- 1Electrical and Electronics Engineering, Velagapudi Ramakrishna Siddhartha Engineering College, Vijayawada, India
- 2Electrical and Electronics Engineering, Vardhaman College of Engineering, Hyderabad, India
- 3Computer Science and Engineering Department, Spoorthy Engineering College, Hyderabad, India
- 4School of Electronics Engineering, VIT-AP University, Amaravati, India
- 5Faculty of Information Technology and Electrical Engineering, Norwegian University of Science and Technology, Trondheim, Norway
- 6School of Physics, Engineering and Technology, University of York, York, United Kingdom
- 7Fukushima Renewable Energy Institute, AIST (FREA), Koriyama, Japan
Profit maximization is crucial for both producers and customers in power grids, particularly in deregulated energy markets. By focusing on profit maximization measures, power grids can improve economic viability and sustainability for all stakeholders. The growing demand for electricity in modern civilization poses a significant challenge to the power grid’s ability to meet it. To address these difficulties, the power industry is attempting to integrate renewable sources into existing power facilities. Because of its unpredictable character, the presence of renewable energy in the electricity system becomes increasingly important and adds complexity to the grid. Given the intermittent nature of renewable sources, an energy storage device is required in this scenario. During the low-power use phase, the grid has ample power. Meanwhile, energy storage devices can be utilized to store extra power in the low power demand phase while maintaining the safety and stability of the power network during peak demand periods. V2G (Vehicle-to-Grid) technology is commonly employed to address the uncertainty of renewable resources while maintaining system stability. By using automobiles as transportable storage devices, V2G can make the best use of excess electricity during low-energy hours. Wind energy’s volatility causes imbalances, lowering system profitability. With wind energy integration, power system profitability can be improved by analyzing uncertainties and employing mitigation strategies. To overcome the issue, the research optimizes the use of V2G and TCSC (thyristor-controlled series compensator). V2G permits bidirectional power flow, lowering imbalance pricing (IP) while considering power demand. TCSC can increase the transient stability of transmission lines, hence reducing line faults. TCSC’s involvement in the system increases profitability by lowering congestion expenses. Strategic TCSC placement increases overall profitability. The approach strives to offer efficient, environmentally friendly power at a reasonable price while addressing the needs of both power providers and customers. This study also looks into the effects of V2G mixing on voltage stability, LMP (locational marginal price), and the effectiveness of a TCSC in a deregulated electrical system. MiPower software is used to simulate operation and find the optimal placement of the storage unit within the IEEE 14-bus system.
1 Introduction
The thermal power plant is regarded as the principal source of electricity generation. This power plant requires a lot of fossil fuel for combustion. Fossil fuels emit massive amounts of CO2, causing global warming (Chauhan et al., 2021). This urges world leaders to integrate renewable energy sources, which will decrease the depletion of nonrenewable resources (Latif et al., 2020). Wind power is often regarded as one of the most efficient renewable energy sources due to its multiple advantages and possible applications (Dey et al., 2020). Gamel and Sultan (2023) emphasized wind energy as a cost-effective and fast-growing technology that contributes to resource conservation, rural power system enhancement, and carbon emission reduction. Nonetheless, because renewable sources are not dispatchable, energy storage facilities are required to retain any excess energy generated during low-demand periods (Hussain et al., 2020a). This stored energy can then be used during peak demand. Choosing the best location for renewable sources and energy storage facilities is critical to maximizing the system’s profits. To maximize economic value, the power environment is shifted from regulated to competitive (Das A. et al., 2022a). The deregulated system refers to an environment in which competitive power exists. In a regulated system, the consumers have no choice as it is a monopoly type of system where all the laws and rules are set by the government and no private bodies can be involved. This results in the electricity system being dominated by a single company. The absence of choice has led to an increase in the burden for consumers. However, the deregulation environment has provided them with a beneficial option. In a competitive electrical system, customers have the freedom to select their power producers based on both production cost and quality (Farooq et al., 2022). The power generating companies try to provide the best quality and pricing. The incorporation of wind farms is crucial to alleviate the burden on thermal power plants. The volatile nature of wind plants is challenging when combined with thermal power plants, as it can result in system instability and security concerns. To address this issue, V2G technology can be employed to support the electrical grid during periods when renewable energy sources are not accessible (Nsonga et al., 2017).
Wind power offers numerous advantages in the power sector, making it a valuable renewable energy source. It is the cleanest form of electricity, contributing significantly to reducing greenhouse gas emissions (Choubey, 2019; Das et al., 2023). The expansion of wind power, along with solar PV, is crucial for reducing emissions, requiring increased power sector flexibility through geographical balancing and electricity storage (Zietsman et al., 2022). Countries like China, India, and Brazil are aggressively adopting wind energy to improve their energy matrices, with China being the largest producer of wind power globally. Wind power presents a feasible solution to electricity problems, especially in regions with high wind density like coastal areas (Ma et al., 2015). Wind power exhibits an unpredictable and stochastic nature due to the variability and uncertainty associated with wind characteristics, impacting power system operations significantly (Barik et al., 2021).
Energy storage incorporation in power systems can effectively store and manage energy for various applications, enhancing overall system performance and reliability (Thakre et al., 2023; Tappeta et al., 2022). The V2G concept involves electric vehicles (EVs) interacting with the power grid to provide services like returning electricity to the grid or regulating its power, benefiting car owners, power firms, aggregators, and society (Mojumder et al., 2022). Ustun et al. (2021) focuses on optimizing charging and discharging control algorithms to maximize system stability while meeting constraints within the V2G system. The transition to electric vehicles is driven by factors like global warming awareness and the need to reduce carbon footprints, leading to the exploration of V2G technology where parked vehicles can generate electricity for the grid, potentially offsetting parking costs (Naja et al., 2023). The vehicle-solar-grid (VSG) integration concept utilizes solar inverters and EV batteries to provide ancillary services to the grid, demonstrating practical implementation and revenue generation possibilities (Nguyen et al., 2023). This technique is a component of an intelligent power grid (smart grid) that utilizes information technology to regulate energy usage. The benefits of EVs are two-fold. To begin with, the ability to charge in both directions and engage in V2G interactions enables the enhancement and intelligent scheduling of a building’s energy management system without the need for massive construction, hence avoiding significant capital costs. Secondly, bidirectional charging reduces operational electricity expenses.
The paper (Bilal et al., 2023) deliberates on the technical and economic feasibility of grid and renewable-powered energy systems for electric vehicle charging stations in India, with an emphasis on lowering prices and guaranteeing power supply stability. Jirdehi and Tabar (2023) focuses on establishing the best allocation of battery charging and swapping stations combined with wind and solar units to minimize overall cost and voltage variation in the long-term growth planning of EV infrastructures. A unit commitment model for a V2G system connected to a smart power grid has been considered with varying degrees of plug-in electric vehicle (PEV) penetration in Egbue et al. (2022). The model combines regulated charging and discharging, as well as accounting for battery deterioration, and demonstrates that efficient scheduling of PEVs reduces generating costs while leveling the load profile. By combining EVs and other distributed energy resources, the research (Armaghan et al., 2023) suggests a short-time optimization technique to improve a smart distribution network’s resilience during inclement weather. Different EV kinds, management programs, and uncertainties such as EV mobility, state of charge, arrival/departure time, and weather-related problems are all taken into consideration by the technique. The basic goal of this proposition is to make it easier to provide electricity to thermal power plants at considerably lower prices while also maximizing the multiple benefits that society stands to gain from this novel method (Abdolrasol et al., 2021). During an emergency, the use of local resources to meet local power demands can have a significant influence. Plug-in electric vehicles (PEVs) can generate portable power, while distributed generators (DGs) such as solar panels or wind farms can also provide electricity (Hussain et al., 2020b). Shehata et al. (2022) introduced AG-PSO-GWO, a novel combination of heuristics used in a multi-objective framework. The primary purpose of this technique is to optimize the positioning of FACTS controllers in power grids to reduce active power system losses, voltage variation, and total operating expenses. Basu et al. (2023) proposed an optimization technique to find the best site and parameter configurations for TCSC in a deregulated electricity market. The usage of TCSC was designed to resolve issues raised during the installation of wind turbines in the system. Dawn et al. (2023) proposed a profit expansion scheme by optimal placement of TCSC in the presence of disequilibrium price.
