- 1Electric Power Research Institute, China Southern Power Grid, Guangzhou, China
- 2Tsinghua Berkeley Shenzhen Institute, Shenzhen International Graduate School, Tsinghua University, Shenzhen, China
- 3Shenzhen Power Supply Bureau, China Southern Power Grid, Shenzhen, China
Massive and diversified distributed energy resources (DERs) have great potential for improving the flexibility of power system operations. However, given the wide distribution, small capacity, and variable ownership subject characteristics of DERs, virtual power plants (VPPs) are suitable for aggregating the flexible DERs for participation in the wholesale energy market. Extant studies have focused on obtaining bidding strategies in multiple electricity markets. However, there are shortcomings in the relevant market mechanisms and temporal relationships for participating in energy and ancillary service markets. This work proposes a joint market temporal rolling bidding strategy for VPPs in the wholesale market based on real unified electricity market mechanisms in the southern region of China. Accordingly, the proposed method enhances the market-oriented operational profits of VPPs.
1 Introduction
Distributed energy resources (DERs) have gained wide importance for their huge potential in enhancing flexibility toward carbon neutrality (Lin et al., 2024). Virtual power plants (VPPs) often participate in power grid operations by aggregating and coordinating massive DERs on the user side, thereby improving the safety, reliability, economy, and flexibility of the power system operations greatly (Yang et al., 2024), while also assisting the DERs with participation in multiple electricity markets (Lin et al., 2023a). The European Union, Australia, United States, and Japan have extensively explored and demonstrated the applications of VPPs as well as related technologies, which have resulted in relatively mature market mechanisms (Wang et al., 2015a). Over the past decade, investigators have implemented some VPP projects in the United States and European Union for research and application development. Their main purpose was to achieve effective management of large-scale and geographically dispersed DERs (Mashhour and Moghaddas-Tafreshi, 2010), eliminate the intermittent and random effects of renewable energy generation (Lin et al., 2021), improve energy utilization efficiency, tap market potential (Tan et al., 2017), improve the utilization efficiency of distributed energy (Lin et al., 2023b), expand the functions of the electricity market, and achieve coordinated management of energy and load (Lu et al., 2018). In recent years, DERs such as photovoltaics, electric vehicles, and battery energy storage systems have developed rapidly in China, for which VPPs have gradually attracted attention. However, the user base of VPPs in China is relatively small, and the scenarios for participating in power grid interactions and market transactions are relatively few (Zhang et al., 2009). There is a lack of unified trading decision-making research for the profit-making behaviors of VPPs in the market.
The primary objective of a VPP is to consolidate different types of DERs into a cohesive entity that can participate in the wholesale market as a single power plant with a defined hourly output (Yi et al., 2019). VPPs combine various generators and storage systems, each with their own strengths (e.g., high short-term energy storage) and weaknesses (e.g., output uncertainties), in a complementary manner (Koeppel, 2003). Consequently, previous studies have extensively explored the question of how a VPP acting as an agent for all aggregated DERs under its control (Liu et al., 2011) can maximize profits by selling electricity to system operators in the electricity market. The optimal bidding strategy of a VPP in the energy market was discussed by Tang and Yang (2019) through a robust optimization model that handles uncertainties. Additionally, by recognizing that VPPs also provide ancillary services, Wang et al. (2017) proposed an optimal bidding strategy for providing energy and ancillary services to the bulk power system by considering flexible ramping products. Yuanyuan et al. (2023) proposed a VPP declaration strategy for participating in the power market by considering flexible ramping products and uncertainties through a two-stage distributed robust optimization. Fusco et al. (2023) presented a multistage stochastic mixed-integer linear program with binary recourse as an alternative to solve the uncertainties and optimize the unit commitments of power plants and VPPs operating in the day-ahead market. The bidding problem of a VPP in a competitive market was outlined by Wang et al. (2015b) through an infinite repeated game constructed among the VPPs.
