- 1College of Resource and Environment, Hunan University of Technological and Business, Changsha, China
- 2Key Laboratory of Hunan Province for Carbon Neutrality and Intelligent Energy, Changsha, China
- 3College of Economics and Trade, Hunan University of Technological and Business, Changsha, China
Introduction: The digital economy plays a crucial role in achieving synergistic reduction in air pollutants and carbon emissions.
Methods: A super-efficiency slack-based model with undesirable outputs was applied to systematically calculate the efficiency of synergistic air pollutants and carbon emissions governance (ESACG). This study used the difference-in-differences (DID), propensity score matching-DID, quantile DID methods and generalized random forest model to empirically test the impact and its heterogeneity of the digital economy on the ESACG, with the establishment of the National Big Data Comprehensive Pilot Zone as a quasi-natural experiment.
Results: 1) The digital economy significantly improved the ESACG by optimizing industrial structure in source management, improving energy utilization efficiency in process control, and promoting green technological innovation in end blocking. The digital literacy of talent and digital financial support strengthened its enhancing effect, but the digital infrastructure was insignificant. 2) The digital economy significantly enhanced the ESACG in the cross-regional and regional demonstration zones but inhibited it in the pioneering zones. Its impact on the ESACG in big data infrastructure-integrated development zones was insignificant. 3) Between the 25th and 90th quantiles, there was an asymmetric inverted U-shaped influence of the digital economy on the ESACG, with no discernible impact at the 10th quantile. In cities with better economic development and technological innovation, the contribution of the digital economy to the ESACG was more significant.
Discussion: It is necessary to continuously advance the construction of existing pilot zones, steadily expand their coverage, and differentiate between harnessing the experiences of reducing pollution and carbon emissions to formulate strategies for synergistic regional governance.
1 Introduction
In the face of extreme changes in the global climate and increasing environmental pollution, the synergistic control of air pollutants (AP) and carbon emissions (CE) has become a global concern. Unlike the development paths of most developed countries, where they pollute first and treat later, developing countries now face the double threat of AP and CE (Yi et al., 2022). The scale and imbalance of China’s development have led to a longer and more intense overlap between these two problems (Di et al., 2023), making it particularly urgent for China to implement measures for the synergistic governance of AP and CE. The homogeneity of atmospheric pollutants and greenhouse gas emissions confirms the feasibility of synergistic AP and CE governance (China Air Quality Management Assessment Report, 2016). However, there are high regulatory costs and low awareness of climate-related pollution prevention at the district level within the Ministry of Ecology and Environment (Li et al., 2022). In addition, the setting of separate targets for tackling climate change and controlling CE makes it challenging to synergize the control of AP and CE (Yamineva and Liu, 2019). The synergistic governance of AP and CE has become the starting point and focus of China’s efforts to protect its ecological environment. It is also an important initiative for realizing the high-quality development of a low-carbon economy.
The common factor contributing to the growth of AP and CE in China is the continuous consumption of fossil fuels (Dong et al., 2021). However, the digital means provided by the rapid development of the digital economy effectively reduce the consumption of fossil fuels, indicating a new direction for the synergistic governance of AP and CE. First, the digital economy is characterized by permeability and sharing, enabling it to penetrate all aspects of energy production and consumption (Xiao et al., 2023) and monitor AP and CE efficiently in real time using digital technologies such as sensors and image recognition. This provides data support for the synergistic reduction of AP and CE (Khatami er al., 2023). Second, the digital economy applies big data technologies and artificial intelligence to dip deeply into emissions monitoring data and identify primary pollution sources and critical control points (Pinto et al., 2020), then simulate them using mathematical models to develop precise and effective synergistic management programs for pollution and carbon reduction (Adu-Amankwa et al., 2023). Third, a unified digital pollution and carbon reduction regulatory platform built by the digital economy integrates information and data from relevant sectors, uses technologies such as blockchain to strengthen the synergistic linkages of multiple departments (Lu et al., 2022), conducts online transactions to reduce transactions costs, and expands the scale of participation, such as the online trading of CE quotas on carbon trading platforms (Yang and Zhang, 2023), thus providing a common incentive for pollution and carbon reduction.
The central government approved and constructed national-level big data comprehensive pilot zones across the country to promote the development of big data-related industries. These big data pilot zones offer a favorable environment for the development of the digital industry by fully leveraging the role of data elements in lowering barriers to data resources and improving infrastructure coordination (Abdalla et al., 2022). Pilot zones are asked to create advanced big data products, cultivate backbone enterprises and industry talents in big data, and effectively exploit the value of data resources to enhance the government’s management capacity to support economic transformation and upgrades (Wei et al., 2023). By continuously summarizing practical experiences that can be learned, replicated, and disseminated, viable strategies can be formed in pilot areas to boost the sustainable development of the digital economy (Wang et al., 2023). Since 2016, China’s eight national-level big data comprehensive pilot zones1 have been shaped to jointly lead the development of the big data industry in the eastern, central, western, and northeastern regions, providing a broad application space and scenario for digital technology.
The existing literature considers the establishment of big data pilot zones as a proxy variable for the digital economy and studies its impact on sustainable marketing (Chou et al., 2022), polycentric spatial structures (Liu et al., 2021b), smart city construction (Zhao et al., 2021), high-quality economic development (Guo et al., 2023), air pollution (Zhang and Ran, 2023), and carbon emission reduction (Bu et al., 2023). Scholars have further examined the synergistic effect of big data pilot zones on AP and CE using PM2.5 and CO2 emissions, respectively, as explanatory variables (Hu, 2023); however, because regional AP and CE are often the product of energy consumption and economic development, the synergistic system of the two needs to take into account the balance between resource utilization, environmental pollution, and economic development (Purohit et al., 2019).
In general, it is necessary to investigate the synergistic effect of the digital economy on pollution and carbon reduction by establishing big data pilot zones as natural experiments. The existing literature should measure the synergistic effect of AP and CE, and more research is needed on the impact of the digital economy on the efficiency of synergistic AP and CE governance (ESACG). Given this, the possible marginal contributions of this study are as follows: 1) The assessment of the synergistic effects of AP and CE used in most studies starts from the absolute amount of emissions reduction, but AP and CE as undesirable outputs require a more detailed examination in conjunction with efficiency scales. Therefore, a new framework for assessing the synergistic effects of AP and CE was constructed by selecting input-output indicators in the muti-dimensional aspects of economic development, resource utilization, and environmental governance, and AP and CE as undesirable outputs into the super-efficiency slack-based (SBM) model to calculate the ESACG, improving the system of indicators for assessing the synergistic effect of pollution and carbon reduction. 2) The exploration of synergistic governance paths has focused mainly on “source management” and “end blocking” and less on “process control.” Based on a quasi-natural experiment involving setting up big data pilot zones, the transmission mechanisms of the digital economy on the ESACG were examined in an all-around manner from the three dimensions of source management, process control, and end blocking. The possible moderating effects of regional “hard facilities” and “soft environment” were considered, providing a reference for the current governance pathway. 3) In contrast to the difference-in-differences (DID) method, which explores the heterogeneity of treatment effects by grouping regressions or introducing interaction terms, machine learning can better identify the differential impact of the digital economy on the ESACG by algorithmically capturing the treatment effects of each individual. The quantile DID (QDID) method and generalized random forest (GRF) model were deployed to study the heterogeneous impact of the digital economy on the ESACG in terms of experimental zone types, the potential for synergistic emission reduction, and urban resource endowment, facilitating regionally differentiated governance.
The outline of this article is as follows: The second section elaborates on the theoretical mechanisms and research hypotheses. The third section outlines the methodology and data. The fourth section explains the empirical results and provides a further discussion. Finally, we present our conclusions and policy recommendations.
2 Theoretical mechanisms and research hypotheses
2.1 Transmission mechanisms of the digital economy on the ESACG
The rigid constraints of peak carbon targets force carbon emitters to promote CE reduction and symbiotic air pollution reduction through the whole chain. They must pursue energy saving and emissions reduction measures involving source management, process control, and end blocking to reach and lower the peak in a timely manner. After adopting the closed-loop control idea of “strengthen the source, strictly control the process, and optimize the end (Wang et al., 2022),” the impact mechanisms of the digital economy on the ESACG are examined from three channels: source management, process control, and end blocking.
2.1.1 Pathway of source management
China’s industrial sector is a crucial engine of economic growth and accounts for the largest share of energy consumption and CE in the country (Yang et al., 2017). However, because it employs an economic model centered on crude resource support, China’s industrial low-carbon transition is not fully synchronized with its economic growth, which impedes the synergistic governance of AP and CE (Liu et al., 2021a). First, unlike the excessive consumption of tangible resources and energy in traditional industrial production, the digital economy involves data and information as key production factors and is considered green and low-carbon. With the rapid development of the digital economy, Internet-based industries, which are classified as technology-intensive, have gained new opportunities and are less dependent on energy, thus reducing AP and CE (Fan et al., 2021). Second, emerging digital technologies such as big data and artificial intelligence have boosted the digitization and decarbonization of traditional industries, accelerating the digitization of the energy industry. This shift accelerates the creation of an inverted U-shaped inflection point in the Kuznets curve of the environment by forcing the transition from low-end, resource-intensive businesses to high-tech, high-value-added sectors, such as technology-intensive industries (Liu and Dong, 2020). Finally, data integration and internet development infiltrate the industry by integrating traditional production resources (Ivus and Boland, 2015), prompting traditional industries to undergo an all-round whole-chain transformation. These changes improve the operational efficiency of industrial organizations by reducing the energy consumption of production processes while achieving quality and efficiency gains, ultimately realizing a synergistic reduction in AP and CE. Therefore, we propose the following hypothesis.