The authors (Singh and Jaiswal, 2021; Das S. S. et al., 2022) demonstrated how TCSC can increase the transient stability of transmission lines and Available Transfer Capability (ATC). The system’s overall damping torque can be increased by strategically putting TCSC controllers in power networks, such as on certain transmission lines, resulting in improved transient stability and fewer oscillations (Pereira et al., 2022; Nkan et al., 2023). Duana et al. (2023) describes initialization-free distributed algorithms for economic dispatch problems in microgrids. They also present techniques for modeling economic dispatch as distributed optimization problems, which ensure finite-time convergence without requiring a specific setup. A multilayer optimization technique for the daily scheduling of combined heat and power (CHP) units with integrated electrical and thermal storage takes into account a variety of elements to improve system efficiency, as stated by Hamoudi et al. (2023). A novel COVID-19-based optimization algorithm is created in Safiullah et al. (2022) for a hybrid microgrid to reduce system disturbances in a dynamic dispatch problem. The microgrid includes various traditional power sources, renewable energy, and energy storage batteries, all following supply-demand balance, capacity, and ramp-rate constraints. An adaptive and lightweight defect detection model called DGNet is proposed in Lei et al. (2023) to reduce costs and enable real-time detection with limited computing resources. The model includes an adaptive lightweight backbone network and a feature fusion network to maintain accuracy while reducing parameter redundancy. A regulation operation framework for PEV aggregator is proposed in Hou et al. (2017). PEVs can offer regulation services while charging. The objective function accounts for the impact of regulation services on PEV battery charging energy and system constraints. It aims to maximize aggregator earnings. An algorithm is suggested for scheduling PEVs by the aggregator. Hu et al. (2024) introduces a new method for optimizing the daily scheduling of combined heat and power units by integrating electrical and thermal storage systems while considering security constraints.
The developed Hyperparameter Bayesian Optimization of Gaussian Process Regression is applied to an asymmetric double-star induction generator (Hamoudi et al., 2023). Experimental analysis shows its effectiveness in autonomous wind energy conversion systems under varying magnetizing inductance even with the lack of a speed sensor wind turbine gearbox and bearing faults. The study (Amir et al., 2023) identifies potential technologies, operational frameworks, comparison analysis, and practical characteristics. The study offers valuable information on global economic effects, global environmental effects, organization resilience, key challenges, and energy storage technology projections. An optimally tuned gated recurrent unit neural network-based state of health estimation scheme is suggested in Rao et al. (2024) for estimating the state of health in lithium-ion batteries which are widely used in EVs and V2G systems. To estimate health parameters, a vast quantity of data, such as voltage, current, and temperature, is gathered. The data is resampled using the superior Fourier Resampling method and then fed to a machine-learning algorithm. The study (Nadeem et al., 2019) discusses how communication technologies different standards, and protocols can be utilized to integrate distributed energy resources, including EVs.
Several researchers have already studied risk management, however, this study addresses certain crucial issues that have not been addressed before. Table 1 summarizes the research and technological gaps discovered in the literature.
The novelty and contributions of this paper are as follows:
• The research focuses on enhancing the profitability of a deregulated electricity market that includes wind farms by tackling imbalance pricing. By integrating renewable energy sources into power systems, there is a notable potential for increased profitability, particularly in contrast to regulated markets.
• Wind energy’s inherent variability poses a considerable challenge, leading to systemic imbalance costs that directly affect overall profitability. This study emphasizes the importance of accurately quantifying uncertainty and developing analytical strategies to mitigate these issues, aiming to boost the profitability and efficiency of power systems that utilize wind energy.
• The main objective of this research is to address these challenges through the effective implementation of the V2G technique and the optimal placement of TCSC.
• The V2G approach helps to resolve pricing imbalances by facilitating two-way power flow between electric vehicles and the grid, while also considering the power demand within the system. Meanwhile, TCSC contributes to profitability by reducing costs linked to system congestion. Thoughtful placement of TCSC can further enhance overall profitability.
• Ultimately, this strategy aims to deliver the most efficient and eco-friendly power at the lowest possible cost, while satisfying the needs and expectations of both energy providers and consumers.
This problem is solved in three stages to test the suggested method’s robustness against the considered objective functions. The initial stage of the system consists of load in the standard IEEE 14 bus system. The second stage of the system consists of base load and wind energy generation. In the third stage, the system includes a base load, wind power, V2G, and TCSC hybrid systems.
1.1 Motivation of the work
The fundamental goal for performing this research is to improve the financial sustainability and general stability of deregulated electrical networks that rely on wind energy as a primary source. The inherent unpredictability and variability of wind energy resources present significant challenges that must be overcome, necessitating the development and implementation of pioneering and forward-thinking strategies such as the integration of Vehicle-to-Grid (V2G) technology and the strategic deployment of Thyristor-Controlled Series Compensator (TCSC) infrastructure. Both of these unique technology solutions are specially planned and constructed to reduce system imbalances, lowering congestion-related costs, and improve power flow via the grid network. This extensive and in-depth research focuses on delivering efficient, environmentally sustainable power generation at a competitive and favorable cost for both electricity suppliers and end users. The suggested technique and strategy are primarily focused on improving the economic feasibility and environmental sustainability of modern power grids, assuring their long-term viability and resilience in the face of changing energy landscapes.
1.2 Scope of this study
The wide scope of this study endeavor necessitates the investigation of various crucial aspects related to the introduction of wind energy into deregulated electricity grids. The major goal is to increase profitability and improve system efficiency.
• Within the field of profit maximization, the primary goal of this research is to design techniques for profit-maximizing within a wind-integrated unregulated system. This procedure entails a thorough examination of a wide range of elements that impact system profitability, including but not limited to revenue sources, generating costs, and the dynamics of imbalance pricing.
• This paper examines the exploration and prospective use of V2G technologies, which serve as feasible means for minimizing the financial risks inherent in power generation imbalances.
• The study investigates the strategic deployment of TCSC in the electrical grid, to determine the best placements for these components. Such planned placement is critical to improving the system’s overall stability and efficiency, notably in managing power flow and reducing energy losses during transmission.
• The main theme of this study is devoted to a thorough examination of imbalance pricing and its tremendous influence on the system’s overall profitability. The analysis sheds light on the complexities of both positive and negative imbalance pricing, revealing their far-reaching ramifications for renewable energy resource producers functioning in the market.
• The research considers the complex regulatory frameworks that govern deregulated markets, with a focus on the critical role that independent system operators play in regulating electricity system operations. This detailed assessment is critical for acquiring a thorough knowledge of how market dynamics impact consumer advantages and the price mechanisms that govern energy transactions.
• The overall purpose of this research is to provide significant insights into the integration of renewable energy sources, particularly wind power, into current power systems. This study intends to pave the path for a more sustainable and efficient energy environment by tackling the numerous difficulties and possibilities given by renewable energy generation’s inherent unpredictability.
2 Mathematical modelling of components
This section will provide comprehensive evaluations of the mathematical modeling of wind farms, V2G systems, and the TCSC static model.
2.1 Wind farm (WF)
WF’s functioning is dependent on the wind rate in that region. In this work, wind velocity info was first gathered in Vijayawada, Andhra Pradesh, India. After that, real-time data on current wind speed (CWS) and estimated wind speed (EWS) was assembled at an altitude of 10 m. The study also took the average wind turbine hub elevation (h) in India, as 120 m. Equation 1 can be used to compute the rate of wind at 120 m.
The generated power (WP) of a wind farm may be calculated using Equation 2, where Wsh indicates the rate of wind at height h, Ws10 represents the wind rate at 10 m, and N is the Hellman factor, which is equivalent to 0.143.
AD, a, and e denotes the volume of air, turbine sweep area, and the wind farm’s efficiency, respectively. In this scenario, AD is 1.226 kg/m3, e is 0.51, and the turbine rotor radius (r) is 40 m. According to the work, the initial cost for a wind farm is $3.75 per MWh.
2.2 Vehicle to grid system (V2G)
Power flows in both directions in a V2G system, necessitating the use of regulated converters to aid this operation. The total power generated by a V2G system is discussed in the sections. During the charging process, power is transferred from the grid to the battery of the electric vehicle (EV). As mentioned in Equation 3, the EV is charged at a bus station that offers a low locational marginal price (LMP), thereby enhancing the economic viability of the entire system.
