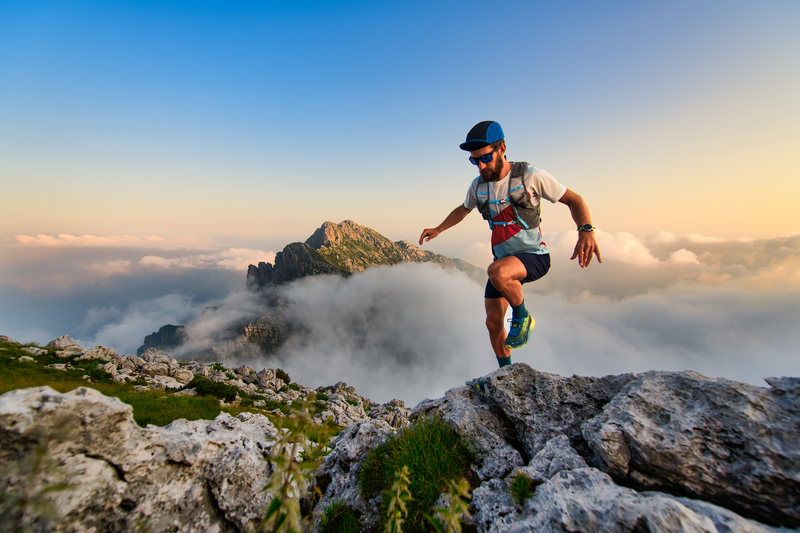
94% of researchers rate our articles as excellent or good
Learn more about the work of our research integrity team to safeguard the quality of each article we publish.
Find out more
REVIEW article
Front. Energy Res. , 11 July 2024
Sec. Process and Energy Systems Engineering
Volume 12 - 2024 | https://doi.org/10.3389/fenrg.2024.1424928
This article is part of the Research Topic Reviews in Energy Research 2024 View all articles
The transition to renewable energy sources is critical for sustainable development, yet integrating these sources into existing power systems poses significant challenges. Energy Storage Systems (ESS) are essential in enhancing the reliability and efficiency of renewable energy systems. Despite growing research, a comprehensive scientometric analysis mapping development and trends in this field is lacking. This study addresses this gap by conducting a detailed scientometric analysis of power systems and new energy research from 2014 to 2023. The novelty of this study lies in its systematic use of advanced bibliometric tools to provide a thorough analysis of the research landscape. Utilizing 425 research articles from the Web of Science database, the study employs CiteSpace to visualize academic networks, identify research hotspots, and outline current trends. Specific methodologies include burst detection to identify significant shifts in research focus, centrality measurement to determine the influence of key studies, and heterogeneous network analysis to map the interconnectedness of various research themes. The analysis reveals extensive international collaborations, with China leading in publication volume (344 articles) and centrality (0.69), followed by the United States (29 articles, centrality 0.53). Significant contributions come from institutions like North China Electric Power University, China Electric Power Research Institute, and Tsinghua University. The findings underscore the importance of international cooperation and the need for broader geographical representation in this research field. This study provides valuable insights into the evolution and current state of power systems and renewable energy research, offering essential guidance for future research and development. The results highlight the critical role of ESS in the transition to renewable energy and suggest directions for future investigations, particularly focusing on enhancing ESS efficiency and reliability and expanding international research collaborations.
In recent years, the field of power systems and new energy has seen rapid growth in research. The number of related journal papers published in the Web of Science database has increased from 3 in 2014 to 125 in 2023. CiteSpace, a prominent visualization tool, is chosen to analyze the academic structure of the field and identify emerging trends in related knowledge areas. When examining complex networks in scientific literature, quantitative research methods are used to gain insights into patterns and intelligence in areas such as electrical energy storage and renewable energy (Chen, 2006). The comprehensive analysis and visualization of these networks allow researchers to spot emerging trends and temporary patterns in their research. The utilization of CiteSpace for systematic quantitative research across different energy and power sectors has received limited attention in the last decade. However, CiteSpace has demonstrated notable effectiveness specifically within the realm of electrical engineering (Zhang et al., 2024).
Bibliometric methods are utilized in a novel manner to improve the analysis of extensive literature databases. This survey showcases visual depictions of collaboration networks, explores evolutionary trends, pinpoints research areas of focus, and contrasts various methodologies within the realm of power systems research (Geissdoerfer et al., 2017; Bjornbet et al., 2021). In essence, this study lays the groundwork and offers direction for the advancement of power systems and new energy sources.
In the realm of power systems and electric vehicles, studies have indicated that North China Electric Power University, China Electric Power Research Institute, and China State Grid Corporation engage in significant collaboration (Zhang et al., 2020; Wang S. J. et al., 2022). This exemplifies the close partnership among key research countries and institutions, underscoring the value of collective efforts in advancing research within this domain. Such collaboration facilitates the exchange of knowledge, resources, and expertise, ultimately enhancing the research capacities of the involved institutions.
In the fields of thermodynamics and sustainable development science, there is notable collaboration among major countries like China, the United States, and the United Kingdom (Ke et al., 2020; Wu H. Y. et al., 2021; Geng et al., 2023). As a result, research attention has shifted towards energy management systems (EMS) specifically tailored for battery energy storage systems (BESS). Distributed mobile energy operation control methods have demonstrated significant potential in energy management applications for large-scale BESS (Liu et al., 2016; Linnenluecke et al., 2020; Ding and Yang, 2022).
Previous research in the field of renewable energy and electrical energy storage, as discussed in reference (Demiroren and Yilmaz, 2010), focused on system configuration and cost analysis using HOMER software to explore the utilization of solar energy, wind energy, and batteries for powering Gokceada Island. Reference (Jindal et al., 2019) also delved into the current status and gaps in distributed renewable energy communication standards, proposing potential pathways for enhancement. Additionally, reference (Dumitrascu et al., 2019) analyzed Romania’s renewable energy potential, the impact of climate change on renewable energy, and associated environmental challenges. These studies offered valuable insights into the development of renewable energy, covering aspects such as cost, communication standards, climate change, and local environmental impacts through practical applications. Reference (Guo et al., 2023) introduced a stochastic optimization method based on the simulated annealing algorithm for optimizing off-grid solar-wind energy and electric energy storage systems. Furthermore, reference (Wang and Liu, 2023) examined the impact of incorporating Bi(Mg2/3Nb1/3)O3 on the electrical energy storage performance of 0.76Bi(0.5)Na (0.5)TiO3-0.24SiTiO3 ceramic, as well as exploring the electrical energy storage capabilities of electronic ceramic materials. Reference (Maghrabie et al., 2023) also highlighted the role of energy storage systems in ensuring fresh water supply by integrating renewable energy with seawater desalination systems. The above references offered innovative ideas in electrical energy storage through novel system optimization methods, material combinations, and system integrations.
In comparison with the current research works, our analysis focuses on the collaboration of major countries in this research field and the co-occurrence of keywords. However, there is a gap in knowledge about bibliometric reviews on topics related to new energy sources in power systems. Therefore, this study aims to:
1) Data Sources and Research Methods: Collect and analyze 425 research articles from the Web of Science database, focusing on publications from 2014 to 2023. Utilize CiteSpace for visualizing academic networks, identifying research hotspots, and outlining current trends through burst detection, centrality measurement, and heterogeneous network analysis.
2) Data Analysis: Examine yearly publication trends to understand research growth in new energy power systems. Identify leading countries and significant international collaborations. Assess contributions from major institutions and their collaborative networks.
3) Visualization Results Analysis: Conduct cluster analysis of keywords to identify prominent research areas and emerging trends. Explore research frontiers and shifting trends through keyword timeline analysis. Review the most influential papers to understand key contributions and foundational research.
4) Discussion of Trends and Research Frontiers: Discuss the effectiveness of CiteSpace in analyzing bibliometric data. Analyze publication trends in major journals, focusing on IEEE Transactions on Power Systems. Examine the evolving focus on distributed generation within power systems, identifying emerging trends and future research directions.
By achieving these objectives, the study offers a comprehensive overview of the current state and future prospects of power systems research.
This study aims to provide a comprehensive scientometric analysis of power systems and new energy research from 2014 to 2023. In this context, the following components are critical to understanding the scope and boundaries of the research:
1) International Collaboration (IC): The study highlights the extensive international collaborations in the field, focusing on contributions from leading countries such as China, the United States, and the United Kingdom. These collaborations are essential for advancing research and development in new energy technologies, sharing knowledge, and leveraging global expertise.
2) Bibliometric Coverage (BC): The research is based on 425 documents sourced from the Web of Science database. This comprehensive dataset provides a broad view of the developments in power systems and new energy research over the past decade. The use of advanced bibliometric tools, particularly CiteSpace, allows for a detailed analysis of academic networks, research hotspots, and emerging trends.
