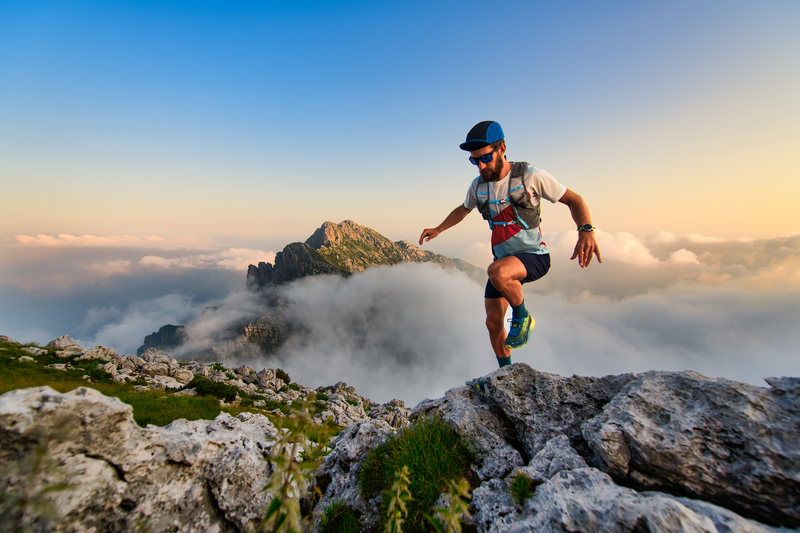
94% of researchers rate our articles as excellent or good
Learn more about the work of our research integrity team to safeguard the quality of each article we publish.
Find out more
EDITORIAL article
Front. Energy Res. , 06 May 2024
Sec. Process and Energy Systems Engineering
Volume 12 - 2024 | https://doi.org/10.3389/fenrg.2024.1413951
This article is part of the Research Topic Key Technologies for Hybrid Energy System Planning and Operation View all 10 articles
Editorial on the Research Topic
Key technologies for hybrid energy system planning and operation
This Research Topic addresses the growing demand for clean and reliable energy in the face of rising electricity consumption and environmental concerns. Traditional fossil fuels dominate power generation, but their accelerated use creates pollution problems. Wind and solar power offer promising renewable alternatives, but their widespread adoption presents challenges. These sources are highly variable and difficult to control due to their dependence on weather conditions. Large-scale integration of wind and solar power can destabilize power grids and threaten their safe operation.
The research presented here explores solutions for integrating these renewable sources effectively. A key approach involves combining wind and solar with controllable power sources like hydropower, thermal power, and battery storage to create hybrid energy systems. Accurate prediction of new energy power generation is crucial for such hybrid systems’ reliable and secure operation.
This collection features nine research articles investigating various aspects of hybrid energy systems:
• Techniques for controlling wind turbines
• Decision-making methods for markets dominated by hydropower
• Optimization models for regional energy integration
• Short-term wind power prediction models
• Decision-making methods for hydropower-dominated markets
The original research by Y. Wang et al. proposed a flexible torque control method for wind turbines participating in frequency regulation. It addresses the issue of transient torque fluctuations during wind speed transitions. The method divides the transition region between operating speeds into sub-regions. It then employs dynamic deloading and flexible torque control within these sub-regions to ensure smooth generator torque transfer. This is achieved without requiring additional sensors, making it simple to implement. The effectiveness of the proposed method is verified through simulations. The study demonstrates that this method can mitigate transient torque fluctuations and improve the overall reliability and efficiency of wind turbine systems.
The original research by Zeng et al. introduced a novel double-layer optimization model tailored for a regional integrated energy system (RIES), emphasizing the integration of concentrating solar power (CSP) stations, carbon emissions management, and integrated demand response (IDR). The primary objective is to minimize both annual planning costs and net pollutant emissions while ensuring optimal system operation. The model operates on two distinct layers: the upper layer employs an enhanced NSGA-II algorithm to configure capacities, focusing on minimizing annual planning costs and net emissions, while the lower layer utilizes a mixed-integer linear programming (MILP) solver to minimize annual operating costs and optimize device output and load curves. Notably, the model encompasses the entire carbon emissions process, including capture, utilization, and trading, and incorporates flexible loads for electricity, heat, cooling, and gas. Simulation results, compared against conventional approaches, underscore several key advantages of the proposed methodology. CSP station integration is found to enhance coordinated optimization, while the improved NSGA-II algorithm demonstrates superior convergence over its conventional counterpart. Furthermore, considering the entirety of carbon emissions alongside IDR leads to reductions in annual operating costs and net carbon emissions. Overall, this innovative approach fosters a more economically viable and environmentally sustainable RIES.
