- 1Department of Neuroscience, Carleton University, Ottawa, ON, Canada
- 2Institut de Santé Publique, d’Épidémiologie et de Développement (ISPED), École de Santé Publique, Université de Bordeaux, Bordeaux, France
- 3Epidemiology and Biostatistics Unit, Centre Armand-Frappier Santé Biotechnologie, Institut National de la Recherche Scientifique, Université du Québec, Laval, QC, Canada
- 4Department of Social and Preventive Medicine, School of Public Health, Université de Montréal, Montreal, QC, Canada
- 5Centre de Recherche du CHUM, Montréal, QC, Canada
- 6Division of Occupational and Environmental Health, Dalla Lana School of Public Health, University of Toronto, Toronto, ON, Canada
- 7Department of Chemical Engineering and Applied Chemistry, University of Toronto, Toronto, ON, Canada
- 8Health Canada, Environmental Health Science and Research Bureau, Ottawa, ON, Canada
- 9School of Epidemiology and Public Health, University of Ottawa, Ottawa, ON, Canada
- 10Division of Epidemiology, Dalla Lana School of Public Health, University of Toronto, Toronto, ON, Canada
Objectives: Environmental exposures play an important role in the development of breast cancer. The incidence of breast cancer is increasing in young women, and its etiology differs from that of older women. Epidemiological studies have provided mixed evidence about whether proximity to urban greenness reduces the risk of breast cancer, but few studies have evaluated this risk in younger women.
Methods: We investigated associations between residentially-based measures of greenness and breast cancer among participants of the Ontario Environmental Health Study (OEHS). The OEHS was a case–control study of Ontario women, 18–45 years of age, who provided questionnaire data between 2013 and 2015. The study included 465 cases diagnosed with a pathologically confirmed primary diagnosis of breast cancer, and 242 population-based controls. Residentially-based measures of greenness, the Normalized Difference Vegetation Index (NDVI) and tree coverage percentage, at 100-, 250-, 500-, and 1,000-m buffers, were assigned to the residential histories of the women. Odds ratios and their 95% confidence intervals (CIs) were estimated using logistic regression, adjusted for potential confounders including traffic-related air pollution [nitrogen dioxide (NO2)].
Results: We found no evidence that an increase in NDVI or tree coverage were significantly associated with breast cancer. The adjusted odds ratio of breast cancer in relation to an interquartile range increase (IQR) in the NDVI (500-m buffer) was 0.86 (95% CI = 0.59–1.13). Similarly, the odds ratio of breast cancer among those in the highest quartile of tree coverage (500-m buffer) relative to the lowest was 1.11 (95% CI = 0.59–2.07). Risk estimates for both measures of greenness did not vary substantially across different buffer distances. Exposure to NO2 was an important confounder in these associations.
Conclusions: Our findings do not support the hypothesis that residential greenness reduces the risk of breast cancer among young women, while highlighting the importance of adjusting for air pollution.
1 Introduction
Breast cancer is the most prevalent cancer in women worldwide (1). In Canada, breast cancer accounted for an estimated one quarter of all newly diagnosed cancer cases, and 14% of deaths among women in 2022 (2). Overall, breast cancer age-standardized incidence rates in Canadian women have slightly declined from 1991 to 2017 by an annual percent change of 0.2% (3). However, between 2000 and 2015, the incidence of breast cancer has increased among Canadian women less than 40 years of age by an average annual value of 0.66% (4). These trends may be partially explained by changes in breast cancer risk factors such as lower birth rates and increased age at first birth (4). However, they are unlikely to be influenced by population screening rates for mammography as, until recently, these procedures were only recommended for those 50 years of age and older (5).
Premenopausal women diagnosed with breast cancer, on average, experience a poorer prognosis relative to those diagnosed at older ages, as they tend to have more aggressive tumors that are less amenable to treatment (6, 7). It is also recognized that women diagnosed with breast cancer at an earlier age are more likely to have a higher genetic susceptibility for breast cancer as demonstrated by the increased presentation of a family history of breast cancer (8) and genetic BRCA mutations when compared with older women (9). The role of other risk factors, particularly obesity, is also recognized to differ by menopausal status (10, 11), suggesting the need for epidemiological studies to characterize these risks separately.
Breast cancer is a multifactorial disease. While several biological and behavioral risk factors including reproductive history, age at menarche, smoking, and alcohol use are well recognized (12), there is an increasing awareness that environmental exposures are important (13). Epidemiological studies of breast cancer have evaluated a diversity of environmental exposures including organophosphate insecticides (14), fine particulate matter (PM2.5), and nitrogen dioxide (NO2) air pollution (15), polycyclic aromatic hydrocarbons (16), and artificial light at night (17). While there have been differences in the risks reported for these exposures across studies, as a whole, the findings suggest that environmental exposures have a greater impact on the risk of premenopausal than postmenopausal breast cancer (14–17).
The urban built environment encapsulates the area around an individual's residence, work, and other locations with which they interact, and this in turn modulates one's behavioral and environmental determinants of health (18, 19). Greenness, also referred to as “green spaces” or “natural vegetation” (20), is a key component of the urban built environment. An expansive literature has shown that greenness has many health benefits including the promotion of physical activity, reduction of stress, enhancement of social interactions, and mitigation of harmful environmental stressors such as noise, urban heat islands, and air pollution (20, 21). Further, epidemiological studies have found that greenness reduces the rates of mortality (22, 23) and the risk of several other diseases and health-related outcomes (24), including cancer (25–27).
To date, a small number of studies have investigated the relationship between greenness and breast cancer. The conclusions from these studies have been inconsistent, with two studies reporting that greenness reduces the risk of breast cancer (27, 28) and four studies finding null results (26, 29–31). An inverse association, as well as a positive association between greenness and breast cancer risk, was even reported in one study that used different measures of greenness (32).
Of the mentioned studies, only two have evaluated whether the association between greenness and breast cancer risk was modified by menopausal status (29, 32). A case–control study of 1,738 cases and 1,910 controls by O'Callaghan-Gordo et al. considered menopausal status as a potential effect modifier (32). The researchers found that an inverse association between the presence of urban green space and the positive association between the Normalized Difference Vegetation Index (NDVI) and breast cancer were not modified by menopausal status. Meanwhile, the Terre-Torras et al. cohort study of 1,798,838 participants found no statistically significant association with either the NDVI or the percentage of greenspace and breast cancer risk in both the pre- and postmenopausal women (29). Differences in findings across the studies are likely due to a number of factors including different measures of greenness, varying spatial resolution of the metrics, use of different buffer intervals, and the ability to control for other risk factors.
