- Energy Development Research Institute China Southern Power Grid, Guangzhou, China
With the continuous development of the electricity market and the gradual expansion of the number and scale of participation in market transactions, the traditional energy trading model has limited the formation of a competitive pattern of multi-agents. In this paper, a new multi-microgrid energy storage alliance energy trading model based on Nash negotiation is proposed. This model takes energy storage, multi-microgrid, and superior power grid enterprises as the main participants and establishes an energy market trading model with “buy–sell” cooperation and competition coexisting within the alliance based on Nash negotiation theory. Through the interaction of electricity between different entities, energy conversion and complementary utilization are increased, achieving reasonable allocation of resources, enhancing the overall flexibility of the alliance, and promoting the local consumption of a high proportion of new energy. The simulation results of the example show that the energy trading model based on Nash negotiation can fully leverage the initiative of demand-side participation in scheduling and improve the utilization rate of energy storage systems while ensuring the payment benefits of all participating entities, which can provide technical support for energy complementarity among multiple entities and provide new technological paths for the sustainable development of energy sharing mechanisms.
1 Introduction
Under the policy of liberalizing electricity generation and consumption plans, diversified market entities such as energy storage, electric vehicles, and microgrids gradually participate in electricity trading. These participants form an alliance to meet their own load needs while transmitting energy to each other, achieving energy mutual assistance trading among multi-market entities within the alliance (Pan et al., 2023), gradually forming a multi-buyer and seller electricity market pattern. This market trading mechanism can achieve reasonable allocation of resources, enhance the overall flexibility of the alliance, and promote the local consumption of a high proportion of new energy (Fang et al., 2022), which can also accelerate carbon peaking, achieve carbon neutrality, and accelerate structural reforms on the energy supply side.
The sharing pattern of energy exchange among multi-market entities can enable users to use resources without ownership. Users can negotiate to form a shared market price, achieving the goal of supply and demand balance and maximizing the use of resources (Sivasankari and Narayanan, 2022). Vernay et al. (2023) provided a detailed explanation of the business model and transaction process of the aforementioned shared governance energy trading mechanism. Ko et al. (2022) pointed out that the shared market can significantly improve economy and resource utilization. Taking shared energy storage as an example, it saves 2.53%–13.82% in electricity costs compared to single user energy storage and increases utilization efficiency by 3.71%–38.98%. In addition, relevant explorations have been made on the pricing mechanisms of shared markets in the trading process, such as methods based on fixed prices (Yin and Yang, 2023), peak valley prices (Shen and Chen, 2022), profit or cost allocation (Siqin et al., 2022), and auction prices (Gabrielli and Willington, 2023), which have been widely studied and applied.
However, as a new business model, the energy exchange within the multi-market entity alliance also faces new difficulties in the comprehensive promotion process, such as the issue of fair transactions between multi-market entities. The interests of different market participants are showing a trend of diversification, and each market entity needs to consider its own and other market participants’ impact on itself when making decisions and how to handle conflicts of interest between different market entities. Nash negotiation (Montazeri et al., 2020) can balance the conflicts of interest between different participating parties and is used to solve the problem of profit distribution between multi-participating parties in the buy and sell process. At present, its most applications in the power system are concentrated in the operation between wind power and multi-hydrogen production stations (Zhao et al., 2023), wind solar hydrogen energy systems (Liu et al., 2023), and so on. There are many participants and types involved in energy trading within a multi-market entity alliance, and different participants have the right to choose and make decisions. In energy trading within the alliance, they will simultaneously act as buyers or sellers. Nash negotiation is one of the best technical means for scientifically analyzing and allocating the complex interest relationships mentioned previously.
Based on the aforementioned analysis, in order to accelerate the development of new energy and promote the local consumption of new energy, a multi-microgrid energy storage alliance energy trading model based on Nash negotiation is constructed. This model takes energy storage, multi-microgrid, and superior power grid enterprises within the multi-microgrid energy storage alliance as the participating entities and constructs a “buy–sell” cooperation and competition coexisting electricity market trading model based on the cooperation and competition relationship between each entity. Then, we establish an optimization decision making model to maximize the payment benefits of each participating entity within the alliance and utilize the improved moth to fire algorithm to solve the optimization decision model. Finally, the effectiveness and feasibility of the energy trading strategy of the multi-microgrid energy storage alliance based on Nash negotiation were demonstrated through simulation. The benefits of the non-cooperative mode (NCM) and cooperative mode (CM) based on Nash negotiation were compared, the initiative of demand-side participation in scheduling in the multi-microgrid was analyzed, and the utilization situation of shared energy storage under different trading modes was explored.
