- 1School of Economics and Trade, Hunan University, Changsha, Hunan, China
- 2School of Economics and Management, Tibet University, Lhasa, Tibet, China
Introduction: Facing the problem that it is difficult to reconcile development and carbon reduction in the energy sector, this study explores the impact mechanism of the development of energy storage industry on low-carbon economy from the perspective of the energy trilemma.
Methods: Using a moderated two-mediation model and data from 275 cities in China, this study explores the differences in the impact mechanisms of the development of the energy storage industry on the low-carbon economy in different regions of China.
Results: This study draws the following conclusions: first, the development of the energy storage industry can promote the green economy by facilitating technical support and the development of new energy industries. Second, financial support can regulate the effect of this mediating role. Third, in China, the contribution of energy storage industry development to green development is significantly higher in the northern region than in the southern region due to the differences in impact mechanisms.
Discussion: The results of this study provide an effective path for developing countries to balance energy sector development, security, and low-carbon.
1 Introduction
Climate change is one of the most pressing human issues worldwide (Kong et al., 2023; Wu et al., 2023). According to the CO₂ Emissions in 2023 published by the International Energy Agency, China’s carbon dioxide emissions will amount to 35% of the world’s total carbon dioxide emissions in 2023, making it the world’s largest carbon dioxide emitter, and thus China’s actions will determine the success or failure of mankind’s response to the climate problem and the development of a low-carbon economy. Carbon emissions from the energy sector account for more than 80% of the total emissions in China, rendering the low-carbon transformation of the energy sector the most important factor affecting the production of a low-carbon economy (Fan and Zhang, 2022). For developing countries such as China, where both economic development and carbon emission reduction are extremely important, how should the energy sector balance development and carbon emission reduction to promote the realization of a low-carbon economy?
The main contribution and novelty of this study are as follows: unlike most existing studies (Azam et al., 2023a; Duan et al., 2023; Li et al., 2023; Azam, 2024), which consider renewable energy to be the key to balancing the developmental and low-carbon characteristics of a low-carbon economy, this study considers that the key to the role of renewable energy lies in the development of the energy storage industry, and for the first time, constructs a system for the impact of the energy storage industry on the green economy. Moreover, this study introduces the theory of energy triple dilemma difficulty research for the first time in the study of green economy issues, and constructs a research theory containing energy triple dilemma. From the perspective of the energy trilemma, this study explores the pathways through which the energy storage industry affects the low-carbon economy and the differences in this mechanism in different regions, and the results of the study provide an effective path for developing countries to balance energy sector development, security and low-carbon.
2 Literature review and theoretical framework construction
The concept of a low-carbon economy was first introduced in an energy white paper titled “Future Energy—Creating a Low-Carbon Economy” that was published by the British government in 2003, which emphasized the need to obtain greater economic benefits with less energy consumption and pollution” (Nannan and Chang, 2018). The concept of a low-carbon economy encompasses the two common issues of development and low-carbon, in addition to which the energy sector has its own special problems. In view of the importance of energy security (Wen et al., 2023a), governments usually consider development, security and low-carbon issues simultaneously when making decisions in the energy sector, and the Energy Trilemma has emerged.
The energy trilemma, which refers to the difficulty in achieving energy affordability and access, energy security, and environmental sustainability, needs to be balanced in all countries (Khan et al., 2022). At present, most research on the energy trilemma focuses on its evaluation system and impact relationship, but few studies have provided solutions to the trilemma. Scholars believe that factors such as the digital economy (Zhao et al., 2024), education (Gibellato et al., 2023), climate finance (Setyowati, 2020), and government price policy (Wei et al., 2021) can help improve the trilemma, while little attention has been paid to the role of the energy sector itself. This study argues that solutions to the energy trilemma should be considered first and foremost in the energy sector compared to measures in other areas.
This study argues that, in terms of energy itself, the main difficulties in developing clean energy are currently the uncertainty, volatility, and low economic efficiency of clean energy access (Liu et al., 2022), which threaten the security, affordability, and access to energy. One recognized solution to this problem is to build a more efficient power system and further develop the energy-storage industry.
In terms of power systems, two aspects are generally discussed: enhancing the resilience of traditional power systems and building new power systems such as virtual power plants and microgrids. In terms of traditional power systems, scholars mainly use probabilistic methods, possibilistic method and other methods to deal with node power uncertainties and network uncertainties. (Aien et al., 2016; Singh et al., 2022), while research has been focused on microgrids, distributed energy resources, virtual power plants, remote-controlled switches, energy management systems, demand responses, and black start units to solve the uncertainty and volatility associated with new energy access (Izadi et al., 2021). In new power systems such as virtual power plants and microgrids, energy storage devices play an irreplaceable role and support the construction of new power systems.
In terms of developing the energy storage industry, the functions of the energy storage system itself; peak shifting, valley filling, and stabilization of the power grid have been discussed for the development of new power systems. According to Tan et al. (2018), the main roles of energy storage are: (1) Stable system output. (2) Emergency power supply. (3) Improving the flexibility of power system dispatch. Energy storage is associated with functions such as chip peak off and fill valley up. In terms of stabilization, energy storage can facilitate the construction of new grid systems, such as microgrids, by allowing exchange between different energy sources, solving the problem of power uncertainty. Li and Wang (2019) believe that “energy storage is expected to support distributed power and the micro-grid, promote the open sharing and flexible trading of energy production and consumption, and realize multi-functional coordination,” while Hannan et al. (2021) state that “autonomous microgrids are required to guarantee reliability, reduce GHG discharges, and allow blended energy resources to meet the innovative and unpredictable demands of a consistent power supply.” Miller and Carriveau (2018) similarly argue that “Arbitrage is another quantifiable benefit of energy storage systems, which can be charged during low-price periods and discharged during high-price periods.”