Most studies on this topic have modeled surrounding greenness using the NDVI (20). The use of NDVI offers several advantages, most notably an objective overall measure of greenness (or vegetation), thereby avoiding potential biases associated with self-reported measures of greenness. However, the NDVI is unable to distinguish between the various features of greenness such as forest areas, grassy fields, and agricultural lands. The ability to incorporate different features of greenness may be relevant from the perspective of providing insights about the plausibility of different pathways influencing breast cancer development.
This study was undertaken to evaluate whether residential greenness is associated with the development of breast cancer in young women. Our analyses address several research gaps within this topic by incorporating data on multiple measures of greenness at different buffer distances. In addition, the study makes use of previously collected case–control data that include a comprehensive series of environmental, occupational, and lifestyle/behavioral risk factors, including air pollution. As greenness represents a modifiable exposure, our findings may help inform urban design policies with the ultimate goal of improving population health.
2 Materials and methods
2.1 Study population
Data were collected from the Ontario Environmental Health Study (OEHS) (33, 34); a population-based case–control study designed to investigate exposure to environmental, dietary, and occupational risk factors for breast cancer in Ontario women 18–45 years of age. Indeed, the primary objective of the OEHS was to assess whether exposure to polybrominated diphenyl ethers (PBDEs) increased the risk of breast cancer in young women (34). The eligible cases were identified from women who had a newly pathologically confirmed primary diagnosis of breast cancer recorded in the Ontario Cancer Registry (OCR) ePATH system between the years 2013 and 2015. The system allows for a timely identification of all newly diagnosed cases in Ontario (35), and allowed OEHS to recruit women and administer a questionnaire to them typically within 2–3 months of diagnosis. The eligible controls were identified by the Institute for Social Research at York University by using random-digit dialing methods from a sample of Ontario women that were frequency-matched within 5-year age groups. All eligible cases and controls who agreed to be contacted, except those living in Thunder Bay, Timmins, and Sault Ste. Marie (areas that are not serviced by the biological collection laboratory Gamma-Dynacare), were sent a formal invitation to participate by mail (including six follow-up attempts for non-responders). All study participates were required to complete online consent and questionnaires in English on the OEHS website. From the 1,181 potential participants who initially responded to the mail-outs, 70.1% of the cases and 47% of the controls participated in this study.
These study participants completed a web-based Environmental Health Questionnaire (EHQ), which collected information on sociodemographic, lifestyle, anthropometric, physical activity, reproductive, family history, occupational history, and other breast cancer risk factors. Of the individuals who gave online consent, four were excluded from the current study because they did not complete the EHQ. Residential histories in the form of postal code, street address, and the years resided were collected for four different time periods: the time of survey, previous address (if they had moved in the previous 2 years), longest address between the age of 10–17, and address at birth. All participants provided a six-character postal code for their residence at the time of the interview. However, information was only available for 12.7% of participants’ postal codes for their previous address, 71.3% for their address during adolescence, and 44.7% for their address at birth. In Canada, six-character postal codes in urban areas typically represent one side of a city block or a single multiunit building, while in rural areas they cover a much larger land area, sometimes even an entire town (36).
2.2 Assignment of residential surrounding greenness and other area-related exposures
Postal codes were linked to the environmental exposure datasets provided by the Canadian Urban Environmental Health Research Consortium (CANUE) (37). CANUE has assembled and maintains a data platform of environmental spatiotemporal exposure surfaces for use in health research. It has assigned these exposure surfaces to Canadian postal codes by using the geographical location represented by the centroid of each postal code. Individual-level residential addresses were linked to greenness exposures and other area-related measures for two time periods: (i) year at time of interview and (ii) 5 years prior to interview. These correspond roughly to the time of diagnosis, and 5 years before diagnosis for the cases. Unfortunately, we were unable to model exposures at earlier periods of the residential history owing to the large amount of missing data, coupled with relatively small overall sample sizes. For those participants who did not provide postal codes but, provided detailed residential addresses, we determined their postal codes by using the Canada Post postal code look up tool and Google Maps (38, 39). The land area for each of the postal codes was obtained from DMTI Spatial Inc. (40).
The NDVI and tree canopy coverage percentage were linked to participants’ postal codes to assign an estimate of an individual-level exposure to residential surrounding greenness. The mean annual NDVI and percentage of average tree canopy coverage estimates were obtained for 100, 250, 500, and 1,000-m buffers from the centroid of the participants’ postal codes.
The NDVI measures the intensity of green vegetation and is derived by using remote satellite sensors that detect land surface reflectance of visible and near-infrared range radiation. The NDVI captures the photosynthetic capacity of vegetation absorbing visible radiation while reflecting near-infrared radiation (41). It ranges from −1 to 1, where the lower range of values represents the absence of vegetation or presence of bodies of water. By contrast, values closer to 1 represent a greater amount of vegetation. Barren surfaces are usually characterized by NDVI values less than 0.2, grasslands by values from 0.2 to 0.4, and values greater than 0.4 indicate increasingly lush vegetation. In our analysis, any negative NDVIs were assigned a value of zero (this only occurred once for an NDVI exposure at the 100 m buffer). Unfortunately, we did not have a spatial surface for water and therefore were unable to isolate or control for the possible beneficial effects of blue spaces. The annual NDVI measures were generated based on scenes captured by satellite data between the first day and the last day of the year. The United States Geological Survey's Landsat 5 and 8 satellites captured scenes every 16 days at a 30-m resolution (42, 43). Accessed via Google Earth Engine, CANUE calculated mean annual NDVI from all cloud-free composites available within that year and assigned annual measurements to the DMTI Spatial Inc. postal codes (44, 45). The pixels within the satellite-captured scenes flagged as cloud covered or cloud shadowed are omitted from the calculations. The measurements of annual mean NDVI were available from 1984 to 2019, which allowed us to retrospectively assign NDVI values to the year at interview (2013–2015) and 5 years prior to interview (2008–2010).
Tree canopy coverage refers to areas covered by vegetation (i.e., tree leaves, branches, and stems) that cover the ground when viewed from above (46). The annual percentage of tree canopy coverage was calculated using data collected by satellites that estimated the percentage of pixels covered with vegetation over 5 m in height. Measurements of tree canopy coverage were derived from Landsat 5 Thematic Mapper and Landsat 7 Enhanced Thematic Mapper Plus based estimates of the Global Forest Cover Change Surface Reflectance product at a 30-m resolution (42, 47, 48). As tree canopy measures were only available for 2010 and 2015, we assigned the values from 2015 to the residence at the time of interview, while we used the 2010 values for the exposure 5 years before interview.