This article’s major innovation points are as follows:
1) An energy trading strategy for multi-microgrid energy storage alliance was proposed based on Nash negotiation
2) Based on the characteristics of the optimization model in this article, the moth to fire algorithm is improved to solve the problem
3) The profitability, demand-side participation and scheduling initiative, and shared energy storage utilization of multi-microgrid energy storage were compared under the NCM and CM based on Nash negotiation
2 Multi-microgrid energy storage alliance energy trading architecture
2.1 Non-cooperative mode
There is a lack of market response and self-regulation ability in China’s existing energy trading. Market entities such as microgrids, new energy stations, energy storage, and controllable loads do not have decision-making and discourse power and still follow the pricing mechanism of power grid enterprise buying, selling, and monopolizing. New energy power generation has been connected to the grid as planned, resulting in large-scale wind and solar abandonment and ineffective utilization of resources. In this energy trading mode, the microgrid is only provided with electricity and natural gas by the superior energy grid and is forced to accept the transaction pricing of the superior power grid. Energy storage belongs to the superior power grid and is used to meet the inertia support and frequency regulation needs of the superior power grid. At the same time, only the superior power grid charges the energy storage to meet the operational needs of the energy storage during the scheduling cycle. Renewable energy sources such as wind power and photovoltaic are managed by power grid enterprises through government electricity prices and tax subsidies, which are planned for grid access. Each participating entity has no other choice except to conduct electricity trading with the superior power grid, resulting in prominent issues such as information asymmetry and opacity and the dominance of power grid enterprises, which is not conducive to the sustainable development of the power economy.
2.2 Cooperative mode based on Nash negotiation
The transaction mode of the multi-microgrid energy storage alliance under the cooperation mode is as follows.
Different from the energy trading under NCM, in Figure 1, all participating entities participate in market-oriented cooperation and competition through reasonable price incentives, promoting the sustainable development of electricity economy. Microgrid, power grid enterprises, and energy storage system form an alliance. The information among participants in the alliance is completely open and transparent, and all participating entities have equal status in the power trading process. Under the premise of ensuring the balance of power supply and demand and safe and stable operation of all participating entities, a true “buy–sell” cooperative and competitive power market trading model was achieved. This model is based on Nash negotiation theory and determines the trading volume and price between each participating entity and other entities through negotiation. Its multi-party governance and sharing electricity trading model can promote healthy competition among participating entities within the alliance and attract more participating entities to join. The specific energy exchange and trading methods are as follows:
1) When the electricity supply of microgrid i is less than the demand, the transaction price can be determined by the superior power grid, energy storage, or other microgrids competing with each other based on the demand of microgrid i.
2) When there is a surplus of electricity in microgrid i, it can be used to compensate for the electricity demand of other microgrids, sold to superior power grids to meet frequency regulation needs, or sold to energy storage to meet operational needs during the scheduling cycle. The price of electricity sold by microgrid i is determined through competition with other participating entities in the alliance.
3) Energy storage is used not only to meet the inertia support and frequency regulation needs of the superior power grid but also to compensate for the electricity demand of the microgrid. The price for selling energy storage is determined through competition with other participating entities in the alliance.
4) When the energy storage needs to be charged to maintain normal operation during the scheduling cycle, the superior power grid and microgrid group can cooperate and compete to determine the transaction price and quantity of electricity charged to the energy storage based on the required charging quantity.
5) For superior power grid enterprises, their inertia support and frequency regulation needs can be met by energy storage or microgrid groups. Based on the electricity required for auxiliary services, energy storage or microgrid groups compete to determine the trading partner, electricity quantity, and price.
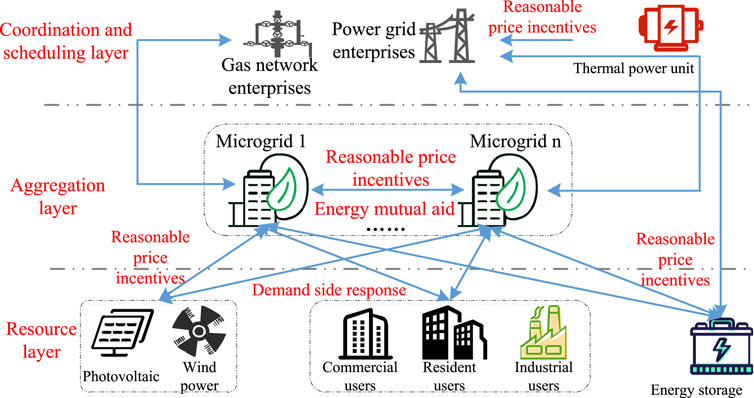
FIGURE 1. Energy trading mechanisms of multi-microgrid energy storage alliance under the cooperative mode.
