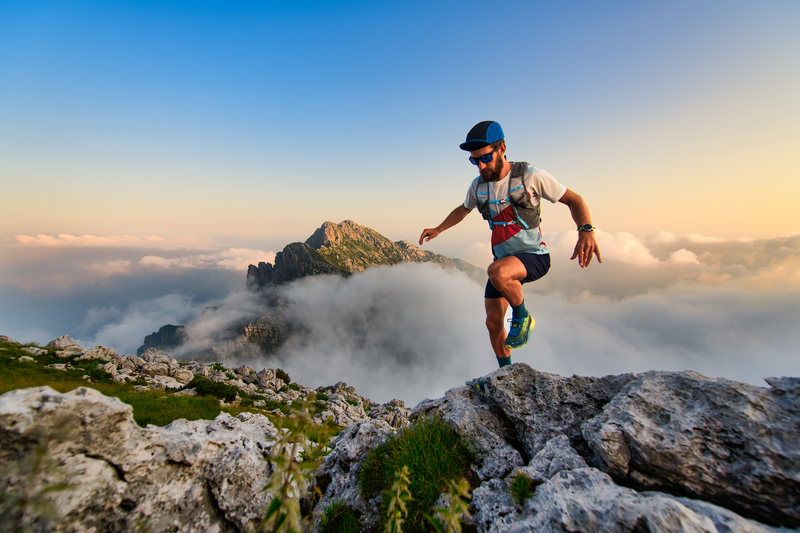
94% of researchers rate our articles as excellent or good
Learn more about the work of our research integrity team to safeguard the quality of each article we publish.
Find out more
ORIGINAL RESEARCH article
Front. Energy Res. , 22 February 2024
Sec. Sustainable Energy Systems
Volume 12 - 2024 | https://doi.org/10.3389/fenrg.2024.1337188
This article is part of the Research Topic New Trends in Energy-Economy-Environment System View all 21 articles
Promoting sustainable development in manufacturing is a paramount goal, with a focus on advancing green innovation. This study constructs a system for evaluating green innovation efficiency and employs the Super-EBM model, incorporating unexpected output, to assess the efficiency of green innovation in 13 cities across the Beijing-Tianjin-Hebei region from 2011 to 2020. The study further conducts dynamic analysis using the Malmquist-Luenberger index. Results reveal that, statically, the overall green innovation efficiency in the manufacturing industry of the Beijing-Tianjin-Hebei region is inefficient. There exists a considerable gap in green innovation efficiency among Beijing, Tianjin, and Hebei, with Beijing and Tianjin demonstrating superior performance compared to Hebei. Substantial variations exist in the green innovation efficiency of manufacturing across different cities in the Beijing-Tianjin-Hebei region. Only Beijing, Qinhuangdao, and Baoding achieve DEA-effective green innovation efficiency in the manufacturing industry, while the other cities do not. Dynamically, the green innovation efficiency of the manufacturing industry in the Beijing-Tianjin-Hebei region is on the rise. There is a varying degree of improvement in green innovation efficiency in Beijing, Tianjin, and Hebei, with Hebei showing the highest improvement, Tianjin ranking second, and Beijing having the least improvement. With the exception of Langfang and Hengshui, the green innovation efficiency in the manufacturing industry is improving in most cities in the Beijing-Tianjin-Hebei region, with Hebei witnessing the most significant improvement. This study aims to integrate “environmental pollution” into the evaluation index system for green innovation efficiency. It assesses green innovation efficiency in the manufacturing industry of the Beijing-Tianjin-Hebei region, considering both static and dynamic perspectives. This clarification offers insights into the level of green innovation, contributing valuable information for the advancement of high-quality development in the regional manufacturing industry.
Since the initiation of economic reforms and opening-up policies, China’s economy has experienced substantial growth, propelling it to the position of the world’s second-largest economy. The Gross Domestic Product (GDP) is expected to surge from 51.9 trillion yuan in 2012 to 121 trillion yuan in 2022, marking a growth of 42.9 percent and contributing over 30 percent to global economic expansion. Nevertheless, despite the rapid economic growth, the nation is grappling with progressively severe environmental challenges. The 2020 Global Environmental Performance Index (EPI) Report reveals that China’s EPI is 37.3, ranking 120th out of 180 regions, while its air quality index is 137th, scoring 27.1. Regarding energy consumption, the 2022 BP Statistical Yearbook of World Energy indicates that China’s total energy consumption rose by 7.1 percent annually. It constitutes 26.5 percent of global consumption, securing the top rank worldwide. Additionally, the energy consumption per unit of GDP remains 1.5 times higher than the global average. Environmental concerns now significantly constrain China’s economic growth. Urgent measures are needed to shift the development paradigm towards achieving sustainable economic growth (Wu et al., 2017; Chen et al., 2020; Yang et al., 2021). Faced with escalating environmental pollution and growing energy consumption concerns, China has introduced a novel development paradigm encompassing “innovation, coordination, greenness, openness, and sharing.” The emphasis is on seamlessly integrating this new development concept across all facets of economic and social progress, steering high-quality economic development in alignment with these principles, and overcoming bottlenecks impeding China’s economic and social progress within the confines of ecological and environmental constraints.
China is in the advanced phase of industrialization and is undergoing a pivotal shift in consumption structure. Focusing on the development of the real economy becomes imperative, and the manufacturing sector, a crucial pillar within it, significantly contributes to shaping a modernized economic system. Nonetheless, the swift growth of the manufacturing sector has concurrently triggered challenges like escalated energy consumption and environmental pollution, consequently impeding the prospect of sustainable economic advancement (Cherniwchan, 2012; Rubashkina, 2015; Chakraborty and Chatterjee, 2017). The pressing concern currently revolves around orchestrating a balance between economic growth and environmental preservation to attain a green, low-carbon transformation. This becomes pivotal for fostering high-quality advancements within the manufacturing sector (Johnstone et al., 2012; Ford et al., 2014). With China’s economic development entering a new phase, the imperative lies in transitioning the economic growth rate to a medium-high pace, the development mode to one focused on quality and efficiency, and the development impetus to an innovation-driven approach (Yuan and Xiang, 2018). The transition of the manufacturing industry from “Made in China” to “Created in China” necessitates a profound emphasis on innovation-driven approaches. Only through enhancement in their innovative capabilities can they avoid getting stuck in a bottleneck of key technologies. Green innovation, as a critical synergy of environmental development, coordinated progress, and innovation-driven initiatives, stands as the linchpin for surmounting resource and environmental limitations while fostering high-quality economic growth (Mitsutsug, 2005; Albrizio et al., 2017).
The synergistic development of Beijing-Tianjin-Hebei has garnered attention due to China’s unbalanced regional development (Liang and He, 2022). Serving as a crucial manufacturing hub in China, the Beijing-Tianjin-Hebei region plays a pivotal role in the country’s economic development. In recent years, the manufacturing industry in Beijing-Tianjin-Hebei has encountered significant challenges, including resource consumption, energy shortages, and environmental pollution. Achieving a green transformation in the high-quality development of the manufacturing industry in Beijing-Tianjin-Hebei has become an urgent necessity, and green innovation is an essential requirement for this transformation (Broekel, 2015; Albort et al., 2016). Accurately assessing the green innovation efficiency in the Beijing-Tianjin-Hebei manufacturing industry is theoretically and practically significant. Formulating targeted green innovation policies, purposefully enhancing innovation efficiency, and fostering high-quality development in manufacturing are crucial.
Building upon this groundwork, the study formulates an evaluation index system for green innovation efficiency. It utilizes the Super-EBM model, incorporating unexpected output, to assess the green innovation efficiency within the manufacturing sector of the Beijing-Tianjin-Hebei region. Additionally, it employs the Malmquist-Luenberger (ML) index to conduct a dynamic analysis of green innovation efficiency, complementing the static evaluation.
