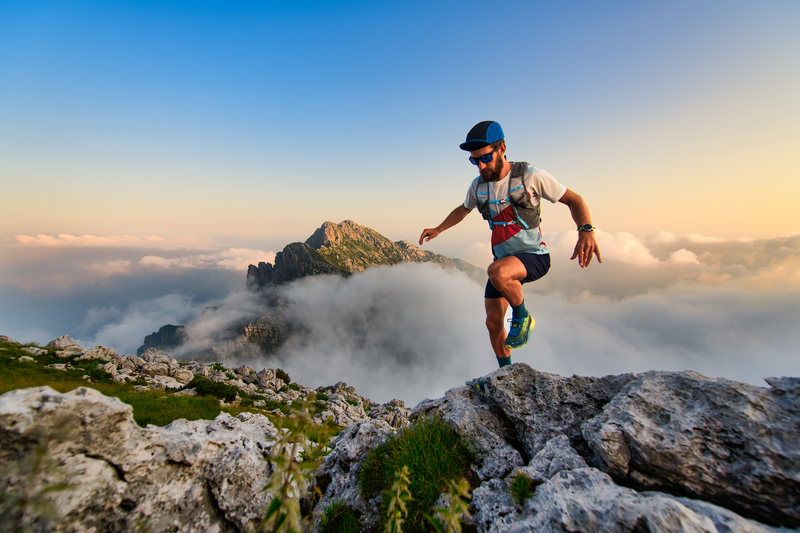
94% of researchers rate our articles as excellent or good
Learn more about the work of our research integrity team to safeguard the quality of each article we publish.
Find out more
OPINION article
Front. Energy Res. , 11 September 2024
Sec. Sustainable Energy Systems
Volume 12 - 2024 | https://doi.org/10.3389/fenrg.2024.1414068
This article is part of the Research Topic Emerging Technologies for the Construction of Renewable Energy-Dominated Power System View all 32 articles
As a typical spatial–temporal flexible resource, mobile energy storage can respond promptly to ensure uninterrupted power supply in case of life safety issues and economic loss due to the consequences of electricity outages (Sun et al., 2022; Sun et al., 2017; Chuangpishit et al., 2023). In addition to emergency power supply, mobile energy storage systems can also provide various ancillary services, including peak shaving and valley filling (Li et al., 2023; Manojkumar et al., 2021; Li X. et al., 2021), distributed renewable energy consumption (Li X. et al., 2021; Zhou et al., 2021; Zhong et al., 2023), and power quality management (Xiong et al., 2020; Cao et al., 2024; Naderi et al., 2021). Nevertheless, energy storage mostly stays in a standby state, thus failing to fully leverage its multiple spatiotemporal applications, leading to low utilization and long investment payback periods (Li et al., 2022). Moreover, the existing literature studies (Wang et al., 2023a; Yang, 2021; Wang et al., 2023b) on the research of power supply trading models mainly focus on the economic efficiency, and the presented charging standards of power supply are uniform. This results in customers with significantly different power supply demands paying the same electricity price, severely undermining customers’ enthusiasm for purchasing emergency supply services. Consequently, this paper aims to offer insightful opinions and discussions on a multi-grade pricing strategy for mobile energy storage systems providing emergency power supply services that meet the differentiated demands of customers.
The main contributions of this paper are twofold, as listed: (1) a hierarchical trading framework is presented for mobile energy storage systems to provide emergency power supply services, and three metrics, namely, power supply capacity, duration of power supply, and response time, are formulated to evaluate the reliability of emergency power supply. (2) A bi-level pricing optimization model based on Stackelberg game is proposed to obtain tiered prices of emergency power supply and customer purchase capacity of emergency power supply, thereby increasing the revenue of mobile energy storage and reducing the emergency power supply cost of customers.
Currently, there is no established pricing mechanism for MESS to provide emergency power supply services in China (Yang et al., 2023). Shang (2021) selected power supply duration as the standard to divide emergency power supply subsidy tariffs. Meanwhile, Yu (2015) suggested that the significance of energy storage in offering differentiated emergency power supply services is primarily demonstrated by the magnitude of emergency standby capacity and response speed. It is known that the higher the emergency power supply capacity and the longer the duration of the emergency power supply, the greater the investment cost of energy storage equipment will be. In addition, the mobile cost of energy storage increases with the amplification of response speed (Sun et al., 2022; Lei et al., 2019). Consequently, power supply capacity, duration of power supply, and response time were selected as the three metrics to delineate the classification of power supply levels in this paper. Based on the typical capacity of mobile energy storage and the historical downtime of customers (Xiao, 2021), three levels have been classified, with higher levels indicating a greater demand for emergency power supply (Zhu and Si, 2023; Zhang et al., 2021). This is shown in detail in Figure 1A, where H1 represents the lowest power supply level, while H3 represents the highest power supply level. Hence, the unit price of emergency power supply
where
Figure 1. Multi-grade pricing strategy for emergency power supply of mobile energy storage. (A) Hierarchical trading framework of the mobile energy storage. (B) Pricing model based on Stackelberg game.
