- 1Academy of Climate Change Education and Research, Kerala Agricultural University, Thrissur, India
- 2Indian Council of Agricultural Research-National Institute of Animal Nutrition and Physiology, Bangalore, India
- 3School of Agriculture and Food Sciences, The University of Queensland, Gatton, QLD, Australia
The effects of climate change on humans and other living ecosystems is an area of on-going research. The ruminant livestock sector is considered to be one of the most significant contributors to the existing greenhouse gas (GHG) pool. However, there are opportunities to combat climate change by reducing the emission of GHGs from ruminants. Methane (CH4) and nitrous oxide (N2O) are emitted by ruminants via anaerobic digestion of organic matter in the rumen and manure, and by denitrification and nitrification processes which occur in manure. The quantification of these emissions by experimental methods is difficult and takes considerable time for analysis of the implications of the outputs from empirical studies, and for adaptation and mitigation strategies to be developed. To overcome these problems, computer simulation models offer substantial scope for predicting GHG emissions. These models often include all farm activities while accurately predicting the GHG emissions, including both direct as well as indirect sources. The models are fast and efficient in predicting emissions and provide valuable information on implementing the appropriate GHG mitigation strategies on farms. Further, these models help in testing the efficacy of various mitigation strategies that are employed to reduce GHG emissions. These models can be used to determine future adaptation and mitigation strategies, to reduce GHG emissions thereby combating livestock induced climate change.
Introduction
Global warming, the rise in the average surface temperature of Earth has been attributed to greenhouse gases (GHGs) like carbon dioxide (CO2), methane (CH4), water vapor (H2O), nitrous oxide (N2O) and hydroflurocarbons (HFCs) through the “greenhouse effect” and is an alarming issue worldwide (Smit et al., 2014; Li et al., 2016). The increasing atmospheric concentrations of GHGs in recent years are primarily due to the anthropogenic activities involving fossil fuel burning, application of nitrogen fertilizers in farming and the rearing and breeding of large ruminants. In actuality GHG's are necessary for human survival. Over millennium GHGs have stabilized in the atmosphere resulting in an average surface temperature of 15°C. Without the heat retention capacity of GHG the Earth would likely be uninhabitable. Natural processes are contributing to increasing levels of atmospheric GHG; however, the aforementioned anthropogenic activities are now contributing to additional warming, leading to rapid climate change (IPCC-Intergovernmental Panel on Climate Change, 2013; Quaghebeur et al., 2015).
According to the IPCC-Intergovernmental Panel on Climate Change (2013), over the period 1880–2012 the average land and ocean temperature increased by 0.85°C (0.65 to 1.06°C), and the last three decades were warmer than any other decade before 1850. The number of cold days and nights have decreased and warm days and night have increased. In addition, the frequency of heat waves has increased over Asia, Europe and Australia (IPCC-Intergovernmental Panel on Climate Change, 2013). The sea surface temperature over the Northern Hemisphere increased compared to the Southern Hemisphere. Over the period 1971 to 2010 the upper ocean (0 to 75 m depth) warmed by 0.11°C (0.09 to 0.13°C). Increasing temperature has resulted in the melting of glaciers and Arctic sea ice. Melting of the Greenland ice sheet has increased from 34Gt yr−1 to 215Gt yr−1 over the period 1992 to 2011 and Antarctic ice sheet melting increased from 30Gt yr−1 to 147Gt yr−1 (IPCC-Intergovernmental Panel on Climate Change, 2013). Due to the melting of ice the sea level has increased by 0.19 m (0.017 to 0.21 m) over the period 1901 to 2010 (IPCC-Intergovernmental Panel on Climate Change, 2013). Rainfall distribution has shown high variability in both the hemispheres. Averaged over the mid-latitudes the Northern Hemisphere has experienced increased in rainfall, whereas the Southern Hemisphere has experienced decreased rainfall. Furthermore, extreme weather events appear to be increasing in frequency globally.
Based on data from 2004, CO2 contributed 77%, CH4 14%, N2O 8%, and HFCs 1% of global GHG emissions (IPCC-Intergovernmental Panel on Climate Change, 2007). The current concentrations of CO2, CH4, and N2O are 395.4 ppm, 1893 ppb, and 326 ppb respectively, having a lifetime of 100–300, 12, and 121 years, respectively. Atmospheric concentrations of CO2 have increased by 16 times between 1900 and 2008 (Le Quéré et al., 2014; Beyzavi et al., 2015). The intensity of warming for each gas is referred to as the Global Warming Potential (GWP). The GWP of each gas is determined relative to the GWP of CO2, which is given a value of one. The GWP of CH4, N2O, and hydroflurocarbons are 23, 296, and 12000 respectively (UNEP, 2012). These gases are naturally occurring, but the increasing concentration due to the anthropogenic effects is of concern. GHG emissions have been determined for the industrial/agricultural sector. Energy generation contributes 26% of total global emissions, industries 19%, land use, land change and forestry 17%, agriculture 14%, residential and commercial buildings 8%, and waste and wastewater contribute 3% (IPCC-Intergovernmental Panel on Climate Change, 2007).
The livestock sector is considered to be a major contributor to climatic change (Steinfeld et al., 2006). FAO (2006) presented an aggregated view about the impacts of livestock on climate change. The direct and indirect effects of animal agriculture on climate change were espoused. The sources of GHG from livestock production systems were determined to be from land use and land change, feed production, animal production, manure management, and processing and transportation. A US Environmental Protection Agency report which was also published in 2006 suggested that by 2020 global enteric CH4 emission would be 2344 Mt CO2–eq/yr and CH4 emissions from manure storage to be at 523 Mt CO2–eq/yr (EPA-US Environmental Protection Agency, 2006). Emissions of N2O from cropping practices were estimated to reach 2937 Mt CO2–eq/yr by 2020 (EPA-US Environmental Protection Agency, 2006).
It is clear that climate change is real and that the forces behind this change are GHG emissions. It is inevitable that renewable energy will form a component of, any attempts to reduce GHG emissions from the livestock sector. However, using renewable energy in livestock production is not enough. The livestock sector needs to focus emissions reduction strategies on management approaches that can be applied to livestock in the field. It has been estimated that approximately 12.5% of the total global GHG emission are from the livestock sector (Steinfeld et al., 2013) and 80% of the total emission from agriculture is from the livestock sector. Approximately 9% of CO2, 36% of CH4, and 64% of N2O is contributed to the livestock production process and 75% of the CO2 emitted from livestock is from ruminants (Prasad et al., 2015). Methane and N2O have a GWP of 23 and 296 with a lifetime of 12 and 114 years, respectively, whereas CO2 has a lifetime of 100–300 years (Le Quéré et al., 2014). Mitigating the emission of CO2 in the livestock sector will be less effective in reducing the effects of GHGs compared to the mitigation of short living gases like CH4 and N2O which are the major GHG from the livestock sector. This review is an attempt to highlight the role of livestock in contributing to climate change through enteric fermentation and manure management. Special emphasis has been given to highlighting the difficulties in conducting on farm mitigation studies and signifying the importance of modeling as an alternative for finding solution in curtaining livestock related climate change.