3) Limitations of the Study: Despite the extensive data collection and analysis, the study has several limitations. First, it relies solely on publications indexed in the Web of Science database, which may exclude relevant research published in other databases or non-indexed journals. Second, the bibliometric analysis is inherently retrospective, potentially missing the most recent developments that have not yet been widely cited. Third, the study focuses on specific keywords and topics, which may not capture the entire scope of research in the field.
4) Presumptions: The study presumes that the Web of Science database provides a representative sample of the most significant research contributions in the field of power systems and new energy. It also assumes that the bibliometric tools and methods used, such as CiteSpace, accurately reflect the structure and dynamics of the research landscape. Furthermore, the study presumes that the identified trends and hotspots are indicative of broader developments in the field.
Web of Science is a comprehensive multidisciplinary core journal database. This article utilizes two databases from the core collection of WOS, namely, the Web of Science Core Collection (Science Citation Index Expanded - SCIE, Social Sciences Citation Index - SSCI) as data sources. The search was conducted using the formula [TS = “power system*” AND “new energy*“] with a search time of 2023-12-23. A total of 477 documents were retrieved using this search term, including 437 research articles, 37 review articles, 1 conference abstract article, and 2 other articles. Of these, Note, Editorial, Business article, Letter and Data Paper type articles are not available. From these, only the 437 research articles were screened, and English-language articles were selected. After carefully reviewing the article titles, abstracts, and contents, any articles that were deemed irrelevant were excluded from the study. This process narrowed down the initial pool of articles to a final selection of 425, which met the criteria for scientific measurement. The distribution of these articles, in terms of the number published each year.
This study utilized a text form of the collected article data, which included complete records and citations. The data was then subjected to in-depth analysis using CiteSpace 6.1. R6 software. The study followed CiteSpace’s standard operational procedures for constructing knowledge maps, which include time slicing, threshold setting, modeling, trimming, merging, and mapping. By leveraging CiteSpace’s key features such as burst detection, centrality measurement, and heterogeneous network analysis, this research visually represents the trajectory of research development, identifying key issues and frontiers in the field (Freeman, 1977; Ghosh and Mount, 1991; Attiratanasunthron and Fakcharoenphol, 2008). In the diagrams depicting networks, every individual node symbolizes authors, institutions, countries, or keywords. The occurrence or citation frequency of each node determines its size, whereas the time of occurrence or citation is represented by the color of the nodes. Notably, nodes with purple borders indicate entities with high betweenness centrality, which mark key turning points or hotspots in the research area (Zheng and Wang, 2019; Li et al., 2020; Wang et al., 2020). Centrality analysis uncovers key nodes within the research network, representing elements with significant influence in the field. This has been demonstrated by Chin et al. (2014) in their development of cytoHubba, a tool for identifying central objects in complex interaction groups, and by Liang et al. (2017) in their bibliometric analysis of acupuncture treatment for low back pain over a 20-year period using CiteSpace (Antoniotti et al., 2013; Chin et al., 2014; Liang et al., 2017; Modrák and Vohradsky, 2018; Wang et al., 2021; Zhang et al., 2021). In this article, we employ the quantitative tools provided by CiteSpace to conduct an extensive bibliometric analysis of research in the field of power systems and new energy. Through critical reading, we extract key research results and gain insights into future development trends in the field.
We used Web of Science analysis tools to classify and archive the collected literature. A quantitative analysis of the annual publication volume, contributing countries, institutions, and authors was conducted using an Excel spreadsheet. Additionally, we employed CiteSpace (6.1. R6) software to analyze co-words, co-occurrences, and emergent words of important noun phrases in document titles, abstracts, and keywords. The objective of this analysis was to identify the development trajectory and research hotspots of new energy technology innovation in power systems. In CiteSpace, we set a 10-year time span with annual time nodes. The node types selected were ‘Country’ and ‘Keywords’, with ‘Cosine’ used as the node intensity and a threshold of ‘Top 20'. To simplify the network, we used the ‘minimum spanning tree’ option, and for analyzing changes in keywords over time, we utilized the ‘time zone’ function.
A scrutiny of the yearly dissemination of scholarly works concerning power systems and new energy exploration in the principal database of scientific literature, Web of Science core collection, throughout the preceding decade exposes that the mean count of publications within this timeframe solely reached 75. Nevertheless, there exists notable advancement in research, which is consistently advancing. In recent times, the quantity of publications and references has exhibited unfaltering expansion. As shown in Figure 1, the number of documents was relatively small before 2014, but experienced rapid growth after 2018. Similarly, the number of citations increased from an initial count of 14 to a total of 1863. Although the number of studies has not yet reached a high level, research efforts are rapidly developing. Furthermore, based on research trends, there is considerable research potential in the coming years, indicating that the research volume will continue to grow.
Figure 1. (2014-2023) Temporal evolution of the total number of articles and citations in the WOS database.
Table 1 presents the total count and proportion of various article types within the domain of power systems and innovative energy storage solutions. The analysis includes research articles, reviews, conference papers, and other types of scholarly contributions. The predominant type of publication is the research article, comprising 437 entries, which accounts for a significant 91.61% of the total publications. Reviews follow with 37 entries, representing 7.76% of the total. Conference papers are scarce, with only 1 entry, constituting 0.21%. Other types of publications, including notes, editorials, business articles, letters, and data papers, were either absent or minimally represented, with the category labeled “Other” contributing just 2 entries (0.42%). The significant proportion of research articles underscores the intensive investigative efforts and the development of new insights and technologies. The relatively smaller percentage of reviews indicates a need for more comprehensive synthesis of existing research, while the minimal presence of other publication types suggests potential areas for further scholarly exploration.
Table 2 delineates the categories and proportions of research articles in the Web of Science (WOS) database related to power systems and innovative energy storage solutions. This categorization provides a detailed overview of the diverse research areas contributing to this field.
The majority of the research articles fall under the category of Engineering, with 163 articles, making up 37.30% of the total. This indicates a strong focus on engineering aspects in the development and optimization of power systems and energy storage solutions. Following this, the Energy Fuels category includes 87 articles, representing 19.91%, reflecting significant research interest in fuel sources and energy efficiency. The Science Technology category encompasses 56 articles (12.81%), showcasing advancements and technological innovations. Computer Science, with 22 articles (5.03%), highlights the integration of computational methods and data analysis in energy research. The Physics category includes 19 articles (4.35%), indicating the foundational scientific principles being explored. The “Other Topics” category includes 28 articles (6.41%), covering miscellaneous subjects related to the field.
The categorization of research articles underscores the multidisciplinary nature of the study of power systems and innovative energy storage solutions. By understanding the distribution of research efforts across various scientific and technological categories, this study provides insights into the current trends and potential future directions. The significance of this research lies in its ability to inform policymakers, researchers, and industry stakeholders about the focal points of scholarly activity.
This study aims to identify the main countries conducting research in the field of new energy research in power systems and examine the academic exchanges and cooperation between these countries. Quantitative analysis was performed on the countries that have published research papers. The analysis utilized CiteSpace software and covered the time period from 2014 to 2023. Figure 2 shows that this analysis is conducted annually, with a top 10 threshold. The resulting analysis produced 52 networks, represented by a country analysis map consisting of nodes and 100 connections. The density of the analysis was calculated to be 0.0754. The importance of a node in the network, based on its position, can be understood by examining the thickness of the purple circle. This measure is known as betweenness centrality and provides insights into the significance of a node in the network (Newman, 2003; Wang et al., 2018; Tao et al., 2022).
In the map (Figure 3), each line represents a collaborative relationship, with the number and color intensity of the lines indicating the frequency of cooperation. Darker and thicker lines denote more frequent collaborations, while lighter and thinner lines indicate less frequent interactions. The map reveals that China and the United States are central hubs in this global network, with numerous and intense lines connecting them to various countries, indicating high levels of international cooperation. Other significant nodes include England, Germany, and Canada, which also show substantial collaboration frequencies with multiple countries. This network visualization underscores the pivotal roles of these countries in driving international research efforts and fostering academic exchanges in the field of new energy systems.
The network density calculated in two parts of this study represents the level of interconnectedness and collaboration among countries in the field of research on new energy in power systems. Network density is a crucial metric as it quantifies the extent of collaboration and information exchange between different countries. A higher network density indicates a more cohesive and integrated research network, where countries frequently collaborate and share knowledge, leading to more robust and comprehensive advancements in the field. The necessity of calculating network density lies in its ability to highlight the collaboration patterns and the central roles of specific countries within the global research network. By understanding these patterns, researchers and policymakers can identify key players, potential collaborators, and gaps in international cooperation. This insight is essential for fostering more effective and strategic partnerships, promoting knowledge dissemination, and accelerating the development of innovative solutions in power systems and energy storage technologies.
Figure 4 and Figure 5 display the top 10 countries with the highest frequency. Frequency indicates the number of times a country is mentioned, while centrality represents the country’s position within the field. The centrality metric reveals the interconnectedness between countries, meaning that the more connections a country has, the higher its centrality. A higher centrality indicates a more significant role in research, reflecting the country’s importance.
