- 1Applied Mathematics, Universidad Politécnica de Madrid, Madrid, Spain
- 2Applied Mathematics and Computer Science in Civil and Marine Engineering, Universidad Politécnica de Madrid, Madrid, Spain
We made use of 3D tomograms from X-ray computed tomography of soil samples from a vineyard in La Rioja (Spain), to explore the ability of Minkowski functionals of connected soil pore space to discriminate between different pore space geometries coming from soils with different management and depth and that, a priori, are expected to have some degree of dissimilarity. We estimate the volume of the connected pore space (V), the surface of the interface soil/void (S), the accumulated mean curvature of that interface (C), and its connectivity (E) out of 3D binary images taken from samples of two different depths of soil where two different managements were undertaken. Logit model showed that V, S, and C are needed to predict soil management and only V is required to infer depths. In our limited experimental circumstances, where we just explain two soil features, it seems natural that not all the four functionals are related to or needed to explain the variety of considered cases of soil structures. Therefore, this could not be an argument to dismiss Minkowski functionals as good candidates as predictors of the geometric structure of soil pore space. Our results suggest just the opposite, and they can be used as discriminants for a wide variety of soil features and behaviors.
1. Introduction
Soil structure is intimately related to soil functioning. Soil structure controls many important biophysical processes in soil-plant-microbial systems, related to microbial population dynamics, mass flow, nutrient cycling and uptake by roots (Young and Crawford, 2004). Besides, soil structure also influences soil's response to changes in the surrounding climate or to agriculture (Pot et al., 2015), carbon protection in soil (Kravchenko et al., 2015) or the transport of water and gas through its complex structure (Lehmann et al., 2006). In particular, many of these phenomena are strongly dependent on pore space geometry. A deeper understanding of the geometrical subtleties of soil's pore space topology and its changes due to agricultural management or depth are essential to modeling the previously mentioned processes and to infer the effect of agricultural management and depth on them.
Soil management is intended to affect soil structure to alter its functioning and enhance its productivity, but the geometrical effect of this, sometimes highly invasive, soil perturbations on pore space geometry is not completely elucidated. It is known that a shift from long periods of intensive agriculture to a natural vegetation cover, increases the contents of organic matter present in the soil and also results in a bigger number of stable micro aggregates (Gryze et al., 2004; Grandy and Robertson, 2007).
The pedologist will manually sample soil in situ and classify its structure among several classes that are, somehow, related to its possible functioning and agricultural yield. For many years now, the use of X-ray computerized tomography (CT) has been used as a source of three-dimensional studies of the geometry of the soil pore space (Peyton et al., 1994; Perret et al., 1999; Pierret et al., 2002; Mees et al., 2003; Lehmann et al., 2006; Wildenschild and Sheppard, 2013). In particular, the use of advanced X-ray CT 3D imaging techniques made possible the visualization and study of the pore space inside soil aggregates. Many studies have shown that agricultural practices as tillage and fertilization directly affect the intra-aggregate pore characteristics (Peth et al., 2008; Kravchenko et al., 2011; Wang et al., 2012; Zhou et al., 2013; Zucca et al., 2013).
The vast amount of 3D geometrical data obtained through X-ray CT has been analyzed using tools borrowed from mathematical morphology and integral geometry (Santaló, 1976; Mecke and Stoyan, 2000, 2002; Banhart, 2008). Among these, Minkowski functionals (Arns et al., 2002; Lehmann et al., 2006) are specially interesting. They provide four fundamental geometrical properties of 3D objects, like soil porosity, embedded in three-dimensional space. These properties are the volume, the boundary surface, the integral mean curvature, and the connectivity of the object. Plus they can be computed efficiently. Hadwiger's theorem shows that any functional defined on any three-dimensional object that meets some natural and self-evident geometrical properties is a linear combination of Minkowski functionals (Santaló, 1976). This mathematical theorem could be interpreted as follows: any information that is geometrically relevant for the characterization of the pore space should be encoded in these four parameters. Then, these functionals could be a suitable quantitatively description of the 3D geometry of soil structure. Mecke (1998) and Roth et al. (2005) characterized 2D porous structures using the variation of Minkowski functionals based on the image binarization threshold variation. The same methodology was used by San José Martínez et al. (2013) on soil pore volumes of CT images of undisturbed soil columns. Mecke (2002) and Vogel et al. (2005) studied the variation of Minkowski funcionals based on the morphological effect of the dilation and erosion operations. This technique was also used by Arns et al. (2002, 2004) to characterize 3D images of Fontainebleau sandstone. Furthermore, Renard and Allard (2013) used the Euler numbers variation subject to erosion and dilation to describe connectivity as a means for characterizing heterogeneous aquifers with 2D models. Schlüter et al. (2011) used Minkowski functionals to describe the soil structure development in response to different fertilizations. Falconer et al. (2012, 2015) used Minkowski functionals to study soil fungal and microbial dynamics as functions of pore architecture and carbon distribution. San José Martínez et al. (2015) described exhaustively the morphology of soil aggregates using Minkowski functionals. This work included both the intra-aggregate pore space and the surface features of the aggregates.