The electricity transferred from the EV battery to the electrical grid in Equation 4 considers the combined average efficiency of the battery and the converter. It is critical to adhere to efficiency restrictions to ensure the converters’ stable operation and to avoid completely exhausting the battery.
The power demand at the ith bus is represented by PDi, while pdi denotes the power demand specifically at that bus. Additionally, C refers to the capacity of the EV battery that requires charging.
E denotes the battery’s efficacy, PDi indicates the total power requirement at the ith bus, and pdi represents the current power need at the ith bus. C represents the charging potential of the EV battery. In EVs, different kinds of batteries are utilized. Table 2 shows the parameters linked to several battery types that are suitable for V2G operations.
The presented work effectively outlines a detailed range of information related to the various costs associated with the implementation of V2G systems. It covers not only the operational expenses but also the physical costs that arise in this context.
2.2.1 Charging and discharging costs
During the charging phase, electricity is drawn from the grid at a relatively low locational marginal price (LMP), while in the discharging phase, power is fed back into the grid at a higher LMP, thus optimizing potential profits for the involved parties. Moreover, the efficiency of these processes depends on the type of battery technology used, which may include lead-acid, lithium-ion, or super-capacitors.
2.2.2 Physical costs
The work highlights the investment costs associated with different types of EV batteries, offering a thorough examination of the financial aspects involved. For example, the investment cost for lithium-ion batteries generally ranges from $1,250 to $1,500 per kilowatt-hour (kWh), while lead-acid batteries typically cost between $400 and $600 per kWh. These physical costs are crucial in shaping the overall financial model, as they encompass both aspects.
2.2.3 Battery life and efficiency
Battery life and efficiency play a vital role in determining the economic practicality and success of V2G systems. For instance, lithium-ion batteries are known to have an efficiency rate of about 90% and can last around 15 years. On the other hand, super-capacitors, while boasting the highest efficiency rates, come with higher installation costs. The efficiency of both charging and discharging cycles greatly affects the overall profits of the system, especially when considering the effects of energy storage over long periods.
2.3 TCSC modelling
TCSC, also known as a FACTS device, is used to enhance power system stability and control. Its principal role in an electrical system is to expand the capacity of transmission lines for power transfer. This is accomplished by injecting an adjustable capacitive reactance into the line, which helps to reduce voltage instability while raising the power transfer limit. TCSCs can help to reduce power system oscillations and increase transient stability. It accomplishes this by providing the system with variable impedance, which may be adjusted to deliver or absorb reactive power as needed to keep the system stable. The installation of a TCSC in a power system can improve power transfer capacity, boost system stability, and lower power losses (Dawn and Tiwari, 2016). These benefits can lead to additional efficient and reliable action of the power system, which ultimately benefits both utilities and customers.
The TCSC’s control typically consists of a voltage regulator that monitors the connection point voltage and a phase-angle controller. To control power flow and increase system stability in high-voltage gearbox systems, thyristor firing angles are adjusted in real-time to maintain the needed level of reactance. A TCSC is a simple and effective way of managing power flow, as seen in Figure 1 with a transmission line. After connecting the TCSC, the reactance of the transmission line (ReacLine) can be calculated using Equations 5, 6.
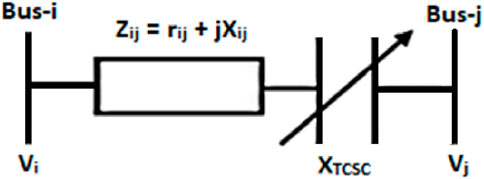
Figure 1. TCSC static model (Dawn and Tiwari, 2016).
The reactance of the line before the placement of TCSC is represented by ReacLine, whereas the reactance of the TCSC devices is represented by XTCSC. The compensation level range for TCSC (CLTCSC) is between −0.7 and 0.2.
3 Proposed work description
In the regulated system, there are no different market players. It is a monopoly type of system where all the rules and regulations are setup by the government particulars. And it is also executed by market players only. So, the customer needs to pay the energy prices, which are decided by the power producers. But there is a market controller (i.e., independent system operator) present in the deregulated system to govern the complete power system. The customers in this particular scenario can enjoy different advantages that bring about positive outcomes for them like, low power costs, ensuring that they can save money on their electricity bills. Additionally, they are also able to benefit from the presence of reliable power quality, which ensures that their electrical devices and appliances function properly and efficiently. Furthermore, another advantage that these customers experience is the presence of a stable voltage profile, that there are no sudden fluctuations or disruptions in the voltage levels of their electricity supply. In a renewable integrated system, the operation of the electrical network is carried out through the concept of power scheduling. It involves the renewable power plant being responsible for providing the market operators with a potential generation scenario for the future. This generation scenario serves as the foundation for initiating the process of power scheduling. It's worth mentioning that the power generation of a renewable power plant may differ from the initial data due to the unpredictable nature of renewable energy sources. This difference between the submitted data and the actual power generation is referred to as the imbalance price.
The imbalance in pricing affects the overall profit of the system. It is important to understand that renewable power producers are granted awards for their surplus power contribution to the grid. These awards are given to them when their power contribution exceeds the amount that was initially submitted in the data. This surplus power contribution is represented by what is known as the positive imbalance price. On the other hand, the negative imbalance price arises when there is insufficient power from WF to the electrical grid. This means that the renewable power plant is not able to meet the required power demand, which results in a negative impact on the overall profit of the system. The system profit, which is denoted as SP(p), is contingent upon several factors. At a given time p, there are several factors to consider, such as the revenue RC(p), the generation cost GC(p), and the imbalance price IP(p). It is important to consider all of these factors when determining the system’s profit. In this paper, the expansion problem is considered as the objective function for maximizing profit. It is important to note that the expansion problem is addressed in Equation 7 of the paper. The objective of maximizing profit is of utmost importance in this context, as it allows for the system to operate efficiently and effectively. By maximizing profit, the system can achieve its goals and objectives in the most optimal manner possible.
The revenue has embraced two portions, i.e., thermal plant revenue
At a specific time p, the function PTher(m,p) is used to symbolize the power that is generated thermally, whereas the symbol LMP(m,p) is utilized to indicate the Locational Marginal Price of the mth thermal generator. The quantity of generators present within the system must be accurately represented by the symbol N. The present wind power, commonly referred to as CWP, and the estimated wind power, known as EWP, play a crucial role in calculating the system imbalance price. If you want to find out the imbalance price of an electrical network that includes wind power, you can consult Equations 10–13 to get the right expression.
At time p, the wind plant’s additional rates and shortage rates are denoted by ER(p) and DR(p) correspondingly. ER(p) represents the additional rates, while DR(p) represents the shortage rates. The wind power generated for the mth unit is represented by EWP(m,p) and CWP(m,p) based on the estimated wind speed and the current wind speed. EWP(m,p) represents the wind power generated based on the estimated wind speed, while CWP(m,p) represents the wind power generated based on the current wind speed. The symbol ∝ is used to represent the imbalance price coefficient. The imbalance price coefficient is an important factor in determining the price for the imbalance between the generated wind power and the system demand. The system generation cost is defined using Equation 14. Equation 14 provides a mathematical representation of the system generation cost, taking into account various factors such as the wind power generated, the wind speed, and the imbalance between the generated power and the system demand. In summary, at time p, the wind plant’s additional and shortage rates, as well as the wind power generated for each unit, are represented by specific symbols and equations, and the system generation cost is calculated using a defined equation.
In this particular study, we will be considering the thermal production cost (GCTher(p)) as in Equation 15 as well as the wind farms’ investment cost (GCWT(p)) at a specific time ‘p'. Additionally, we will also be taking into account the price coefficients of the thermal plant, namely, am, bm, and cm. With the use of the IEEE 14 bus system, we will be carefully analyzing the impact of integrating wind generators at different locations within the power grid. Through this analysis, we will be examining various aspects such as voltage, LMP, generation costs, and profits, all within regulated systems. Furthermore, we will be exploring how the introduction of a deregulated environment can potentially enhance the profitability of the energy system. By investigating the competitive power systems that use demand-side bidding, we hope to gain insights into the potential for higher returns compared to regulated systems. Consequently, renewable energy sources will be strategically selected to maximize the benefits offered by the power system in this specific scenario as well.
