- 1School of Biotechnology and Biomolecular Sciences, UNSW Sydney, Randwick, NSW, Australia
- 2Department of Biological Sciences, Macquarie University, Sydney, NSW, Australia
- 3Australian Antarctic Division, Antarctic Conservation and Management, Kingston, TAS, Australia
Quantification of microbial functional genes enhances predictions of soil biogeochemical process rates, but reliance on low-throughput quantitative PCR (qPCR) limits the scope of ecological studies to a handful of targets. Here, we explore whether microfluidic qPCR (MFQPCR) is a viable high-throughput alternative for functional gene quantification, by evaluating the efficiency, specificity and sensitivity of 29 established and 12 newly designed primer pairs targeting taxonomic, nitrogen-cycling, and hydrocarbon degradation genes in genomic DNA soil extracts, under three different sets of MFQPCR assay conditions. Without curation, commonly-used qPCR primer pairs yielded an extreme range of reaction efficiencies (25.9–100.1%), but when conditions were optimized, MFQPCR produced copy-number estimates comparable to traditional qPCR. To guide microbial soil ecologists considering adoption of MFQPCR, we present suggestions for primer selection, including omission of inosines, degeneracy scores of < 9, amplicon sizes of ≤ 211 bp, and GC content of 32–61%. We conclude that, while the nanoliter reaction volumes, rapid thermocycling and one-size-fits-all reaction conditions of MFQPCR necessitates more stringent primer selection criteria than is commonly applied in soil microbial ecology, the ability to quantify up to 96 targets in 96 samples makes MFQPCR a valuable tool for monitoring shifts in functional community abundances. MFQPCR will particularly suit studies targeting multiple clade-specific functional genes, or when primer design is informed by previous knowledge of the environment.
Introduction
Soil microbial communities perform a dazzling array of ecosystem services, from fixation of atmospheric nitrogen and the release of nutrients from rocks (Landeweert et al., 2001; Levy-Booth et al., 2014), to the degradation of organic pollutants and pesticides (Molina et al., 2009; Kumar et al., 2016). Advances in genomic technologies are rapidly expanding our knowledge of the genetic and taxonomic diversity of microbial communities, unearthing new biogeochemical pathways and revealing whole clades of undescribed bacteria, at a pace that quantification technologies have struggled to keep up with (Jones et al., 2013; Hu et al., 2015; Widder et al., 2016; Kuypers et al., 2018). While high-throughput sequencing has become the mainstay of many microbial ecology and ecotoxicology laboratories, low-throughput quantitative PCR (qPCR) remains the method of choice for accurate quantification of total cell numbers, individual species or functional genes. In soil the quantification of microbial functional genes enhances the prediction of biogeochemical process rates (Hallin et al., 2009; Petersen et al., 2012; Bier et al., 2015; Powell et al., 2015; Graham et al., 2016; Breuillin-Sessoms et al., 2017), but traditional qPCR is laborious and costly, severely limiting the scope of microbial ecology investigations to quantification of just a handful of target genes.
Quantitative PCR uses DNA-complexing fluorophores and real-time detection to quantify the number of gene copies present in an individual reaction and is currently the gold-standard of gene quantification. By including a linear range of standards with known numbers of gene copies, qPCR is used to estimate starting concentrations of the target gene, often down to 101 copies/ μL (Smith and Osborn, 2009). In recent years a number of alternatives to traditional qPCR have arisen which drastically improve throughput by either miniaturizing, automating, digitalizing or multiplexing reactions (Baker, 2010; Huggett et al., 2013). However, these methods do not simultaneously match both the accuracy and flexibility of qPCR. The most promising technologies that are addressing this gap include microarrays such as Geochip, which uses hybridization of an array of > 20,000 probes to semi-quantitate thousands of gene variants in a small number of samples (He et al., 2010); digital PCR, which uses sample partitioning into droplets or nanoliter chambers to detect individual copies of rare targets (Baker, 2012); and microfluidic qPCR (MFQPCR), which uses nanoliter reaction volumes and a system of pressurized valves and microfluidic channels to automate the mixing and thermocycling of up to 96 assays and 96 samples in a single chip (Spurgeon et al., 2008). While microarrays have proven a powerful tool for screening soils for the presence of functional genes (Yergeau et al., 2007), and digital PCR has its niche in detecting a few targets with high sensitivity, both are prohibitively expensive for ecological studies which require the analysis of large numbers of individual samples. MFQPCR has emerged as an attractive alternative, being cost-effective, easily customizable, and as it uses the same chemistry as traditional qPCR, theoretically produces directly comparable results (Miller et al., 2016).
First developed for reverse-transcriptase quantification of gene expression in humans (Spurgeon et al., 2008), Kleyer et al. (2017) recently reported the application of MFQPCR to quantify specific soil bacteria using species-specific primers in batch cultures and sterile sand. MFQPCR has also been successfully applied to environmental samples, detecting pathogenic bacteria and viruses in aquatic environments and in salmon (Ishii et al., 2013, 2014a,b; Byappanahalli et al., 2015; Miller et al., 2016; Bass et al., 2017; Sadik et al., 2017). These studies demonstrated that MFQPCR is sensitive to 2 copies/μL (Ishii et al., 2014a), is specific enough to distinguish between serotypes (Dhoubhadel et al., 2014), and per assay costs are less than half that of conventional qPCR (Ishii et al., 2014a; Miller et al., 2016). Until now, however, these studies have endeavored to use assays which conform to the parameters established in MFQPCR's development as a tool for quantification of gene expression in humans (Spurgeon et al., 2008). That is, using primer sets directed to highly specific target genes for quantification in relatively homogenous, high purity samples that have been purified of PCR-inhibiting contaminants.
Microfluidic qPCR achieves its high-throughput capacity by sacrificing much of the flexibility of qPCR. Recommended MQPCR conditions include primer pairs that are free from degeneracy, produce short amplicons of < 100 bp, and have melt temperatures close to 60°C, thereby enabling up to 96 assays to be run simultaneously, using identical reagent concentrations and thermocycling conditions (Spurgeon et al., 2008). Conversely, due to its low-throughput nature, traditional qPCR allows for assay-by-assay modifications of reaction conditions to suit specific primer pairs and sample types. This flexibility is particularly valuable in the quantification of microbial functional genes, where maximal coverage, rather than specificity, is desired (Gaby and Buckley, 2012). As microbial functional communities are taxonomically diverse, with several distinct clades performing a single substrate transformation, primers targeting a specific gene in one species have little predictive value for determining process rates in the whole community. Instead, degenerate primers are often used to target a single gene in one or multiple clades, with qPCR conditions optimized to ensure acceptable reaction efficiencies (Iwai et al., 2011; Wei et al., 2015; Gaby and Buckley, 2017). Other commonly used deviations from qPCR ‘best-practice’ include longer amplicons to straddle an active site combined with extended elongation times (Baldwin et al., 2003; Lueders and Von Netzer, 2017), the combination of mismatched bases and lower annealing temperatures (Ishii and Fukui, 2001; Frank et al., 2008; Edwards et al., 2011), and the use of additives to combat inhibitors such as humic acids present in soil DNA extracts (Dandie et al., 2007). Given the need for flexibility in primer design and the inability to customize individual assay conditions when using MFQPCR, it is necessary to establish what the boundaries of primer variability are for MFQPCR before it can be considered a high-throughput alternative for the quantification of microbial functional genes in environmental samples.
The aim of this study was to determine whether established qPCR primer pairs targeting microbial functional genes in soil could be applied to MFQPCR with comparable accuracy. As preliminary research showed a high assay failure rate (Crane, 2016), we aimed to determine which primer design parameters were crucial to assay success, by assessing performance of a wide variety of nitrogen cycle and hydrocarbon degradation primers under different combinations of primer concentrations and MFQPCR thermocycling conditions. We used soil DNA extracts from ongoing hydrocarbon ecotoxicology studies in subantarctic and Antarctic soils (Crane, 2016; Mcwatters et al., 2016) to evaluate the sensitivity, specificity and reaction efficiency of 29 established and 12 newly designed primer pairs and three sets of MFQPCR assay conditions. We then evaluated MFQPCR accuracy by comparing MFQPCR and qPCR gene abundance estimates for a subset of genes and soil samples.