The marginal contribution of this study is demonstrated in three main aspects. Firstly, “environmental pollution” is considered as undesirable output. The study introduces a comprehensive evaluation index system for green innovation efficiency, covering input, desired output, and undesirable output. It takes into account the economic, social, and environmental aspects of green innovation, enhancing and refining the efficiency index system. Additionally, the study analyzes the regional green innovation efficiency of the manufacturing industry. The study does not explore green innovation efficiency at the national and provincial levels. Instead, it focuses on 13 cities in the Beijing-Tianjin-Hebei region to assess the green innovation efficiency of the manufacturing industry, elevating the research perspective in this area. Finally, the paper evaluates the efficiency of green innovation from both static and dynamic perspectives. In the analysis of green innovation efficiency, most studies traditionally focus on static analysis, neglecting the dynamic perspective. This paper assesses the efficiency of green innovation in the manufacturing industry of Beijing-Tianjin-Hebei from both static and dynamic perspectives. This analysis clarifies the level of green innovation in the region and enhances research methods for green innovation efficiency.
This paper is structured as follows: Section 2 comprises a literature review focusing on the definition of green innovation and the methodology for assessing green innovation efficiency. Section 3 details the research design, emphasizing the model configuration, development of the green innovation efficiency assessment index system, and data sources. Section 4 presents an empirical study concentrating on the green innovation efficiency within the manufacturing industry of the Beijing-Tianjin-Hebei region, examining both static and dynamic perspectives. Section 5 elaborates on the primary findings. In Section 6, research conclusions, policy implications, limitations, and future prospects are presented.
Green innovation gained prominence in academic research during the 1990s. There is no consensus among scholars regarding the definition of green innovation. Beisea and Rennings (2005) define green innovation as the adoption of new technologies, processes, and products to prevent or reduce environmental harm. Building upon this, Akbari et al. (2022) suggest that green innovation can mitigate environmental pollution, conserve energy, and foster sustainable development aligning environmental protection with corporate competitiveness. Additionally, other scholars highlight green innovation as a creative endeavor that reconciles economic and environmental gains, simultaneously enhancing environmental quality and product performance (Feng et al., 2018; Li and Shen, 2021; Liu et al., 2023). Green innovation primarily targets economic and environmental advantages, striving for the dual objectives of economic growth and environmental safeguarding.
The assessment of green innovation efficiency presently involves two primary approaches: one utilizes stochastic Frontier (SFA) models founded on parametric methods. Several researchers have empirically studied the innovation efficiency within China’s high-tech sectors employing the SFA method (Han, 2010; Yi et al., 2019; Chao, 2020). Meanwhile, Gupta and Barua employed the SFA model to gauge firms’ green innovation capability, focusing on SMEs in their research (Gupta and Barua, 2017). The second approach involves the utilization of Data Envelopment Analysis (DEA) models, rooted in non-parametric methods. Because the stochastic Frontier (SFA) approach is limited to assessing the efficiency of a single output, and green innovation efficiency involves multiple inputs and outputs, scholars generally favor the data envelopment analysis (DEA) approach (Fernando et al., 2010). In early studies, many scholars predominantly employed traditional radial models, like CCR and BCC models, when choosing DEA models. Lee and Park utilized the DEA model to assess the innovation efficiency of Asian countries, revealing Singapore as having the highest innovation efficiency, whereas China and Korea exhibit comparatively lower innovation efficiency (Lee and Park, 2005). Li and Yang (2020) evaluated the technical efficiency of China’s provincial telecommunication industry using the DEA model, revealing notable regional variations in the technical efficiency of China’s telecommunication industry. With research progress, an increasing number of scholars favor non-radial DEA models, particularly employing the SBM model to gauge green innovation efficiency. For instance, Feng developed a DEA-SBM model to assess the green innovation efficiency of Chinese industrial firms, comparing it to the conventional DEA-CCR model, demonstrating the DEA-SBM model’s alignment with actuality in measuring innovation efficiency (Feng, 2013). Conversely, Cao et al. (2022) employed a non-radial, variable returns to scale Super-SBM model, observing a fluctuating upward trend in China’s industrial green innovation efficiency. Additionally, other scholars have expanded the application of the SBM model to assess green innovation efficiency (He et al., 2015; Tavassoli et al., 2020; Ding et al., 2022; Hashem et al., 2022).
In recent years, scholars have diversified the application of the DEA model, integrating it with various other models to assess green innovation efficiency. Ren et al. (2014) devised a DEA-RAM model, encompassing green, innovation, and economic efficiencies, to gauge the green innovation efficiency within Shanxi province’s industry. Some researchers opt to merge the DEA model with the Luenberger index for their investigations. Tang et al. (2015) developed a non-radial BML-DEA model using the BM directional distance function, DEA model, and Luenberger index to assess industrial environmental efficiency in China. Consequently, Xu and Deng (2022) employed the super-efficient network SBM model and the Malmquist-Luenberger index to appraise the efficiency of green technology innovation within Chinese industrial enterprises. Han et al. (2018) and other scholars developed a two-stage input-output indicator system, employing a dynamic two-stage DEA model to assess technological innovation efficiency in Chinese high-tech enterprises. Building on this work, researchers applied a three-stage network DEA model in empirical studies. Li and Jian (2022) utilized a three-stage chain linkage network DEA model to gauge the innovation efficiency of China’s regional high-tech industries. Their findings indicate that the innovation efficiency in most Chinese regions is suboptimal, necessitating measures for improvement.
Constructing the indicator system is a crucial aspect of the DEA model for assessing the efficiency of green innovation. In earlier studies, many scholars overlooked non-desired outputs in constructing the indicator system. For instance, Nasierowski and Arcelus employed regional production efficiency and the number of patents as output indicators (Nasierowski and Arcelus, 2003). Nevertheless, certain researchers have categorized output indicators into stages. In their work, Ye et al. considered the number of invention patents, patent applications, and technology market turnover as first-stage outputs. They also included the output value of new products, the value of export deliveries, the real GDP growth rate, and the industry’s value added in GDP as second-stage indicators to assess the performance of the high-tech industry (Ye et al., 2012). With the introduction of the new development concept and China’s growing emphasis on green innovation and development, scholars are increasingly focusing on environmental factors and integrating them into output indicators, thereby enhancing the rationality of the indicator system construction. Xiao et al. (2020) characterized undesirable outputs by employing the environmental composite index and energy consumption per unit of industrial GDP as environmental indicators. Building upon this, Wang et al. (2023) concluded that environmental pollution had the most significant impact on the ecological environment, employing carbon dioxide emissions and waste collection volume as metrics for undesirable output.
Based on a literature review, current academic research on green innovation efficiency has developed a comprehensive system. However, there is limited research on the green innovation effectiveness in the manufacturing industry of the Beijing-Tianjin-Hebei region. This paper examines the green innovation efficiency of the manufacturing industry in the Beijing-Tianjin-Hebei region from static and dynamic perspectives. It also provides policy recommendations to enhance the green innovation capacity of the manufacturing industry in the region and promote the transition to a green innovation mode.