According to the analysis of the interactive mechanism between energy storage and customers, the hierarchical trading framework for energy storage providing emergency power supply services is established, as depicted in Figure 1A. On one hand, mobile energy storage strategically sets electricity prices to maximize the benefits for emergency power supply, but on the other hand, power supply customers optimize the emergency power supply capacity to achieve the maximum utility during power outages. Therefore, it is a sequential decision process, thereby constituting a Stackelberg game relationship dominated by energy storage, which can be expressed as a bi-level mathematical optimization model (Sun et al., 2022; Xu et al., 2023). The upper level of this model aims to maximize the revenue of mobile energy storage providing emergency power supply services, considering the energy storage investment constraint, individual rational constraint, and premium pricing constraint. The lower level takes the purchase decision constraint into account to maximize the customer utility of emergency power supply. As the leader in this game relationship, mobile energy storage takes the initiative to make electricity price decisions. Meanwhile, customers functioning as followers in the model adapt their electricity purchase strategies in response to the pricing decisions. Subsequently, the leader revises the initial decision framework, creates new decisions, and shares them with all the followers. Then, the followers adjust their power supply strategy again based on the latest electricity prices. This iterative process continues until equilibrium is achieved, resulting in optimal tiered pricing of emergency power supply and optimal power supply capacity purchase strategies of customers.
(a) The utility function of customers purchasing emergency power supply considering outage loss.
The utility function of emergency power supply on the customer’s side is determined by calculating the difference between the reduced economic losses after choosing the power supply service and the emergency power supply service fee paid, which is formulated as Equation 2:
where
where
(b) The income function of mobile energy storage providing emergency power supply services.
Mobile energy storage is typically kept in a standby state, only being utilized to provide an emergency power supply in the event of a power outage (Cao et al., 2024; Jiang et al., 2021). Considering energy storage resource reuse strategies to enhance its capacity utilization efficiency, the standby capacity for emergency power supply can be used for peak–valley arbitrage and distributed renewable energy consumption in electricity trading to maximize revenue (Li et al., 2023) (Zhong et al., 2023). Therefore, the income function of mobile energy storage is composed of the emergency power supply service income, peak–valley arbitrage income, distributed renewable energy consumption income, and power supply service cost, which is formulated as Equation 5:
where
where U is the number of customers; D is the annual operating days of energy storage;
The multi-grade pricing of a mobile energy storage system is designed as a one-leader-multi-follower bi-level optimization problem in Figure 1B, where the mobile energy storage is the leader in the upper-level problem and the multi-type customers are the followers in the lower-level problem (Ding et al., 2023). In the upper-level problem, the energy storage aims to maximize its operating income by optimizing the expected revenue from emergency power supply services, with multi-grade electricity price (
(a) Upper-level problem: maximize the benefits of energy storage for emergency power supply, which can be defined in Equations 10–13.
Constraints (11)–(13) are the energy storage investment constraint, individual rational constraint, and premium pricing constraint, respectively. Here,
(b) Lower-level problem: maximize the utility of emergency power supply for customers, which can be defined in Equations 14, 15.
where
It is evident that the objective function involves the multiplication of decision variables, but a bi-level nonlinear optimization problem cannot be directly solved by the available commercial solver. Since the objective functions for energy storage and customers are all in the linear space for their decision variables, Karush–Kuhn–Tucker (KKT) theory is used to transform the lower-level problem into constraints of the upper-level problem (Zhou et al., 2023). Therefore, the bi-level multi-grade pricing problem can be converted into the game equilibrium single-level MILP model (Chuangpishit et al., 2023), which can be optimized by the Gurobi solver based on MATLAB.