Materials and Methods
Soil Samples and DNA Extraction
To evaluate MFQPCR reaction efficiencies, we used 217 soil DNA extracts from hydrocarbon ecotoxicology studies conducted on subantarctic Macquarie Island (Crane, 2016), and at Casey station, East Antarctica (Mcwatters et al., 2016). Samples from Macquarie Island were collected from a two-year in situ mesocosm study investigating the effect of a residual hydrocarbon mixture (spiked into clean soils) on native microbial soil communities. Samples from four separate biopiles at Casey Station were collected as part of a large-scale remediation project to evaluate hydrocarbon biodegradation rates and the effects of the active remediation treatments on the indigenous microbial population over 5 years. Soil samples (50g) were collected in sterile plastic 50 ml tubes, immediately sealed and stored at −20°C until analyzed. Total community genomic DNA (gDNA) was extracted in triplicate from 0.3 to 0.5 g soil using the FastDNA SPIN Kit for soil (MP Biomedicals) and quantified spectrophotometrically using the PicoGreen double-strand DNA kit (Life Technologies) on the ClarioSTAR® microplate reader (BMG Labtech). DNA lysates were diluted in nuclease- free water; Macquarie Island samples to an optimal range of 7–8 ng μL−1, (min. 3.3 ng μL−1, max 10.7 ng μL−1) and Casey Station samples were all diluted 10-fold. An inter-plate calibrator (IPC) was generated by mixing 10 randomly selected Macquarie Island gDNA extracts to a final concentration of 8.7 ng μL−1.
Primers and Standard Generation
Primers targeting universal genes for Fungi, Bacteria, Archaea, Acidobacteria, and Betaproteobacteria were selected from the literature, in addition to a suite of primers for nitrogen cycling and hydrocarbon degrading genes in Bacteria and Archaea (Table 1). In accordance with qPCR best practice (Rodríguez et al., 2015), primer pairs with minimal degeneracy and small amplicon sizes were preferentially selected. Twelve additional nitrogen cycle primers with no degeneracy and amplicon sizes of < 200 bp were designed with PRISE2 (Huang et al., 2014) using sequences downloaded from FunGene (Fish et al., 2013) and GenBank. Standards for copy number quantification were generated either by PCR amplification of environmental gDNA (Shahsavari et al., 2016) or through artificially synthesized gBlock Gene Fragments (Integrated DNA Technologies) (Table 2). Representative sequences for gBlock standards were sourced from NCBI using Primer-BLAST (Table S3) (Ye et al., 2012) and each gBlock comprised of five different standard sequences. For PCR-derived standards, PCR reactions were conducted in 25 μL volumes containing 1 μL template, 1x GoTaq Flexi Buffer; pH 8.5 (Promega), 400 nM each primer (Integrated DNA Technologies), 250 μM each dNTP (Bioline), 160 μg ml-1 BSA, 0.625 U GoTaq polymerase (Promega) and optimized concentrations of MgCl2 (Promega) (Table S4). Thermocycling conditions consisted of 94°C for 2 min, then 35 cycles of 94°C for 45 s, annealing for 45 s, 72°C for 45 s, with a final extension at 72°C for 10 min. Annealing temperatures were optimized for each primer pair (Table S4). PCR products were purified using QIAquick PCR purification columns (QIAGEN) and quantified spectrophotometrically. Copy numbers were calculated and standard curves generated using serial dilution from 102 to 109 copies/μL. Standards were pooled for use with MFQPCR, with final concentrations of 102-108 copies/μL for EUK and Eub, and 101-107 copies/μL for all other assays.
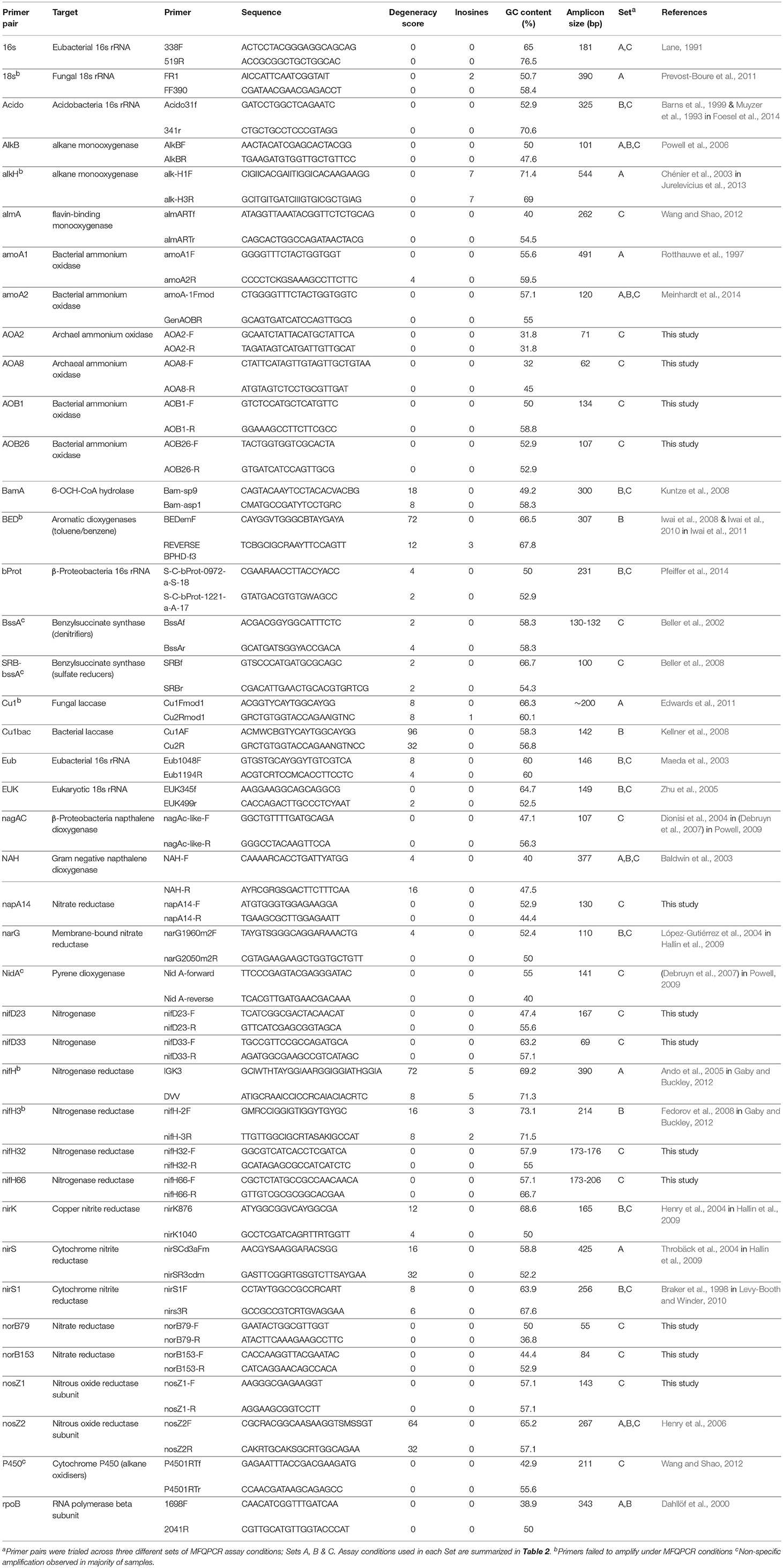
Table 1. Primers pairs targeting taxonomic, nitrogen-cycling and hydrocarbon degradation genes selected for this study.
MFQPCR
MFQPCR assays were run in three separate sets using Evagreen® chemistry and two different Fluidigm Dynamic Array™ Integrated Fluidic Circuits (IFCs): A single 48.48 IFC (Set A), three 48.48 IFCs (Set B), and a single 96.96 IFC (Set C). Primer selection (Table 1), primer concentration, extension times, sample source, and method of standard generation were different for each of the three Sets used (Table 2). An inter-plate calibrator sample (IPC) was run in triplicate across the three 48.48 IFCs in Set B (Bi, Bii, and Biii) to enable the evaluation of intra- and inter-run variation. Specific target amplification (STA) and MFQPCR were conducted at the Ramaciotti Center for Genomics (UNSW Australia, Sydney, Australia). Samples (gDNA) and 7-point standards were pre-amplified with a 50 nM primer pool using TaqMan PreAmp Master Mix (ThermoFischer Scientific). STA cycling conditions were 95°C for 2 min, then 14 cycles of 96°C for 15 s and 60°C for 4 min. Products were treated with 8 U Exonuclease I (New England Biolabs) at 37°C for 30 min and 80°C for 15 min, diluted 1 in 5 with DNA suspension buffer (TEKnova) and stored at −20°C overnight. For MFQPCR, gDNA and assays were loaded into the reaction chambers of a 48.48 or 96.96 IFC using an MX or HX IFC controller respectively, according to the manufacturer's Evagreen® protocol. The array was then placed in a BioMark HD™ for thermo-cycling; 95°C for 1 min, followed by 35 cycles of 96°C for 5 s and 60°C for 20 s or 25 s (Table 2), followed by melt curve analysis for 60–95°C at a ramp rate of 1°C/3 s.