Residential-based measurements of area-level socioeconomic status and air pollution were also obtained through the CANUE datasets, and these values were similarly linked to participants’ postal codes. The Canadian Marginalization Index (CAN-Marg) is a census-based summary measure of deprivation and marginalization (49, 50). This area-level socioeconomic measure is derived at the census dissemination area level, which is the smallest possible geographical area that typically captures approximately 400–700 residents. We used the 2006 CAN-Marg Index, which incorporates four dimensions of marginalization: residential instability, material deprivation, ethnic concentration, and dependency. A summary measure was calculated by consolidating the quintile rank scores for these four dimensions into a summary measure (51).
Our analysis also considered the spatiotemporal surfaces of ambient NO2, which is a marker of traffic-related air pollution (52). The methodology used to create this surface has been previously described in detail by Hystad et al. (53). In brief, the surface was created by using a national surface land use regression (LUR) model to estimate annual ambient NO2 concentration in parts per billion (ppb) for individual postal code locations across Canada available from 1984 to 2016 (45, 53). This LUR model incorporated fixed site monitoring data, remote sensing data at a spatial resolution 10 × 10 km, geographic land use variables, and deterministic gradients (53). This resulted in a spatial surface of NO2 at a resolution of 10 m. Estimates of NO2 from this surface were previously linked to the centroids of all Canadian postal codes. This allowed for the annual average NO2 measurements to be assigned to the postal codes provided by the participants.
We chose to prioritize modeling NO2, rather than PM2.5, in our adjusted models to control for air pollution's potential confounding role in the association between greenness and breast cancer. NO2 has been shown to have substantially greater within-city variability than PM2.5 (54) and exhibits a stronger correlation with greenness that also varies within urban areas (55, 56). It is our view that this is the preferred approach to adjusting for possible confounding by air pollution. Our approach to control for NO2 is consistent with other studies that have evaluated the health benefits of greenness (57–60).
2.3 Other covariates
We considered the potential confounding influence for an extensive series of breast cancer risk factors that were collected in the EHQ. The selection of confounding factors was guided by our review of previous literature that investigated the association of greenness and breast cancer risk. In addition, we conducted bivariate analyses to identify those factors related to both case status and greenness. Following this, the variables included in our models were: participants’ age at interview (continuous variable), race and ethnicity, highest level of education achieved (high school or below, non-university certificate or trade school, Bachelor's degree or higher), body mass index [(BMI) categorized based on the WHO classification scheme (61) as underweight/normal weight (<25 kg/m2), overweight (25 to <30 kg/m2), and obese (≥30 kg/m2)], smoking status (never smoker, ever smoker), parity (parous, nulliparous), and family history of breast cancer (yes, no). Race and ethnicity were self-reported by the study participants. Data on race and ethnicity were collected as part of an OEHS questionnaire. We categorized race and ethnicity as either White or all other races and ethnicities combined (which included participants identifying as Aboriginal, Arab, Black, East Asian, Jewish, Latin American/Hispanic, South Asian, and other groups not listed) owing to the small number of participants that precluded a more detailed classification. For BMI and smoking variables, values are representative of participants’ height and weight measurements and smoking habits 2 years prior to the interview. CAN-Marg and NO2, previously described, were also considered as possible confounding variables.
2.4 Statistical analysis
We undertook analyses to describe the distribution of potential covariates and characterize the study participants. Specifically, for each variable we provided a summary of the distributional characteristics, and estimated the odds ratios in relation to case status. We also calculated the mean NDVI (at a 500-m buffer) across the levels of each classification variable. The distributional characteristics for area-related environmental exposures were described, along with the assessment of Pearson correlation coefficients between greenness (NDVI and tree coverage percentage), air pollution (NO2), and CAN-Marg exposures to describe the direction and strength of associations between these variables. Multivariable logistic regression was used to estimate odds ratios and their 95% confidence intervals (CIs) between greenness and incident breast cancer risk. In our primary analysis, we modeled the annual mean NDVI and tree coverage percentage based on a 500-m residential buffer at the time of survey completion. Our analysis was conducted at a 500-m buffer as this is a commonly used distance within greenness literature. This buffer distance covers areas within approximately a 15-min walk from a participant's residence. We categorized measures of greenness based on quartiles to evaluate the possibility of a non-linear association. We also modeled NDVI as a linear term and expressed the odds ratio in relation to an interquartile range increase.
Four models were fit to evaluate the effects of adjusting for different sets of potential confounding factors. The first model (M1) assessed associations with a minimal adjustment for age. The second model (M2) further included covariates for sociodemographic, behavioral, and reproductive risk factors (race and ethnicity, BMI, smoking, parity, and family history of breast cancer). Individual (highest education completed) and area-level (CAN-Marg summary quintiles) socioeconomic variables were introduced into Model 3 (M3). Finally, for the fully adjusted model (M4), the analysis was extended to incorporate the potential confounding effect of traffic-related air pollution (NO2).
A number of sensitivity analyses were undertaken. Specifically, we explored whether associations between greenness and breast cancer varied across different buffer distances (100, 250, 500, and 1,000 m). This was conducted for both annual mean NDVI and tree coverage percentage. In addition, we estimated odds ratios and their confidence intervals using measures of greenness defined 5 years before the time of interview, and for duration of time lived at current residence (stratified by less than 5 years and 5 years or more). All statistical analysis was performed using SAS software, version 9.4.
3 Results
Overall, 465 cases and 242 controls participated in this study. The study participants predominantly self-identified as White (80.5%), were 30 years of age or older (92.4%), and had a university education (55.7%) (Table 1). Participants’ residences were typically located within urban postal codes (85.9%). The land areas of the postal codes differed substantially based on whether the residence was in an urban or rural area. Specifically, the median land area of urban postal codes was 0.02 km2 (IQR = 0.01–0.03 km2), while for rural codes it was 109.87 km2 (IQR = 69.29–201.61 km2).
For the most part, the magnitude and direction of the age-adjusted odds ratios were consistent with established knowledge regarding risk factors associated with breast cancer in premenopausal women. As seen in Table 1, there are increased odds of breast cancer among smokers, alcohol drinkers, and those with a family history of breast cancer. Further, we observed reduced odds of breast cancer for parous individuals and those who were obese. However, decreased physical activity, an established risk factor for breast cancer (62), was not significantly associated with breast cancer risk. For most risk factors, the mean NDVI at a 500-m buffer did not vary substantially across factor levels. However, there were noticeable differences in the mean NDVI by race and ethnicity (White = 0.46; all other races and ethnicities = 0.38), and across levels of marginalization (least deprived = 0.48; most deprived = 0.39).