3 Energy trading mechanisms for multi-microgrid energy storage alliance based on Nash negotiation
3.1 Energy trading mode
Nash negotiation, also known as the bargaining model, is one of the earliest studied problems in game theory and an important theoretical basis for cooperative games (Churkin et al., 2021). The purpose of bargaining is to hope for greater benefits for oneself, but due to conflicts of interest among the participating parties, the degree of benefits is limited, and beyond the boundaries, the negotiation will break down. For the participating entities in the shared alliance in this article, applying Nash negotiation theory can yield
where
This article is based on the Nash negotiation cooperation model, establishing an alliance consisting of four participating entities: superior power grid enterprises, microgrid A, microgrid B, and energy storage. To maintain generality, this article selects general comprehensive energy system architecture, including equipment such as electricity, heat, cooling, natural gas, and energy storage, as the structural framework of the microgrid. The specific structure is shown in the work of Li et al. (2021), including a gas turbine (GT), waste heat recovery (WHR), gas boiler (GB), and electric cooler (EC). The controllable equipment is the elastic load, which is divided into the reducible electrical load (RLe) and transferable cold/heat load (TLc/TLh) according to the type of electricity used. The optimization process of negotiating the electricity trading volume and corresponding trading prices between each participating entity and other entities is shown in Figure 2.
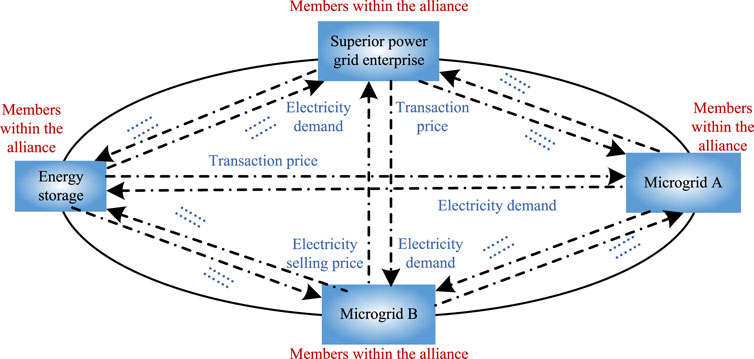
FIGURE 2. Optimization process of energy mutual assistance among participating entities in Nash negotiations.
Under the CM based on Nash negotiation, each participating entity negotiates the electricity trading volume and corresponding trading price with other entities. Driven by the electricity trading volume and trading price with other entities, the participating entities have the following optimization process:
1) Superior power grid enterprises optimize internal thermal power units
2) Microgrids optimize the scheduling plan for controllable resources
3) The energy storage system optimizes the hourly charging and discharging capacities
The transaction prices decided by each entity will affect the electricity trading volume between other entities and that entity. The electricity trading volume will further affect the scheduling plan of controllable resources within each participating entity and also the entity’s decision making on trading prices.
In this “buy–sell” cooperative and competitive electricity market trading model, energy conversion and complementary utilization can be increased through the interaction of electricity between different entities, thereby improving the overall revenue of the shared energy storage alliance. When the overall revenue of the alliance increases, the revenue of each participating entity also increases accordingly. During the optimization process, the information of each participating entity is transmitted to each other, ultimately achieving the Pareto–Nash equilibrium.
3.2 Energy trading process
The energy trading strategy of the multi-microgrid energy storage alliance based on Nash negotiation mainly aimed at the day-ahead scale electricity trading in the spot market. To ensure complete transparency of information among participants in the alliance and equal status of all participants in the electricity trading process, the trading process is shown in Figure 3. Taking a day-ahead scale scheduling process as an example, it is described as follows:
1) Data packets are generated from the capacity of thermal power units in the superior power grid, rated capacity and power of energy storage, and various load demands in the microgrid and broadcasted to the participating entities of the multi-microgrid energy storage alliance.
2) After receiving the demand for natural gas, the superior gas network formulates the unit natural gas price and forms a data packet again, which is broadcasted to the multi-microgrid energy storage alliance.
3) After receiving two broadcasts of information, the participating entities in the multi-microgrid energy storage alliance obtain the optimal transaction plan based on the Nash negotiation model of each participating entity.
4) Transaction prices and quantities are negotiated into data packets broadcasted to the entire network to reach consensus.
5) Permits are issued for transactions that have already reached a consensus for confirmation. The transaction is declared invalid without reaching a consensus, and all participating entities synchronously update their status and requirements before proceeding to step one again.
4 Energy trading model for multi-participants based on Nash negotiation
4.1 Superior power grid enterprises
4.1.1 Economic benefits
The benefits of the superior power grid when the energy trading mode is the NCM and CM are expressed as follows:
where
where
where
In the NCM, the superior power grid only has its own thermal power units and energy storage coordinated to meet the frequency regulation needs. Therefore, Eq. 4 can be rewritten as
4.1.2 Constraint condition
The superior power grid enterprise also has the following constraints.