Sources of GHGs in Livestock Farms
According to Steinfeld et al. (2006), global livestock agriculture was responsible for 7516 Mt per year of CO2 equivalents (CO2 eq) or 18% of the anthropogenic GHG emissions annually. It is from the animal and manure emissions that 37% of global agricultural CH4 and N2O arise and the remainder is associated with cropping and deforestation (EPA-US Environmental Protection Agency, 2006). The various sources of GHGs from livestock farms are described in Figure 1. Globally dairy animals, including cull cows and dual purpose beef cattle account for approximately 4% of anthropogenic GHG emissions (FAO-Food and Agriculture Organization of the United Nations, 2010). In developed countries the GHG emissions from dairy production are generally lower than in developing countries due to the higher productivity (Hagemann et al., 2011), and better feed quality. According to the EEA (2011), beef and dairy cattle are estimated to contribute 2.1 and 1.2% respectively to anthropogenic GHG inventories in the European Union (EU) whereas in the United States (US) the contributions are 2.75 and 0.55% respectively (EPA-US Environmental Protection Agency, 2006). However, in developed countries where pastoral agriculture is a significant portion of the economy, such as Ireland and New Zealand or developing countries like Brazil and India, the emission contribution from dairy production to the national inventory will be higher (FAO-Food and Agriculture Organization of the United Nations, 2010). It is misleading if the CH4 emissions from the livestock sector are examined only as a proportion of total anthropogenic GHG emission (Knapp et al., 2014). Methane emissions are dependent upon the population size of the ruminants, their productivity, and manure handling system. Reducing CH4 and N2O emissions from livestock production are focused on because they are less expensive to mitigate than CO2 emissions (EPA-US Environmental Protection Agency, 2006; FAO-Food and Agriculture Organization of the United Nations, 2010; Shafer et al., 2011; Gerber et al., 2013).
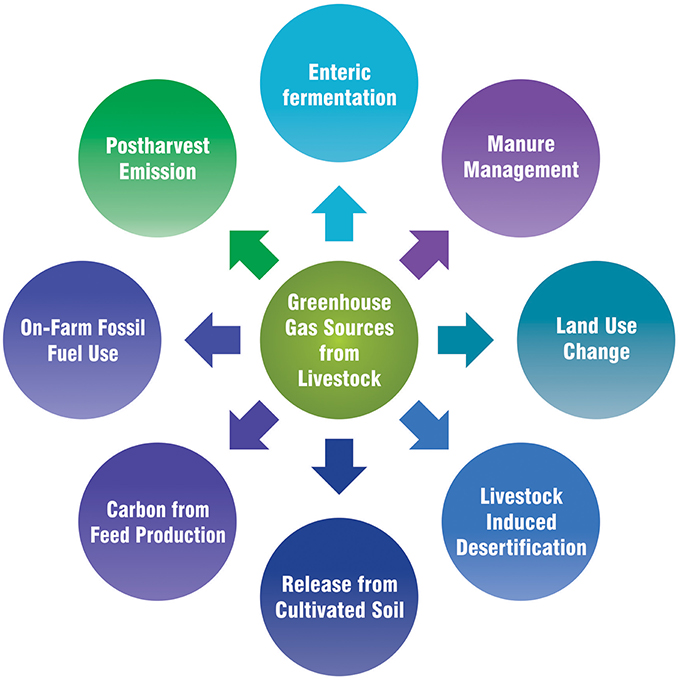
Figure 1. Different sources of GHGs from livestock farms (Adopted from Sejian et al., 2015).
Enteric fermentation causes the emission of CH4, which is a by-product of the breaking down of carbohydrate molecules into soluble particles by methanogens residing within the rumen. Thus, formed CH4 is eructated by the animal and becomes a GHG. Feed quality is a major determinant of CH4 production. High fiber content (cellulose) in the feed will increase CH4 emission. Methane is also produced, as is N2O via the breakdown of manure. When manure is managed in a liquid form the organic matter contained in it are exposed to anaerobic bacteria that decompose the manure and in the process GHGs are formed and liberated. The formation of N2O is by nitrifying and denitrifying bacteria which reside in the soil. The emission of N2O from manure depends upon the nitrogen and carbon content of manure, and for the duration of the storage and type of treatment (IPCC-Intergovernmental Panel on Climate Change, 2006). The oxidation of ammonia nitrogen to nitrate nitrogen (nitrification) is a necessary prerequisite for the emission of N2O from stored animal manures. Nitrification happens in aerobic condition, whereas denitrification occurs in anaerobic conditions in which the nitrites and nitrates are transformed to N2O and dinitrogen (N2). In order for N2O to be produced from manure, nitrites and nitrates are required (under anaerobic conditions) which then allow the formation of the oxidized forms under aerobic conditions (IPCC-Intergovernmental Panel on Climate Change, 2006).
Nitrogen fertilizer, animal manure applications to land and urine deposition by grazing animals are the main sources of emitted N2O (Brown et al., 2001). Unlike CH4, N2O production can only take place if specific conditions are met, i.e., the combined processes of anaerobic and aerobic bacteria: (1) Nitrification, transformation of ammonium to nitrate (aerobic); and (2) Denitrification, formation of nitrogen gas from nitrate reduction (anaerobic). Oxygen content, moisture content and soil conditions will influence N2O emission. Normally due to the anaerobic conditions prevailing in manure, nitrification and denitrification doesn't occur. But when forced and controlled aeration of liquid manure or solid manure for removing organic matter (OM), nitrogen and water (drying) then denitrification occurs after aeration (nitrification). A mixture of manure and straw/litter, results in partial compaction and this forms favorable conditions for passive aeration, resulting in uncontrolled nitrification and denitrification (Groenestein and Van Faassen, 1996). The other sources of GHG emission from livestock farms are, livestock related land use change, feed production, on farm fossil fuel use and post-harvest emission (Steinfeld et al., 2006).