Much have been said about the effect that soil tillage and depth have on the geometry of pore space. In this work we focus our attention in another key question: are Minkowski functionals good candidates as indicators of soil structure? We use Minkowski functionals obtained from three-dimensional X-ray CT images of cubic regions of undisturbed soil columns than were extracted from a vineyard in northern Spain as data set to tackle this question. These samples came from different depths of a soil where two different soil management practices were undertaken. In this investigation, first, we elucidate the influence of soil tillage and depth on Minkowski functionals as representative geometrical parameters. Then, we face the study of the power of these functionals to discriminate between different types of soil tillage and different depths.
2. Materials and Methods
Soil column samples were taken from an experimental farm, called “La Grajera” which belongs to La Rioja Regional Government (La Rioja, northern Spain). Only the Ap horizon was sampled. Its depth varied between 20 and 39 cm due to the slope. Two different soil managements have been employed, since 2004. These soil managements were established in-between the vines rows, with a row spacing of 5.80 m. The managements tested were (i) tillage and (ii) no tillage with soil natural crop cover. The tillage was done with a cultivator up to depth of 15 cm. The frequency was once every 4–6 weeks, as required to control the weeds that affected the grapevines growth cycle. The dominant species commonly found in vineyards of La Rioja were annual grass and herb. This was the permanent cover crop in between the rows with no tillage. More details on the site can be found in Peregrina et al. (2010). A total of 12 soil columns were extracted in December 2010 using cylinders made out of PVC. The dimensions were 7.5 cm inside diameter and 60 cm height. These cylinders were percussion drilled in the space between the rows. In total, three strips were selected per treatment, six in total. Therefore, two columns per strip and six per treatment were collected.
Soil images were attained with a X-ray CT that used a Feinfocus FXE 225.51 microfocus beam source tube and a PerkinElmer amorphous silicon (a-Si) detector with 2,048 × 2,048 pixels. Operation acceleration voltage was 190kV (53μA) and target power was 20 W. The resulting images were in 16-bit grayscale. The shallow soil layer was analyzed by scanning the top 15 cm of each column. Images were taken of them from the first 7.5 cm and from the 7.5 to 15 cm layer below soil surface. Six images were taken for each layer and treatment, resulting in a total of 24 images. Each column scan resulted in a stack of 1,706 images. Voxel size in 3D reconstructions was 50μm per side. Prior to segmentation, images were enhanced by applying two consecutive 3D nonlinear filters (Müter et al., 2012). The first one, an Unsharp Mask, is used to enhance the edges between the soild and void phases, whilst the second one, a Median Filter, is used to reduce the noise present in the image. Images were segmented using the local adaptive method (Sauvola and Pietikäinen, 2000; Naveed et al., 2012). Cubic volumes 5.1 cm lineal size (= 10243 voxels) were cut out of the cylinders and used as regions of interest for the study of soil structure. Reconstructions of the soil pore volumes inside columns can be seen in Figure 1. More detailed information can be found in San José Martínez et al. (2017).