H1a. The digital economy can improve the ESACG through industrial structure optimization in source management.
2.1.2 Pathway of process control
Regarding process control, the ESACG can be enhanced from the enterprise, residential, and governmental perspectives. Enterprise departments are connected across network communications on digital platforms to dynamically collect energy flow data closely related to enterprise emission activities in real time (Xu et al., 2022). Enterprise production and services are optimized to prevent needless losses of energy resources throughout the manufacturing process, consequently enhancing energy efficiency and assisting in achieving the ESACG. In addition, by reducing information barriers and technical constraints in traditional resource use, digital technology has led to the digital transformation of enterprises, promoting the advancement of energy utilization technology (Yang et al., 2021). On the residential side, the digital economy has created favorable conditions for the rise of the sharing economy, which emphasizes the elastic matching of supply and demand to achieve dynamic, timely, accurate, and efficient supply demand matching for optimal resource allocation and avoiding unnecessary new outputs (Bai and Sun, 2021), as well as saving energy and reducing waste, the carbon footprints, and emissions (Plewnia and Guenther, 2018). Regarding the government’s role, digital technologies provide stable price signals and incentives for emissions reduction through a sound environmental regulatory system and improved efficiency of environmental information management to enhance the effective regulation of energy supply and use in the public sector and the market’s ability to withstand external shocks (Ren et al., 2022; Yang and Zhao, 2022). Thus, we propose the following hypothesis.
H1b. The digital economy can improve the ESACG by improving energy utilization efficiency in process control.
2.1.3 Pathway of end blocking
Regarding end blocking, green technological innovation has a crucial impact on the digital economy’s ability to improve the ESACG. By boosting clean energy research and experimental development (R&D) (Chen et al., 2023) and promoting the exploitation of pollution control technologies by compensating for the loss of profits incurred through the payment of pollution control fees, the digital economy may foster green technological innovation. It accelerates the incubation and transformation of green and low-carbon technology innovation in cities, feeding AP and CE reduction with green innovation output (Wang et al., 2022), thus effectively improving the ESACG. However, the knowledge spillover effect brought about by the digital economy has compelled businesses to speed up the adoption of innovative pollution control techniques and promote urban green technological innovation (Meng et al., 2023). Consequently, the urban innovation environment attracts talent and funding for R&D and the use of clean technological in the pollution absorption initiative, thereby improving the ESACG-enhancement effects in the digital economy. Furthermore, green technological innovation has increased the government’s capacity to monitor and gather environmental information from polluting businesses. This aids businesses in taking targeted actions to control and eliminate emitted pollutants, which improves their ability to reduce AP and CE (Shi et al., 2018). In general, green technological innovation facilitates a green digital transformation, provides cleaner production and precision tools, which synergizes with economy-wide end-of-pipe pollution control to jointly improve efficiency and equity in reducing AP and CE (Rao et al., 2021). Thus, we propose the following hypothesis.
H1c. The digital economy can improve the ESACG through the end blocking of green technological innovation.
Based on the above analysis, we propose the following comprehensive hypothesis.
H1. The growth of the digital economy can enhance the ESACG.
2.2 Moderating effects of the digital economy on the ESACG
For the digital economy to release the synergistic reduction effect of AP and CE it requires material, human, and financial support. This will ensure that the information technology on which the digital economy relies can maximize the services needed for the urban management of contamination and environmental protection (Shi et al., 2018). Digital infrastructure, with its advantages of spillover and sharing, is the primary carrier of the growth of the digital economy; it is the “hard facility” that supports the regional synergistic reduction of AP and CE. Simultaneously, the digital literacy of talent and digital financial support should be available in the digital economy era. This is also a significant “soft environment” affecting the ESACG.
The information network formed by the construction of digital infrastructure accelerates information dissemination by reducing information asymmetry and promoting the formation of informal environmental regulation, mainly through the Internet (Li et al., 2017). Moreover, informal environmental regulations shaped by the amplification and ripple effects of network platforms exert massive public opinion pressure to force highly polluting and energy-intensive industries to undergo green transformation and upgrading, facilitating the ESACG. Regarding the digital literacy of talent, increasing regional human digital literacy strengthens the information service capacity of the digital industry, which decreases the cost of matching regional talent and creates a “reservoir” of green technical assets (Zhang and Shi, 2019). In turn, the multiplying effect of the digital economy on the ESACG can be released continuously and steadily by providing high-level human digital capital for each link in the closed-loop control. Digital finance shows an innovative mode of operation compared with traditional financial institutions, ensuring the continued growth of the digital economy. With the massive amount of information provided by big data technology, which can be used to evaluate enterprises at a lower cost, digital finance offers diversified financing channels for enterprises to allow financial resources to precisely match the capital needs of green R&D and achieve green goals, effectively alleviating the enterprises’ financing constraints (Zhang and Hu, 2023). In addition, through the provision of green financial products and services, digital finance improves the transparency of environmental information, expands green investment and financing channels to widen the difference between risk spillover of green assets and traditional assets, forming a green and low-carbon economic and financial system (Duan et al., 2023).
Consequently, increasing green R&D investment promotes the implementation and application of green R&D results, facilitating the collaborative function of the digital economy in reducing AP and CE. The above analysis lead us to the following hypothesis.
H2. Digital infrastructure, digital literacy of talent, and digital financial support positively moderate the digital economy, improving the ESACG.
Figure 1 presents the framework of the digital economy’s influence the ESACG.
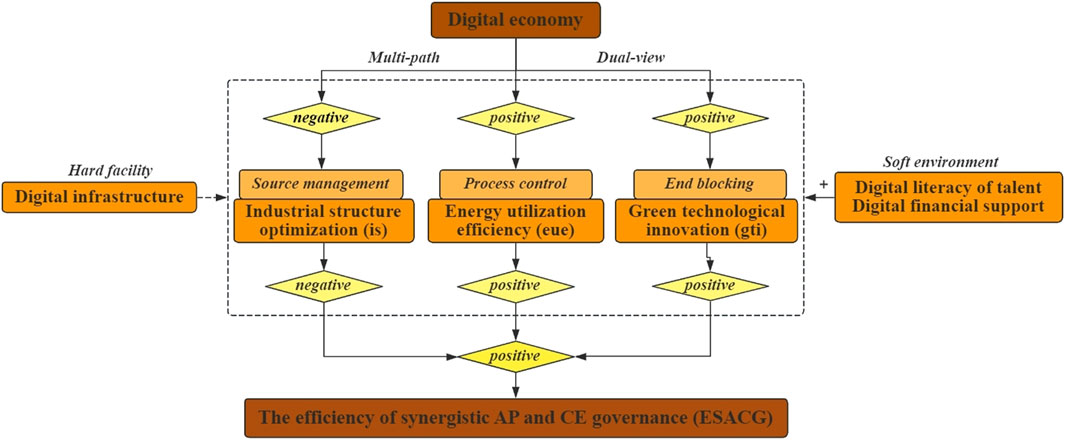
FIGURE 1. Mechanisms analysis of the digital economy affecting the ESACG. Note: The dashed line indicated that the effect was not significantly exerted.
3 Methodology and data
3.1 Empirical methodology
3.1.1 Benchmark regression model
Pilot zones are mainly concentrated at the provincial level. However, owing to the lack of representativeness of the provincial data and significant differences between provinces, we used municipal data as the research sample. Meanwhile, the traditional DID model applies to the cases in which policies coincide, but the pilot zone policy used in this study uses batches for piloting. Therefore, we employed a multi-period DID model to evaluate whether the digital economy affects the ESACG:
Furthermore, the multi-period DID model, although effective in mitigating endogeneity problems in assessing policy effects, cannot remove the selectivity bias of the sample. The combination of propensity score matching (PSM) and DID eliminated this bias:
where i stands for the city, t represents the year, and efficiencyit is the ESACG of city i in year t; didit indicates a dummy variable denoting the city’s pilot status, and its coefficient α1 reflects the impact of the digital economy on the ESACG in pilot cities. If city i start implementing the policy in year t, didit = 1; otherwise, didit = 0. Controlit is a set of control variables and λ represents its corresponding coefficient; μi and νt indicate the fixed effects of city and time, respectively; α0 stands for the intercept term and εit denotes the stochastic error term.