MFQPCR Data Analysis
Data were analyzed using the Real-Time PCR Analysis software, version 4.1.2 (Fluidigm), using default quality threshold of 0.65 and linear baseline correction. Peak sensitivity was set at 7, peak ratio threshold at 0.7, and melt temperature (Tm) ranges were set individually based on the peaks observed in standards, as per the manufacturers' recommendations. Both Tm ranges and threshold cycle (Ct) values were manually normalized to the mean across intra-chip replicate assays. Individual reactions were excluded from analysis if they failed any of the melt curve quality parameters, were outside 0.5 Ct of other replicates, or had a peak outside the set Tm range. Calibration curves were created in the Calibration Curve View Module using the known copy numbers in standards, and the R2 calculated from an OLS regression for each assay. Calibrated relative concentrations were then exported to MS Excel for conversion to copies/g of soil, analysis and modeling.
Traditional qPCR
Traditional qPCR was conducted with three primer pairs; amoA, narG, and bamA, for comparison with MFQPCR results obtained for Set B. Reactions were carried out using optimized qPCR conditions; in 20 μL volumes containing 1 × QuantiFast SYBR Green PCR Master Mix (Qiagen), 500 nM each primer, and 1.25 μL template gDNA. Each 96-well plate consisted of a 7-point standard curve (102-108 copies/μL), no-template control (NTC), inter-plate calibrator sample (IPC) and 23 randomly selected samples, all in triplicate. Thermocycling was conducted with a CFX96 TouchTM Real-Time PCR Detection System (Bio-Rad) with a hot start of 95°C for 5 min, followed by 40 cycles of 94°C for 20 s and 60°C for 50 s, and melt curve analysis from 50 to 95°C at a ramp rate of 0.5°C/5 s. Analysis of qPCR data were conducted with the CFX manager software (Bio-Rad). Replicates with >0.5 Ct variation were examined, and outliers discarded. Specificity was confirmed with melt peak analysis and reactions were discarded if non-specific amplification was evident. The average Ct values across replicates were determined and copy numbers were calculated based on linear regression of the standard curve. Standard curve efficiencies and copy numbers were converted to copies/g of soil for subsequent analysis.
Analysis of Reaction Efficiencies
Mean reaction efficiencies (percentage increase of template in each round of thermocycling) of samples and standards were calculated from observed increases in fluorescence using the LinRegPCR program (version 2015.3) (Ramakers et al., 2003). For qPCR data, non-baseline corrected data were exported from the CFX manager software and the raw fluorescence values imported into LinRegPCR. For microfluidic data, as the Fluidigm software does not allow for the export of raw fluorescence data, a constant baseline was first applied in the Real-Time PCR Analysis software version 4.1.2 (Fluidigm), and the data reanalyzed. Normalized fluorescence intensity values for all samples or standards, to 20 decimal places, were exported gene by gene, which does not allow for the identification of individual reactions but allows for group analysis of all samples or standards for each assay. Computation of efficiencies in LinRegPCR was conducted as per the program instructions (Ramakers et al., 2003). Noisy samples, where a continuous increase could not be identified, were excluded from “Window of Linearity” calculations and “strictly continuous log-linear phase” criteria was applied to baseline estimations. Samples were also excluded from mean efficiency calculations if they had no plateau. In accordance with qPCR best practice, we considered reaction efficiencies over 90% to be optimal (Rodríguez et al., 2015).
Results
Evaluation of MFQPCR Assay Quality
In this study, we used three different sets of assay conditions (Sets A, B, and C), to evaluate variations in primer concentrations, extension times, soil samples, methods of standard generation, and the size and number of IFC chips used (Table 2). In total 41 qPCR pairs were evaluated, 29 of which were selected from the literature as they had been used previously in qPCR (Table 1). Additionally, 12 nitrogen cycle primer pairs with no degeneracy and amplicons of < 200 bp in length were designed here. Overall, primer degeneracy ranged from 0 to 96, and amplicon sizes from 55 to 544 bp. Other factors, such as GC content, melt temperature, homo-, and hetero- dimer complementarity, were not considered in the selection of primers but were also found to be variable (Table S1).
MFQPCR reaction efficiencies (Figure 1), were highly variable, ranging from 25.9% (Cu1, Set A) to 100.1% (EUK, Set B), with five assays failing completely to amplify the target sequence under MFQPCR conditions (18s, alkH, BED, nifH, nifH3). All five failed assays contained at least one inosine residue in either the forward or reverse primer, and exclusion of primers containing inosines in Set C eradicated further assay failure. Moderate negative correlations between efficiency and amplicon size (r = 0.45) and degeneracy (r = 0.38) were observed when data from the three Sets were pooled (Figures 2A,B). Of the other primer characteristics examined, weak, non-linear relationships with reaction efficiency were detected (Figures 2C–E). While the GC content of gBlock standards, analyzed in Set C, had a moderate negative correlation with reactionefficiency (r = −0.66, Figure 2F).
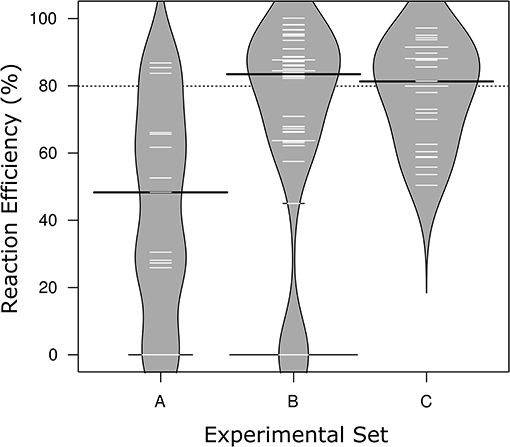
Figure 1. Distribution of reaction efficiencies across the three Sets of MFQPCR assay conditions. Higher primer concentrations in Sets B & C corresponded with improved efficiencies, and exclusion of primers with inosines corresponds with the elimination of failed/zero efficiencies in Set C. Further differences in assay conditions are outlined in Table 2. Black lines show the medians; white lines represent mean efficiency score of individual assays (n = 15, 48, 27, respectively); polygons represent the estimated density of the data. Efficiencies are based on standards only.