3.1 Constraints for resolving of power flow problem
To carry out Optimal Power Flow (OPF) solutions, it is essential to take into consideration a variety of continuous and discrete constraints. These constraints play a pivotal role in ensuring the security and operational limits of the system are maintained. The representation of transmission loss and power demand is denoted by Ploss and PL, in Equations 16, 17 correspondingly. The line conductance of the connection between buses ‘x' and ‘y' is indicated by Gi. Furthermore, the voltage magnitude of bus ‘x' and bus ‘y' are represented by '|Vx|' and '|Vy|' respectively. Similarly, the voltage angle of bus ‘x' and bus ‘y' are denoted by 'δx' and 'δy' respectively. All these concepts are represented in Equations 18–22:
The bus ‘m’ is equipped with both lower and upper voltage limits, denoted as ‘Vmmin’ and ‘Vmmax’ respectively. These limits serve as boundaries to ensure that the voltage at bus ‘m’ remains within acceptable levels. Similarly, the voltages at bus ‘m’ are also subject to lower and higher phase angle limits, denoted as '∅mmin’ and '∅mmax’. These limits govern the range within which the phase angles of the voltages at bus ‘m’ can vary. To provide a comprehensive understanding of the system, it is important to consider the total number of transmission lines present. In this context, this total number is referred to as ‘NTL’. It represents the collective count of all the transmission lines in the system, serving as a vital metric in analyzing the system’s overall transmission capabilities.
When focusing specifically on the bus ‘m’, it is crucial to take into account the actual power it possesses. This actual power is subject to lower and upper limits, denoted as ‘PGmmin’ and ‘PGmmax’ respectively. These limits establish the range within which the actual power of bus ‘m’ can fluctuate, ensuring that it remains within acceptable parameters. Lastly, the reactive power of the bus ‘m’ is also subject to limits. The minimum and maximum values of this reactive power are denoted as ‘QGmmin’ and ‘QGmmax’ respectively. These limits define the boundaries within which the reactive power of bus ‘m’ can vary, allowing for effective management and control of the system. In summary, the bus ‘m’ is governed by various limits and constraints. These include voltage limits, phase angle limits, power limits, and reactive power limits. These limits ensure that the bus ‘m’ operates within safe and reliable parameters, contributing to the overall stability and performance of the system. The analysis of an IEEE 14-bus system, which has been carried out, has taken into consideration all the fundamental conditions that are relevant to this particular system. To calculate the revenues, generation costs, and profit, the utilization of the Mipower software has been employed.
The step-by-step process that has been followed to achieve these specific results is clearly outlined in Figure 2. It is imperative to note that there are multiple stages involved in the completion of the proposed work, each of which has been meticulously executed. One of the key aspects that has been examined is the placement of the wind farm at various load buses within the IEEE 14-bus system. For each scenario, the profit has been accurately calculated, ensuring a comprehensive analysis. Based on the highest profit that has been attained after considering the wind farm’s placement, the optimal location for the wind farm has been recommended. In terms of the capacity of the wind farm, it has been arbitrarily set at 7 MW, which serves as a benchmark for this study. The primary objective of this research endeavor is to enhance the overall profit of the system by effectively utilizing V2G technology, while also taking into account the imbalance price. Furthermore, to further maximize the system’s profit and alleviate any transmission line congestion, the installation of a Thyristor Controlled Series Capacitor (TCSC) has been executed with utmost precision.
The optimization problem that arises within the context of a deregulated power system is profoundly and significantly influenced by the inherent stochastic characteristics associated with power production, consumer demand, and the pricing mechanisms in play. The complex interplay among these various factors has substantial implications for the overall equilibrium of the power system, reflecting the complex dynamics at work:
• The Unpredictability of Power Production: The incorporation of renewable sources, with a particular emphasis on wind power, serves to introduce a notable degree of variability into the overall power generation process. This lack of predictability can result in significant mismatches between the available supply and the actual demand, leading to consequential fluctuations in market prices that can destabilize the system. The analysis presented in this paper underscores the fact that the stochastic nature of wind power generation has a profound impact on system operations, thereby necessitating the development and implementation of energy storage solutions, such as V2G technology, to effectively manage excess generation during periods of low demand, all while ensuring reliable stability during times of peak demand when energy usage is at its highest.
• Consumer Demand Variability: The variability in consumer demand is also subject to considerable fluctuations, which are influenced by numerous factors, including the time of day, prevailing weather conditions, and the broader context of economic activity. In the context of a deregulated market environment, consumers possess the autonomy to select their preferred power suppliers based on considerations of pricing and the quality of service offered. This inherent choice creates an atmosphere of competition among various producers, compelling them to adopt and implement strategies that can effectively accommodate the fluctuations in demand while simultaneously maintaining their profitability. The research presented in this paper emphasizes that the competitive landscape encourages power producers to continually enhance and improve their service offerings to attract and retain customers in a highly competitive market.
• Price Dynamics: The complex interaction between supply and demand within a deregulated market framework gives rise to dynamic pricing mechanisms that are inherently responsive to real-time fluctuations in demand and the availability of renewable energy sources. The paper provides a detailed discussion on how the strategic implementation of V2G technology can play a pivotal role in stabilizing market prices by enabling electric vehicles to supply power back to the grid during periods of heightened demand, thereby mitigating the effects of imbalance pricing and ultimately enhancing the overall profitability of the system.
• Participant Strategies: Diverse market participants, encompassing power producers, consumers, and independent system operators, engage in the adoption of various strategies aimed at maximizing their respective benefits within the marketplace. Producers may choose to invest in advanced technologies and innovative energy storage solutions as a means to strengthen their competitive edge. Conversely, consumers actively seek to minimize their costs by selecting power suppliers that can provide the most favorable prices and the highest quality of service. The paper notes that the presence and role of independent system operators within a deregulated environment are crucial in regulating these multifaceted interactions, thereby ensuring a delicate balance between supply and demand while promoting equitable pricing practices across the board.
• Equilibrium Challenges: The equilibrium of the power system faces significant challenges stemming from the need to effectively balance these stochastic elements, which are inherently unpredictable and variable. If the supply consistently falls short of meeting the overall demand, it can lead to escalating prices and potential shortages that threaten system integrity. Conversely, an oversupply situation can result in depressed prices and diminished profitability for producers, creating an unsustainable market dynamic. The paper suggests that optimizing the strategic placement of TCSC and leveraging V2G technology can serve as effective measures to mitigate these challenges, thereby enhancing the overall stability and profitability of the power system.
In summary, the inherently stochastic nature of power production, consumer demand, and pricing dynamics plays an indispensable and critical role in shaping the equilibrium of a deregulated power system. The interactions among the various market participants, which are driven by their respective strategies as well as the overarching regulatory framework, are essential for the maintenance of a stable and efficient market environment that benefits all stakeholders involved.
4 V2G integration
The utilization of advanced technologies and the significant rise in importance of the cardinal uprising have paved the way for the extensive adoption of intricate solutions. Among these solutions, V2G technology stands out as it effectively addresses issues concerning power quality, voltage instability, and the maintenance of a stable grid frequency. The V2G system functions by establishing a connection between the electric vehicle charging station and the electrical grid. In a power environment where regulations are relaxed, electricity prices are significantly reduced during off-peak hours, but they rise sharply during peak times.
The V2G system configuration is depicted in Figure 3. V2G system is used to return the energy stored in the EV to the power grid. The diagram shows two power flows. Therefore, this particular system is called a bidirectional system. During charging time, the grid supplies power to the battery bank of EVs, whereas in discharging time, power is returned to the grid from the EV’s battery bank. The V2G system comprises three primary functional blocks: AC-DC bi-directional converter, DC-AC bi-directional converter, and Control and management system. AC-DC bidirectional converter converts AC to DC power for charging the EV’s battery. The bidirectional DC-AC converter converts the direct current from the EV battery bank into alternating current to fulfill the electrical grid necessity. The control and management system consists of many interconnected microprocessors that effectively control the flow of electricity in the V2G unit.