3.1.2 Intermediary effect model
To examine the potential indirect effect mechanisms of the digital economy on the ESACG, three intermediary variables-industrial structure (lnis), energy utilization efficiency (eue), and green technological innovation (lngti)-were added to the intermediary effects model. Baron and Kenny (1986) proposed the fractional steps method for verifying research hypotheses, and the specific model setup is as follows:
where β0 and γ0 are intercept terms; β1 and γ1 are the coefficients of didit; medit are the mediating variables, including lnisit, eueit, and lngtiit,; and θ denotes their influencing coefficients. δ and φ represent the coefficients of the control variables, whereas ηit and ξit represent the stochastic error terms. μi and νt are the same as above. Equation (3) examines the effect of medit on the ESACG and Eq. 4 simultaneously explores the effect of the digital economy and medit on the ESACG. The intermediary effect model consists of Eqs. (1), (3) and (4). If either β1 or θ is insignificant, further testing of the possible mediating effects using the Sobel test is required.
3.1.3 Moderating effect model
Three interactive terms (did*di, did*lndlt, and did*lndfs) were constructed to evaluate the moderating effects of three moderating factors: digital infrastructure (di), digital literacy of talents (lndlt), and digital financial support (lndfs). The existence of a moderating effect depends on the significance of the coefficient of the interaction term in Eq. 5:
where modit is denoted as diit, lndltit, and lndfsit, respectively. If ϕ is significantly positive or negative, modit is strengthening or weakening the digital economy’s contribution to the ESACG. The remaining variables are outlined above.
3.2 Variable selection
3.2.1 Core explanatory variable: the digital economy (didit)
Sixty-seven cities under the jurisdiction of the provinces, municipalities, and autonomous regions housing big data pilot zones were designated as the treatment group, and the remaining 211 cities were set as the control group. The dummy variable treatmentit in the experimental group was assigned 1; otherwise, it was assigned 0. Combined with the time of the pilot zone establishment, the establishment of pilot cities was set to 2016, except for Guizhou Province, where the policy pilot time was set to 2015. The time dummy variable postit took the value of 1 in the year in which the policy was piloted and in subsequent years; otherwise, it was assigned the value of 0. The policy dummy variable didit was the proxy for this study’s core explanatory variable: the digital economy.
3.2.2 Explained variable: Efficiencyit
The homogeneity of AP and CE has generated many in-depth assessments of their mutual synergistic effects using synergistic control coordinate systems, pollutant cross-elasticity analysis, and coupled coordination models (Alimujiang and Jiang, 2020; Tang et al., 2022). However, the measurement methods involved in most studies start from the absolute amount of emissions reduction and do not consider that regional AP and CE are often the products of multiple factors. Therefore, we calculated the synergistic effects of AP and CE in detail by combining the efficiency scales.
To measure the ESACG more reasonably and accurately, resource consumption, environmental management, and other indicators were selected to form a comprehensive index system (Wu and Guo, 2016; Li et al., 2022) (Table 1). It is worth mentioning how we measured AP and CE: urban CE were obtained by adding CE from electricity, gas and liquefied petroleum gas; transport and thermal energy consumption; and air pollutant emissions normalized by the air pollutant equivalent coefficients specified in the Environmental Protection Tax Act. The specific calculations were as follows.
where
The traditional data envelopment analysis (DEA) models used in the extant literature do not consider variable slackness and angle selection, which leads to an overestimating of efficiency, in addition to external factors and random errors that interfere with the results (He et al., 2023). The SBM-DEA model proposed by Tone (2001) incorporates slack variables directly into the objective function of production, overcoming the problem of ignoring environmental and resource factors when measuring efficiency. An undesirable super-efficiency SBM-DEA model was developed to measure the ESACG using MaxDEA software, as follows: Suppose that the ESACG of n DMUs is measured, denoted as
where ρ is the ESACG of DMU and
3.2.3 Mechanism variables
Mediating variables. Because secondary industries are highly polluting, a reasonable and advanced industrial structure effectively improves the ESACG. Industrial structure (lnis) was measured using the natural logarithm of secondary industry value-added as a percentage of GDP (Tang et al., 2022), a negative indicator. Energy intensity represents the energy cost required to obtain a unit of GDP accurately and directly. Therefore, energy intensity was commonly used to measure a region’s energy utilization efficiency (eue) (Xue and Zhou, 2022), with the inverse of the energy intensity serving as a proxy variable. The level of green technological innovation (lngti) was measured by the number of green patent applications in each city obtained by searching the green patent classification codes published by the World Intellectual Property Organization (Liu and Dong, 2020).
Moderating variables. The level of digital infrastructure (di) was expressed as a composite index obtained by applying the entropy weight method to the percentage of employees in the information transmission, software, and computer services industries; the total amount of telecommunication services per capita; the number of Internet broadband access subscribers per 100 people; and the number of cell phone subscribers per 100 people (Wen and Zhong, 2022). The number of university students per 100,000 residents in the area was employed to measure the digital literacy of talent (lndlt) (Eshet, 2004). The proxy variable for digital financial support (lndfs) was the Digital Inclusive Finance Index provided by Peking University’s Digital Finance Research Center (Guo et al., 2020).
3.2.4 Control variables
The following variables that affect the ESACG were considered to mitigate the bias introduced into the empirical study by the omitted variables. The logarithm of GDP per capita defined the level of economic development (lnedl) (Yin et al., 2015). The logarithm of year-end people per unit of administrative region was employed to calculate population density (lnpd) (Liu et al., 2021a). The ratio of real foreign investment to GDP was used to measure foreign direct investment (fdi) (Li et al., 2018). The ratio of general fiscal budget revenue to general fiscal budget expenditure was used to measure the degree of fiscal decentralization (fdd) (Yao et al., 2020). The percentage of the city’s resident population with an undergraduate degree or higher was used to calculate human capital (hc).
3.3 Data sources
Given the availability and uniformity of data, panel data from 278 cities from 2006 to 2019 were chosen for quantitative estimation. The number of green patent applications was obtained from the State Intellectual Property Office2. The Digital Inclusive Finance Index was obtained from the Digital Finance Research Center of Peking University3. The list of each policy pilot was obtained from official documents or websites issued by the State4. Other original city data were mainly obtained from statistics such as the China City Statistical Yearbook, China Regional Economic Statistical Yearbook, China City Construction Statistical Yearbook, China Statistical Yearbook, and statistical bulletins of each city5. A linear interpolation method was applied to fill in any missing data. In addition, all economic variables involving prices were treated as constant, with 2006 as the base period. Continuous variables were subjected to 1% and 99% tailings on both sides to exclude interference from extreme values. Table 2 presents the variables’ descriptive statistics.
4 Empirical results and further discussion
4.1 Benchmark regression results
We gradually added time and city two-way fixed effects and control variables to eliminate the impact of individual and temporal differences. Parallel to this, the PSM-DID estimation method based on radius matching was further adapted for robustness testing to overcome the synergistic differences in the trend in changes among pilot cities and other cities and to reduce the estimation bias of the DID method. The results are shown in Columns (3) and (4) of Table 3. According to the DID estimation results presented in Columns (1) and (2) of Table 3, with or without the control variables, the digital economy improved the ESACG at the 1% significance level, which is consistent with H1. The PSM-DID estimation results shown in Columns (3) and (4) of Table 3 confirm the benchmark regression results and illustrate the importance of the synergistic governance impact of the digital economy on AP and CE.
The above results are consistent with the finding of Hong (2023) that the development of the digital economy in Chinese cities had a significant pollution and carbon reduction effect, and the conclusions of Zhang and Ran (2023) and Bu et al. (2023) that the establishment of the pilot zone reduced AP and CE, respectively, which is in line with the theoretical expectation that the digital economy enhanced the effectiveness of collaborative governance. The reason for this is that big data pilot zones have a sound digital infrastructure, which provides technical support for various types of environmental monitoring tools, realizes the information linkage of environmental sectors and forms a more efficient environmental governance model, thus promoting the synergistic process of urban pollution and carbon reduction.
4.1.1 Parallel trend test results
The treatment and control groups must adhere to the parallel trend hypothesis in which there is no discernible difference in the ESACG, which is a prerequisite for adopting the multi-period DID model. Considering that the pilot policy was implemented in batches, the event study method was adopted to examine the time trend of the digital economy’s impact on the ESACG. The results of the parallel trend test showed that, before policy implementation, the estimated coefficients essentially fell around 0, and the upper and lower limits of the confidence intervals both contained 0, indicating that the parallel trend hypothesis was satisfied.
4.1.2 Placebo test results
The placebo test utilizes a nonparametric permutation test to prevent estimate bias due to omitted explanatory variables (Lu et al., 2021). A non-replicated random sampling method was used to generate a list of pilot cities in a comprehensive big data test area. The estimated probability density distribution of the coefficients obtained after 500 replicate regressions indicated that the distribution of the estimated coefficients was approximately around one and normal. The benchmark regression coefficient (0.0634) fell in the low tail of the probability density distribution, indicating that other unobserved factors did not influence the synergistic reduction effect of the digital economy.
4.1.3 Robustness test results
The robustness tests included excluding other policies, lagging the explanatory variables by one period, excluding special cities, and considering multi-period DID method weight heterogeneity. Tables 4–6 present the specific findings of the robustness tests.