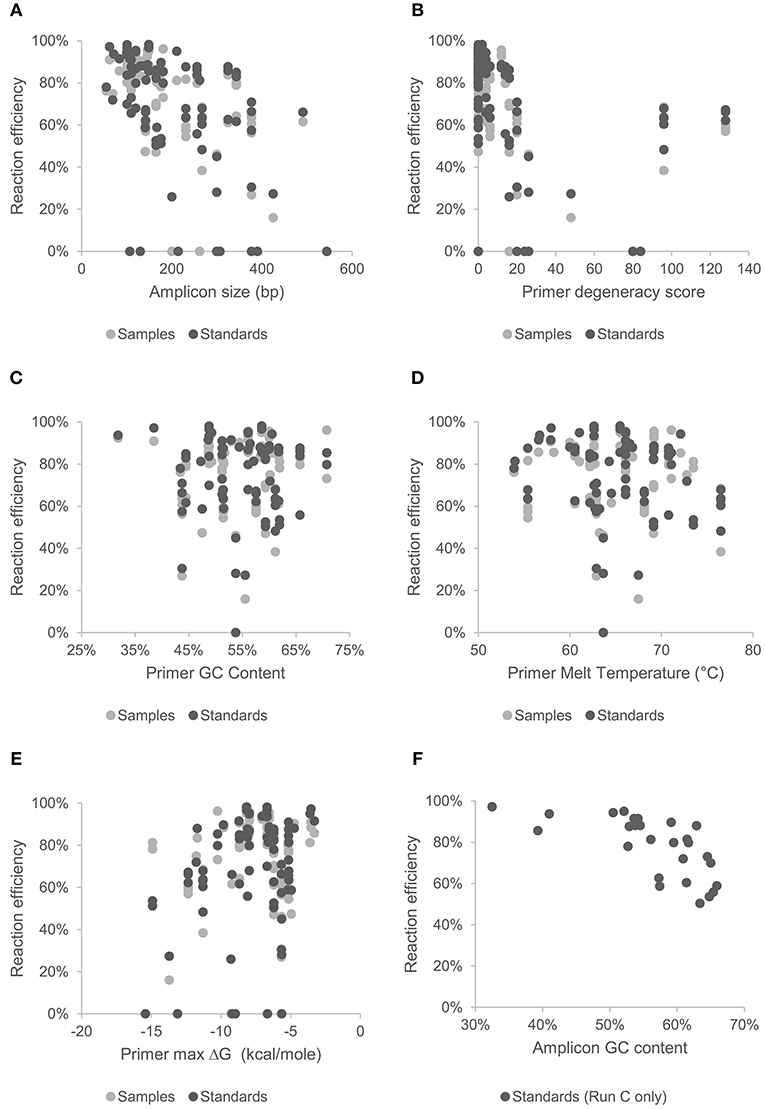
Figure 2. Effect of amplicon size (A), and primer characteristics; degeneracy (B), GC content (C), melt temperature (D), and hetero-dimer ΔG (E), on mean reaction efficiency of samples and standards across all chips and assays. Effect of GC content of gBlock artificially synthesized standards (Set C) on reaction efficiency are shown in (F).
Reaction efficiencies exhibited a strong Set effect, reflecting a combination of both assay conditions and primer selection, with the highest median efficiency observed in Set B (Figure 1). Increased primer concentrations in Set B (700 nM) compared to Set A (500 nM) improved efficiency of all assays used in both Sets. However, results varied widely from an improvement of 5% for amoA2 through to 29.1% nirK indicating that in Set A, primer concentration was limiting the reaction. In Set C, efficiencies of several assays were lower than in Set B, likely due to the smaller reaction volumes (6.7 nl vs. 10.1 nl) and the GC content of the gBlock standards used. The addition of T4 gene 32 to a subset of six samples and two assays (amoA2 and nifH66) in Set C did not produce a detectable effect on efficiencies or copy numbers compared to samples without this additive. Across the three experiments, all assays exhibited similar reaction efficiencies for standards and samples, except for four assays in Set C (AlkB, nirS1, nifH66, and nifD23; Table S2). Efficiencies in these four assays were 21–28% lower in standards compared to samples, reflecting a discordance between the GC rich representative sequences used in the gBlock, and those present in the environmental samples. Characteristics of MFQPCR assays displaying optimal efficiencies (≥ 90%) are summarized in Box 1.
Box 1. What makes an optimal MFQPCR assay?
The following characteristics defined assays in this study with efficiencies greater than 90%:
□ Amplicon length of 62 - 211 bp
□ Individual degeneracy score of 0 - 8
□ Combined degeneracy score (F+R) of 0 - 12
□ No inosine residues
□ GC content of 32 - 61%
□ Predicted melt temperature of 57 - 71°C
□ Hetero-dimer ΔG of (-9.8) – (-3.5)
□ GC content of standards 33 - 59%
□ Primer concentration of 700 nM
□ Soil gDNA extracts diluted ≥ 5-fold
Standard curves were linear over between 4 and 7 orders of magnitude, with sensitivity ranging from 101 to 104 copies/μL and R2 values from 0.919 to 1.000. In Set A, 13 samples exceeded detection limits for 16S, and in one chip of Set B the maximal value of accurate detection for AlkB, amoA2, Cu1bac, Eub, and narG was 106 copies/μL, and 105 for EUK. Amplification in all other standards and samples fell within the maximal range of detection. Low levels of non-specific amplification in the NTC was observed for 16S in Set A and Bprot and Eub in Set C, but in all cases amplification was > 5 Ct below all standards and samples analyzed. Non-specific amplification, determined by melt curve analysis, was observed in most samples for BssA, NidA, SRB-BssA, and P450, suggesting these assays were lacking specificity. An increase in primer concentration in Sets B & C did not appear to be associated with any increase in non-specific amplification. The intra-run coefficient of variance ranged from 0% to 26.5%, and the inter-run coefficient of variance ranged from 2.3% to 24.1% (Figure S1).
Comparison of MFQPCR and qPCR
Three primer pairs; amoA2, narG, and bamA, were used in a comparison between traditional and microfluidic qPCR. Mean efficiencies of standards and samples were higher with MFQPCR for amoA2 and narG, and slightly lower for bamA (Figure S2). BamA had very low efficiencies in both qPCR and MFQPCR assays and most samples failed melt-curve analysis due to the presence of an additional peak that did not correspond to that observed for the standards (Figure S3). MFQPCR and qPCR copy number estimates for amoA2 and narG were within one order of magnitude of each other for samples diluted ≥ 4-fold (Figure 3), while MFQPCR estimates were substantially lower than those of qPCR for samples at dilution factors of 3 or less. These results indicated that MFQPCR was more sensitive to the presence of inhibitors compared to traditional qPCR, and samples should be diluted accordingly.
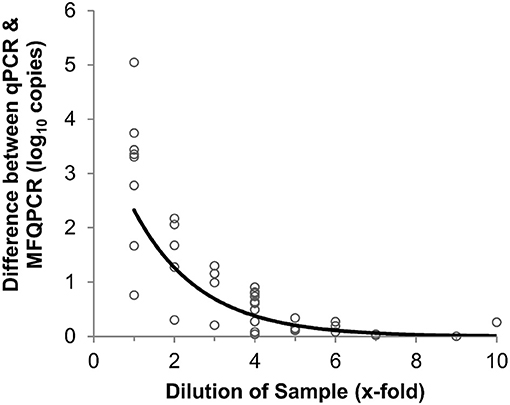
Figure 3. Effect of sample dilution on accuracy of MFQPCR copy number estimates. Large discrepancies between qPCR and MFQPCR estimates are evident when gDNA soil extracts are diluted 4-fold or less. Data points are a combination of estimates for amoA2 and narG under Set B assay conditions (summarized in Table 2).
Discussion
Analyzing the efficiencies of a wide range of published qPCR primer pairs under various run conditions and against over 200 soil gDNA extracts allowed us to determine which parameters were most essential to MFQPCR assay success (Box 1). The combination of nanoliter reaction volumes, rapid thermocycling and one-size-fits-all reaction conditions of MFQPCR required more stringent primer selection criteria, compared to common practices in environmental qPCR studies. In the case of assays targeting functional genes the most problematic requirement outlined here was for primers with degeneracy scores of ≤ 8. These constraints excluded many broad-coverage primers, which rely on degeneracy to achieve adequate coverage of taxonomically-diverse functional communities (Gaby and Buckley, 2012).
In addition to assay conditions and primer parameters, we trialed the use of artificially synthesized gBlock standards using sequences randomly selected from databases. This use of gBlock standards increased the accuracy of standard dilutions, produced greater inter-assay correlation, and reduced preparation time by eliminating the need to source representative cultures or generate amplicons from the environment. However, as the sequences used to design the gBlock standards were selected randomly, several amplicons exhibited different melt peaks and significant discrepancies in efficiencies compared to environmental samples. This introduces new inaccuracies, as differences in standard and sample efficiencies lead to an under- or over-estimation of copy numbers and hence invalidate estimation of absolute copy numbers (Ramakers et al., 2003; Bru et al., 2008).
It is important to emphasize that these guidelines are based on observed efficiencies under specific assay conditions, and alterations of thermocycling times, reagent concentrations or the use of additives could be manipulated to relax the stringent constraints outlined here (Box 1). Indeed, we found that increased primer concentrations in Sets B and C drastically improved assay efficiencies, ostensibly as it remedied the reduced primer/template ratio that results from using degenerate mixtures of primers (Figure 1) (Rose et al., 1998; Gaby and Buckley, 2017). Similarly, employing additives such as T4 gene 32 (Dandie et al., 2007) or an alternative soil DNA extraction kit (Mahmoudi et al., 2011) could potentially alleviate the inhibition observed in undiluted samples (Figure 3). The selection of chemistry is also clearly important; while we observed unusual sensitivity to inosine residues compared to those reported for qPCR (Zheng et al., 2008), this phenomenon has not been reported for inosine-containing MFQPCR assays that use TaqMan chemistry (Ishii et al., 2014a) or for Evagreen® chemistry with the Access Array™ (Oshiki et al., 2018).