The theoretical model constructed in this study is presented in Figure 1. The development of energy storage industry can contribute to the solution of the energy trilemma through the product market and factor market, and can also contribute to the solution of the energy trilemma through the improvement of the new electric power system, and then through the product market and factor market. As far as the product market is concerned, Gissey et al. (2019) suggest that “energy storage offers the flexibility required to integrate renewable generation into electricity systems,” while Martinez-Duart et al. (2015) stated that “storage can make dispatchability much more effective by reducing the curtailment periods of low demand, therefore increasing the capacity factors of renewable systems.” Therefore, the article argues that the development of the energy storage industry will promote the development of new energy industries and products, thus contributing to the solution of the energy trilemma and the development of a green economy. Regarding factor markets, Wu and Jiang. (2021) argued that industrial integration and agglomeration brought about by industrial development promote technological innovation. Ren et al. (2023a) argued that “Given the conflict between economic development and environmental protection, green innovation of firms is a good way to ease this conflict”. This paper argues that the development of the energy storage industry will promote technological innovation, which in turn will contribute to the solution of the energy trilemma. Miller and Carriveau. (2018) argue that energy storage as a solution is limited more by finance than by technology itself. Funding affects not only the development of the energy storage industry but also new energy industries and other industrial and factor markets. Therefore, this paper argues that although capital belongs to the factor market (Wen et al., 2023b), it plays a role in influencing the entire impact process (Azam et al., 2023b), and its moderating effect on the overall impact needs to be analyzed separately.
This study focuses on how the development of the energy storage industry affects energy transition and explores the relationship between the development of the energy storage industry, technical support, new energy industries, and energy transition. The relationship presented in Figure 2 is based on Figure 1, with the following assumptions:
Hypothesis 1. Development of the energy storage industry can contribute to the development of a low-carbon economy.
Hypothesis 2. The energy-storage industry can contribute to the development of a low-carbon economy through technical support.
Hypothesis 3. The development of the energy storage industry can promote the development of a low-carbon economy by promoting the development of new energy industries.
Hypothesis 4. Financial support plays a moderating role in the direct impact that energy storage industry development has on energy transition.
Hypothesis 5. Financial support moderates the impact that energy storage industry development has on energy transition through technological support.
Hypothesis 6. Financial support plays a moderating role in the development of the energy storage industry through the impact that new energy has on energy transition.
3 Model construction and data
3.1 Model construction
In this study, the moderated two-mediation model was chosen to test the model, which has the following advantages: 1. The mediation effect and the moderating effect can be explored at the same time. 2. It can explain the linear model better and help in conducting the review.
To verify the impact of the energy storage industry on the low-carbon economy, a basic regression model was constructed:
where
A moderating effect model was also constructed to verify the impact of developing the energy storage industry on a low-carbon economy under financial support constraints:
where
Based on previous analysis of the energy transition mediation mechanism, the following mediation model was constructed to verify the mediating role that energy transition has on the development of the energy storage industry and a low-carbon economy:
where
To further explore the mediating role that technical support and the development level of a new energy industry has on the energy storage industry development of a low-carbon economy under technological constraints, the following moderated mediation model was constructed:
Eqs 8, 9 were used to explore the moderating effect of financial constraints on the first half of the mediating role of the energy storage industry in influencing the green economy through technical support, and Eqs 10, 11 were used to explore the moderating effect of financial constraints on the second half of this mediating role. Eqs 12, 13 were used to explore the moderating effect of financial constraints on the first half of the mediating role of the energy storage industry in influencing the green economy through the new energy industry, and Eqs 14, 15 were used to explore the moderating effect of financial constraints on the second half of the mediating role.
3.2 Variable measures and descriptions
3.2.1 Core explanatory variables
This study uses low carbon economy as the dependent variable. According to Wang et al. (2019),
The carbon emissions of each city were calculated from the sum of emissions produced by transportation and construction, industrial processes, agriculture, forestry, land-use changes, waste disposal activities, purchased electricity, and heating and cooling. The emission factors were adopted from the Inventory and Guidelines for Provincial-Level Greenhouse Gas Emissions (Trial) and the IPCC Emission Factor Database. The emissions from the various sectors were obtained from the China Energy Statistical Yearbook, China Industry Statistical Yearbook, China City Statistical Yearbook, China Statistical Yearbook on the Environment, and statistical yearbooks at various administrative levels.
This study uses the level of development of the energy storage industry as the independent variable. Liu et al. (2023) concluded that hybrid shared energy storage significantly reduces carbon emissions; therefore, the level of development in the energy storage industry was selected as the explanatory variable, and the number of energy storage enterprises in each city was used to measure the development level of the energy storage industry. The numbers of energy storage enterprises and companies were obtained by searching the term “energy storage” in the Aiqicha Database. The level of development in the energy storage industry was treated logarithmically, and the results denoted using lnES.