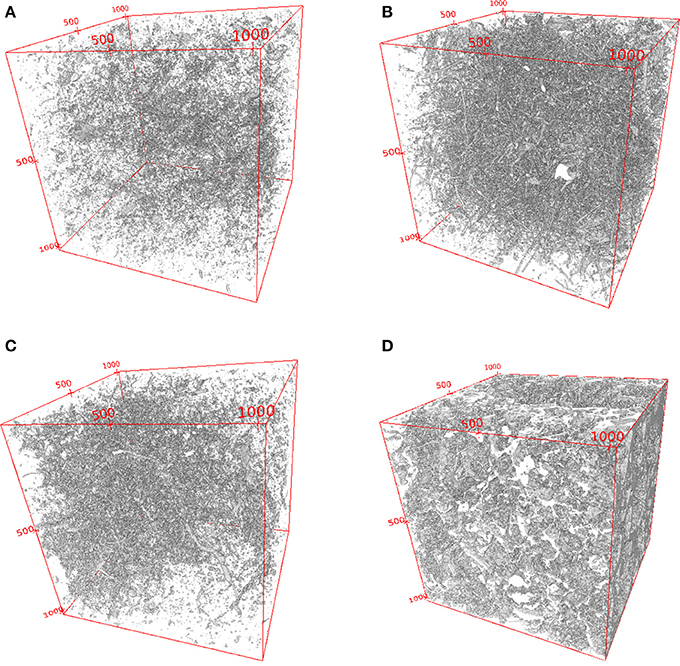
Figure 1. Three-dimensional reconstruction pores space (black). Ticks in the axes correspond to pixel number. (A) Top soil sample cube from conventional tillage. (B) Bottom soil sample cube from conventional tillage. (C) Top soil sample cube from permanent cover crop of natural vegetation. (D) Bottom soil sample cube permanent cover crop of natural vegetation.
When the binary images were available, a computer program (Michielsen and Raedt, 2001) was used to measure geometrical properties and derive the Minkowski functionals. For a 3D object K we can obtain the volume (V), the boundary surface (S), its integral mean curvature (C), and the connectivity (E) of the object of interest as follows
where nc is the number of open cubes, nf the number open squares or faces, ne the number of open edges, and nv the number of vertices that the voxels of the object K has. The property of two voxels being connected must be properly defined to be able to efficiently compute the topological properties of connected voxels by just using the total numbers of vertices, edges, faces and cubes defined above. In this work, two pore space voxels were considered as connected when they had either a face, an edge or a vertex in common (Michielsen and Raedt, 2001). Pores space voxels connected to the boundaries of the sample will be referred to as connected porosity. This is the object of interest in this work.
Soil samples come from two different soil management techniques, i.e., tillage, which will be noted as SMt; and natural cover, or no tillage, noted as SMc. Besides, two different depths are analyzed, first one, the layer between the soil surface and a depth of 7.5 cm, noted as DP1, while the second one ranges from 7.5 to 15 cm, noted as DP2. A total of n = 22 samples were considered, divided in the two above described factors with two levels. The inhomogeneity of sampling numbers among factors is due to the loss of a deep sample in the SMt class, and the loss of a superficial sample of the SMc class. We investigate if soil factors, tillage and depth, have any influence on the Minkowski functionals V, S, C, and E. For this purpose ANOVA factor analysis, with two factors and two levels in each factor, was carried out. Additionally, this procedure establishes possible interactions among the factors: sometimes the effect that a factor has on a response variable is dependent on the level at which the other factor is. To check the validity of the model, normality and homoscedasticity of residues were checked. We use Kolmogorov-Smirnov test and Levene test, respectively. We found that at 0.05 significance level the normality and homoscedasticity hypothesis cannot be rejected.