While previous environmental studies using MFQPCR have examined correlations between MFQPCR and qPCR copy number estimates (Ishii et al., 2014a; Byappanahalli et al., 2015), our study is one of the first to explicitly examine individual MFQPCR assay efficiencies. In addition to the discrepancies between sample and standard efficiencies discussed above, we also observed major variation in the efficiencies of unoptimized assays (25.9- 100.1%). Neither of these sources of inaccuracy would have been detected without analysis in LinRegPCR (Ramakers et al., 2003). This finding highlights the need for MFQPCR uptake to be accompanied by uniform and transparent reporting of experimental data including efficiencies, in accordance with MIQE guidelines (Bustin et al., 2009, 2013). Efficiency analysis is currently hampered by the inability of regular users of Real-Time PCR Analysis (Fluidigm) software to export raw fluorescence data without a cumbersome work-around, which may explain the lack of efficiency reporting in most MFQPCR studies. We anticipate that with increased adoption of MFQPCR, greater emphasis will be placed on the establishment of MFQPCR ‘best-practice’ protocols, similar to the Digital MIQE guidelines for digital PCR (Huggett et al., 2013), and that qPCR efficiency analysis software like LinRegPCR will allow data importation from MFQPCR programs (Ramakers et al., 2003).
To ensure MFQPCR assays are of high-efficiency while capitalizing on the increased throughput that the platform affords, we suggest that selection of both primers and representative sequences for gBlock standards be informed by knowledge of which gene variants are present in the environment in question. This approach could be taken further, by harnessing recent advances in high-resolution melting curve (HRM) analysis, which allows the identification and quantification of multiple gene variants based on differences in melt temperatures (Hjelmso et al., 2014). In our study, assays were excluded from analysis if there was severe separation of standard and sample melt peaks. In the case of bamA, early samples and standards had a different melt peak profile to later samples (Figure S3), but the inability to confirm the identity of this new peak meant that such data were considered to be non-specific and were thus discarded. Such shifts in the abundance of different gene variants potentially reflect not non-specific amplification, but adaptation of the community. By designing gBlock standards that cover a range of gene variants, in combination with HRM, such diversity could be quantified reliably. This capability is not far off, with a Single Nucleotide Polymorphism (SNP) melting curve analysis method already available on the Fluidigm BioMark platform [e.g., (Kim et al., 2017)].
Conclusion
MFQPCR is a valuable tool for quantifying microbial functional communities in soil, provided primer pair and assay conditions are stringently curated. When optimal conditions were met, MFQPCR allowed reliable, simultaneous quantification of taxonomic, nitrogen-cycling and hydrocarbon degradation genes in over 200 gDNA extracts from subantarctic and Antarctic soils. MFQPCR will be of greatest utility if multiple low-degeneracy, clade-specific primers can be designed, or if primer selection is guided by prior knowledge of the specific environment, such as that revealed by metagenomic or predictive metagenomic surveys (Bonilla-Rosso et al., 2016; Mukherjee et al., 2017). Employed in such a fashion, MFQPCR will fill a valuable niche between high-throughput taxonomic sequencing and low-throughput functional gene qPCR, allowing accurate quantification of microbial ecosystem services, such as clade-specific resolution of microbial functional guilds involved in biogeochemical nutrient cycling. We propose that MFQPCR will be particularly useful in monitoring the response of sensitive functional groups such as ammonia-oxidizers to environmental disturbances (Van Dorst et al., 2014), or for the accurate prediction of biogeochemical process rates (Breuillin-Sessoms et al., 2017).
Author Contributions
All authors contributed to the study design. BF coordinated the study. SC and JvD carried out the experimental procedure. SC conducted the data analysis and drafted the manuscript. All authors finalized, read and approved the manuscript.
Funding
Australian Antarctic Science Grants (AAS-4135 and AAS-4036) and UNSW internal funding supported this research.
Conflict of Interest Statement
The authors declare that the research was conducted in the absence of any commercial or financial relationships that could be construed as a potential conflict of interest.
The handling editor and reviewer RF declared their involvement as co-editors in the Research Topic, and confirm the absence of any other collaboration.
Acknowledgments
The authors would like to thank all the scientists and logistical personal stationed at Macquarie Island for the 2014 & 2015 field seasons, and Casey Station field seasons 2011 - 2016. Special thanks go to Ingrid Errington for Macquarie Island sample collection, and to Tanya Raymond for comments on the manuscript.
Supplementary Material
The Supplementary Material for this article can be found online at: https://www.frontiersin.org/articles/10.3389/fenvs.2018.00145/full#supplementary-material
References
Ando, S., Goto, M., Meunchang, S., Thongra-Ar, P., Fujiwara, T., Hayashi, H., et al. (2005). Detection of nifH sequences in sugarcane (Saccharum officinarum L.) and pineapple (Ananas comosus [L.] Merr.). Soil Sci. Plant Nutri. 51, 303–308. doi: 10.1111/j.1747-0765.2005.tb00034.x
Baker, M. (2010). Clever PCR: more genotyping, smaller volumes. Nat. Methods 7, 351–356. doi: 10.1038/nmeth0510-351
Baldwin, B. R., Nakatsu, C. H., and Nies, L. (2003). Detection and enumeration of aromatic oxygenase genes by multiplex and real-time PCR. Appl. Environ. Microbiol. 69, 3350–3358. doi: 10.1128/AEM.69.6.3350-3358.2003
Barns, S. M., Takala, S. L., and Kuske, C. R. (1999). Wide distribution and diversity of members of the bacterial kingdom Acidobacterium in the environment. Appl. Environ. Microbiol. 65, 1731–1737.
Bass, A., Hinch, S., Teffer, A., Patterson, D., and Miller, K. (2017). A survey of microparasites present in adult migrating Chinook salmon (Oncorhynchus tshawytscha) in south-western British Columbia determined by high-throughput quantitative polymerase chain reaction. J. Fish Dis. 40, 453–477. doi: 10.1111/jfd.12607
Beller, H. R., Kane, S. R., Legler, T. C., and Alvarez, P. J. (2002). A real-time polymerase chain reaction method for monitoring anaerobic, hydrocarbon-degrading bacteria based on a catabolic gene. Environ. Sci. Technol. 36, 3977–3984. doi: 10.1021/es025556w
Beller, H. R., Kane, S. R., Legler, T. C., Mckelvie, J. R., Sherwood Lollar, B., Pearson, F., et al. (2008). Comparative assessments of benzene, toluene, and xylene natural attenuation by quantitative polymerase chain reaction analysis of a catabolic gene, signature metabolites, and compound-specific isotope analysis. Environ. Sci. Technol. 42, 6065–6072. doi: 10.1021/es8009666
Bier, R. L., Bernhardt, E. S., Boot, C. M., Graham, E. B., Hall, E. K., Lennon, J. T., et al. (2015). Linking microbial community structure and microbial processes: an empirical and conceptual overview. FEMS Microbiol. Ecol. 91:fiv113. doi: 10.1093/femsec/fiv113
Bonilla-Rosso, G., Wittorf, L., Jones, C. M., and Hallin, S. (2016). Design and evaluation of primers targeting genes encoding NO-forming nitrite reductases: implications for ecological inference of denitrifying communities. Sci. Rep. 6:39208. doi: 10.1038/srep39208
Braker, G., Fesefeldt, A., and Witzel, K-P. (1998). Development of PCR primer systems for amplification of nitrite reductase genes (nirK and nirS) to detect denitrifying bacteria in environmental samples. Appl. Environ. Microbiol. 64, 3769–3775.