1) Power balance constraint:
The superior power grid enterprise sells electricity to the microgrid and energy storage to ensure the balance of power supply and demand in the microgrid and the demand for energy storage operation.
2) Frequency modulation capacity demand constraint:
where
3) Thermal power unit operational constraints:
The thermal power unit operational constraints including output constraint and climbing constraint are shown in Eqs 8, 9, respectively.
where
where
4.2 Energy storage
4.2.1 Economic benefits
The benefits of energy storage when the energy trading mode is the NCM and CM are expressed as follows:
Equation 10 shows that the income from the energy storage is the income from selling electricity to other market entities minus the cost of purchasing electricity from other market entities to charge energy storage while maintaining normal operation of energy storage. According to the analysis in Section 2.1, in the NCM, energy storage’s energy is only sold to the superior power grid enterprise to meet the frequency regulation needs of the power grid and can only accept power supply from the superior power grid. In this case,
Based on the energy storage economic benefits shown in Eq. 10, it can be inferred that the total power of energy storage charging at time t is
4.2.2 Constraint condition
The energy storage also needs to meet the following constraints during operation.
1) Energy storage charging/discharging power constraint:
where
2) Energy storage charging/discharging state constraint:
In order to avoid simultaneous charging and discharging of energy storage at the same time period, there is the following constraint:
3) Energy storage charging/discharging power constraint during the total scheduling cycle:
At the same time, in order to ensure the sustainable development and healthy operation of the energy storage, the sum of the charging and discharging powers of the energy storage during the total scheduling cycle is set to 0.
4) Energy storage capacity constraint:
To avoid deep charging and discharging of energy storage, in order to delay the usage time of energy storage, the use of energy storage is generally forcibly stopped when the energy storage capacity is low or high. In this case, there is a capacity constraint for energy storage, as shown in Eq. 14. The capacity of energy storage at current time t is related to the charging and discharging powers at that time and the capacity at the previous time. The specific calculations are shown in Eqs 15, 16.
where
where
where
4.3 Microgrid
4.3.1 Economic benefits
The benefits of the microgrid in the energy trading mode of the NCM and CM are expressed as follows:
where
where
where
In the NCM, microgrids only purchase energy from superior power grids and gas grids, and the cost of energy purchase is expressed as follows:
where
In the CM, microgrids can accept energy supply from any other participating entity within the alliance; at the same time, when there is an energy surplus within the microgrid, energy can also be mutually beneficial to meet the needs of other market entities. Based on the aforementioned analysis, the energy purchase cost is expressed as follows:
where i is the collection of market entities that have energy interactions with the analyzed microgrid, including other microgrids, superior power grids, and energy storage.
The CCHP units in the microgrid have the following conversion relationships:
where
4.3.2 Constraint condition
The microgrid also needs to meet the following constraints during operation.
1) Energy balance constraint:
The real-time power balance including electrical energy is shown in Eq. 23. For cold and hot energy, due to its large inertia and storage capacity, only the cooling and heating needs can be guaranteed, as shown in Eq. 24.
where
where
2) CCHP unit operation constraints:
The operational constraints of CCHP units in the microgrid are simplified as upper and lower bound constraints for energy conversion:
where
3) Controllable load call constraints:
The call of the controllable load should be within its maximum load loss ratio, as shown in Eq. 26. The transferable cold and hot loads should ensure that the total amount of transferred power remains unchanged during the total scheduling cycle and should also include upper and lower limit constraints on the transferred power and total transfer amount constraint. Transferable hot load is taken as an example to illustrate:
where
where
5 Solution of the energy trading model
The energy trading model of the multi-microgrid energy storage alliance has the characteristics of non-linearity and complex constraints. To solve the aforementioned model, a novel swarm intelligence algorithm, the improved moth–flame optimization (IMFO) algorithm, is proposed. The solution flowchart is shown in Figure 4. The improvement strategies are as follows:
1) Average entropy initialization is introduced to ensure global diversity.
2) Levy flight is introduced to avoid “precocity” in the algorithm.
3) Variable scale chaotic strategy is adopted to increase local search performance.
4) The scaling factor concept is integrated in differential thinking into the MFO algorithm to enhance the algorithm’s ability to solve large-scale problems.
The specific strategy collaboration can be found in the work of Wu et al. (2023).
In order to overcome the randomness of the metaheuristic algorithm during the solving process, the results of the examples in this paper are all the optimal values of the improved moth to flame algorithm after running independently for 30 times. For complex constraints such as energy storage, elastic load operation constraints, and power balance equation constraints, dynamic relaxation constraint processing (He et al., 2021) is used to ensure the feasibility of the solution.