Besides the previous test, we analyze if Minkowski functionals are capable of predicting the particular level of any of the soil available factors that a particular sample is in. Moreover, we want to identify which of the functionals is more relevant in the role of predicting the level of the corresponding soil factor. Classical linear regression is out of the question as the dependent variable is a qualitative binary response variable. A Logit, or logistic regression model, comes at hand: it is a specifically designed non-linear regression model for binary responses. Basically, the nonlinearity forces the estimated values to be in the [0, 1] interval. As this is a regression analysis, it allows us to identify the variables which are most important at explaining the difference among the possible soil levels. We will determine the probability of a given sample to belong to a given soil treatment or a given soil depth based on a given combination of the values of the Minkowski functionals of that sample. The predictive power of the Minkowski functionals is related to the number of samples that are correctly assigned using the model probability function. With a logarithmic transformation we have the following expression for the probability of a given sample belongs to a given soil treatment or a given soil depth
In this expression, X = (1, X1, …, X4), βj are the coefficient of the regression and εi is a random variable with mean 0 and independent values. Variables Xi correspond, in order, to Minkowski functionals, V, S, C, and E. Therefore, there are four explicative variables, or predictors and we need to estimate five values: the independent term β0 and the coefficients of the four independent variables β1, …, β4. The value of the response will be 1 if the probability obtained, for a given combination of the Minkowski functionals, is strictly bigger than 0.5, and zero in the other case. To evaluate the statistical significance of the estimated parameters, a test of hypothesis is performed. The null hypothesis H0:β0 = β1 = β2 = β3 = β4 = 0 that all parameters are equal to zero, i.e., they have no effect on the soil factors, is tested. Finally, to analyze the goodness-of-fit of the Logit model, we made use of a “leave-one-out-cross-validation” (LOOCV) procedure.
3. Results and Discussion
3.1. Geometrical Attributes V, S, C, and E as Response Variables
The calculated Minkowski functionals for the different images can be found in Table 1. Their mean values and standard deviations are collected in Table 2. We performed the ANOVA test for each Minkowski functional, V, S, C, and E, in order to evaluate the statistical significance of the parameters of the model. Table 3 shows ANOVA results for Minkowski functionals as response variables.
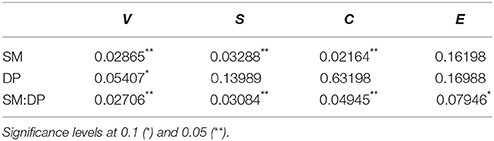
Table 3. Results of ANOVA's test of p-values for Minkowski functionals as response variables of two factors (SM and DP) with two levels (SMt/SMc and DP1/DP2).
When using a significance level of 0.05, soil management and the interaction between soil management and soil depth are statistically significant at explaining V. When using a significance level of 0.1, then soil depth also becomes statistically significant. In order to interpret the interaction between the two factors, we can see, in Figure 2A, that when in a soil that has undergone tillage (SMt), the mean values of V for the two available soil depths are not visually different. On the other hand, when the soil has kept the natural cover (SMc), there are differences in the mean values of V, meaning that this soil use has no effect on the mean value of the volume of soil pore network, thus it is not altering the soil volume at any depth. On the other hand, tillage removes the soil cover up to a depth of approximately 15cm. This practice homogenizes soil structure, whilst when soil is kept with its natural cover, this does not happen. Thus, Minkowski functional V captures this expected difference in soil structure due to soil management.
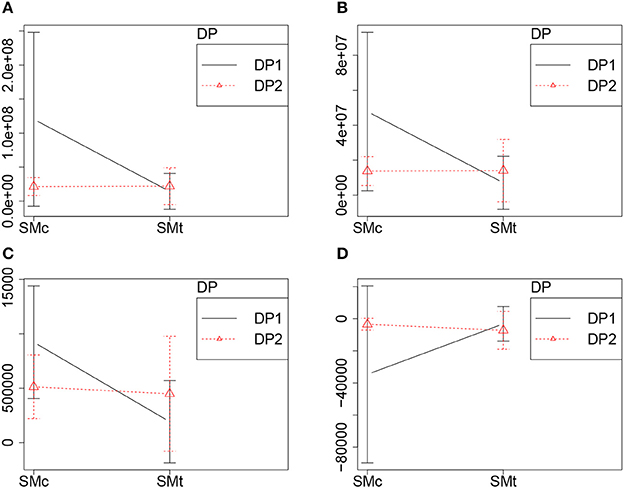
Figure 2. Two factor interaction for (A) V, (B) S, (C) C, and (D) E. Horizontal axis represents the soil management factor and vertical axis the value of the Minkowski functional. The depth factor is represented by alternating colors.