Breuillin-Sessoms, F., Venterea, R. T., Sadowsky, M. J., Coulter, J. A., Clough, T. J., and Wang, P. (2017). Nitrification gene ratio and free ammonia explain nitrite and nitrous oxide production in urea-amended soils. Soil Biol. Biochem. 111, 143–153. doi: 10.1016/j.soilbio.2017.04.007
Bru, D., Martin-Laurent, F., and Philippot, L. (2008). Quantification of the detrimental effect of a single primer-template mismatch by real-time PCR using the 16S rRNA gene as an example. Appl. Environ. Microbiol. 74, 1660–1663. doi: 10.1128/AEM.02403-07
Bustin, S. A., Benes, V., Garson, J., Hellemans, J., Huggett, J., Kubista, M., et al. (2013). The need for transparency and good practices in the qPCR literature. Nat. Methods 10, 1063–1067. doi: 10.1038/nmeth.2697
Bustin, S. A., Benes, V., Garson, J. A., Hellemans, J., Huggett, J., Kubista, M., et al. (2009). The MIQE guidelines: minimum information for publication of quantitative real-time PCR experiments. Clin. Chem. 55, 611–622. doi: 10.1373/clinchem.2008.112797
Byappanahalli, M. N., Nevers, M. B., Whitman, R. L., and Ishii, S. (2015). Application of a microfluidic quantitative polymerase chain reaction technique to monitor bacterial pathogens in beach water and complex environmental matrices. Environ. Sci. Technol. Lett. 2, 347–351. doi: 10.1021/acs.estlett.5b00251
Chénier, M. R., Beaumier, D., Roy, R., Driscoll, B. T., Lawrence, J. R., and Greer, C. W. (2003). Impact of seasonal variations and nutrient inputs on nitrogen cycling and degradation of hexadecane by replicated river biofilms. Appl. Environ. Microbiol. 69, 5170–5177. doi: 10.1128/AEM.69.9.5170-5177.2003
Crane, S. L. (2016). Microfluidic qPCR for Microbial Ecotoxicology in Soil: A Pilot Study. [master's thesis]. Sydney: UNSW Sydney
Dahllöf, I., Baillie, H., and Kjelleberg, S. (2000). rpoB-based microbial community analysis avoids limitations inherent in 16S rRNA gene intraspecies heterogeneity. Appl. Environ. Microbiol. 66, 3376–3380. doi: 10.1128/AEM.66.8.3376-3380.2000
Dandie, C., Miller, M., Burton, D., Zebarth, B., Trevors, J., and Goyer, C. (2007). Nitric oxide reductase-targeted real-time PCR quantification of denitrifier populations in soil. Appl. Environ. Microbiol. 73, 4250–4258. doi: 10.1128/AEM.00081-07
Debruyn, J. M., Chewning, C. S., and Sayler, G. S. (2007). Comparative quantitative prevalence of Mycobacteria and functionally abundant nidA, nahAc, and nagAc dioxygenase genes in coal tar contaminated sediments. Environ. Sci. Technol. 41, 5426–5432. doi: 10.1021/es070406c
Dhoubhadel, B. G., Yasunami, M., Yoshida, L-M., Thi, H. a. N., Thi, T. H. V., Dang, D. A., et al. (2014). A novel high-throughput method for molecular serotyping and serotype-specific quantification of Streptococcus pneumoniae using a nanofluidic real-time PCR system. J. Med. Microbiol. 63, 528–539. doi: 10.1099/jmm.0.071464-0
Dionisi, H. M., Chewning, C. S., Morgan, K. H., Menn, F-M., Easter, J. P., and Sayler, G. S. (2004). Abundance of dioxygenase genes similar to Ralstonia sp. strain U2 nagAc is correlated with naphthalene concentrations in coal tar-contaminated freshwater sediments. Appl. Environ. Microbiol. 70, 3988–3995. doi: 10.1128/AEM.70.7.3988-3995.2004
Edwards, I. P., Zak, D. R., Kellner, H., Eisenlord, S. D., and Pregitzer, K. S. (2011). Simulated atmospheric N deposition alters fungal community composition and suppresses ligninolytic gene expression in a northern hardwood forest. PLoS ONE 6:e20421. doi: 10.1371/journal.pone.0020421
Fedorov, D., Ivanova, E., Doronina, N., and Trotsenko, Y. A. (2008). A new system of degenerate oligonucleotide primers for detection and amplification of nifHD genes. Microbiology 77, 247–249. doi: 10.1134/S0026261708020215
Fish, J. A., Chai, B., Wang, Q., Sun, Y., Brown, C. T., Tiedje, J. M., et al. (2013). FunGene: the functional gene pipeline and repository. Front. Microbiol. 4:291. doi: 10.3389/fmicb.2013.00291
Foesel, B. U., Nägele, V., Naether, A., Wüst, P. K., Weinert, J., Bonkowski, M., et al. (2014). Determinants of Acidobacteria activity inferred from the relative abundances of 16S rRNA transcripts in German grassland and forest soils. Environ. Microbiol. 16, 658–675. doi: 10.1111/1462-2920.12162
Frank, J. A., Reich, C. I., Sharma, S., Weisbaum, J. S., Wilson, B. A., and Olsen, G. J. (2008). Critical evaluation of two primers commonly used for amplification of bacterial 16S rRNA genes. Appl. Environ. Microbiol. 74, 2461–2470. doi: 10.1128/AEM.02272-07
Gaby, J. C., and Buckley, D. H. (2012). A comprehensive evaluation of PCR primers to amplify the nifH gene of nitrogenase. PLoS ONE 7:e42149. doi: 10.1371/journal.pone.0042149
Gaby, J. C., and Buckley, D. H. (2017). The use of degenerate primers in qPCR analysis of functional genes can cause dramatic quantification bias as revealed by investigation of nifH primer performance. Microbial. Ecol. 74:701–708. doi: 10.1007/s00248-017-0968-0
Graham, E. B., Knelman, J. E., Schindlbacher, A., Siciliano, S., Breulmann, M., Yannarell, A., et al. (2016). Microbes as engines of ecosystem function: when does community structure enhance predictions of ecosystem processes? Front. Microbiol. 7:214. doi: 10.3389/fmicb.2016.00214
Hallin, S., Jones, C. M., Schloter, M., and Philippot, L. (2009). Relationship between N-cycling communities and ecosystem functioning in a 50-year-old fertilization experiment. ISME J. 3, 597–605. doi: 10.1038/ismej.2008.128
He, Z., Deng, Y., Van Nostrand, J. D., Tu, Q., Xu, M., Hemme, C. L., et al. (2010). GeoChip 3.0 as a high-throughput tool for analyzing microbial community composition, structure and functional activity. ISME J. 4:1167. doi: 10.1038/ismej.2010.46
Henry, S., Baudoin, E., López-Gutiérrez, J. C., Martin-Laurent, F., Brauman, A., and Philippot, L. (2004). Quantification of denitrifying bacteria in soils by nirK gene targeted real-time PCR. J. Microbiol. Methods 59, 327–335. doi: 10.1016/j.mimet.2004.07.002
Henry, S., Bru, D., Stres, B., Hallet, S., and Philippot, L. (2006). Quantitative detection of the nosZ gene, encoding nitrous oxide reductase, and comparison of the abundances of 16S rRNA, narG, nirK, and nosZ genes in soils. Appl. Environ. Microbiol. 72, 5181–5189. doi: 10.1128/AEM.00231-06
Hjelmso, M. H., Hansen, L. H., Baelum, J., Feld, L., Holben, W. E., and Jacobsen, C. S. (2014). High-resolution melt analysis for rapid comparison of bacterial community compositions. Appl. Environ. Microbiol. 80, 3568–3575. doi: 10.1128/AEM.03923-13
Hu, H-W., Chen, D., and He, J-Z. (2015). Microbial regulation of terrestrial nitrous oxide formation: understanding the biological pathways for prediction of emission rates. FEMS Microbiol. Rev. 39, 729–749. doi: 10.1093/femsre/fuv021
Huang, Y-T., Yang, J-I., Chrobak, M., and Borneman, J. (2014). PRISE2: Software for designing sequence-selective PCR primers and probes. BMC Bioinformat. 15:317. doi: 10.1186/1471-2105-15-317
Huggett, J. F., Foy, C. A., Benes, V., Emslie, K., Garson, J. A., Haynes, R., et al. (2013). The digital MIQE guidelines: minimum information for publication of quantitative digital PCR experiments. Clin. Chem. 59, 892–902. doi: 10.1373/clinchem.2013.206375
Ishii, K., and Fukui, M. (2001). Optimization of annealing temperature to reduce bias caused by a primer mismatch in multitemplate PCR. Appl. Environ. Microbiol. 67, 3753–3755. doi: 10.1128/AEM.67.8.3753-3755.2001