Significance of Reducing GHG from the Livestock Sector
From the above discussion, it is evident that livestock act as an important source of GHG emissions and any attempt to develop mitigation strategies to reduce emissions may be beneficial in slowing climate change. Furthermore, CH4 production through enteric fermentation also contributes to the dietary energy loss. In addition, nutrient use efficiency decreases due to CH4 synthesis. Any factor (feed or management) that reduces nutrient use efficiency will result in greater CH4 emissions. Deficiencies in nutrient requirements for rumen microorganisms reduce microbial growth efficiency, which further reduces microbial biomass resulting in reduced digestibility of foodstuffs and reduced feed intake. In order to combat this, as a consequence of the interspecies hydrogen transfer, the bacteria which are syntrophic to the methanogens produce 1 ATP per molecule of glucose which they utilize for growth and biomass production. It is important to note that this energy is not utilized by the animal. Hence, any attempt to reduce livestock related GHG emissions, apart from the goal of reducing their impact on climate change, may also improve production efficiency of livestock by preventing the dietary energy loss.
Difficulties Associated with Experimental Reduction
The production of GHGs through enteric fermentation and manure management is a complex process. The quantification of these gases often requires complex and expensive equipment. Additionally the collection and measurement of GHGs are time consuming. Further, the mitigation strategies designed for a particular ecological zone will not necessarily be suitable in another zone due to the complexities in the rumen microbial population. In addition, the feeding habits of the animals and the feed resources available may not be the same across agro-ecological zones. Furthermore, the climatic conditions prevailing in a particular locality are a crucial factor influencing GHG production. All these factors are involved in livestock's contribution to GHG emissions and hence climate change. The complexity of various farming systems makes it difficult to identify appropriate mitigation strategies that can be universally applied. There is every chance that even if a strategy is identified by conventional experiments, by the time the work is completed other components of this complex might influence the gas production in a different way. This means that expensive studies need to be repeated numerous times. Hence, research efforts are needed to identify strategies that may be cost effective, less time consuming and with wider applicability.
Significance of Modeling
Projections indicate that by 2050 animal production is expected to increase by 80% compared to 2005 (Alexandratos and Bruinsma, 2012). There is an increasing global demand for milk and meat, and this demand is being met with increased production from pasture based systems (Fiala, 2008; Thornton, 2010). Projections show that the global annual growth rate of beef to 2050 will be 1.2%, which is very close to the annual growth rate of 1.3% for total meat production to 2050 (Alexandratos and Bruinsma, 2012). Hence there is an urgent need to identify simpler cost effective technologies to quantify GHG emissions and to find appropriate solutions for climate change. Computer simulation models are valuable tools for the study of feedback and feed forward interactions between mitigation of GHG emissions and adaptation to climate change in ruminant based production systems. These models offer substantial scope for identifying solutions to livestock related climate change. The models will also provide strategic direction for Government policies related to climate change and food security. It is inevitable that the models will have complex interactions among farm components and climate systems. Tools and models are being developed to estimate GHG emission from livestock systems in the form of process-based simulation (Schils et al., 2007b), emission factor calculations (Amani and Schiefer, 2011; Colomb et al., 2012), and life cycle assessments (LCA)-based approaches (De Vries and de Boer, 2010; De Boer et al., 2011; Cowie et al., 2012). These models have wide acceptance in the scientific community due to the efforts made to improve the understanding of the effect of various systems and changes in farm performance. Further, these models may serve as an alternative for the expensive, time consuming and technically difficult experimentation in a field and farm scale (Bryant and Snow, 2008).
Modeling of GHGs in Livestock Farms
The primitive models which used the prediction equations corresponding to the nutrient uptake of the animals and the subsequent emission of gases were evaluated. These models are commonly referred to as Empirical/Statistical models. They use simple and uncomplicated regression equations based on feed characteristics. However, these models were used in very costly extensive experiments. The environmental changes and the microbial populations residing in the rumen are not included in these models. No factors other than the feed characteristics are studied. The interactions of various other systems are not evaluated. Further, these models cannot be used to predict the changes in CH4 emissions outside the range they were developed for. Hence, to overcome these drawbacks, mechanistic/dynamic models which simulate CH4 emissions based on a mathematical description of ruminal fermentation biochemistry were developed. These models are not costly and they evaluate the complexities associated with enteric CH4 emission. Mitigation measures can be assessed for their effectiveness under varying scenarios of climate and feed intake at field level. The information pertaining to the climate of the particular ecological zone and the routine management practices being followed in livestock farms is also included in these models (Del Prado et al., 2009; Cullen and Eckard, 2011; Graux et al., 2011, 2012; Bell et al., 2012). These models also take into account the information on microbial population and their efficiency in CH4 production rate. However, the complexity of the systems involved in a mechanistic model makes it difficult to operate. The rapid dynamic changes in metabolic flux during lactation, especially in late pregnancy and early lactation are also difficult to quantify using these models. The models use empirical equations derived from statistical analysis to simulate the enteric CH4 emission. These equations have limitations in their ability to quantify the characteristics of the animals and diets they use (Ellis et al., 2010). The success rate for accurately predicting the GHG emission using these models relies heavily on the quality of the input data, such as the chemical composition of the diet, degradation rates of feed components, and passage rates.
Components of Modeling
Input flows and output flows in the livestock sector depends upon the management practices and the environmental conditions prevailing at the site. For each gas the emission mechanism is different. Carbon dioxide emissions depend on C intake and the fixation processes linked with respiration and the direct energy use. Methane emission occurs due to the enteric fermentation in the rumen and the manure management under anaerobic conditions. Denitrification and nitrification processes in manure storages and soils, and the leaching of NO3 and volatilization of NH3 results in N2O emission. Although these are all the primary pathways by which the models predict the GHGs that are being produced from livestock farms, emissions from other sectors beyond the boundaries of the farm have been considered in few models. The models have used different approaches to incorporating all of the components into a single system. The objectives of each model differ: such as GHG emission estimates, GHG mitigation measures and implications of various adaptation and mitigation strategies in on the farm. The various components in the models have to be interrelated effectively if the objective (correct output from the model) is to be achieved. To adequately analyze animal productivity, emission estimates, feeding practices, and animal type the models need to be quantified based on metabolic parameters. Manure management is incorporated into the model by quantifying the flows transformations of manure on the farm and the emission are simulated. Further, information pertaining to housing of the animals, manure storage facilities, treatment of manure and application of manure in the field is programmed into these models. The different livestock related activities and their contribution to existing GHG pool are described in Figure 2.