5 Assumptions for the presented models
The presented model focuses on the optimization of profit maximization within the context of a wind-integrated, deregulated power system by employing V2G technologies alongside the strategic placement of TCSC operates under a multitude of foundational assumptions that are pivotal in steering its comprehensive modeling and analytical framework.
• Deregulated Market Environment: The analytical models presuppose the existence of a competitive power market characterized by the operation of numerous stakeholders who actively engage in trading activities, which stands in stark contrast to a regulated system where a singular authoritative entity exerts control over both pricing mechanisms and the supply chain. This particular assumption is of principal importance as it lays the groundwork for a significant analysis of profit maximization strategies that are specifically designed for the unique dynamics of a deregulated market context.
• Integration of Renewable Energy: The paper posits that the incorporation of renewable energy sources, with a particular emphasis on wind power, into the existing electricity grid infrastructure is an essential assumption. This integration is recognized for its propensity to introduce significant variability and unpredictability into power generation patterns, thereby necessitating the implementation of advanced energy storage solutions such as V2G technologies to adeptly manage and stabilize these inherent fluctuations in generation capacity.
• Use of V2G Technology: The analytical frameworks employed in the study assume the operational deployment of V2G technology, which empowers electric vehicles to function as dynamic mobile energy storage units. This innovative technology is conceived to enable bidirectional power flow, thereby assisting in the mitigation of imbalance prices and contributing to the enhancement of overall system stability, particularly during critical peak demand periods when energy consumption surges.
• TCSC Placement: The research articulates the assumption that the strategic placement of TCSC within the power infrastructure can significantly boost the transient stability of the overall power system. The models developed in this context hinge on the assumption that the implementation of TCSC technologies can effectively alleviate congestion costs associated with power transmission and, in doing so, enhance the overall profitability of the energy market participants.
• Power Scheduling Mechanism: The models articulated in the study assume the presence of a modern power scheduling mechanism wherein renewable power generation facilities furnish market operators with various generation scenarios. This scheduling process is predicated on the understanding that actual power generation outputs may deviate from initial forecasts, which is a direct consequence of the unpredictable nature of renewable energy sources, thus necessitating flexible scheduling practices to accommodate these variances.
• Economic Viability: The main assumption that permeates the study is the notion that the maximization of profit is of critical importance for both energy producers and consumers operating within the power grid ecosystem. The models are precisely designed to enhance the economic viability and sustainability of all stakeholders engaged in the electricity market, thereby ensuring a balanced and equitable distribution of resources and benefits.
These foundational assumptions collectively constitute the base upon which the methodologies and analytical explorations are constructed in this work, thereby steering the inquiry into profit maximization strategies within a complex power system environment.
6 Results and discussions
The effectiveness, durability, and viability of the proposed method have been evaluated using an IEEE 14-bus network. This test system entails 14 buses, five generators, and 20 lines. The slack bus, designated as bus no. 1, has been defined within the system. The system data for this test system has been obtained from Dawn and Tiwari (2016). The entire process has been executed using Mi-Power software. The study and analysis of this system have been conducted in the following stages:
Stage 1: Optimum placement of wind plant.
Stage 2: Comparison of economic parameters between deregulated and regulated systems.
Stage 3: Imbalance price measurement and check its influence on economic profit.
Stage 4: Implementation of the V2G method for economic profit enhancement.
Stage 5: Placement of TCSC for further profit improvements.
6.1 Stage 1: optimum placement of wind plant
The wind farm does not incur any running costs; however, there are costs associated with investment and labor, which makes it necessary to carefully find the optimum placement of the wind power plant to maximize profits. To determine the best position, the wind farm (referred to as WF) was strategically placed at various load buses within the system, and the resulting profits were meticulously calculated. The position that yielded the maximum system profit was ultimately chosen as the optimal position for the wind farm. In this case, a wind farm capacity of 7 MW was selected randomly, but with a purpose. To thoroughly assess the applicability and effectiveness of the projected technique, 9 cases/scenarios, including the base base/scenario were meticulously chosen and then comprehensively detailed in Table 3. The analysis of the impact and influence of wind farm allocation on economic parameters was meticulously carried out for both regulated and deregulated power environments, meticulously considering and meticulously examining all the specified cases.
Table 4 provides a comprehensive and detailed analysis that covers all aspects of revenues, gen. costs, and profits for a regulated environment. The placement of WF strategically performed at different load buses throughout the system played a crucial role in enabling the computation and evaluation of thermal revenues, gen. costs, and profits. Furthermore, it is worth mentioning that the power produced by the different generator buses, along with their corresponding LMP were meticulously and accurately recorded, ensuring a highly precise and reliable analysis. The noteworthy outcome of this comprehensive assessment has revealed that the optimal location for the WF is at bus # 9, specifically in Case-4, as it produces the most extensive profit.
The transformation of the electrical system from regulated to deregulated was achieved through the implementation of demand-end bidding, with the bidding process conducted from load bus 6. Wind energy, just like the regulated system, was implemented at different load buses to ensure a properly functioning and controlled system. In this regard, the thermal plant revenues, gen. costs, and profit were meticulously calculated, taking into consideration the power capacity that was generated and the LMP associated with it. It is seen that, in Table 5 the wind farm was strategically placed at bus number 14, resulting in the highest system profit, particularly in Case-4.
This finding highlights the significant impact and positive outcome that can be achieved by carefully selecting the appropriate location for wind energy installation. To further demonstrate the effectiveness of this approach, the OPF (optimal power flow) solution for the base scenario was determined using the MiPower software and is visually represented in Figure 4. This visualization serves as a clear and concise representation of the successful implementation of wind energy in the system.
6.2 Stage 2: comparison of economic parameters between deregulated and regulated systems
Nowadays the use of deregulated systems is increasing very sharply throughout the world due to the economic assistance to the system players. This work is also influenced by the economically beneficial concept of a deregulated system and comparative studies have been performed here in terms of revenue, system generation cost, and profit between the deregulated and regulated system. Figure 5 shows the revenue comparison where it is revealed that the revenue is higher in a regulated system than the competitive system. In the entire research, the revenue represents the earnings of the power producers. Therefore, reducing the revenue signifies a lighter financial burden on customers about energy prices, which is the chief aim of the competitive system.
The aggregate expenses associated with electricity production, encompassing transmission and distribution costs, are denoted as the generation cost. This incorporates the costs of equipment, raw materials, installation, labor, and other relevant expenditures. As illustrated in Figure 6, the gen. cost in the regulated power system exceeds that of the competitive one. This discrepancy arises from the heightened competition among power producers in the deregulated system, compelling them to embrace advanced technologies to improve power quality and concurrently reduce generation costs to attract a larger customer base.
The profit is the balance that remains after subtracting the cost of production from revenue. In this paper, it is denoted in $/h. Figure 7 illustrates a comparison between the profitability of regulated systems and deregulated systems, indicating that regulated systems generate greater profits than their deregulated counterparts. This implies that power producers benefit from the implementation of deregulated systems, which in turn benefits the customers. The examination of Figure 7 brings us to the inference that the positioning of wind farms leads to higher profits for both controlled and market-based power systems when compared to systems without wind farms. This discovery may incentivize power providers to increase investments in renewable power plants, aiming for enhanced economic returns.
This study deeply explores the impact that wind farms have on systems that are both regulated and deregulated. To provide a clear example, a fixed 7 MW wind farm is used as an illustrative example. The findings of this study suggest that by enhancing the integration of wind power, there is the potential for market participants to experience increased profits. This is particularly beneficial for customers as it offers them social advantages. It is important to note that in a competitive power environment, the effectiveness of the electrical system is reliant on two key factors. Firstly, the economic aspects are controlled by Locational Marginal Prices (LMP). Secondly, the stability and power quality of the system are influenced by the system’s voltage profile. The study emphasizes that both regulated and deregulated systems experience the highest profits in case 4 and case 9, according to the research conducted. To further analyze these cases, comparative examinations of LMP and voltage profiles were carried out. Figures 8, 9 offer a graphical depiction that showcases the contrast between locational marginal prices in the regulated and deregulated systems for case 4 and case 9, correspondingly.