In this study, technical support and the development level of new energy industries are used as mediating variables. Zhu et al. (2023) argue that the technological effects of green technological innovation play a key role in low-carbon economies. Therefore, technical support was adopted as a mediating variable in this study. Because most technological innovations brought about by the development of the energy storage industry are green technologies, the number of green patents (GP) granted in each city, which was based on the WIPO Green Patent List from the State Intellectual Property Office, was used to represent the level of technological support. The number of green patents granted was treated logarithmically, and the results denoted using lnGP.
Murshed et al. (2021) argue that “nonfossil fuel and hydroelectricity consumption have been proven to abate the carbon footprint levels.” Therefore, the level of new energy industry development (NE) was adopted as the mediating variable, with the number of new energy industries in each city used to measure the level of new energy industry development in the city. The number of new energy enterprises was obtained by searching “new energy” in the Aiqicha Database and included all enterprises with “new energy” in their names. The development level of the new energy industry was treated logarithmically, and the results denoted using lnNE.
This study uses financial support as a moderating variable. Miller and Carriveau. (2018) argue that energy storage as a solution is limited more by finance than by technology itself. Ren et al. (2022), Ren et al. (2022) argue that digital finance and financing constraints affect factors such as green innovation and energy prices. Considering data availability, the year-end balance of Chinese Yuan loans from financial institutions (FS) was therefore used to represent the level of financial support, with samples selected from the China Urban Statistical Yearbook. Financial support was also treated logarithmically, and the results denoted lnFS.
3.2.2 Control variables
Time (year), the number of jobs in non-private units (EMP), share of secondary industries in GDP (SEC), Number of industrial enterprises with main business income of 20 million yuan or more in the current year (NUM), number of full-time faculty members in colleges and universities (TEA), and built-up area (BUA) were used as control variables. The number of non-private firms was set as the control for labor market effects in the factor market. The number of full-time teachers in higher education was set to control for non-green technology in the factor market. The built-up area was used to control land elements of the factor market. The proportion of GDP accounted for by secondary industries and the number of industrial enterprises above a designated size were set to control the state of the product market from the perspectives of economic structure and economic volume, respectively.
Time, the number of non-private sector employees, share of secondary industries in GDP, number of industrial enterprises above large scale, number of full-time faculty members in colleges and universities, and built-up area were obtained from the China Urban Statistical Yearbook. Control variables other than time were dimensionless, and the effect of magnitude was eliminated using maximum-minimum standardization. The standardized variables were denoted EMPm, SECm, NUMm, TEAm, and BUAm.
3.2.3 Substitution of variables
In order to be in the robustness test, the dependent, mediating, moderating and control variables were replaced in this study.
In terms of the substitution of dependent variables, the use of different carbon emission coefficients to calculate carbon emissions will be different, which means that different organizations have different carbon emissions in their statistics. The CO2 emissions per unit of total GDP from the China Public Policy and Green Development Database were thus used as the CO2 emissions per unit of GDP, denoted EW2, for the robustness test in this study.
In terms of the replacement of mediator variables, the enterprises obtained by searching the keywords “new energy industry” in the Aiqicha Database includes those related to the entire new energy industry chain, and the statistical scope is wider. Enterprise Research*Social Science Data was therefore used as the mediator variable for the robustness test because the number of enterprises in this database is relatively small and the statistical range is narrow. The data were logarithmized and denoted lnNE2.
In terms of the substitution of moderating variables, the development of commercial banking and financial institutions depends on loan issuance and deposit acquisition. Therefore, the level of financial support in the robustness test was measured using the year-end Chinese Yuan balances of various deposits in financial institutions. The data were logarithmically treated and denoted using lnFS2.
In terms of the replacement of control variables, the land elements in the China Urban Statistical Yearbook are represented using both built-up area and the facility land area, with the difference being that the built-up area is the actual number of land elements, while the built-up land area is the number of land elements available in the plan. In this study, the land element represented using construction land in the China Urban Statistical Yearbook was used as a control variable for the robustness test. The data were standardized and denoted using BUAm2.
As the latest data in the China Public Policy and Green Development Database and Enterprise Research*Social Science Big Data are from 2020, 574 datapoints from 2016 to 2020 were used in the robustness test.
3.3 Data sources
The data from 275 cities in China from 2017 to 2021 were used in this study. Samples with missing data were not included. The final dataset comprised 1,107 datapoints. All data used in this study were obtained from the China City Statistical Yearbook, Aiqicha Database, and the State Intellectual Property Office.
4 Results
4.1 Descriptive statistics and correlation analysis
Table 1 lists the descriptive statistical results of the variables involved in this study and the correlation between the variables. From the core explanatory variables, the level of development of the energy storage industry has a significant negative correlation with the carbon emissions per unit of GDP, with a coefficient of −0.28 (p < 0.01), and the development of the energy storage industry may lead to a reduction in carbon emissions per unit of GDP, which is consistent with Hypothesis 1. The development of new energy industry and the number of green patents are significantly negatively correlated with unit GDP carbon emissions, with coefficients of −0.257 (p < 0.01) and −0.316 (p < 0.01), respectively, and the development of new energy industry and technological upgrading may lead to the reduction of unit GDP carbon emissions, consistent with hypotheses 2 and 3. The level of financial support are significantly negatively correlated with unit GDP carbon emissions, the coefficient is −0.361 (p < 0.01), financial support may lead to the reduction of unit GDP carbon emissions. From the control variables, time, the proportion of secondary industry, labor force elements, the number of college teachers, the number of industrial enterprises, the area of built-up areas are positively correlated with unit GDP carbon emissions, that is, with industrialization, urbanization, labor force increase with the green technology other than science and technology is detrimental to the development of green economy.
