- Department of Wind and Energy Systems, Technical University of Denmark, Roskilde, Denmark
We study the possible effect of climate change on the extreme wind over northern Europe using data from 18 models of the Sixth Phase of the Coupled Model Intercomparison Project (CMIP6) and the high-emission Shared Socioeconomic Pathway 5-8.5 (SSP585) scenario. We use the spectral correction method to correct the 6-hourly wind speeds and calculate the 50-year wind at an equivalent temporal resolution of 10 min, consistent with the International Electrotechnical Commission (IEC) standard. We obtain the possible effect of climate change through the comparison of the extreme wind parameters, including the 50-year wind and the 95%-percentile of the wind speed, and the change in turbine class at 50 m, 100 m and 200 m, between a near future period (2020–2049) and the historic period (1980–2009). The analysis shows an overall increase in the extreme winds in the North Sea and the southern Baltic Sea, but a decrease over the Scandinavian Peninsula and most of the Baltic Sea. However, the suggested change is not significant enough to conclude whether higher or lower classes of turbines will be needed in this area in the future.
1 Introduction
Extreme winds can cause great loss to society and the 50-year wind,
The scale and speed of wind energy development and deployment have never been so great and it will continue in the future (GWEC, 2022), but we also live in a world where climate is changing potentially affecting wind farm siting parameters like the 50-year return wind. Therefore, it is a relevant question to ask how climate change will impact extreme winds in the future and what this change implies for the cost of wind energy planning and development.
Extreme winds have not been studied as extensively as some other meteorological parameters such as extreme temperature and precipitation. The studies of extreme winds have also mostly been done though historical data from reanalysis and regional downscaling. Reanalysis data has been a popular choice for extreme wind estimation due to its global coverage and long-term availability, including European Centre for Medium-Range Weather Forecasts (ECMWF) reanalysis ERA-40 (e.g., Della-Marta et al., 2009), reanalysis from the National Centers for Environmental Prediction and the National Center for Atmospheric Research (NCEP/NCAR) (e.g., Larsén and Mann, 2009), the National Oceanic and Atmospheric Administration (NOAA) 20th century reanalysis (e.g., Donat et al., 2011), the 5th generation reanalysis from the ECMWF, ERA5 (e.g., Pryor and Bartelmie, 2021; Imberger and Larsén, 2022), Climate Four-Dimensional Data Assimilation (CFDDA) (e.g., Hansen et al., 2016), Climate Forecast System Reanalysis (CFSR) (e.g., Larsén and Kruger, 2014) and Modern-Era Retrospective analysis for Research and Applications version 2 MERRA2 (e.g., Imberger and Larsén, 2022). The temporal resolution of these data ranges from 1 to 6 h, and the spatial grid spacing from about 25 km to a couple of hundreds of kilometers. This resolution is too coarse and not ideal for site-specific extreme wind calculations. Using data from mesoscale model simulations is expected to improve the extreme wind estimation, as shown in, e.g., Bastine et al. (2018) and Larsén et al. (2021), where data from the Weather Research and Forecasting (WRF) model with spatial grid spacing of 3 km were used. The only global coverage of extreme wind that has applied microscale modeling with spatial grid spacing of 275 m has been created within the Global Atlas for Siting Parameters (GASP) project (Larsén et al., 2022). The above-mentioned studies look at issues related to the calculation of extreme winds through model data of different spatial and temporal resolution, and focus on the estimation of extreme winds using historical data, rather than the impact of climate change.
In recent decades, Regional Climate Models (RCMs) have been used to study the impact of climate change on extreme wind. However, these studies founded on several generations of RCMs do not seem to have reached a consensus. These studies include, for example, the PRUDENCE project (Prediction of Regional Scenarios and Uncertainties for Defining European climate change Risks and Effects), a EU Framework Program project that ended in 2004 (WCRP Working Group on Coupled Modeling (WGCM), 2004), ENSEMBLES (Ensemble-Based Predictions of climate changes and Their Impacts), a successor to PRUDENCE which finished in 2009 (van der Linden and Mitchell, 2009) and CORDEX (Coordinated Regional Downscaling Experiment, Giorgi and Gutowski, 2015), based on data known from the Fifth Phase of the Coupled Model Intercomparison Project (CMIP5; Taylor et al., 2012). The RCMs included in the PRUDENCE project have a spatial grid spacing of 50 km, ENSEMBLES 25 km and Euro-CORDEX 12 km. With PRUDENCE, Beniston et al. (2007) (with both high and low emission scenarios A2 and B2), Schwierz et al. (2010) (with SRES1 A2 scenario), and Rockel and Woth (2007) (also with SRES A2 scenario) studied the climate change on extreme wind using the 90th, 98th, and 99th percentiles of wind speed, as well as the gust and the
To follow up these investigations, this study examines the possible climate change impact on the extreme winds using the new, CMIP6 data, here particularly the 50-year wind
The CMIP6 data are available every 6 h, which is a resolution too coarse for the purpose of calculating the extreme wind, according the IEC standard, where a temporal resolution of 10 min is needed (IEC, 2019). To relate our calculation and analysis to the IEC standard for turbine design, and hence to be able to address the impact of climate change from the perspectives of wind energy application, we downscale the CMIP6 time series of wind speed in the temporal domain using a fast and simple spectral model that is well established for wind energy applications. This method, called the spectral correction method, corrects the CMIP6 time series to an equivalent 10-min temporal resolution and it is introduced in Section 2.3. Data, including CMIP6 and reanalysis data, are introduced in Section 2, followed by the results in Section 3. The discussion of the results and the conclusions are in Section 4 and Section 5, respectively.
For readability, a list of Abbreviations is provided at the end of this paper.
2 Data and methods
We use the output from CMIP6 models to assess the climate change impact on the extreme wind over two periods: the historical period (his-Period, 1980–2009) and the near future period (fut-Period, 2020–2049) from the CMIP6 historical and SSP585 simulations, respectively (Eyring et al., 2016). These two periods are relevant for a turbine’s life time of 20–30 years for a wind farm being planned in 2020. When assessing the CMIP6 data quality, we use three reanalysis data sets and measurements from the three FINO (Forschungsplattformen in Nord-und Ostsee) masts in the North Sea and the Baltic Sea for comparison with CMIP6 from his-Period.
The CMIP6 data are introduced in Section 2.1 and the reanalysis data in Section 2.2. When calculating
2.1 The CMIP6 data
Consistent with the study of wind resources using CMIP6 data in Hahmann et al. (2022), we use the 18 models listed in Table 1 for the high emission scenario SSP585. Among the many CMIP6 models these 18 are chosen for the same reason as given in Hahmann et al. (2022): the availability of model outputs of surface pressure, temperature and humidity in addition to the wind components
To reduce the data download volume, we follow Hahmann et al. (2022) and crop the CMIP6 model data domain to approximately cover the area
2.2 The reanalysis data
The reanalysis data is used to assess the reliability of the CMIP6 wind speeds in describing extreme winds in the historic period in this region through a qualitative description of the spatial distribution and patterns of
Three reanalysis data sets are used: 1) the CFSR (Saha et al., 2010) available at hourly temporal resolution with a grid spacing of about 40 km; 2) the MERRA2 (Gelaro et al., 2017) available hourly with a grid spacing of
Wind speed diagnostics are available at different heights in the three reanalysis: 10 m (CFSR), 10 m and 50 m (MERRA2), and at 10 m and 100 m (ERA5). A single common height for comparison with hub height would be ideal. However, extrapolating wind speeds from a height, i.e., 10 m or 50 m, to a typical modern turbine hub height (
2.3 The spectral correction method and the annual maximum method
The IEC standard requires that the 50-year return wind estimate are based on time series equivalent to a temporal resolution of 10 min. Thus, we cannot use the estimation of
where
Please refer to Larsén et al. (2012) for details of the derivation. Equations 1, 2 show clearly that
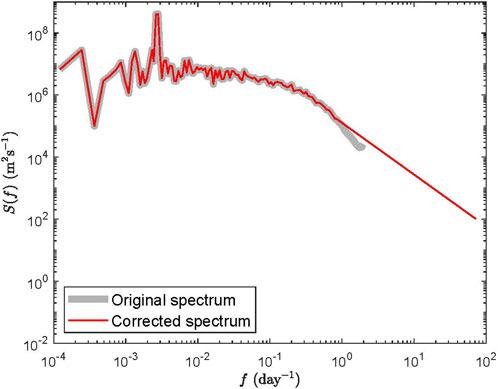
Figure 1. An example of the spectrum of the 6-hourly time series of wind speed at 100 m from the CNRM-CM6-1 model of historical period and the corrected spectrum to equivalent resolution of 10-min. The time series is from the location 56.7309
The spectral correction method uses the spectral model from Larsén et al. (2013) to estimate the tail of the distribution for
where the value of the coefficient
The spectral behavior is related to the energy transfer and cascade across atmospheric flow of different scales, following the law of physics. We assume the same set of physics laws in the future as in the historic data, and therefore do not introduce climate change in the spectra of the wind speed. This assumption should be reasonable, as demonstrated by the example of similar spectra from both the historical and future periods in Supplementary Figure S1, shown in Supplementary Appendix SA.