Since the maximum power supply capacity standard is 250 kW in Section 2, this paper selects the lithium iron phosphate batteries as the energy storage battery, with an energy storage inverter capacity of 250 kW. The cost of the energy storage vehicle body is 150,000 yuan, with an annual labor cost of 100,000 yuan (Gong et al., 2022). Basic parameters and other energy storage parameters are explained in Fang et al. (2023). The scenario considers 20 emergency power supply customers, and parameters for power supply customers are referenced in the literature (Wang et al., 2023b; Fang et al., 2023; Chen, 2015; Li J. et al., 2021). In order to evaluate the effectiveness of the multi-grade pricing method for emergency power supply of mobile energy storage, this paper designs three cases to conduct a comparative analysis of energy storage economics. Case 1 is the multi-grade pricing strategy proposed in this paper. In case 2, mobile energy storage provides emergency power supply without considering grading. In case 3, energy storage only engages in peak–valley arbitrage and distributed renewable energy consumption, and customers independently configure energy storage as backup power at their own expense in case of blackout. Furthermore, based on the parameters provided above, calculations are performed according to formulas 4 to 8, and the results of the economic benefits (Fang et al., 2023) of mobile energy storage under different cases are shown in Table 1.
Compared with cases 2 and 3, the total revenue of case 1 is the highest, while the total cost is the highest in Table 1, as the increased revenue of emergency power supply in case 1 far outweighs the increase in cost. This demonstrates that emergency power supply services can significantly increase the annual operating income of energy storage and that emergency power supply services provided by energy storage have strong commercial prospects.
Mobile energy storage plays a crucial role in peak shaving and valley filling, distributed renewable energy consumption, and power quality management, especially in ensuring the reliability of power supply. In this paper, a comprehensive overview of the multi-grade pricing strategy for emergency power supply of the mobile energy storage system is conducted. The key findings of this paper can be summarized as follows: 1) the hierarchical pricing metrics of the emergency power supply service provided by mobile energy storage are proposed, which consist of the power supply capacity, power supply duration, and response time. These metrics achieve a precise delineation of emergency power supply levels, having specific significance for the formulation of customized power service grade standards in the future. 2) A bi-level pricing optimization model based on Stackelberg game is proposed to obtain optimal tiered pricing of emergency power supply and optimal power supply capacity purchase strategies of customers, achieving interest equilibrium between mobile energy storage and consumers.
Numerous studies (Wang et al., 2023a; Yang, 2021; Xiao, 2021; Jiang et al., 2021) have been conducted on the configuration of mobile energy storage. The power emergency center optimizes and schedules mobile energy storage based on customers’ demand for power supply within the emergency mechanism (Xiao, 2021). Therefore, combining the configuration of energy storage and the emergency power supply needs of customers within a certain region, the tiered pricing model proposed in this paper can be applied to calculate the electricity prices for different levels of emergency power supply. In the existing power market, energy storage can profit by providing multiple customized power services (Fang et al., 2023). Thus, according to the results of the emergency power supply price and customers’ demand for power supply, the emergency power supply service can be paid on a per-use basis.
During the process of mobile energy storage transporting to designated power supply points, the traffic network is highly complex. The multi-grade pricing model in this paper does not take into account real-time scheduling issues of mobile energy storage, only calculating the annual operational costs but ignoring daily or real-time operational conditions. The next step could integrate real-time scheduling of mobile energy storage into the multi-grade pricing model.
JB: writing–original draft and writing–review and editing. XG: conceptualization and writing–original draft. XY: data curation, formal analysis, and writing–review and editing.
The author(s) declare that financial support was received for the research, authorship, and/or publication of this article. This work was sponsored by the Guangdong Basic and Applied Basic Research Foundation (2023A1515110105) and the Scientific Research Startup Fund for Shenzhen High-Caliber Personnel of SZPT (No. 6022310042k).
The authors declare that the research was conducted in the absence of any commercial or financial relationships that could be construed as a potential conflict of interest.
All claims expressed in this article are solely those of the authors and do not necessarily represent those of their affiliated organizations, or those of the publisher, the editors, and the reviewers. Any product that may be evaluated in this article, or claim that may be made by its manufacturer, is not guaranteed or endorsed by the publisher.