Ishii, S., Kitamura, G., Segawa, T., Kobayashi, A., Miura, T., Sano, D., et al. (2014a). Microfluidic quantitative PCR for simultaneous quantification of multiple viruses in environmental water samples. Appl. Environ. Microbiol. 80, 7505–7511. doi: 10.1128/AEM.02578-14
Ishii, S., Nakamura, T., Ozawa, S., Kobayashi, A., Sano, D., and Okabe, S. (2014b). Water quality monitoring and risk assessment by simultaneous multipathogen quantification. Environ. Sci. Technol. 48, 4744–4749. doi: 10.1021/es500578s
Ishii, S., Segawa, T., and Okabe, S. (2013). Simultaneous quantification of multiple food and waterborne pathogens by use of microfluidic quantitative PCR. Appl. Environ. Microbiol. 00205–00213. doi: 10.1128/AEM.00205-13
Iwai, S., Chai, B., Sul, W. J., Cole, J. R., Hashsham, S. A., and Tiedje, J. M. (2010). Gene-targeted-metagenomics reveals extensive diversity of aromatic dioxygenase genes in the environment. ISME J. 4, 279–285. doi: 10.1038/ismej.2009.104
Iwai, S., Johnson, T. A., Chai, B., Hashsham, S. A., and Tiedje, J. M. (2011). Comparison of the specificities and efficacies of primers for aromatic dioxygenase gene analysis of environmental samples. Appl. Environ. Microbiol. 77, 3551–3557. doi: 10.1128/AEM.00331-11
Iwai, S., Kurisu, F., Urakawa, H., Yagi, O., Kasuga, I., and Furumai, H. (2008). Development of an oligonucleotide microarray to detect di-and monooxygenase genes for benzene degradation in soil. FEMS Microbiol. Lett. 285, 111–121. doi: 10.1111/j.1574-6968.2008.01223.x
Jones, C. M., Graf, D. R., Bru, D., Philippot, L., and Hallin, S. (2013). The unaccounted yet abundant nitrous oxide-reducing microbial community: a potential nitrous oxide sink. ISME J. 7, 417–426. doi: 10.1038/ismej.2012.125
Jurelevicius, D., Alvarez, V. M., Peixoto, R., Rosado, A. S., and Seldin, L. (2013). The use of a combination of alkB primers to better characterize the distribution of alkane-degrading bacteria. PLoS ONE 8:e66565. doi: 10.1371/journal.pone.0066565
Kellner, H., Luis, P., Zimdars, B., Kiesel, B., and Buscot, F. (2008). Diversity of bacterial laccase-like multicopper oxidase genes in forest and grassland Cambisol soil samples. Soil Biol. Biochem. 40, 638–648. doi: 10.1016/j.soilbio.2007.09.013
Kim, H., Yoon, J. B., and Lee, J. (2017). Development of fluidigm SNP type genotyping assays for marker-assisted breeding of chili pepper (Capsicum annuum L.). Korean J. Horticult. Sci. Technol. 35, 465–479. doi: 10.12972/kjhst.20170050
Kleyer, H., Tecon, R., and Or, D. (2017). Resolving species level changes in a representative soil bacterial community using microfluidic quantitative PCR. Front. Microbiol. 8:2017. doi: 10.3389/fmicb.2017.02017
Kumar, A., Trefault, N., and Olaniran, A. O. (2016). Microbial degradation of 2,4-dichlorophenoxyacetic acid: insight into the enzymes and catabolic genes involved, their regulation and biotechnological implications. Crit. Rev. Microbiol. 42, 194–208. doi: 10.3109/1040841X.2014.917068
Kuntze, K., Shinoda, Y., Moutakki, H., Mcinerney, M. J., Vogt, C., Richnow, H. H., et al. (2008). 6-Oxocyclohex-1-ene-1-carbonyl-coenzyme A hydrolases from obligately anaerobic bacteria: characterization and identification of its gene as a functional marker for aromatic compounds degrading anaerobes. Environ. Microbiol. 10, 1547–1556. doi: 10.1111/j.1462-2920.2008.01570.x
Kuypers, M. M., Marchant, H. K., and Kartal, B. (2018). The microbial nitrogen-cycling network. Nat. Rev. Microbiol. 16, 263–276 doi: 10.1038/nrmicro.2018.9
Landeweert, R., Hoffland, E., Finlay, R. D., Kuyper, T. W., and Van Breemen, N. (2001). Linking plants to rocks: ectomycorrhizal fungi mobilize nutrients from minerals. Trends Ecol. Evol. 16, 248–254. doi: 10.1016/S0169-5347(01)02122-X
Lane, D. (1991). “16S/23S rRNA sequencing,” in Nucleic Acid Techniques in Bacterial Systematics, eds E. Stackebrandt and M Goodfellow (John Wiley and Sons: Chichester, UK), 115–175.
Levy-Booth, D. J., Prescott, C. E., and Grayston, S. J. (2014). Microbial functional genes involved in nitrogen fixation, nitrification and denitrification in forest ecosystems. Soil Biol. Biochem. 75, 11–25. doi: 10.1016/j.soilbio.2014.03.021
Levy-Booth, D. J., and Winder, R. S. (2010). Quantification of nitrogen reductase and nitrite reductase genes in soil of thinned and clear-cut Douglas-fir stands by using real-time PCR. Appl. Environ. Microbiol. 76, 7116–7125. doi: 10.1128/AEM.02188-09
López-Gutiérrez, J. C., Henry, S., Hallet, S., Martin-Laurent, F., Catroux, G., and Philippot, L. (2004). Quantification of a novel group of nitrate-reducing bacteria in the environment by real-time PCR. J. Microbiol. Methods 57, 399–407. doi: 10.1016/j.mimet.2004.02.009
Lueders, T., and von Netzer, F. (2017). “Primers: functional genes for anaerobic hydrocarbon degrading microbes,” in Hydrocarbon and Lipid Microbiology Protocols, eds T. J. McGenity, K. N. Timmis and B. Nogales (Berlin; Heidelberg: Springer), 39–55.
Maeda, H., Fujimoto, C., Haruki, Y., Maeda, T., Kokeguchi, S., Petelin, M., et al. (2003). Quantitative real-time PCR using TaqMan and SYBR Green for Actinobacillus actinomycetemcomitans, Porphyromonas gingivalis, Prevotella intermedia, tetQ gene and total bacteria. FEMS Immunol. Med. Microbiol. 39, 81–86. doi: 10.1016/S0928-8244(03)00224-4
Mahmoudi, N., Slater, G. F., and Fulthorpe, R. R. (2011). Comparison of commercial DNA extraction kits for isolation and purification of bacterial and eukaryotic DNA from PAH-contaminated soils. Can. J. Microbiol. 57, 623–628. doi: 10.1139/w11-049
Mcwatters, R., Wilkins, D., Spedding, T., Hince, G., Raymond, B., Lagerewskij, G., et al. (2016). On site remediation of a fuel spill and soil reuse in Antarctica. Sci. Total Environ. 571, 963–973. doi: 10.1016/j.scitotenv.2016.07.084
Meinhardt, K. A., Bertagnolli, A., Pannu, M., Strand, S., Brown, S., and Stahl, D. A. (2014). Evaluation of revised PCR primers for more inclusive quantification of ammonia-oxidizing archaea and bacteria. Environ. Microbiol. Rep. 7, 354–363. doi: 10.1111/1758-2229.12259
Miller, K. M., Gardner, I. A., Vanderstichel, R., Burnley, T., Angela, D., Li, S., et al. (2016). Report on the Performance Evaluation of the Fluidigm BioMark Platform for High-Throughput Microbe Monitoring in Salmon. Fisheries and Oceans Canada, Ecosystems and Oceans Science.
Molina, M. C., González, N., Bautista, L. F., Sanz, R., Simarro, R., Sánchez, I., et al. (2009). Isolation and genetic identification of PAH degrading bacteria from a microbial consortium. Biodegradation 20, 789–800. doi: 10.1007/s10532-009-9267-x
Mukherjee, A., Chettri, B., Langpoklakpam, J. S., Basak, P., Prasad, A., Mukherjee, A. K., et al. (2017). Bioinformatic approaches including predictive metagenomic profiling reveal characteristics of bacterial response to petroleum hydrocarbon contamination in diverse environments. Sci. Rep. 7:1108. doi: 10.1038/s41598-017-01126-3
Muyzer, G., De Waal, E. C., and Uitterlinden, A. G. (1993). Profiling of complex microbial populations by denaturing gradient gel electrophoresis analysis of polymerase chain reaction-amplified genes coding for 16S rRNA. Appl. Environ. Microbiol. 59, 695–700.
