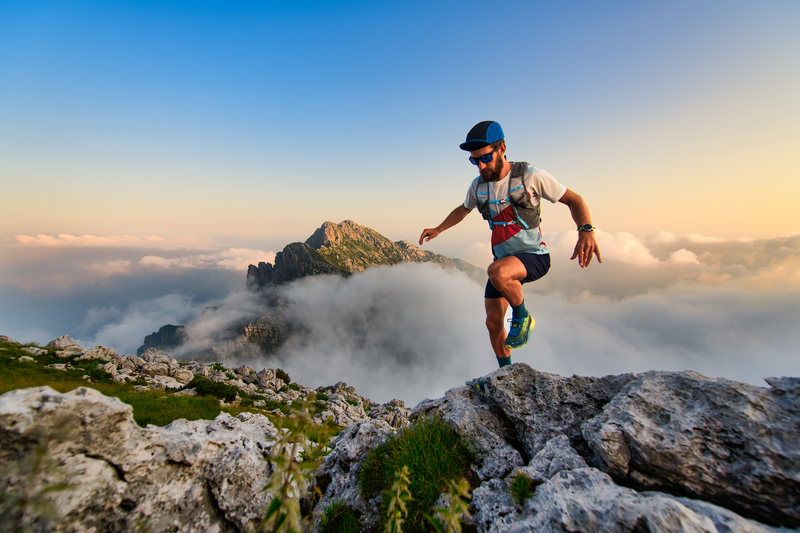
95% of researchers rate our articles as excellent or good
Learn more about the work of our research integrity team to safeguard the quality of each article we publish.
Find out more
EDITORIAL article
Front. Energy Res. , 09 December 2024
Sec. Wind Energy
Volume 12 - 2024 | https://doi.org/10.3389/fenrg.2024.1531689
This article is part of the Research Topic Online Monitoring of Wind Power Plants using Digital Twin Models View all 10 articles
Editorial on the Research Topic
Online monitoring of wind power plants using digital twin models
In the fast-evolving renewable energy sector, offshore wind technology has made significant strides, evolving from bottom-fixed turbines to the integration of floating turbines. These developments have led to a critical focus on reducing Operational Expenditure (OPEX) and improving turbine reliability through predictive maintenance that uses real-time data to forecast and mitigate potential failures. Digital Twin models have acted as an enabling technology for performing predictive maintenance. This research topic brings together a set of nine studies dedicated to addressing the technical challenges and practical applications of digital twin models in wind energy, aiming to lay the groundwork for more sustainable, reliable, and cost-effective wind energy systems.
The featured articles explore various aspects of digital twin technology, from architectural development to fault detection, all with the goal of optimizing wind turbine maintenance. For instance, Marykovskiy et al. in Architecting a Digital Twin for Wind Turbine Rotor Blade Aerodynamic Monitoring, presents a novel digital twin system, Aerosense, designed for rotor blade monitoring using microelectromechanical sensors. Built on systems engineering principles, Aerosense uses microelectromechanical sensors to provide comprehensive monitoring and predictive modeling, moving the industry toward more adaptable and integrated digital twin frameworks.
Another significant contribution, Fault Detection of a Wind Turbine Generator Bearing Using Interpretable Machine Learning by Bindingsbø et al. addresses the challenge of detecting faults in generator bearings—a common issue that can compromise turbine performance. This study presents a machine learning-based approach for identifying temperature anomalies indicative of bearing faults, using Supervisory Control and Data Acquisition (SCADA) data. The emphasis on interpretable machine learning underscores the need for transparency and accountability in predictive maintenance, essential for broader adoption of digital twin models in wind energy.
This research topic also delves into powertrain degradation and control. In Yaw Misalignment in Powertrain Degradation Modelling for Wind Farm Control in Curtailed Conditions, Moghadam et al. examine how yaw misalignment affects powertrain components and propose a damage-aware control framework for efficient power distribution within wind farms. This study highlights the integration of degradation metrics into farm-level control, demonstrating how digital twin models can simultaneously address efficiency and durability through advanced control strategies, and allow operators to make informed decisions about the power setpoints of individual turbines within large farms.
Another key study, Optimal Operation Strategy for Distribution Network with High Penetration of Dispersed Wind Power by Duan et al. addresses the integration of distributed wind power into local grids. This research presents an optimization model to enhance network performance while balancing high wind penetration and voltage stability. It highlights the potential of digital twins to manage complex, large-scale networks, providing essential decision-making support.