The original research by Liu et al. presented a pioneering approach to coordinated scheduling for hydro-wind-solar power systems, taking into account both peak shaving and navigation objectives. With the rapid expansion of wind and solar energy, grid stability faces challenges due to their intermittent nature. Although hydropower offers flexibility, its conventional operation might clash with other functionalities like navigation. To tackle these challenges, the proposed model incorporates uncertainty quantification via a Gaussian mixture model for wind and solar power forecasts. This integration enables the model to effectively manage the inherent variability of these renewable sources. Additionally, the model utilizes the ε-constraint method to achieve Pareto optimality, effectively balancing the conflicting goals of peak shaving and navigation. Key contributions of this study include the development of a multi-objective hydro-wind-solar scheduling model that accounts for both peak shaving and navigation, uncertainty quantification of wind and solar power forecasts using a Gaussian mixture model, and the transformation of chance constraints into solvable linear constraints through error bounds. The model’s effectiveness is demonstrated through a real-world application on the Jinghong-Ganlanba cascade hydropower system, with further analysis conducted on the impact of wind and solar factors on its performance. This research provides valuable insights for power grid operators aiming to integrate renewable energy sources while upholding system reliability and addressing navigation requirements.
The methods paper by Wu et al. proposed a novel safety check method for the electricity spot market that optimizes tie-line power to maximize effective reserve resources within the power system. This approach aims to ensure the stability of the market and the safety of regional power grids. The key challenge addressed is the difficulty of accurately evaluating effective reserves in the current spot market environment. This is due to factors like limited real-time data and the impact of new energy sources with fluctuating power generation. The proposed method tackles this challenge by incorporating a safety check during the optimization process. This check considers tie-line equipment limitations, reserve capacity, and various constraints of the spot market clearing model. The model itself is linear, facilitating practical implementation in power-dispatching applications.
Th original research article by Zhong investigated the application of deep learning for improved power load forecasting and market price prediction in the face of complex energy systems and market volatility. Deep learning has emerged as a powerful tool for time series forecasting, and this research proposes a novel framework that integrates and optimizes multiple deep learning models to achieve superior prediction accuracy and reliability. The proposed framework leverages the strengths of three distinct deep learning architectures: Artificial Neural Networks (ANNs), Long Short-Term Memory (LSTM) networks, and transformers. ANNs provide a versatile foundation for the model, while LSTMs, known for their proficiency in sequence modeling, generate initial predictions. The framework further incorporates transformers, specifically their self-attention mechanism, to capture long-range dependencies within the data. This combined approach significantly enhances the overall model performance. The effectiveness of the proposed framework is evaluated through experiments using various public datasets. The performance is compared to both traditional forecasting methods and a single-model deep learning approach. The results conclusively demonstrate that the integrated framework surpasses other methods in predicting both power load and market prices.
The methods article by Wu et al. proposed a novel method for predicting hydroelectricity generation using a combination of meteorological similar days and the XGBoost machine learning model. Accurate prediction of hydroelectricity supply and demand is crucial for efficient resource management, reliable power supply, and minimizing the impact of natural disasters. The proposed method leverages historical meteorological and runoff data to identify days with weather patterns similar to the current day. These “similar days” form a dataset used to train an XGBoost model. The trained model then predicts future runoff based on the current meteorological data, which can be used to estimate future hydroelectricity generation. The effectiveness of the proposed method is evaluated using data from a hydroelectricity cluster in Yunnan, China. The results demonstrate high prediction accuracy and stability, highlighting its potential as a valuable tool for hydroelectricity prediction.