For Minkowski functional S, results of the ANOVA test (Table 3), showed that at the 0.05 significance level, only soil management and the interaction between the two factors are statistically significant. On the other hand, depth has no influence on the mean value of S. The interaction between the two factors can be interpreted using Figure 2B, which is very similar to the graph for the interaction of the factors for V. When soil underwent tillage, the mean values of S are similar for both depths, whilst they are different for a naturally covered soil. The homogenization effect of tillage observed for V is also valid for S.
In the case of C response variable, we have (Table 3) the same conclusions as for the model with response variable V or S: both the soil management and the interaction between the factors are statistically significant. The interaction between the factors for C can be seen in Figure 2C, where we observe the same behavior as we did for the previous functionals V and S.
Lastly, for the model with response variable E (Table 3), only the interaction between soil management and soil depth is statistically significant but with a significance level of 0.1. None of the other factors alone has influence on the mean value of E. Figure 2D shows the effect of the interaction of the factors in the mean value of E, which is similar to the previous interaction graphs.
We conclude that mean values of V, S, and C become statistically different when altering soil management with a significance level of 0.1. However mean values of V become statistically different when depth changes. It is worth noting that interactions were observed between the two factors for all geometrical attributes V, S, C, and E, with a significance level of 0.1. A soil that has undergone tillage (SMt), did not show different mean values of functionals for the two available soil depths. But, when the soil has kept the natural cover (SMc), there are differences in the mean values of these geometrical attributes. Our results suggest that Minkowski functionals capture the expected difference in soil structure due to soil management when measured at different depths.
3.2. Geometrical Attributes V, S, C, and E as Predictors
First we consider that the response variable is the factor type of soil management in the Logit model. We use the level SMt as 1, and SMc as 0 in the model. Thus, the model estimates the probability that a certain sample has undergone tillage, i.e., belongs to the level SMt. Table 4 shows the estimation of the coefficients of the regression model and the p-values associated with the hypothesis tests for those coefficients to be equal to zero. We conclude that all the coefficients are equal to zero except β0, as all the p-values are bigger than the habitual reference values. This could lead to conclude that the response variable is independent of the Minkowski functionals, but this is not reasonable looking at the results of the previous part of this work. A plausible explanation could be the existence of multicolinearity among variables. To study this possible effect the values of the functionals are plotted against one another. Figure 3 is a matrix of dispersion graphs for all the functionals. All of them present highly aligned graphs. To further analyze this possible colinearity, Table 5 presents the coefficients of determination among the functionals. It can be observed that C is the least correlated functional, to the rest. Taking into account this information and the results of ANOVA, it seems reasonable to use V, S, and C as predictors in the Logit model. Table 6 shows the results in this case. Now, besides β0, also the coefficients for V, S, and C, are statistically significant at a level of 0.1.
Now we are considering that soil depth level is the response variable in the Logit model. In this case we take the level DP2 as the value 1, and DP1 as 0 for the model. Thus, the model will estimate the probability that a certain sample belongs to the level DP2. Table 7 shows the values of the estimated coefficients for this model, along with the p-values. In this case only β1, the coefficient for V, is statistically significant at a level of 0.05. This result is also coherent with the results obtained previously in the ANOVA test.
In order to asses the ability of these models to predict the level of factor types SM and DP, we made use of a “leave-one-out-cross-validation” (LOOCV) procedure. With this test, the percentage of failure for predicting the SM was 26.3%, and for predicting DP it was 26.0%.
Interesting to see is that a combination of the three, V, S, and C, discriminates between soil management practices, whilst only V is necessary to discriminate between soil depth levels. These results suggest that Minkowski functionals are good predictors of soil management and depth. Nevertheless not all of them are needed to discriminate between the different imprint that soil management or depth has left on pore space geometry. For the soils considered in this investigation, connectivity, as measured by E, does not play a major role as a predictor neither for soil management nor for soil depth.
4. Conclusions
In this investigation we made use of 3D tomograms from X-ray CT of soil samples from a vineyard in La Rioja (Spain), to explore the ability of Minkowski functionals of connected soil pore space to discriminate between different pore space geometries that, a priori, are expected to have some degree of dissimilarity. To do so we measured the volume of the connected pore space (V), the surface of the interface soil/void (S), the accumulated mean curvature of that interface (C) and its connectivity (E). These are the Minkowski functionals for the soil pore space.