The spectral correction method has been applied in research and industry for
The
Here
where
The effect of using different length of time series on the calculation of
2.4 FINO masts measurements
We use the results of

Table 2. Sites and their coordinates, data period and coverage and the height where data are analyzed.
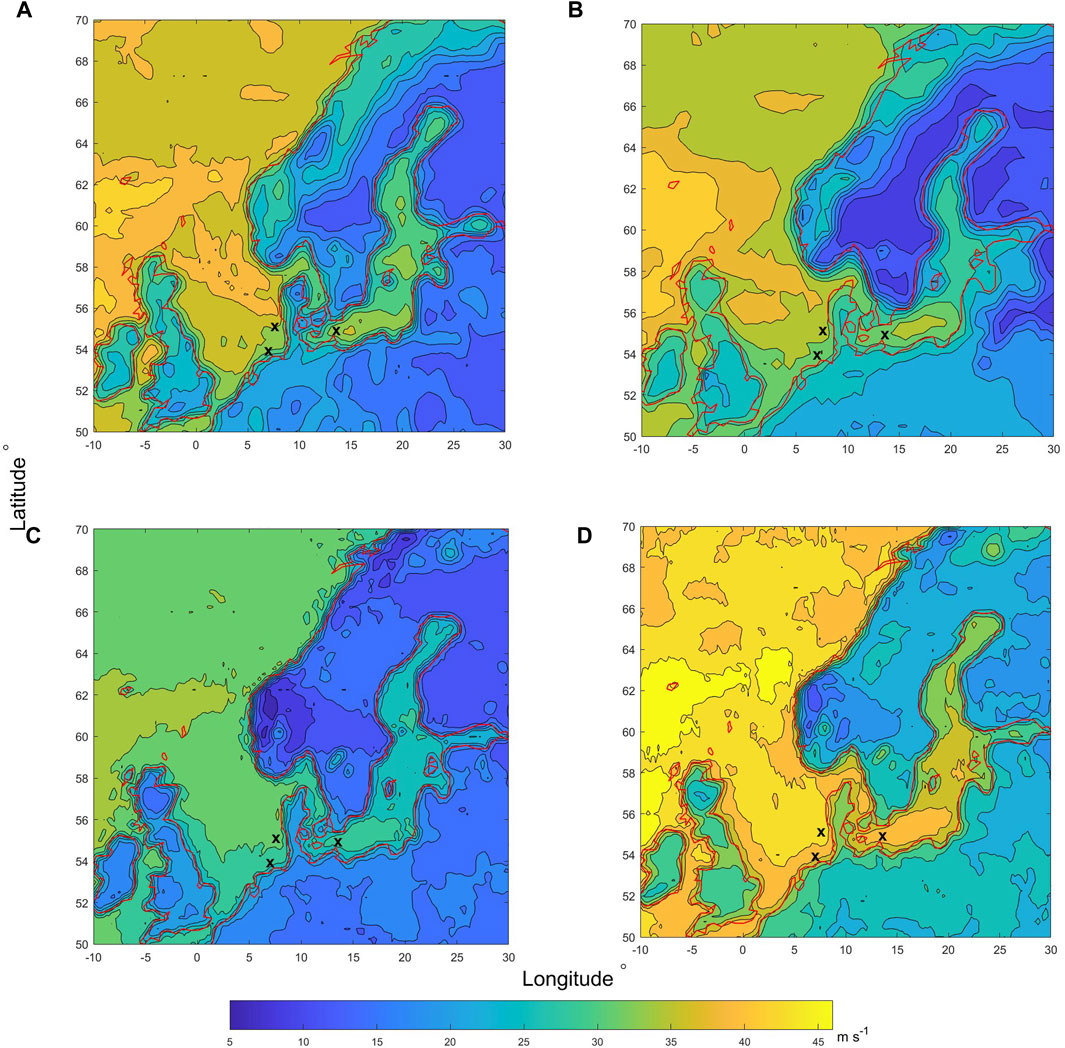
Figure 2. Spatial distribution of the spectral corrected 50-year wind
2.5 Statistical significance
When using climate model output, it is always a challenge to quantify or qualify the systematic signals and uncertainties. In climate studies, the
Following the IEC standard, turbine classes I, II, III and TC (for most severe storms such as tropical cyclones) are defined based on the threshold 10-min values of
We compute the difference between the fut-Period and the his-Period using Equations 7-10. The difference of
with
The relative difference is defined as
and mean relative difference is
A similar calculation for the second variable
where SI is for significant increase, MI is for medium increase, neutral is for no obvious change, MD is for medium decrease and SD is for significant decrease.
A probability distribution of
To roughly assess how much of the changes in
where
To help qualifying the CMIP6 model reliability, we compare the estimate of
To further assess the robustness of the results from the 18 models, we also use sub-samples from the 18 models depending on several performance indices as presented in Section 3.1.2, thus forming two additional selections of models. The statistics are compared with those from the 18 models.