First, the synergistic impacts of the digital economy on AP and CE may be over- or underestimated because of the possibility that other digital, environmental, and low-carbon policies in the same area and time frame influence the ESACG. Hence, six representative pilot policies in the three policy categories were re-estimated as policy dummy variables in the baseline regression model, including “Broadband China Pilot Policy” (bc), “Smart City Pilot Policy” (sc), “Air Pollution Prevention and Control Action Plan” (ap), “Sulfur Dioxide Emission Trading Pilot Policy” (sdet), “Low-carbon City Pilot Policy” (lc), and “Carbon Emissions Trading Pilot Policy” (cet). Table 4 presents the empirical results, which demonstrate that all regression coefficients were significantly positive at the 1% level when considering other policy considerations, sequentially or simultaneously. In other words, the driving influence of the digital economy on the ESACG continued after eliminating other policy shocks.
Second, to exclude the effect of different time trends faced by cities in the treatment and control groups prior to the establishment of the experimental area, joint city and time fixed effects were added to the model to better control for the interference of factors that changed over time for each city sample, as shown in Column (1) of Table 5. Third, the explanatory variables were lagged by one period before being empirically estimated to account for the possibility that the pilot zone did not have an immediate influence due to the time-leg effect and to avoid joint equation bias, as shown in Column (2) of Table 5. Finally, special cities were excluded from the analysis. Regarding the synergistic reduction in AP and CE, cities with greater administrative levels tended to have more onerous restrictions, stricter constraints, and heavier governance, which may have affected the baseline results. Therefore, as indicated in Column (3) of Table 5, the regressions were conducted again after excluding the city samples corresponding to municipalities, provincial capitals, and planned cities. The core explanatory variable’s coefficients were all positive and significant at the 1% level in the regression results after the above treatment, indicating the robustness of the digital economy’s driving effect on the ESACG.
Finally, when groups are treated at various times, the traditionally estimated coefficients are viewed as the weighted average sum of the treatment effects across the treated samples at each time point, which may be negatively weighted, leading to a weak regression result. We drew on the methodology of Chaisemartin and D’ Haultfoeuille (2020) to calculate the share of negative weights and found no such share; therefore, the results of the benchmark regression can be considered robust. After the treatment effect test, which considered the heterogeneity of the multi-period DID method (Gardner, 2021; Sun and Abraham, 2021), the results in Table 6 show that the coefficient of the core explanatory variable was still significantly positive, and the benchmark regression result was reliable.
4.2 Intermediary and moderating effect analysis
4.2.1 Transmission mechanisms of the digital economy on the ESACG
A three-step approach was applied to verify the transmission mechanisms of the digital economy affecting the ESACG. The empirical results of the final two steps, which correspond to Eqs. 3, 4, are shown in Table 7. As at least one of the coefficients β1 and θ corresponding to the three mediating variables were insignificant, the Sobel test was applied to identify possible mediating mechanisms (Table 8).
In the source management stage, the mediating variable of industrial structure corresponded to a Sobel test value of 0.0071, indicating that the digital economy improved the ESACG through industrial structure optimization, which was consistent with the findings of Henry (2023) and H1a. This may be because China’s energy consumption is mainly concentrated in high-energy-consuming industries in the polluting and carbon-emitting sectors. By significantly reducing the share of secondary industry, particularly heavy industries, the digital economy helps promote the transition to a low-carbon green industry, affecting the synergistic reduction in AP and CE. The digitization of heavy industry should be accelerated to reduce its environmental footprint and industrial policies should be rationally formulated to guide the industry’s sustainable development.
During process control, the Sobel test value corresponding to the mechanism of energy utilization efficiency was 0.0062, which indicates that improving energy utilization efficiency played a role in the digital economy’s impact on the ESACG, verifying H1b. The study of Contini et al. (2023) found that digital technology improved energy utilization efficiency in the industrial production process to realize the effect of pollution and carbon reduction, supporting the finding of this paper. This may be because the digital economy connects economic agents with lower transaction costs, achieves precise matching of market supply and demand, optimizes global resource factor allocation, and reduces unnecessary energy efficiency losses to improve energy utilization efficiency. Enhancing energy utilization efficiency is essential for achieving synergistic AP and CE reductions. We should vigorously promote the digitization and intelligence of energy systems; formulate policies to support the research, development, and application of energy efficiency enhancement technologies; and establish an assessment mechanism linking energy efficiency and pollution emissions to fully tap the emissions reduction potential of energy efficiency enhancement as a pathway.
In the end-blocking stage, the mediation test for the channel of green technological innovation corresponded to a p-value of 0.0009, which shows that green technological innovation partially mediated the synergistic reduction in AP and CE in the digital economy, confirming H1c. The finding of Kurniawan et al. (2022) that digital technology promoted green innovation and a circular economy to achieve the end-of-pipe governance was similar to our result. Owing to China’s long-standing crude economy and high share of energy-intensive industries, clean pollutant recovery technologies cannot fully absorb residual pollutant emissions. The digital economy makes it possible for businesses to lower their barriers to entry for green technological innovation, unleashing their incentive for green technological innovation, which sparks the development of better pollution control and carbon management technologies, thus boosting the ESACG. To make full use of green technological innovation to reduce emissions, China should increase financial support for environmental technological innovation; establish a green technological intellectual property protection system; and strengthen green technological cooperation between enterprises, universities, and scientific institutes.
Through the above analysis, the shared path for the digital economy to exert the synergistic effect of AP and CE lied in the whole chain of energy saving and emission reduction initiatives of source management, process control, and end blocking within the city. However, Sun and Deng (2022) found that environmental policies only inhibited CE in source prevention and process control, which was at odds with our findings. This may be because environmental policies are more concerned on administrative control and punishment, with limited incentives for corporate technological innovation and a greater emphasis on aggregate control; however, big data pilot zones provide better digital technology support and incentives to promote green technological innovation (Yuan and Pan, 2023), and digital technology governance is characterized by fine-grained, dynamic optimization, achieving more efficient end-of-pipe emissions reduction.
4.2.2 Moderating effects of the digital economy on the ESACG
To further examine the possible moderating effects of cities’ “hard facilities” and “soft environment” in the digital economy-driven ESACG process, we introduced interaction terms between the policy variable didit and the moderating variables and observed the significance of the corresponding coefficients to determine the moderating effect. The regression results are presented in Table 9.
The results in Column (1) of Table 9 show that the coefficient of the interaction term (did*di) between the digital economy and digital infrastructure failed the significance test, in line with the theory of scale economics and cost-benefit analysis. While digital infrastructure is beneficial in guiding industries toward intensive green development and achieving intelligent and efficient environmental governance, the manufacture and use of new digital facilities also exacerbates the dependence on fossil energy in the electricity and basic materials sectors (Sadorsky, 2012; Park et al., 2018), which is detrimental to the synergistic AP and CE reduction in cities. In parallel, antiquated traditional infrastructures aggravate environmental pollution (Higón et al., 2017). These two effects cancel each other out, indicating that digital infrastructure cannot significantly enhance the digital economy’s impact on the ESACG. Therefore, to guide its sustainable transformation, it is necessary to promote the transition from digital infrastructure to renewable energy, improve the recycling systems of digital facilities, and strengthen regulations that eliminate pollution from traditional infrastructure.
The results in Column (2) of Table 9 show that the coefficient of the interaction term (did*lndlt) between the digital economy and digital literacy of talent was significantly positive, indicating that digital literacy has a positive moderating influence on the digital economy, taking on a synergistic role in reducing AP and CE, in line with H2 and the theory of human capital. Digital literacy of talent enhances the acquisition of digital technological knowledge. As regional talent’s digital knowledge and skills continue to improve, they ensure that the digital industry’s development demand for human capital is met and that the ESACG-driven effects of the digital economy are more fully realized. To provide solid human capital support for the development of the digital economy, the government should increase the cultivation of digitally skilled talent, encourage enterprises to conduct digital skills training for employees, and improve evaluation and incentive mechanisms for digital talent to create a favorable digital learning environment.
The results in Column (3) of Table 9 show that the coefficient of the interaction term (did*lndfs) between the digital economy and digital financial support was significantly positive, demonstrating that digital financial support reinforces the positive impact of the digital economy on the ESACG, supporting H2. A possible reason for these results is that the diversified financing channels offered by digital financial support provide funding for enterprises’ green innovation R&D, which stimulates their motivation for green technological innovation. Additionally, digital finance effectively reduces enterprises’ financing costs owing to its inclusive nature, ensuring that they have sufficient funds to absorb and apply green innovation technologies that are advantageous for synergistic AP and CE reduction. In general, digital financial support to play a reinforcing role is mainly realized through two mechanisms of providing financial support and reducing transaction costs, which is consistent with the theory of information economics and transaction cost of digital finance. Thus, the way to employ the moderating role of digital finance is for the government to formulate preferential policies to reduce the financial costs of green innovative enterprises, establish green credit policies and standards to guide the flow of funds to green industries, and encourage financial institutions to innovate green financial products. Digital collaborative governance models between enterprises and financial institutions should be promoted to facilitate sustainable corporate financialization in response to corporate financialization crises caused by climate uncertainty (Ren et al., 2023).