The control of the system revenue is directly under the jurisdiction of the LMP as it aids in the depiction of the selling price for the power that is generated. The introduction of a deregulated system has resulted in a decrease in the LMP value for individual buses, subsequently leading to a drop in the power selling charge. As a result, customers will now have to pay a reduced quantity for energy consumption. A comparison between the regulated and the deregulated system can be observed in Figures 10, 11 for case-4 and case-9 respectively, effectively showcasing the profile of the voltage within the system. It is of utmost importance to maintain a consistent voltage level across all buses as it greatly contributes to the enhancement of the security and stability of the system. The outcomes derived from the analysis indicate that the implementation of a deregulated environment has had a positive impact on the overall voltage profile of the system.
6.3 Stage 3: imbalance price measurement and check its influence on economic profit
The evaluation of the economic impact of the competitive power network connected to renewable energy must consider the ramifications of imbalanced prices. The uncertain and capricious nature of renewable sources presents a notable peril to the electrical grid, as there is a possibility that renewable power plants may not meet the predetermined power demand. To address this dilemma, two possible avenues can be pursued: the application of cutting-edge forecasting technologies and the integration of energy storage devices. Nevertheless, it is important to note that the effectiveness of the forecasting process is hindered by certain limitations, thus rendering it an inefficient solution. Consequently, the implementation of energy storage devices emerges as an indispensable measure to ensure the maintenance of scheduled power and the minimization of potential economic losses.
The survey that was conducted on the day aimed to gather data on the current wind speed, also known as CWS. Simultaneously, the data from the previous day was considered as the estimated wind speed, referred to as EWS. It's important to mention that the difference between these two wind speeds directly affects wind generation. This impact then leads to a difference between the expected power generation and the resulting profits. This difference, commonly known as the generation of imbalance price, represents the gap between the current and expected data. It's crucial to emphasize that real-time current and estimated wind speed data were specifically gathered for the city of Vijayawada (Time and Date website, 2024). These data points were carefully collected and organized in Table 6, providing a comprehensive overview of the wind speeds in the area. Due to the unpredictable nature of wind speed, the data was captured at hourly intervals and recorded at a height of 10 m. Additionally, the probable wind speed at the wind turbine’s hub height of 120 m was also measured for a more detailed analysis. As a result, wind generation calculations were conducted using this data for different hours.
In case the present wind velocity surpasses the predicted wind velocity, the power-producing firms are compensated through an award. If the wind speed at the present moment is found to be lower than the wind speed that was initially estimated, the companies responsible for generating electricity are subjected to a penalty as a consequence of their inability to fulfill their commitment to delivering the electricity that was promised. Upon analyzing the recorded wind speed data, it becomes evident that there have been instances of repetitive scenarios, which have unfortunately not been taken into consideration.
This is a significant oversight considering the potential impact of these repetitive situations on the overall operations of the wind farm. The wind farm, which is located on bus number 14 as previously determined in Stage 2, plays a crucial role in the generation of electricity. To accurately determine the cost of imbalances, the calculations consider both the current and projected wind power. These calculations are essential for assessing the financial impact of any imbalances in wind power generation. To further illustrate this, Table 6 provides a detailed overview of how imbalance prices can affect the overall profit generated by the wind farm. It's important to note that the estimated profit mentioned here refers to the profit that would be achieved without considering imbalance prices. On the other hand, the actual profit represents the profit obtained when the imbalance price is taken into account. Figure 12 presents a comparative analysis of the profit with and without considering imbalance prices. It is seen that in many hours the actual profit is reduced as compared to the estimated profit which happens due to the negative imbalance prices.
6.4 Stage 4: implementation of V2G technology for profit enhancement
To avoid such situations and eliminate the imbalance cost discussed in step 3 above, V2G technology can be used. The V2G technology utilizes the energy stored in electric vehicles to support the grid during high-demand periods, while the EVs draw power from the grid during low-demand periods. When demand increases, the stored energy in the EVs can be retrieved to meet the required demand. Table 7 provides a comprehensive and detailed representation of the significant impact that the prices of imbalance have on the actual profit. This analysis takes into account a substantial period, specifically 24 h, to ensure a comprehensive understanding of the method employed.
By carefully considering and eliminating any duplicates or situations with similar wind speeds, we are left with a total of 17 unique cases that are of utmost importance in our examination. It is important to highlight that in this specific study, the wind farm has been strategically placed at the most lucrative bus within the deregulated system, specifically bus #14. Upon closer examination of the data presented in Table 8, it becomes abundantly clear that there is a noticeable change in profit as a direct result of the imbalance. It is evident that the penalty associated with the imbalance significantly outweighs the profit that is generated. This discovery serves to highlight the immense importance of taking the necessary steps to remove the cost of imbalance.
It is worth noting that a higher penalty ultimately leads to even greater losses, further emphasizing the urgency to address this issue. Fortunately, there exists a promising solution that can help mitigate the potential losses that arise from the imbalance. The integration of V2G technology, which operates based on utilizing batteries, presents a viable and effective strategy to avoid such detrimental financial outcomes. By leveraging this innovative technology, it becomes possible to effectively manage and regulate the flow of energy, thereby minimizing the impact of imbalance on the overall profit. This forward-thinking approach has the potential to revolutionize the energy sector and ensure a more sustainable and financially viable future. The EV fleet capacity considered here is 3 MW. The final efficiency varies accordingly for each battery. The utilizing capacity is crucial for the amount of output power of the EV. The better the efficiency, the better the output power, and the better the profit. When prices become imbalanced, the EV fleet can provide the necessary power to prevent further imbalance. The power that is provided by the fleet of electric vehicles is subsequently subtracted, and the power that is left over after this deduction is then utilized to calculate the overall profit. To achieve the maximum possible profit within the power system, the implementation of V2G technology can prove to be highly advantageous. At present, the effectiveness of V2G technology largely depends on the utilization of lithium-ion batteries. Our comprehensive analysis involved a thorough examination of the impact that various types of batteries, including lithium-ion, lead-acid, and supercapacitor batteries, have on the overall profit of the system when they are integrated into the power system. Table 8 illustrates the impact of these batteries on the power grid’s profit. It's worth noting that the system being considered is deregulated, as it's more profitable than the regulated system.
In the situation that is specifically being considered in this charging scenario, it becomes quite apparent that the level of increase or improvement in terms of the overall amount of energy stored or supplied to the battery remains constant and uniform in every single instance or situation that is being analyzed or evaluated, and this is primarily because the battery in question is already filled or replenished to its maximum capacity or level. However, when it comes to discharging, the gain varies depending on the specific cases. This discrepancy arises from the fact that different batteries possess different output efficiencies. Out of the three battery options that were taken into consideration, it can be observed that the supercapacitor proves to be the most efficient among them. However, it is crucial to emphasize that the supercapacitor also entails a higher cost when it comes to its installation. In the particular situation being examined, the EV experiences discharge in extreme proximity to the generator bus, which is directly associated with the concept of V2G as well as the EV’s battery storage unit. While the EV can charge from a bus, it can discharge in various buses. Consequently, this method proves to be advantageous in safeguarding the electrical grid from severe faulty conditions.
From the values in Table 9, we can see that the system gains are excellent when the V2G system is integrated, even though there is an imbalance in the power network. Figure 13 illustrates the comparative gains in power systems due to the presence of V2G systems. The graphical representation of the profit in the system is depicted in the figure. The findings of the study provide evidence that the incorporation of V2G technology into the power grid yields noteworthy consequences, particularly when the V2G system is fueled by an appropriate battery or energy storage system. The outcomes of the investigation demonstrate that the inclusion of the V2G system within the power system framework has a considerable impact. This impact is particularly pronounced and noteworthy in situations where the V2G system is appropriately powered by a suitable battery or energy storage system. The profit increases after the implementation of the V2G method, regardless of whether there is an imbalance price scenario or not.
6.5 Stage 5: placement of TCSC for further profit improvements
In a vast power network, transmission line congestion may arise from failures or abnormalities in buses, generators, or lines, incurring additional costs for power customers through congestion charges added to energy selling prices. To mitigate this issue, the study explores the application of Thyristor Controlled Series Capacitor (TCSC) to enhance the thermal limit of transmission lines and alleviate congestion problems. The strategic conduction of the placement of TCSC in this research was carried out with the utmost care and precision, ensuring that the system profit was maximized and economic benefits were delivered to all market players involved. A thorough analysis was conducted to determine the optimal position for the placement of TCSC. This analysis involved adjusting compensation levels for all transmission lines within a range of −0.7 to 0.2 and calculating the system generation cost for each case.