However, existing research methods have not accounted for the correlations between different market mechanisms and have not considered the orderly trading of VPPs in multiple markets that does not maximize their profits. At the same time, extant bidding methods cannot effectively reduce the risks involved in market transactions as they do not take into account the penalty for breach of contract when dealing with multiple types of markets. The various VPP trading models available at present do not provide assessments for the application of VPP trading at different levels in various types of actual electricity markets, resulting in weak applicability, limited profit margins, and severe impact of single-market price fluctuations on profits. These models do not fully realize the market values of the VPPs and lack consideration of their market return risks.
The present study aims to fully explore the participation mechanisms of VPPs in energy and ancillary service markets. By considering the electricity markets in the southern region of China as an example, we studied the mechanisms and policies of VPPs participating in different energy, frequency regulation, demand–response, and reserve markets to build the participation architecture of these VPPs. Based on this architecture, clear bidding strategies are designed for the VPPs in these electricity markets. The major contributions of this study are as follows:
The remainder of this paper is organized as follows. Section 2 presents the model formulation for the VPP bidding strategies in the joint energy–reserve–frequency–demand-response market; Section 3 presents the joint market temporal rolling method. Section 4 presents the numerical results, and Section 5 presents the conclusions of this work.
2 Model formulation
The following assumptions are made to develop the model for VPP bidding in the day-ahead and real-time markets:
2.1 Day-ahead market
VPPs have recently participated in energy, demand–response, frequency regulation, and interprovincial reserve markets with the objective of maximizing profits, which include revenues from multiple markets minus the costs associated with internal generation, energy storage operations, and load management. The objective function consists of three parts as follows: 1) revenues of the VPP participating in the energy, demand–response, frequency regulation, and cross-provincial reserve markets; 2) internal generation and energy storage operation costs; 3) adjustable load subsidy within the VPP. The bidding model P1 of the VPP participating in the day-ahead market is given by
Equation 1 is the objective function, which is the expected benefit from the day-ahead market;
2.2 Real-time market
The VPPs also participate in the energy, demand–response, frequency regulation, and interprovincial reserve markets in real time. Here, the VPP submits the frequency regulation capacity and mileage to the frequency regulation market, responds to demand based on the system instructions, and determine the reserve as well as adjusted reserve trading capacities. The objective function entails maximization of the profits, which includes returns from participation in multiple markets minus the costs of additional generation and additional load management. Then, the bidding model P2 of the VPP participating in the real-time market is given by
The objective function given by Equation 20 represents the real-time profits of the VPP from multiple markets. Equation (21) gives the output adjustment of the generators, while Equation 22 accounts for the reserve adjustment
3 Joint market temporal rolling bidding algorithm
According to the Guangdong Electricity Market Spot Energy Trading Rules of 2022 (Bureau, 2022a), Southern Regional Frequency Modulation Auxiliary Service Market Trading Rules (Trial) (Bureau, 2022b), Southern Regional Power Reserve Auxiliary Service Market Trading Rules (Draft for Comments) of 2022 (Bureau, 2022c), and Implementation Rules for VPPs in Shenzhen (Development of Shenzhen, 2022), the detailed operational timetables for VPPs participating in various markets are as shown in Figure 1. Based on these timetables, we derive the joint market temporal rolling bidding framework in terms of two types of operations, namely day-ahead and real-time operations. The joint market temporal rolling bidding framework in multiple electricity markets is as shown in Figure 2.
In the day-ahead stage, the VPP operators receive market information released by the market managers before 12:00; confirm their eligibility to participate; and jointly apply to the energy, frequency regulation, and demand–response markets from 12:00 to 13:00. The market clearance results are then collected before 17:00. Thus, in the joint declaration stage of the energy, frequency regulation, and demand–response markets, the VPP operator solves model P1 based on the predicted market prices and obtains the corresponding bidding capacities of the different markets on the previous day. The bidding volume of the reserve market is regarded as a reference for subsequent single-market bidding. Normally, the results of market clearance for the energy, frequency regulation, and demand–response markets are released before 16:30. This means that the VPP operator would have already obtained the cleared results of the preorder market before optimizing the interprovincial reserve decision, i.e., the market price and winning capacity are known quantities. The VPP operator then predicts the interprovincial reserve market price and solves model P1 to obtain the reserve market bidding volume. These steps are shown in Algorithm 1 below.
