252. doi: 10.1007/s10327-006-0274-z
Mowlick, S., Hirota, K., Takehara, T., Kaku, N., Ueki, K., and Ueki, A. (2012). Development of anaerobic bacterial community consisted of diverse clostridial species during biological soil disinfestation amended with plant biomass. Soil Sci. Plant Nutr. 58, 273–287. doi: 10.1080/00380768.2012.682045
Mowlick, S., Takehara, T., Kaku, N., Ueki, K., and Ueki, A. (2013). Proliferation of diversified clostridial species during biological soil disinfestation incorporated with plant biomass under various conditions. Appl. Microbiol. Biotechnol. 97, 8365–8379. doi: 10.1007/s00253-012-4532-z
Nipperess, D., and Wilson, P. (2017). PDcalc: An Implementation of the Phylogenetic Diversity (PD) Calculus in R. 0.3.1.9 ed. Available online at: https://github.com/davidnipperess/PDcalc
Nipperess, D. A., Faith, D. P., and Barton, K. (2010). Resemblance in phylogenetic diversity among ecological assemblages. J. Veg. Sci. 21, 809–820. doi: 10.1111/j.1654-1103.2010.01192.x
Oksanen, J., Blanchet, F. G., Kindt, R., Legendre, P., Minchin, P. R., O'Hara, R. B., et al. (2016). Vegan: Community Ecology Package. R package version 2.3-5 ed. Available online at: https://CRAN.R-project.org/package=vegan
Oren, A. (2014). “The family Rhodocyclaceae,” in The Prokaryotes, 4th Edn., eds. E. Rosenberg, E. F. DeLong, S. Lory, E. Stackebrandt, and F. Thompson (New York, NY: Springer), 975–998.
Pett-Ridge, J., and Firestone, M. K. (2005). Redox fluctuation structures microbial communities in a wet tropical soil. Appl. Environ. Microbiol. 71, 6998–7007. doi: 10.1128/aem.71.11.6998-7007.2005
Philippot, L., Andersson, S. G. E., Battin, T. J., Prosser, J. I., Schimel, J. P., Whitman, W. B., et al. (2010). The ecological coherence of high bacterial taxonomic ranks. Nat. Rev. Microbiol. 8, 523–529. doi: 10.1038/nrmicro2367
Price, M. N., Dehal, P. S., and Arkin, A. P. (2010). FastTree 2-approximately maximum-likelihood trees for large alignments. PLoS ONE 5:9490. doi: 10.1371/journal.pone.0009490
Pruesse, E., Peplies, J., and Glockner, F. O. (2012). SINA: accurate high-throughput multiple sequence alignment of ribosomal RNA genes. Bioinformatics 28, 1823–1829. doi: 10.1093/bioinformatics/bts252
Quast, C., Pruesse, E., Yilmaz, P., Gerken, J., Schweer, T., Yarza, P., et al. (2013). The SILVA ribosomal RNA gene database project: improved data processing and web-based tools. Nucleic Acids Res. 41, D590–D596. doi: 10.1093/nar/gks1219
Rahman, M., Khatun, A., Liu, L., and Barkla, B. J. (2018). Brassicaceae mustards: traditional and agronomic uses in Australia and New Zealand. Molecules 23:E231. doi: 10.3390/molecules23010231
Richter, H., Molitor, B., Diender, M., Sousa, D. Z., and Angenent, L. T. (2016). A narrow pH range supports butanol, hexanol, and octanol production from syngas in a continuous co-culture of Clostridium ljungdahlii and Clostridium kluyveri with in-line product extraction. Front. Microbiol. 7:1773. doi: 10.3389/fmicb.2016.01773
Rodrigues, R. R., Rodgers, N. C., Wu, X. W., and Williams, M. A. (2018). COREMIC: a web-tool to search for a niche associated CORE MICrobiome. PeerJ 6:4395. doi: 10.7717/peerj.4395
Saunders, R. M. (1985). Rice bran: composition and potential food uses. Food Rev. Int. 1, 465–495. doi: 10.1080/87559128509540780
Schneider, S. M., Rosskopf, E. N., Leesch, J. G., Chellemi, D. O., Bull, C. T., and Mazzola, M. (2003). United States Department of Agriculture–agricultural research service research on alternatives to methyl bromide: pre-plant and post-harvest. Pest Manag. Sci. 59, 814–826. doi: 10.1002/ps.728
Shennan, C., Muramoto, J., Koike, S., Baird, G., Fennimore, S., Samtani, J., et al. (2018). Anaerobic soil disinfestation is an alternative to soil fumigation for control of some soilborne pathogens in strawberry production. Plant Pathol. 67, 51–66. doi: 10.1111/ppa.12721