Oshiki, M., Segawa, T., and Ishii, S. (2018). Nitrogen cycle evaluation (NiCE) chip for the simultaneous analysis of multiple N-cycle associated genes. Appl. Environ. Microbiol. 02615–02617. doi: 10.1128/AEM.02615-17
Petersen, D. G., Blazewicz, S. J., Firestone, M., Herman, D. J., Turetsky, M., and Waldrop, M. (2012). Abundance of microbial genes associated with nitrogen cycling as indices of biogeochemical process rates across a vegetation gradient in Alaska. Environmental Microbiology 14, 993–1008. doi: 10.1111/j.1462-2920.2011.02679.x
Pfeiffer, S., Pastar, M., Mitter, B., Lippert, K., Hackl, E., Lojan, P., et al. (2014). Improved group-specific primers based on the full SILVA 16S rRNA gene reference database. Environ. Microbiol. 16, 2389–2407. doi: 10.1111/1462-2920.12350
Powell, J. R., Welsh, A., and Hallin, S. (2015). Microbial functional diversity enhances predictive models linking environmental parameters to ecosystem properties. Ecology 96, 1985–1993. doi: 10.1890/14-1127.1
Powell, S. M. (2009). “Potential use of real-time PCR to assess changes in the microbial population structure and function during bioremediation of polar soils,” in Polar Microbiology, eds A. K. Bej, J. Aislabie, and R. M. Atlas, (Boca Raton, FL: CRC Press), 357–371. doi: 10.1201/9781420083880-c16
Powell, S. M., Ferguson, S. H., Bowman, J. P., and Snape, I. (2006). Using real-time PCR to assess changes in the hydrocarbon-degrading microbial community in Antarctic soil during bioremediation. Microbial. Ecol. 52, 523–532. doi: 10.1007/s00248-006-9131-z
Prevost-Boure, N. C., Christen, R., Dequiedt, S., Mougel, C., Lelievre, M., Jolivet, C., et al. (2011). Validation and application of a PCR primer set to quantify fungal communities in the soil environment by real-time quantitative PCR. PLoS ONE 6:e24166. doi: 10.1371/journal.pone.0024166
Ramakers, C., Ruijter, J. M., Deprez, R. H. L., and Moorman, A. F. (2003). Assumption-free analysis of quantitative real-time polymerase chain reaction (PCR) data. Neurosci. Lett. 339, 62–66. doi: 10.1016/S0304-3940(02)01423-4
Rodríguez, A., Rodríguez, M., Córdoba, J. J., and Andrade, M. J. (2015). Design of primers and probes for quantitative real-time PCR methods. PCR Primer Design 31–56. doi: 10.1007/978-1-4939-2365-6_3
Rose, T. M., Schultz, E. R., Henikoff, J. G., Pietrokovski, S., Mccallum, C. M., and Henikoff, S. (1998). Consensus-degenerate hybrid oligonucleotide primers for amplification of distantly related sequences. Nucl. Acids Res. 26, 1628–1635. doi: 10.1093/nar/26.7.1628
Rotthauwe, J-H., Witzel, K-P., and Liesack, W. (1997). The ammonia monooxygenase structural gene amoA as a functional marker: molecular fine-scale analysis of natural ammonia-oxidizing populations. Appl. Environ. Microbiol. 63, 4704–4712.
Sadik, N. J., Uprety, S. R., Nalweyiso, A., Kiggundu, N., Banadda, N. E., Shisler, J. L., et al. (2017). Quantification of multiple waterborne pathogens in drinking water, drainage channels, and surface water in Kampala, Uganda during seasonal variation. GeoHealth 1, 258–269. doi: 10.1002/2017GH000081
Shahsavari, E., Aburto-Medina, A., Taha, M., and Ball, A. S. (2016). A quantitative PCR approach for quantification of functional genes involved in the degradation of polycyclic aromatic hydrocarbons in contaminated soils. MethodsX 3, 205–211. doi: 10.1016/j.mex.2016.02.005
Smith, C. J., and Osborn, A. M. (2009). Advantages and limitations of quantitative PCR (Q-PCR)-based approaches in microbial ecology. FEMS Microbiol. Ecol. 67, 6–20. doi: 10.1111/j.1574-6941.2008.00629.x
Spurgeon, S. L., Jones, R. C., and Ramakrishnan, R. (2008). High throughput gene expression measurement with real time PCR in a microfluidic dynamic array. PLoS ONE 3:e1662. doi: 10.1371/journal.pone.0001662
Throbäck, I. N., Enwall, K., Jarvis, Å., and Hallin, S. (2004). Reassessing PCR primers targeting nirS, nirK and nosZ genes for community surveys of denitrifying bacteria with DGGE. FEMS Microbiol. Ecol. 49, 401–417. doi: 10.1016/j.femsec.2004.04.011
Van Dorst, J., Siciliano, S. D., Winsley, T., Snape, I., and Ferrari, B. C. (2014). Bacterial targets as potential indicators of diesel fuel toxicity in subantarctic soils. Appl. Environ. Microbiol. 80, 03939–03913. doi: 10.1128/AEM.03939-13
Wang, W., and Shao, Z. (2012). Genes involved in alkane degradation in the Alcanivorax hongdengensis strain A-11-3. Appl. Microbiol. Biotechnol. 94, 437–448. doi: 10.1007/s00253-011-3818-x
Wei, W., Isobe, K., Nishizawa, T., Zhu, L., Shiratori, Y., Ohte, N., et al. (2015). Higher diversity and abundance of denitrifying microorganisms in environments than considered previously. ISME J. 9:1954. doi: 10.1038/ismej.2015.9
Widder, S., Allen, R. J., Pfeiffer, T., Curtis, T. P., Wiuf, C., Sloan, W. T., et al. (2016). Challenges in microbial ecology: building predictive understanding of community function and dynamics. ISME J. 10:2557. doi: 10.1038/ismej.2016.45
Ye, J., Coulouris, G., Zaretskaya, I., Cutcutache, I., Rozen, S., and Madden, T. L. (2012). Primer-BLAST: a tool to design target-specific primers for polymerase chain reaction. BMC Bioinform. 13:134. doi: 10.1186/1471-2105-13-134
Yergeau, E., Kang, S., He, Z., Zhou, J., and Kowalchuk, G. A. (2007). Functional microarray analysis of nitrogen and carbon cycling genes across an Antarctic latitudinal transect. ISME J. 1, 163–179. doi: 10.1038/ismej.2007.24
Zheng, L., Gibbs, M. J., and Rodoni, B. C. (2008). Quantitative PCR measurements of the effects of introducing inosines into primers provides guidelines for improved degenerate primer design. J. Virol. Methods 153, 97–103. doi: 10.1016/j.jviromet.2008.07.029
Keywords: microfluidic qPCR, quantitative PCR, functional genes, nitrogen cycle, hydrocarbon degradation, microbial community, terrestrial ecology, biogeochemical cycles
Citation: Crane SL, van Dorst J, Hose GC, King CK and Ferrari BC (2018) Microfluidic qPCR Enables High Throughput Quantification of Microbial Functional Genes but Requires Strict Curation of Primers. Front. Environ. Sci. 6:145. doi: 10.3389/fenvs.2018.00145
Received: 19 July 2018; Accepted: 08 November 2018;
Published: 26 November 2018.
Edited by:
Luiz Fernando Wurdig Roesch, Federal University of Pampa, BrazilReviewed by:
Satoshi Ishii, University of Minnesota Twin Cities, United StatesRoberta Fulthorpe, University of Toronto Scarborough, Canada
Copyright © 2018 Crane, van Dorst, Hose, King and Ferrari. This is an open-access article distributed under the terms of the Creative Commons Attribution License (CC BY). The use, distribution or reproduction in other forums is permitted, provided the original author(s) and the copyright owner(s) are credited and that the original publication in this journal is cited, in accordance with accepted academic practice. No use, distribution or reproduction is permitted which does not comply with these terms.
*Correspondence: Belinda C. Ferrari, Yi5mZXJyYXJpQHVuc3cuZWR1LmF1