Figure 4. Total number of publications issued by the Top10 Countries/Regions. (Top 10 countries that have issued new energy journal articles for power systems in the past 10 years).
Figure 5. Centrality of publications issued by the Top10 Countries/Regions. (Top 10 countries that have issued new energy journal articles for power systems in the past 10 years).
Figure 4 and Figure 5 show that China is the leading country in the field of research on new energy in power systems, with 344 publications, which is significantly more than other countries. This indicates China’s strong interest and rapid advancement in hydrogen fuel cell technology, positioning it as a frontrunner in this domain (Xu et al., 2020; Cheng and Lv, 2021; Shi et al., 2024). China’s centrality is 0.69, suggesting that cross-border cooperation is relatively common for China. The United States ranks second with 29 publications, while Iran ranks third with 16 publications. In terms of centrality, the United States and Iran have significantly different centrality values compared to China, indicating relatively little international cooperation for these two countries. Spain, Poland, and Denmark have the lowest centrality values and almost no international cooperation. On the other hand, England, Canada, and Singapore have centrality values exceeding 0.1, indicating that research in these countries is the result of cooperation among multinational researchers. The research on China, the United States, and Iran started in 2014 and has shown a relatively high frequency, highlighting the foundational role played by these three countries.
An institutional analysis map was generated using institutions in CiteSpace, revealing a network of 236 nodes and 196 connections. The density of this network was calculated to be 0.0071. The nodes representing North China Electric Power University, China Electric Power Research Institute, and Tsinghua University were found to be densely connected and primarily focused on data. This suggests that these institutions play a central role in the research field. The visual clustering and thick connecting lines further indicate that these institutions are involved in extensive collaborative efforts and engage in high levels of research activity. These findings provide evidence of strong academic exchanges in the field of power system blockchain technology research (Wang N. et al., 2019; Gu et al., 2024; Shukla et al., 2024).
Many research institutions in Figure 6, such as North China Electric Power University, China Electric Power Research Institute, Tsinghua University, Southeast University, and China Three Gorges University, actively engage in collaborations. These partnerships exhibit distinctive regional characteristics, highlighting the importance of cross-institutional research and collaboration in fostering academic exchanges within the Source-grid-load system research field (Wen et al., 2016; Xi et al., 2018; Zhou et al., 2020; Gu et al., 2021; Lu et al., 2021; Guo et al., 2022).
This article also presents a ranking of the top 10 publishing institutions in the field of new energy research, as shown in Figure 7 North China Electric Power University has the highest number of publications, with 47 articles, surpassing other institutions. On the other hand, Guangxi University ranks relatively low, indicating that it publishes more frequently but has less collaboration with other institutions. This observation can also be deduced from the publication years. North China Electric Power University and China Electric Power Research Institute published their research earlier, suggesting their significant contributions to photovoltaic system research. China Three Gorges University, with 11 publications, secured the fourth position. It is noteworthy that these publications were made in 2018, indicating a relatively late focus on wind energy research by China Three Gorges University. However, this institution’s centrality value of 0.08 is relatively high, suggesting recent attention towards wind energy research. China Three Gorges University has conducted in-depth research on new energy reliability assessment and has provided valuable insights into the current cutting-edge research direction.
Figure 7. Centrality vs Count by Institution. (Top 10 organizations that have published articles on the field of new energy and power system in the last decade).
The present study utilizes Citespace software to perform cluster analysis on keywords. In order to ensure the accuracy of cluster classification, the panthfinder algorithm is employed to cut connections. The findings of this analysis are visually presented in Figure 8, which effectively depicts the research topics pertaining to power systems and new energy over the course of the previous decade. The cluster numbers assigned in the figure correspond to the topics derived from clustering the keywords through the LLR algorithm. Furthermore, the results reveal a total of 11 distinct clusters, and the detailed information regarding the clustering for each cluster is provided in the accompanying figure.
Figure 8. Clustering of Top11 keywords in the field of new energy for power systems in the last decade.
The objective of this research is to examine the evolution of keyword hotspots in the realm of new energy research within the field of electrical engineering. To achieve this, we employed the Bursts detection algorithm from Citespace software for analysis. The primary aim of this study is to identify the emergence of keywords and showcase their intensity in the domain of new energy in the power system. For a comprehensive understanding of the emergence intensity and the duration of hotspots, please refer to Table 3. The presented information displays blue lines indicating the time intervals of emergence, while the red line segments represent the start and end years of burst durations within each topic category.
According to Table 3, the hot spots in the energy field were prevalent from 2014 to 2018. During the period between 2016 and 2018, the research focus shifted towards integration, management, and emission. However, with the passage of time, 2019 witnessed the emergence of new topics and hot spots, including photovoltaic systems, efficiency, synchronous generators, and stability. These developments highlight that new energy power generation systems, power system stability, and power system efficiency are currently at the forefront of research in this field.
This study utilizes Citespace to analyze the changes in keywords over time in the field. The analysis node selected for generating the keyword time graph is Timeline. To generate the current graph, keywords with smaller nodes are omitted, with the specific indicators and thresholds as follows: time slice set to 1 and the threshold chosen as g-index = 5.
The timeline presented in Figure 9 illustrates the distribution of keywords over time in the research fields of energy, power systems, and modeling. The relatively even distribution of keywords indicates that research topics in these fields are constantly evolving and becoming more diverse. This suggests a dynamic academic environment where researchers continuously adapt and expand their focus to address the evolving theoretical and practical challenges in these fields. The emergence of new keywords over time reflects the natural evolution of the field, driven by factors such as new technologies, changes in regulations, and shifts in global energy demand. These changes necessitate the development of new modeling and system analysis methods in the power and energy sectors. Specifically, during the period of 2016–2017, the field of ‘Advances and Innovations in Renewable Energy Integration’ witnessed the emergence of themes such as integration, management, emission, and power systems. This indicates that the initial focus of renewable energy integration was on power systems, and it continued to prioritize power system research (Li et al., 2016; Wang X. et al., 2019; Lago et al., 2019; Liu Z. C. et al., 2021; Wang W. Z. et al., 2022; Lu et al., 2024). During the period of 2017–2018, the research field of ‘Source-Grid-Load-Storage Coordination Planning Technology’ focused on the hot topics of ‘technology’ and ‘model’. This indicated a strong emphasis on integrating new energy resources into power systems, with a central focus on the technological and modeling aspects (Yang et al., 2018; Wu H. et al., 2021; Chen et al., 2022). Between 2019 and 2021, the research field of ‘Regional Photovoltaic Power Systems’ experienced a surge in themes such as photovoltaic system efficiency, synchronous generator, and stability, highlighting the evolving focus on these aspects of photovoltaic power systems (Lei et al., 2014; Wang S. et al., 2023; Wang S. J. et al., 2023). In the years 2023–2024, the research domain of ‘Hybrid Renewable Energy’ witnessed emerging themes such as virtual synchronous generators, wind turbines, and reliability assessment, reflecting the evolving priorities in this field (Anderson and Grover, 2003; Al-Rawashdeh et al., 2023; Liu D. et al., 2023).
Figure 9. Heat Map of Keyword Activity. (Emergence of Top 20 keywords in the field of new energy in power systems in the past 10 years, and the shade of red represents the magnitude of centrality).
Based on Table 4, the publication that garnered the most citations between 2014 and 2023 is titled ‘Enhancing Intraday Load Forecasting Accuracy by Utilizing Customer Behavior Similarities in Smart Meter Data’ from the academic journal IEEE TRANSACTIONS ON SMART GRID, with a total of 301 citations. Conversely, the periodical APPLIED ENERGY boasts an impact factor of around 11 over the previous 5-year period and has accumulated 187 citations, hosting the article ‘A Comprehensive Review of Recent Advancements and Evolving Challenges in Peer-to-peer Energy Systems for Interconnected Communities’. These two articles delve into advanced energy technology. The first article presents a decision-making framework, while the second article focuses on predictive modeling using machine learning algorithms (Quilumba et al., 2015; Jindal et al., 2016; Tushar et al., 2021; Du et al., 2023; Wang et al., 2024; Yan et al., 2024). The most cited recent study, titled ‘Energy Management and Operational Control Methods for Grid Battery Energy Storage Systems,’ provides a comprehensive examination of advancements in battery energy storage systems and their applications. The study specifically focuses on how these systems can enhance the flexibility, economy, and security of power systems. It delves into various application scenarios and management techniques for large-scale battery storage systems. This study, conducted by Li and Wang in 2021, is a valuable resource for understanding the potential of battery energy storage systems (Tong et al., 2017; Li and Wang, 2021; Huang et al., 2023). Moving on to the next two articles, the first one is titled ‘Research and application of a new hybrid forecast model - taking wind speed forecasting in China as an example.’ This article highlights the importance of forecasting and planning in energy systems. It particularly emphasizes the need for effective strategies and models to forecast and manage energy demand, taking into account the variability of renewable energy sources. By focusing on wind speed forecasting in China, the authors, Wang, J., Wang, Y., and Jiang, P., in 2015, aim to provide insights into developing more accurate and reliable forecasting methods (Wang et al., 2015; Verma et al., 2016; Galván et al., 2021). The second article discussed in this paragraph is titled ‘Designing a low-carbon UK power system in 2050 that is robust to spatial, temporal, and inter-annual changes in weather.’ This article also emphasizes the significance of forecasting and planning in energy systems, specifically in designing resilient low-carbon power systems. The authors, Zeyringer, M., Price, J., et al., in 2018, highlight the importance of considering the variability and unpredictability of weather conditions in the design of future power systems. Their research aims to provide insights into developing power systems that can withstand and adapt to these changes (Huang and McElroy, 2015; Zeyringer et al., 2018; Liu Z. M. et al., 2024).
