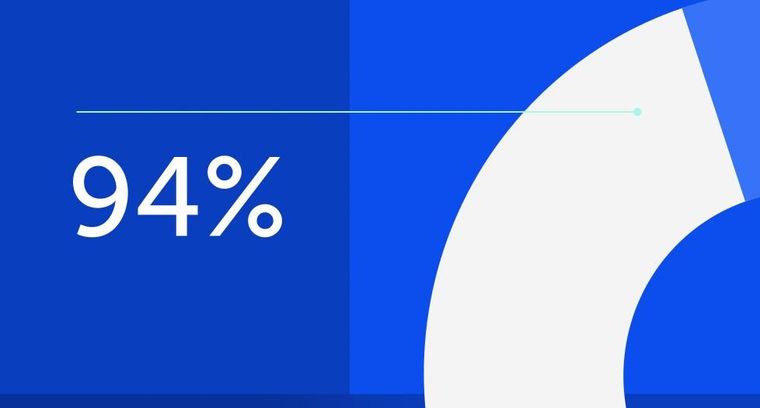
94% of researchers rate our articles as excellent or good
Learn more about the work of our research integrity team to safeguard the quality of each article we publish.
Find out more
MINI REVIEW article
Front. Energy Res., 29 December 2023
Sec. Process and Energy Systems Engineering
Volume 11 - 2023 | https://doi.org/10.3389/fenrg.2023.1324450
This article is part of the Research TopicLow-Carbon Oriented Market Mechanism and Reliability Improvement of Multi-energy SystemsView all 28 articles
With the growth of electricity consumers purchasing green energy and the development of digital energy trading platforms, the role of digitalized retail electricity markets in the low-carbon transition of electric energy systems is becoming increasingly crucial. In this circumstance, the research work on retail electricity markets needs to be further analyzed and expanded, which would facilitate the efficient decision-making of both market players and policymakers. First, this paper introduces the latest developments in the retail electricity market under low-carbon energy transition and analyzes the limitations of the existing research works. Second, from three aspects of power trading strategy, retail pricing methodology, and market risk management, it provides an overview of the existing operation and mechanism design strategies of the retail electricity market; then, it provides a systematic introduction to the evaluation system and monitoring methodology of electricity markets, which is not sufficient for the current digitalized retail electricity markets. Finally, the issues regarding operation evaluation and platform optimization of the current digitalized retail electricity market are summarized, and the research topics worth further investigations are recommended.
To realize the low-carbon transition of energy systems and the sustainable development of society, the installed capacity of renewable energy sources has achieved substantial growth worldwide (AlAshery et al., 2019; Erdogan et al., 2023; Lu et al., 2023). Under this context, it is necessary to have an efficient electricity market to achieve the optimal allocation of renewable energy resources and provide effective incentives for the production and consumption of green electricity (Yang et al., 2021). The retail electricity market serves as a bridge connecting electricity retailers and customers. With extensive access to various types of distributed energy resources, such as distributed photovoltaics and electric vehicles, the trading volume of the retail electricity market has been growing rapidly, and the needs of retail market players are becoming more and more diversified (Hampton et al., 2022). In this circumstance, the increased needs and risk levels have put forward higher requirements for retail electricity market construction and operation.
To face the above challenges, a digital energy trading platform can be utilized to carry out online electricity retail transactions, which can reduce the operation costs of trading entities and improve the transparency, fairness, and efficiency of the retail electricity market (Yang et al., 2021). In the major electricity market worldwide, digital retail trading platforms have the functions of retail package management, electronic contracting, information disclosure, etc., which can significantly reduce the communication and marketing costs between electricity consumers and retailers. In Japan, electricity consumers can log into the electricity package price comparison website and enter their basic information, such as the address zip code, household size, and characteristics of electricity consumption, to calculate the electricity cost of various packages (Fang and Wang, 2023). In Australia, a third-party website can help electricity consumers choose an electricity seller by considering multiple factors, including residential location, type of residence, and household size, as well as the percentage of green electricity in the package (Nelson et al., 2018). In Texas, United States, retail customers can also access the package comparison website, where the historical electricity consumption, acceptable tariff range, rate plan, renewable energy ratio, and other information about packages can be compared (Joskow, 2008). In the provincial electricity markets in China, online electricity retail trading has been established in Yunnan, Guangdong, Zhejiang, Shanxi, and other provinces and regions, and more provinces will join them in the future (Yang, 2021). In December 2022, the platform of Guangdong’s retail electricity market was launched, and as of 31 March 2023, a total of 36,187 electricity consumers in Guangdong had signed the retail electricity contracts with 158 electricity retailers through the digital trading platform.