We first analyzed the Minkowski functionals as response variable of soil management, tillage vs. natural cover; and depth, from topsoil to 7.5cm, and from there to 15cm. We observed, with a significance level of 0.1, that mean values of V, S, and C become statistically different when altering soil management. Also interactions were observed between the two factors for geometrical attributes V, S, and C. A soil that has undergone tillage (SMt), did not show different mean values of these functionals for the two available soil depths. But, when the soil has kept the natural cover (SMc), there are differences in the mean values of these geometrical attributes. Tillage mixes the soil cover up to a depth of approximately 15cm. This practice of soil management homogenizes soil structure, whilst when soil is kept with its natural cover crop, this does not happen. A similar interaction shows connectivity E. These results suggest that Minkowski functionals V, S, and C capture this expected difference in soil structure due to soil tillage when measured at different depths. On the other hand, depth has influence only on the volume of the connected pore space. This result should be regarded at the light of the fact that both factors showed interaction for all the Minkowski functionals, as explained above.
Logit model showed that V, S, and C are needed to discriminate between soil tillage practices and only V is required to infer depths. As only a limited type of soil structures were at hand, it seems fair that not all the four functionals are needed to infer the limited variety of considered cases of soil structures. Therefore, our results suggest that Minkowski functionals could be good predictors of the geometric structure of soil pore space. Nevertheless, this study should be extended to a larger number and variety of samples. This first study suggests that it is reasonable to further develop this theoretical framework. Future work could be to assess the predicting ability of this promising functionals as predictors of soil features related to changes in pore geometric structure.
Author Contributions
FS has design the experimental, survey column extraction, X-ray CT imaging and the image analysis for binarization and enhance process. CG-G has carried out the estimation of Minkowski functionals. LM has completed the statistical analysis.
Conflict of Interest Statement
The authors declare that the research was conducted in the absence of any commercial or financial relationships that could be construed as a potential conflict of interest.
Acknowledgments
This research work was funded by Spain's Plan Nacional de Investigación Científica, Desarrollo e Innovación Tecnológica (I+D+i), under ref. AGL2015-69697-P. One of the authors (LM) would like to thank Prof. G. Palomo for enlightening discussions.
References
Arns, C., Knackstedt, M., and Mecke, K. (2002). “Characterizing the morphology of disordered materials,” in Morphology of Condensed Matter. LNP 600, eds K. R. Mecke and D. Stoyan (Berlin: Springer Verlag), 37–74.
Arns, C., Knackstedt, M., and Mecke, K. (2004). Characterization of irregular spatial structures by parallel sets and integral geometric measures. Colloids Surf. A Physicochem. Eng. Asp. 241, 351–372. doi: 10.1016/j.colsurfa.2004.04.034
Banhart, J. (ed.). (2008). Advanced Tomographic Methods in Materials Research and Engineering. Oxford: Oxford University Press.
Falconer, R., Battaia, G., Schmidt, S., baveye, P., Chenou, C., and Otten, W. (2015). Microscale heterogeneity explains experimental variability and non-linearity in soil organic matter mineralisatins. PLoS ONE 10:e0123774. doi: 10.1371/journal.pone.0123774
Falconer, R., Houston, A., Otten, W., and Baveye, P. (2012). Emergent behaviour of soil fungal dynamics: influence of soil architecture and water distribution. Soil Sci. 177, 111–119. doi: 10.1097/SS.0b013e318241133a
Grandy, A., and Robertson, G. (2007). Land-use intensity effects on soil organic carbon accumulation rates and mechanisms. Ecosystems 10, 58–73. doi: 10.1007/s10021-006-9010-y
Gryze, S. D., Six, J., Paustian, K., Morris, S., Paul, E., and Merckx, R. (2004). Soil organic carbon pool changes following land-use conversions. Glob. Change Biol. 10, 1120–1132. doi: 10.1111/j.1529-8817.2003.00786.x
Kravchenko, A., Negassa, W., Guber, A., and Rivers, M. (2015). Protection of soil carbon within macro-aggregates depends on intra-aggregate pore characteristics. Sci. Report. 5:16261. doi: 10.1038/srep16261
Kravchenko, A., Wang, W., Smucker, A., and Rivers, M. (2011). Long-term differences in tillage and land use affect intra-aggregate pore heterogeneity. Soil Sci. Soc. Am. J. 75, 1658–1666. doi: 10.2136/sssaj2011.0096
Lehmann, P., Wyss, P., Flisch, A., Lehmann, E., Vontobel, P., Krafczyk, M., et al. (2006). Tomographical imaging and mathematical description of porous media used for the prediction of fluid distribution. Vadose Zone J. 5, 80–97. doi: 10.2136/vzj2004.0177
Mecke, K. (1998). Integral geometry and statistical physics. Int. J. Mod. Phys. B 12, 861–899. doi: 10.1142/S0217979298000491
Mecke, K. (2002). The shape of parallel surfaces: Porous media, fluctuating interfaces and complex fluids. Phys. A 314, 655–662. doi: 10.1016/S0378-4371(02)01047-6
Mecke, K., and Stoyan, D. (eds.). (2000). Statistical Physics and Spatial Statistics. The Art of Analyzing and Modeling Spatial Structures and Pattern Formation. LNP 554. Berlin: Springer Verlag.