4.2 Regression analysis
4.2.1 Mediating effect test
As shown in Table 2, the 95% confidence intervals for both the direct and indirect benefits did not pass through 0, and the p-values are all less than 0.05, indicating that both are significant. Moreover, the mediating effect that the energy storage industry had on green development through technical support and new energy industries was incomplete because both belonged to the product and factor markets, meaning that the mediating effect of the energy storage industry on the green economy through the product and factor markets was incomplete. The total effect coefficient between the number of enterprises in the energy storage industry and carbon emissions per unit of GDP was −0.6388, indicating that for every 1% increase in the energy storage industry, the carbon emissions generated are reduced by 0.6388 million tons per 10,000 yuan of GDP. The development of the energy storage industry is thus favorable for the development of the carbon economy, verifying Hypothesis 1. The direct effect coefficient of 0.2782 for energy storage on carbon emissions per unit of GDP indicates that every 1% increase in the number of enterprises in the energy storage industry directly increases the carbon emissions generated per 10,000 yuan of GDP by 0.2782 million tons. The indirect effect coefficient of the energy storage industry on carbon emissions per unit of GDP was 0.917, indicating that although the growth in the number of enterprises in the energy storage industry leads directly to an increase in carbon emissions, indirectly, every 1% increase in the number of enterprises in the energy storage industry directly reduces the carbon emissions generated per 10,000 yuan of GDP by 0.917 million tons. These results indicate that the development of the energy storage industry itself is not conducive to a green economy; however, the indirect effects of energy storage are favorable to a green economy.
In terms of the indirect effects of the energy storage industry, the coefficient for the influence on the green economy through the market for new energy products was 0.5783. This datum indicates that for every 1% increase in the number of enterprises in the energy storage industry, the carbon emissions generated for every 10,000 yuan of GDP will be reduced by 0.5783 million tons These results indicate that the development of the energy storage industry promotes a green economy by promoting the development of new energy industries, supporting Hypothesis 3. In terms of the indirect effect of the energy storage industry, the coefficient for the influence that green technology has on the green economy was −0.3387, which indicates that every 1% increase in the number of enterprises in the energy storage industry will reduce the carbon emissions generated for each 10,000 yuan of GDP by 0.3387 million tons. These results indicate that the energy storage industry contributes to the green economy through technological support, thus supporting Hypothesis 2.
4.2.2 Direct effect test with moderation
Hierarchical analysis was used to test the moderating effect model (Wen and Ye, 2014). The first step in this method is to determine whether the moderating variable (financial support) has a direct effect.
As shown in Table 3, the confidence interval prediction of the mediated direct effect interaction term (lnES*LnFS) did not contain 0, and the p-value was less than 0.05; therefore, the moderated direct effect was significant, and Hypothesis 4 was supported. The effect of the energy storage industry on carbon emissions per unit of GDP was negative, the coefficient of the interaction term between the energy storage industry and financial support was positive, and the financial support weakened the direct effect. As financial support increases, the scale of the energy storage industry expands and expands, and the carbon emissions directly caused by the energy storage industry increase.
4.2.3 Test for moderated mediation effect
To verify the moderated mediation mechanism, the first (lnES > lnGP, lnES > lnNE) and second halves (lnNW, lnGP > EW) of the mediation effect were tested separately. The results are summarized in Table 4.
The moderating effects in the first half were significant. With the development of the new energy industry as a mediating variable, the energy storage industry positively affected the new energy industry; however, the interaction term between the energy storage industry and financial support had a negative effect on the new energy industry, weakening the mediating effect. In other words, the development of the energy storage industry will bring about an enhancement to the development of the new energy industry, and this enhancement lies largely in the market and financial support of the new energy industry by the energy storage industry, and therefore, with the fulfillment of the financial support, this positive promotion effect is weakened.
The confidence interval predicted by the interaction term did not contain 0, and the moderating effect was significant. With new energy industry development as the mediating variable, the energy storage industry positively affected green technology support. The interaction term of the energy storage industry and financial support had a negative effect on green technology support, weakening the mediating effect, and the confidence interval predicted by the interaction term did not contain zero, indicating a significant effect. In other words, the development of the energy storage industry promotes green technological innovation, and much of this promotion lies in the fact that the development of the energy storage industry provides financial security for green technological innovation, and thus this positive promotion is weakened as financial support is met.
In the second half of mediation, some effects were significant, while others were not. With the development of the new energy industry as the mediating variable, the new energy industry negatively affected carbon emissions per unit of GDP, and the interaction term of the new energy industry and financial support negatively affected carbon emissions per unit of GDP, enhancing the mediating effect. However, the confidence interval in the prediction of its interaction term contained 0, and the moderating effect was insignificant. In other words, the impact of financial support may be minimal in the reduction of carbon emissions from the development of new energy industries.
With technical support as the mediating variable, the technical support negatively affected the carbon emissions per unit of GDP while the interaction term between the new energy industry and financial support positively affected carbon emissions per unit of GDP, weakening the mediating effect. The confidence interval predicted by the interaction term did not contain 0, and the moderating effect was significant. In other words, green technology will reduce carbon emissions, and this reduction is based on the scale of existing industries, while financial support, although it will promote the application of green technology, such application will lead to the large-scale construction of related industries, a process that may, on the contrary, increase carbon emissions.