In the context of the rapid growth of renewable energy sources and the development of digital trading platforms, it is necessary to conduct a comprehensive overview and analysis of the operation and evaluation methods of digitalized retail electricity markets. However, the current works (Möst and Dogan, 2010; Bublitz et al., 2019; Bao et al., 2021; Liu et al., 2022) on electricity markets mainly review the methodologies on the wholesale side, and Yang et al. (2017) and Hampton et al. (2022) focused only on the decision-making of the retailers and consumers, respectively, which did not involve the evaluation of the retail market or an analysis of the digital energy trading platform.
Therefore, this paper reviews and analyzes the operation and evaluation of digitalized retail electricity markets, and its contributions mainly include the following: 1) the research works on retail electricity markets, considering the dependent operation and evaluation approaches, are systematically reviewed; 2) the research gaps in the current research works are pointed out, which include the systematic evaluation of retail electricity markets and in-depth analysis of digital energy trading platforms; and 3) referring to other e-commerce platforms, such as Amazon and TikTok, suggestions on improving the operation and evaluation of digitalized retail electricity markets are put forward.
As the main decision-makers in retail electricity markets, electricity retailers and consumers have been comprehensively investigated in the existing works, which is summarized in Figure 1. In this section, the operation strategies for retailers and consumers mainly focus on power trading optimization, retail pricing scheme, and market risk management.
1) Power trading strategy optimization. The intermittent renewable energy resources increased the risk levels of electricity retailers in the wholesale electricity market, which needs to be managed by using proper retail pricing and contract design strategies in retail electricity contracts. In the existing works, the trading strategies of electricity retailers in multi-type power markets have been developed using advanced optimization techniques considering various flexible resources, such as battery storages, demand response (DR) programs, and virtual bids (Slouma et al., 2023; Wei et al., 2023). Ghazvini et al. (2015) and Khojasteh (2022) developed multi-objective optimization models for electricity retailers, where disaster recovery scheduling and retail packages have been investigated in detail, respectively. Jacquet et al. (2023) maximized the electricity retailer’s profit by designing a contract menu for customers, where a quadratic regularization model of customer responses is adopted to improve the robustness of the model, and Luo et al. (2017) proposed a personalized power retail solution recommendation system for residential users using collaborative filtering technology by considering users’ energy consumption patterns. Lu et al. (2022) analyzed the trading strategies for large retailers in China’s medium- and long-term electricity markets, while Charwand et al. (2014) focused on medium-term planning for electricity retailers and formulated a multi-objective framework to balance profit and sales prices to customers. Feuerriegel and Neumann (2014) optimized electricity procurement with DR for retailers, which can significantly reduce procurement costs and peak costs. Liu et al. (2020) explored data-driven decision-making strategies for electricity retailers using actor–critic and deep Q-learning approaches. Lu et al. (2021) introduced a comprehensive power cost optimization algorithm for electricity retailers, and its economic feasibility is verified in China’s electricity spot market.
2) Developing retail pricing schemes. The design of efficient retail pricing schemes is crucial for the retailer to encourage consumers to participate in the retail markets, and the green retail contracts can also facilitate the renewable energy consumption of the consumers. The Stackelberg game model was employed by Sekizaki et al. (2016) and Zugno et al. (2013) to obtain flexible pricing strategies for retailers, where the flexible interactions between market players and weather-related random parameters have been taken into account. Mahmoudi-Kohan et al. (2010) proposed an annual pricing framework for electricity retailers using the clustering technology based on consumers’ load profiles. Yang et al. (2018) optimized the time-of-use electricity price structure and level using data mining technology, given the number of price blocks using real power consumption data. Zhang et al. (2023a) proposed a two-stage customized retail price design based on personalized DR incentives, while Nojavan and Zare (2018) proposed a real-time pricing model by comparing time-sharing and fixed pricing. Qiu et al. (2020) introduced a three-level optimization model to capture the retailer’s pricing strategies’ implications and wholesale market price DR, and it can demonstrate the interactions and assess demand flexibility’s influence. Naseri et al. (2022) addressed the challenges faced by retailers, and the Seq2Seq algorithm and reinforcement learning were used for customer demand prediction and tariff mechanism design, respectively.