6 Example analyses
6.1 Situation description
Based on the alliance structure in Figure 1, the participating individuals of the multi-microgrid energy storage alliance in the calculation example are determined including superior power grid enterprise, energy storage, microgrid A, and microgrid B. The efficiency values of CCHP units in the microgrid mainly refer to the work of Roy and Das (2023), and some units such as waste heat recovery devices have been modified according to the actual situation. The call cost coefficients of each CCHP unit refer to the work of Ma et al. (2023), and the specific settings are shown in Table 1. Based on the actual situation and the work of Gough et al. (2023), the virtual power plant is extended to a virtual energy plant, and the schedulable loads and parameters of various virtual energy plants contained within two microgrids are shown in Table 1.
The maximum proportion of the load that can be reducible during the operation is the proportion of the total load that can be reducible in the microgrid. The daily forecast of various loads and renewable energy output in each microgrid is shown in Figure 5.
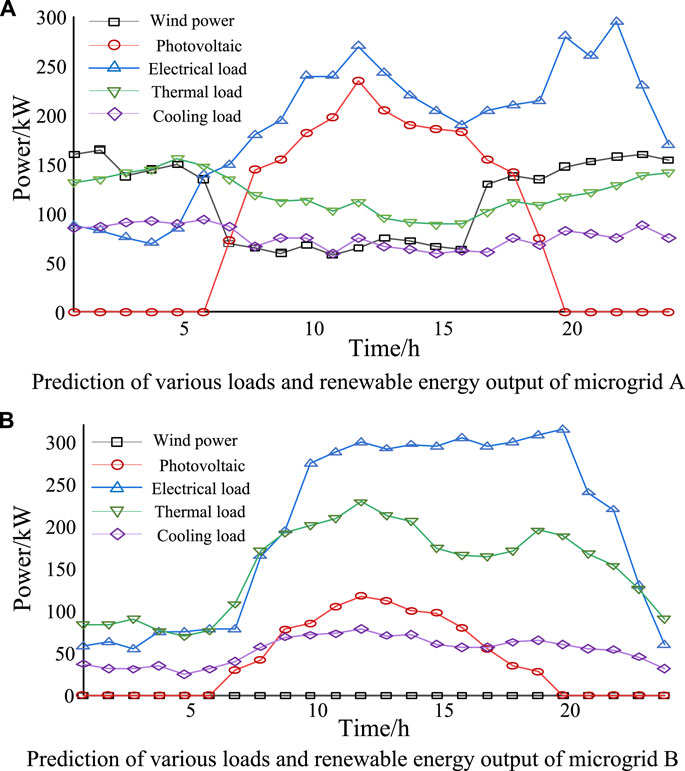
FIGURE 5. Prediction of various loads and renewable energy outputs of each microgrid. (A) Prediction of various loads and renewable energy output of microgrid A, and (B) Prediction of various loads and renewable energy output of microgrid B.
6.2 Analysis of energy trading results in the non-cooperative mode
In the NCM, both electricity and gas prices are set by the superior power grid and gas grid, as shown in Figure 6, and other participating entities are forced to accept them. The operating cost coefficients are
The scheduling plans for each CCHP unit in microgrids A and B are shown in Figures 7A, B; the controllable load scheduling plan is shown in Figures 7C, D, and the scheduling plans for the superior power grid and energy storage are shown in Figure 7E.
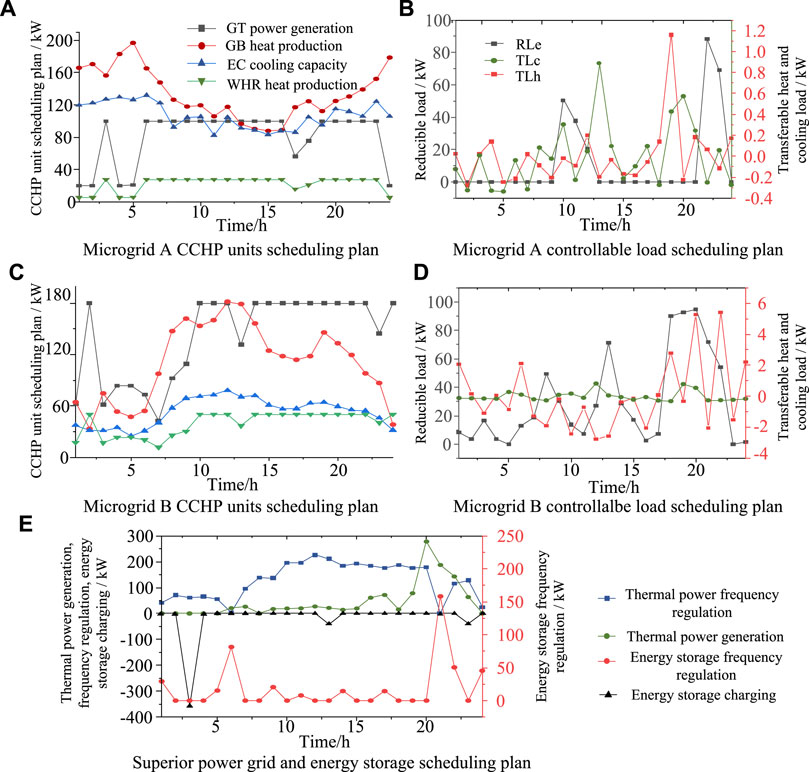
FIGURE 7. Microgrid, superior power grid, and energy storage scheduling plan in the non-cooperative mode. (A) Microgrid A CCHP units scheduling plan, (B) Microgrid A controllable load scheduling plan, (C) Microgrid B CCHP units scheduling plan, (D) Microgrid B controllable load scheduling plan, and (E) Superior power grid and energy storage scheduling plan.