3 Results
For each model grid point,
3.1 Extreme wind in the historic period
3.1.1 Reanalysis data
The 50-year wind
As mentioned in Section 1, a global atlas of
Due to the differences in the various reanalysis data it is difficult to decide which one of them is the best without a systematic validation with measurements. But a global database of such measurements does not exist. Thus, we focus on the characteristics of the wind distribution that are shared by these reanalysis data and use them to discuss the quality of the CMIP6 data for his-Period. We use the following wind characteristics that are present in the three reanalyses as basic quality check for the CMIP6 data: (a) A 50-year wind at 100 m
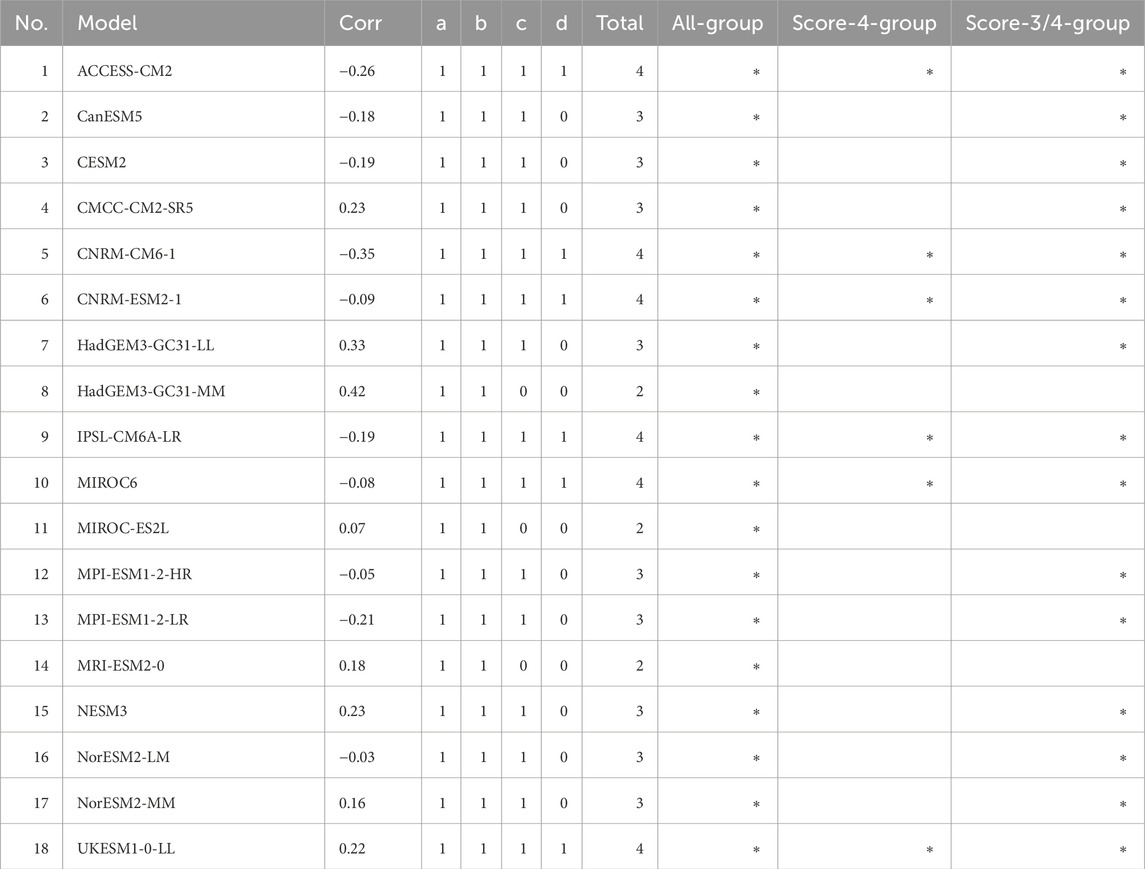
Table 4. Score of the large-scale extreme wind for the his-Period for the 18 CMIP6 models (labeled as defined in Table 1) using the four wind characteristics defined in Section 3.1.2. Also shown are the three model groups. The third column “corr” is the correlation between
3.1.2 CMIP6 data
We use the same procedure as used for the reanalysis data, e.g., through the spectral correction method, Annual Maximum Method and the Gumbel distribution fit, to calculate
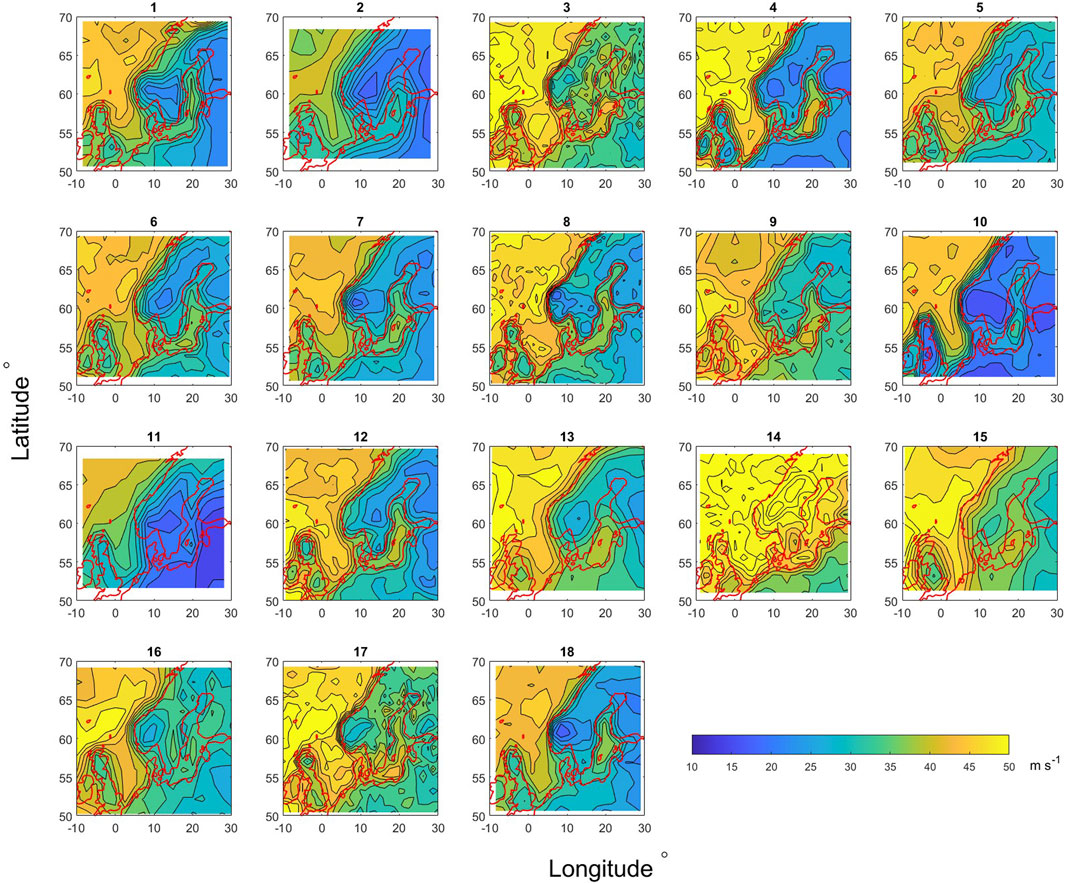
Figure 3. The 50-year wind at 100 m for the 18 CMIP6 models for the his-period,