Overall, as shown in Table 5, mediation with the new energy industry as a mediating variable was simultaneously regulated by financial support; the mediating effect was enhanced, and carbon emissions per unit of GDP decreased, proving Hypothesis 5. Although financial support has a significant effect on both the first and second half of the mediation effect, the effects of the two segments weaken each other, and this path of mediation effect may not be significant in terms of the total process of financial support simultaneously moderating the mediation effect with technical support as the mediating variable. Hypothesis 6 may need to be explored further.
To further explore the moderating roles of the energy storage industry, new energy industry, green patents, and green development, and technological support, the new energy industry and carbon emissions per unit of GDP were divided into high and low groups based on the previous and following standard deviations. Simple slope tests and effect analysis was performed, and the results plotted.
The results, as shown in subplot (A) of Figure 3, indicated that in the first half of mediation with technical support as the mediating variable, a high level of financial support had a significant positive effect on the technical support (p = 0.0000, β = 0.2576), and a low level of financial support had positive and significant effect on technical support (p = 0.0000, β = 0.4505). The results, as shown in subplot (B) of Figure 3, indicated that in the first half of mediation with the new energy industry as the mediating variable, both high and low levels of financial support had a significant positive effect on the development of the new energy industry, at (p = 0.0000, β = 0.3535) and (p = 0.0000, β = 0.4551), respectively. The results, as shown in subplot (C) of Figure 3, indicated that in the second half of mediation with technical support as the mediating variable, financial support had a non-significant effect on carbon emissions per unit of GDP when high (p = 0.1481, β = 0.2640) and a negative significant effect on carbon emissions per unit of GDP when low (p = 0.0137, β = −0.3019).
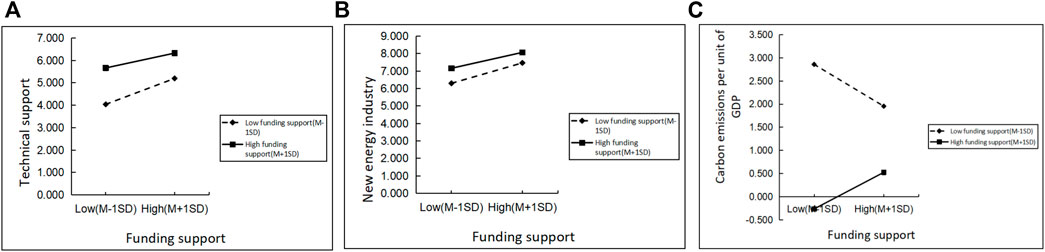
Figure 3. Simple effect analysis chart. (A) The moderating effect of financial support on the development of energy storage industry and technological support. (B) The moderating effect of financial support on the development of energy storage industry and development of new energy industry. (C) The moderating effect of financial development on the relationship between technological support and carbon emission per unit of GDP.
4.3 Robustness tests
Replacing the explanatory, mediating, and moderating variables with re-descriptive statistics, correlation analysis, and regression analysis indicated that Hypothesis 2, 5, 6 were valid with robust conclusions, while Hypothesis 1, 3, 4 were non-robust. The results are shown in Table 6.
As shown in Table 6, the signs of the correlation coefficients of the independent, mediator, and moderator variables with carbon emissions per unit of GDP in the robustness test were consistent with those obtained previously; thus, the correlations were robust. The signs of the correlation coefficients obtained for the control variables, which represent the proportion of the output of secondary industries in the industrial structure and the time and carbon emissions per unit of GDP, were not consistent with those obtained previously; thus, the effects of the control variables were not robust.
As shown in Table 7 the robustness test indicated that the direct effect of the energy storage industry on carbon emissions per unit of GDP was not significant and the indirect mediating effect with technical support as a mediating variable had a significant impact, while the mediating effect of the new energy industry was not significant. Because the direct benefits were not robust, no more robustness tests were performed for these effects.
As shown in Tables 8, 9, the mediating effects indicated that financial support was significant in mediating both the before and after stages of mediation with other factors. Financial support had a robust moderating effect in both the first and second halves of mediation with technical support as the mediating variable, and an unrobust moderating effect in the second half with the new energy industry as the mediating variable.
4.4 Heterogeneity analysis
Since the degree of economic development and social customs differ in both the four major economic regions and North and South China, heterogeneity was tested from two perspectives: (1) North and South China, and (2) the four major economic regions. The difference between the north and south has been widely discussed in recent years; however, the division between the provinces and cities in the north and south remains unclear. The northwestern, northeastern, and northern local provinces article are collectively referred to as northern, and the Qinghai–Tibetan and southern provinces as southern in this study.
As seen in Table 10, of the four major economic regions, the highest total effect of the energy storage industry on green development was observed in the northeast, which is likely because of the strong industrial base in this region. However, the development of the energy storage industry was weak, and the benefits associated with the development of the energy storage industry were stronger. The lowest total benefit of the energy storage industry for green development was in the west of the country, where the benefit to the low-carbon economy was not significant, probably because the industrial base is poor in this area. The energy storage industry does not benefit from the development of new energy sources, and it is difficult to deal with carbon emissions from the development of the energy storage industry itself.