Data envelopment analysis (DEA) is commonly employed for evaluating the efficacy of green innovation. Traditional DEA models are typically radial, disregarding slack variables in inputs and outputs, potentially leading to biased efficiency values and an inability to incorporate non-expected outputs. Conversely, a non-radial Slack-Based Measure (SBM) model utilizing slack variables can integrate non-desirable outputs, yet it risks compromising the ratio between target and actual input-output values. Building upon this, Tone and Tsutsui introduced an Enhanced Additive-Based Model (EBM) incorporating a hybrid distance function that amalgamates radial and non-radial characteristics. It preserves the radial ratio between target and actual values and accommodates slack variable variability between factors, thus addressing limitations present in both radial and non-radial models (Tone and Tsutsui, 2010). Owing to the challenge faced by EBM models in comparing the efficiency values of effective decision-making units, this study leverages research by Andersen and Petersen (1993) and Sheng (2022) Eqs 1, 2 to develop a Super-EBM model. This model not only encompasses non-expected outputs but also circumvents the constraints of traditional DEA and SBM models. Moreover, it differentiates between the concurrent presence of multiple decision-making units in effective scenarios. The specific formula is outlined below:
where k is the number of Decision Making Units (DMUs);
The Super-EBM model statically analyzes efficiency levels, lacking dynamic evaluation capabilities. In contrast, the Malmquist Index enables dynamic assessment of efficiency. The traditional Malmquist index, calculated using the output distance function, has limitations; it cannot account for non-desired outputs. In contrast, the Malmquist-Luenberger index, derived from the Malmquist index and computed with the directional distance function, overcomes these limitations. It facilitates measurement of dynamic green innovation efficiency, considering non-desired outputs. This study utilizes the Malmquist-Luenberger (ML) index to assess the dynamic efficiency of green innovation, drawing insights from the works of Chung et al. (1997), Fare et al. (2001), and Wei et al. (2023) Eqs 3–5. Considering non-expected output, the ML index for the period t to t+1 is expressed as:
The ML index can be further decomposed into an index of change in technical efficiency (EC) and an index of change in technical level (TC). Expressed as respectively:
Among them,
This paper combines existing literature research and redefines the input-output indicators in green innovation activities. It constructs the following indicator system based on the principles of scientific, systematic, and operable indicator selection.
Human and capital inputs are necessary for the smooth operation of activities in green innovation. Additionally, energy inputs are incorporated to emphasize the environmentally friendly aspect of innovative processes. Human input typically encompasses labor contributions in innovative processes, often measured by the count of individuals involved in scientific and technological endeavors and the full-time equivalent of R&D personnel. The full-time equivalent (FTE) of R&D staff stands as a globally recognized measure of personnel engagement, being somewhat comprehensive and universally applicable. In line with the research of Yao et al. (2022), this study opts to use FTE of R&D staff as a representation of human input. Capital input generally denotes financial investments in innovative processes, constituting an essential expenditure in innovation. Internal R&D expenditure is consistently monitored. Consequently, this study draws on the findings of Cao et al. (2022) and selects internal R&D expenditure as the representation of capital input. Regarding energy input, the manufacturing industry needs to consider energy consumption in the development process. Enterprises must allocate specific resources before achieving innovative success, often evaluated through total energy consumption. This study selects total energy consumption as a representation of energy intake, drawing from the research conducted by Zheng and Xu (2023).
Considering the environmental impact of energy consumption, this paper divides the output index into desired and undesired components. The expected output is primarily evaluated from two perspectives: innovation and economic outcomes. Considerable uncertainty arises as the number of awarded patents is sensitive to regional regulations, while the number of patent applications signifies the most original innovation output. This better reflects the outcomes of company innovation operations. Therefore, this paper refers to the study conducted by Sun et al. (2022) and chooses the number of patent applications to represent innovation output. Economic production encompasses investments in innovative R&D and the subsequent realization of economic benefits through transformation. Typically, it is measured through revenue generated from new product sales. According to Fan and Gu (2022), the sales revenue of new products is selected to reflect economic output. Environmental pollution level is predominantly attributed to undesirable output. Recently, the Beijing-Tianjin-Hebei region has experienced severe air pollution, significantly compromising air quality. Based on the research of Chen et al. (2022), this paper chooses industrial wastewater emissions, industrial sulfur dioxide emissions, and industrial smoke (dust) emissions to represent environmental pollution outputs. The specific index system is detailed in Table 1.
The focus of this study includes the 13 cities within the Beijing-Tianjin-Hebei region spanning the years 2011–2020, with a specific emphasis on the manufacturing sector above a certain scale. The data used in this study were extracted from several yearbooks published by the National Bureau of Statistics from 2011 to 2020. These include the China Statistical Yearbook on Environment, the China Statistical Yearbook on Energy, the Beijing Statistical Yearbook, the Tianjin Statistical Yearbook, the Hebei Statistical Yearbook, and the statistical yearbooks of Hebei’s prefecture-level cities. Linear interpolation was employed to address gaps in the data. The specifics are outlined in Table 2.
This study utilizes MaxDEA Ultra 8 software to assess the green innovation efficiency of the manufacturing industry in the Beijing-Tianjin-Hebei region from 2011 to 2020. The evaluation involves the application of the Super-EBM model and the green innovation efficiency index system described earlier.
At the global level, as shown in Figure 1, the green innovation efficiency value for the manufacturing industry in the Beijing-Tianjin-Hebei region fluctuates between 0.6 and 0.8, falling short of achieving efficiency. This suggests, to some extent, that the manufacturing industry in the Beijing-Tianjin-Hebei region might experience limited utilization of resource elements and insufficient transformation of economic outcomes and innovations during green innovation activities. There is ample room for improvement in the overall level of green innovation. Examining the temporal trend, the green innovation efficiency value of the manufacturing industry in the Beijing-Tianjin-Hebei region exhibits a general upward trajectory, increasing from 0.686 in 2011 to 0.781 in 2020, marking an overall growth of 9.5%. This suggests that, with the implementation of Beijing-Tianjin-Hebei synergistic development, the level of green innovation in the manufacturing industry in the Beijing-Tianjin-Hebei region has improved to some extent. The green innovation efficiency of the manufacturing industry in the Beijing-Tianjin-Hebei region has progressed through three phases. The initial phase, spanning from 2011 to 2014, witnessed a fluctuating upward trend in the region’s green innovation efficiency. During this period, China introduced policies aimed at optimizing the industrial structure, thereby enhancing the green innovation efficiency within the manufacturing sector of the Beijing-Tianjin-Hebei region. The second stage, occurring between 2014 and 2016, experienced a slight downward trend in the green innovation efficiency of the manufacturing industry within the Beijing-Tianjin-Hebei region. During this phase, the recently initiated Beijing-Tianjin-Hebei collaborative development strategy encountered challenges and difficulties, potentially contributing to the decline. These obstacles may include issues such as the uniformity of the manufacturing industry layout and the lack of rationality in industry transfers. During the third stage, post-2016, the green innovation efficiency in the manufacturing industry of the Beijing-Tianjin-Hebei region exhibits a stable upward trajectory. In this period, coinciding with the 13th Five-Year Plan, China introduced novel development policies for the manufacturing sector. Concurrently, through the comprehensive execution of the Beijing-Tianjin-Hebei coordinated development strategy, continuous adjustments and optimizations have been made to the manufacturing industry structure, resulting in a consistent upward trend in green innovation efficiency within the Beijing-Tianjin-Hebei region.
At the provincial and municipal levels, as illustrated in Figure 2, Beijing exhibits the highest green innovation efficiency in the manufacturing sector, followed by Tianjin, and Hebei shows the lowest efficiency. This pattern aligns with the overall economic strength development in these three regions. Regarding the temporal evolution trend, the green innovation efficiency of manufacturing industries in Beijing and Hebei exhibits consistency, displaying an overall upward trend. Beijing experienced growth from 1.161 in 2011 to 1.226 in 2020, marking an overall increase of 6.5 percent. Similarly, Hebei Province witnessed growth from 0.612 in 2011 to 0.721 in 2020, reflecting an overall increase of 10.9 percent. Notably, the green innovation efficiency of Tianjin’s manufacturing industry generally exhibits the characteristic of a cyclic pattern, initially rising, then falling, and subsequently rising again. The accelerated implementation of Beijing-Tianjin-Hebei coordinated development has driven industrial structure upgrades and innovation. The elimination of low-quality, high-pollution, and high-energy-consuming enterprises, coupled with increased focus on ecological environmental protection and construction, has resulted in heightened green innovation in the manufacturing industry in the Beijing-Tianjin-Hebei region, despite fluctuations. Specifically, Beijing consistently exhibits manufacturing green innovation efficiency values exceeding 1, while Tianjin consistently surpasses this threshold except in 2018 and 2019. In contrast, Hebei’s manufacturing green innovation efficiency values fluctuate within the range of 0.6–0.8. Beijing’s efficiency value is nearly double that of Hebei, while Tianjin consistently surpasses Hebei’s efficiency value, except in 2019 when it slightly lags behind. It is evident that the green innovation efficiency of the manufacturing industry in Beijing and Tianjin has nearly reached an efficient state, whereas Hebei’s manufacturing industry has not attained this level of efficiency. This observation underscores the highly unbalanced development of the manufacturing industry in the Beijing-Tianjin-Hebei region, indicating a considerable distance yet to be covered in achieving collaborative development.