We use the four characteristics, (a)–(d), as described at the end of Section 3.1.1 to give a score 0 or 1 to the 18 models. The results of this scoring are summarized in Table 4. The four criteria are generous, and therefore the scoring is qualitative; all models satisfy the basic quality criteria (a) and (b). In the end, we have 6 models with highest score 4 (models 1, 5, 6, 9, 10, 18) and 3 models with lowest score 2 (models 8, 11, 14). As introduced in Section 2.5, to explore the sensitivity of results to the collection of model data, in addition to the analysis using all 18 models (called “All-group”), we also perform the data analysis using two sub-groups of models: “Score-4-group” (including all models of a score of 4: 6 models), and “Score-3/4-group” (including models with a score of 3 or 4: 15 models). The model grouping is also shown in Table 4.
We validate the calculations of
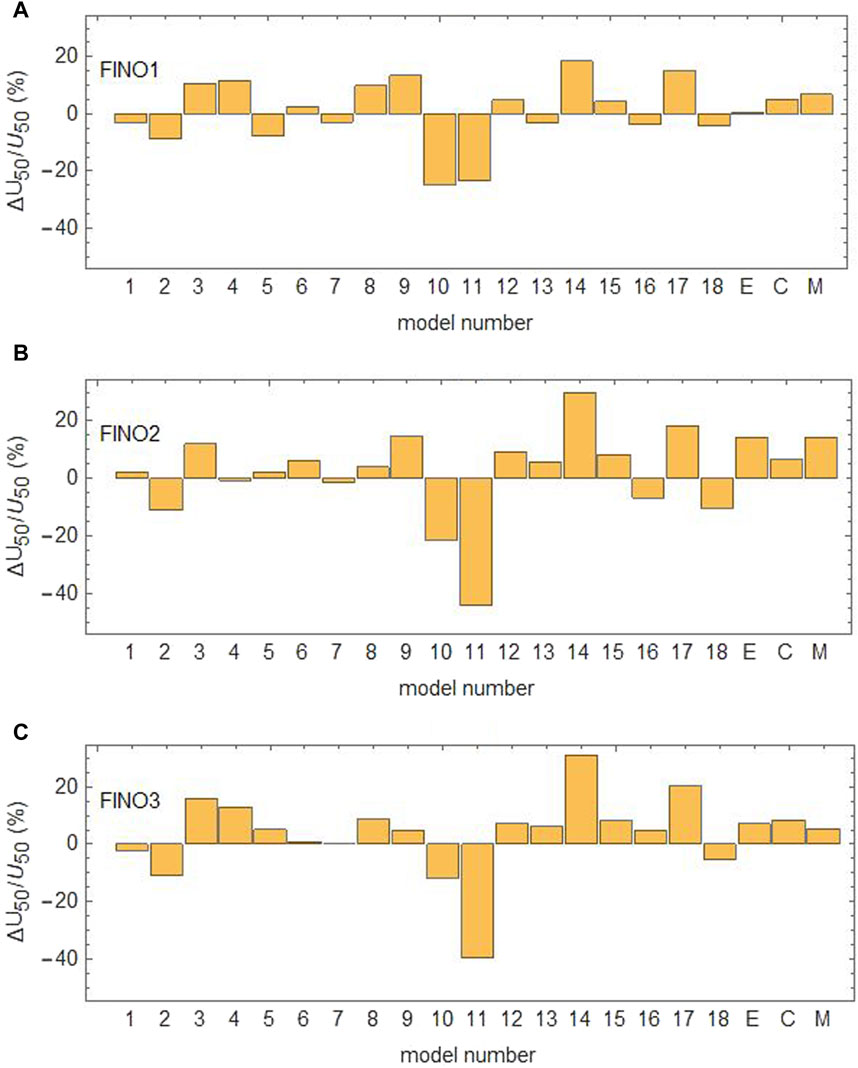
Figure 4. Comparison of
3.2 Climate change impact on the extreme wind
The effect of climate change on the extreme wind is estimated through parameters defined in Section 2.5. This includes the mean and relative differences in
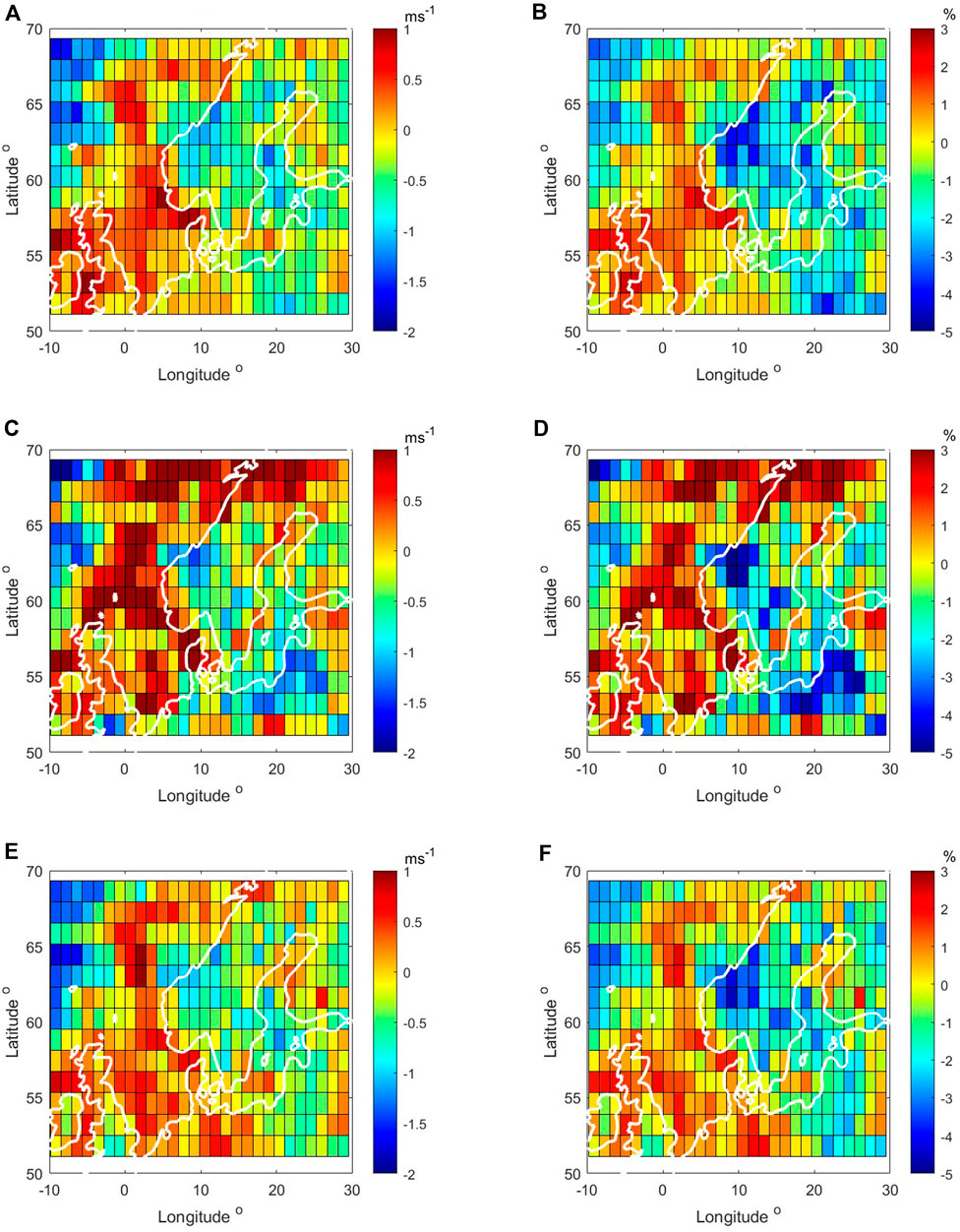
Figure 5. Spatial distribution of model mean difference
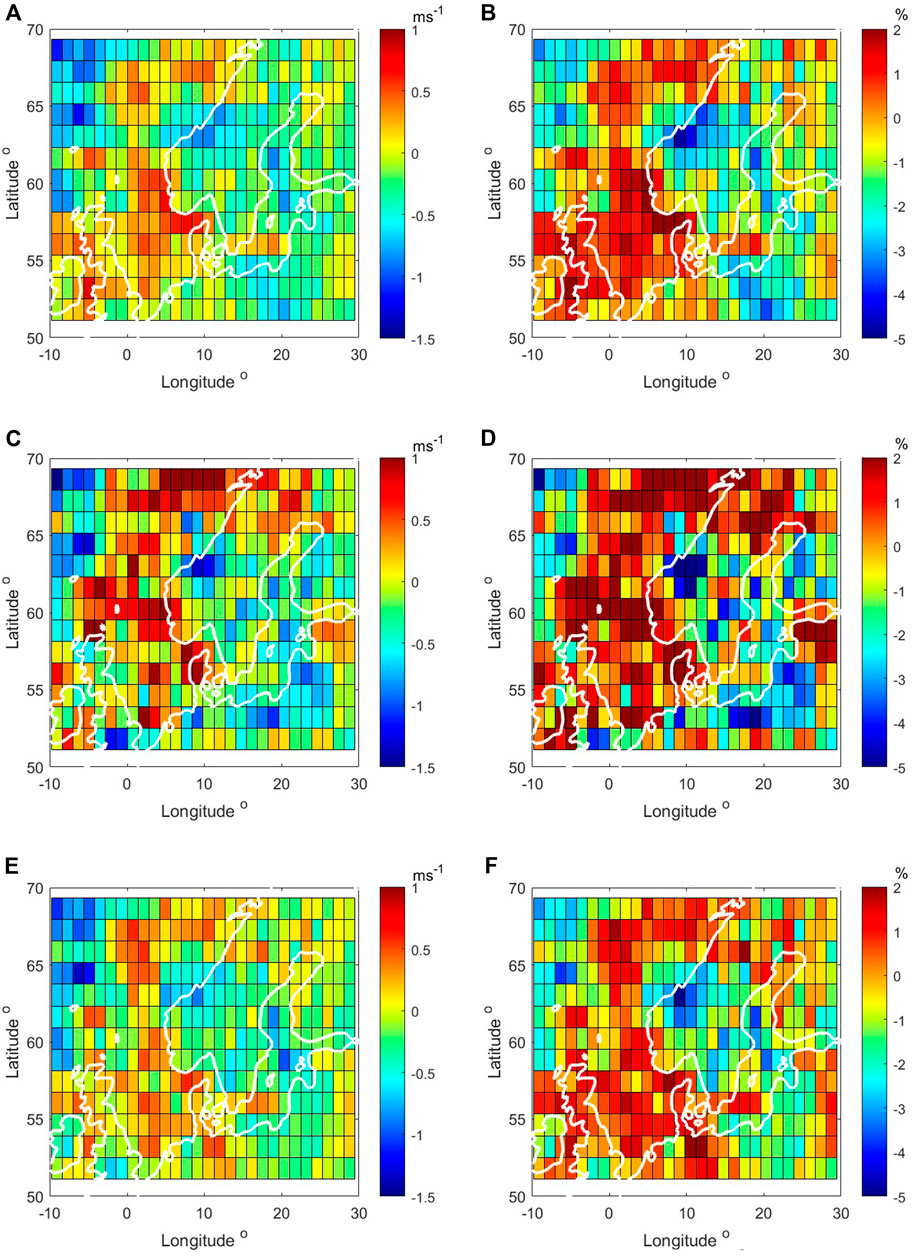
Figure 6. Same as Figure 5, but for the 95% quantile of the wind speed at 100 m. For model All-group (A, B), Score-4-group (C, D) and Score-3/4-group (E, F).