The distributional characteristics and Pearson's correlation coefficients of the residentially-based environmental exposures are presented in Table 2. As anticipated, the NDVI and tree coverage percentage were positively correlated with each other (r = 0.44), while inversely correlated with NO2 (r = −0.47 and r = −0.59, respectfully). Both greenness measures were inversely correlated with greater deprivation (r = −0.28 for the NDVI and r = −0.16 for tree canopy). We observed high correlations between greenness measured at multiple buffer distances (Supplementary Table S1). Environmental exposures based on measurements (i) at interview and (ii) 5 years prior to interview were similar; however, the mean measurements of tree coverage percentage and NO2 were marginally higher 5 years before the interview (Supplementary Table S2).
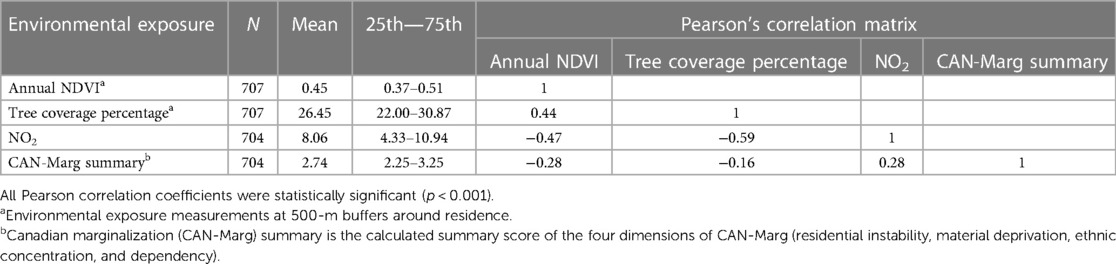
Table 2. Pearson's correlation matrix and distributional statistics of area-level exposures at participants’ residence during the time of the interview.
In the fully adjusted logistic model, an interquartile range increase (IQR = 0.14) in the annual mean NDVI within a 500-m buffer distance was not significantly associated with breast cancer risk (OR = 0.86; 95% CI = 0.65–1.13) during the time of the interview (Table 3). Similarly, a non-statistically significant association was observed for average annual tree coverage percentage at a 500-m buffer at the interview and breast cancer risk among those in the upper quartile relative to the lowest quartile (OR = 1.11; 95% CI = 0.59–2.07). Of note, in our models that were not adjusted for NO2, an inverse association between greenness and breast cancer was observed (Table 3). The addition of NO2 to these models strongly attenuated the association between greenness and breast cancer, producing a non-significant result.
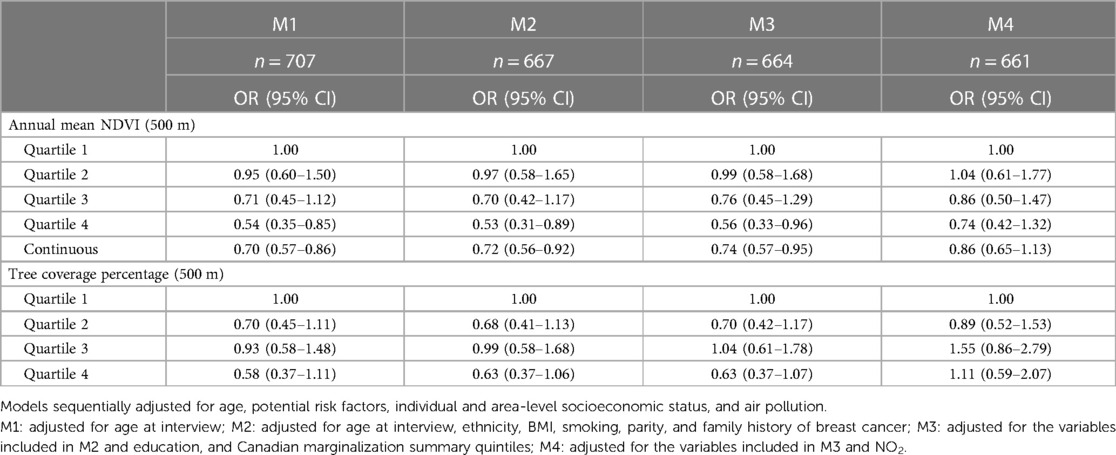
Table 3. Odds ratios and 95% CIs between breast cancer and annual mean NDVI and tree coverage percentage at 500 m buffer distance around residential address at the time of the interview.
No substantial differences were observed when comparing the odds ratios of breast cancer for the annual mean NDVI and tree coverage percentage at 100, 250, 500, and 1,000-m buffers (Supplementary Table S3). For an IQR increase in the annual mean NDVI, the odds ratios of breast cancer for participants living less than 5 years at current residence was slightly decreased in comparison with participants who had resided for 5 years or more (respectively, OR = 0.83; 95% CI = 0.52–1.34 and OR = 0.94; 95% CI = 0.66–1.35) (Supplementary Table S4). The surrounding NDVI at participants’ current and 5 years’ prior interview address were found to have similar estimates (respectively, OR = 0.86; 95% CI = 0.65–1.13 and OR = 0.95; 95% CI = 0.66–1.36) (Supplementary Table S5).
4 Discussion
Overall, we found that there was no statistically significant association between residential greenness and the risk of breast cancer in women 45 years of age and younger. The risk estimates for young women did not materially change across multiple buffer distances (100, 250, 500, and 1,000 m), and similar results were obtained for residential greenness measures at the time of interview compared with 5 years before. Interestingly, we found that adjusting for traffic-related air pollution (NO2) strongly attenuated the initial inverse association observed with greenness.
A cohort study based in Catalonia, Terre-Torras et al., was the only other study we identified that characterized estimates for associations between residential greenness and breast cancer in young women (29). Within this cohort, premenopausal breast cancer cases had a median age of 38 (IQR = 34–41) years, resembling our population's age structure. They reported that an IQR increase of NDVI (HR = 0.989; 95% CI = 0.966–1.012) and greenspace percentage (HR = 0.991; 95% CI = 0.963–1.020) were not related to the risk of premenopausal breast cancer. This finding is consistent with our own.
Our study had the advantage of using two greenness metrics, namely, the NDVI and tree coverage percentage. While NDVI has its strengths as a quantifiable and standardized metric for greenness exposure, it also has limitations. Even though NDVI facilitates comparisons between studies conducted in different locations and time periods, it only captures overall greenness and does not provide insights on specific types of greenness or the usability of the greenspace. We lacked data for blue spaces, and thus were unable to differentiate low values that could be the result of water, or impervious surfaces. The moderate Pearson's correlation we found between NDVI and tree coverage (r = 0.44) suggests that these two metrics capture overlapping features of greenness, yet they also have some distinct features.