Shennan, C., Muramoto, J., Lamers, J., Mazzola, M., Rosskopf, E. N., Kokalis-Burelle, N., et al. (2014). Anaerobic soil disinfestation for soil borne disease control in strawberry and vegetable systems: current knowledge and future directions. Acta Hortic. 1044, 165–175. doi: 10.17660/ActaHortic.2014.1044.20
Shrestha, U., Auge, R. M., and Butler, D. M. (2016). A meta-analysis of the impact of anaerobic soil disinfestation on pest suppression and yield of horticultural crops. Front. Plant Sci. 7:1254. doi: 10.3389/fpls.2016.01254
Strauss, S. L., Greenhut, R. F., McClean, A. E., and Kluepfel, D. A. (2017). Effect of anaerobic soil disinfestation on the bacterial community and key soilborne phytopathogenic agents under walnut tree-crop nursery conditions. Plant Soil 415, 493–506. doi: 10.1007/s11104-016-3126-4
Strauss, S. L., and Kluepfel, D. A. (2015). Anaerobic soil disinfestation: a chemical-independent approach to pre-plant control of plant pathogens. J. Integr. Agric. 14, 2309–2318. doi: 10.1016/s2095-3119(15)61118-2
Team, R. C. (2017). R: A Language and Environment for Statistical Computing. Vienna: R Foundation for Statistical Computing.
Teclu, D., Tivchev, G., Laing, M., and Wallis, M. (2009). Determination of the elemental composition of molasses and its suitability as carbon source for growth of sulphate-reducing bacteria. J. Hazard. Mater. 161, 1157–1165. doi: 10.1016/j.jhazmat.2008.04.120
Villemur, R., Lanthier, M., Beaudet, R., and Lepine, F. (2006). The Desulfitobacterium genus. FEMS Microbiol. Rev. 30, 706–733. doi: 10.1111/j.1574-6976.2006.00029.x
von Mering, C., Hugenholtz, P., Raes, J., Tringe, S. G., Doerks, T., Jensen, L. J., et al. (2007). Quantitative phylogenetic assessment of microbial communities in diverse environments. Science 315, 1126–1130. doi: 10.1126/science.1133420
Wang, Q., Garrity, G. M., Tiedje, J. M., and Cole, J. R. (2007). Naive Bayesian classifier for rapid assignment of rRNA sequences into the new bacterial taxonomy. Appl. Environ. Microbiol. 73, 5261–5267. doi: 10.1128/aem.00062-07
Ward, N. L., Challacombe, J. F., Janssen, P. H., Henrissat, B., Coutinho, P. M., Wu, M., et al. (2009). Three genomes from the phylum Acidobacteria provide insight into the lifestyles of these microorganisms in soils. Appl. Environ. Microbiol. 75, 2046–2056. doi: 10.1128/aem.02294-08
Keywords: anaerobic soil disinfestation, plant pathogen control, organic amended soils, soil microbial communities, tree crop agriculture
Citation: Poret-Peterson AT, Albu S, McClean AE and Kluepfel DA (2019) Shifts in Soil Bacterial Communities as a Function of Carbon Source Used During Anaerobic Soil Disinfestation. Front. Environ. Sci. 6:160. doi: 10.3389/fenvs.2018.00160
Received: 07 September 2018; Accepted: 18 December 2018;
Published: 09 January 2019.
Edited by:
Luiz Fernando Wurdig Roesch, Federal University of Pampa, BrazilReviewed by:
Suvendu Das, Gyeongsang National University, South KoreaAfnan Khalil Ahmad Suleiman, Netherlands Institute of Ecology (NIOO-KNAW), Netherlands
Copyright © 2019 Poret-Peterson, Albu, McClean and Kluepfel. This is an open-access article distributed under the terms of the Creative Commons Attribution License (CC BY). The use, distribution or reproduction in other forums is permitted, provided the original author(s) and the copyright owner(s) are credited and that the original publication in this journal is cited, in accordance with accepted academic practice. No use, distribution or reproduction is permitted which does not comply with these terms.
*Correspondence: Amisha T. Poret-Peterson, YW1pc2hhLnBvcmV0cGV0ZXJzb25AYXJzLnVzZGEuZ292
†Present Address: Sebastian Albu, California Department of Food and Agriculture, Plant Pest Diagnostics Center, Sacramento, CA, United States