At the city level, significant variations in green innovation efficiency exist among manufacturing industries in different cities within the Beijing-Tianjin-Hebei region (see Table 3). In the Beijing-Tianjin-Hebei region, the average green innovation efficiency of manufacturing industries ranks from highest to lowest as follows: Beijing, Qinhuangdao, Baoding, Tianjin, Handan, Langfang, Zhangjiakou, Hengshui, Tangshan, Xingtai, Shijiazhuang, Chengde, and Cangzhou. Specifically, the efficiency values for Beijing, Qinhuangdao, and Baoding all exceed 1, indicating that the green innovation efficiency of the manufacturing industry in these cities has reached an efficient state. Serving as the capital of China, Beijing exhibits a high standard of economic development, characterized by the dominance of advanced and high-end manufacturing sectors. These include the automobile, pharmaceutical, and computer, communication, and electronic equipment industries, known for their precision and low environmental impact. Furthermore, Beijing boasts abundant capital and human resources, coupled with elevated levels of R&D innovation and technology. It also provides a conducive environment for green innovation in manufacturing. Qinhuangdao, among the earliest cities to commence manufacturing in China, possesses a robust manufacturing base and a favorable ecological environment. Consequently, this contributes to a high level of efficiency in green innovation within the manufacturing industry. Geographically proximate to Beijing and Tianjin, Baoding enjoys a strategic location with convenient and rapid transportation. It possesses abundant labor resources and is dedicated to ecological development. As part of the synergistic industrial development in the Beijing-Tianjin-Hebei region, Baoding actively facilitates the transfer of industries from Beijing and Tianjin. This positions Baoding at the forefront of green innovation efficiency in the manufacturing industry.
The cities ranked with a mean green innovation efficiency in manufacturing exceeding 0.5 are Tianjin, Handan, Tangshan, Xingtai, Zhangjiakou, Langfang, and Hengshui, respectively. The mean green innovation efficiency of Tianjin’s manufacturing industry is 0.959, but in 2018 and 2019, it surpasses This could be attributed to Tianjin’s abundant human resources, distinct location advantages, and robust scientific and technological innovation capability. However, during 2018 and 2019, despite increased human and capital innovation inputs, the innovation and economic outputs relatively declined, leading to the green innovation efficiency of its manufacturing industry falling below 1, indicating inefficiency. Handan’s manufacturing industry has an average green innovation efficiency of 0.852. The industry’s green innovation efficiency generally increased before 2019 but showed a sharp decline after that year. Shijiazhuang, Chengde, and Cangzhou exhibit manufacturing green innovation efficiency mean values below 0.5, standing at 0.429, 0.4, and 0.341, correspondingly. Despite fluctuations, these cities’ manufacturing green innovation efficiency values remain consistently low. Shijiazhuang leads in investment in labor, funding, and energy within Hebei Province. However, the prevalence of highly polluting and energy-intensive industries results in a low green innovation efficiency within its manufacturing sector. Despite Chengde’s focus on ecological construction, its low-quality labor force, limited scientific and technological innovation, and weak industrial foundation contribute to its inefficient green innovation efficiency. Cangzhou suffers from a weak industrial structure, lagging R&D and innovation, and a relatively subpar ecological environment, impacting its manufacturing industry’s green innovation efficiency.
This study employes the ML index to dynamically analyze the efficiency of green innovation in the manufacturing industry, building upon static analysis. The ML index measures the efficiency of green innovation in the Beijing-Tianjin-Hebei manufacturing industry, along with its derived technical efficiency change index (EC) and technical level change index (TC), calculated using MaxDEA Ultra 8 software.
At the global level, as shown in Table 4, the average ML index value for green innovation efficiency in the manufacturing industry of the Beijing-Tianjin-Hebei region exceeds 1. The average growth in green innovation efficiency within the manufacturing sector is 8%, signifying an overall upward trend in green innovation within the Beijing-Tianjin-Hebei manufacturing industry. Further decomposition of the ML index reveals that, during the assessment period, the average values for both the technical efficiency change index and the technical level change index of green innovation in the manufacturing industry of Beijing-Tianjin-Hebei surpass 1. The average growth rates are 4.9% for the technical efficiency change index and 3.9% for the technical level change index. This indicates that technical efficiency and technical level collectively drive the enhancement of green innovation in the manufacturing industry of the Beijing-Tianjin-Hebei region, with the degree of technical efficiency change exceeding that of technological level. Specifically, prior to 2015, the average ML index for green innovation efficiency in the manufacturing industry of the Beijing-Tianjin-Hebei region was below 1. Subsequently, post-2015, the average ML index exceeded 1. This suggests that the adoption of the Beijing-Tianjin-Hebei coordinated development strategy has led to a continual improvement in the level of green innovation within the manufacturing industry of the Beijing-Tianjin-Hebei region. This improvement has also contributed to a certain extent to the high-quality development of the economy in the Beijing-Tianjin-Hebei region.
At the provincial and municipal levels, as depicted in Figure 3, the ML index values for green innovation efficiency in the manufacturing sectors of Beijing, Tianjin, and Hebei exceed 1. Notably, Hebei registers the highest ML index value, trailed by Tianjin, with Beijing recording the lowest. The dynamic efficiency of green innovation has, on average, risen by 8.3% in Hebei and 6.8% in Tianjin, contrasting with Beijing’s more modest average growth rate of 5.6%. This indicates that the manufacturing industry in Beijing, with its high level of green innovation, faces more limited developmental opportunities compared to Hebei, resulting in slower growth. Hebei’s economic prowess, technological advancement, and innovation level significantly lag behind those of Beijing. Nevertheless, recent years have witnessed significant improvements in Hebei’s industrial structure, economic development level, scientific and technological innovation, and resource allocation, owing to national policy adjustments and the coordinated development strategy among Beijing, Tianjin, and Hebei, thereby boosting the green innovation efficiency within Hebei’s manufacturing sector. The technological efficiency change index for Beijing, Tianjin, and Hebei is 1.006, 1.014, and 1.056, respectively, upon further division of the ML index. On average, the technological efficiency change index increased by 0.6% in Beijing and 1.4% in Tianjin, while Hebei experienced significant growth of 5.6%, surpassing the Beijing-Tianjin region. The technological level change index for Beijing, Tianjin, and Hebei is 1.050, 1.066, and 1.036, respectively. The average growth rates of the technical level change index are 5.0% in Beijing, 6.6% in Tianjin, and 3.6% in Hebei, all of which are lower than the rates in the Beijing-Tianjin region. The technical level change index for green innovation in the manufacturing industry is higher than the technical efficiency change index in Beijing and Tianjin. This demonstrates that the enhancement of green innovation in the manufacturing industry in Beijing and Tianjin is attributed to technological progress, whereas in Hebei, it is attributed to improvements in technical efficiency.