3) Market risk management. Different risk assessment methods have been investigated by the market players or system operators to handle the uncertainties on both wholesale and retail sides (Deng. et al., 2023; Xiao et al., 2023). To investigate the risk management for integrated energy service providers in the retail market, Guo et al. (2021) proposed an optimal pricing method based on value at risk (VaR), considering multi-energy DR. By using the CVaR and scenario generation techniques, the impacts of risk preferences and market conditions on the trading patterns of retailers were analyzed in detail (Sun et al., 2021). Karandikar et al. (2010) offered a method for strategic retailers to assess their benefits and quantify the risks in bilateral transactions. Zhang et al. (2022b) presented a credit evaluation and risk measurement mechanism for electricity retailers to manage risks in a dynamic market using the Bayesian best–worst method (BBWM) cloud model. Yu and Sun (2019) addressed risk assessment for independent electricity retailers in China and constructed a multi-level electricity market portfolio optimization model. To manage diverse contracts and sales prices, Charwand and Moshavash (2014) proposed an information gap decision theory-based method to evaluate retailer strategies, which could serve as a tool to assess risk levels and determine risk preferences.
The retail electricity market plays a crucial role in achieving multi-type policy targets, such as carbon neutrality, energy transition, and national unified electricity market construction in China. Defeuilley (2009) critiqued the impact of introducing competition on the supply side of the retail electricity market, and the flaws in the theoretical concepts from the Austrian School are addressed when the stability of short- and medium-term retail markets is evaluated. Mulder and Zomer (2016) revealed the effectiveness of renewable energy labeling systems and their limitations in Dutch retail electricity markets toward net zero. Peng and Tao (2018) addressed the existing issues and achievements by investigating an inter-regional power trading model in China, which focuses on quantifying the impact of cost, electricity price, and revenue on the behavior of electricity retailers. Guo et al. (2020) addressed risk assessment in China’s evolving retail electricity markets, and a hybrid multi-criteria decision-making method combining the Bayesian best–worst method and matter–element extension model was proposed. Yue et al. (2023) investigated the electricity price linkage mechanism between wholesale and retail sides in electricity markets for different applications with various users. Yuan et al. (2022) proposed the retail electricity market under the framework of constructing a national unified electricity market in China, where characteristics of the emerging entities are taken into account. Liang et al. (2022) proposed a carbon neutrality-driven architecture for the retail electricity trading platform to improve market efficiency and satisfy the diverse needs of different market entities. To ensure credible and efficient retail electricity trading of distributed energy resources, a blockchain-based online platform was developed by Chen et al. (2022). Zishan et al. (2022) examined the strategic choices of electricity retailers participating in the market, considering uncertain parameters in DR programs. Sun et al. (2023) proposed an incentive mechanism involving government, retailers, and residents to promote renewable energy consumption, and its effectiveness is verified by using realistic data in the winter. To reduce uncertainty and disclose privacy, the concepts of data revenues and costs were illustrated, and power-side and data-side trading frameworks were proposed for electricity retailers (Wang et al., 2022).
To ensure the efficient operation of wholesale and retail electricity markets, it is necessary to construct reliable monitoring and evaluation systems, which can avoid illegal bidding and other undesirable market behaviors that may decrease market efficiency (Li et al., 2004). Additionally, the environmental indicators suitable for retail electricity markets need to be developed to evaluate the energy transition status on the demand side. Xu et al. (2021) analyzed the establishment of a power market operation evaluation system and put forward the basic principle of power market operation monitoring to adapt to China’s power market reform. As a whole, the assessment of the electricity market needs to include a regulatory information collection system to provide data support, a market evaluation to generate early warnings, and a regulatory information dissemination system to show the evaluation results (Li et al., 2004). Moreover, the operation processes of the power market also need to be evaluated, and the main indicators of evaluation can be divided into four categories: economy, security, fairness, and environmental protection (Xu et al., 2021). To monitor and evaluate the electricity market, market structure, market balance, trading results, bidding strategy, and supplier status should be considered to ensure the healthy development of the market (Liu et al., 2004). The market power is a crucial content to be included to effectively assess and monitor the electricity market (Liu et al., 2023), where the evaluation system can be constructed according to the structure–conduct–performance model of industrial economics, followed by real-time evaluation and the corresponding decision-making by combining the fuzzy comprehensive evaluation method (Zhang et al., 2006). The residual supply index and the market parameter dependence index can be taken into account to access the market conditions (Dolmatova et al., 2021).