In summary, the strengthening effects of regional “soft environment” are consistent with its supporting role for the development of digital economy industry, in line with the theoretical expectations of this paper; however, the empirical conclusion that regional “hard facility” fail to play a reinforcing role is not in keeping with the mainstream view that digital infrastructure reduce AP and CE, which enriches the application of theories of scale economics and cost-benefit analysis in the field of digital emissions reduction. The reason may be related to the carbon rebound effect of digital infrastructure construction proposed by Lan and Zhu (2023).
4.3 Heterogeneity analysis
Whereas the traditional linear regression model focuses on the average treatment effect of the policy, the GRF model can estimate individual treatment effects as well as the average treatment effect of the policy. Figure 2 shows the distribution of the estimation treatment effects. The figure shows that the individual treatment effects were scattered between −0.1 and 1.1, with most of them concentrated around 0.1, indicating that the digital economy has significant differences in affecting the ESACG. The heterogeneity of treatment effects in the sample was tested using the optimal linear prediction method proposed by Chernozhukov et al. (2018). According to the results of the heterogeneity test (see Table 10), the average treatment effects were significantly positive. When not clustered, the differential coefficient was significantly positive, indicating heterogeneity of the synergistic reduction effects. As a result, the impacts of heterogeneity on the experimental zone type, potential for synergistic emissions reduction, and urban resource endowments were determined.
4.3.1 Heterogeneity analysis of the experimental zone type
The integrated big data pilot zones were divided into pioneering pilot zones (Guizhou Province), cross-regional pilot zones (Beijing-Tianjin-Hebei and Pearl River Delta), regional demonstration pilot zones (Shanghai Municipality, Henan Province, Chongqing Municipality, and Shenyang City), and big data infrastructure-integrated development pilot zones (Inner Mongolia). Because the four types of pilot zones have different functional positioning and focus, which may differentiate the digital economy’s impact on the ESACG, cities belonging to different categories of pilot zones were analyzed along with other non-test zone cities to form experimental and control group.
The empirical results in Table 11 show that the digital economy had a significant impact on improving the ESACG in the cross-regional and regional demonstration pilot zones, and the promotion effect was more pronounced in the regional demonstration pilot zones than in cross-regional ones; however, the construction of the pioneering pilot zones dampened the ESACG, and its impact on the ESACG in big data infrastructure-integrated development zones was insignificant. These findings are different from what was expected: pioneering pilot zones were expected to have a positive impact on enhancing the ESACG due to their emphasis on cutting-edge innovations in the digital economy. It is possible that the rapid expansion of big data infrastructure has harmed the environment because the pioneering pilot zones have overemphasized digital technological innovation and neglected the supporting work on pollution and carbon reduction, and that emissions reduction policy and regulation have not been perfected. Big data technology improved resource utilization efficiency, so the establishment of a infrastructure-integrated development pilot zone was expected to have a positive effect on emissions reduction. The positioning of such pilot areas indicates they should strengthen their green and intensive development based on the full use of regional energy and climate conditions. However, the current situation of the Inner Mongolia Autonomous Region’s overreliance on the resource-consuming development model and disproportionate economic output has obscured the driving effect of the digital economy on the ESACG. Conclusions obtained in the cross-regional and regional demonstration pilot areas were in line with theoretical expectations. Because cities in these two pilot zones have better digital infrastructures, deeper integration of big data applications with traditional industries, and innovative ecological construction of big data industry services, enabling them to better balance the development of green and digital industries. Cross-regional pilot zones are often not limited to the province in which they are located but are intended to reach neighboring provinces and the entire country. By contrast, regional demonstration pilot zones can better develop policy systems and regulations tailored to the region’s conditions; therefore, the policy dividend effect of establishing regional demonstration pilot zones is more significant than that of other zones.
The most relevant literature only divides the test area into two batches for heterogeneity analysis (Geng et al., 2023), and our subdividing them into four categories for heterogeneity test, which is a new perspective and direction that has not yet been covered by the exiting literature, providing a new idea of category division and a research framework for the future assessment study of the pilot area. Based on this, pioneering pilot zones should optimize the layout of digital infrastructure and strengthen the environmental impact assessment of projects to prevent the adverse environmental effects of digital construction. Cross-regional pilot zones should enhance resource sharing and complementarity, promote the development of digital coordination among regions, and build regional cooperation mechanisms for digital environment governance. Regional demonstration pilot zones can develop the digital economy in conjunction with local characteristics and explore the best pathways for coordination between digitization and greening within the region. Big data infrastructure-integrated development pilot zones must change their development model, leverage their location and resource advantages to promote green digitization, and establish a unified mechanism for assessing and monitoring the digital environment’s impact to explore effective paths for digital green transformation in the region.
4.3.2 Heterogeneity analysis of the potential for synergistic emissions reduction
Variances in the potential for synergistic emissions reduction cause the policy dividend of the digital economy to influence the pilot regions differently because there are considerable differences in the degree of government governance among regions. The quantile DID method was adopted to explore the differential impact at various ESACG levels, and the results are presented in Table 12.
The panel quantile regression test found a positive effect of the digital economy on the ESACG between the 25th and 90th quantiles, with the strength of the effect increasing and then decreasing in an approximately asymmetric inverted “U” shape, with the maximum effect at the 50th quantile. However, the digital economy had no significant impact on the ESACG in cities in the 10th quantile, possibly because the regions with low ESACG need to transform their industrial sectors, foster new industries, and reduce their reliance on traditional industries. This, coupled with an inadequate synergistic governance mechanism, lowers the intrinsic motivation of local government to reduce AP and CE. By comparison, the regions in the remaining quantiles have a relatively good foundation for synergistic governance, providing room for exploring digital resources to empower synergistic AP and CE reduction. Therefore, for regions where the ESACG is located between the 25th and 90th quantiles, we can further explore ways to digitally empower them, advocate for and support emerging technology enterprises to carry out innovative practices, develop more digital technologies related to emissions reduction, and strengthen the cultivation and exchange of talent, thus promoting the in-depth integration of the digital economy and emissions reduction industries. Regions with ESACG in the 10th quantile must learn from the advanced paradigm of green digital development, increase support for industrial sector transformation and the cultivation of emerging industries, and establish a government-guided fund to subsidize and support local enterprises in implementing green technology reforms and innovations, helping them break away from their dependence on traditional industries.
In fact, the heterogeneity analysis of the potential for synergistic emissions reduction differs from the expected result that the synergistic effect of pollution and carbon reduction in the digital economy improves with the increase of ESACG quantiles. Hu (2023) used a quantile regression model and found that the pollution reduction effect of the digital economy is characterized by increasing marginal effect and the marginal effect of its carbon reduction is not obvious, which is at odds with our conclusions. This may be because the inverted “U” shape is more in conformity with the law of diminishing marginal effects of the digital economy, where the absorption effect of digitization and the room for improvement are greatest at medium levels of the ESACG, while there is limited room for improvement at high levels, and insufficient absorptive capacity at lower levels. Our findings are consistent with the idea that the level of governance matches the absorption effect of digitization and provide new evidence of the diminishing marginal effect of the digital economy.
4.3.3 Heterogeneity analysis of urban resource endowment
Because resource endowment differences in the economic base, human capital, and technology innovation among cities stimulate national output growth to varying degrees (Inuwa et al., 2023), it was necessary to further explore the synergistic effects of the digital economy on the ESACG in different cities with different resource endowments. The importance ranking results show that the two factors most crucial in influencing synergistic AP and CE reduction were the levels of economic development and technological innovation, as indicated by GDP per capita and the number of patent applications, respectively. Figure 3 illustrates the relationship between the two essential variables and the ESACG.
The figure on the left reveals a significant increase in ESACG returns to the digital economy as economic development levels increase. Among the less-developed regions in China, where economic development is less than RMB 25,000, digital economy development had the least driven impact on the ESACG. The economic development in these cities is often accompanied by significant energy consumption, resulting in little synergistic emissions reduction from the digital economy. At an economic development level of approximately RMB 50,000, ESACG returns from digital economy development in developed regions reached their peak and then stabilized. This is because the marginal benefits diminish when economic development reaches a certain level. The reduction in energy consumption intensity gradually decreases without major industrial changes or technological innovation, and the impact then tapers (Mirza and Kanwal, 2017). In less-developed areas, infrastructure inputs must be increased and network coverage and utilization should be improved to provide good basic conditions for developing the digital economy. Simultaneously, it is necessary to develop a distinctive digital economy based on local conditions to drive the economic level up through industrial upgrades. In regions with medium-to-high levels of economic development, it is necessary to continue to promote the deep integration of digital technology with traditional industries for industrial transformation and upgrading and to establish a sound digital environmental governance system. Furthermore, interregional digital infrastructure connectivity should be strengthened to prevent the widening of the digital divide between the two types of regions, and the sharing of digitization results should be promoted to achieve collaborative cross-regional governance.