Control strategies for grid integration and stability are also central to An MPC Based Active and Reactive Power Coordinated Control Strategy of PMSG Wind Turbines to Enhance the Support Capability by Ma et al. The study advances model predictive control methods for regulating active and reactive power, underscoring the role of digital twins in managing grid support functions traditionally handled by conventional units. This contributes to a broader vision of the utilization of digital twin models in future grid where renewable sources play a more active role in maintaining grid stability.
Available Power Estimation of Wind Farms Based on Deep Spatio-temporal Neural Networks by Liu et al. illustrates the potential of artificial intelligence in digital twin applications. This study leverages deep learning techniques to forecast available power based on historical data, demonstrating how spatio-temporal neural networks can enhance prediction accuracy. The focus on turbine-level data highlights the potential of digital twins for fine-grained, turbine-specific insights, allowing operators to make informed decisions about individual turbines within large farms.
In addition to simulations, the research topic also includes an experimental study performed by Xiong et al. in Experimental study of Dynamic Characteristics of an Ultra-Large Jacket Offshore Wind Turbine under Wind and Wave Loads Using Aero-Hydro-Structural Elastic Similarities, which provides a physical testing framework for offshore turbine stability under extreme conditions. This study underscores the importance of empirical validation in digital twin models, ensuring their reliability across diverse operational conditions.
Power management and fault resilience are addressed by contributions such as A Model Predictive Control Strategy for Enhancing Fault Ride Through in PMSG Wind Turbines Using SMES and Improved GSC Control by Abdelkader et al. which introduces a novel strategy that combines superconducting magnetic energy storage (SMES) with model predictive control (MPC). This strategy boosts fault resilience by providing reactive power support during grid disruptions, showcasing how digital twins can predict and preempt faults, minimizing downtime.
Finally, Study on Obtaining Real Power Curve of Wind Turbines by Using SCADA Data by Dai et al. explores methods for deriving accurate power curves from SCADA data, an essential function of digital twins in assessing wind turbine performance. This study contributes to more precise performance assessments, directly supporting predictive maintenance and operational planning.
Together, these studies represent a comprehensive approach to tackling the challenges of digital twin modeling for predictive maintenance in wind energy. They collectively address the three core layers of digital twin realization—data acquisition, dynamic model processing, and decision support—each layer contributing to a robust framework for optimizing turbine performance. As digital twin technology continues to mature, these contributions highlight the technical challenges and the immense potential of predictive maintenance, steering the wind energy industry towards a more sustainable and efficient future.
FK: Writing–original draft, Writing–review and editing. AK: Writing–review and editing. ZG: Writing–review and editing.
The author(s) declare that no financial support was received for the research, authorship, and/or publication of this article.
The authors declare that the research was conducted in the absence of any commercial or financial relationships that could be construed as a potential conflict of interest.
The authors declare that no Generative AI was used in the creation of this manuscript.
All claims expressed in this article are solely those of the authors and do not necessarily represent those of their affiliated organizations, or those of the publisher, the editors and the reviewers. Any product that may be evaluated in this article, or claim that may be made by its manufacturer, is not guaranteed or endorsed by the publisher.
Keywords: digital twin models, predictive maintenance, offshore wind energy, fault detection, machine learning applications, renewable energy systems, wind turbine optimization, grid integration
Citation: Khazaeli Moghadam F, Keprate A and Gao Z (2024) Editorial: Online monitoring of wind power plants using digital twin models. Front. Energy Res. 12:1531689. doi: 10.3389/fenrg.2024.1531689
Received: 20 November 2024; Accepted: 25 November 2024;
Published: 09 December 2024.
Edited and reviewed by:
David Howe Wood, University of Calgary, CanadaCopyright © 2024 Khazaeli Moghadam, Keprate and Gao. This is an open-access article distributed under the terms of the Creative Commons Attribution License (CC BY). The use, distribution or reproduction in other forums is permitted, provided the original author(s) and the copyright owner(s) are credited and that the original publication in this journal is cited, in accordance with accepted academic practice. No use, distribution or reproduction is permitted which does not comply with these terms.
*Correspondence: Farid Khazaeli Moghadam, ZmFyaWRraGF6YWVsaUBnbWFpbC5jb20=
Disclaimer: All claims expressed in this article are solely those of the authors and do not necessarily represent those of their affiliated organizations, or those of the publisher, the editors and the reviewers. Any product that may be evaluated in this article or claim that may be made by its manufacturer is not guaranteed or endorsed by the publisher.
Research integrity at Frontiers
Learn more about the work of our research integrity team to safeguard the quality of each article we publish.