In the real-time stage, the VPP operators participate in the real-time energy, frequency regulation, demand–response, and cross-provincial backup markets. The declaration clearance time and market rolling method vary among the different markets. First, by predicting the market prices and conducting joint market declarations, the energy and demand–response market bidding volumes obtained by solving model P2 are considered as the current market bidding volumes with a 15 min duration, while the frequency regulation and interprovincial reserve market bidding volumes are considered as the current market bidding volumes with a 1 h duration. After 15 min, given the real-time frequency regulation and reserve market prices as well as the intermediate scalar, the prices of the energy and demand–response markets are obtained. The bidding volumes of the energy and demand–response markets obtained by solving model P2 are considered as the current market bidding volumes. The joint bidding process is repeated after 1 h until 24:00. These steps are shown in Algorithm 2 below.
The proposed market bidding problems P1, P2 can be easily solved using off-the-shelf solvers (e.g., Gurobi) and the proposed algorithms.
Algorithm 1.Framework of the joint market temporal rolling bidding method for VPP participation in the day-ahead market.
Input:
The predicted market prices:
Output:
The bidding quantities in different markets:
1.Solve the joint market bidding model P1 to determine the bidding quantities in the energy, frequency regulation, and demand–response markets (
2.Once the energy, frequency regulation, and demand–response markets are cleared, input the cleared quantities and prices (
3.Solve the single-market bidding model and obtain the bidding quantity in the reserve market (
Algorithm 2.Framework of the joint market temporal rolling bidding method for VPP participation in the real-time market.
Input:
The predicted market prices:
Output:
The bidding quantities in different markets:
1.Solve the joint market bidding model P2 to determine the bidding quantities in the frequency regulation and demand–response markets (
2.Once the frequency regulation and demand–response markets are cleared, input the cleared quantities and prices (
3.Solve P2 and obtain the bidding quantities in the energy and reserve markets (
4.For each hour in the real-time market, repeat steps 1–3.
5. return
4 Simulation
In this study, the modified IEEE 9-bus system was considered as the test system. A single VPP was built using several DERs, like distributed generators, photovoltaic panels, storage systems, and flexible loads. The detailed system setting is shown in the supplementary material along with the parameters of the DERs as well as the predicted prices of the energy and ancillary service markets. The 24 h bidding results are shown in Figure 3, representing the bidding quantities in the energy, demand–response, reserve, and frequency regulation markets. The bidding quantity in the energy market includes the day-ahead base terms and real-time adjustments, while that in the ancillary service market includes the combined bidding quantities from the day-ahead and real-time markets because we assume that the prediction for the ancillary service market does not have any error. It is clear that the VPP operator only needs to bid once in the frequency regulation market because of the market mechanism.