Figures 7A, C show that microgrids A and B can achieve energy supply and demand balance within their respective regions by dispatching CCHP units. In order to reduce the cost of purchasing electricity from microgrids, the main power supply equipment, the gas turbine, reaches its rated power at multiple times, and the power curve is driven by real-time electricity prices. Figures 7B, D show that in the NCM, except for the participation of the RLe-type load in scheduling during certain periods, TLh/c did not effectively participate in scheduling, and the initiative of the demand side was not fully utilized. Figure 7E shows that the frequency regulation demand of the superior power grid is mainly led by the thermal power unit, and the energy storage cooperates to complete the total frequency regulation demand. From the scheduling curve of the energy storage, it can be seen that energy storage is not effectively utilized in the NCM.
6.3 Analysis of energy trading results in the cooperative mode
In the CM, all participating entities in the alliance determine the transaction price and electricity consumption through negotiation. The trading price is set with different fluctuation ranges according to the peak/valley of electricity consumption (Mei et al., 2023), as shown in Table 2.
Starting from the transaction methods and scheduling plans of participating entities in the alliance in the NCM and CM, this section compares the revenue situation of the two modes, analyzes the initiative of demand side participation, and explores the utilization of energy storage under different transaction modes.
6.3.1 Comparison of revenue
The comparison of the costs and benefits of the microgrid, superior power grid, and energy storage between the NCM and CM is shown in Table 3.
Table 3 shows that in the CM, the cost of the microgrid group is reduced by 31.06% compared to the NCM and the cost of the superior power grid is reduced by 10.11%. This is because in the NCM, the microgrid only receives power from the superior power grid and has no other options except for electricity trading with the superior power grid. This makes it difficult for microgrids to effectively consume surplus electricity while meeting their own energy needs, resulting in resource waste. The abandoned electricity of microgrids in the NCM is shown in Figure 8. The CM grants participants the right to choose and make decisions, and the microgrid sells surplus electricity to any other entity, thereby reducing costs.
Figure 9 shows the average transaction price of electricity sold by entities within the alliance under the CM. The transaction prices during each period are controlled by the alliance and are within the pre-set peak/valley fluctuation range, which is energy storage > microgrid B > superior grid > microgrid A. This is because microgrid A contains a large amount of wind and solar power generation, and renewable energy is not included in the power generation cost in the text, greatly reducing the purchase cost of microgrid A and making the transaction price of electricity sold through microgrid A the lowest. The energy storage does not contain energy generation devices and can only maintain its own charging and discharging balance by purchasing electricity, resulting in the highest transaction price for selling electricity through energy storage. Microgrid B and the superior grid each contain a small number of photovoltaic and thermal power units with intermittent characteristics, and the power generation cost of thermal power units is lower than the cost of purchasing electricity from other entities. Therefore, the transaction price of electricity sold through microgrid B and the superior grid is between shared energy storage and microgrid A.
6.3.2 Comparison of demand-side response levels
Under the CM, the scheduling plan for controllable loads in microgrids A and B is shown in Figure 10.
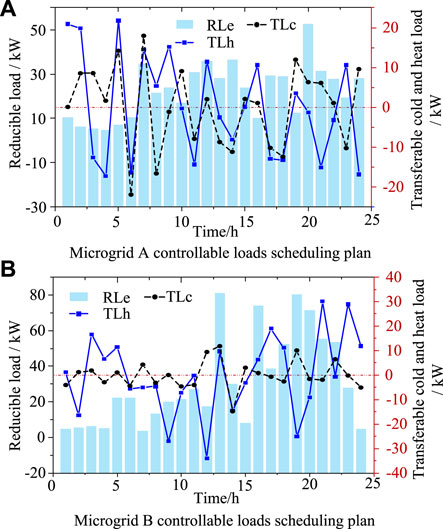
FIGURE 10. Microgird controllable load scheduling plan in the cooperative mode. (A) Microgrid A controllable loads scheduling plan, and (B) Microgrid B controllable loads scheduling plan.