The literature data collected in this manuscript are obtained from WOSCC, while the CiteSpace software utilizes information from not only WOSCC but also other database networks. Other popular bibliometric software options include VOSViewer and NetDraw. Specifically, CiteSpace, which was developed by Professor Chaomei Chen, is highly regarded in the field of knowledge mapping due to its efficient techniques for visualizing information (Xie, 2015a; Chen C., 2018; Lin and Zhang, 2023). The collected literature data in this manuscript are derived from WOSCC, and the CiteSpace software uses information from a variety of database networks, not just WOSCC. Among the popular bibliometric software tools available, VOSViewer and NetDraw are also commonly used. Notably, CiteSpace, developed by Professor Chaomei Chen, is widely respected within the field of knowledge mapping because of its highly effective approaches for visualizing information (Synnestvedt et al., 2005; Xie, 2015b; Chen C. M., 2018). The articles excel in exploring various aspects of a field, such as collaboration, key points, internal structure, trends, and dynamics (Awad et al., 2014; Wang et al., 2016; Xu et al., 2021; Qin et al., 2022; Sabe et al., 2022; Qian et al., 2023). It is evident that bibliometric software possesses unique advantages, with CiteSpace being a comprehensive tool.
This research paper presents a comprehensive scientometric analysis of the field of Power Systems & Electric Vehicles, focusing on Distributed Generation research. It explores various factors such as publication outputs, international collaborations, and co-occurrence analyses of subject categories and keywords. The findings indicate a significant increase in research interest over the past decade, with a total of 751 journal articles published in this field. However, the distribution of this interest is not equal worldwide, with North America, Europe, and Asia being the main areas of focus (Wang and Wang, 2015; Connell et al., 2017; AlKuwaiti et al., 2024; Schneider et al., 2018; Park et al., 2012; Du and Mohammadi, 2023). One of the noteworthy observations from this analysis is the substantial international collaboration between different regions. The United States and China emerge as the most active contributors, accounting for half of the global publications. Interestingly, the United States shows a higher level of collaboration compared to China (Yang et al., 2013; Li et al., 2014; Muratori and Rizzoni, 2016; Liu Q. P. et al., 2021). In addition, several prolific institutions like the United States Department of Energy (DOE), Argonne National Laboratory, Tsinghua University, Nanyang Technological University, and Nanyang Technological University National Institute of Education (NIE) Singapore are involved in extensive inter-institutional cooperation, as evident from their publications (Zeng et al., 2014; Wang and Chiang, 2016; Hoang et al., 2021; Barth et al., 2023; Liu J. et al., 2023). These findings highlight the global interest and collaboration in the field of Distributed Generation research within the Power Systems & Electric Vehicles domain. The research community, particularly in North America, Europe, and Asia, has shown significant engagement and involvement in advancing knowledge and understanding in this area. The active participation of institutions such as the United States Department of Energy, Argonne National Laboratory, Tsinghua University, Nanyang Technological University, and Nanyang Technological University National Institute of Education (NIE) Singapore further strengthens the collaborative efforts in this field (Brenna et al., 2013; Wanik et al., 2015; Bai et al., 2018; Sun et al., 2019; Zhou et al., 2022; Kurundkar and Vaidya, 2023).
This research presents numerous distinct benefits. Initially, it conducts a methodical examination of the investigations on dispersed generation of sustainable energy via bibliometrics, supplying a comprehensive guide for scholars intrigued by this realm (Ali et al., 2016; Chen et al., 2018; Wei et al., 2023a). Furthermore, unlike conventional reviews, bibliometric analysis has the potential to offer a more all-encompassing comprehension of the prevalent and state-of-the-art concerns in the field. Notwithstanding the escalating inquiries in the sphere of new energy automobiles, most researchers and institutions hail from the United States and China, leading to an evident regional characterization (Azizi et al., 2022; Wei et al., 2023b; Gao et al., 2023). Further exploration and continuous observation are required to determine whether institutions and researchers from various nations are producing profounder, more avant-garde insights into this subject matter. However, there exist certain limitations to this study. The restricted accessibility of literature in this domain curtails the outcomes of the quantitative analysis of the literature and may not entirely exemplify all facets of the research area (Esmaeilion et al., 2022; De Castro et al., 2024; Su et al., 2024). Furthermore, the design of the software presents challenges in depicting recently published high-level literature in visual analysis outputs in comparison to older studies. Future enhancements in these realms will be crucial for augmenting the accuracy of trend forecasting (Azari et al., 2024; Liu C. et al., 2024; Jiang et al., 2024).
In recent years, the research direction of Energy Storage Systems (ESS), Renewable Energy Sources (RES), and Energy Efficiency Systems (EES) has undergone significant changes, reflecting the evolving priorities and technological advancements in the field. The integration of RES into existing power grids has highlighted the critical role of ESS in ensuring grid stability and reliability. This integration necessitates advancements in EMS designed for BESS and the implementation of distributed mobile energy operation control methods.
The trend analysis indicates a growing focus on deep learning and artificial intelligence applications to optimize the performance and efficiency of ESS. Researchers are increasingly exploring the use of machine learning algorithms to predict energy demand and supply, enhance battery management systems, and improve the overall efficiency of renewable energy systems.
Moreover, there is a notable shift towards enhancing the power quality and reliability of RES through advanced control strategies and optimization techniques. The development of hybrid systems combining multiple energy storage technologies is also gaining traction, offering a more resilient and efficient solution for renewable energy integration.
Looking forward, the future research directions in this domain are expected to focus on several key areas:
1) Enhancing ESS Efficiency and Reliability: Continued research into advanced battery technologies, including solid-state batteries and flow batteries, is essential to improve the energy density, lifespan, and safety of ESS.
2) Integration of AI and Machine Learning: The application of AI and machine learning in energy management and control systems will be crucial for optimizing the performance of ESS and RES, enabling smarter and more adaptive energy systems.
3) Development of Hybrid Energy Storage Solutions: Exploring the synergy between different energy storage technologies will provide more flexible and robust solutions for balancing energy supply and demand.
4) International Collaboration and Knowledge Sharing: Strengthening international research collaborations will facilitate the sharing of knowledge, resources, and best practices, accelerating the development and deployment of innovative energy solutions.
We address the issue of understanding the development process and knowledge frontiers of power systems and new energy research from 2014 to 2023. The conclusions are given as follows:
1) Significant International Collaboration: The study reveals extensive international cooperation, with major contributions from countries such as China, the United States, and the United Kingdom. Leading institutions like North China Electric Power University and China Electric Power Research Institute play pivotal roles in advancing research.
2) Key Research Areas: The analysis identifies critical research areas including Energy Management Systems (EMS) for Battery Energy Storage Systems (BESS), distributed mobile energy operation control methods, and the broader implications for power system stability and efficiency. These areas are essential for the effective integration of renewable energy sources into power systems.
3) Emerging Trends and Shifting Focus: Recent years have seen a shift towards topics such as deep learning, power quality, and renewable energy integration. These emerging trends reflect the evolving priorities and technological advancements in the field, highlighting the dynamic nature of power systems research.
4) Future Research Directions: The study provides a roadmap for future investigations, emphasizing the need to enhance the efficiency and reliability of Energy Storage Systems (ESS). It calls for greater international collaboration and the exploration of new technologies and methods for renewable energy integration. Future researchers are encouraged to build on these findings to address the identified gaps and push the boundaries of current knowledge.
By achieving these objectives, this study offers a comprehensive overview of the current state and future prospects of power systems research, emphasizing the critical role of ESS in the transition to renewable energy. The insights provided serve as a strategic guide for future research and development efforts, ensuring that the most critical and impactful areas receive the necessary attention and resources to advance sustainable energy systems.