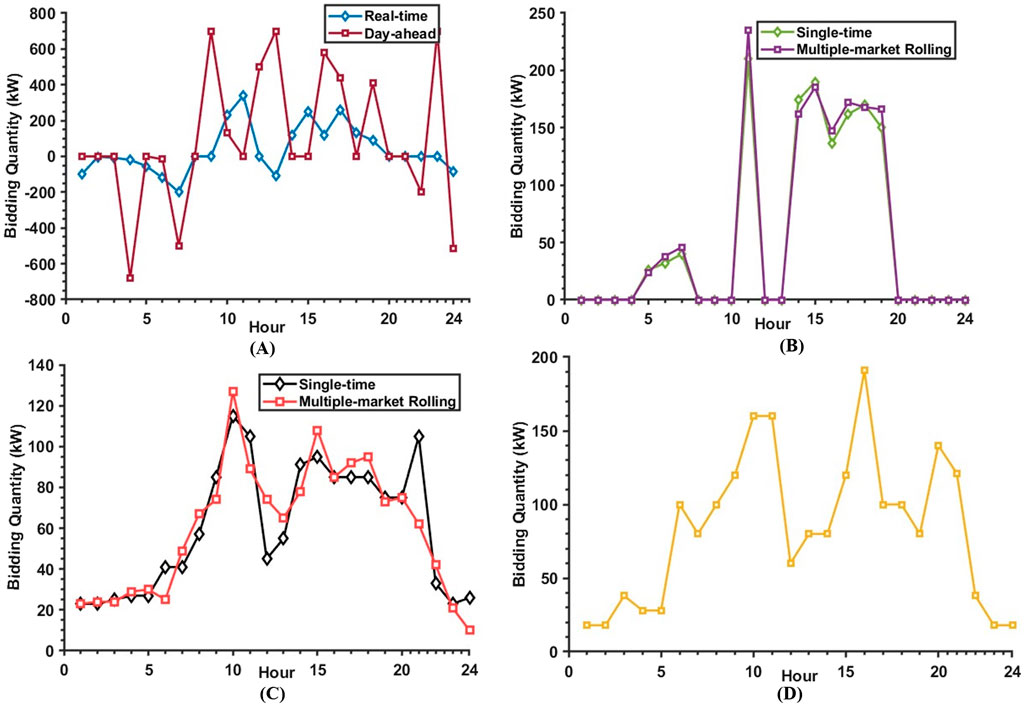
Figure 3. Bidding quantities in the (A) energy, (B) demand–response, (C) reserve, and (D) frequency regulation markets.
According to Figure 3, during the peak load periods, the ability of the VPP to provide frequency regulation is limited because its internal energy balance must be prioritized. At 16:00, the peak photovoltaic output is not during the peak load period, so the VPP provides more frequency regulation capacity. Demand–response is mainly focused on peak shaving during the peak periods, such as 11:00–12:00 and 14:00–20:00. The simulations also allow the VPP to provide valley filling services, with the main valley filling period being 5:00–8:00. Based on the market rolling method, the VPP operator knows the clearance volumes for the real-time frequency regulation and reserve markets, so that they can obtain more accurate bidding volumes. During the peak load periods, the ability of the VPP to provide reserve power is limited because its internal energy balance is prioritized. In the rolling mode of the market, the real-time market has specific periods in which the upward reserve is needed, and the call capacities are subsidized on the basis of the real-time electricity prices, so there is a large difference in the real-time market returns. Moreover, the VPP operator can change their role in the energy market by either selling electricity to or buying it from the energy market according to the changing tendencies of the market prices. In summary, using the market rolling model to optimize the decision-making of VPPs participating in different types of markets can increase the total revenue by about 13% compared to solving the bidding model once; these results are shown in Table 1. Moreover, we tested the total time required to solve the proposed algorithms. Indeed, the improved bidding strategy decreases the solution time, with a slight but worthwhile sacrifice in computing efficiency for higher participation profits.
5 Conclusion
This work first proposes bidding strategies for VPPs in multiple electricity markets based on local, real market mechanisms, thereby enabling a large and solid application scale to the proposed method. Participation architectures are designed for the VPP operator in multiple markets according to official policies and market mechanisms. Based on these improvements, the joint market temporal rolling method is proposed. Then, the bidding models for the VPP in the energy and ancillary service markets are presented with their solution algorithms. These approaches allow the VPP operator to bid in multiple markets precisely while achieving greater market returns. Simulations were performed to illustrate the proposed strategies, which show decent performances that are in agreement with the expected conclusions noted during model formulation.
Data availability statement
The original contributions presented in the study are included in the article/supplementary material; further inquiries can be directed to the corresponding author.