With renewable energy sources being connected to the power grid at a tremendous rate of growth, the quality and continuity of the power supply in the power system is being significantly challenged (Rietveld et al., 2015). To achieve this goal, intelligent devices for monitoring and controlling the status of power grids need to be deployed to maintain the safe and stable operation of power grids (Liu et al., 2013; Yang et al., 2023a). However, the current smart grid monitoring system often encounters problems such as poor portability of monitoring terminals, oversimplified monitoring systems, and low security of the information system (Fu et al., 2017). To judge the operation status and trend of the power grid, evaluation indexes can be constructed based on the stochastic matrix and qualitative trend analysis using operation data collected from the power grid (Yang et al., 2023b). It is also possible to utilize big data in combination with information fusion technology to provide the assessment and prediction of key equipment in a smart grid (Wang et al., 2011). The monitoring system can be used in conjunction with the smart grid to assess and evaluate the status of the power system and the information system, which help the staff make correct decisions and reduce losses (Fu et al., 2017). Additionally, if the operating conditions are relatively harsh, the monitoring system needs to take into account typhoon, ice, snow, lightning, and many other unfavorable factors to avoid the occurrence of serious accidents (Wang et al., 2012). In the process of monitoring and evaluating smart grids, different models can be established by considering different detection needs so as to obtain the best monitoring and evaluation effects (Falahati et al., 2013).
Based on the evaluation indexes proposed in the research works on market evaluation and monitoring, an evaluation framework with various evaluation indexes for electricity markets is shown in Table 1, which includes six primary evaluation indicators and secondary evaluation indicators. It is shown that most market structure and management indicators, such as the Herfindahl–Hirschman index (HHI), top-m, and timely rate of information disclosure indexes, are easy to be applied to retail electricity markets. However, some indicators on market behavior and development are not suitable due to the different frameworks, policies, and rules between retail and wholesale electricity markets. For instance, the centralized market clearing mechanism is widely used in wholesale electricity markets, while bilateral contracts are adopted by the players in retail electricity markets.
Existing research efforts have conducted comprehensive studies on decision optimization and mechanism design strategies for the retail electricity market. However, the current strategies did not consider the specific features and advantages of digital retail platforms in depth. It is also necessary to have systematic evaluation systems to provide references for the optimization and design of retail electricity markets, which also help improve the operation performance and risk management capability. Additionally, current monitoring and evaluation approaches for electricity market operation mainly focus on the wholesale side, which might not be suitable for retail electricity markets.
Therefore, to further improve the operational efficiency of retail electricity markets toward low-carbon transition, it is necessary to carry out further research in the following aspects:
1) It is necessary to conduct in-depth comparative analyses of the operational characteristics of wholesale and retail power markets and establish a quantitative and systematic evaluation index system for retail electricity markets so as to provide a basis for the optimal market mechanism design.
2) As the electricity retail platform has certain similarities with e-commerce platforms, such as TikTok and Amazon, it can refer to the operation and management strategies of existing e-commerce platforms and fully utilize those platform’s multi-dimensional data. This can help improve the operation and service levels of electricity retail trading platforms under low-carbon transition.
3) Since there are large numbers of players in retail electricity markets, which are diversified and large in scale, we can make better use of the artificial intelligence technology to conduct in-depth analyses of the market data, better understand the behavioral characteristics of various retail trading entities, improve the low-carbon operational efficiency, and enrich market service strategies.
XH: conceptualization, formal analysis, funding acquisition, methodology, project administration, resources, software, supervision, validation, writing–original draft, and writing–review and editing. WT: conceptualization, funding acquisition, project administration, supervision, writing–original draft, and writing–review and editing. YX: conceptualization, resources, software, validation, and writing–review and editing. WY: conceptualization, formal analysis, resources, supervision, and writing–review and editing. ZZ: formal analysis, investigation, resources, software, and writing–review and editing. YH: conceptualization, formal analysis, resources, software, and writing–review and editing. DX: conceptualization, formal analysis, investigation, project administration, software, supervision, writing–original draft, and writing–review and editing. YC: formal analysis, investigation, and writing–review and editing. RC: conceptualization, formal analysis, investigation, and writing–review and editing.
The author(s) declare that financial support was received for the research, authorship, and/or publication of this article. The authors would like to acknowledge the support from the Science and Technology Project of Guangdong Power Exchange Center Co., Ltd (Project No. GDKJXM20222599).
Authors XH, WT, YX, WY, ZZ, and YH were employed by Guangdong Power Exchange Center Co., Ltd.
The remaining authors declare that the research was conducted in the absence of any commercial or financial relationships that could be construed as a potential conflict of interest.
All claims expressed in this article are solely those of the authors and do not necessarily represent those of their affiliated organizations, or those of the publisher, the editors, and the reviewers. Any product that may be evaluated in this article, or claim that may be made by its manufacturer, is not guaranteed or endorsed by the publisher.