There have been few previous attempts to evaluate specific features or types of greenness in relation to breast cancer risk. An exception is the work by O'Callaghan-Gordo et al., which found a reduced odds ratio for the presence of an “urban green area” within a 300-m residential buffer (OR = 0.65, 95% CI = 0.49–0.86), while the presence of agricultural land (OR = 1.33, 95% CI = 1.07–1.65) and an IQR increase in surrounding NDVI (32). Conversely, Zare Sakhvidi et al., a cohort study from France, found no associations between residential proximity to urban greenspaces, forests, or agricultural lands and breast cancer risk (28). While there has been some consideration of different types of green spaces, epidemiological studies that capture the amount of time participants spend in these areas.
The proposed mechanisms explaining the hypothesis that greenness reduces the risk of breast cancer have mainly described ways that physical activity and air pollution drive the relationship with greenness to improve an individual's wellbeing and health (21). Our analyses of data from the OEHS indicated that physical activity was not related to the risk of breast cancer, and therefore, does not fit the criteria of either a mediator or confounder of the greenness and breast cancer relationship. O'Callaghan-Gordo et al. similarly found physical activity did not substantially change their risk estimates when considered as a confounder (31). Markevych et al. have described that overall greenness may not necessarily equate to promoting physical activity, but could rely more on the perception of its safety, accessibility, and type of greenness (21).
The addition of air pollution strongly attenuates the relationship between greenness and breast cancer, as the odds ratio for an IQR increase in NDVI at a 500 m buffer changed from 0.74 (95% CI = 0.57–0.95) to 0.86 (95% CI = 0.65–1.13). In urban areas, NO2 is inversely correlated with greenness, which largely results from the fact that urban environments with green spaces are void of roadways. Greenness also contributes to lower levels of air pollution as vegetation has been shown to reduce concentrations of ambient pollution (63). Terre-Torras et al., the only other study specifying risk estimates among younger women, also adjusted for NO2, but their associations between overall greenness and breast cancer prior to adjustment were already null (29). A similar role of NO2 was observed in another Canadian study of greenness and incident breast cancer (64). The findings from our study highlight the importance of epidemiological studies to control for the confounding influence of traffic-related air pollution.
There are several important study limitations that should be acknowledged. This includes a small sample size which limits the ability to detect an association. In addition, the small sample sizes limited our approach to adjust for the confounding influence of other variables, and we acknowledge there may be residual confounding. Even though our study is population-based, our associations may not be readily generalized to all women in Ontario. The OEHS excluded participants from Northern Ontario who did not live close to a biological collection laboratory (usually, at least a 3 h drive). Although we were able to examine the relationship with respect to the density of forests inferred by tree coverage percentage, further investigations may be warranted to clarify whether other aspects of greenness around residences may impact breast cancer risk among young women.
We recognize that modeling exposure to residential greenness during the time of the interview or 5 years prior may not capture the most etiologically relevant exposure period for breast cancer. The latency time to a solid tumor like breast cancer may be over a decade from the time of first exposure to a harmful environmental stimuli, or during periods of increased vulnerability (e.g., puberty and pregnancy) (65). We were limited in our ability to assess earlier exposure periods because of a large number of residential data missing. Specifically, only 74.3% of the participants had information on their residence 5 years before the interview, while 43.7% of the participants had residential information for 10 years before the interview.
Lastly, although a population-based methodology was used to obtain cases and controls, OEHS encountered challenges acquiring the desired number of controls due to low response rates. This possibly reflects, in part, a lack of willingness or logistical challenges in providing biological specimens. The original aim of the study was to characterize the risk of breast cancer in relation to exposures to PBDEs, which were determined using blood samples. The lower response rate among the controls compared with the cases raises the possibility of selection bias. However, when we evaluated recognized breast cancer risk factors for young women (e.g., BMI, parity, smoking), overall, we observed that they demonstrated expected directional effects that were similar to those previously published (66). Moreover, the reported odds ratios for greenness and breast cancer risk did not substantially change with the addition of socioeconomic variables, which is typically correlated with participation rates. This suggests that our study findings are not unduly influenced by participation bias.
The study possesses several strengths. As studies focusing on breast cancer in younger women remain scarce, our findings offer valuable evidence with respect to this subpopulation. In addition, breast cancer cases were identified through a provincial cancer registry, and therefore our study is population based. Moreover, greenness exposure estimates were derived using the NDVI and linked to participants residential addresses and are thus objective measures of exposure that are not susceptible to recall bias. Another notable advantage of the study is the extensive collection of information on environmental and other relevant risk factors. The OEHS's focus on research of environmental exposures affecting breast cancer allowed for a comprehensive examination of potential confounders within this study. Furthermore, the study was able to investigate whether a specific type of greenness such as tree coverage had a different association with breast cancer risk compared with the overall surrounding greenness. Access to partial residential histories allowed us to conduct sensitivity analyses, which did not reveal substantial change of observed associations after evaluating a lag time of 5 years prior to interview for participants’ duration at current address and environmental exposure levels.
5 Conclusion
The lack of a statistically significant association between greenness and breast cancer incidence determined by our study suggests that urban greenness does not confer a reduced risk of breast cancer in premenopausal women. Future studies that investigate greenness and breast cancer should account for air pollution's role within the studied relationship, and efforts should be made to better account for the time participants spend in green spaces. While our findings do not support the hypothesis that greenness reduces the risk of breast cancer, we recognize that urban greenness does contribute to a number of health benefits.
Data availability statement
The data analyzed in this study are subject to the following licenses/restrictions: the dataset is not available for public release and was only available for the researchers participating in this study. This is in accordance with our ethics, and the informed consent by participants. Requests to access these datasets should be directed to the corresponding author.
Ethics statement
The studies involving humans were approved by Carleton University Research Ethics Board-B (CUREB-B). CUREB-B is constituted and operates in compliance with the Tri-Council Policy Statement: Ethical Conduct for Research Involving Humans (TCPS2). The study was conducted in accordance with the local legislation and institutional requirements. The participants provided their written informed consent to participate in this study.
Author contributions
CW: Data curation, Formal Analysis, Investigation, Methodology, Writing – original draft, Writing – review & editing. PV: Conceptualization, Funding acquisition, Methodology, Supervision, Writing – review & editing. BP: Investigation, Writing – review & editing. MÉ-P: Conceptualization, Methodology, Writing – review & editing. JB: Funding acquisition, Writing – review & editing, Data curation. EL: Funding acquisition, Writing – review & editing. SH: Funding acquisition, Writing – review & editing.
Funding
The authors declare financial support was received for the research, authorship, and/or publication of this article.