In this study, we exclusively utilized the main Web of Science database for quantitative analysis. The search terms were limited to the subject part of the content. Although we made efforts to ensure literature saturation and conducted a thorough review during literature selection, it is possible that certain gaps in the literature still exist. Consequently, some relevant literature might not have been included in our search. It is important to acknowledge that the results obtained from this study may be subjective.
BD: Conceptualization, Data curation, Formal Analysis, Funding acquisition, Investigation, Methodology, Project administration, Resources, Software, Supervision, Validation, Visualization, Writing–original draft, Writing–review and editing. ZG: Conceptualization, Funding acquisition, Investigation, Methodology, Resources, Software, Writing - original draft. AM: Data curation, Funding acquisition, Project administration, Writing–review and editing YT: Funding acquisition, Investigation, Resources, Writing–review and editing. ML: Data curation, Funding acquisition, Writing–review and editing. YY: Funding acquisition, Visualization, Writing–review and editing. XL: Conceptualization, Funding acquisition, Investigation, Methodology, Project administration, Resources, Software, Supervision, Writing–review and editing.
The author(s) declare that financial support was received for the research, authorship, and/or publication of this article.
The authors are grateful to the editors and reviewers for their helpful comments and suggestions.
The authors declare that the research was conducted in the absence of any commercial or financial relationships that could be construed as a potential conflict of interest.
All claims expressed in this article are solely those of the authors and do not necessarily represent those of their affiliated organizations, or those of the publisher, the editors and the reviewers. Any product that may be evaluated in this article, or claim that may be made by its manufacturer, is not guaranteed or endorsed by the publisher.
Ali, A., David, R., and Mahmoud, K. (2016). Adaptive multi-objective optimization for power loss minimization and voltage regulation in distribution systems. in Proc. 2016 Eighteenth Int. Middle East Power Syst. Conf. MEPCON, 573–578.
AlKuwaiti, R. H., El-Sayed, W. T., Farag, H. E. Z., Al-Durra, A., and El-Saadany, E. F. (2024). Power system resilience against climatic faults: an optimized self-healing approach using conservative voltage reduction. Int. J. Electr. Power & Energy Syst. 155, 109519. doi:10.1016/j.ijepes.2023.109519
Al-Rawashdeh, H., Al-Khashman, O. A., Al Bdour, J. T., Gomaa, M. R., Rezk, H., Marashli, A., et al. (2023). Performance analysis of a hybrid renewable-energy system for green buildings to improve efficiency and reduce GHG emissions with multiple scenarios. Sustainability 15, 7529. doi:10.3390/su15097529
Anderson, G. O., and Grover, H. K. (2003). “Energy efficient design of an office building: a case study, Power and Energy Systems,” in Proceedings, 7th IASTED int. Multi-conf. On power and energy syst., 191–197.
Antoniotti, M., Bader, G. D., Caravagna, G., Crippa, S., Graudenzi, A., and Mauri, G. (2013). GeStoDifferent: a Cytoscape plugin for the generation and the identification of gene regulatory networks describing a stochastic cell differentiation process. Bioinformatics 29, 513–514. doi:10.1093/bioinformatics/bts726
Attiratanasunthron, N., and Fakcharoenphol, J. (2008). A running time analysis of an Ant Colony Optimization algorithm for shortest pathsin directed acyclic graphs. Inf. Process. Lett. 105, 88–92. doi:10.1016/j.ipl.2007.08.013
Awad, A. S. A., El-Fouly, T. H. M., and Salama, M. M. A. (2014). Optimal distributed generation allocation and load shedding for improving distribution system reliability. Electr. Power Compon. Syst. 42 (6), 576–584. doi:10.1080/15325008.2014.880962
Azari, M., Show, P. L., Du, R., and Huang, X. (2024). Editorial: advanced biotechnologies towards energy-efficient wastewater treatment plants. Front. Microbiol. 15, 1407478. doi:10.3389/fmicb.2024.1407478
Azizi, N., Esmaeilion, F., Moosavian, S. F., Yaghoubirad, M., Ahmadi, A., Aliehyaei, M., et al. (2022). Critical review of multigeneration system powered by geothermal energy resource from the energy, exergy, and economic point of views. Environ. Sci. Ecol. 10, 4859–4889. doi:10.1002/ese3.1296
Bai, L., Wang, J., Wang, C., Chen, C., and Li, F. (2018). Distribution locational marginal pricing (DLMP) for congestion management and voltage support. IEEE Trans. Power Syst. 33, 4061–4073. doi:10.1109/TPWRS.2017.2767632
Barth, D., Mautor, T., Watel, D., and Weisser, M. (2023). Configuring an heterogeneous smartgrid network: complexity and approximations for tree topologies. Arxiv 89, 223–257. doi:10.1007/s10898-023-01338-0
Bjornbet, M. M., Skaar, C., Fet, A. M., and Schulte, K. O. (2021). Circular economy in manufacturing companies: a review of case study literature. J. Clean. Prod. 294, 126268. doi:10.1016/j.jclepro.2021.126268
Brenna, M., De Berardinis, E., Carpini, L. D., Foiadelli, F., Paulon, P., Petroni, P., et al. (2013). Automatic distributed voltage control algorithm in smart grids applications. IEEE Trans. Smart Grid 4 (2), 877–885. doi:10.1109/TSG.2012.2206412
Chen, B., Chen, C., Wang, J. H., and Butler-Purry, K. L. (2018). Sequential service restoration for unbalanced distribution systems and microgrids. IEEE Trans. Power Syst. 33, 1507–1520. doi:10.1109/TPWRS.2017.2720122
Chen, C. (2006). CiteSpace II: detecting and visualizing emerging trends and transient patterns in scientific literature. J. Am. Soc. Inf. Sci. Technol. 57, 359–377. doi:10.1002/asi.20317
Chen, C. (2018a). “Visualizing and exploring scientific literature with CiteSpace: an introduction to a half-day tutorial,” in Proceedings of ACM CHIIR conference (CHIIR18). New Brunswick, NJ, USA, March 11-15, 2018 Association for Computing Machinery Inc, 2.
Chen, C. M. (2018b). Visualizing and exploring scientific literature with CiteSpace. CHIIR'18 Proc. 2018 Conf. Hum. Inf. Interact. Retr., 369–370. doi:10.1145/3176349.3176897
Chen, H., Zhang, H., Dai, Q., Wang, W., and Wang, M. (2022). A low-carbon planning model for regional power systems with generation-load-storage coordination considering new energy resources' consumption. Math. Probl. Eng. 2022, 1–15. doi:10.1155/2022/6071901
Cheng, W. S., and Lv, H. Q. (2021). “Research on cloud evaluation of rural e-commerce development level in China,” in 2021 2nd international conference on E-commerce and internet technology (ECIT 2021), 200–205.
Chin, C. H., Chen, S. H., Wu, H. H., Ho, C. W., Ko, M. T., and Lin, C. Y. (2014). cytoHubba: identifying hub objects and sub-networks from complex interactome. BMC Syst. Biol. 8, S11. doi:10.1186/1752-0509-8-s4-s11
Connell, A.O', Smith, J., and Keane, A. (2017). “Distribution feeder hosting capacity analysis,” in 2017 IEEE PES innovative Smart grid technologies conference Europe (ISGT-Europe). IEEE.
De Castro, C., Resende, E. M., Prast, A. E., and Abreu, F. (2024). Nanotechnology boosts the production of clean energy via nanoparticle addition in anaerobic digestion. Front. Nanotechnol. 6. doi:10.3389/fnano.2024.1406344
Demiroren, A., and Yilmaz, U. (2010). Analysis of change in electric energy cost with using renewable energy sources in Gokceada, Turkey: an island example. Renew. Sustain. Energy Rev. 14, 323–333. doi:10.1016/j.rser.2009.06.030
Ding, X., and Yang, Z. (2022). Knowledge mapping of platform research: a visual analysis using VOSviewer and CiteSpace. Electron. Commer. Res. 22, 787–809. doi:10.1007/s10660-020-09410-7
Du, C. Y., Lv, Y. C., Cao, J., Zhu, H., Zhang, Y., Zou, Y. L., et al. (2023). Removal of oxytetracycline from water by S-doped MIL-53(Fe): synergistic effect of surface adsorption and persulfate activation. Environ. Res. 239, 116842. doi:10.1016/j.envres.2023.116842
Du, Y., and Mohammadi, J. (2023). Equitable coordination in multi-agent power systems: impacts of computation granularity. arXiv:2311.12190.