Mecke, K., and Stoyan, D. (eds.). (2002). Morphology of Condensed Matter. LNP 600. Berlin: Springer Verlag.
Mees, F., Swennen, R., van Geet, M., and Jacobs, P. editors (2003). Applications of X-Ray Computed Tomography in Geosciences. Spec. Publ. 215. London: Geological Society of London.
Michielsen, K., and Raedt, H. D. (2001). Integral–geometry morphological image analysis. Phys. Rep. 347, 461–538. doi: 10.1016/S0370-1573(00)00106-X
Müter, D., Pedersen, S., Sørensen, H., Feidenhans'l, R., and Stipp, S. (2012). Improved segmentation of X-ray tomography data from porous rocks using a dual filtering approach. Comput. Geosci. 49, 131–139. doi: 10.1016/j.cageo.2012.06.024
Naveed, M., Moldrup, P., Arthur, E., Wildenschild, D., Eden, M., Lamandé, M., et al. (2012). Revealing soil structure and functional macroporosity along a clay gradient using X-ray computed tomography. Soil Sci. Soc. Am. J. 77, 403–411. doi: 10.2136/sssaj2012.0134
Peregrina, F., Larrieta, C., Ibáñez, S., and García-Escudero, E. (2010). Labile organic matter, aggregates, and stratification ratios in a semiarid vineyard with cover crops. Soil Sci. Soc. Am. J. 74, 2120–2130. doi: 10.2136/sssaj2010.0081
Perret, J., Prasher, S., Kantzas, A., and Langford, C. (1999). Three-dimensional quantification of macropore networks in undisturbed soil cores. Soil Sci. Soc. Am. J. 63, 1530–1543. doi: 10.2136/sssaj1999.6361530x
Peth, S., Horn, R., Beckmann, F., Donath, T., Fischer, J., and Smucker, A. (2008). Three-dimensional quantification of intra-aggregate pore-space features using synchrotron radiation-based microtomography. Soil Sci. Soc. Am. J. 72, 897–907. doi: 10.2136/sssaj2007.0130
Peyton, R., Gantzer, C., Anderson, S., Haeffner, B., and Pfeifer, P. (1994). Fractal dimension to describe soil macropore structure using X-ray computed tomography. Water Resour. Res. 30, 691–700. doi: 10.1029/93WR02343
Pierret, A., Capowiez, Y., Belzunces, L., and Moran, C. (2002). 3D reconstruction and quantification of macropores using X-ray computed tomography and image analysis. Geoderma 106, 247–271. doi: 10.1016/S0016-7061(01)00127-6
Pot, V., Peth, S., Monga, O., Vogel, L., Genty, A., Garnier, P., et al. (2015). Three-dimensional distribution of water and air in soil pores: comparison of two-phase two-relaxation-times lattice-Boltzmann and morphological model outputs with synchrotron X-ray computed tomography data. Adv. Water Resour. 84, 87–102. doi: 10.1016/j.advwatres.2015.08.006
Renard, P., and Allard, D. (2013). Connectivity metrics for subsurface flow and transport. Adv. Water Resour. 51, 168–196. doi: 10.1016/j.advwatres.2011.12.001
Roth, R., Boike, J., and Vogel, H. (2005). Quantifying permafrost patterns using Minkowski densities. Permafrost Periglacial Process. 16, 277–290. doi: 10.1002/ppp.531
San José Martínez, F., Caniego, F., and García-Gutiérrez, C. (2017). Lacunarity of soil macropore space arrangement of ct images: effect of soil management and depth. Geoderma 287, 80–89. doi: 10.1016/j.geoderma.2016.09.007