As far as direct effects are concerned, the strongest direct effect of the energy storage industry on green development was in the west, where the highest carbon emissions from the energy storage industry were observed, which could be due to this region being technologically backwards and difficulty dealing with the carbon emissions from the energy storage industry itself. The weakest direct effect of the energy storage industry on green development was in the east; however, it was not significant, probably because the carbon emissions, carbon trading, and other supporting hardware and software are more complete and technology is more advanced in the east than in the other regions, meaning that the region can effectively deal with the carbon emissions associated with development of the energy storage industry.
The strongest indirect effect of the energy storage industry was in the northeast, which may be due to the technical support and new energy industry in this region, while the weakest was in the east. Two intermediary channels were effective: the energy storage industry had a strong driving effect, and the northeast region lacked the development of new energies; thus, the development of the energy storage industry can enhance the marginal effect energy storage. While the eastern region had a certain foundation for technological and new energy industry development, the marginal effect of developing the energy storage industry was weaker. The two intermediary channels in the eastern region were ineffective, leading to a weak indirect effect and the total effect of support for the green economy being particularly low.
In terms of the two major Chinese regions, the north and the south, the total contribution of developing the energy storage industry on the green economy was stronger in the north because the direct effect of energy storage development was not significant and the indirect effect was stronger in the north than in the south of the country. Little difference was observed between the north and south in terms of the indirect effects of green technologies; however, the coefficient for new energy industries was more than four times higher in the north than in the south, probably because southern China is more cloudy with high precipitation, rendering industries such as photovoltaics less efficient in this part of the country.
As shown in Table 11, heterogeneity was analyzed according to the north and south for mediating effects against moderation. Financial support significantly affected mediation by the new energy industry as a mediating variable in both the south and the north, and the total moderating effect that financial support had on technical support as a mediating variable was unsatisfactory. The total moderation associated with the new energy industry as a mediating variable indicated that the moderating effect of financial support was stronger in the north than in the south, probably because northern China is poorer than southern China; therefore, the marginal benefit of financial resources was higher in this region.
5 Conclusion
This study examines the mechanism of the energy storage industry’s impact on the green economy and explores the variation of this impact mechanism in different regions of China. First, we test the mediating effect of new energy industry and green technology in this mechanism by using a double mediating effect model. Second, we introduced moderating variables in the dual mediating effect to explore whether the government and large plutocrats can regulate this mediating mechanism through financial support. Finally, we calculate the differences in this mechanism in different regions of China, in order to provide differentiated guidance for reducing carbon emissions in China.
There are three main conclusions. First, the development of the energy storage industry promotes the development of the green economy, and this effect is realized through two mediating effects: in the first path, the development of the energy storage industry promotes the development of the new energy industry, which in turn promotes the green development; in the second path, the development of the energy storage industry promotes the green technology innovation, which in turn promotes the green development.
Second, according to the results of the moderating effect, we find that financial support does not necessarily enhance the impact of the energy storage industry on green development. In the direct effect of energy storage industry on green development, financial support is unfavorable to the reduction of carbon emissions. In the mediating effect with new energy development as the mediating variable, financial support favors the construction of carbon emissions. In the mediating effect with green technology as the mediating variable, financial support is inconsistent in the direction of influence in the first and second halves of the mediating effect, making its total effect insignificant. The reason why financial support does not have the same effect in each path is that financial support expands the production scale of some industries, but this expansion of production may increase carbon emissions.
Third, based on the results of the heterogeneity test, we find that for the four major economic regions, the highest total effect of the energy storage industry on green development is in the Northeast, and the lowest is in the West. In terms of North and South, the total effect of energy storage industry on promoting green economy is stronger in North China than in South China. The magnitude of the energy storage industry’s impact on the green development coefficient may be related to the local industrial base and new energy industry base; when the industrial base is too low, it is difficult for the local area to deal with the carbon emissions of the energy storage industry itself.
Based on the results of the study, this study concludes that, first, governments should focus on controlling the direct carbon emissions of the energy storage industry when developing the energy storage industry, and they should also focus on the indirect effects of the new energy industry and green technological innovations to reduce carbon emissions. Second, governments should be prudent in stimulating the development of the energy storage industry by providing financial elements. Third, when laying out the energy storage industry, the government should choose regions with a better industrial base and better development of new energy industry.
The contribution of the research results can be summarized at both the theoretical and practical levels. At the theoretical level, the research introduces the theory of energy trilemma difficulty research for the first time according to the special problems in the energy field, and constructs the research theory containing the energy trilemma when researching the green economy problems. Moreover, the study deepens the thinking about green economy, considers that the development of energy storage industry is an important reason for the new energy industry to play a role in green economy, and constructs for the first time the influence system of energy storage industry on green economy. At the practical level, the study explores the impact of financial support, which is an important hand of the government in influencing industrial development and green economy, on the influence mechanism, and explores the differences in the influence mechanism of financial support in different regions of China, which provides differentiated guidance for China to build a green economy through the energy storage industry.
Undeniably, the study has some limitations. This study only uses Chinese data to explore the impact mechanism of energy storage industry development on green economy, and the research results may not be practiced in some other countries, and data from more countries can be used to explore the impact of energy storage industry in future studies. In addition, the results of the robustness test of the study are not very good, because the production of energy storage industry increases carbon emissions and reduces carbon emissions through the mediating effect. Future research can build a more in-depth and robust theoretical system.