At the city level, see Table 5, all cities in the Beijing-Tianjin-Hebei region, except Langfang and Hengshui, exhibit ML index values exceeding 1. Moreover, the majority of Hebei’s cities demonstrate significantly higher ML index values compared to Beijing and Tianjin. The green innovation levels of most cities in the Beijing-Tianjin-Hebei manufacturing sector have improved to some degree, with a more significant increase observed in the Hebei region. This observation reflects the attainment of specific outcomes in the coordinated development of Beijing-Tianjin-Hebei. Specifically, the mean ML index values for green innovation efficiency in manufacturing industries in Langfang and Hengshui are 0.957 and 0.961, respectively. This suggests a declining trend in the dynamic efficiency of green innovation in these manufacturing sectors during the examination period. This decline is primarily attributed to the dual influence of changes in technical efficiency and technological level. Notably, the impact of changes in technical efficiency is more pronounced, signifying a deficiency in technological innovation capacity, irrational allocation of resource factors, and other issues in the manufacturing industry of these two cities. Consequently, this results in a decrease in the green dynamic efficiency of their manufacturing sectors. Additionally, in Shijiazhuang, Xingtai, Chengde, and Cangzhou, the mean technical efficiency change index for manufacturing green innovation surpasses the technology level change index. This implies that the enhancement in the dynamic efficiency of manufacturing green innovation in these cities primarily stems from improved technical efficiency. Conversely, in Tangshan, Qinhuangdao, Handan, Baoding, and Zhangjiakou, the boost in dynamic efficiency for manufacturing green innovation primarily results from technological progress.
Cultivating green innovation is crucial for enhancing the quality of regional development. The industrial sector in the Beijing-Tianjin-Hebei region is grappling with notable challenges, particularly concerning resource utilization, energy deficits, and environmental pollution. Consequently, green innovation stands as a crucial requirement for achieving sustainable long-term development (Liu et al., 2021). This study applies the Super-EBM model and the Malmquist-Luenberger index to evaluate the efficiency of green innovation in the manufacturing sector within the Beijing-Tianjin-Hebei region. This approach contributes to the enrichment and expansion of pertinent research content, thereby fostering the coordinated development of the region.
Based on static analysis, the research indicates that the overall green innovation efficiency in the Beijing-Tianjin-Hebei manufacturing industry has not yet achieved efficiency, aligning with the findings of Wu and Chen (2017). There is a significant disparity in the level of development within the Beijing-Tianjin-Hebei region. With China emphasizing the five development concepts of “innovation, coordination, green, openness, and sharing” and implementing the coordinated development strategy for Beijing-Tianjin-Hebei, the region has increasingly focused on green and innovative development. It has actively facilitated the transformation and upgrading of the manufacturing industry, progressing toward high-quality manufacturing development. Provincially, Beijing and Tianjin exhibit significantly higher green innovation efficiency compared to Hebei, with Hebei’s green innovation efficiency score below 1. Beijing and Tianjin possess significantly stronger economic development capabilities than Hebei, with a greater abundance of personnel, capital, material resources, and robust scientific and technological innovation capabilities. Despite Hebei having greater resource endowments, its economic development lags behind. The traditional manufacturing industry is more widely distributed, and the R&D level is inadequate, resulting in Hebei’s manufacturing industry lagging significantly in green innovation efficiency compared to Beijing and Tianjin. This poses a challenge to the transformation and modernization of the Hebei manufacturing industry (Lin and Meng, 2020; Ying et al., 2021). At the city level, we infer that Beijing, Qinhuangdao, and Baoding have achieved DEA-effective manufacturing green innovation efficiency, surpassing other cities. Beijing leads in green innovation efficiency within the manufacturing sector, attributed to its dominance in advanced and high-end manufacturing, along with a conducive environment for green innovation. Qinhuangdao and Baoding secured the second and third positions, respectively. Qinhuangdao’s manufacturing industry has a stable foundation and an optimal environmental setting, while Baoding’s advantageous geographical location and convenient transportation position its manufacturing green innovation efficiency at the forefront. Tianjin experienced a significant decline in green innovation efficiency during 2018–2019, leading to a decrease in its overall rating. Other cities in Hebei exhibit lower green innovation efficiency, resulting in a lower ranking. This implies that Hebei faces significant challenges in transitioning the manufacturing industry to a green innovation mode within the Beijing-Tianjin-Hebei region. This finding contrasts slightly with the results of Zhu et al. (2022), possibly due to differences in study methodologies, the constructed evaluation index system, and the scope of research.
The findings of dynamic study indicate an advancing total green innovation level in the manufacturing industry of the Beijing-Tianjin-Hebei region. Analyzing the ML index reveals that the increase in the green innovation level is mainly linked to improved technological efficiency. Before the adoption of the coordinated development strategy in 2015, the ML index was consistently below 1. After implementation, the ML index exceeds 1, and the green innovation level in the Beijing-Tianjin-Hebei region is steadily increasing. Provincially, Beijing, Tianjin, and Hebei all have ML index values above 1, with Hebei being the highest, Tianjin the second highest, and Beijing the lowest. Similarly, the increase in green innovation in Beijing and Tianjin is primarily attributed to technological growth, while Hebei gains from enhanced technical efficiency (Zhou and Shao, 2023). The green innovation level in the industrial sector in Beijing has consistently shown resilience, but there is less room for improvement compared to Hebei. Resulting from the shift in national policies and the recent transformation and growth of Hebei, there has been a substantial enhancement in its resource allocation, R&D innovation, and economic development, leading to a consistent rise in its green innovation level in the manufacturing industry. Beijing and Tianjin host numerous research institutes with generous talent subsidies, leading to the retention of exceptional experts and advanced technological capabilities. This difference in innovation efficiency improvement among Beijing, Tianjin, and Hebei is highlighted by Dong et al. (2022). At the city level, excluding Langfang and Hengshui, ML index values in the remaining cities all exceed one. The primary reason for the decline in the dynamic efficiency of green innovation in the manufacturing industries of Langfang and Hengshui is the fluctuation in technical efficiency. There is no doubt that the two cities still face significant constraints in terms of resource distribution, technological innovation capability, and other areas. Through the implementation of coordinated development in the Beijing-Tianjin-Hebei region, Beijing has downsized non-capital functions. Additionally, Beijing and Tianjin are initiating the relocation of certain manufacturing enterprises to Hebei (Zhang, 2020). In 2017, the proposal to establish the Xiongan New Area in Hebei Province emerged, aiming to catalyze high-quality manufacturing development in the Beijing-Tianjin-Hebei region. Hebei has actively pursued the transfer of industries between Beijing and Tianjin, implementing continuous industrial transformation, upgrading, and strategic layout. This has contributed to the ongoing improvement of green innovation efficiency in the manufacturing sector.
This study assesses the green innovation efficiency of the manufacturing industry in the Beijing-Tianjin-Hebei region from 2011 to 2020. It achieves this by establishing an index system for green innovation efficiency evaluation and utilizing the Super-EBM model, which incorporates non-expected outputs. Subsequently, the study dynamically evaluates the green innovation efficiency of the manufacturing industry using the ML index based on static analyses. The research yields the following conclusions:
From a static perspective, the average green innovation efficiency in the manufacturing industry of the Beijing-Tianjin-Hebei region is consistently below one, indicating inefficiency. Regarding temporal evolution, the green innovation efficiency in the manufacturing industry of Beijing-Tianjin-Hebei exhibits a fluctuating upward trend. Secondly, there is a notable disparity in green innovation efficiency among the manufacturing industries of Beijing, Tianjin, and Hebei, with Beijing and Tianjin significantly surpassing Hebei. This aligns with their respective overall economic strengths. Except for a few years, both Tianjin and Beijing manufacturing industries have consistently surpassed the production Frontier in green innovation efficiency. However, Hebei’s values fall short of the Frontier, highlighting a notable disparity among Hebei, Beijing, and Tianjin. In terms of temporal evolution, the green innovation efficiency of the manufacturing industry in Beijing and Hebei shows a consistent overall upward trend. In contrast, Tianjin follows a pattern of “initial rise, subsequent fall, followed by a rise again.” Finally, the green innovation efficiency of industrial sectors exhibits significant variation among cities in the Beijing-Tianjin-Hebei region. Beijing, Qinhuangdao, and Baoding are the only cities with a median green innovation efficiency score above one, indicating DEA effectiveness. In contrast, the other cities have scores below one, indicating DEA ineffectiveness.