Author contributions
TM: data curation, investigation, project administration, supervision, validation, and writing–original draft. HL: conceptualization, data curation, formal analysis, methodology, resources, software, validation, visualization, writing–original draft, and writing–review and editing. RC: conceptualization, data curation, investigation, methodology, project administration, resources, and writing–review and editing. JL: investigation, methodology, software, supervision, validation, and writing–review and editing. BZ: data curation, funding acquisition, methodology, project administration, supervision, validation, writing–review and editing, resources, and visualization. WZ: funding acquisition, methodology, project administration, resources, visualization, investigation, supervision, validation, and writing–review and editing. TW: funding acquisition, investigation, resources, supervision, validation, visualization, software, and writing–review and editing.
Funding
The authors declare that financial support was received for the research, authorship, and/or publication of this article. This work is supported by the Science and Technology Project of China Southern Power Grid (grant no. SZKJXM20220036/09000020220301030901283).
Conflict of interest
TM, RC, JL, BZ, WZ, and TW were employed by the China Southern Power Grid.
The remaining author declares that the research was conducted in the absence of any commercial or financial relationships that could be construed as a potential conflict of interest.
The authors declare that this study received funding from China Southern Power Grid. The funder had the following involvement in the study: study design, interpretation of data, the writing of this article.
Publisher’s note
All claims expressed in this article are solely those of the authors and do not necessarily represent those of their affiliated organizations, or those of the publisher, the editors, and the reviewers. Any product that may be evaluated in this article, or claim that may be made by its manufacturer, is not guaranteed or endorsed by the publisher.
References
Bureau, S. C. E. R. (2022a). Guangdong electricity market Spot energy trading Rules 2022. Available at: https://nfj.nea.gov.cn/nfjdoc/aa9c1ba4-c055-4a6a-8b33-72e5203d42bf.pdf.
Bureau, S. C. E. R. (2022b). Guangdong frequency modulation auxiliary service market trading Rules (Trial). Available at: https://nfj.nea.gov.cn/file_upload/20180831/48741535676232760_5da41239-065b-41d3-8d4d-0ce94b5cae43.pdf.
Bureau, S. C. E. R. (2022c). Southern regional power reserve auxiliary service market trading Rules (draft for Comments) 2022. Available at: https://nfj.nea.gov.cn/nfjdoc/cb26c894-a421-4e37-b877-2fd6534689ef.pdf.
Development of Shenzhen, R. C. (2022). Implementation Rules for virtual power plants in shenzhen. Available at: https://fgw.sz.gov.cn/gkmlpt/content/11/11351/mpost_11351896.html.
Fusco, A., Gioffrè, D., Castelli, A. F., Bovo, C., and Martelli, E. (2023). A multi-stage stochastic programming model for the unit commitment of conventional and virtual power plants bidding in the day-ahead and ancillary services markets. Applied Energy 336, 120739. doi:10.1016/j.apenergy.2023.120739
Lin, H., Guo, Y., Zhou, J., Xu, M., Wu, W., Sun, H., et al. (2023a). “A review on virtual power plants: development progress and market participation mechanisms,” in 2023 IEEE 7th conference on energy internet and energy system integration (EI2) (IEEE), 2359–2364.
Lin, H., Nazir, F. U., Pal, B. C., and Guo, Y. (2023b). A linearized branch flow model considering line shunts for radial distribution systems and its application in volt/var control. J. Mod. Power Syst. Clean Energy 11, 1191–1200. doi:10.35833/mpce.2022.000382
Lin, H., Shen, X., Guo, Q., and Sun, H. (2021). “Accuracy and application scope analysis for linearized branch flow model in radial distribution systems,” in IEEE power and energy society general meeting (PESGM). IEEE, 1–5.