Data availability statement
The original contributions presented in the study are included in the article/Supplementary Material, further inquiries can be directed to the corresponding author.
Author contributions
JC: Writing–original draft, Writing–review and editing. HL: Writing–original draft, Writing–review and editing.
Funding
The authors declare financial support was received for the research, authorship, and/or publication of this article. This work was funded by The National Social Science Fund of China (Number: 21EH218).
Conflict of interest
The authors declare that the research was conducted in the absence of any commercial or financial relationships that could be construed as a potential conflict of interest.
Publisher’s note
All claims expressed in this article are solely those of the authors and do not necessarily represent those of their affiliated organizations, or those of the publisher, the editors and the reviewers. Any product that may be evaluated in this article, or claim that may be made by its manufacturer, is not guaranteed or endorsed by the publisher.
Supplementary material
The Supplementary Material for this article can be found online at: https://www.frontiersin.org/articles/10.3389/fenrg.2024.1413057/full#supplementary-material
References
Aien, M., Hajebrahimi, A., and Fotuhi-Firuzabad, M. (2016). A comprehensive review on uncertainty modeling techniques in power system studies. Renew. Sustain. Energy Rev. 57, 1077–1089. doi:10.1016/j.rser.2015.12.070
Azam, A. (2024). How environmental policy stringency, foreign direct investment, and eco-innovation supplement the energy transition: new evidence from NICs. Sustainability 16 (7), 3033. doi:10.3390/su16073033
Azam, A., Ateeq, M., Shafique, M., Rafiq, M., and Yuan, J. (2023a). Primary energy consumption-growth nexus: the role of natural resources, quality of government, and fixed capital formation. Energy 263, 125570. doi:10.1016/j.energy.2022.125570
Azam, A., Rafiq, M., Shafique, M., and Yuan, J. (2023b). Interpreting the dynamic nexus between green energy, employment, fossil fuel energy, and human development index: a panel data investigation. Energies 16 (7), 3132. doi:10.3390/en16073132
Duan, X., Xiao, Y., Ren, X., Taghizadeh-Hesary, F., and Duan, K. (2023). Dynamic spillover between traditional energy markets and emerging green markets: implications for sustainable development. Resour. Policy 82, 103483. doi:10.1016/j.resourpol.2023.103483
Fan, A., and Zhang, D. (2022). Study on carbon dioxide emissions in China's energy sector. Low. Carbon World 12 (04), 99–101. doi:10.16844/j.cnki.cn10-1007/tk.2022.04.010
Gibellato, S., Ballestra, L. V., Fiano, F., Graziano, D., and Gregori, G. L. (2023). The impact of education on the Energy Trilemma Index: a sustainable innovativeness perspective for resilient energy systems. Appl. Energy 330, 120352. doi:10.1016/j.apenergy.2022.120352
Gissey, G. C., Subkhankulova, D., Dodds, P. E., and Barrett, M. (2019). Value of energy storage aggregation to the electricity system. Energy policy 128, 685–696. doi:10.1016/j.enpol.2019.01.037
Hannan, M. A., Wali, S. B., Ker, P. J., Abd Rahman, M. S., Mansor, M., Ramachandaramurthy, V. K., et al. (2021). Battery energy-storage system: a review of technologies, optimization objectives, constraints, approaches, and outstanding issues. J. Energy Storage 42, 103023. doi:10.1016/j.est.2021.103023
Izadi, M., Hosseinian, S. H., Dehghan, S., Fakharian, A., and Amjady, N. (2021). A critical review on definitions, indices, and uncertainty characterization in resiliency-oriented operation of power systems. Int. Trans. Electr. Energy Syst. 31 (1), e12680. doi:10.1002/2050-7038.12680
Khan, I., Zakari, A., Dagar, V., and Singh, S. (2022). World energy trilemma and transformative energy developments as determinants of economic growth amid environmental sustainability. Energy Econ. 108, 105884. doi:10.1016/j.eneco.2022.105884
Kong, S., Li, H., and Tan, S. (2023). Carbon markets, energy transition, and green development: a moderated dual-mediation model. Front. Environ. Sci. 11, 1257449. doi:10.3389/fenvs.2023.1257449
Li, X., and Wang, S. (2019). Energy management and operational control methods for grid battery energy storage systems. CSEE J. Power Energy Syst. 7 (5), 1026–1040. doi:10.17775/CSEEJPES.2019.00160
Li, Y., Yan, C., and Ren, X. (2023). Do uncertainties affect clean energy markets? Comparisons from a multi-frequency and multi-quantile framework. Energy Econ. 121, 106679. doi:10.1016/j.eneco.2023.106679
Liu, H., Khan, I., Zakari, A., and Alharthi, M. (2022a). Roles of trilemma in the world energy sector and transition towards sustainable energy: a study of economic growth and the environment. Energy Policy 170, 113238. doi:10.1016/j.enpol.2022.113238