AlAshery, M. K., Xiao, D., and Qiao, W. (2019). Second-order stochastic dominance constraints for risk management of a wind power producer's optimal bidding strategy. IEEE Trans. Sustain. Energy 11 (3), 1404–1413. doi:10.1109/tste.2019.2927119
Bao, M., Ding, Y., Zhou, X., et al. (2021). Risk assessment and management of electricity markets: a review with suggestions. CSEE J. Power Energy Syst. 7 (6), 1322–1333. doi:10.17775/CSEEJPES.2020.04250
Bublitz, A., Keles, D., Zimmermann, F., Fraunholz, , and Fichtner, W. (2019). A survey on electricity market design: insights from theory and real-world implementations of capacity remuneration mechanisms. Energy Econ. 80, 1059–1078. doi:10.1016/j.eneco.2019.01.030
Charwand, M., Ahmadi, A., Heidari, A. R., and Esmaeel Nezhad, A. (2014). Benders decomposition and normal boundary intersection method for multiobjective decision making framework for an electricity retailer in energy markets. IEEE Syst. J. 9 (4), 1475–1484. doi:10.1109/JSYST.2014.2331322
Charwand, M., and Moshavash, Z. (2014). Midterm decision-making framework for an electricity retailer based on information gap decision theory. Int. J. Electr. Power and Energy Syst. 63, 185–195. doi:10.1016/j.ijepes.2014.05.054
Chen, A., Xu, Y., Wang, H., Xiao, C., Geng, J., Yu, S., et al. (2022). Design and implementation of electricity power retail business platform based on blockchain. J. Glob. Energy Interconnect. 5 (4), 331–338. doi:10.19705/j.cnki.issn2096-5125.2022.04.003
Chen, J., Sheng, G., Wu, J., et al. (2018). Application status and prospect of big data technology in smart grid. High. Volt. Appar. 54 (1), 35–43. doi:10.13296/j.1001-1609.hva.2018.01.006
Defeuilley, C. (2009). Retail competition in electricity markets. Energy Policy 37 (2), 377–386. doi:10.1016/j.enpol.2008.07.025
Deng, S., Xiao, D., Liang, Z., Chen, J., Huang, Y., and Chen, H. (2023). Information gap decision theory-based optimization of joint decision making for power producers participating in carbon and electricity markets. Energy Rep. 9, 74–81. doi:10.1016/j.egyr.2023.08.052
Dolmatova, M., Kozlovskiy, D., Khrustaleva, O., Sultanova, T., and Vasin, A. (2021). Market parameters dependent indices for competition evaluation in electricity market. Electr. Power Syst. Res. 190, 106762. doi:10.1016/j.epsr.2020.106762
Erdogan, S., Pata, U. K., and Solarin, S. A. (2023). Towards carbon-neutral world: the effect of renewable energy investments and technologies in G7 countries. Renew. Sustain. Energy Rev. 186, 113683. doi:10.1016/j.rser.2023.113683
Falahati, B., Fu, Y., and Mousavi, M. J. (2013). Reliability modeling and evaluation of power systems with smart monitoring. IEEE Trans. smart grid 4 (2), 1087–1095. doi:10.1109/tsg.2013.2240023
Fang, D., and Wang, P. (2023). Optimal real-time pricing and electricity package by retail electric providers based on social learning. Energy Econ. 117, 106442. doi:10.1016/j.eneco.2022.106442
Feuerriegel, S., and Neumann, D. (2014). Measuring the financial impact of demand response for electricity retailers. Energy Policy 65, 359–368. doi:10.1016/j.enpol.2013.10.012
Fu, W., Gong, L., Chen, H., and Pan, G. (2017). Research and design of smart grid monitoring control via terminal based on iOS system. IOP Conf. Ser. Earth Environ. Sci. IOP Publ. 69 (1), 012095. doi:10.1088/1755-1315/69/1/012095
Ghazvini, M. A. F., Soares, J., Horta, N., Neves, R., Castro, R., and Vale, Z. (2015). A multi-objective model for scheduling of short-term incentive-based demand response programs offered by electricity retailers. Appl. energy 151, 102–118. doi:10.1016/j.apenergy.2015.04.067
Guo, S., Zhang, W., and Gao, X. (2020). Business risk evaluation of electricity retail company in China using a hybrid MCDM method. Sustainability 12 (5), 2040. doi:10.3390/su12052040
Guo, Z., Xu, M., Li, R., et al. (2021). Optimal strategy of integrated energy retail market based on VaR theory. Electr. Power Constr. 42 (06), 9–16. doi:10.12204/j.issn.1000-7229.2021.06.002
Hampton, H., Foley, A., Del, R., Smyth, B., Laverty, D., and Caulfield, B. (2022). Customer engagement strategies in retail electricity markets: a comprehensive and comparative review. Energy Res. Soc. Sci. 90, 102611. doi:10.1016/j.erss.2022.102611