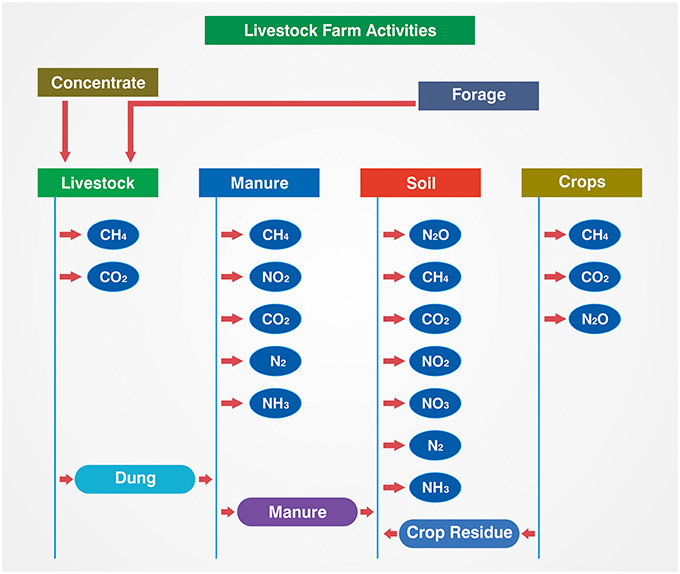
Figure 2. Pictorial representation of different livestock related activities and their contribution to GHG pool.
In addition, the dry matter (DM) volume and the liquid content of manure is dynamically tracked for the C and N fractions (Olesen et al., 2006; Chardon et al., 2012; Rotz et al., 2012). Often CH4 is modeled using the IPCC Tier 2 approach (IPCC-Intergovernmental Panel on Climate Change, 2006) while NH3 and N2O emissions are modeled by volatilization and aerobic condition of manure respectively (Rotz et al., 2012).
For the estimation of N2O emission and C sequestration management practices and their interactions between soil, animal, plant and weather conditions are evaluated. The process based models look into the various interactions and dynamic changes happening to the N pathway (Li et al., 2012) such as denitrification and nitrification processes where N intermediates for N2O and N2 production (Firestone and Davidson, 1989). Temporal variability of N2O depends upon the temperature, rainfall pattern and the amount of N substrate availability and by proper modeling of climate and management practices it is evaluated.
For grazing animals the pasture availability is very important and it can be modeled as the function of soil water, N availabilities and weather condition. From the empirical equations (Foley et al., 2011) mechanistic models are developed by incorporating soil characteristics, ambient temperature and solar radiation as the driving factors (Del Prado et al., 2011; Rotz et al., 2012). Different grazing systems can be included in the model (rotational grazing being the most difficult one to simulate; Graux et al., 2011). Spatial variability of the pastures is incorporated in some models, while others assume uniform distribution over the whole field. The variations in GHG emission with soil properties can be analyzed accurately using this simulation (Linn and Doran, 1984; Ruser et al., 2006).
Soil C constitutes the other component in the field. Soil is the third largest global C pool (Lal, 2008). Improved management of grasslands for increased forage production has the potential to increase C stocks (Freibauer et al., 2004; Rees et al., 2005). The C stock and GHG emission are interrelated, and by modifying the quality and composition of manure, land use changes, variability in feed intake and wider variations in the microbial activity will change the metabolic functions and thereby emission quantity (Vellinga and Hoving, 2011). Natural sources and sinks are not included in the models as they are insignificant contributors to CO2 changes in the atmosphere. During long term analyses C sequestration is not considered because C assimilated equals C stored and emitted C from the farm (Del Grosso et al., 2002; Matthews et al., 2010).
Many models have been developed to analyze animal productivity in different environmental conditions and the associated GHG emission. MITERRA- (a Europen model) is an environmental assessment model used to assess the effects of the implementation of NH3 and NO3 measures and policies on the GHG emissions such as CH4, N2O, and CO2. The MITERRA-Europe model is partly based on the models GAINS (Greenhouse Gas and Air Pollution Interactions and Synergies) and CAPRI (Common Agricultural Policy Regionalised Impact), complemented with an N leaching module, a soil C module and a module for mitigation (Lesschen et al., 2011). It measures the emission from enteric fermentation, manure management, N2O emission, fertilizer production, organic soil cultivation, liming, and fossil fuel use. The data for livestock numbers, crop areas, and animal production are inputted from the databases of FAO and Eurostat and for emission factors from IPCC, GAINS and spatial environmental data. This model is able to simulate nitrogen and GHG emissions however, at the global scale further validation and model comparisons are needed to increase the confidence intervals for the model. The feed intake parameter needs to be further tested and parameterized. Table 1 describes the different models available and their merits and demerits.
The Integrated Model to Assess the Global Environment (IMAGE) model studies the long term effects of human activities on the environment. The regional production of food, animal production, timber harvest, local climatic conditions and terrain the evaluation are incorporated within the model. The land use and cover are efficiently simulated in this model (Kram and Stehfest, 2006; Neumann et al., 2011). The model evaluates the efficient population needed in a region and estimates the feed requirement by the animals (Bouwman et al., 2005; Neumann et al., 2011). IMAGE distinguishes the pastoral livestock systems based on the grazing ruminants and mixed and landless (industrial) production systems, integrating crop and livestock production where animals are fed with a mixture of crops, grasses, and fodder and crop residues.
The LEITAP model which is based upon the Global Trade Analysis Project (GTAP) model was developed to evaluate the changes associated with GHG emission based on the number of animals on a national level. It is formulated based on the neoclassical microeconomic theory multiregional, multisectorial, static, and the applied general equilibrium model. Projections for livestock numbers and agricultural land use were calculated for the EU for 2010, 2020, and 2030 (Neumann et al., 2011). For the estimation of GHG emissions from livestock farms within the EU a model named FarmGHG was created. This model evaluates the emission of CH4 and N2O released from farms and the CO2, CH4, and N2O emission from feed, fertilizer and imported energy. This model was developed to quantify the effects of management practices on emissions of GHGs. The model proposed that by increasing N use, efficiency the emission of GHG can be reduced.
The DairyWise model was developed to estimate GHG emissions from dairy farms. This empirical model integrates all the major systems in the dairy into a whole farm model. Inputs such as farm management, herd type, cropping plan, soil characteristics, grass and feed management, buildings and equipment are quantified in this model (Schils et al., 2007a). The CH4 emission is calculated from the enteric fermentation and N2O is calculated from the manure management. Both direct and indirect N2O emissions are simulated where direct emissions for N inputs through fertilizers, manure application, biological fixation, and urine excreted during grazing, crop residues, and peat oxidation (Schils et al., 2007b).