Cao, Y., Zhou, B., Chung, C. Y., Wu, T., Ling, Z., and Shuai, Z. (2024). A coordinated emergency response scheme for electricity and watershed networks considering spatio-temporal heterogeneity and volatility of rainstorm disasters. IEEE Trans. Smart Grid 15, 3528–3541. doi:10.1109/TSG.2024.3362344
Chen, J. (2015). Research on reducing power loss of important power users in power distribution network. Telecom Power Technol. doi:10.19399/j.cnki.tpt.2015.06.011
Chuangpishit, S., Katiraei, F., Chalamala, B., and Novosel, D. (2023). Mobile energy storage systems: a grid-edge technology to enhance reliability and resilience. IEEE Power Energy Mag. 21, 97–105. doi:10.1109/MPE.2023.3246899
Ding, Y., Xu, Q., Hao, L., and Xia, Y. (2023). A stackelberg game-based robust optimization for user-side energy storage configuration and power pricing. Energy 283, 128429. doi:10.1016/j.energy.2023.128429
Fang, J., He, D., Pei, Z., Peng, Z., Bao, J., Liu, W., et al. (2023). A multi-grade pricing strategy for distributed energy storage considering default risks of customized power services. J. Shanghai Jiaot. Univ. doi:10.16183/j.cnki.jsjtu.2023.481
Gong, C., Wu, M., and Sun, L. (2022). Economic benefit analysis of mobile energy storage based on improved genetic algorithm. Electr. Eng. doi:10.19768/j.cnki.dgjs.2022.05.003
Jiang, X., Chen, J., Zhang, W., Wu, Q., Zhang, Y., and Liu, J. (2021). Two-step optimal allocation of stationary and mobile energy storage systems in resilient distribution networks. J. Mod. Power Syst. Clean Energy 9, 788–799. doi:10.35833/MPCE.2020.000910
Lei, S., Chen, C., Zhou, H., and Hou, Y. (2019). Routing and scheduling of mobile power sources for distribution system resilience enhancement. IEEE Trans. Smart Grid 10, 5650–5662. doi:10.1109/TSG.2018.2889347
Li, J., Han, J., Liu, X., Zhang, Y., Zhang, Y., Zhang, X., et al. (2021b) “Optimal capacity allocation of anti-disaster mobile power vehicles based on dynamic programming,” in 2021 IEEE 4th international Conference on renewable Energy and power engineering (REPE). doi:10.1109/REPE52765.2021.9617024
Li, J., Mu, G., Zhang, J., Li, C., Yan, G., Zhang, H., et al. (2023). Dynamic economic evaluation of hundred megawatt-scale electrochemical energy storage for auxiliary peak shaving. Prot. Control Mod. Power Syst. 8, 50. doi:10.1186/s41601-023-00324-8
Li, L., Cao, X., and Zhang, S. (2022). Shared energy storage system for prosumers in a community: investment decision, economic operation, and benefits allocation under a cost-effective way. J. Energy Storage 50, 104710. doi:10.1016/j.est.2022.104710
Li, X., Wang, L., Yan, N., and Ma, R. (2021a). Cooperative dispatch of distributed energy storage in distribution network with PV generation systems. IEEE Trans. Appl. Supercond. 31, 1–4. doi:10.1109/TASC.2021.3117750
Manojkumar, R., Kumar, C., Ganguly, S., and Catalão, J. P. S. (2021). Optimal peak shaving control using dynamic demand and feed-in limits for grid-connected PV sources with batteries. IEEE Syst. J. 15, 5560–5570. doi:10.1109/JSYST.2020.3045020
Naderi, E., K. C., B., Ansari, M., and Asrari, A. (2021). Experimental validation of a hybrid storage framework to cope with fluctuating power of hybrid renewable energy-based systems. IEEE Trans. Energy Convers. 36, 1991–2001. doi:10.1109/TEC.2021.3058550
Shang, B. (2021). Study on optimal configuration and operation method of user side energy storage. Beijing, China: Beijing Jiaotong University. doi:10.1016/j.ijepes.2022.108621
Sun, S., Yang, Q., and Yan, W. (2017). Optimal temporal-spatial PEV charging scheduling in active power distribution networks. Prot. Control Mod. Power Syst. 2, 34. doi:10.1186/s41601-017-0065-x
Sun, W., Liu, W., Zhang, J., and Tian, K. (2022). Bi-level optimal operation model of mobile energy storage system in coupled transportation-power networks. J. Mod. Power Syst. Clean Energy 10, 1725–1737. doi:10.35833/MPCE.2020.000730
Wang, F., Ou, Y., and Xin, X. (2023a) “Operation strategy analysis of power supply ensuring microgrid with photovoltaic and energy storage,” in Electric power automation equipment. doi:10.16081/j.epae.202210020