Dumitrascu, M., Grigorescu, I., Micu, D., Mocanu, I., Vrinceanu, A., Mitrica, B., et al. (2019). Renewable energy, climate change and environmental challenges in Romania. Proc. IEEE PES Innov. Smart Grid Technol. Eur., 419. doi:10.1109/ISGTEurope.2019.8905552
Esmaeilion, F., Soltani, M., and Nathwani, J. (2022). Assessment of a novel solar-powered polygeneration system highlighting efficiency, exergy, economic and environmental factors. Desalination 540, 116004. doi:10.1016/j.desal.2022.116004
Freeman, L. C. (1977). A set of measures of centrality based on betweenness. Sociometry 40, 35–41. doi:10.2307/3033543
Galván, I. M., Huertas-Tato, J., Rodríguez-Benítez, F. J., Arbizu-Barrena, C., Pozo-Vázquez, D., and Aler, R. (2021). Evolutionary-based prediction interval estimation by blending solar radiation forecasting models using meteorological weather types. Appl. Soft Comput. 109, 107531. doi:10.1016/j.asoc.2021.107531
Gao, M., Wei, Y., He, Y., Zhang, D., Tian, Y., Huang, B., et al. (2023). Fuzzy controller-based design and simulation of an automatic parking system. J. Softw. Eng. Appl. 16 (9), 505–520. doi:10.4236/jsea.2023.169025
Geissdoerfer, M., Savaget, P., Bocken, N. M. P., and Hultink, E. J. (2017). The Circular Economy: a new sustainability paradigm? J. Clean. Prod. 143, 757–768. doi:10.1016/j.jclepro.2016.12.048
Geng, Y., Zhang, N., and Zhu, R. (2023). Research progress analysis of sustainable smart grid based on CiteSpace. J. Energy Strategy Rev. 48, 101111. doi:10.1016/j.esr.2023.101111
Ghosh, S. K., and Mount, D. M. (1991). An output-sensitive algorithm for computing visibility graphs. SIAM J. Comput. 20, 888–910. doi:10.1137/0220055
Gu, S. H., Xu, W. H., Xi, K. L., Luo, A. X., Fan, K. Q., and Wang, F. (2024). High-performance piezoelectric energy harvesting system with anti-interference capability for smart grid monitoring. Renew. Energy 221, 119742. doi:10.1016/j.renene.2023.119742
Gu, S. Z., Geng, M. Y., and Lan, L. (2021). Attention-based fault-tolerant approach for multi-agent reinforcement learning systems. Entropy 23 (9), 1133. doi:10.3390/e23091133
Guo, E. Y., He, B., and Zhang, J. L. (2023). Effects of photovoltaic panel type on optimum sizing of an electrical energy storage system using a stochastic optimization approach. J. Energy Storage 72, 108581. doi:10.1016/j.est.2023.108581
Guo, J. L., Liu, Q., and Chen, E. Q. (2022). A deep reinforcement learning method for multimodal data fusion in action recognition. IEEE Signal Process. Lett. 29, 120–124. doi:10.1109/LSP.2021.3128379
Hoang, A. T., Pham, V. V., and Nguyen, X. P. (2021). Integrating renewable sources into energy system for smart city as a sagacious strategy towards clean and sustainable process. J. Clean. Prod. 305, 127161. doi:10.1016/j.jclepro.2021.127161
Huang, H., Li, Y. G., and Liu, H. Z. (2023). Collaborative optimization strategy of source-grid-load-storage considering dynamic time series complementarity of multiple storages. IET Gener. Transm. Distrib. 17 (18), 4007–4023. doi:10.1049/gtd2.12862
Huang, J. L., and McElroy, M. B. (2015). A 32-year perspective on the origin of wind energy in a warming climate. Renew. Energy 77, 482–492. doi:10.1016/j.renene.2014.12.045
Jiang, Y., Fan, M., and Fan, Y. (2024). Does energy transition policy enhance urban green innovation capabilities? a quasi-natural experiment based on China’s new energy demonstration city policy. Front. Environ. Sci. 12. doi:10.3389/fenvs.2024.1377274
Jindal, A., Kumar, N., and Singh, M. (2016). A data analytical approach using support vector machine for demand response management in smart grid. 2016 IEEE Power Energy Soc. General Meet. (PESGM). doi:10.1109/pesgm.2016.7741457
Jindal, A., Marnerides, A. K., Gouglidis, A., Mauthe, A., and Hutchison, D. (2019). Communication standards for distributed renewable energy sources integration in future electricity distribution networks. Proc. IEEE Int. Conf. Acoust. Speech Signal Process., 8390–8393. doi:10.1109/ICASSP.2019.8683149
Ke, L. X., Lu, C. C., Shen, R., Lu, T. T., Ma, B., and Hua, Y. P. (2020). Knowledge mapping of drug-induced liver injury: a scientometric investigation (2010-2019). Front. Pharmacol. 11, 842. doi:10.3389/fphar.2020.00842
Kurundkar, K. M., and Vaidya, G. A. (2023). Voltage control ancillary service through grid-connected microgrid, its pricing by optimized active power and reactive power management using IEHO-TOPSIS approach. J. Sci. Technol. Trans. Electr. Eng. 48, 229–250. doi:10.1007/s40998-023-00655-0
Lago, J., Sogancioglu, E., Suryanarayana, G., De Ridder, F., and De Schutter, B. (2019). Building day-ahead bidding functions for seasonal storage systems: a reinforcement learning approach. IFAC Pap. 52 (4), 488–493. doi:10.1016/j.ifacol.2019.08.258
Lei, Y., Zhou, S. P., Xia, Y. J., Hu, G., and Shu, X. (2014). Research on short-term load prediction including the photovoltaic system, energy develop. Pts 1-4. Adv. Mater. Res., 860–863. doi:10.4028/www.scientific.net/AMR.860-863.135
Li, D., Dai, F. M., Xu, J. J., and Jiang, M. D. (2020). Characterizing hotspots and frontier landscapes of diabetes-specific distress from 2000 to 2018: a bibliometric study. Biomed Res. Int. 2020, 8691451. doi:10.1155/2020/8691451
Li, H., Li, G., Wu, Y., Wang, Z., and Wang, J. (2016). Operation modeling of power systems integrated with large-scale new energy power sources. Energies 9, 810. doi:10.3390/en9100810
Li, J., Ma, X. Y., Liu, C. C., and Schneider, K. P. (2014). Distribution system restoration with microgrids using spanning tree search. IEEE Trans. Power Syst. 29, 3021–3029. doi:10.1109/TPWRS.2014.2312424
Li, X., and Wang, S. (2021). Energy management and operational control methods for grid battery energy storage systems. CSEE J. Power Energy Syst., 1026–1040. doi:10.17775/CSEEJPES.2019.00160
Liang, Y.-D., Li, Y., Zhao, J., Wang, X.-Y., Zhu, H.-Z., and Chen, X.-H. (2017). Study of acupuncture for low back pain in recent 20 years: a bibliometric analysis via CiteSpace. J. Pain Res. 10, 951–964. doi:10.2147/JPR.S132808
Lin, Z. R., and Zhang, C. (2023). Current situation analysis of Chinese variable housing research based on CiteSpace analysis. J. Asian Archit. Build. Eng., 1–18. doi:10.1080/13467581.2023.2292092
Linnenluecke, M. K., Marrone, M., and Singh, A. K. (2020). Conducting systematic literature reviews and bibliometric analyses. Aust. J. Manag. 45, 175–194. doi:10.1177/0312896219877678
Liu, C., Wang, C., Yang, S., Wang, W., Zhao, L., and Li, Q. (2024b). Catalyst or obstacle? Green innovation and total factor energy efficiency. Front. Environ. Sci. 12. doi:10.3389/fenvs.2024.1397462
Liu, D., Li, G. Y., Wu, X. Z., Wang, J., Hu, A. J., Yan, Q. D., et al. (2023a). Comparative analysis of heating characteristics of convective-radiant systems using various terminal air source heat pumps. Energy Build. 301, 113701. doi:10.1016/j.enbuild.2023.113701
Liu, J., Zhao, S., Li, Y., and Sun, Z. (2023b). Development of an interval double-stochastic carbon-neutral electric power system planning model: a case study of Fujian province, China. J. Clean. Prod. 425, 138877. doi:10.1016/j.jclepro.2023.138877
Liu, Q. P., Dereli, R. K., Flynn, D., and Casey, E. (2021b). Demand response through reject water scheduling in water resource recovery facilities: a demonstration with BSM2. Water Res. 188, 116516. doi:10.1016/j.watres.2020.116516
Liu, X., Sun, Y., Xu, H., Jia, P., Wang, S., Dong, L., et al. (2016). Combining scientometrics with patent-metrics for CTI service in R&D decision-making: practices of national science library of CAS. Anticip. Future Innov. Pathw. Through Large Data Anal., 321–339. doi:10.1007/978-3-319-39056-7_17
Liu, Z. C., Peng, D. G., Yao, J., and Zhu, J. F. (2021a). Economic analysis and research on investment return of energy storage participating in thermal power peak and frequency modulation. 2021 Power Syst. Green Energy Conf. (PSGEC), 373–378. doi:10.1109/PSGEC51302.2021.9542156
Liu, Z. M., Li, M. Q., Virguez, E., and Xie, X. M. (2024a). Low-carbon transition pathways of power systems for Guangdong-Hongkong-Macau region in China. Energy & Environ. Sci. 17 (1), 307–322. doi:10.1039/d3ee02181e
Lu, N., Wang, G. Y., Su, C. G., Ren, Z. M., Peng, X. Y., and Sui, Q. (2024). Medium- and long-term interval optimal scheduling of cascade hydropower-photovoltaic complementary systems considering multiple uncertainties. Appl. Energy 353, 122085. doi:10.1016/j.apenergy.2023.122085
Lu, X., Xia, S. W., Sun, G. Z., Hu, J. J., Zou, W. W., Zhou, Q., et al. (2021). Hierarchical distributed control approach for multiple on-site DERs coordinated operation in microgrid. Int. J. Electr. Power & Energy Syst. 129, 106864. doi:10.1016/j.ijepes.2021.106864
Maghrabie, H. M., Olabi, A. G., Rezk, A., Radwan, A., Alami, A. H., and Abdelkareem, M. A. (2023). Energy storage for water desalination systems based on renewable energy resources. Energies 16, 3178. doi:10.3390/en16073178
Modrák, M., and Vohradsky, J. (2018). Genexpi: a toolset for identifying regulons and validating gene regulatory networks using time-course expression data. BMC Bioinforma. 19, 137. doi:10.1186/s12859-018-2138-x
Muratori, M., and Rizzoni, G. (2016). Residential demand response: dynamic energy management and time-varying electricity pricing. IEEE Trans. Power Syst. 31, 1108–1117. doi:10.1109/TPWRS.2015.2414880
Newman, M. E. J. (2003). The structure and function of complex networks. SIAM Rev. 45, 167–256. doi:10.1137/S003614450342480
Park, K. W., Kim, J. B., and Lee, D. Z. (2012). Applying the DC distribution system constructed commercial buildings. in Int. Conf. Renew. Energy Res. Appl. (ICRERA).