Comparing Figure 10 with Figures 7B, D, it can be seen that under the influence of the “buy–sell” competitive market trading model, the CM can promote the demand side to fully leverage its initiative. Driven by transaction prices, the demand side in the microgrid participates in scheduling as much as possible during peak electricity consumption periods. The microgrid sells surplus electricity to other participating entities in the alliance to obtain more profits, which is consistent with the analysis of the results shown in Table 3.
6.3.3 Comparison of energy storage utilization
Under the CM, the charging/discharging plan for shared energy storage within the alliance is shown in Figure 11. Comparing Figure 11 with Figure 7E, it can be seen that energy storage belongs to the superior power grid under the NCM, and there is only electricity exchange with the superior power grid, which makes the energy storage system not effectively utilized. In the CM, energy storage transactions are conducted among multiple parties, motivated by price incentives, to purchase electricity from market entities with lower transaction prices while meeting the electricity demand of other participating entities, in order to maintain their own charging and discharging balance. Under this trading mode, the utilization rate of the energy storage system has increased, and the profits have also correspondingly increased, which is consistent with the results of the increased benefits of shared energy storage in Table 3.
7 Conclusion
Against the backdrop of accelerating the transformation of energy supply side structure in China, a multi-microgrid energy storage alliance energy trading strategy based on Nash negotiation is proposed for the electricity market mechanism and trading mode of multi-market entities in the micro grid with a high proportion of renewable energy access, with a “buy–sell” electricity market pattern. Through simulation, the following conclusions can be drawn:
1) A “buy–sell” cooperative and competitive electricity market trading model was constructed based on Nash negotiations, promoting healthy competition among participating entities within the alliance, attracting more participants to join, and promoting sustainable development of the electricity economy
2) The cooperation model based on Nash negotiation can significantly reduce/improve the costs/benefits of participating entities in energy trading
3) The cooperation model based on Nash negotiation can fully leverage the initiative of demand-side participation in scheduling, improve the utilization rate of energy storage systems, and promote the sustainable development of effective energy utilization and sharing mechanisms
In subsequent research, the regulatory needs of various market entities at different time scales will be considered, and further research will be conducted on the configuration and operation strategies of shared energy storage systems under stable support needs at multi-time scales.
Data availability statement
The raw data supporting the conclusion of this article will be made available by the authors, without undue reservation.
Author contributions
HQ: methodology, visualization, and writing–review and editing. LW: investigation, validation, and writing–review and editing. SW: investigation and writing–original draft. JZ: validation, visualization, and writing–original draft.
Funding
The authors declare that no financial support was received for the research, authorship, and/or publication of this article.
Conflict of interest
The authors declare that the research was conducted in the absence of any commercial or financial relationships that could be construed as a potential conflict of interest.
Publisher’s note
All claims expressed in this article are solely those of the authors and do not necessarily represent those of their affiliated organizations, or those of the publisher, the editors, and the reviewers. Any product that may be evaluated in this article, or claim that may be made by its manufacturer, is not guaranteed or endorsed by the publisher.
References
Churkin, A., Bialek, J., Pozo, D., Sauma, E., and Korgin, N. (2021). Review of Cooperative Game Theory applications in power system expansion planning. Renew. Sustain. Energy Rev. 145, 111056. doi:10.1016/j.rser.2021.111056
Fang, G., Yang, K., Tian, L., and Ma, Y. (2022). Can environmental tax promote renewable energy consumption? — An empirical study from the typical countries along the Belt and Road. Energy 260, 125193. doi:10.1016/j.energy.2022.125193
Gabrielli, M. F., and Willington, M. (2023). Estimating damages from bidding rings in first-price auctions. Econ. Model. 126, 106404. doi:10.1016/j.econmod.2023.106404
Gough, M., Santos, S. F., Javadi, M. S., Home-Ortiz, J. M., Castro, R., and Catalão, J. P. S. (2023). Bi-level stochastic energy trading model for technical virtual power plants considering various renewable energy sources, energy storage systems and electric vehicles. J. Energy Storage 68, 107742. doi:10.1016/j.est.2023.107742