The Sustainable and Integrated Management Systems for Dairy Production (SIMS-Dairy) model simulates biodiversity, landscape, animal welfare, soil quality and product quality (Del Prado and Scholefield, 2006). It focuses on the management practices in the livestock sector and aims to obtain a sustainable system. The possible impacts of mitigation strategies on the emission of GHG are studied in this model. Emission from soil, animal excreta as manure, or urine and emissions from the rumen are analyzed (Schils et al., 2007b).
The FARM SIMulation (FarmSim) model simulates GHG emission from nine interacting modules. The flow of product among various components of the farm system is included in this model. PASIM model where GHGs exchanged over the different grassland types on the farm are integrated with IPCC Tier 1 and Tier 2 methodology, where emissions from cropland and cattle housing are evaluated and included in the FarmSim model. The structure of the farm, including the area, type of crop(s), grassland and herd type, the number of herd per type, the area of grasslands, the crops and the feeding and waste management systems are inputted into the model.
The Integrated Farm System Model (IFSM) integrates the biological and physical processes of a crop, beef or dairy farm in order to simulate crop production, feed use and manure output over a period of time while at the same time incorporating the weather parameters (Rotz et al., 2009). The crop production of alfalfa, grass, corn, soybean, and small grain crops can be predicted based on daily soil and weather conditions. The feed consumed by an animal and the response (e.g., average daily gain) are related to the nutrient content of the feed. The manure quality and quantity is based upon the nutrient content of the feed consumed. When simulating GHG emission modules for the balance of C will need to be based upon processes like crop production, animal respiration and microbial respiration plus manure management. Certain criteria have been formulated for the potential evaluation of the models The models should (i) simulate the processes by which CO2 emissions will be affected when farm management practices change; (ii) represent every process influencing GHG emissions; (iii) predict the observed data in the past for its accuracy under different potential conditions; and (iv) accurate data should be available for the accurate simulation.
The Global Livestock Environmental Assessment Model (GLEAM) explores the environmental implications of on farm production practices (Gerber et al., 2013). Its development is based upon five modules which reproduce the main elements of livestock supply chains: (1) Herd module; this module evaluate the number of animals per GIS grid cell, where they have been managed with different farming systems; the herd characteristics and structure of each cell are studied under this module; (2) Manure module; evaluates the manure production from the GIS cell from each animal type; (3) Feed module; calculates the various components in the feed, nutrient content and the emissions per kg of feed given; (4) System module; incorporates the herd, manure and feed modules to determine the energy requirement by each animal type, the annual production from each GIS cell, emissions produced from manure management, enteric fermentation and feed production, and (5) Allocation module; calculates the total GHG emission from the farm incorporating all the direct, indirect and post farm emissions. GLEAM uses geo-referenced data to calculate the GHG emissions from the farm. The information regarding the productivity and the practices undertaken to increase livestock production is collected at various levels of aggregation such as at a country level, agro-ecological zones, or a combination of these. The main data sources are Gridded Livestock of the World (FAO-Food and Agriculture Organization of the United Nations, 2007), National Inventory Reports of Annex I countries (UNFCCC-United Nations Framework Convention on Climate Change., 2009), International Food Policy Research Institute (IFPRI), Life Cycle Inventory data from SIK, International Agricultural Research (CGIAR) and statistics from FAO (FAOSTAT, 2009).
Advantages of Modeling Over Conventional Experiments
The models outlined above are being used for simulating and predicting the GHG emission from the livestock sector. However, further development is required to improve the accuracy of the model outputs. Components of the model must include modules which are comprised of animal, crop, soil, and climate data. Models need to be developed based upon the various animal characteristics, their feeding habits, metabolic fluxes, microbial population, manure management, farm management, and climatic conditions. These models should be region specific rather than global as the components of model may be different for different regions. Furthermore, the parameters that are standardized for one agro-ecological zone may not be the same for another. In addition, the climatic conditions will also differ between regions. For example the body weight of a cow in Europe will be different from that of Asia. Any differences will result in errors if the same standardized values made for another region are used. The model assumptions have to be changed according to the conditions prevailing in respective regions. These regionalized models can be effectively used to simulate and predict the GHG emissions from livestock enterprises. These models could become an alternative solution for livestock related climate change by initiating quick actions to mitigate such emissions. Doing experiments in the field may take years to quantify the emissions and to analyze the implications of various mitigation strategies employed for the reduction of emission. However, through modeling each variation in any of the targeted parameters can be identified real time. Using simulation models we can vary conditions at the farm level that affect the metabolic mechanism of animals and fermentation processes. The model outputs can then be used to evaluate potential mitigation strategies. Projection of emissions from the animals can be projected into the future. This is not possible in experimental conditions. The projections can be used to formulate the appropriate mitigation strategies for the future, thus making management strategies more systematic and efficient.
Conclusion
Livestock undoubtedly need to be a priority focus as the global community seeks to address the challenge of climate change. The magnitude of the discrepancy between the estimates illustrates the need to provide the climate change community and policy makers with accurate GHG emission estimates and information about the link between agriculture and climate. Improving the global estimates of GHG attributed from livestock systems is of paramount importance. This is not only to define the magnitude of the impact of livestock on climate change, but to understand their contribution relative to other sources of GHG. Estimates of GHG emission through experiments under different production system is practically impossible and with growing awareness of global warming and its continuous negative impact on agricultural production systems, attention should be directed toward immediate mitigation strategies to curtail such emissions.
The complexity of various farming systems makes it difficult to identify appropriate mitigation strategies that can be universally applied. Hence simulation models offer huge scope as these models may serve as an alternative for the expensive, time consuming and technically difficult experimentation in a field and farm scale. Such information will enable effective mitigation options to be designed to reduce emissions and improve the sustainability of the livestock sector, while continuing to provide livelihoods and food for a wide range of people.
A synthesis of the available literature suggests that the mechanistic models are superior to empirical models in accurately predicting the CH4 emission from farms. The latest development in prediction model is the IFSM which is a process-based whole-farm simulation technique. The IFSM takes into account the entire livestock farm operations, including breed of animal, production stage, available feed resources, grazing information, pasture management, manure handling, and local weather condition. It is possible through these models to evaluate the variations in GHG emission by altering any of the targeted parameters in real time mode. Thus, these models could become an alternative solution for livestock related climate change by initiating quick actions to mitigate such emissions.