The Canadian Institute of Health Research provided funding for this study (Funding reference #: DA6 184674). The Ontario Health and Environment Study was supported by the Canadian Cancer Society (Funding reference #: 700865, RG-11).
Acknowledgments
The case–control data were provided by the Ontario Health Environmental Study (OEHS). The following built environmental exposures were provided by the Canadian Urban Environmental Health Research Consortium (CANUE): (i) NDVI metrics, indexed to DMTI Spatial Inc. postal codes; (ii) Tree canopy metrics, indexed to DMTI Spatial Inc. postal codes; (iii) Canadian Marginalization Index (CAN-Marg), indexed to DMTI Spatial Inc. postal codes; and (iv) Nitrogen dioxide data, indexed to DMTI Spatial Inc. postal codes. We would like to further acknowledge that the intellectual property rights to the tree canopy metrics dataset obtained from CANUE belong to the University of Maryland, Department of Geographical Sciences, and NASA.
Conflict of interest
The authors declare that the research was conducted in the absence of any commercial or financial relationships that could be construed as a potential conflict of interest.
Publisher's note
All claims expressed in this article are solely those of the authors and do not necessarily represent those of their affiliated organizations, or those of the publisher, the editors and the reviewers. Any product that may be evaluated in this article, or claim that may be made by its manufacturer, is not guaranteed or endorsed by the publisher.
Supplementary material
The Supplementary Material for this article can be found online at: https://www.frontiersin.org/articles/10.3389/fenvh.2023.1274563/full#supplementary-material
References
1. Bray F, Ferlay J, Soerjomataram I, Siegel RL, Torre LA, Jemal A. Global cancer statistics 2018: GLOBOCAN estimates of incidence and mortality worldwide for 36 cancers in 185 countries. Cancer J Clin. (2018) 68(6):394–424. doi: 10.3322/caac.21492
2. Brenner DR, Poirier A, Woods RR, Ellison LF, Billette JM, Demers AA, et al. Projected estimates of cancer in Canada in 2022. Can Med Assoc J. (2022) 194(17):E601–7. doi: 10.1503/cmaj.212097
3. Canadian Cancer Advisory Committee in collaboration with the Canadian Cancer Society, Statistics Canada, and the Public Health Agency of Canada. Canadian Cancer Statistics 2021. Toronto, ON: Canadian Cancer Society (2021).
4. Heer E, Ruan Y, Mealey N, Quan ML, Brenner DR. The incidence of breast cancer in Canada 1971–2015: trends in screening-eligible and young-onset age groups. Can J Public Health. (2020) 111:787–93. doi: 10.17269/s41997-020-00305-6
5. Klarenbach S, Sims-Jones N, Lewin G, Singh H, Thériault G, Tonelli M, et al. Recommendations on screening for breast cancer in women aged 40–74 years who are not at increased risk for breast cancer. Can Med Assoc J. (2018) 190(49): E1441–51. doi: 10.1503/cmaj.180463
6. Dufour O, Houvenaeghel G, Classe JM, Cohen M, Faure C, Mazouni C, et al. Early breast cancer in women aged 35 years or younger: a large national multicenter French population-based case control-matched analysis. Breast. (2023) 68:163–72. doi: 10.1016/j.breast.2023.02.004
7. Gnerlich JL, Deshpande AD, Jeffe DB, Sweet A, White N, Margenthaler JA. Elevated breast cancer mortality in women younger than age 40 years compared with older women is attributed to poorer survival in early-stage disease. J Am Coll Surg. (2009) 208:341–7. doi: 10.1016/j.jamcollsurg.2008.12.001
8. Pharoah PDP, Day NE, Duffy S, Easton DF, Ponder BAJ. Family history and the risk of breast cancer: a systematic review and meta-analysis. Int J Cancer. (1998) 71(5):800–9. doi: 10.1002/(sici)1097-0215(19970529)71:5%3C800::aid-ijc18%3E3.0.co;2-b
9. Gomez-Flores-Ramos L, Alvarez-Gomez RM, Villarreal-Garza C, Wegman-Ostrosky T, Mohar A. Breast cancer genetics in young women: what do we know? Mutat Res Rev Mutat Res. (2017) 774:33–45. doi: 10.1016/j.mrrev.2017.08.001
10. Chollet-Hinton L, Anders CK, Tse CK, Bell MB, Yang YC, Carey LA, et al. Breast cancer biologic and etiologic heterogeneity by young age and menopausal status in the Carolina breast cancer study: a case-control study. Breast Cancer Res. (2016) 18:79. doi: 10.1186/s13058-016-0736-y
11. García-Estévez L, Cortés J, Pérez S, Calvo I, Gallegos I, Moreno-Bueno G. Obesity and breast cancer: a paradoxical and controversial relationship influenced by menopausal status. Front Oncol. (2021) 11:705911. doi: 10.3389/fonc.2021.705911
12. Łukasiewicz S, Czeczelewski M, Forma A, Baj J, Sitarz R, Stanisławek A. Breast cancer—epidemiology, risk factors, classification, prognostic markers, and current treatment strategies—an updated review. Cancers (Basel). (2021) 13(17):4287. doi: 10.3390/cancers13174287
13. Hiatt RA, Brody JG. Environmental determinants of breast cancer. Annu Rev Public Health. (2018) 39:113–33. doi: 10.1146/annurev-publhealth-040617-014101
14. Engel LS, Werder E, Satagopan J, Blair A, Hoppin JA, Koutros S, et al. Insecticide use and breast cancer risk among farmers’ wives in the agricultural health study. Environ Health Perspect. (2017) 125(9):097002. doi: 10.1289/EHP1295
15. Gabet S, Lemarchand C, Guénel P, Slama R. Breast cancer risk in association with atmospheric pollution exposure: a meta-analysis of effect estimates followed by a health impact assessment. Environ Health Perspect. (2021) 129(5):057012. doi: 10.1289/EHP8419
16. Lee DG, Burstyn I, Lai AS, Grundy A, Friesen MC, Aronson KJ, et al. Women’s occupational exposure to polycyclic aromatic hydrocarbons and risk of breast cancer. Occup Environ Med. (2019) 76(1):22–9. doi: 10.1136/oemed-2018-105261