San José Martínez, F., Munoz, F., Caniego, F., and Peregrina, F. (2013). Morphological functions to quantify three-dimensional tomograms of macropore structure in a vineyard soil with two different management regimes. Vadose Zone J. 12:vzj2012.0208. doi: 10.2136/vzj2012.0208
San José Martínez, F., Ortega, F. M., Monreal, F. C., Kravchenko, A., and Wang, W. (2015). Soil aggregate geometry: measurements and morphology. Geoderma 237–238, 36–48. doi: 10.1016/j.geoderma.2014.08.003
Sauvola, J., and Pietikäinen, M. (2000). Adaptive document image binarization. Patt. Recogn. 33, 225–236. doi: 10.1016/S0031-3203(99)00055-2
Schlüter, S., Weller, U., and Vogel, H.-J. (2011). Soil-structure development including seasonal dynamics in a long-term fertilization experiment. J. Plant Nutr. Soil Sci. 174, 395–403. doi: 10.1002/jpln.201000103
Vogel, H., Hoffmann, H., and Roth, K. (2005). Studies of crack dynamics in clay soil. I. Experimental methods, results, and morphological quantification. Geoderma 125, 203–211. doi: 10.1016/j.geoderma.2004.07.009
Wang, W., Kravchenko, A., Smucker, A., Liang, W., and Rivers, M. (2012). Intra-aggregate pore characteristics: X-ray computed microtomography analysis. Soil Sci. Soc. Am. J. 76, 1159–1171. doi: 10.2136/sssaj2011.0281
Wildenschild, D., and Sheppard, A. (2013). X-ray imaging and analysis techniques for quantifying pore-scale structure and processes in subsurface porous medium systems. Adv. Water Resour. 51, 217–246. doi: 10.1016/j.advwatres.2012.07.018
Young, I., and Crawford, J. (2004). Interactions and self-organization in the soil-microbe complex. Science 304, 1634–1637. doi: 10.1126/science.1097394
Zhou, H., Peng, X., Perfect, E., Xiao, T., and Peng, G. (2013). Effects of organic and inorganic fertilization on soil aggregation in an Ultisol as characterized by synchrotron based X-ray micro-computed tomography. Geoderma 23, 195–196. doi: 10.1016/j.geoderma.2012.11.003
Keywords: soil structure, soil pore space, X-ray computed tomography, mathematical morphology, Minkowski functional, soil management
Citation: San José Martínez F, Martín L and García-Gutiérrez C (2018) Minkowski Functionals of Connected Soil Porosity as Indicators of Soil Tillage and Depth. Front. Environ. Sci. 6:55. doi: 10.3389/fenvs.2018.00055
Received: 01 March 2018; Accepted: 25 May 2018;
Published: 13 June 2018.
Edited by:
Wilfred Otten, Cranfield University, United KingdomReviewed by:
David Montagne, AgroParisTech Institut des Sciences et Industries du Vivant et de L'environnement, FranceMariusz Cycoń, Medical University of Silesia, Poland
Steffen Schlüter, Helmholtz-Zentrum für Umweltforschung (UFZ), Germany
Copyright © 2018 San José Martínez, Martín and García-Gutiérrez. This is an open-access article distributed under the terms of the Creative Commons Attribution License (CC BY). The use, distribution or reproduction in other forums is permitted, provided the original author(s) and the copyright owner are credited and that the original publication in this journal is cited, in accordance with accepted academic practice. No use, distribution or reproduction is permitted which does not comply with these terms.
*Correspondence: Fernando San José Martínez, ZmVybmFuZG8uc2Fuam9zZUB1cG0uZXM=