The figure on the right demonstrates that cities with greater technological innovation are conducive to unlocking synergistic reduction effects. This reasoning comes from the nature of the digital economy, which is embedded in technological innovation and dictates that its development level and quality are limited by the local innovation capacity. Establishing big data pilot zones in cities with high levels of technological innovation can encourage the emergence of new digital businesses and technologies, thereby releasing larger synergistic reduction benefits for AP and CE. The positive impact of technological innovation in cities plateaued after rapid growth, as the diffusion of new technological innovation had a certain time lag. The key to fully unleashing the synergistic effects of the digital economy to reduce AP and CE lies in the continuous promotion of technological innovation. Therefore, we should invest in scientific research; build a suitable mechanism for cooperation between industry, academia, and research; formulate policies to support digital innovation; and focus on developing environmentally friendly digital technologies. We should establish a linkage assessment mechanism between technological innovation and environmental benefits, continuously conduct digital innovation experiments, and form a virtuous cycle of technological innovation and emissions reduction.
5 Conclusions and policy recommendations
5.1 Conclusions
Using panel data of 278 prefecture-level cities from 2006 to 2019, the SBM model was adopted to calculate the ESACG, and multi-period DID and PSM-DID models were adopted to empirically examine the effect of the digital economy on the ESACG, with the establishment of the National Big Data Comprehensive Pilot Zone as a proxy variable. Intermediary and moderating effect models were introduced to explore pathways and moderating mechanisms. Moreover, the quantile DID method and GRF model were used to analyze the differential impact of the digital economy on the ESACG. The main findings of this study are as follows: 1) Developing the digital economy significantly increased the ESACG. This finding held after several robustness tests. 2) The digital economy promoted the ESACG by optimizing the industrial structure in source management, improving energy utilization efficiency in process control, and facilitating green technological innovation in end blocking. 3) The digital literacy of talent and digital financial support strengthened the enhancing effect of the digital economy on the ESACG, while the strengthening effect of digital infrastructure was insignificant. 4) The digital economy had a significant impact on improving the ESACG in pilot cities in the cross-regional and regional demonstration pilot zones; however, it inhibited the ESACG in pilot cities in the pioneering pilot zones, and the impact on pilot cities in the big data infrastructure-integrated development pilot zone was insignificant. 5) The digital economy positively impacted the ESACG between the 25th and 90th quantiles, and the impact intensity increased then decreased in an asymmetrical inverted “U” shape, with the greatest impact at the 50th quantile. However, the effect of the digital economy on cities in the 10th percentile of ESACG was insignificant. 6) The ESACG returns to the digital economy significantly increased as economic development and technological innovation levels increased, and their effects tended to level off.
5.2 Policy recommendations
Based on the above findings, some policy implications can be drawn: First, China should continuously and intensively promote the construction of existing pilot zones and the critical role of big data technology in all aspects of environmental governance. Pilot cities should focus on improving data-sharing mechanisms and enhancing their capacity for urban environmental monitoring and information sharing. Cutting-edge technologies, such as digital twins and artificial intelligence, can be used to establish a sophisticated environmental management system that fully unlocks the coordination of reducing pollution and CE during the development of the digital economy by optimizing the industrial structure and enhancing energy efficiency. Simultaneously, pilot cities should increase green R&D subsidies and promote the research and promotion of pollution treatment and remediation technologies to strengthen the application of green technological innovation in the construction of pilot zones. China should provide full play to the vital role of green technological innovation in the end link, such as post-pollution control, and realize the whole process of environmental management from source management and process control to data driven end blocking, thus improving the ESACG of the pilot cities comprehensively. Second, it is important to summarize the successful experiences of existing pilot zones and steadily expand the coverage of the test areas. If the existing conditions for the declaration of the National Big Data Comprehensive Pilot Zone are met, the government should appropriately increase the environmental performance assessment criteria and optimize the screening mechanism for pilot zones to select more exemplary and representative pilot areas. A new round of pilot construction should be carried out in areas with challenging environmental governance tasks and good digital infrastructure, while considering the differences in the level of economic development and technological innovation in different regions. The selection of pilot areas requires adequate consideration of the talent and financial element guarantees, prioritizing the coverage of regions with strong digital talent resources and support from financial institutions from the green digital economy. When expanding the area covered by the pilot zone, attention should be paid to leveraging the lessons learned and promoting the formation of a replicable and scalable model for digital emissions reduction. Finally, China should promote differentiated and precise governance strategies and categorize and guide the construction and promotion of pilot zones. Cross-regional and regional demonstration pilot zones will further enhance data networking sharing and establish a joint interregional responsibility assessment mechanism for emissions reduction targets to realize the demonstration effects of regional governance. Pioneering pilot zones should improve infrastructure construction planning, rationalize the layout of digital infrastructure, avoid blind construction and duplication, optimize urban digital management modes, and monitor the environmental impact of big data applications throughout the entire process to reduce the adverse effects of policy pilots on the effectiveness of emissions reduction. Big data infrastructure pilot zones should fully use their regional advantages, increase resource integration efforts, and strengthen cross-regional cooperation with the eastern and central regions’ industries, talent, and application advantages, thereby realizing green-intensive development. In addition, key supervision should be implemented for pilot zones with low ESACG and resource support should be increased to enhance the autonomous governance capacity of such pilot zones. China should promote the successful model experiences of the pilot zones by considering local realities and continue to encourage the pilot zones to deepen their reform and innovation to promote the in-depth integration of digitization tools with pollution and carbon reduction governance.
Data availability statement
The original contributions presented in the study are included in the article/Supplementary material, further inquiries can be directed to the corresponding author.
Author contributions
BY: Conceptualization, Funding acquisition, Investigation, Methodology, Writing–original draft, Writing–review and editing. PK: Investigation, Methodology, Writing–original draft, Supervision. XD: Funding acquisition, Writing–original draft.
Funding
The author(s) declare that financial support was received for the research, authorship, and/or publication of this article. This research is supported by the Major Project of Changsha Science and Technology planning Project (kh2301012), the Key Project of the Hunan Education Department (21A0371), the Natural Science Foundation of Environmental Protection Department of Hunan Province (HBKF2022004), and Research Foundation of the Department of Natural Resources of Hunan Province (Grant No. 20230141ST).
Conflict of interest
The authors declare that the research was conducted in the absence of any commercial or financial relationships that could be construed as a potential conflict of interest.
Publisher’s note
All claims expressed in this article are solely those of the authors and do not necessarily represent those of their affiliated organizations, or those of the publisher, the editors and the reviewers. Any product that may be evaluated in this article, or claim that may be made by its manufacturer, is not guaranteed or endorsed by the publisher.
Footnotes
1In September 2016, the National Development and Reform Commission (NDRC), and the Ministry of Industry and Information technological (MIIT), and the Central Internet Information Office (CIIO) approved Guizhou Province to become the first national-level big data comprehensive pilot zone. In October 2016, a total of seven regions, including Beijing-Tianjin-Hebei, the Pearl River Delta, Shanghai Municipality, Henan Province, Chongqing Municipality, Shenyang Municipality, and the Inner Mongolia Autonomous Region, promoted the construction of national-level big data comprehensive pilot zones.
2State Intellectual Property Office: https://www.cnipa.gov.cn/
3Digital Finance Research Center of Peking University: http://idf.pku.edu.cn
4Cyberspace Administration of China (Big Data Pilot Zone): http://www.cac.gov.cn/index.htm
5National Bureau of Statistics: http://www.stats.gov.cn/
References
Abdalla, W., Renukappa, S., and Suresh, S. (2022). An evaluation of critical knowledge areas for managing the COVID-19 pandemic. J. Knowl. Manag. 26 (10), 2634–2667. doi:10.1108/JKM-01-2021-0083
Adu-Amankwa, N. A. N., Rahimian, F. P., Dawood, N., and Park, C. (2023). Digital Twins and Blockchain technologies for building lifecycle management. Autom. Constr. 155, 105064. doi:10.1016/j.autcon.2023.105064
Alimujiang, A., and Jiang, P. (2020). Synergy and co-benefits of reducing CO2 and air pollutant emissions by promoting electric vehicles--A case of Shanghai. Energy sustain. Dev. 55, 181–189. doi:10.1016/j.esd.2020.02.005
Bai, X. J., and Sun, X. Z. (2021). Impact of Internet development on total factor carbon productivity: induced by cost, innovation, or demand? China Popul. Res. Environ. 31 (10), 105–117.