Future Perspectives
Farmers typically adopt the most cost-effective and easy-to-adapt options. The services provided by the models have currently no market value among the farming community, but may become valuable in the future. Although many modeling studies are being undertaken, they do not have the capability to quantify the potential interactions among ecosystem services. The rapid dynamic changes in metabolic flux during lactation, especially in late pregnancy and early lactation have to be rectified in future models. There is also a need to integrate the effects of climate change on plant protection issues, pollination and risks from pathogens. Because this can affect the safety of the feed quality given to the animals and the microbial population is affected badly by the pesticide actions within the rumen. A balanced systems-based approach to quantify synergies and trade-offs is still lacking in current models because of the inherent complexity of some of these relationships. Multifunctionality in farms implies greater levels of heterogeneity in farming systems, and hence increase the complexity of the farm scenarios to be modeled.
Author Contributions
VJ-Literature collection and introduction preparation; VS-Preparation of outline and editing manuscript; MB-Collected information and prepared write up on sources of GHGs in livestock farms; AR-Collected information and prepared write up on significance of reducing GHG from the livestock sector; AL-Collected information and prepared write up on difficulties associated with experimental reduction; YA-Collected information and prepared write up on significance of modeling; MS-Collected information and prepared write up on modeling of GHGs in livestock farms; RB-Collected information and prepared write up on advantages of modeling over conventional experiments; JG-Prepared conclusion and future perspectives.
Conflict of Interest Statement
The authors declare that the research was conducted in the absence of any commercial or financial relationships that could be construed as a potential conflict of interest.
References
Alexandratos, N., and Bruinsma, J. (2012). World Agriculture Towards 2030/2050: The 2012 Revision. ESA Working Paper No. 12-03. Rome: FAO.
Amani, P., and Schiefer, G. (2011). Review on suitability of available LCIA methodologies for assessing environmental impact of the food sector. Int. J. Food Syst. Dyn. 2, 194–206. doi: 10.18461/ijfsd.v2i2.228
Bell, M. J., Eckard, R. J., and Cullen, B. R. (2012). The effect of future climate scenarios on the balance between productivity and greenhouse gas emissions from sheep grazing systems. Livest. Sci. 147, 126–138. doi: 10.1016/j.livsci.2012.04.012
Beyzavi, M. H., Stephenson, C. J., Liu, Y., Karagiaridi, O., Hupp, J. T., and Farha, O. K. (2015). Metal–organic framework-based catalysts: chemical fixation of CO2 with epoxides leading to cyclic organic carbonates. Front. Energy Res. 2:63. doi: 10.3389/fenrg.2014.00063
Bouwman, A. F., Hoek, K. W., Eickhout, B., and Soenario, I. (2005). Exploring changes in world ruminant production systems. Agr. Sys. 84, 121–153. doi: 10.1016/j.agsy.2004.05.006
Brown, L., Armstrong Brown, S., Jarvis, S. C., Syed, B., Goulding, K. W. T., Phillips, V. R., et al. (2001). An inventory of nitrous oxide emissions from agriculture in the UK using the IPCC methodology: emission estimate, uncertainty and sensitivity analysis. Atmos. Environ. 35, 1439–1449. doi: 10.1016/S1352-2310(00)00361-7
Bryant, J. R., and Snow, V. O. (2008). Modelling pastoral farm agro-ecosystems: a review. New Zealand J. Agr. Res. 51, 349–363. doi: 10.1080/00288230809510466
Chardon, X., Rigolot, C., Baratte, C., Espagnol, S., Raison, C., Martin-Clouaire, R., et al. (2012). MELODIE: a whole-farm model to study the dynamics of nutrients in dairy and pig farms with crops. Animal 6, 1711–1721. doi: 10.1017/S1751731112000687
Chianese, D. S., Rotz, C. A., and Richard, T. L. (2009). Whole-farm gas emissions: a review with application to a Pennsylvania dairy farm. Appl. Eng. Agric. 25, 431–442. doi: 10.13031/2013.26895
Colomb, V., Bernoux, M., Bockel, L., Chotte, J. L., Martin, S., Martin-Phipps, C., et al. (2012). Review of GHG calculators in agriculture and forestry sectors. A Guideline and Appropriate Choice and Use of Landscape Based Tools. Available online at: http://www.fao.org/fileadmin/templates/ex_act/pdf/Review_existingGHGtool_FR.pdf
Cowie, A., Eckard, R., and Eady, S. (2012). Greenhouse gas accounting for inventory, emissions trading and life cycle assessment in the land-based sector: a review. Crop Pasture Sci. 63, 284–296. doi: 10.1071/CP11188
Cullen, B. R., and Eckard, R. J. (2011). Impacts of future climate scenarios on the balance between productivity and total greenhouse gas emissions from pasture based dairy systems in south-eastern Australia. Anim. Feed Sci. Tech. 166–167, 721–735. doi: 10.1016/j.anifeedsci.2011.04.051
De Boer, I. J. M., Cederberg, C., Eady, S., Gollnow, S., Kristensen, T., Macleod, M., et al. (2011). Greenhouse gas mitigation in animal production: towards an integrated life cycle sustainability assessment. Curr. Opin. Environ. Sustain. 3, 423–431. doi: 10.1016/j.cosust.2011.08.007
Del Grosso, S., Ojimaa, D., Parton, W., Mosier, A., Peterson, G., and Schimel, D. (2002). Simulated effects of dryland cropping intensification on soil organic matter and greenhouse gas exchanges using the DAYCENT ecosystem model. Environ. Pollut. 116, 75–83. doi: 10.1016/S0269-7491(01)00260-3
Del Prado, A., Misselbrook, T., Chadwick, D., Hopkins, A., Dewhurst, R. J., Davison, P., et al. (2011). SIMSDAIRY: a modelling framework to identify sustainable dairy farms in the UK. Framework description and test for organic systems and N fertiliser optimisation. Sci. Total Environ. 409, 3993–4009. doi: 10.1016/j.scitotenv.2011.05.050
Del Prado, A., and Scholefield, D. (2006). Use of SIMSDAIRY modelling framework system to specify sustainable UK dairy farms. Delivering sustainability within profitable farming systems — is it possible? Asp. Appl. Biol. 80, 73–80. doi: 10.1017/S0021859608007727
Del Prado, A., Shepherd, A., Wu, L., Topp, C., Moran, D., Tolkamp, B., et al. (2009). Modelling the Effect of Climate Change on Environmental Pollution Losses from Dairy Systems in the UK. BC3 Working Paper Series 2009-07. Bilbao: Basque Centre for Climate Change (BC3).