Jacquet, Q., Van, A. W., Alasseur, C., et al. (2023). Quadratic regularization of bilevel pricing problems and application to retail electricity markets. Eur. J. Operational Res. doi:10.1016/j.ejor.2023.05.006
Joskow, P. L. (2008). Lessons learned from electricity market liberalization. Energy J. 29 (2), 1–34. doi:10.5547/issn0195-6574-ej-vol29-nosi2-3
Karandikar, R. G., Khaparde, S. A., and Kulkarni, S. V. (2010). Strategic evaluation of bilateral contract for electricity retailer in restructured power market. Int. J. Electr. Power and Energy Syst. 32 (5), 457–463. doi:10.1016/j.ijepes.2009.09.018
Khojasteh, M. (2022). Multi-objective energy procurement strategy of electricity retail companies based on normalized normal constraint methodology. Int. J. Electr. Power and Energy Syst. 135, 107281. doi:10.1016/j.ijepes.2021.107281
Li, J., Mu, G., Zhang, J., Li, C., Yan, G., Zhang, H., et al. (2023). Dynamic economic evaluation of hundred megawatt-scale electrochemical energy storage for auxiliary peak shaving. Prot. Control Mod. Power Syst. 8 (3), 50–868. doi:10.1186/s41601-023-00324-8
Li, R., Liu, D., He, G., et al. (2004). Operating monitoring and evaluating system of electricity market. Automation Electr. Power Syst. 28 (14), 18–22. doi:10.2174/0929866043478455
Liang, Z., Xie, Y., Xiuzhen, H., et al. (2022). Research on the trading mode of electricity market retail platform driven by carbon neutrality. Electr. Meas. Instrum. 59 (08), 47–57. doi:10.19753/j.issn1001-1390.2022.08.006
Liu, D., Li, R., Chen, X., He, G., and Zhou, S. (2004). Surveillance indices and evaluating system of electricity market. Automation Electr. Power Syst. 28, 16–21. doi:10.2174/0929866043478455
Liu, J., Wang, J., and Cardinal, J. (2022). Evolution and reform of UK electricity market. Renew. Sustain. Energy Rev. 161, 112317. doi:10.1016/j.rser.2022.112317
Liu, Y., Liu, J., Liu, T., Guan, X., and Sun, Y. (2013). Security risks evaluation toolbox for smart grid devices. Comput. Commun. Rev. 43 (4), 479–480. doi:10.1145/2486001.2491693
Liu, Y., Tang, Y., Liu, M., Bie, F., He, Y., and Zhang, Y. (2023). Market power monitoring and mitigation mechanism of spot market under new power system. Int. Conf. Comput. Graph. Artif. Intell. Data Process. 12604, 437–450. doi:10.1117/12.2674578
Liu, Y., Zhang, D., and Gooi, H. B. (2020). Data-driven decision-making strategies for electricity retailers: a deep reinforcement learning approach. CSEE J. Power Energy Syst. 7 (2), 358–367. doi:10.17775/CSEEJPES.2019.02510
Lu, G., Wang, Z., Bhatti, U., and Fan, X. (2023). Recent progress in carbon dioxide capture technologies: a review. Clean Energy Sci. Technol. 1 (1), 32. doi:10.18686/cest.v1i1.32
Lu, T., Zhang, W., and Ding, X. (2021). Operation strategy of electricity retailers based on energy storage system to improve comprehensive profitability in China’s electricity spot market. Energies 14 (19), 6424. doi:10.3390/en14196424
Lu, T., Zhang, W., Wang, Y., Xie, H., and Ding, X. (2022). Medium-and long-term trading strategies for large electricity retailers in China’s electricity market. Energies 15 (9), 3342. doi:10.3390/en15093342
Luo, F., Ranzi, G., Wang, X., and Dong, Z. Y. (2017). Social information filtering-based electricity retail plan recommender system for smart grid end users. IEEE Trans. Smart Grid 10 (1), 95–104. doi:10.1109/TSG.2017.2732346
Maass, H., Çakmak, H. K., Bach, F., et al. (2015). Data processing of high-rate low-voltage distribution grid recordings for smart grid monitoring and analysis. EURASIP J. Adv. Signal Process., 1–21. doi:10.1186/s13634-015-0203-4
Mahmoudi-Kohan, N., Moghaddam, M. P., and Sheikh-El-Eslami, M. K. (2010). An annual framework for clustering-based pricing for an electricity retailer. Electr. Power Syst. Res. 80 (9), 1042–1048. doi:10.1016/j.epsr.2010.01.010
Möst, D., and Dogan, K. (2010). A survey of stochastic modelling approaches for liberalised electricity markets. Eur. J. Operational Res. 207 (2), 543–556. doi:10.1016/j.ejor.2009.11.007