Baron, R. M., and Kenny, D. A. (1986). The moderator-mediator variable distinction in social psychological research: conceptual, strategic, and statistical considerations. J. Pers. Soc. Psychol. 51 (6), 1173–1182. doi:10.1037/0022-3514.51.6.1173
Bu, H., Li, G. M., Yu, X. Y., and Xun, Z. (2023). Is smart carbon emission reduction justified in China? Evidence from national big data comprehensive pilot zones. Heliyon 9 (7), e17744. doi:10.1016/J.HELIYON.2023.E17744
Chaisemartin, C. D., and D' Haultfoeuille, X. (2020). Two-way fixed effects estimators with heterogeneous treatment effects. Am. Eco. Rev. 110 (9), 2964–2996. doi:10.1257/aer.20181169
Chen, X. H., Zhou, P., and Hu, D. B. (2023). Influences of the ongoing digital transformation of the Chinese Economy on innovation of sustainable green technologies. Sci. Total Environ. 875, 162708. doi:10.1016/j.scitotenv.2023.162708
Chernozhukov, V., Demirer, M., Duflo, E., and Fernández-Val, I. (2018). Generic machine learning inference on heterogeneity treatment effects in randomized experiments, with an application to immunization in India. NBER Work. Pap., 24678. doi:10.3386/w24678
China Air Quality Management Assessment Report (2016). Atmospheric pollutants and greenhouse gases have the same roots and origins, and air pollution control has a carbon-reducing effect. China Environ. Sci. 36 (10), 2929.
Chou, S. F., Horng, J. S., Liu, C. H., Yu, T. Y., and Kuo, Y. T. (2022). Identifying the critical factors for sustainable marketing in the catering: the influence of big data applications, marketing innovation, and technological acceptance model factors. J. Hosp. Tour. Manag. 51, 11–21. doi:10.1016/j.jhtm.2022.02.010
Contini, G., Peruzzini, M., Bulgarelli, S., and Bosi, G. (2023). Developing key performance indicators for monitoring sustainability in the ceramic industry: the role of digitization and industry 4.0 technologies. J. Clean. Prod. 414, 137664. doi:10.1016/j.jclepro.2023.137664
Di, K. S., Chen, W. D., Zhang, X. N., Shi, Q. M., Cai, Q. L., Li, D. L., et al. (2023). Regional unevenness and synergy of carbon emission reduction in China’s green low-carbon circular economy. J. Clean. Prod. 420, 138436. doi:10.1016/j.jclepro.2023.138436
Dong, Z. F., Zhou, J., Bi, F. F., Song, Y. C., Zhang, Z. Y., Peng, C., et al. (2021). Research on synergistic policy of climate change and ecological environment protection. Chin. J. Environ. Manage. 13 (1), 25–34. doi:10.16868/j.cnki.1674-6252.2021.01.025
Duan, K., Liu, Y., Yan, C., and Huang, Y. (2023). Differences in carbon risk spillovers with green versus traditional assets: evidence from a full distributional analysis. Energy Econ., 107049. doi:10.1016/j.eneco.2023.107049
Eshet, Y. (2004). Digital literacy: a conceptual framework for survival skills in the digital era. J. Educ. Multi. Hyper. 13 (1), 93–106.
Fan, S. S., Peng, S. N., and Liu, X. X. (2021). Can smart city policy facilitate the low-carbon economy in China? A quasi-natural experiment based on pilot city. Complexity 2021, 9963404. doi:10.1155/2021/9963404
Gardner, J. (2021). Two-stage differences in differences. Economics,2207 , 05943. doi:10.48550/arXiv.2207.05943
Geng, W., Wang, X. Y., and Wang, X. Y. (2023). The impact of national big data comprehensive pilot zone on FDI inflow: from the perspective of quantity and quality. Int. Econ. Trade Res. 39 (1), 19–35. doi:10.13687/j.cnki.gjjmts.2023.01.003
Guo, B. N., Wang, Y., Zhang, H., Liang, C. Y., Feng, Y., and Hu, F. (2023). Impact of the digital economy on high-quality urban economic development: evidence from Chinese cities. Econ. Model. 120, 106194. doi:10.1016/j.econmod.2023.106194
Guo, F., Wang, J. Y., Wang, F., Kong, T., Zhang, K., and Cheng, Z. Y. (2020). Measuring China’s digital financial inclusion: index compilation and spatial characteristic. China Econ. Q. 19 (4), 1401–1418. doi:10.13821/j.cnki.ceq.2020.03.12
He, J. L., Lau, W. T., and Liu, Y. J. (2023). Innovative production efficiency in Chinese high-tech industries during the 13th five-year plan: evidence from a three-stage DEA model. Green Low-Carbon Econ., 1–12. doi:10.47852/bonviewGLCE3202910
Henry, A. (2023). An examination of the relationship between industry structure, debt and multilateral environmental agreements and environmental performance. J. Environ. Manage. 336, 117270. doi:10.1016/j.jenvman.2023.117270
Higón, D. A., Gholami, R., and Shirazi, F. (2017). ICT and environmental sustainability: a global perspective. Telematics Inf. 34 (4), 85–95. doi:10.1016/j.tele.2017.01.001
Hong, J. T. (2023). Research on the impact of digital economy on pollution and carbon reduction in Chinese cities. Anhui Jianzhu Univ. doi:10.27784/d.cnki.gahjz.2023.000286
Hu, J. (2023). Synergistic effect of pollution reduction and carbon emission mitigation in the digital economy. J. Environ. Manage. 337, 117755. doi:10.1016/j.jenvman.2023.117755
Inuwa, N., Bello, M., and Sani, M. B. (2023). Resource curse in WAIFEM member countries: an application of seemingly unrelated regression. Green Low-Carbon Econ. doi:10.47852/bonviewGLCE3202485
Ivus, O., and Boland, M. (2015). The employment and wage impact of broadband deployment in Canada. Can. J. Econ. 48 (5), 1803–1830. doi:10.1111/caje.12180
Khatami, F., Vilamová, Š., Cagno, E., Cagno, P. B., Neri, A., and Cantino, V. (2023). Efficiency of consumer behaviour and digital ecosystem in the generation of the plastic waste toward the circular economy. J. Environ. Manage. 325, 116555. doi:10.1016/j.jenvman.2022.116555
Kurniawan, T. A., Othman, M. H. D., Hwang, G. H., and Gikas, P. (2022). Unlocking digital technologies for waste recycling in Industry 4.0 era: a transformation towards a digitization-based circular economy in Indonesia. J. Clean. Prod. 357, 131911. doi:10.1016/j.jclepro.2022.131911
Lan, M. D., and Zhu, Y. K. (2023). Digital infrastructure construction, carbon total factor productivity, and carbon rebound effect. Environ. Sci. Pollut. R. 30 (38), 88968–88985. doi:10.1007/S11356-023-28738-9
Li, H. M., Wang, J., Yang, X., Wang, Y. F., and Wu, T. (2018). A holistic overview of the progress of China’s low-carbon city pilots. Sustain. Cities Soc. 42, 289–300. doi:10.1016/j.scs.2018.07.019
Li, X., Yang, C. Y., and Cao, J. H. (2017). Does Internal public opinion help alleviate haze pollution? --Analyzing the spatial spillover effect of haze pollution. Econ. D. 6, 45–57.