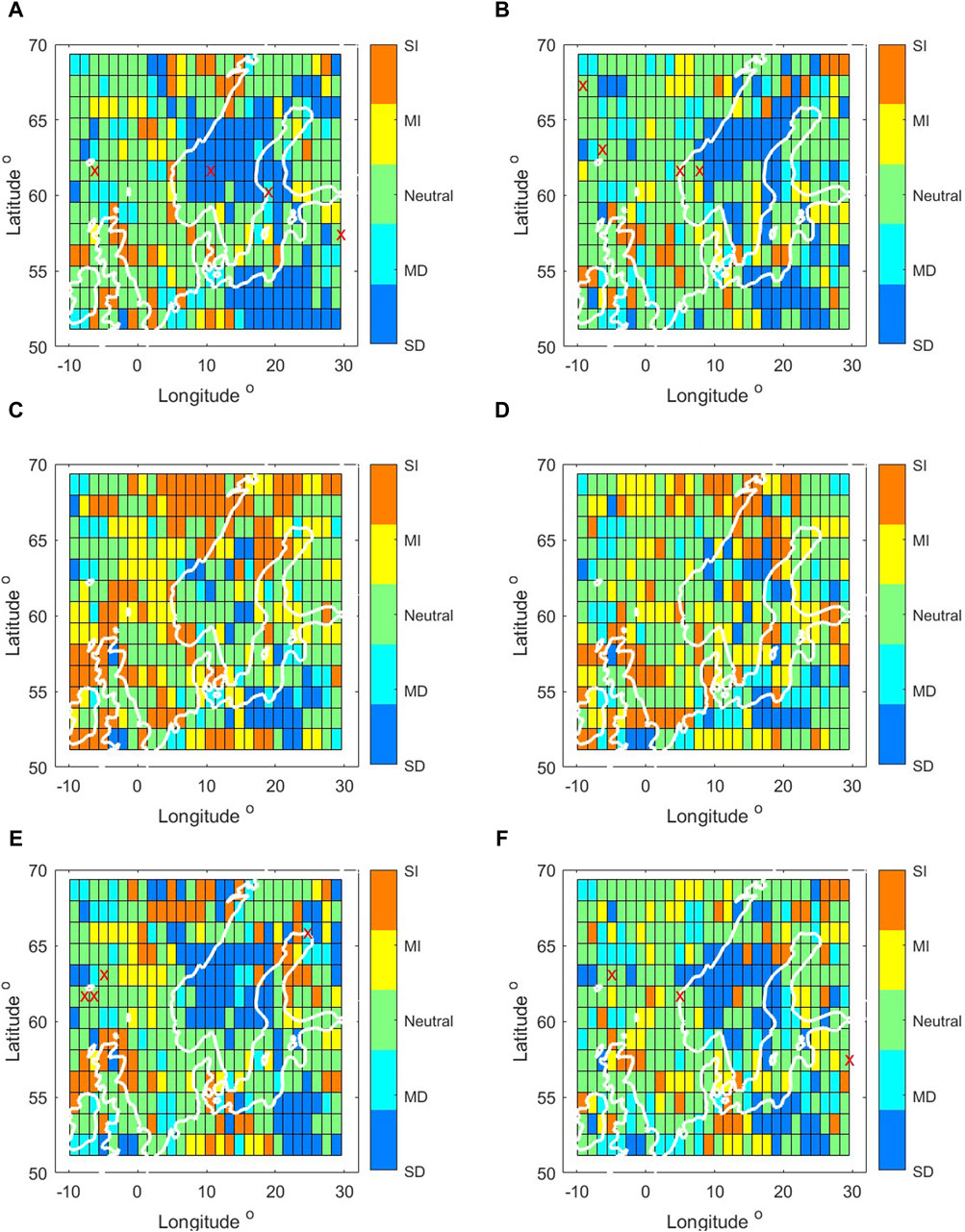
Figure 7. Range (SI–significant increase, MI–medium increase, neutral–no obvious change, MD–medium decrease, SD–significant decrease, see text) in relative change in
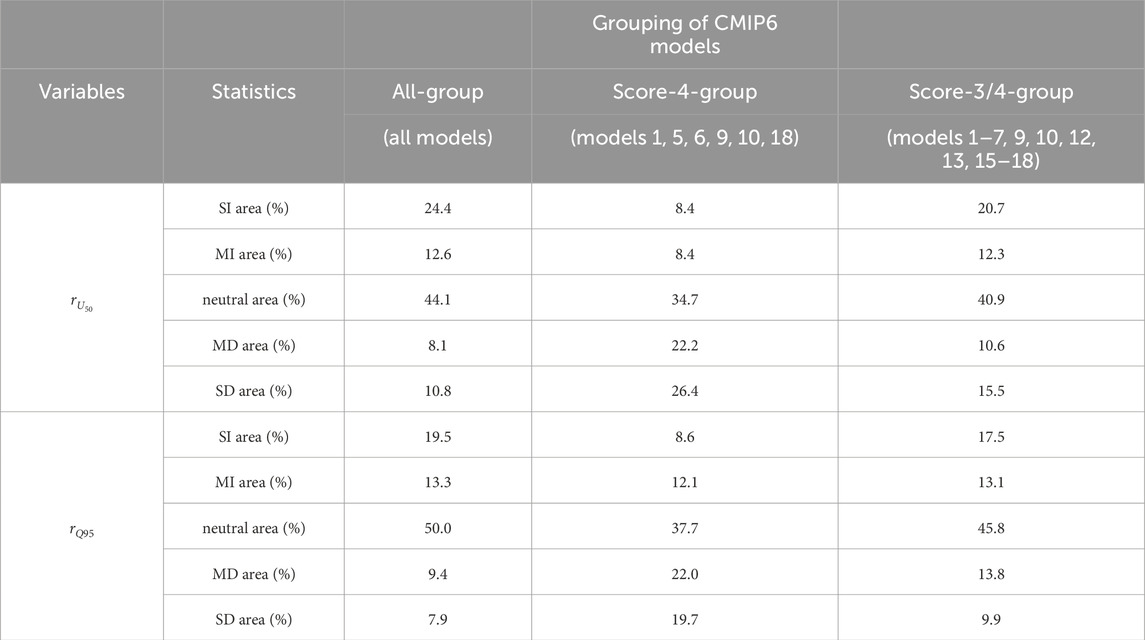
Table 5. Summary of the statistics of change in the extreme wind
We first explore the effect of climate change on
Figures 5, 6 show high consistency of the spatial patterns of
Table 5 summarizes the statistics for the three groupings. The All-group is rather similar to the Score-3/4-group, while the Score-4-group, which has only 6 models, shows slightly larger differences but consistent results. For the majority of grid points in the model domain, most models indicate no obvious change (“neutral”
The correlation coefficient between
To discuss the relevance and importance of the climate change effect on the extreme winds, we relate the extreme wind change to the turbine class as defined in the IEC standard (IEC, 2019) (Table 3). The corresponding changes in turbine class are calculated for each CMIP6 model and each grid point. When requiring the level of statistical significance for climate studies, 80% of the samples, be consistent in the signals, all grid points suggest no TCL-change. It is still so when lowering the level from 80% to 50%. Behind this conclusion lies the fact that individual models indicate locations with TCL-change rather differently, and therefore do not conform to statistical significance for TCL-change. Here we use the Score-4-group as an example; the TCL-change at 100 m in the six models are shown in Figure 8. For each grid point, the majority of the models suggest no TCL-change, but each model has its own locations for the red (stronger TCL) and blue (weaker TCL) boxes. For instance, model 1 (Figure 8A) suggests a lower turbine class in the near future over the water in the north, and a higher turbine class in part of the southern North Sea; model 9 (Figure 8D) has contradicting suggestions. Model 5 (Figure 8B) suggests higher TCL in the coast areas of Norway, Finland, Denmark, the Baltic Sea and south of the North Sea, to which model 18 (Figure 8F) has contradicting suggestions (except for the most northern part of the United Kingdom). These results suggest that in this region there is no conclusive trend in the change in the extreme winds that will provoke a shift towards the need for higher TCL in the future. A change towards a higher TCL would make wind energy development more expensive. The same analysis was performed at 50 and 200 m; the conclusions are the same as at 100 m.