The results of this analysis revealed that the optimal position for TCSC was at line number 3, with a compensation level of −0.25. It was determined that this particular placement scenario resulted in the lowest system generation cost, thus solidifying it as the ideal position for TCSC within the system. Moreover, to further enhance the accuracy and effectiveness of the research, the study also incorporated the V2G method. This method was utilized to calculate the overall system profit in conjunction with the placement of TCSC. Figure 14, which is provided as a visual representation, depicts a comprehensive comparison of the profit achieved with and without the implementation of imbalance prices for the V2G method after the placement of TCSC. The findings derived from this analysis indicate that the placement of TCSC, when combined with the V2G method, has a significant positive impact on the overall system profit. This further highlights the importance and effectiveness of TCSC placement in maximizing economic benefits.
The entire research was executed utilizing the Mi Power software. The early phases of the research were structured to cater to both regulated and deregulated system environments. Upon determining that the deregulated system offered superior economic benefits, the subsequent phases of the work were exclusively carried out within the deregulated environment.
7 Conclusion
This article focuses on increasing profit and reducing risk by incorporating renewable energy sources, primarily wind energy sources, into existing thermal power plants. Companies that use renewable energy sources can increase their profits while also contributing to a more sustainable and ecologically responsible future. Renewable energy is simple to implement into a deregulated system because it allows for several players. The main disadvantage of renewable energy sources is their unpredictability, which causes mismatches in the system, particularly imbalance pricing. To address this issue, energy storage technologies such as EVs, PHS, and BESS can be deployed. V2G not only serves as an energy storage device, but it can also carry electricity from one location to another. The deployment of V2G technology has the potential to increase the profitability of the electricity system by alleviating the current price imbalance, as identified in recent research. During peak hours, EVs can be discharged at any point, preventing a grid blackout. The battery of an EV is charged with a low LMP (power selling price) and discharged at a high LMP. As a result, the system’s profits are automatically boosted. The TCSC placement of deregulated power systems has also improved transient stability, decreased congestion, minimized power losses, and increased transmission line load capacity, all of which benefit energy customers economically. The findings from the empirical analysis give persuasive evidence that wind farms have the inherent ability to drastically reduce reliance on fossil fuels within the complex frameworks of electricity networks. Furthermore, it is worth noting that supplementary remedies such as V2G and TCSC can be effectively used to ensure the steadfastness and equilibrium of the electrical grid by systematically reducing congestion within the system and efficiently maximizing the economic advantages and benefits that the system can derive from such a course of action.
Data availability statement
The original contributions presented in the study are included in the article/supplementary material, further inquiries can be directed to the corresponding authors.
Author contributions
SD: Writing–original draft, Writing–review and editing. AR: Writing–original draft, Writing–review and editing. MR: Writing–original draft, Writing–review and editing. SSD: Writing–original draft, Writing–review and editing. KR: Writing–original draft, Writing–review and editing. UC: Writing–original draft, Writing–review and editing. TU: Writing–original draft, Writing–review and editing.
Funding
The author(s) declare that no financial support was received for the research, authorship, and/or publication of this article.
Conflict of interest
The authors declare that the research was conducted in the absence of any commercial or financial relationships that could be construed as a potential conflict of interest.
Publisher’s note
All claims expressed in this article are solely those of the authors and do not necessarily represent those of their affiliated organizations, or those of the publisher, the editors and the reviewers. Any product that may be evaluated in this article, or claim that may be made by its manufacturer, is not guaranteed or endorsed by the publisher.
References
Abdolrasol, M. G. M., Hannan, M. A., Sarker, M. R., and Ker, P. J. (2021). Energy management scheduling for microgrids in the virtual power plant system using artificial neural networks. Energies 14, 6507. doi:10.3390/en14206507
Amir, M., Deshmukh, R. G., Khalid, H. M., Said, Z., Raza, A., Muyeen, S. M., et al. (2023). Energy storage technologies: an integrated survey of developments, global economical/environmental effects, optimal scheduling model, and sustainable adaption policies. J. Energy Storage 72 (Part E), 108694. doi:10.1016/j.est.2023.108694
Armaghan, S. M., Asgharzadeh, F., Khanghah, B. Y., and Ashraf, H. R. (2023). Resilient operation of electric vehicles considering grid resiliency and uncertainties. Int. Trans. Electr. Energy Syst. 2023, 5320002. doi:10.1155/2023/5320002
Barik, A. K., Das, D. C., Latif, A., Hussain, S. M. S., and Ustun, T. S. (2021). Optimal voltage–frequency regulation in distributed sustainable energy-based hybrid microgrids with integrated resource planning. Energies 14, 2735. doi:10.3390/en14102735
Basu, J. B., Dawn, S., Saha, P. K., Chakraborty, M. R., Alsaif, F., Alsulamy, S., et al. (2023). Risk mitigation and profit improvement of a wind-fuel cell hybrid system with TCSC placement. IEEE Access 11, 39431–39447. doi:10.1109/access.2023.3267163
Bilal, M., Ahmad, F., and Rizwan, M. (2023). Techno-economic assessment of grid and renewable powered electric vehicle charging stations in India using a modified metaheuristic technique. Energy Convers. Manag. 284, 116995. doi:10.1016/j.enconman.2023.116995
Chauhan, A., Upadhyay, S., Khan, M. T., Hussain, S. M. S., and Ustun, T. S. (2021). Performance investigation of a solar photovoltaic/diesel generator based hybrid system with cycle charging strategy using BBO algorithm. Sustainability 13, 8048. doi:10.3390/su13148048
Choubey, A. (2019). Utilization of maximum power in airfoil blade of horizontal Axis wind turbine by the concept of cfd analysis. J. Of Urban and Environ. Eng. 13 (2), 266–273. doi:10.4090/juee.2019.v13n2.266273
Das, A., Dawn, S., Gope, S., and Ustun, T. S. (2022a). A strategy for system risk mitigation using FACTS devices in a wind incorporated competitive power system. Sustainability 14, 8069. doi:10.3390/su14138069
Das, S. S., Das, A., Dawn, S., Gope, S., and Ustun, T. S. (2022b). A joint scheduling strategy for wind and solar photovoltaic systems to grasp imbalance cost in competitive market. Sustainability 14 (9), 5005. doi:10.3390/su14095005
Das, S. S., Kumar, J., Dawn, S., and Salata, F. (2023). Existing stature and possible outlook of renewable power in comprehensive electricity market. Processes 11 (6), 1849. doi:10.3390/pr11061849
Dawn, S., Das, S. S., Ramesh, M., Inkollu, S. R., Pandraju, T. K. S., Alsaif, F., et al. (2023). Profit expansion of a solar integrated day-ahead system by placement of TCSC and fuel cell in the presence of disequilibrium price. IEEE Access 11, 111812–111831. doi:10.1109/access.2023.3315747
Dawn, S., and Tiwari, P. K. (2016). Improvement of economic profit by optimal allocation of TCSC and UPFC with wind power generators in double auction competitive power market. Electr. Power Energy Syst. 80, 190–201. doi:10.1016/j.ijepes.2016.01.041