Mulder, M., and Zomer, S. P. E. (2016). Contribution of green labels in electricity retail markets to fostering renewable energy. Energy Policy 99, 100–109. doi:10.1016/j.enpol.2016.09.040
Naseri, N., Talari, S., Ketter, W., and Collins, J. (2022). Dynamic retail market tariff design for an electricity aggregator using reinforcement learning. Electr. Power Syst. Res. 212, 108560. doi:10.1016/j.epsr.2022.108560
Nelson, T., McCracken-Hewson, E., Whish-Wilson, P., and Bashir, S. (2018). Price dispersion in Australian retail electricity markets. Energy Econ. 70, 158–169. doi:10.1016/j.eneco.2017.12.026
Nojavan, S., and Zare, K. (2018). Optimal energy pricing for consumers by electricity retailer. Int. J. Electr. Power and Energy Syst. 102, 401–412. doi:10.1016/j.ijepes.2018.05.013
Peng, X., and Tao, X. (2018). Cooperative game of electricity retailers in China's spot electricity market. Energy 145, 152–170. doi:10.1016/j.energy.2017.12.122
Qiu, D., Papadaskalopoulos, D., Ye, Y., and Strbac, G. (2020). Investigating the effects of demand flexibility on electricity retailers’ business through a tri-level optimisation model. IET Generation, Transm. Distribution 14 (9), 1739–1750. doi:10.1049/iet-gtd.2019.1433
Rietveld, G., Braun, J. P., Martin, R., Wright, P., Heins, W., Ell, N., et al. (2015). Measurement infrastructure to support the reliable operation of smart electrical grids. IEEE Trans. Instrum. Meas. 64 (6), 1–1363. doi:10.1109/TIM.2015.2406056
Sekizaki, S., Nishizaki, I., and Hayashida, T. (2016). Electricity retail market model with flexible price settings and elastic price-based demand responses by consumers in distribution network. Int. J. Electr. Power and Energy Syst. 81, 371–386. doi:10.1016/j.ijepes.2016.02.029
Slouma, S., Boulaares, W., Maity, S., Jemni, A., and Alimi, S. E. (2023). Optimal design of stand-alone photovoltaic system based on battery storage system: a case study of Borj Cedria in Tunisia. Clean Energy Sci. Technol. 1 (1), 28. doi:10.18686/cest.v1i1.28
Sun, B., Li, M., Wang, F., and Xie, J. (2023). An incentive mechanism to promote residential renewable energy consumption in China's electricity retail market: a two-level Stackelberg game approach. Energy 269, 126861. doi:10.1016/j.energy.2023.126861
Sun, B., Wang, F., Xie, J., and Sun, X. (2021). Electricity Retailer trading portfolio optimization considering risk assessment in Chinese electricity market. Electr. Power Syst. Res. 190, 106833. doi:10.1016/j.epsr.2020.106833
Wang, B., Guo, Q., and Yu, Y. (2022). Mechanism design for data sharing: an electricity retail perspective. Appl. Energy 314, 118871. doi:10.1016/j.apenergy.2022.118871
Wang, K., Cai, W., Deng, Y., et al. (2012). Application and management information platform for transmission line monitoring system. High. Volt. Eng. 38 (5), 1274–1280. doi:10.3969/j.issn.1003-6520.2012.05.035
Wei, C., Wang, Y., Shen, Z., Xiao, D., Bai, X., and Chen, H. (2023). AUQ–ADMM algorithm-based peer-to-peer trading strategy in large-scale interconnected microgrid systems considering carbon trading. IEEE Syst. J., 1–12. doi:10.1109/JSYST.2023.3290775
Xiao, D., Chen, H., Cai, W., Wei, C., and Zhao, Z. (2023). Integrated risk measurement and control for stochastic energy trading of a wind storage system in electricity markets. Prot. Control Mod. Power Syst. 8, 60. doi:10.1186/s41601-023-00329-3
Xu, M., Song, W., Tuo, J., Liu, D., and Li, T. (2021). Research on operation monitoring system of spot electricity market. IOP Conf. Ser. Earth Environ. Sci. 621, 012042. doi:10.1088/1755-1315/621/1/012042
Yang, J., Dong, Z. Y., Wen, F., Chen, Q., and Liang, B. (2021). Spot electricity market design for a power system characterized by high penetration of renewable energy generation. Energy Convers. Econ. 2 (2), 67–78. doi:10.1049/enc2.12031
Yang, J., Sun, W., Ma, M., et al. (2023b). Evaluation of operation state of power grid based on random matrix theory and qualitative trend analysis. Energies 16 (6), 2855. doi:10.3390/en16062855
Yang, J., Zhao, J., Luo, F., Wen, F., and Dong, Z. Y. (2017). Decision-making for electricity retailers: a brief survey. IEEE Trans. Smart Grid 9 (5), 4140–4153. doi:10.1109/TSG.2017.2651499