Qian, H. C., Zhang, F., and Qiu, B. (2023). Deciphering the evolution, frontier, and knowledge clustering in sustainable city planning: a 60-year interdisciplinary review. Sustainability 15 (24), 16854. doi:10.3390/su152416854
Qin, Z. J., Chen, X. W., Hou, Y. H., Liu, H., and Yang, Y. D. (2022). Coordination of preventive, emergency and restorative dispatch in extreme weather events. IEEE Trans. Power Syst. 37 (4), 2624–2638. doi:10.1109/TPWRS.2021.3123247
Quilumba, F. L., Lee, W. J., Huang, H., Wang, D. Y., and Szabados, R. L. (2015). Using smart meter data to improve the accuracy of Intraday load forecasting considering customer behavior Similarities. IEEE Trans. Smart Grid 6, 911–918. doi:10.1109/TSG.2014.2364233
Sabe, M., Pillinger, T., Kaiser, S., Chen, C., Taipale, H., Tanskanen, A., et al. (2022). Half a century of research on antipsychotics and schizophrenia: a scientometric study of hotspots nodes bursts and trends. Neurosci. Biobehav. Rev. 136, 104608. doi:10.1016/j.neubiorev.2022.104608
Schneider, K. P., Mather, B. A., Pal, B. C., Ten, C.-W., Shirek, G. J., Zhu, H., et al. (2018). Analytic considerations and design basis for the IEEE distribution test feeders. IEEE Trans. Power Syst. 33, 3181–3188. doi:10.1109/TPWRS.2017.2760011
Shi, M. F., Li, X. M., and Xu, C. B. (2024). Two-stage site selection of hydrogen refueling stations coupled with gas stations considering cooperative effects based on the CRITIC-ITFAHP-MABAC method: a case study in Beijing. Int. J. Hydrogen Energy 49, 1274–1292. doi:10.1016/j.ijhydene.2023.09.168
Shukla, S., Hussain, S., Irshad, R. R., Alattab, A. A., Thakur, S., Breslin, J. G., et al. (2024). Network analysis in a peer-to-peer energy trading model using blockchain and machine learning. Comput. Stand. & Interfaces 88, 103799. doi:10.1016/j.csi.2023.103799
Su, C., Shen, J., Feng, Z. K., Fattah, I. M. R., and Xiong, Y. (2024). Editorial: key technologies for hybrid energy system planning and operation. Front. Energy Res. 12. doi:10.3389/fenrg.2024.1413951
Sun, H., Guo, Q., Qi, J., Ajjarapu, V., Bravo, R., Chow, J., et al. (2019). Review of challenges and research opportunities for voltage control in smart grids. IEEE Trans. Power Syst. 34, 2790–2801. doi:10.1109/TPWRS.2019.2897948
Synnestvedt, M. B., Chen, C., and Holmes, J. H. (2005). CiteSpace II: visualization and knowledge discovery in bibliographic databases. AMIA Annu. Symp. Proc. AMIA Symp. 2005, 724–728.
Tao, L., Kong, S. Z., He, L. Z., Zhang, F., Li, X. H., Jia, T., et al. (2022). A sequential-path tree-based centrality for identifying influential spreaders in temporal networks. Chaos Solit. Fractals 165, 112766. doi:10.1016/j.chaos.2022.112766
Tong, S., Fung, T., Klein, M. P., Weisbach, D. A., and Park, J. W. (2017). Demonstration of reusing electric vehicle battery for solar energy storage and demand side management. J. Energy Storage 11, 200–210. doi:10.1016/j.est.2017.03.003
Tushar, W., Yuen, C., Saha, T. K., Morstyn, T., Chapman, A. C., Alam, M. J. E., et al. (2021). Peer-to-peer energy systems for connected communities: a review of recent advances and emerging challenges. Appl. Energy 282, 116131. doi:10.1016/j.apenergy.2020.116131
Verma, T., Tiwana, A. P. S., Reddy, C. C., Arora, V., and Devanand, P. (2016). Data analysis to generate models based on neural network and regression for solar power generation forecasting. in 2016 7th Int. Conf. Intelligent Syst, Model. Simul. (ISMS), 97–100.
Wang, C., Wang, X. R., Long, X. S., Xia, D. M., Ben, D. F., and Wang, Y. (2020). Publication trends of research on acute lung injury and acute respiration distress syndrome during 2009-2019: a 10-year bibliometric analysis. Am. J. Transl. Res. 12, 6366–6380. doi:10.1155/2020/8691451
Wang, H., and Liu, H. B. (2023). Enhanced electrical energy storage performance under low electric fields in Bi(Mg2/3Nb1/3)O3-modified 0.76Bi0.5Na0.5TiO3-0.24SiTiO3 ceramics. J. Mater. Sci. Mater. Electron. 34, 659. doi:10.1007/s10854-023-10071-z
Wang, J., Li, C., and Xia, C. Y. (2018). Improved centrality indicators to characterize the nodal spreading capability in complex networks. Appl. Math. Comput. 334, 388–400. doi:10.1016/j.amc.2018.04.028
Wang, J., Wang, Y., and Jiang, P. (2015). The study and application of a novel hybrid forecasting model - a case study of wind speed forecasting in China. Appl. Energy 143, 472–488. doi:10.1016/j.apenergy.2015.01.038
Wang, N., Zhou, X., Lu, X., Guan, Z., Wu, L., Du, X., et al. (2019a). When energy trading meets blockchain in electrical power system: the state of the art. Appl. Sci.-Basel 9, 1561. doi:10.3390/app9081561
Wang, S., and Chiang, H. D. (2016). Multi-objective service restoration of distribution systems using user-centered methodology. Int. J. Electr. Power & Energy Syst. 80 (80), 140–149. doi:10.1016/j.ijepes.2016.01.021
Wang, S., Ju, Z., and Zhang, D. (2023a). New energy power planning of photovoltaic power system for a smart city based on genetic algorithm. J. Test. Eval. 51, 1674–1685. doi:10.1520/JTE20220105
Wang, S. J., Liu, C., Liang, K., Cheng, Z. Y., Kong, X., and Gao, S. (2022a). Wind speed prediction model based on improved VMD and sudden change of wind speed. Sustainability 14 (14), 8705. doi:10.3390/su14148705
Wang, S. J., Zhang, D., and Ju, Z. H. (2023b). New energy power planning of photovoltaic power system for a smart city based on genetic algorithm. J. Test. Eval. 51 (3), 1674–1685. doi:10.1520/JTE20220105
Wang, S. Y., Luo, L. Y., Wu, A. P., Wang, D. X., Wang, L., Jiao, Y. Q., et al. (2024). Recent advances in tailoring zeolitic imidazolate frameworks (ZIFs) and their derived materials based on hard template strategy for multifunctional applications. Coord. Chem. Rev. 498, 215464. doi:10.1016/j.ccr.2023.215464
Wang, T. Q., Sun, L. M., Hao, L. X., Guo, Y., Zhang, Y., and Feng, X. (2021). Visualized analysis of research hotspots in acupuncture-neuro immunoregulation based on bibliometrics. Acupunct. Electro-Therapeutics Res. 46, 259–270. doi:10.3727/036012921X16281724938078
Wang, W. Z., Li, Y. X., Dong, X. Y., Chang, H., Yang, J. T., Ma, X. P., et al. (2022b). Study on the typical business model of clean energy utilization system based on flexible resources. in IEEE/IAS Ind. Com. Power Syst. Asia (I and CPS Asia 2022), 1277–1282.