Dey, P. P., Das, D. C., Latif, A., Hussain, S. M. S., and Ustun, T. S. (2020). Active power management of virtual power plant under penetration of central receiver solar thermal-wind using butterfly optimization technique. Sustainability 12, 6979. doi:10.3390/su12176979
Duana, Y., Zhaob, Y., and Hu, J. (2023). An initialization-free distributed algorithm for dynamic economic dispatch problems in microgrid: modeling, optimization, and analysis. Sustain. Energy, Grids Netw. 34, 101004. doi:10.1016/j.segan.2023.101004
Egbue, O., Uko, C., Aldubaisi, A., and Santi, E. (2022). A unit commitment model for optimal vehicle-to-grid operation in a power system. Electr. Power Energy Syst. 141, 108094. doi:10.1016/j.ijepes.2022.108094
Farooq, Z., Rahman, A., Hussain, S. M. S., and Ustun, T. S. (2022). Power generation control of renewable energy based hybrid deregulated power system. Energies 15, 517. doi:10.3390/en15020517
Gamel, S. A., and Sultan, Y. A. (2023). Winds of power: data analysis for the relationship between wind speed, gust, and power output. J. Eng. Res. 7 (26), 189–194. doi:10.21608/erjeng.2023.239780.1265
Hamoudi, Y., Amimeur, H., Aouzellag, D., Abdolrasol, M. G. M., and Ustun, T. S. (2023). Hyperparameter bayesian optimization of Gaussian process regression applied in speed-sensorless predictive torque control of an autonomous wind energy conversion system. Energies 16, 4738. doi:10.3390/en16124738
Hou, M., Zhao, Y., and Ge, X. (2017). Optimal scheduling of the plug-in electric vehicles aggregator energy and regulation services based on grid to vehicle. Int. Trans. Electr. Energy Syst. 27 (6), e2364. doi:10.1002/etep.2364
Hu, J., Zou, Y., and Soltanov, N. (2024). A multilevel optimization approach for daily scheduling of combined heat and power units with integrated electrical and thermal storage. Expert Syst. Appl. 250, 123729. doi:10.1016/j.eswa.2024.123729
Hussain, S. M. S., Aftab, M. A., Ali, I., and Ustun, T. S. (2020b). IEC 61850 based energy management system using plug-in electric vehicles and distributed generators during emergencies. Int. J. Electr. Power and Energy Syst. 119, 105873. doi:10.1016/j.ijepes.2020.105873
Hussain, S. M. S., Aftab, M. A., Nadeem, F., Ali, I., and Ustun, T. S. (2020a). Optimal energy routing in microgrids with IEC 61850 based energy routers. IEEE Trans. Industrial Electron. 67 (6), 5161–5169. doi:10.1109/tie.2019.2927154
Jirdehi, M. A., and Tabar, V. S. (2023). Multi-objective long-term expansion planning of electric vehicle infrastructures integrated with wind and solar units under the uncertain environment considering demand side management: a real test case. Sustain. Cities Soc. 96, 104632. doi:10.1016/j.scs.2023.104632
Latif, A., Paul, M., Das, D. C., Hussain, S. M. S., and Ustun, T. S. (2020). Price based demand response for optimal frequency stabilization in ORC solar thermal based isolated hybrid microgrid under salp swarm technique. Electronics 9, 2209. doi:10.3390/electronics9122209
Lei, Y., Yanrong, C., Hai, T., Ren, G., and Wenhuan, W. (2023). DGNet: an adaptive lightweight defect detection model for new energy vehicle battery current collector. IEEE Sensors J. 23 (23), 29815–29830. doi:10.1109/jsen.2023.3324441
Ma, B. X. Z., Geng, Y., Heck, P., Ren, W., Tobias, M., Maas, A., et al. (2015). A life cycle co-benefits assessment of wind power in China. Renew. Sustain. Energy Rev. 41, 338–346. doi:10.1016/j.rser.2014.08.056
Mojumder, M. R. H., Antara, F. A., Hasanuzzaman, M., Alamri, B., and Alsharef, M. (2022). Electric vehicle-to-grid (V2G) technologies: impact on the power grid and battery. Sustainability 14, 13856. doi:10.3390/su142113856
Nadeem, F., Aftab, M. A., Hussain, S. M. S., Ali, I., Tiwari, P. K., Goswami, A. K., et al. (2019). Virtual power plant management in smart grids with XMPP based IEC 61850 communication. Energies 12, 2398. doi:10.3390/en12122398
Naja, R., Soni, A., and Carletti, C. (2023). Electric vehicles energy management for vehicle-to-grid 6G-based smart grid networks. J. Sens. Actuator Netw. 12 (79), 79. doi:10.3390/jsan12060079
Nguyen, V. T., Nguyen, H. V. P., Truong, T. B. T., and Truong, Q. V. (2023). A virtual synchronous generator control method in microgrid with vehicle-to-grid system. J. Electr. Eng. 74 (6), 492–502. doi:10.2478/jee-2023-0057
Nkan, I. E., Okpo, E. E., and Inyang, A. B. (2023). Enhancement of power systems transient stability with TCSC: a case study of the Nigerian 330 kV, 48-bus network. Int. J. of Multidiscip. Res. and Analysis 06 (10). doi:10.47191/ijmra/v6-i10-37
Nsonga, P., Hussain, S. M. S., Garba, A., Ustun, T. S., and Ali, I. (2017). “Performance evaluation of electric vehicle ad-hoc network technologies for charging management,” in 2017 IEEE PES Asia-Pacific power and energy engineering Conference. 08-10 November 2017. Bangalore, India: IEEE, 1–5.
Pereira, J. S., Belati, E. A., Nascimento, C. F., Silva, P. F., and Rossoni, P. (2022). A mathematical programming approach for allocation and analysis of TCSC in power transmission systems. IEEE Lat. Am. Trans. 20 (7), 2001–2009. doi:10.1109/tla.2021.9827473
Rao, K. D., Anand, N. V., Pandraju, T. K. S., Alsaif, F., and Ustun, T. S. (2024). Optimally tuned gated recurrent unit neural network-based state of health estimation scheme for lithium ion batteries. IEEE Access 12, 58597–58607. doi:10.1109/access.2024.3392432
Safiullah, S., Rahman, A., Lone, S. A., Hussain, S. M. S., and Ustun, T. S. (2022). Novel COVID-19 based optimization algorithm (C-19BOA) for performance improvement of power systems. Sustainability 14, 14287. doi:10.3390/su142114287
Shehata, A. A., Tolba, M. A., El-Rifaie, A. M., and Korovkin, N. V. (2022). Power system operation enhancement using a new hybrid methodology for optimal allocation of FACTS devices. Energy Rep. 8, 217–238. doi:10.1016/j.egyr.2021.11.241
Singh, S., and Jaiswal, S. P. (2021). Enhancement of ATC of micro grid by optimal placement of TCSC. Mater. Today Proc. 34 (3), 787–792. doi:10.1016/j.matpr.2020.05.161
Tappeta, V. S. R., Appasani, B., Patnaik, S., and Ustun, T. S. (2022). A review on emerging communication and computational technologies for increased use of plug-in electric vehicles. Energies 15, 6580. doi:10.3390/en15186580
Thakre, M. P., Thakre, P. M., Tapre, P. C., Pawase, R. S., Kadlag, S. S., Kadam, D. P., et al. (2023). Integration of storage technology oversight: power system and computer engineering analogy. Bull. Electr. Eng. Inf. 12 (6), 3228–3237. doi:10.11591/eei.v12i6.4879
Time and date website, (2024). Available at: www.timeanddate.com. Accessed on: April 10, 2024.
Ustun, T. S., Hussain, S. M. S., Syed, M. H., and Dambrauskas, P. (2021). IEC-61850-Based communication for integrated EV management in power systems with renewable penetration. Energies 14, 2493. doi:10.3390/en14092493
Keywords: deregulated system, wind power, vehicle-to-grid, thyristor-controlled series compensator, system profit, imbalance price
Citation: Dawn S, Ramakrishna A, Ramesh M, Das SS, Rao KD, Cali U and Ustun TS (2024) Profit maximization of a wind-integrated deregulated system using V2G techniques and TCSC placement. Front. Energy Res. 12:1400745. doi: 10.3389/fenrg.2024.1400745
Received: 14 March 2024; Accepted: 13 September 2024;
Published: 26 September 2024.
Edited by:
Michael Carbajales-Dale, Clemson University, United StatesReviewed by:
Mohamed Salem, University of Science Malaysia (USM), MalaysiaHaris M. Khalid, University of Dubai, United Arab Emirates
Copyright © 2024 Dawn, Ramakrishna, Ramesh, Das, Rao, Cali and Ustun. This is an open-access article distributed under the terms of the Creative Commons Attribution License (CC BY). The use, distribution or reproduction in other forums is permitted, provided the original author(s) and the copyright owner(s) are credited and that the original publication in this journal is cited, in accordance with accepted academic practice. No use, distribution or reproduction is permitted which does not comply with these terms.
*Correspondence: Umit Cali, dW1pdC5jYWxpQG50bnUubm8=; Taha Selim Ustun, c2VsaW0udXN0dW5AYWlzdC5nby5qcA==