The original article by P. Wang et al. proposed a novel ensemble model for short-term wind power prediction that addresses the challenges posed by the high randomness and volatility of wind power. The model leverages a combination of three techniques: Ensemble Empirical Mode Decomposition (EEMD), Gated Recurrent Unit (GRU), and Markov Chain (MC). Initially, the EEMD algorithm decomposes historical wind power sequences into stationary subsequences, reducing the impact of random fluctuations and noise. Subsequently, the GRU model predicts each subsequence, and the aggregated predicted values yield preliminary forecasts. Finally, the MC is employed to refine the prediction accuracy. Extensive numerical experiments, particularly on the ZMS wind farm’s spring dataset, demonstrate the superiority of the proposed EEMD-GRU-MC model over six benchmark models in terms of various evaluation metrics. Notably, the model achieves a mean absolute error (MAE) of 1.37 MW, a root mean square error (RMSE) of 1.97 MW, and a mean absolute percentage error (MAPE) of 1.76%. Moreover, the model exhibits high computational efficiency, requiring an average of approximately 35 min for accurate daily wind power prediction after 30 iterations. Thus, the ensemble model based on EEMD-GRU-MC holds promise for short-term wind power forecasting.
The Policy and practice review article by Han et al. proposed a new scheduling method for large hydropower plants in China’s evolving electricity market. The method addresses challenges caused by complex factors like uncertain electricity prices, diverse settlement rules, and inter-provincial transmission lines. It incorporates Latin hypercube sampling and K-means clustering to account for electricity price fluctuations. The study also establishes a performance measure for priority electricity considering settlement rules and proposes methods to handle inter-regional transmission connections and hydraulic coupling in cascaded hydropower plants. The complex non-linear problem is then transformed into a solvable mixed-integer linear programming model using the Big M method.
Cascade hydropower producers face two risks in medium- and long-term electricity markets: bidding risks during bidding and operational risks during scheduling due to uncertain water flow and market prices. The methods article by Lu et al. proposed a new decision-making method for such producers in a hydropower-dominated market in Southwest China. The method combines Joint Information Gap Decision Theory (IGDT) and Prospect Theory to address these risks. IGDT is used in a robust optimization model to find the maximum allowable variation in water flow and market prices while meeting expected revenue targets. Prospect Theory is then used to consider producer risk tolerance when developing bidding strategies. To avoid accuracy Research Topic caused by curve fitting in traditional solvers, the paper employs a nonlinear programming method combined with an improved stepwise optimization algorithm. The effectiveness of the method is verified using a real-world cascade hydropower station example. The results show that the method can determine the acceptable range of variation for water flow and market prices under different revenue targets and can be used to develop bidding and operation plans that consider producer risk tolerance.
CS: Writing–review and editing. JS: Writing–review and editing. Z-kF: Writing–review and editing. IF: Writing–original draft. YX: Writing–review and editing.
The authors declare that the research was conducted in the absence of any commercial or financial relationships that could be construed as a potential conflict of interest.
All claims expressed in this article are solely those of the authors and do not necessarily represent those of their affiliated organizations, or those of the publisher, the editors and the reviewers. Any product that may be evaluated in this article, or claim that may be made by its manufacturer, is not guaranteed or endorsed by the publisher.
Keywords: energy forecasting, power grid optimization, wind turbine control, short-term prediction models, optimization algorithms, ensemble forecasting, hydro-wind-solar scheduling, market decision-making methods
Citation: Su C, Shen J, Feng Z-k, Fattah I and Xiong Y (2024) Editorial: Key technologies for hybrid energy system planning and operation. Front. Energy Res. 12:1413951. doi: 10.3389/fenrg.2024.1413951
Received: 08 April 2024; Accepted: 22 April 2024;
Published: 06 May 2024.
Edited and reviewed by:
Ellen B. Stechel, Arizona State University, United StatesCopyright © 2024 Su, Shen, Feng, Fattah and Xiong. This is an open-access article distributed under the terms of the Creative Commons Attribution License (CC BY). The use, distribution or reproduction in other forums is permitted, provided the original author(s) and the copyright owner(s) are credited and that the original publication in this journal is cited, in accordance with accepted academic practice. No use, distribution or reproduction is permitted which does not comply with these terms.
*Correspondence: IMR Fattah, cml6d2FudWwuYnVldEBnbWFpbC5jb20=
Disclaimer: All claims expressed in this article are solely those of the authors and do not necessarily represent those of their affiliated organizations, or those of the publisher, the editors and the reviewers. Any product that may be evaluated in this article or claim that may be made by its manufacturer is not guaranteed or endorsed by the publisher.
Research integrity at Frontiers
Learn more about the work of our research integrity team to safeguard the quality of each article we publish.