Wang, F., Ou, Y., and Xin, X. (2023b). Optimal configuration of user-side energy storage considering power supply transaction mode and capacity market. High. Volt. Eng. doi:10.13336/j.1003-6520.hve.20230205
Xiao, K. (2021). Mobile energy storage planning and scheduling under different scenario in distribution network. Hangzhou, China: Zhejiang University. doi:10.27461/d.cnki.gzjdx.2021.000453
Xiong, L., Liu, X., Zhao, C., and Zhuo, F. (2020). A fast and robust real-time detection algorithm of decaying DC transient and harmonic components in three-phase systems. IEEE Trans. Power Electron. 35, 3332–3336. doi:10.1109/TPEL.2019.2940891
Xu, C., Xu, C., Zhang, T., Si, L., Yang, J., Yu, H., et al. (2023). Stackelberg game-based three-stage optimal pricing and planning strategy for hybrid shared energy storage. Front. Energy Res. 11. doi:10.3389/fenrg.2023.1273929
Yang, T. (2021). Research on power boosting and operation strategy of charging pile based on distributed mobile energy storage. Beijing, China: Beijing Jiaotong University. doi:10.26944/d.cnki.gbfju.2021.001979
Yang, Z., Gao, T., Liu, Y., Huang, X., Gao, S., Zhao, Q., et al. (2023). A two-stage pricing strategy for electric vehicles participating in emergency power supply for important loads. Electr. Power Syst. Res. 218, 109239. doi:10.1016/j.epsr.2023.109239
Yu, L. (2015). Energy storage system service and value assessment in smart grid. Beijing, China: North China Electric Power University. doi:10.7666/d.Y2879251
Zhang, H., Ma, S., Ding, T., Lin, Y., and Shahidehpour, M. (2021). Multi-Stage multi-zone defender-attacker-defender model for optimal resilience strategy with distribution line hardening and energy storage system deployment. IEEE Trans. Smart Grid 12, 1194–1205. doi:10.1109/TSG.2020.3027767
Zhong, J., Li, Y., Cao, Y., Tan, Y., Peng, Y., Zhou, Y., et al. (2023). Robust coordinated optimization with adaptive uncertainty set for a multi-energy microgrid. IEEE Trans. Sustain. Energy 14, 111–124. doi:10.1109/TSTE.2022.3203299
Zhou, B., Fang, J., Ai, X., Cui, S., Yao, W., Chen, Z., et al. (2023). Storage right-based hybrid discrete-time and continuous-time flexibility trading between energy storage station and renewable power plants. IEEE Trans. Sustain. Energy 14, 465–481. doi:10.1109/TSTE.2022.3215839
Zhou, B., Zou, J., Chuang, C., Wang, H., Liu, N., Voropai, N., et al. (2021). Multi-microgrid energy management systems: architecture, communication, and scheduling strategies. J. Mod. Power Syst. Clean Energy 9, 463–476. doi:10.35833/MPCE.2019.000237
Keywords: bi-level optimization, emergency power supply, mobile energy storage, multi-grade pricing, Stackelberg game
Citation: Bao J, Gao X and Yan X (2024) Opinions on the multi-grade pricing strategy for emergency power supply of mobile energy storage systems. Front. Energy Res. 12:1414068. doi: 10.3389/fenrg.2024.1414068
Received: 08 April 2024; Accepted: 27 August 2024;
Published: 11 September 2024.
Edited by:
Anant Kumar Verma, Universidad de O’Higgins, ChileReviewed by:
Ziqing Zhu, Hong Kong Polytechnic University, Hong Kong, SAR ChinaCopyright © 2024 Bao, Gao and Yan. This is an open-access article distributed under the terms of the Creative Commons Attribution License (CC BY). The use, distribution or reproduction in other forums is permitted, provided the original author(s) and the copyright owner(s) are credited and that the original publication in this journal is cited, in accordance with accepted academic practice. No use, distribution or reproduction is permitted which does not comply with these terms.
*Correspondence: Xiang Gao, Z2FveGlhbmdAc3pwdS5lZHUuY24=
Disclaimer: All claims expressed in this article are solely those of the authors and do not necessarily represent those of their affiliated organizations, or those of the publisher, the editors and the reviewers. Any product that may be evaluated in this article or claim that may be made by its manufacturer is not guaranteed or endorsed by the publisher.
Research integrity at Frontiers
Learn more about the work of our research integrity team to safeguard the quality of each article we publish.