Wang, X., Chang, J., Meng, X., and Wang, Y. (2019b). Hydro-thermal-wind-photovoltaic coordinated operation considering the comprehensive utilization of reservoirs. Energy Convers. Manag. 198, 111824. doi:10.1016/j.enconman.2019.111824
Wang, Y., Chen, C., Wang, J., and Baldick, R. (2016). Research on resilience of power systems under natural disasters: a review. IEEE Trans. Power Syst. 31, 1604–1613. doi:10.1109/TPWRS.2015.2429656
Wang, Z. Y., and Wang, J. H. (2015). Self-healing resilient distribution systems based on sectionalization into microgrids. IEEE Trans. Power Syst. 30, 3139–3149. doi:10.1109/TPWRS.2015.2389753
Wanik, M. Z. C., Ibrahim, A. A., Shareef, H., and Huat, T. J. (2015). Microgrid operation for a low voltage network with renewable energy sources for losses minimisation and voltage control. in 2015 Australas. Univ. Power Eng. Conf. (AUPEC).
Wei, Y., Gao, M., Xiao, J., Liu, C., Tian, Y., and He, Y. (2023a). Research and implementation of traffic sign recognition algorithm model based on machine learning. J. Softw. Eng. Appl. 16 (06), 193–210. doi:10.4236/jsea.2023.166011
Wei, Y., Zhang, D., Gao, M., Tian, Y., He, Y., Huang, B., et al. (2023b). Breast cancer prediction based on machine learning. J. Softw. Eng. Appl. 16, 348–360. doi:10.4236/jsea.2023.168018
Wen, G. H., Hu, G. Q., Hu, J. Q., Shi, X. L., and Chen, G. R. (2016). Frequency regulation of source-grid-load systems: a compound control strategy. IEEE Trans. Ind. Inf. 12, 69–78. doi:10.1109/TII.2015.2496309
Wu, H., Liu, K., Zhou, C., and Lu, Y. C. (2021b). “Research on line loss improvement of station area in distribution network by modular photovoltaic energy storage system,” in 2021 IEEE IAS ind. And com. Power syst. Asia (IEEE I&CPS Asia), 1285–1289.
Wu, H. Y., Zhou, Y., Wang, Y. L., Tong, L. J., Wang, F. C., Song, S. R., et al. (2021a). Current state and future directions of intranasal delivery route for central nervous system disorders: a scientometric and visualization analysis. Front. Pharmacol. 12, 717192. doi:10.3389/fphar.2021.717192
Xi, L., Chen, J., Huang, Y., Xu, Y., Liu, L., Li, Y., et al. (2018). Smart generation control based on multi-agent reinforcement learning with the idea of the time tunnel. Energy 153, 977–987. doi:10.1016/j.energy.2018.04.042
Xie, P. (2015a). Study of international anticancer research trends via co-word and document co-citation visualization analysis. Scientometrics 105 (1), 611–622. doi:10.1007/s11192-015-1689-0
Xie, P. (2015b). Study of international anticancer research trends via co-word and document co-citation visualization analysis. Scientometrics 105 (1), 611–622. doi:10.1007/s11192-015-1689-0
Xu, C., Wu, Y., and Dai, S. (2020). What are the critical barriers to the development of hydrogen refueling stations in China? A modified fuzzy DEMATEL approach. Energy Policy 142, 111495. doi:10.1016/j.enpol.2020.111495
Xu, D., Wang, Y. L., Wang, K. T., Wang, Y., Dong, X. R., Tang, J., et al. (2021). A scientometrics analysis and visualization of depressive disorder. Curr. Neuropharmacol. 19 (6), 766–786. doi:10.2174/1570159X18666200905151333
Yan, Q., Lu, Z. Y., Liu, H., He, X. T., Zhang, X. H., and Guo, J. L. (2024). Short-term prediction of integrated energy load aggregation using a bi-directional simple recurrent unit network with feature-temporal attention mechanism ensemble learning model. Appl. Energy 355, 122159. doi:10.1016/j.apenergy.2023.122159
Yang, B., Sang, B. Y., Yu, J. C., and Li, G. J. (2018). “Research on energy storage capacity configuration method based on demand response,” in 2018 China int Conf. on Electricity Distrib. (CICED), 2290–2294.
Yang, H., Tang, S. Q., Zeng, Z., He, X. M., Zhao, R. X., and Kuroda, M. (2013). Demand response of inverter air conditioners and applications in distribution system voltage stability enhancement. in 2013 Int. Conf. Elect. Mach. Syst. (ICEMS), 954–959.
Zeng, B., Zhang, J. H., Yang, X., Wang, J. H., Dong, J., and Zhang, Y. Y. (2014). Integrated planning for transition to low-carbon distribution system with renewable energy generation and demand response. IEEE Trans. Power Syst. 29, 1153–1165. doi:10.1109/TPWRS.2013.2291553
Zeyringer, M., Price, J., Fais, B., Li, P. H., and Sharp, E. (2018). Designing low-carbon power systems for Great Britain in 2050 that are robust to the spatiotemporal and inter-annual variability of weather. Nat. Energy 3, 395–403. doi:10.1038/s41560-018-0128-x
Zhang, J. H., Zhang, Y. X., Hu, L. Y., Huang, X. X., Liu, Y. F., Li, J. Y., et al. (2021). Global trends and performances of magnetic resonance imaging studies on acupuncture: a bibliometric analysis. Front. Neurosci. 14, 620555. doi:10.3389/fnins.2020.620555
Zhang, Y., Pan, G., Chen, B., Han, J., Zhao, Y., and Zhang, C. (2020). Short-term wind speed prediction model based on GA-ANN improved by VMD. Renew. Energy 156, 1373–1388. doi:10.1016/j.renene.2019.12.047
Zhang, Y. S., Lu, J., Chang, T. Y., Tang, X. L., Wang, Q., Pan, D., et al. (2024). A bibliometric review of Glycyrrhizae Radix et Rhizoma (licorice) research: Insights and future directions. J. Ethnopharmacol. 321, 117409. doi:10.1016/j.jep.2023.117409
Zheng, K., and Wang, X. (2019). Publications on the association between cognitive function and pain from 2000 to 2018: a bibliometric analysis using CiteSpace. Med. Sci. Monit. 25, 8940–8951. doi:10.12659/msm.917742
Zhou, Q., Tian, Z., Shahidehpour, M., Liu, X., Alabdulwahab, A., and Abusorrah, A. (2020). Optimal consensus-based distributed control strategy for coordinated operation of networked microgrids. IEEE Trans. Power Syst. 35 (3), 2452–2462. doi:10.1109/TPWRS.2019.2954582
Keywords: renewable energy, energy storage systems, energy efficiency improvements, scientometric analysis, CiteSpace visualization
Citation: Dong B, Guo Z, Mulat A, Tian Y, Lu M, Yuan Y and Liu X (2024) Leveraging heterogeneous networks to analyze energy storage systems in power systems and renewable energy research: a scientometric study. Front. Energy Res. 12:1424928. doi: 10.3389/fenrg.2024.1424928
Received: 28 April 2024; Accepted: 10 June 2024;
Published: 11 July 2024.
Edited by:
Farbod Esmaeilion, K.N.Toosi University of Technology, IranReviewed by:
Alireza Mahmoudan, K.N.Toosi University of Technology, IranCopyright © 2024 Dong, Guo, Mulat, Tian, Lu, Yuan and Liu. This is an open-access article distributed under the terms of the Creative Commons Attribution License (CC BY). The use, distribution or reproduction in other forums is permitted, provided the original author(s) and the copyright owner(s) are credited and that the original publication in this journal is cited, in accordance with accepted academic practice. No use, distribution or reproduction is permitted which does not comply with these terms.
*Correspondence: Xianchen Liu, eGxpdTA3M0BmaXUuZWR1, ZGJvd2UwMjFAZml1LmVkdQ==
†ORCID: Bowen Dong, orcid.org/0009-0004-5138-7441
‡These authors share first authorship
Disclaimer: All claims expressed in this article are solely those of the authors and do not necessarily represent those of their affiliated organizations, or those of the publisher, the editors and the reviewers. Any product that may be evaluated in this article or claim that may be made by its manufacturer is not guaranteed or endorsed by the publisher.
Research integrity at Frontiers
Learn more about the work of our research integrity team to safeguard the quality of each article we publish.