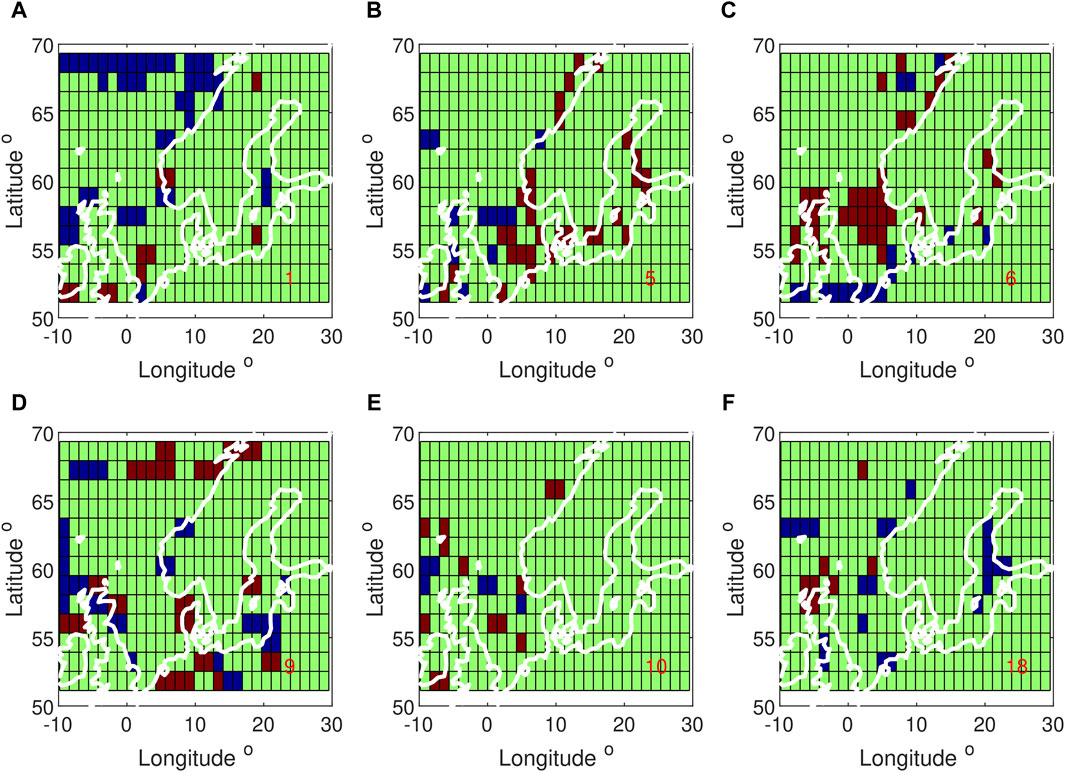
Figure 8. Change in the future TCL for 100 m in the six models in Score-4-group: (A) model 1: ACCESS-CM2; (B) model 5: CNRM-CM6-1; (C) model 6: CNRM-ESM2-1; (D) model 9: IPSL-CM6A-LR; (E) model 10: MIROC6; (F) model 18: UKESM1-0-LL. The colors indicate: one class stronger (red), one class weaker (blue) and no changes (green).
4 Discussion
We investigated the climate change effect on the 50-year wind, which is one of the key design parameters for wind turbines. We used outputs from 18 CMIP6 models, which is about one third of all models submitted to CMIP6. We also chose to use the high-emission SSP585 scenario, following Hahmann et al. (2022). Such a selection faces the question whether this collection of models is sufficient for drawing robust conclusions. The models were chosen because of the availability of a number of variables needed for deriving the wind speeds at turbine hub heights from the values at model levels (Hahmann et al., 2022). In addition, in terms of wind resources, Hahmann et al. (2022) showed good agreement with observations for the historical period for the North Sea for at least 16 of the 18 models. This is however not directly transferable to extreme winds. With this limitation, we note that the analysis and conclusions drawn here may be updated in the future when more relevant data are made available by the community. At the same time, it is worth finding out what the 18 models suggest regarding the climate change effect on the extreme wind and more relevantly to wind energy application, the change in the choice of turbine class.
A common challenge in analyzing ensemble data is to separate the “signal” from the “noise,” as signals can be buried in the group of models (e.g., Outten and Esau, 2013; Smith et al., 2020). Smith et al. (2020) pointed it out: “Quantifying signals and uncertainties in climate models is essential for the detection, attribution, prediction and projection of climate change.” Even within different reanalysis products that should be representative of the historic data, there are already significant discrepancies in their estimations of extreme winds, here, particularly over land. The measurements used here are from three offshore sites, thus evaluation based on the FINO locations would strongly underrate both the model scatter and wind extreme changes over the region as the whole. Our approach for “extracting signals” of the effect of climate change include the comparison of the large scale distribution pattern of the extreme wind between the CMIP6 model data that are consistently presented by the three reanalysis data, and the average of the increase and decrease in the extreme wind parameters in the model ensemble. It must be noted that the chosen analysis approach is based on the assumption that consistent behaviour between the CMIP6 models is an indicator for higher probability of occurrence under the given scenario (e.g., Christensen et al., 2019). This assumption is commonly used in the IPCC assessment report (IPCC, 2021). There are systematic and consistent patterns for increased and decreased extreme winds that can be identified in certain regions, for both
Among the many studies on climate change impact, the impact on extreme wind conditions does not lead to a clear conclusion. The following patterns have been identified in earlier studies using different model outputs and scenarios: (a) Overall increasing extreme wind parameters in Northern Europe (e.g., Donat et al., 2011; Pryor et al., 2012; Christensen et al., 2022); (b) Increase over the Baltic Sea (e.g., Nikulin et al., 2011; Christensen et al., 2022); (c) Small insignificant increase with large spread in the southern part of the Baltic Sea. Christensen et al. (2022) found from 72 EURO-CORDEX RCP8.5 simulations very little agreement even on the sign of wind speed change. With the current study, some key statistics can be summarized (see, e.g., Table 5), including: about 40% of domain area corresponds to no considerable change, 20% corresponds to significant increase and a slightly smaller area suggests significant decrease. Geographically, quite a large area of the North Sea corresponds to an average increase, and most part of the Baltic Sea and the Scandinavian Peninsula corresponds to an average decrease. Most analyses here also support a medium increase in southern part of the Baltic Sea. While the analysis has also shown some degree of sensitivity regarding the models used, the overall conclusions are consistent (Figures 5–7). For all five ranges of
It should be noted that here for both his-Period and fut-Period, 30-year long data are used in connection with Equations 4–6. Data length of 30-year is considered a sufficient length for the calculation of 50-year return wind for the studied area (e.g., Larsén et al., 2015). However, if an extreme wind event has a return period longer than 30-year, it could not be detected in our calculations.
We assess the importance of the effects of climate change on the extreme wind (and thus on wind turbine siting), by relating the results to the IEC standards and discuss how it affects the design criteria for turbines. There is a high diversity between models on the general analysis of extreme wind and there is no consensus anywhere in the studied domain regarding if it will be more expensive or cheaper in the future to install wind turbines (Figure 8). Most models suggest no change in turbine class over most grid points in the study area. Using different reference model grids and interpolation methods to convert different model data to common grid points do not change the conclusion. It is expected that a high resolution regional modeling downscaled from the CMIP6 data will bring much more spatial variability. The corresponding regional model output will be valuable for updating the analysis in this study. Such a downscaling to a spatial resolution of a few kilometers is a big project and it will take the community some time to accomplish. However, these regional modeling is often done with historical land use classes, which limits its usefulness to wind-related studies. This study only downscales the CMIP6 data in temporal domain using the spectral correction method, so that the calculated extreme wind of the time series matches that of a 10-min time series (requirement from the IEC standard). The spatial details, however, are thus not resolved. For the 18 CMIP6 models, the quality of the extreme wind simulation, which is assessed through the comparison of extreme wind distribution pattern with reanalysis data and comparison with values from measurements at three offshore sites, did not suggest that higher resolution gives better agreement with measurements. This is likely because the spatial resolution of all of these model data are too coarse and all the sites are offshore. These data have a spatial resolution ranging from about 80 km to a couple of hundreds of kilometers. This will of course introduce uncertainties and thus we can only discuss the effect of climate change on a large scale.
5 Conclusion
Eighteen CMIP6 ensemble members are used to assess climate change impact on extreme wind over Northern Europe. There is a large diversity of suggestions of impacts between the model members. The analysis shows an overall increase in the extreme winds in the North Sea and southern part of the Baltic Sea, but a decrease in the Scandinavian Peninsula and most part of the Baltic Sea. However, the wind climate change as projected by the CMIP6 SSP585 models does not suggest more expensive or cheaper turbines will need to be installed in the area.
Data availability statement
The datasets presented in this study can be found in online repositories. The names of the repository/repositories and accession number(s) can be found in the article/Supplementary Material.
Author contributions
XL: Conceptualization, Data curation, Formal Analysis, Funding acquisition, Methodology, Resources, Writing–original draft, Writing–review and editing. MI: Data curation, Formal Analysis, Investigation, Validation, Writing–review and editing. ÁH: Data curation, Formal Analysis, Writing–review and editing.
Funding
The author(s) declare that financial support was received for the research, authorship, and/or publication of this article. This study is supported by the GASPOC project (EUDP 64020-1043) and EU Horizon project DTWO (HE 101146689).
Acknowledgments
The ERA5 data were downloaded from ECWMF and Copernicus climate change Service Climate Data Store. MERRA2 data are downloaded from the Distributed Active Archive Center (GSFC DAAC), https://doi.org/10.5067/VJAFPLI1CSIV. FINO 1, 2, and 3 were supplied by German Federal Maritime And Hydrographic Agency (BSH). CFSR data have been retrieved from the Research Data Archive of the National Center for Atmospheric Research (https://doi.org/10.5065/D6513W89). The CMIP6 multi-model ensemble data were downloaded through the distributed data archive developed and operated by the Earth System Grid Federation (ESGF; https://esgf.llnl.gov/). We thank our colleague Andrea Hahmann for advice and comments, and for preparing the CMIP6 data.