Yang, J., Zhao, J., Wen, F., and Dong, Z. (2018). A model of customizing electricity retail prices based on load profile clustering analysis. IEEE Trans. Smart Grid 10 (3), 3374–3386. doi:10.1109/TSG.2018.2825335
Yang, W., Zhang, E., Zhao, J., Zhao, Y., Tang, K., Cui, Y., et al. (2023a). Dawn of clean energy: enhanced heat transfer, radiative cooling, and firecracker-style controlled nuclear fusion power generation system. Clean Energy Sci. Technol. 1 (1), 61. doi:10.18686/cest.v1i1.61
Yang, X. (2021). “Design and application of electric power retail platform based on E-commerce mode in yunnan province,” in 2021 IEEE Sustainable Power and Energy Conference (iSPEC), Nanjing, China, December, 2021, 2432–2437. doi:10.1109/iSPEC53008.2021.9735681
Yao, L., Li, J., Li, T., et al. (2009). Design and application of novel power quality monitoring and evaluation system. Proc. Chin. Soc. Univ. Electr. Power Syst. its Automation. doi:10.1002/smr.397
Yu, X., and Sun, Y. (2019). Trading risk control model of electricity retailers in multi-level power market of China. Energy Sci. Eng. 7 (6), 2756–2767. doi:10.1002/ese3.457
Yuan, H., Dong, X., Liu, Q., et al. (2022). Framework design in national unified electricity market system. Power Syst. Technol. 46 (12), 4852–4862. doi:10.13335/j.1000-3673.pst.2022.0592
Yue, Z., Xie, W., Lin, Z., et al. (2023). Study on electricity price transmission mechanism from wholesale side to retail side in spot market. Power Demand Side Manag. 25 (3), 6–13. doi:10.3969/j.issn.1009-1831.2023.03.002
Zhang, C., Qiu, J., Yang, Y., and Zhao, J. (2023a). Residential customers-oriented customized electricity retail pricing design. Int. J. Electr. Power and Energy Syst. 146, 108766. doi:10.1016/j.ijepes.2022.108766
Zhang, J., Zhang, L., Cheng, Y., et al. (2006). Market power warning in electricity market based on SCP model and fuzzy algorithm. Automation Electr. Power Syst. doi:10.1016/S1005-8885(07)60093-4
Zhang, Y., Zhao, H., Li, B., and Qi, Z. (2023b). Research on credit rating and risk measurement of electricity retailers based on Bayesian Best Worst Method-Cloud Model and improved Credit Metrics model in China's power market. Energy 252, 124088. doi:10.1016/j.energy.2022.124088
Zishan, F., Akbari, E., Montoya, O. D., Giral-Ramírez, D. A., and Nivia-Vargas, A. M. (2022). Electricity retail market and accountability-based strategic bidding model with short-term energy storage considering the uncertainty of consumer demand response. Results Eng. 16, 100679. doi:10.1016/j.rineng.2022.100679
Keywords: retail electricity market, low carbon, operation strategy, evaluation method, risk management
Citation: Hu X, Tan W, Xie Y, Yang W, Zeng Z, Huang Y, Xiao D, Chi Y and Cheng R (2023) Operation and evaluation of digitalized retail electricity markets under low-carbon transition: recent advances and challenges. Front. Energy Res. 11:1324450. doi: 10.3389/fenrg.2023.1324450
Received: 19 October 2023; Accepted: 29 November 2023;
Published: 29 December 2023.
Edited by:
Minglei Bao, Zhejiang University, ChinaCopyright © 2023 Hu, Tan, Xie, Yang, Zeng, Huang, Xiao, Chi and Cheng. This is an open-access article distributed under the terms of the Creative Commons Attribution License (CC BY). The use, distribution or reproduction in other forums is permitted, provided the original author(s) and the copyright owner(s) are credited and that the original publication in this journal is cited, in accordance with accepted academic practice. No use, distribution or reproduction is permitted which does not comply with these terms.
*Correspondence: Dongliang Xiao, ZHhpYW9Ac2N1dC5lZHUuY24=
Disclaimer: All claims expressed in this article are solely those of the authors and do not necessarily represent those of their affiliated organizations, or those of the publisher, the editors and the reviewers. Any product that may be evaluated in this article or claim that may be made by its manufacturer is not guaranteed or endorsed by the publisher.
Research integrity at Frontiers
Learn more about the work of our research integrity team to safeguard the quality of each article we publish.