Lin, H., Shen, X., Guo, Y., Ding, T., and Sun, H. (2024). A linear distflow model considering line shunts for fast calculation and voltage control of power distribution systems. Applied Energy 357, 122467. doi:10.1016/j.apenergy.2023.122467
Liu, C., Shahidehpour, M., and Wang, J. (2011). Coordinated scheduling of electricity and natural gas infrastructures with a transient model for natural gas flow. Chaos Interdiscip. J. Nonlinear Sci. 21, 025102. doi:10.1063/1.3600761
Lu, T., Wang, Z., Wang, J., Ai, Q., and Wang, C. (2018). A data-driven stackelberg market strategy for demand response-enabled distribution systems. IEEE Trans. Smart Grid 10, 2345–2357. doi:10.1109/tsg.2018.2795007
Mashhour, E., and Moghaddas-Tafreshi, S. M. (2010). Bidding strategy of virtual power plant for participating in energy and spinning reserve markets—part i: problem formulation. IEEE Trans. Power Syst. 26, 949–956. doi:10.1109/tpwrs.2010.2070884
Tan, Z., Wang, G., Ju, L., Tan, Q., and Yang, W. (2017). Application of cvar risk aversion approach in the dynamical scheduling optimization model for virtual power plant connected with wind-photovoltaic-energy storage system with uncertainties and demand response. Energy 124, 198–213. doi:10.1016/j.energy.2017.02.063
Tang, W., and Yang, H.-T. (2019). Optimal operation and bidding strategy of a virtual power plant integrated with energy storage systems and elasticity demand response. IEEE Access 7, 79798–79809. doi:10.1109/access.2019.2922700
Wang, J., Zhong, H., Tang, W., Rajagopal, R., Xia, Q., Kang, C., et al. (2017). Optimal bidding strategy for microgrids in joint energy and ancillary service markets considering flexible ramping products. Applied Energy 205, 294–303. doi:10.1016/j.apenergy.2017.07.047
Wang, Y., Ai, X., Tan, Z., Yan, L., and Liu, S. (2015a). Interactive dispatch modes and bidding strategy of multiple virtual power plants based on demand response and game theory. IEEE Trans. Smart Grid 7, 510–519. doi:10.1109/tsg.2015.2409121
Wang, Y., Ai, X., Tan, Z., Yan, L., and Liu, S. (2015b). Interactive dispatch modes and bidding strategy of multiple virtual power plants based on demand response and game theory. IEEE Trans. Smart Grid 7, 510–519. doi:10.1109/tsg.2015.2409121
Yang, L., Li, H., Zhang, H., Wu, Q., and Cao, X. (2024). Stochastic-distributionally robust frequency-constrained optimal planning for an isolated microgrid. IEEE Trans. Sustain. Energy, 1–15. doi:10.1109/tste.2024.3404434
Yi, Z., Xu, Y., Gu, W., and Wu, W. (2019). A multi-time-scale economic scheduling strategy for virtual power plant based on deferrable loads aggregation and disaggregation. IEEE Trans. Sustain. Energy 11, 1332–1346. doi:10.1109/tste.2019.2924936
Yuanyuan, Z., Huiru, Z., and Bingkang, L. (2023). Distributionally robust comprehensive declaration strategy of virtual power plant participating in the power market considering flexible ramping product and uncertainties. Applied Energy 343, 121133. doi:10.1016/j.apenergy.2023.121133
Keywords: bidding strategy, distributed energy resource, electricity market, virtual power plant, market mechanism
Citation: Mao T, Lin H, Cheng R, Li J, Zhou B, Zhao W and Wang T (2024) A joint market temporal rolling bidding strategy for virtual power plants in the wholesale market. Front. Energy Res. 12:1473180. doi: 10.3389/fenrg.2024.1473180
Received: 30 July 2024; Accepted: 09 September 2024;
Published: 03 October 2024.
Edited by:
Lun Yang, Xi’an Jiaotong University, ChinaReviewed by:
Lirong Deng, Shanghai University of Electric Power, ChinaZhongkai Yi, Harbin Institute of Technology, China
Copyright © 2024 Mao, Lin, Cheng, Li, Zhou, Zhao and Wang. This is an open-access article distributed under the terms of the Creative Commons Attribution License (CC BY). The use, distribution or reproduction in other forums is permitted, provided the original author(s) and the copyright owner(s) are credited and that the original publication in this journal is cited, in accordance with accepted academic practice. No use, distribution or reproduction is permitted which does not comply with these terms.
*Correspondence: Hanyang Lin, linhy22@mails.tsinghua.edu.cn