From a dynamic perspective, the green innovation efficiency of the manufacturing industry in the Beijing-Tianjin-Hebei region has generally increased. Both technical efficiency and technical level contribute to this improvement, with technical efficiency showing a more pronounced change than technical level. The average ML index value for green innovation efficiency in the manufacturing sector of the Beijing-Tianjin-Hebei region exceeds one, showing an average growth of 8%. In comparison, the indices for changes in technological efficiency and technological level stand at 4.9% and 3.9%, respectively. Secondly, the green innovation efficiency of the manufacturing sectors in Beijing, Tianjin, and Hebei has generally improved, though with significant variations among them. The ML index values for green innovation efficiency in Beijing, Tianjin, and Hebei’s manufacturing sectors are all above one, with Hebei leading, Tianjin following, and Beijing trailing. Technological progress drives the improvement in green innovation in the manufacturing sectors of Beijing and Tianjin, while Hebei benefits from enhanced technical efficiency in advancing its green innovation. Ultimately, most cities in the Beijing-Tianjin-Hebei region have seen consistent improvements in manufacturing industry green innovation efficiency, with Hebei showing the most significant increase. Apart from Langfang and Hengshui, the average ML index value for green innovation efficiency in the remaining cities of the Beijing-Tianjin-Hebei region exceeds one, with Hebei’s values notably surpassing those of Beijing and Tianjin.
Firstly, enhancing the industrial structure and promoting green development. On the one hand, Beijing and Tianjin should enhance the transformation of both old and new driving forces. This involves actively transforming or eliminating industries with high pollution and energy consumption. The focus should be on developing high-end manufacturing sectors like automobile, pharmaceutical, computer, communication, and electronic equipment manufacturing. Moreover, there is a need to increase investment in research and development (R&D) for the advancement of high-tech industries and facilitate the co-development of such sectors. Drive the industrial sector of the entire Beijing-Tianjin-Hebei region towards high-end and environmentally friendly development. On the other hand, the government is obligated to guide the industrial transfer, actively promote the relocation of industries to Hebei province, and adjust the spatial layout. Efforts should be made to promote the complementary cooperation of talents and other innovative factors, optimize innovation resource allocation, and thereby facilitate the coordinated expansion of the industrial structure in the Beijing-Tianjin-Hebei region. On one hand, Hebei should actively engage in the industrial transfer from Beijing and Tianjin, while also undergoing a positive transformation to promote the development of industrial clusters and economies of scale. On the other hand, Hebei should encourage the upgrade of industrial structures based on its own resource endowment features and implement actions that rely on local conditions. Hebei should enhance and strengthen traditional competitive industries such as steel, equipment manufacturing, petrochemicals, and food. Simultaneously, it should eliminate conventional industries with high pollution and energy consumption. Additionally, Hebei should cultivate and strengthen strategic emerging industries, including next-generation technologies, biomedicine, new energy, and novel materials, while optimizing its manufacturing layout. The aim is to promote the development of the manufacturing industry structure through a low-carbon and green path.
Secondly, enhancing technical innovation capabilities and optimizing resource allocation. Leveraging their respective strengths, Beijing and Tianjin should enhance collaboration between enterprises, universities, and research institutes. They should ensure universities consistently produce high-quality professionals for enterprises, foster ongoing technological innovation, strengthen independent innovation capabilities, and advance the level of green innovation in manufacturing. Neighboring cities with low green efficiency, such as Langfang, Chengde, and Tangshan, should receive targeted assistance. Additionally, a platform for sharing green innovative technology should be established to facilitate the development of the collaborative innovation system in the Beijing-Tianjin-Hebei region and promote the green transformation and upgrading of industries. Hebei should, on one hand, transform its traditional commercial model. It should prioritize innovative investment in the innovation process, proactively engage in technological change and innovation, increase investment in industrial R&D, establish a fully functional technology transformation platform and achievement trading platform, and facilitate the transformation of numerous scientific and technological achievements. On the other hand, it is essential to nurture innovative entrepreneurs who can lead in innovation, promote the deep integration of industries, universities, and research institutes, optimize input-output factors in the green innovation process in manufacturing, reduce production and operation costs, and enhance the efficiency of green innovation in manufacturing.
Ultimately, promoting ecological and environmental management to enhance economic efficiency. In recent years, the Beijing-Tianjin-Hebei region has faced severe air pollution and compromised air quality. On one hand, the government should rigorously enforce corporate pollution emissions licensing and management, establish a robust ecological and environmental protection management system with synergistic mechanisms, increase investment in industrial pollution control in the Beijing-Tianjin-Hebei region, enhance the utilization rate of pollutants by converting them into other valuable resources, and heighten enterprise awareness of green development. This will contribute to enhancing the economic efficiency of enterprises. On the other hand, as the manufacturing sector’s industrial structure undergoes upgrades, Beijing and Tianjin should enhance environmental protection efforts. This involves implementing comprehensive systems for total energy and resource management and conservation, as well as promoting the recycling of energy elements. Hebei should reconsider its initial extensive expansion of the manufacturing industry and implement stringent control over enterprise pollution emissions. A corresponding punitive mechanism can be established to reinforce the establishment of a corporate environmental responsibility system. Additionally, relevant departments can organize comprehensive pollution treatment measures to reduce emissions from the manufacturing industry, aiming to enhance resource utilization and support the development of high-quality manufacturing.
Due to limitations in research data availability and other factors, the green innovation efficiency evaluation index system developed in this study still exhibits deficiencies. There may be additional indicators with stronger relevance that are not incorporated into the evaluation index system, warranting improvement. Furthermore, following the assessment of green innovation efficiency in the manufacturing industry of the Beijing-Tianjin-Hebei region, there is an opportunity to delve into the spatial and temporal characteristics, as well as the influencing factors impacting green innovation efficiency. This aspect can be explored more comprehensively in future studies.
The raw data supporting the conclusion of this article will be made available by the authors, without undue reservation.
CZ: Conceptualization, Formal Analysis, Methodology, Supervision, Writing–review and editing. MY: Conceptualization, Methodology, Software, Validation, Writing–original draft, Writing–review and editing. ZZ: Writing–review and editing, Funding acquisition. ML: Conceptualization, Methodology, Writing–review and editing.
The author(s) declare financial support was received for the research, authorship, and/or publication of this article. The research was funded by the National Natural Science Foundation of China (71973051 and 71703009), and the Social Science Foundation of Beijing (16GLB019 and 16GLC063).
Author ZZ was employed by Henan Yuzhan Precision Technology Limited Company.
The remaining authors declare that the research was conducted in the absence of any commercial or financial relationships that could be construed as a potential conflict of interest.
All claims expressed in this article are solely those of the authors and do not necessarily represent those of their affiliated organizations, or those of the publisher, the editors and the reviewers. Any product that may be evaluated in this article, or claim that may be made by its manufacturer, is not guaranteed or endorsed by the publisher.