Li, Z. H., Bai, T. T., and Tang, C. (2022). How does the low-carbon city pilot policy affect the synergistic governance efficiency of carbon and smog? Quasi-experimental evidence from China. J. Clean. Prod. 373, 133809. doi:10.1016/j.jclepro.2022.133809
Liu, B., and Dong, Z. Q. (2020). Has the energy bias of technological progress induced carbon lock-in? Ind. Econ. Rev. 11 (4), 133–148. doi:10.14007/j.cnki.cjpl.2020.04.010
Liu, J. Y., Woodward, R. T., and Zhang, Y. J. (2021a). Has carbon emissions trading reduced PM2.5 in China? Environ. Sci. Technol. 55 (10), 6631–6643. doi:10.1021/acs.est.1c00248
Liu, X. B., Yan, X. D., Wang, W., Titheridge, H., Wang, R., and Liu, Y. (2021b). Characterizing the polycentric spatial structure of Beijing Metropolitan Region using carpooling big data. Cities 109, 103040. doi:10.1016/j.cities.2020.103040
Liu, X. Y., Duan, Z. Y., Shan, Y. L., Duan, H. Y., Wang, S., Song, J. N., et al. (2019). Low-carbon developments in northeast China: evidence from cities. Appl. Energy 236, 1019–1033. doi:10.1016/j.apenergy.2018.12.060
Lu, J., Yan, Y., and Wang, T. X. (2021). The microeconomic effects of green credit policy: from the perspective of technological innovation and resource reallocation. China Ind. Econ. 394 (1), 174–192. doi:10.19581/j.cnki.ciejournal.2021.01.010
Lu, Y. S., Li, Y., Tang, X. J., Cai, B. W., Wang, H., Liu, L., et al. (2022). STRICTs: a blockchain-enabled smart emission cap restrictive and carbon permit trading system. Appl. Eenrg. 313, 118787. doi:10.1016/j.apenergy.2022.118787
Meng, Z. Y., Li, W. B., Chen, C. F., and Guan, C. H. (2023). Carbon emission reduction effects of the digital economy: mechanisms and evidence from 282 cities in China. Land 12 (4), 773. doi:10.3390/land12040773
Mirza, F. M., and Kanwal, A. (2017). Energy consumption, carbon emissions and economic growth in Pakistan: dynamic causality analysis. Renew. Sust. Energy Rev. 72, 1233–1240. doi:10.1016/j.rser.2016.10.081
Park, Y., Meng, F. C., and Baloch, M. A. (2018). The effect of ICT, financial development, growth, and trade openness on CO2 emissions: an empirical analysis. Environ. Sci. Pollut. Res. 25, 30708–30719. doi:10.1007/s11356-018-3108-6
Pinto, J. A., Kumar, P., Alonso, M. F., Andreao, W. L., Pedruzzi, R., Espinosa, S. I., et al. (2020). Kriging method application and traffic behavior profiles from local radar network database: a proposal to support traffic solutions and air pollution control strategies. Sustain. Cities Soc. 56, 102062. doi:10.1016/j.scs.2020.102062
Plewnia, F., and Guenther, E. (2018). Mapping the sharing economy for sustainability research. Manage. Decis. 56 (3), 570–583. doi:10.1108/MD-11-2016-0766
Purohit, P., Amann, M., Kiesewetter, G., Rafaj, P., Chaturvedi, V., Dholakia, H. H., et al. (2019). Mitigation pathways towards national ambient air quality standards in India. Environ. Int. 133, 105147. doi:10.1016/j.envint.2019.105147
Rao, N. D., Kiesewetter, G., Min, J., Pachauri, S., and Wagner, F. (2021). Household contributions to and impacts from air pollution in India. Nat. Sustain. 4 (10), 859–867. doi:10.1038/s41893-021-00744-0
Ren, X., Li, Y., Qi, Y., and Duan, K. (2022). Asymmetric effects of decomposed oil-price shocks on the EU carbon market dynamics. Energy 254, 124172. doi:10.1016/j.energy.2022.124172
Ren, X. H., Li, W. C., Duan, K., and Zhang, X. R. (2023). Does climate policy uncertainty really affect corporate financialization? Environ. Dev. Sustain. 1–19. doi:10.1007/s10668-023-02905-x
Sadorsky, P. (2012). Information communication technological and electricity consumption in emerging economies. Energy Policy 48, 130–136. doi:10.1016/j.enpol.2012.04.064
Shi, D. Q., Ding, H., Wei, P., and Liu, J. J. (2018). Can smart city construction reduce environmental pollution. China Ind. Econ. 363 (6), 117–135. doi:10.19581/j.cnki.ciejournal.2018.06.008
Sun, H., and Deng, Y. Y. (2022). The effect of “Reducing Pollution and Carbon Emissions” in environmental policy: a study based on the perspective of pollution fee collection. China. Econ. Stud. 3, 115–129. doi:10.19365/j.issn1000-4181.2022.03.07
Sun, L. Y., and Abraham, S. (2021). Estimating dynamic treatment effects in event studies with heterogeneous treatment effects. J. Econ. 225 (2), 175–199. doi:10.1016/j.jeconom.2020.09.006
Tang, X. B., Zhang, Y., Cao, L. Z., Zhang, J. M., and Chen, X. H. (2022). Spatio-temporal characteristics and influencing mechanism of synergistic effect of pollution and carbon emission reduction in China. Res. Environ. Sci. 35 (10), 2252–2263. doi:10.13198/j.issn.1001-6929.2022.08.10
Wang, H., Sun, H., Xiao, H. Y., and Xin, L. (2022). Synergistic effects and paths of pollution and carbon reduction under the constraint of carbon peak goal. China Popul. Res. Environ. 32 (11), 96–108.
Wang, W., Zhang, H. G., Sun, Z. Y., Wang, L. H., Zhao, J. Y., and Wu, F. Z. (2023). Can digital policy improve corporate sustainability? Empirical evidence from China’s national comprehensive big data pilot zones. Telecommun. Policy 102617. doi:10.1016/j.telpol.2023.102617
Wei, X. H., Jiang, F., and Yang, L. S. (2023). Does digital dividend matter in China’s green low-carbon development: environmental impact assessment of the big data comprehensive pilot zones policy. Environ. Impact Asses. 101, 107143. doi:10.1016/j.eiar.2023.107143
Wen, H. W., and Zhong, Q. M. (2022). Digital infrastructure and enterprise total factor productivity--Evidence from Chinese listed companies. Soft Sci. 36 (8), 64–71. doi:10.13956/j.ss.1001-8409.2022.08.09
Wu, J. X., and Guo, Z. Y. (2016). Research on the convergence of carbon dioxide emissions in China: a continuous dynamic distribution approach. Stat. Res. 33 (1), 54–60. doi:10.19343/j.cnki.11-1302/c.2016.01.008
Xiao, Y., Wu, S., Liu, Z. Q., and Lin, H. J. (2023). Digital economy and green development: empirical evidence from China’s cities. Front. Environ. Sci. 11, 1124680. doi:10.3389/fenvs.2023.1124680
Xu, W. X., Zhou, J. P., and Liu, C. J. (2022). The impact of digital economy on urban carbon emissions: based on the analysis of spatial effects. Geogr. Res. 41 (1), 111–129.
Xue, F., and Zhou, M. L. (2022). Can the energy-consuming right transaction system improve energy utilization efficiency? China Popul. Res. Environ. 32 (1), 54–66.
Yamineva, Y., and Liu, Z. (2019). Cleaning the air, protecting the climate: policy, legal and institutional nexus to reduce black carbon emissions in China. Environ. Sci. Policy 95, 1–10. doi:10.1016/j.envsci.2019.01.016
Yang, W., and Zhang, N. (2023). Does the carbon emissions trading scheme improve carbon total factor productivity? Evidence from Chinese cities. Green Low-Carbon Econ. doi:10.47852/bonviewGLCE3202905
Yang, X., and Zhao, S. G. (2022). Low-carbon emission reduction effect of digital economy empowering regional green development. Res. Econ. Manag. 43 (12), 85–100. doi:10.13502/j.cnki.issn1000-7636.2022.12.006
Yang, X. D., Wu, H. T., Ren, S. Y., Ran, Q. Y., and Zhang, J. N. (2021). Does the development of the Internet contribute to air pollution control in China? Mechanism discussion and empirical test. Struct. Change Econ. D. 56, 207–224. doi:10.1016/j.strueco.2020.12.001
Yang, Z. B., Fan, M. T., Shao, S., and Yang, L. L. (2017). Does carbon intensity constraint policy improve industrial green production performance in China? A quasi-DID analysis. Energy Econ. 68, 271–282. doi:10.1016/j.eneco.2017.10.009
Yao, X. L., Zhang, X. L., and Guo, Z. (2020). The tug of war between local government and enterprises in reducing China’s carbon dioxide emissions intensity. Sci. Total. Environ. 710, 136140. doi:10.1016/j.scitotenv.2019.136140
Yi, L., Yang, T. T., Du, X., Yang, L., and Deng, W. (2022). Collaborative pathways of pollution reduction and carbon abatement: typical countries’ driving mechanisms and their implications for China. China Popul. Res. Environ. 32 (9), 53–65.
Yin, J. H., Zheng, M. Z., and Chen, J. (2015). The effects of environmental regulation and technical progress on CO2 Kuznets curve: an evidence from China. Energy Policy, 77, 97. doi:10.1016/j.enpol.2014.11.008
Yuan, S., and Pan, X. F. (2023). Inherent mechanism of digital technology application empowered corporate green innovation: based on resource allocation perspective. J. Environ. Manage. 345, 118841. doi:10.1016/j.jenvman.2023.118841
Zhang, H., and Shi, L. (2019). Digital economy: new driving force in the new era. J. Beijing Jiaot. Univ. Soc. Sci. Ed. 18 (2), 10–22. doi:10.16797/j.cnki.11-5224/c.20190410.003
Zhang, Y. R., and Ran, C. J. (2023). Effect of digital economy on air pollution in China? New evidence from the “national big data comprehensive pilot area” policy. Econ.Anal. Policy 79, 986–1004. doi:10.1016/j.eap.2023.07.007
Zhang, Y. Y., and Hu, S. C. (2023). Impact of digital inclusive finance on the coordinated development of economy and environment: mediating effect of innovation. Soft Sci. 37. (9), 23–30. doi:10.13956/j.ss.1001-8409.2023.09.04
Keywords: the digital economy, synergistic reduction effect, air pollutants, carbon emissions, difference-in-differences, generalized random forest
Citation: Yin B, Kuang P and Deng X (2023) The effect of synergistic pollution and carbon reduction in the digital economy: Quasi-experimental evidence from Chinese cities. Front. Energy Res. 11:1267355. doi: 10.3389/fenrg.2023.1267355
Received: 26 July 2023; Accepted: 07 November 2023;
Published: 17 November 2023.
Edited by:
Michael Carbajales-Dale, Clemson University, United StatesReviewed by:
Kun Duan, Huazhong University of Science and Technology, ChinaMahmood Ahmad, Shandong University of Technology, China
Copyright © 2023 Yin, Kuang and Deng. This is an open-access article distributed under the terms of the Creative Commons Attribution License (CC BY). The use, distribution or reproduction in other forums is permitted, provided the original author(s) and the copyright owner(s) are credited and that the original publication in this journal is cited, in accordance with accepted academic practice. No use, distribution or reproduction is permitted which does not comply with these terms.
*Correspondence: Xinhui Deng, eGhkZW5nMjAwN0AxMjYuY29t