17. Urbano T, Vinceti M, Wise LA, Filippini T. Light at night and risk of breast cancer: a systematic review and dose–response meta-analysis. Int J Health Geogr. (2021) 20(1):1–26. doi: 10.1186/s12942-021-00297-7
18. Frank LD, Iroz-Elardo N, MacLeod KE, Hong A. Pathways from built environment to health: a conceptual framework linking behavior and exposure-based impacts. J Transp Health. (2019) 12:319–35. doi: 10.1016/j.jth.2018.11.008
19. Renalds A, Smith TH, Hale PJ. A systematic review of built environment and health. Fam Community Health. (2010) 33(1):68–78. doi: 10.1097/FCH.0b013e3181c4e2e5
20. James P, Banay RF, Hart JE, Laden F. A review of the health benefits of greenness. Curr Epidemiol Rep. (2015) 2:131–42. doi: 10.1007/s40471-015-0043-7
21. Markevych I, Schoierer J, Hartig T, Chudnovsky A, Hystad P, Dzhambov AM, et al. Exploring pathways linking greenspace to health: theoretical and methodological guidance. Environ Res. (2017) 158:301–17. doi: 10.1016/j.envres.2017.06.028
22. Gascon M, Triguero-Mas M, Martínez D, Dadvand P, Rojas-Rueda D, Plasència A, et al. Residential green spaces and mortality: a systematic review. Environ Int. (2016) 86:60–7. doi: 10.1016/j.envint.2015.10.013
23. Rojas-Rueda D, Nieuwenhuijsen MJ, Gascon M, Perez-Leon D, Mudu P. Green spaces and mortality: a systematic review and meta-analysis of cohort studies. Lancet Planet Health. (2019) 3(11):e469–77. doi: 10.1016/S2542-5196(19)30215-3
24. Yang BY, Zhao T, Hu LX, Browning MHEM, Heinrich J, Dharmage SC, et al. Greenspace and human health: an umbrella review. Innovation. (2021) 2(4):100164. doi: 10.1016/j.xinn.2021.100164
25. Zare Sakhvidi MJ, Yang J, Mehrparvar AH, Dzhambov AM, Ebrahimi AA, Dadvand P, et al. Exposure to greenspace and cancer incidence, prevalence, and mortality: a systematic review and meta-analyses. Sci Total Environ. (2022) 838:156180. doi: 10.1016/j.scitotenv.2022.156180
26. Huang YJ, Lee PH, Chen LC, Lin BC, Lin C, Chan TC. Relationships among green space, ambient fine particulate matter, and cancer incidence in Taiwan: a 16-year retrospective cohort study. Environ Res. (2022) 212:113416. doi: 10.1016/j.envres.2022.113416
27. Kayyal-Tarabeia I, Michael Y, Lensky IM, Blank M, Agay-Shay K. Residential greenness and site-specific cancer: a registry based cohort of 144,427 participants with a 21-years of follow-up, Tel-Aviv district, Israel. Environ Res. (2022) 212:113460. doi: 10.1016/j.envres.2022.113460
28. Zare Sakhvidi MJ, Yang J, Siemiatycki J, Dadvand P, de Hoogh K, Vienneau D, et al. Greenspace exposure and cancer incidence: a 27-year follow-up of the French GAZEL cohort. Sci Total Environ. (2021) 787:147553. doi: 10.1016/j.scitotenv.2021.147553
29. Terre-Torras I, Recalde M, Díaz Y, de Bont J, Bennett M, Aragón M, et al. Air pollution and green spaces in relation to breast cancer risk among pre and postmenopausal women: a mega cohort from Catalonia. Environ Res. (2022) 214:113838. doi: 10.1016/j.envres.2022.113838
30. Datzmann T, Markevych I, Trautmann F, Heinrich J, Schmitt J, Tesch F. Outdoor air pollution, green space, and cancer incidence in Saxony: a semi-individual cohort study. BMC Public Health. (2018) 18(1):1–10. doi: 10.1186/s12889-018-5615-2
31. Wang Q, Goldberg MS, Labrèche F, Ho V. The association between the incidence of post-menopausal breast cancer and residential greenness. Cancer Epidemiol. (2022) 76:102094. doi: 10.1016/j.canep.2021.102094
32. O’Callaghan-Gordo C, Kogevinas M, Cirach M, Castaño-Vinyals G, Aragonés N, Delfrade J, et al. Residential proximity to green spaces and breast cancer risk: the multicase-control study in Spain (MCC-Spain). Int J Hyg Environ Health. (2018) 221(8):1097–106. doi: 10.1016/j.ijheh.2018.07.014
33. Siddique S, Harris SA, Kosarac I, Latifovic L, Kubwabo C. Urinary metabolites of organophosphate esters in women and their relationship with serum lipids: an exploratory analysis. Environ Pollut. (2020) 263:114110. doi: 10.1016/j.envpol.2020.114110
34. Harris SA, Boucher B, Thompson C, Kuwabo C, Cotterchio M, Knight J, et al. The development of a comprehensive exposure assessment strategy for polybrominated diphenyl ethers (PBDEs) for use in observational epidemiology studies. ISEE Conf Abstr. (2013). doi: 10.1289/isee.2013.P-1-22-27
35. Cancer Care Ontario. How we collect cancer registry data. Available online at: https://www.cancercareontario.ca/en/data-research/accessing-data/technical-information/cancer-registry-data-collection (accessed April 27, 2018).
36. Canada Post. Addressing guidelines (2022). Available online at: https://www.canadapost-postescanada.ca/cpc/en/support/articles/addressing-guidelines/postal-codes.page (accessed May 11, 2023).
37. CANUE. The Canadian Urban Environmental Health Research Consortium. Toronto (2023). Available online at: https://canue.ca/ (accessed April 10, 2023).
38. Google. Google Maps. Available online at: https://www.google.com/maps (accessed April 11, 2023).
39. Canada Post Corporation. Find a postal code. Available online at: https://www.canadapost-postescanada.ca/cpc/en/tools/find-a-postal-code.page (accessed April 11. 2023).
41. Robinson NP, Allred BW, Jones MO, Moreno A, Kimball JS, Naugle DE, et al. A dynamic Landsat derived normalized difference vegetation index (NDVI) product for the conterminous United States. Remote Sens. (2017) 9(863):863. doi: 10.3390/rs9080863
42. USGS Landsat 5 TM TOA reflectance (Orthorectified), 1984 to 2011. Available online at: https://explorer.earthengine.google.com/detail/LANDSAT/LC8_L1T_TOA (accessed July 2017).
43. USGS Landsat 8 TOA reflectance (Orthorectified), 2013 to 2017. Available online at: https://explorer.earthengine.google.com/detail/LANDSAT/LC8_L1T_TOA (accessed July 2017).