De Vries, M., and de Boer, I. J. M. (2010). Comparing environmental impacts for livestock products: a review of life cycle assessments. Livest. Sci. 128, 1–11. doi: 10.1016/j.livsci.2009.11.007
EEA (European Environment Agency) (2011). The Application of Models Under the European Union's Air Quality Directive: A Technical Reference Guide. EEA Technical report No 10/2011. Available online at: http://www.eea.europa.eu/publications/fairmode
Ellis, J. L., Bannink, A., France, J., Kebreab, E., and Dijkstra, J. (2010). Evaluation of enteric methane prediction equations for dairy cows used in whole farm models. Glob. Change Biol. 16, 3246–3256. doi: 10.1111/j.1365-2486.2010.02188.x
EPA-US Environmental Protection Agency (2006). Available online at: http://www.epa.gov/methane/sources.html
FAO-Food Agriculture Organization of the United Nations (2007). Gridded Livestock of the World 2007. Rome: FAO-Food and Agriculture Organization of the United Nations
FAO-Food Agriculture Organization of the United Nations (2010). Greenhouse Gas Emissions from the Dairy Sector. A Life Cycle Assessment. FAO-Food and Agriculture Organization of the United Nations.
Fiala, N. (2008). Meeting the demand: an estimation of potential future greenhouse gas emissions from meat production. Ecol. Econom. 67, 412–419. doi: 10.1016/j.ecolecon.2007.12.021
Firestone, M. K., and Davidson, E. A. (1989). “Microbiological basis of NO and N2O production and consumption in soil,” in Exchange of Trace Gases between Terrestrial Ecosystems and the Atmosphere, eds M. O. Andreae and D. S. Schimel (Chichester: John Wiley & Sons), 7–21.
Foley, P. A., Crosson, P., Lovett, D. K., Boland, T. M., O'Mara, F. P., and Kenny, D. A. (2011). Whole-farm systems modelling of greenhouse gas emissions from pastoral suckler beef cow production systems. Agri. Ecosyst. Environ. 142, 222–230. doi: 10.1016/j.agee.2011.05.010
Freibauer, A., Rounsevell, M. D. A., Smith, P., and Verhagen, J. (2004). Carbon in the agricultural soils of Europe. Geoderma 122, 1–23. doi: 10.1016/j.geoderma.2004.01.021
Gerber, P. J., Steinfeld, H., Henderson, B., Mottet, A., Opio, C., Dijkman, J., et al. (2013). Tackling Climate Change through Livestock: A Global Assessment of Emissions and Mitigation Opportunities. Rome: Food and Agriculture Organization of the United Nations (FAO).
Graux, A. I., Gaurut, M., Agabriel, J., Baumont, R., Delagarde, R., Delaby, L., et al. (2011). Development of the pasture simulation model for assessing livestock production under climate change. Agri. Ecosyst. Environ. 144, 69–91. doi: 10.1016/j.agee.2011.07.001
Graux, A. I., Lardy, R., Bellocchi, G., and Soussana, J. F. (2012). Global warming potential of French grassland-based dairy livestock systems under climate change. Reg. Environ. Change 12, 751–763. doi: 10.1007/s10113-012-0289-2
Groenestein, C. M., and Van Faassen, H. G. (1996). Volatilization of ammonia, nitrous oxide and nitric oxide in deep-litter systems for fattening pigs. J. Agri. Eng. Res. 65, 269–274. doi: 10.1006/jaer.1996.0100
Hagemann, M., Hemme, T., Ndambi, A., Alqaisi, O., and Sultana, M. N. (2011). Benchmarking of greenhouse gas emissions of bovine milk production systems for 38 countries. Anim. Feed Sci. Technol. 166–167, 46–58. doi: 10.1016/j.anifeedsci,.2011.04.002
IPCC-Intergovernmental Panel on Climate Change (2006). IPCC Guidelines for National Greenhouse Gas Inventories, Vol. 4: Agriculture, Forestry and Other Land Use. Hayama: IGES.
IPCC-Intergovernmental Panel on Climate Change (2007). Climate Change 2007: Synthesis Report. Contribution of Working Groups I, II and III to the Fourth Assessment Report of the Intergovernmental Panel on Climate Change, p. 104. Geneva, Switzerland, IPCC. Available online at: http://www.ipcc.ch/publications_and_data/publications_ipcc_fourth_assessment_report_synthesis_report.htm
IPCC-Intergovernmental Panel on Climate Change (2013). “Summary for Policymakers,” in Climate Change 2013: The Physical Science Basis. Contribution of Working Group I to the Fifth Assessment Report of the Intergovernmental Panel on Climate Change. New York, NY; Cambridge: Cambridge University Press), 1–30.
Knapp, J. R., Laur, G. L., Vadas, P. A., Weiss, W. P., and Tricarico, J. M. (2014). Invited review: enteric methane in dairy cattle production: quantifying the opportunities and impact of reducing emissions. J. Dairy Sci. 97, 3231–3261. doi: 10.3168/jds.2013-7234
Kram, T., and Stehfest, E. (2006). “The IMAGE model: history, current status and prospects,” in Integrated modelling of global environmental change, eds A. F. Bouwman, T. Kram, and K. K. Goldewijk (Bilthoven: Netherlands Environmental Agency), 7–24.
Lal, R. (2008). Carbon sequestration. Philos. Trans. R. Soc. 363, 815–830. doi: 10.1098/rstb.2007.2185
Le Quéré, C., Moriarty, R., Andrew, R. M., Peters, G. P., Ciais, P., Friedlingstein, P., et al. (2014). Global Carbon Budget 2014. Earth Syst. Sci. Data 7, 521–610. doi: 10.5194/essdd-7-521-2014
Lesschen, J. P., van den Berg, M., Westhoekb, H. J., Witzkec, H. P., and Oenema, O. (2011). Greenhouse gas emission profi les of European livestock sectors. Anim. Feed Sci. Technol. 166–167, 16–28. doi: 10.1016/j.anifeedsci.2011.04.058
Li, C., Salas, W., Zhang, R., Krauter, C., Rotz, A., and Mitloehner, F. (2012). Manure-DNDC: a biogeochemical process model for quantifying greenhouse gas and ammonia emissions from livestock manure systems. Nutr. Cycl. Agroecosys. 93, 163–200. doi: 10.1007/s10705-012-9507-z
Li, Q., Gupta, S., Tang, L., Quinn, S., Atakan, V., and Riman, R. E. (2016). A novel strategy for carbon capture and sequestration by rHLPD Processing. Front. Energy Res. 3:53. doi: 10.3389/fenrg.2015.00053
Linn, D. M., and Doran, J. W. (1984). Effect of water-filled pore space on carbon dioxide and nitrous oxide production in tilled and nontilled soils. Soil Sci. Soc. Am. J. 48, 1267–1272. doi: 10.2136/sssaj1984.03615995004800060013x
Matthews, R. A., Chadwick, D. R., Retter, A. L., Blackwell, M. S. A., and Yamulki, S. (2010). Nitrous oxide emissions from small-scale farmland features of UK livestock farming systems. Agri. Ecosys. Environ. 136, 192–198. doi: 10.1016/j.agee.2009.11.011
Neumann, K., Verburg, P. H., Elbersen, B., Stehfest, E., and Woltjer, G. B. (2011). Multi-scale scenarios of spatial-temporal dynamics in the European livestock sector. Agri. Ecosys. Environ. 140, 88–101. doi: 10.1016/j.agee.2010.11.015
Olesen, J. E., Schelde, K., Weiske, A., Weisbjerg, M. R., Asman, W. A. H., and Djurhuus, J. (2006). Modelling greenhouse gas emissions from European conventional and organic dairy farms. Agri. Ecosys. Environ. 112, 207–220. doi: 10.1016/j.agee.2005.08.022
Prasad, R. J., Sourie, S. J., Cherukuri, V. R., Fita, L., and Merera, C. E. (2015). Global warming: genesis, facts and impacts on livestock farming and mitigation strategies. Int. J.Agric. Innov. Res. 3, 2319–1473.