Akbari, M., Padash, H., Parizi, Z. S., Rezaei, H., Shahriari, E., and Khosravani, A. (2022). A bibliometric review of green innovation research: identifying knowledge domain and network. Qual. Quantity 56 (6), 3993–4023. doi:10.1007/s11135-021-01295-4
Albort-Morant, G., Leal-Millán, A., and Cepeda-Carrión, G. (2016). The antecedents of green innovation performance: a model of learning and capabilities. J. Bus. Res. 69 (11), 4912–4917. doi:10.1016/j.jbusres.2016.04.052
Albrizio, S., Kozluk, T., and Zipperer, V. (2017). Environmental policies and productivity growth: evidence across industries and firms. J. Environ. Econ. Manag. 81, 209–226. doi:10.1016/j.jeem.2016.06.002
Andersen, P., and Petersen, N. C. (1993). A procedure for ranking efficient units in data envelopment analysis. Manag. Sci. 39 (10), 1261–1264. doi:10.1287/mnsc.39.10.1261
Beise, M., and Rennings, K. (2005). Lead markets and regulation: a framework for analyzing the international diffusion of environmental innovations. Ecol. Econ. 52 (1), 5–17. doi:10.1016/j.ecolecon.2004.06.007
Broekel, T. (2015). Do cooperative research and development (R&D) subsidies stimulate regional innovation efficiency? Evidence from Germany. Reg. Stud. 49 (7), 1087–1110. doi:10.1080/00343404.2013.812781
Cao, K. (2020). An empirical test of technological innovation efficiency in equipment manufacturing industry based on SFA. Statistics Decis. 36 (20), 72–75. doi:10.13546/j.cnki.tjyjc.2020.20.015
Cao, L., Yang, H. C., and Li, L. S. (2022). Spatial and temporal differentiation characteristics and dynamic evolution of industrial green innovation efficiency. Stud. Sci. Sci. 40 (10), 1895–1906. doi:10.16192/j.cnki.1003-2053.20220304.001
Chakraborty, P., and Chatterjee, C. (2017). Does environmental regulation indirectly induce upstream innovation? New evidence from India. Res. Policy 46 (5), 939–955. doi:10.1016/j.respol.2017.03.004
Chen, B., Peng, W. B., and Liu, Y. F. (2022). Spatio-temporal evolution and driving factors of green innovation efficiency of the urban agglomeration in the middle reaches of the yangtze river. Econ. Geogr. 42 (9), 43–49. doi:10.15957/j.cnki.jjdl.2022.09.005
Chen, W., Wang, X. F., Peng, N., Wei, X., and Lin, C. R. (2020). Evaluation of the green innovation efficiency of Chinese industrial enterprises: research based on the three-stage chain network SBM model. Math. Problems Eng. 2020, 1–11. doi:10.1155/2020/3143651
Cherniwchan, J. (2012). Economic growth, industrialization, and the environment. Resour. Energy Econ. 34 (4), 442–467. doi:10.1016/j.reseneeco.2012.04.004
Chung, Y. H., Fare, R., Grosskopf, S., and Rosskopf, S. (1997). Productivity and undesirable outputs: a directional distance function approach. J. Environ. Manag. 51 (3), 229–240. doi:10.1006/jema.1997.0146
Ding, J., Liu, B. L., and Shao, X. F. (2022). Spatial effects of industrial synergistic agglomeration and regional green development efficiency: evidence from China. Energy Econ. 112, 106156–106211. doi:10.1016/j.eneco.2022.106156
Dong, H. Z., Cao, Z. X., and Zhang, R. J. (2022). Identification of two-stage green innovation efficiency and influencing factors in China's high-tech industries. Statistics Decis. 38 (6), 44–49. doi:10.13546/j.cnki.tjyjc.2022.06.009
Fan, D. C., and Gu, X. M. (2022). An analysis of the key influencing factors of technological innovation efficiency in high-tech industries: an empirical study based on the DEA-Malmquist and Bayesian Model Averaging approach. Sci. Res. Manag. 43 (1), 70–78. doi:10.19571/j.cnki.1000-2995.2022.01.008
Fare, R., Grosskopf, S., and Pasurka, J. R. (2001). Accounting for air pollution emissions in measures of state manufacturing productivity growth. J. Regional Sci. 41 (3), 381–409. doi:10.1111/0022-4146.00223
Feng, Z. J. (2013). Research on industrial enterprises' green innovation efficiency in China. Forum Sci. Technol. China 2, 82–88. doi:10.13580/j.cnki.fstc.2013.02.015
Feng, Z. J., Zeng, B., and Ming, Q. (2018). Environmental regulation, two-way foreign direct investment, and green innovation efficiency in China’s manufacturing industry. Int. J. Environ. Res. public health 15 (10), 2292. doi:10.3390/ijerph15102292
Fernando, J. S., Iturriagagoitia, M. J., Zofio, L. J., and Elena, C. M. (2010). Evaluating research efficiency within national R&D programmes. Res. Policy 40 (2), 230–241. doi:10.1016/j.respol.2010.10.005
Ford, A. J., Steen, J., and Verreynne, M. (2014). How environmental regulations affect innovation in the Australian oil and gas industry: going beyond the Porter Hypothesis. J. Clean. Prod. 84 (1), 204–213. doi:10.1016/j.jclepro.2013.12.062
Gupta, H., and Barua, M. K. (2017). Supplier selection among SMEs on the basis of their green innovation ability using BWM and fuzzy TOPSIS. J. Clean. Prod. 152, 242–258. doi:10.1016/j.jclepro.2017.03.125
Han, B., Su, Y., Li, T., and Wan, M. (2018). Technological innovation performance of high-tech enterprises based on two-stage DEA model. Sci. Res. Manag. 39 (3), 11–19. doi:10.19571/j.cnki.1000-2995.2018.03.002
Han, J. (2010). An empirical analysis of China's high---technology industry innovation efficiency based on SFA. Stud. Sci. Sci. 28 (03), 467–472. doi:10.16192/j.cnki.1003-2053.2010.03.016
Hashem, O., Meisam, S., and Ali, E. (2022). Evaluating sustainable efficiency of decision-making units considering undesirable outputs: an application to airline using integrated multi-objective DEA-TOPSIS. Environ. Dev. Sustain. 25 (7), 5899–5930. doi:10.1007/s10668-022-02285-8
He, F., Zhu, L. Y., Ma, D. D., and Jiang, W. (2015). Research on the green technical efficiency of China s iron and steel enterprise. China Ind. Econ. 7, 84–98. doi:10.19581/j.cnki.ciejournal.2015.07.006
Johnstone, N., Haščič, I., Poirier, J., Hemar, M., and Michel, C. (2012). Environmental policy stringency and technological innovation: evidence from survey data and patent counts. Appl. Econ. 44, 2157–2170. doi:10.1080/00036846.2011.560110
Lee, H., and Park, Y. (2005). An international comparison of R&D efficiency: DEA approach. Asian J. Technol. Innovation 13 (2), 207–222. doi:10.1080/19761597.2005.9668614
Li, D., and Shen, W. (2021). Can corporate digitalization promote green innovation? The moderating roles of internal control and institutional ownership. Sustainability 13 (24), 13983. doi:10.3390/su132413983
Li, P. Z., and Jian, L. R. (2022). Research on innovation process efficiency of regional high-tech industry. Stud. Sci. Sci. 40 (4), 713–722+733. doi:10.16192/j.cnki.1003-2053.20210720.002
Li, Z. Y., and Yang, S. H. (2010). Technical efficiency of provincial telecom: regional difference and affecting factors. China Ind. Econ. 8, 129–139. doi:10.19581/j.cnki.ciejournal.2010.08.013
Liang, W. J., and He, P. (2022). Research on regional coordinated innovation monitoring system in beijing-tianjin-hebei region: a comparison of regional coordinated innovation with yangtze river delta. Stat. Res. 39 (3), 132–141. doi:10.19343/j.cnki.11-1302/c.2022.03.009
Lin, P., and Meng, N. N. (2020). Quality measurement and dynamic deconstruction of Beijing-Tianjin-Hebei regional economic development under environmental constraints: from the perspective of green total factor productivity. Econ. Geogr. 40 (9), 36–45. doi:10.15957/j.cnki.jjdl.2020.09.004
Liu, H. M., Long, J. C., and Shen, Z. H. (2023). Research on the impact of digital transformation on corporate green innovation. Sci. Res. Manag. 44 (10), 22–34. doi:10.19571/j.cnki.1000-2995.2023.10.003