Conflict of interest
The authors declare that the research was conducted in the absence of any commercial or financial relationships that could be construed as a potential conflict of interest.
Publisher’s note
All claims expressed in this article are solely those of the authors and do not necessarily represent those of their affiliated organizations, or those of the publisher, the editors and the reviewers. Any product that may be evaluated in this article, or claim that may be made by its manufacturer, is not guaranteed or endorsed by the publisher.
Supplementary material
The Supplementary Material for this article can be found online at: https://www.frontiersin.org/articles/10.3389/fenrg.2024.1404791/full#supplementary-material
Footnotes
1Special Report on Emissions Scenarios (SRES) published by the Intergovernmental Panel on climate change (IPCC).
References
Abild, J. (1994). Application of the wind atlas method to extremes of wind climatology. Forskningscenter Risoe. Risoe-R-722(EN).
Bastine, D., Larsén, X., Witha, B., Dörenkämper, M., and Gotschall, J. (2018). Extreme winds in the new european wind atlas. J. Phys. Conf. Ser. 1102, 012006. doi:10.1088/1742-6596/1102/1/012006
Beniston, M., Stephenson, D. B., Christensen, O. B., Ferro, C. A. T., Frei, C., Goyette, S., et al. (2007). Future extreme events in european climate: an exploration of regional climate model projections. Clim. Change 81, 71–95. doi:10.1007/s10584-006-9226-z
Boucher, O., Servonnat, J., Albright, A. L., Aumont, O., Balkanski, Y., Bastrikov, V., et al. (2020). Presentation and evaluation of the IPSL-CM6A-LR climate model. J. Adv. Model. Earth Syst. 12, e2019MS002010. doi:10.1029/2019MS002010
Brasseur, G., and Carlson, D. (2015). Future directions for the world climate research programme. EOS 96. doi:10.1029/2015EO033577
Cherchi, A., Fogli, P. G., Lovato, T., Peano, D., Iovino, D., Gualdi, S., et al. (2019). Global mean climate and main patterns of variability in the CMCC-CM2 coupled model. J. Adv. Model. Earth Syst. 11, 185–209. doi:10.1029/2018MS001369
Christensen, J., Larsen, M., Christensen, O. e. a., Drews, M., and Stendel, M. (2019). Robustness of european climate projections from dynamical downscaling. Clim. Dyn. 53, 4857–4869. doi:10.1007/s00382-019-04831-z
Christensen, O. B., Kjellström, E., Dieterich, C., Gröger, M., and Meier, H. E. M. (2022). Atmospheric regional climate projections for the baltic sea region until 2100. Earth Syst. Dyn. 13, 133–157. doi:10.5194/esd-13-133-2022
Clausen, N.-E., Larsén, X., Pryor, S., and Drews, M. (2012). Wind power, in “Climate Change and Energy Systems—Impacts, Risks and Adaption in the Nordic and Baltic countries”. Editors T. Thorsteinn, and B. Halldór (TemaNord: Nordic Council of Ministers), 147–160. No. 502 in TemaNord. Print: Arco Grafisk A/S.
Danabasoglu, G., Lamarque, J.-F., Bacmeister, J., Bailey, D. A., DuVivier, A. K., Edwards, J., et al. (2020). The community earth system model version 2 (cesm2). J. Adv. Model. Earth Syst. 12, e2019MS001916. doi:10.1029/2019MS001916
[Dataset] WCRP Working Group on Coupled Modeling (WGCM) (2004). Prediction of regional scenarios and uncertainties for defining european climate change risks and effects (prudence). Available at: https://www.wcrp-climate.org/modelling-wgcm-mip-catalogue/modelling-wgcm-mips-2/288-modelling-wgcm-catalogue-prudence (Accessed March, 2024).
Della-Marta, P., Mathis, H., Frei, C., Liniger, M., Kleinn, J., and Appenzeller, C. (2009). The return period of wind storms over europe. Int. J. Climatol. 29, 437–459. doi:10.1002/joc.1794
Donat, M. G., Leckebusch, G. C., Wild, S., and Ulbrich, U. (2011). Future changes in european winter storm losses and extreme wind speeds inferred from GCM and RCM multi-model simulations. Nat. Hazards Earth Syst. Sci. 11, 1351–1370. doi:10.5194/nhess-11-1351-2011
Dörenkämper, M., Olsen, B. T., Witha, B., Hahmann, A. N., Davis, N. N., Barcons, J., et al. (2020). The making of the new European wind atlas - Part 2: production and evaluation. Geosci. Model Dev. 13, 5079–5102. doi:10.5194/gmd-13-5079-2020
Eyring, V., Bony, S., Meehl, G. A., Senior, C. A., Stevens, B., Stouffer, R. J., et al. (2016). Overview of the coupled model Intercomparison project phase 6 (CMIP6) experimental design and organization. Geosci. Model Dev. 9, 1937–1958. doi:10.5194/gmd-9-1937-2016
Gelaro, R., McCarty, W., Suárez, M. J., Todling, R., Molod, A., Takacs, L., et al. (2017). The modern-era retrospective analysis for research and applications, version 2 (merra-2). J. Clim. 30, 5419–5454. doi:10.1175/jcli-d-16-0758.1
Giorgi, F., and Gutowski, W. J. (2015). Regional dynamical downscaling and the CORDEX initiative. Annu. Rev. Environ. Resour. 40, 467–490. doi:10.1146/annurev-environ-102014-021217
Hahmann, A. N., García-Santiago, O., and Peña, A. (2022). Current and future wind energy resources in the north sea according to CMIP6. Wind Energy Sci. 2022, 2373–2391. doi:10.5194/wes-7-2373-2022
Hajima, T., Watanabe, M., Yamamoto, A., Tatebe, H., Noguchi, M. A., Abe, M., et al. (2020). Development of the miroc-es2l earth system model and the evaluation of biogeochemical processes and feedbacks. Geosci. Model Dev. 13, 2197–2244. doi:10.5194/gmd-13-2197-2020
Hansen, B. O., Larsén, X. G., Kelly, M., Rathmann, O. S., Berg, J., Bechmann, A., et al. (2016). Extreme wind calculation applying spectral correction method—test and validation. Tech. Rep. Wind Energy Dep, DTU Wind Energy E-0098.
Hersbach, H., Bell, B., Berrisford, P., Hirahara, S., Horányi, A., Muñoz-Sabater, J., et al. (2020). The ERA5 global reanalysis 146, 1999–2049. doi:10.1002/qj.3803
Hosking, J., Wallis, J. R., and Wood, E. F. (1985). Estimation of the generalized extreme value distribution by the method of probability-weighted moments. Technometrics 27, 251–261. doi:10.1080/00401706.1985.10488049
IEC (2019). “IEC 61400-1 Ed4: wind turbines - Part 1: design requirements,” in standard. Geneva, Switzerland: International Electrotechnical Commission.