Quaghebeur, M., Nielsen, P., Horckmans, L., and Mechelen, D. V. (2015). Accelerated carbonation of steel slag compacts: development of high-strength construction Materials. Front. Energy Res. 3:52. doi: 10.3389/fenrg.2015.00052
Rees, R. M., Bingham, I. J., Baddeley, J. A., and Watson, C. A. (2005). The role of plants and land management in sequestering soil carbon in temperate arable and grassland ecosytems. Geoderma 128, 130–154. doi: 10.1016/j.geoderma.2004.12.020
Rotz, C. A., Corson, M. S., Chianese, D. S., and Coiner, C. U. (2009). The Integrated Farm System Model: Reference Manual. University Park, PA: USDA-ARS Pasture Systems and Watershed Management Research Unit.
Rotz, C. A., Corson, M. S., Chianese, D. S., Montes, F., Hafner, S. D., Jarvis, R., et al. (2012). Integrated Farm System Model: Reference Manual. USDA Agricultural Research Service. Available online at: http://www.ars.usda.gov/Main/docs.htm?docid521345
Ruser, R., Flessa, H., Russow, R., Schmidt, G., Buegger, F., and Munch, J. C. (2006). Emission of N2O, N2 and CO2 from soil fertilized with nitrate: effect of compaction, soil moisture and rewetting. Soil Biol. Biochem. 38, 263–274. doi: 10.1016/j.soilbio.2005.05.005
Saletes, S., Fiorelli, J., Vuichard, N., Cambou, J., Olesen, J. E., Hacala, S., et al. (2004). “Greenhouse gas balance of cattle breeding farms and assessment of mitigation options,” in Greenhouse Gas Emissions from Agriculture. Mitigation Options and Strategies, eds M. Kaltschmitt and A. Weiske (Leipzig: Institute for Energy and Environment), 203–208.
Schils, R. L. M., de Haan, M. H. A., Hemmer, J. G. A., van den Polvan Dasselaar, A., de Boer, J. A., Evers, A. G., et al. (2007b). DairyWise, a whole-farm dairy model. J. Dairy Sci. 90, 5334–5346. doi: 10.3168/jds.2006-842
Schils, R. L. M., Olesen, J. E., del Prado, A., and Soussana, J. F. (2007a). A review of farm level modelling approaches for mitigating greenhouse gas emissions from ruminant livestock systems. Livest. Sci. 112, 240–251. doi: 10.1016/j.livsci.2007.09.005
Sejian, V., Hyder, I., Ezeji, T., Lakritz, J., Bhatta, R., Ravindra, J. P., et al. (2015). “Global warming: role of livestock,” in Climate Change Impact on Livestock: Adaptation and Mitigation, eds V. Sejian, J. Gaughan, L. Baumgard, C. S. Prasad, C. S (New Delhi: Springer-Verlag GMbH Publisher), 141–170.
Shafer, S. R., Walthall, C. L., Franzluebbers, A. J., Scholten, M., Meijs, J., Clark, H., et al. (2011). Emergence of the global research alliance on agricultural greenhouse gases. Carbon Manag. 2, 209–214. doi: 10.4155/cmt.11.26
Smit, B., Park, A. A., and Gadikota, G. (2014). The grand challenges in carbon capture, utilization, and storage. Front. Energy Res. 2:55. doi: 10.3389/fenrg.2014.00055
Steinfeld, H., Gerber, P., Wassenaar, T., Castel, V., Rosales, M., and de Haan, C. (2006). Livestock's Long Shadow, Environmental Issues and Options. Livestock, Environment, and Development Initiative. Rome: United Nations Food and Agriculture Organization.
Steinfeld, H., Hann, O., and Black Burn, H. (2013). Livestock-Environment Interactions, Issues and Options. Rome: FAO of United Nations.
Thornton, P. K. (2010). Livestock production: recent trends, future prospects. Philos. Trans. R. Soc. Lond B: Biol. Sci. 365, 2853–2867. doi: 10.1098/rstb.2010.0134
UNFCCC-United Nations Framework Convention on Climate Change (2009). Annex I Party GHG Inventory Submissions. Copenhagen: UNFCCC-United Nations Framework Convention on Climate Change.
Keywords: GHG, IFSM, livestock methane, mitigation, modeling, nitrous oxide
Citation: Jose VS, Sejian V, Bagath M, Ratnakaran AP, Lees AM, Al-Hosni YAS, Sullivan M, Bhatta R and Gaughan JB (2016) Modeling of Greenhouse Gas Emission from Livestock. Front. Environ. Sci. 4:27. doi: 10.3389/fenvs.2016.00027
Received: 16 February 2016; Accepted: 23 March 2016;
Published: 14 April 2016.
Edited by:
Yuhua Duan, United States Department of Energy-National Energy Technology Laboratory, USACopyright © 2016 Jose, Sejian, Bagath, Ratnakaran, Lees, Al-Hosni, Sullivan, Bhatta and Gaughan. This is an open-access article distributed under the terms of the Creative Commons Attribution License (CC BY). The use, distribution or reproduction in other forums is permitted, provided the original author(s) or licensor are credited and that the original publication in this journal is cited, in accordance with accepted academic practice. No use, distribution or reproduction is permitted which does not comply with these terms.
*Correspondence: Veerasamy Sejian, ZHJzZWppYW5AZ21haWwuY29t