Liu, L., Yao, X., Qi, X., and Han, Y. (2023). Low-carbon economy configuration strategy of electro-thermal hybrid shared energy storage in multiple multi-energy microgrids considering power to gas and carbon capture system. J. Clean. Prod. 428, 139366. doi:10.1016/j.jclepro.2023.139366
Martinez-Duart, J. M., Hernandez-Moro, J., Serrano-Calle, S., Gomez-Calvet, R., and Casanova-Molina, M. (2015). New frontiers in sustainable energy production and storage. Vacuum 122, 369–375. doi:10.1016/j.vacuum.2015.05.027
Miller, L., and Carriveau, R. (2018). A review of energy storage financing—learning from and partnering with the renewable energy industry. J. Energy storage 19, 311–319. doi:10.1016/j.est.2018.08.007
Murshed, M., Ahmed, Z., Alam, M. S., Mahmood, H., Rehman, A., and Dagar, V. (2021). Reinvigorating the role of clean energy transition for achieving a low-carbon economy: evidence from Bangladesh. Environ. Sci. Pollut. Res. 28, 67689–67710. doi:10.1007/s11356-021-15352-w
Nannan, W., and Chang, Y. C. (2018). EFFECTIVENESS OF LOW-CARBON GOVERNANCE IMPLEMENTATION IN CHINA. Environ. Eng. Manag. J. (EEMJ) 17 (3), 601–609. doi:10.30638/eemj.2018.061
Ren, X., Qin, J., Jin, C., and Yan, C. (2022). Global oil price uncertainty and excessive corporate debt in China. Energy Econ. 115, 106378. doi:10.1016/j.eneco.2022.106378
Ren, X., Xia, X., and Taghizadeh-Hesary, F. (2023a). Uncertainty of uncertainty and corporate green innovation—evidence from China. Econ. Analysis Policy 78, 634–647. doi:10.1016/j.eap.2023.03.027
Ren, X., Zeng, G., and Zhao, Y. (2023b). Digital finance and corporate ESG performance: empirical evidence from listed companies in China. Pacific-Basin Finance J. 79, 102019. doi:10.1016/j.pacfin.2023.102019
Setyowati, A. B. (2020). Mitigating energy poverty: mobilizing climate finance to manage the energy trilemma in Indonesia. Sustainability 12 (4), 1603. doi:10.3390/su12041603
Singh, V., Moger, T., and Jena, D. (2022). Uncertainty handling techniques in power systems: a critical review. Electr. Power Syst. Res. 203 (6–7), 107633. doi:10.1016/j.epsr.2021.107633
Tan, Z., Tan, Q., and Wang, Y. (2018). A critical-analysis on the development of energy storage industry in China. J. Energy Storage 18, 538–548. doi:10.1016/j.est.2018.05.013
Wang, H., Chen, Z., Wu, X., and Nie, X. (2019). Can a carbon trading system promote the transformation of a low-carbon economy under the framework of the porter hypothesis? empirical analysis based on the PSM-DID method. Energy policy 129, 930–938. doi:10.1016/j.enpol.2019.03.007
Wei, Y., Zou, Q. P., and Lin, X. (2021). Evolution of price policy for offshore wind energy in China: trilemma of capacity, price and subsidy. Renew. Sustain. Energy Rev. 136, 110366. doi:10.1016/j.rser.2020.110366
Wen, F., Chen, M., Zhang, Y., and Miao, X. (2023a). Oil price uncertainty and audit fees: evidence from the energy industry. Energy Econ. 125, 106852. doi:10.1016/j.eneco.2023.106852
Wen, F., Lin, D., Hu, L., He, S., and Cao, Z. (2023b). The spillover effect of corporate frauds and stock price crash risk. Finance Res. Lett. 57, 104185. doi:10.1016/j.frl.2023.104185
Wen, Z., and Ye, B. (2014). Analyses of mediating effects: the development of methods and models. Adv. Psychol. Sci. 22 (05), 731–745. doi:10.3724/sp.j.1042.2014.00731
Wu, B., Zhu, P., Yin, H., and Wen, F. (2023). The risk spillover of high carbon enterprises in China: evidence from the stock market. Energy Econ. 126, 106939. doi:10.1016/j.eneco.2023.106939
Wu, G., and Jiang, J. (2021). Productive services clustering, industrial integration and technological innovation. Shanghai Econ. Res. 7, 69–80. doi:10.19626/j.cnki.cn31-1163/f.2021.07.007
Zhao, C., Dong, K., Liu, Z., and Ma, X. (2024). Is digital economy an answer to energy trilemma eradication? The case of China. J. Environ. Manag. 349, 119369. doi:10.1016/j.jenvman.2023.119369
Keywords: energy storage industry, low-carbon economy, moderated dual mediation model, new energy industry, energy trilemma
Citation: Chen J and Li H (2024) Impact of energy storage industry development on the low-carbon economy: evidence from China. Front. Energy Res. 12:1413057. doi: 10.3389/fenrg.2024.1413057
Received: 06 April 2024; Accepted: 11 June 2024;
Published: 09 July 2024.
Edited by:
Xiaohang Ren, Central South University, ChinaReviewed by:
Yan Zheng, Hainan University, ChinaAnam Azam, Fraunhofer Institute for Systems and Innovation Research ISI (FHG), Germany
Copyright © 2024 Chen and Li. This is an open-access article distributed under the terms of the Creative Commons Attribution License (CC BY). The use, distribution or reproduction in other forums is permitted, provided the original author(s) and the copyright owner(s) are credited and that the original publication in this journal is cited, in accordance with accepted academic practice. No use, distribution or reproduction is permitted which does not comply with these terms.
*Correspondence: Hanzun Li, MTQzMzUwNzg5OUBxcS5jb20=