Imberger, M., and Larsén, X. (2022). Sensitivity and quality assessment of the global 50-year return winds using reanalysis products and measurements. Wind. Annu. Event. (ilbao, Spain), April, 2022. https://windeurope.org/annual2022/
IPCC (2021). Climate change 2021: the physical science basis. Contribution of working group I to the sixth assessment report of the intergovernmental panel on climate change. Cambridge, United Kingdom, and New York, USA: Cambridge University Press. doi:10.1017/9781009157896.002
Kawai, H., Yukimoto, S., Koshiro, T., Oshima, N., Tanaka, T., Yoshimura, H., et al. (2019). Significant improvement of cloud representation in the global climate model mri-esm2. Geosci. Model Dev. 12, 2875–2897. doi:10.5194/gmd-12-2875-2019
Larsén, X., Kruger, A., Floors, R., Cavar, D., and Hahmann, A. (2021). Extreme gust atlas over South Africa. EGU General Assem. 2021. online, 19–30 Apr 2021, EGU21-5829. doi:10.5194/egusphere-egu21-5829
Larsén, X., and Mann, J. (2006). The effects of disjunct sampling and averaging time on maximum mean wind speeds. J. Wind Eng. Ind. Aerodyn. 94, 581–602. doi:10.1016/j.jweia.2006.01.020
Larsén, X., and Mann, J. (2009). Extreme winds from the NCEP/NCAR reanalysis data. Wind Energy 12, 556–573. doi:10.1002/we.318
Larsén, X., Mann, J., Rathmann, O., and Jørgensen, H. E. (2015). Uncertainties of the 50-year wind from short time series using generalized extreme value distribution and generalized pareto distribution. Wind Energy 18, 59–74. doi:10.1002/we.1683
Larsén, X. G., Davis, N., Hannesdóttir, Á., Kelly, M., Svenningsen, L., Slot, R., et al. (2022). The global atlas for siting parameters project: extreme wind, turbulence, and turbine classes. Wind Energy 25, 1841–1859. doi:10.1002/we.2771
Larsén, X. G., Du, J., Bolanos, R., Imberger, M., Kelly, M., Badger, M., et al. (2019). Estimation of offshore extreme wind from wind-wave coupled modeling. Wind Energy 22, 1043–1057. doi:10.1002/we.2339
Larsén, X. G., and Kruger, A. (2014). Application of the spectral correction method to reanalysis data in South Africa. J. Wind Eng. Industrial Aerodynamics 133, 110–122. doi:10.1016/j.jweia.2014.08.002
Larsén, X. G., Ott, S., Badger, J., Hahmann, A. H., and Mann, J. (2012). Recipes for correcting the impact of effective mesoscale resolution on the estimation of extreme winds. J. Appl. Meteorology Climatol. 51, 521–533. doi:10.1175/JAMC-D-11-090.1
Larsén, X. G., Vincent, C. L., and Larsen, S. (2013). Spectral structure of mesoscale winds over the water. Q. J. R. Meteorological Soc. 139, 685–700. doi:10.1002/qj.2003
Mauritsen, T., Bader, J., Becker, T., Behrens, J., Bittner, M., Brokopf, R., et al. (2019). Developments in the mpi-m earth system model version 1.2 (mpi-esm1.2) and its response to increasing co2. J. Adv. Model. Earth Syst. 11, 998–1038. doi:10.1029/2018MS001400
Müller, W. A., Jungclaus, J. H., Mauritsen, T., Baehr, J., Bittner, M., Budich, R., et al. (2018). A higher-resolution version of the max planck institute earth system model (mpi-esm1.2-hr). J. Adv. Model. Earth Syst. 10, 1383–1413. doi:10.1029/2017MS001217
Nikulin, G., Kjellström, E., Hansson, U., Strandberg, G., and Ullerstig, A. (2011). Evaluation and future projections of temperature, precipitation and wind extremes over europe in an ensemble of regional climate simulations. Tellus A 63, 41–55. doi:10.1111/j.1600-0870.2010.00466.x
Outten, S., and Esau, I. (2013). Extreme winds over europe in the ensembles regional climate models. Atmos. Chem. Phys. 13, 5163–5172. doi:10.5194/acp-13-5163-2013
Outten, S., and Sobolowski, S. (2021). Extreme wind projections over europe from the euro-cordex regional climate models. Weather Clim. Extrem. 33, 100363. doi:10.1016/j.wace.2021.100363
Pryor, S., and Bartelmie, R. J. (2021). A global assessment of extreme wind speeds for wind energy applications. Nat. Energy 6, 268–276. doi:10.1038/s41560-020-00773-7
Pryor, S. C., Barthelmie, R. J., Clausen, N. E., Drews, M., MacKeller, N., and Kjellstrom, E. (2012). Analyses of possible changes in intense and extreme wind speeds over northern Europe under climate change scenarios. Clim. Dyn. 38, 189–208. doi:10.1007/s00382-010-0955-3
Rockel, B., and Woth, K. (2007). Extremes of near-surface wind speed over europe and their future changes as estimated from an ensemble of RCM simulations. Clim. Change 81, 267–280. doi:10.1007/s10584-006-9227-y
Saha, S., Moorthi, S., Pan, H.-L., Wu, X., Wang, J., Nadiga, S., et al. (2010). The ncep climate forecast system reanalysis. Bull. Am. Meteorological Soc. 91, 1015–1058. doi:10.1175/2010BAMS3001.1
Schwierz, C., Kollner-Heck, P., Mutter, E., Bresch, D., Vidale, P., Wild, M., et al. (2010). Modelling european winter wind storm losses in current and future climate. Clim. Change 101, 485–514. doi:10.1007/s10584-009-9712-1
Séférian, R., Nabat, P., Michou, M., Saint-Martin, D., Voldoire, A., Colin, J., et al. (2019). Evaluation of cnrm earth system model, cnrm-esm2-1: role of earth system processes in present-day and future climate. J. Adv. Model. Earth Syst. 11, 4182–4227. doi:10.1029/2019MS001791
Seland, Ø., Bentsen, M., Olivié, D., Toniazzo, T., Gjermundsen, A., Graff, L. S., et al. (2020). Overview of the Norwegian earth system model (noresm2) and key climate response of cmip6 deck, historical, and scenario simulations. Geosci. Model Dev. 13, 6165–6200. doi:10.5194/gmd-13-6165-2020
Sellar, A. A., Walton, J., Jones, C. G., Wood, R., Abraham, N. L., Andrejczuk, M., et al. (2020). Implementation of u.k. earth system models for cmip6. J. Adv. Model. Earth Syst. 12, e2019MS001946. doi:10.1029/2019MS001946
Smith, D., Scaife, A., Eade, R. e. a., Athanasiadis, P., Bellucci, A., Bethke, I., et al. (2020). North atlantic climate far more predictable than models imply. Nature 583, 796–800doi. doi:10.1038/s41586-020-2525-0
Swart, N. C., Cole, J. N. S., Kharin, V. V., Lazare, M., Scinocca, J. F., Gillett, N. P., et al. (2019). The canadian earth system model version 5 (canesm5.0.3). Geosci. Model Dev. 12, 4823–4873. doi:10.5194/gmd-12-4823-2019
Tatebe, H., Ogura, T., Nitta, T., Komuro, Y., Ogochi, K., Takemura, T., et al. (2019). Description and basic evaluation of simulated mean state, internal variability, and climate sensitivity in miroc6. Geosci. Model Dev. 12, 2727–2765. doi:10.5194/gmd-12-2727-2019
Taylor, K. E., Stouffer, R. J., and Meehl, G. A. (2012). An overview of CMIP5 and the experiment design. Bull. Am. Meteorological Soc. 93, 485–498. doi:10.1175/BAMS-D-11-00094.1
Tilo, Z., Andrew, L., Martin, D., Lenton, A., Bodman, R. W., Dix, M., et al. (2020). The Australian earth system model: ACCESS-ESM1.5. J. South. Hemisphere Earth Syst. Sci. 70, 193–214. doi:10.1071/ES19035
van der Linden, P., and Mitchell, J. (2009). Climate change and its impacts at seasonal, decadal and centennial timescales. Tech. Rep, Met Office Hadley Centre, FitzRoy Road, Exeter EX1 3PB, UK.
Voldoire, A., Saint-Martin, D., Sénési, S., Decharme, B., Alias, A., Chevallier, M., et al. (2019). Evaluation of cmip6 deck experiments with cnrm-cm6-1. J. Adv. Model. Earth Syst. 11, 2177–2213. doi:10.1029/2019MS001683
Yang, Y., Wang, B., Cao, J. e. a., and Li, J. (2020). Improved historical simulation by enhancing moist physical parameterizations in the climate system model NESM3.0. Clim. Dyn. 54, 3819–3840. doi:10.1007/s00382-020-05209-2
Glossary
Keywords: climate change, 50-year wind, CMIP6 data, IEC standard, reanalysis data
Citation: Larsén XG, Imberger M and Hannesdóttir Á (2024) The impact of Climate Change on extreme winds over northern Europe according to CMIP6. Front. Energy Res. 12:1404791. doi: 10.3389/fenrg.2024.1404791
Received: 21 March 2024; Accepted: 07 August 2024;
Published: 22 August 2024.
Edited by:
Davide Astolfi, University of Perugia, ItalyReviewed by:
Zhiping Tian, Chinese Academy of Sciences (CAS), ChinaIgor Esau, Nansen Environmental and Remote Sensing Center (NERSC), Norway
Rui Chang, China Meteorological Administration, China
Copyright © 2024 Larsén, Imberger and Hannesdóttir. This is an open-access article distributed under the terms of the Creative Commons Attribution License (CC BY). The use, distribution or reproduction in other forums is permitted, provided the original author(s) and the copyright owner(s) are credited and that the original publication in this journal is cited, in accordance with accepted academic practice. No use, distribution or reproduction is permitted which does not comply with these terms.
*Correspondence: Xiaoli Guo Larsén, eGdhbEBkdHUuZGs=