Liu, P. Z., Zhang, L. Y., Heather, T., and Yan, Z. Y. (2021). Analysis on spatio-temporal characteristics and influencing factors of industrial green innovation efficiency—from the perspective of innovation value chain. Sustainability 14 (1), 342–362. doi:10.3390/su14010342
Mitsutsugu, H. (2005). Environmental regulation and the productivity of Japanese manufacturing industries. Resour. Energy Econ. 28 (4), 299–312. doi:10.1016/j.reseneeco.2005.11.001
Nasierowski, W., and Arcelus, F. (2003). On the efficiency of national innovation systems. Socio-Economic Plan. Sci. 37 (3), 215–234. doi:10.1016/s0038-0121(02)00046-0
Ren, Y., Niu, C. H., Niu, T., and Yao, X. L. (2014). Theoretical modelling and empirical research on green innovation efficiency. J. Manag. World 7, 176–177. doi:10.19744/j.cnki.11-1235/f.2014.07.020
Rubashkina, Y., Galeotti, M., and Verdolini, E. (2015). Environmental regulation and competitiveness: empirical evidence on the Porter Hypothesis from European manufacturing sectors. Energy Policy 83, 288–300. doi:10.1016/j.enpol.2015.02.014
Sheng, D. T. (2022). Calculation and analysis of High quality economic development efficiency in the central region. Mod. Bus. 2 (4), 85–87. doi:10.14097/j.cnki.5392/2022.04.039
Sun, Z. R., Fan, J., Sun, Y., and Liu, H. C. (2022). Structural characteristics and influencing factors of spatial correlation network of green scientific and technological innovation efficiency in China. Econ. Geogr. 42 (3), 33–43. doi:10.15957/j.cnki.jjdl.2022.03.004
Tang, L. W., Hu, Z. Y., and Zhang, Y. J. (2015). The measurement of regional industrial environmental performance based on non-radial BML-DEA model. Stat. Res. 32 (3), 21–28. doi:10.19343/j.cnki.11-1302/c.2015.03.003
Tavassoli, M., Fathi, A., and Saen, F. R. (2020). Developing a new super-efficiency DEA model in the presence of both zero data and stochastic data: a case study in the Iranian airline industry. Benchmarking Int. J. 28 (1), 42–65. doi:10.1108/bij-01-2020-0044
Tone, K., and Tsutsui, M. (2010). An epsilon-based measure of efficiency in DEA -A third pole of technical efficiency. Eur. J. Operational Res. 207 (3), 1554–1563. doi:10.1016/j.ejor.2010.07.014
Wang, S., Han, J. Y., and Zhao, X. D. (2023). Research on tourism development efficiency and influencing factors in the Yellow River Basin of Inner Mongolia based on undesired output. J. Arid Land Resour. Environ. 37 (8), 195–200. doi:10.13448/j.cnki.jalre.2023.201
Wei, Q. F., Tan, J., and Shi, L. N. (2023). Study on industrial green TFP measurement and spatial differentiation in Chengdu-Chongqing Shuangcheng Economic Circle. Soft Sci. 37 (2), 103–111. doi:10.13956/j.ss.1001-8409.2023.02.14
Wu, K., Shi, J., and Yang, T. (2017). Has energy efficiency performance improved in China? non-energy sectors evidence from sequenced hybrid energy use tables. Energy Econ. 67, 169–181. doi:10.1016/j.eneco.2017.08.010
Wu, Y. Q., and Chen, Y. (2017). An empirical study on manufacturing competitiveness in Beijing-Tianjin-Hebei region: based on the analysis of three economic censuses. J. Hebei Univ. Econ. Bus. 37 (6), 119–126. doi:10.14178/j.cnki.issn1007-2101.2016.06.021
Xiao, R. Q., Shen, L., and Qian, L. (2020). Study on the green innovation efficiency and its influencing factors of industrial enterprises in provinces along " the belt and road. Soft Sci. 34 (8), 37–43. doi:10.13956/j.ss.1001-8409.2020.08.07
Xu, Y. H., and Deng, H. T. (2022). Green total factor productivity in Chinese cities: measurement and causal analysis within a new structural economics framework. J. Innovation Knowl. 7 (4), 100235. doi:10.1016/j.jik.2022.100235
Yang, H. C., Li, L. S., and Liu, Y. B. (2021). Evaluation of the innovation-driven force of regional manufacturing industry and its differences. Stud. Sci. Sci. 39 (10), 1908–1920. doi:10.16192/j.cnki.1003-2053.20210312.006
Yao, M. C., Duan, J. J., Zhang, R. J., and Xuan, Z. Y. (2022). Spatial correlation structure and influencing mechanism of green innovation efficiency in China's high-tech industry. Resour. Environ. Yangtze Basin 31 (11), 2345–2356. doi:10.11870/cjlyzyyhj202211002
Ye, R., Yang, J. F., and Chang, Y. K. (2012). Efficiency measurement and decomposition of China's provincial hi-tech industry. J. Quantitative Technol. 29 (7), 3–17+91. doi:10.13653/j.cnki.jqte.2012.07.001
Yi, M., Peng, J. C., and Wu, C. (2019). Innovation efficiency of high-tech industries based on stochastic frontier method in China. Sci. Res. Manag. 40 (11), 22–31. doi:10.19571/j.cnki.1000-2995.2019.11.003
Ying, L. M., Li, M. H., and Yang, J. (2021). Agglomeration and driving factors of regional innovation space based on intelligent manufacturing and green economy. Environ. Technol. Innovation 22, 101398–101413. doi:10.1016/j.eti.2021.101398
Yuan, B., and Xiang, Q. (2018). Environmental regulation, industrial innovation and green development of Chinese manufacturing: based on an extended CDM model. J. Clean. Prod. 176 (1), 895–908. doi:10.1016/j.jclepro.2017.12.034
Zhang, Y. L. (2020). Research on green innovation efficiency of manufacturing industry in Beijing-Tianjin-Hebei region. Value Eng. 39 (14), 108–109. doi:10.14018/j.cnki.cn13-1085/n.2020.14.047
Zheng, Z. Y., and Xu, Y. B. (2023). Carbon emission trading mechanism, family control and green innovation efficiency. Chin. J. Environ. Manag. 15 (5), 53–66. doi:10.16868/j.cnki.1674-6252.2023.05.053
Zhou, J. Y., and Shao, M. W. (2023). Evaluation of green innovation efficiency in Chinese provincial regions under high-quality development and its influencing factors: an empirical study based on hybrid data envelopment analysis and multilevel mixed-effects tobit models. Sustainability 15 (14), 11079–11145. doi:10.3390/su151411079
Keywords: Beijing-Tianjin-Hebei region, manufacturing, green innovation efficiency, Super-EBM model, Malmquist-Luenberger index
Citation: Zhong C, Yu M, Zhang Z and Lu M (2024) Green innovation efficiency measurement of manufacturing industry in the Beijing-Tianjin-Hebei region of China based on Super-EBM model and Malmquist-Luenberger index. Front. Energy Res. 12:1337188. doi: 10.3389/fenrg.2024.1337188
Received: 12 November 2023; Accepted: 31 January 2024;
Published: 22 February 2024.
Edited by:
Michael Carbajales-Dale, Clemson University, United StatesReviewed by:
Hong-Dian Jiang, China University of Geosciences, ChinaCopyright © 2024 Zhong, Yu, Zhang, Lu. This is an open-access article distributed under the terms of the Creative Commons Attribution License (CC BY). The use, distribution or reproduction in other forums is permitted, provided the original author(s) and the copyright owner(s) are credited and that the original publication in this journal is cited, in accordance with accepted academic practice. No use, distribution or reproduction is permitted which does not comply with these terms.
*Correspondence: Milin Lu, bHVtaWxpbkBiaXB0LmVkdS5jbg==
Disclaimer: All claims expressed in this article are solely those of the authors and do not necessarily represent those of their affiliated organizations, or those of the publisher, the editors and the reviewers. Any product that may be evaluated in this article or claim that may be made by its manufacturer is not guaranteed or endorsed by the publisher.
Research integrity at Frontiers
Learn more about the work of our research integrity team to safeguard the quality of each article we publish.