He, Z., Wang, C., Wang, Y., Wei, B., Zhou, J., Zhang, H., et al. (2021). Dynamic programming with successive approximation and relaxation strategy for long-term joint power generation scheduling of large-scale hydropower station group. Energy 222, 119960. doi:10.1016/j.energy.2021.119960
Ko, G., Amankwah-Amoah, J., Appiah, G., and Larimo, J. (2022). Non-market strategies and building digital trust in sharing economy platforms. J. Int. Manag. 28, 100909. doi:10.1016/j.intman.2021.100909
Li, X., Wang, W., and Wang, H. (2021). Hybrid time-scale energy optimal scheduling strategy for integrated energy system with bilateral interaction with supply and demand. Appl. Energy 285, 116458. doi:10.1016/j.apenergy.2021.116458
Liu, L., Zhai, R., and Hu, Y. (2023). Performance evaluation of wind-solar-hydrogen system for renewable energy generation and green hydrogen generation and storage: energy, exergy, economic, and enviroeconomic. Energy 276, 127386. doi:10.1016/j.energy.2023.127386
Ma, X., Liu, S., Zhao, S., Zong, Q., and Liu, H. (2023). The optimal configuration of distributed generators for CCHP micro-grid based on double-layer operation strategy and improved NSGA-III algorithm. Energy Build. 293, 113182. doi:10.1016/j.enbuild.2023.113182
Mei, S., Tan, Q., Liu, Y., Trivedi, A., and Srinivasan, D. (2023). Optimal bidding strategy for virtual power plant participating in combined electricity and ancillary services market considering dynamic demand response price and integrated consumption satisfaction. Energy 284, 128592. doi:10.1016/j.energy.2023.128592
Montazeri, M., Kebriaei, H., and Araabi, B. N. (2020). Learning pareto optimal solution of a multi-attribute bilateral negotiation using deep reinforcement. Electron. Commer. Res. Appl. 43, 100987. doi:10.1016/j.elerap.2020.100987
Pan, J., Liu, X., and Huang, J. (2023). Multi-level games optimal scheduling strategy of multiple virtual power plants considering carbon emission flow and carbon trade. Electr. Power Syst. Res. 223, 109669. doi:10.1016/j.epsr.2023.109669
Roy, N. B., and Das, D. (2023). Probabilistic optimal power allocation of dispatchable DGs and energy storage units in a reconfigurable grid-connected CCHP microgrid considering demand response. J. Energy Storage 72, 108207. doi:10.1016/j.est.2023.108207
Shen, M., and Chen, J. (2022). Optimization of peak-valley pricing policy based on a residential electricity demand model. J. Clean. Prod. 380, 134761. doi:10.1016/j.jclepro.2022.134761
Siqin, Z., Niu, D., Li, M., Gao, T., Lu, Y., and Xu, X. (2022). Distributionally robust dispatching of multi-community integrated energy system considering energy sharing and profit allocation. Appl. Energy 321, 119202. doi:10.1016/j.apenergy.2022.119202
Sivasankari, G. S., and Narayanan, K. (2022). Demand response approach in the presence of plug-in hybrid electric vehicle for profit maximization of utility. Energy Convers. Manag. X 15, 100263. doi:10.1016/j.ecmx.2022.100263
Vernay, A.-L., Sebi, C., and Arroyo, F. (2023). Energy community business models and their impact on the energy transition: lessons learnt from France. Energy Policy 175, 113473. doi:10.1016/j.enpol.2023.113473
Wu, J., Zhao, P., Li, L., Shi, F., and Li, B. (2023). Multi-microgrids distributed peer-to-peer energy trading in distribution system considering uncertainty risk. Int. J. Electr. Power & Energy Syst. 152, 109234. doi:10.1016/j.ijepes.2023.109234
Yin, L., and Yang, S. (2023). Oil price returns and firm’s fixed investment: a production pattern. Energy Econ. 125, 106896. doi:10.1016/j.eneco.2023.106896
Keywords: Nash negotiation, cooperative and competitive, multi-buyer and seller, multi-energy complementary, energy trading
Citation: Qiao H, Wu L, Wen S and Zhang J (2023) Energy trading model for multi-microgrid energy storage alliance based on Nash negotiation. Front. Energy Res. 11:1306317. doi: 10.3389/fenrg.2023.1306317
Received: 03 October 2023; Accepted: 23 October 2023;
Published: 06 November 2023.
Edited by:
Meiheriayi Mutailipu, Xinjiang University, ChinaReviewed by:
Qingkun Tan, Consultant, Beijing, ChinaZhongfu Tan, North China Electric Power University, China
Xinyue Dong, North China Electric Power University, China
Copyright © 2023 Qiao, Wu, Wen and Zhang. This is an open-access article distributed under the terms of the Creative Commons Attribution License (CC BY). The use, distribution or reproduction in other forums is permitted, provided the original author(s) and the copyright owner(s) are credited and that the original publication in this journal is cited, in accordance with accepted academic practice. No use, distribution or reproduction is permitted which does not comply with these terms.
*Correspondence: Huiting Qiao, qiaoht@csg.cn