44. Gorelick N, Hancher M, Dixon M, Ilyushchenko S, Thau D, Moore R. Google earth engine: planetary-scale geospatial analysis for everyone. Remote Sens Environ. (2017) 202:18–27. doi: 10.1016/j.rse.2017.06.031
46. National Capital Commission. Tree canopy assessment Canada’s capital region. (2019). Available online at: https://ncc-ccn.gc.ca/our-plans/tree-canopy-assessment-canada-capital-region#:∼:text=Tree%20canopy%20is%20defined%20as,ground%20when%20viewed%20from%20above (accessed May 11, 2023).
47. Sexton JO, Song XP, Feng M, Noojipady P, Anand A, Huang C, et al. Global, 30-m resolution continuous fields of tree cover: Landsat-based rescaling of MODIS vegetation continuous fields with LIDAR-based estimates of error. Int J Digit Earth. (2013) 6(5):427–48. doi: 10.1080/17538947.2013.786146
48. CanMap postal code suite v2010 and v2015 [computer file] Markham: DMTI Spatial Inc. (2010 and 2015).
49. Matheson FI, Dunn JR, Smith KLW, Moineddin R, Glazier RH. Development of the Canadian marginalization Index: a new tool for the study of inequality. Can J Public Health. (2012) 103(S2):S12–6. doi: 10.1007/bf03403823
51. Matheson FI, Dunn JR, Smith KLW, Moineddin R, Glazier RH. CAN-Marg: Canadian marginalization index user guide version 1.0. (2012). Available online at: https://canue.ca/wp-content/uploads/2018/07/CANMARG_user_Guide_2012.pdf (accessed April 20, 2023).
52. Levy JI, Greco SL, Melly SJ, Mukhi N. Evaluating efficiency-equality tradeoffs for mobile source control strategies in an urban area. Risk Anal. (2009) 29(1):34–47. doi: 10.1111/j.1539-6924.2008.01119.x
53. Hystad P, Setton E, Cervantes A, Poplawski K, Deschenes S, Brauer M, et al. Creating national air pollution models for population exposure assessment in Canada. Environ Health Perspect. (2011) 119(8):1123–9. doi: 10.1289/ehp.1002976
54. Wang Y, Bechle MJ, Kim SY, Adams PJ, Pandis SN, Pope CA, et al. Spatial decomposition analysis of NO2 and PM2.5 air pollution in the United States. Atmos Environ. (2020) 241:117470. doi: 10.1016/j.atmosenv.2020.117470
55. Villeneuve PJ, Jerrett M, Su JG, Burnett RT, Chen H, Wheeler AJ, et al. A cohort study relating urban green space with mortality in Ontario, Canada. Environ Res. (2012) 115:51–8. doi: 10.1016/j.envres.2012.03.003
56. Crouse DL, Pinault L, Balram A, Brauer M, Burnett RT, Martin RV, et al. Complex relationships between greenness, air pollution, and mortality in a population-based Canadian cohort. Environ Int. (2019) 128: 292–300. doi: 10.1016/j.envint.2019.04.047
57. Fong KC, Hart JE, James P. A review of epidemiologic studies on greenness and health: updated literature through 2017. Curr Environ Health Rep. (2018) 5(1):77–87. doi: 10.1007/s40572-018-0179-y
58. Villeneuve PJ, Lam S, Tjepkema M, Pinault L, Crouse DL, Osornio-Vargas AR, et al. Residential proximity to greenness and adverse birth outcomes in urban areas: findings from a national Canadian population-based study. Environ Res (2022) 204(Pt C):112344. doi: 10.1016/j.envres.2021.112344
59. Klompmaker JO, Janssen NAH, Bloemsma LD, Marra M, Lebret E, Gehring U, et al. Effects of exposure to surrounding green, air pollution and traffic noise with non-accidental and cause-specific mortality in the Dutch national cohort. Environ Health. (2021) 20(1):82. doi: 10.1186/s12940-021-00769-0
60. Bloemsma LD, Wijga AH, Klompmaker JO, Janssen NAH, Smit HA, Koppelman GH, et al. The associations of air pollution, traffic noise and green space with overweight throughout childhood: the PIAMA birth cohort study. Environ Res. (2019) 169:348–56. doi: 10.1016/j.envres.2018.11.026
61. World Health Organization. A healthy lifestyle—WHO recommendations. Available online at: https://www.who.int/europe/news-room/fact-sheets/item/a-healthy-lifestyle—who-recommendations (accessed April 17, 2023).
62. Chen X, Wang Q, Zhang Y, Xie Q, Tan X. Physical activity and risk of breast cancer: a meta-analysis of 38 cohort studies in 45 study reports. Value Health. (2019) 22(1):104–28. doi: 10.1016/j.jval.2018.06.020
63. Diener A, Mudu P. How can vegetation protect US from air pollution? A critical review on green spaces’ mitigation abilities for air-borne particles from a public health perspective—with implications for urban planning. Sci Total Environ. (2021) 796:148605. doi: 10.1016/j.scitotenv.2021.14860
64. Villeneuve P, Mansouri R, Crouse D, To T, Wall C, Miller A. Greenness, obesity and incident breast cancer: evidence from the Canadian national breast screening study. Environ Epidemiol. (2019) 3:416. doi: 10.1097/01.EE9.0000610660.55734.0d
65. Terry MB, Michels KB, Brody JG, Byrne C, Chen S, Jerry J, et al. Environmental exposures during windows of susceptibility for breast cancer: a framework for prevention research. Breast Cancer Res. (2019) 21:96. doi: 10.1186/s13058-019-1168-2
Keywords: residential greenness, urban built environments, breast cancer, premenopausal, case–control study
Citation: Waddingham CM, Villeneuve PJ, Le Provost B, Parent M-É, Brook JR, Lavigne E and Harris SA (2024) Residential surrounding greenness is not associated with incident breast cancer in young women in Ontario, Canada. Front. Environ. Health 2:1274563. doi: 10.3389/fenvh.2023.1274563
Received: 8 August 2023; Accepted: 13 December 2023;
Published: 5 January 2024.
Edited by:
Brent Finley, Stantec, CanadaReviewed by:
Andrea Kaifie-Pechmann, University Hospital RWTH Aachen, GermanyPayel Kundu, Stantec, United States
Dylan Steigerwald, Stantec, Canada
Rachel Freid, Stantec, United States
© 2024 Waddingham, Villeneuve, Le Provost, Parent, Brook, Lavigne and Harris. This is an open-access article distributed under the terms of the Creative Commons Attribution License (CC BY). The use, distribution or reproduction in other forums is permitted, provided the original author(s) and the copyright owner(s) are credited and that the original publication in this journal is cited, in accordance with accepted academic practice. No use, distribution or reproduction is permitted which does not comply with these terms.
*Correspondence: Paul J. Villeneuve cGF1bC52aWxsZW5ldXZlQGNhcmxldG9uLmNh