- 1School of Science, Shandong Jianzhu University, Jinan, Shandong, China
- 2School of Management Engineering, Shandong Jianzhu University, Jinan, Shandong, China
- 3Kunming Rail Transit Group Co., Ltd., Kunming, Yunnan, China
Low carbonization of the traction system is the key to low-carbon rail transit operation, and its preliminary plan decision plays a decisive role in whether low carbon can be achieved in later stages. Therefore, how to achieve scientific decisions of energy storage traction systems in a low-carbon background is a problem that needs to be solved. The innovation of this paper is as follows: first, aiming at the reality of the rail transit energy storage traction system, a decision index system of the energy storage traction system which contains seven attributes and 18 criteria is constructed; second, aiming at the uncertainty of decision information and the decision makers’ aversion to risk, the decision model adapted to the energy storage traction system decision is constructed based on the interval Pythagorean intuitionistic fuzzy number and VIKOR model principle. The decision index system and decision model together constitute the decision framework. The case study results show that the decision index system can provide scientific guidance for the decision of the energy storage traction system, and the decision model can provide risk aversion type decision results with good robustness.
1 Introduction
On 22 September 2020, at the 75th United Nations General Assembly, President Xi Jinping proposed that China’s carbon dioxide emissions should strive to reach the peak by 2030 and strive to achieve the dual carbon goals of carbon peaking and carbon neutrality by 2060. Therefore, all fields are facing an important transformation of low-carbon development. From a global perspective, compared to other fields, such as construction and industry, low-carbon transportation development has always been a global challenge due to factors such as high resource utilization and unreasonable energy consumption. Meanwhile, due to the rapid development of urbanization in China, the rapid development of the transportation industry has brought about a sharp increase in pressure on energy conservation and emission reduction.
In the transportation industry, rail transit has been favored by government departments due to its advantages, such as large volume, fast speed, dense schedules, safety and comfort, high punctuality rate, all-weather operation, and low freight costs. Its proportion in the transportation industry is increasing day by day. The main energy consumed by rail transit is the use of electricity generated by burning coal, and its rapid growth will greatly increase carbon emissions, which is not conducive to achieving the dual carbon target of China.
The main energy consumption of rail transit comes from the traction system in the electrical system. Therefore, in the context of dual carbon targets, to achieve the low-carbon development of rail transit, the low-carbon development of the traction system is crucial, and an energy storage traction system (ESTS) is one of the important directions for the low-carbon development of the traction system.
In the decision stage of newly built rail transit projects, the design unit will provide multiple sets of ESTS plans. However, how to scientifically select suitable plans based on the characteristics of specific rail transit lines is an urgent problem that needs to be solved in the current low-carbon development process of rail transit.
1.1 Literature review on the decision index system of an energy storage traction system plan for rail transit
The energy storage devices in the ESTS can be divided into capacitor-based energy storage devices and flywheel energy storage devices (Dan et al., 2020), and capacitor-based energy storage devices are widely used. Decision-making research on the ESTS mainly focuses on the following aspects: when it is a renovation project, that is, the traction system has been determined, the main research focus is on the selection of energy storage batteries, for example, Hou Pengqi’s research on the plan of energy storage systems based on supercapacitors through ESTS simulation (Pengqi et al., 2022); when it is a new project and the energy storage system and traction system are not determined, the optimization research of the system is mainly based on simulation, for example, Dong Wenzhe’s research on the optimization operation of integrated hybrid energy storage and the RPC traction power supply system (Wenzhe et al., 2023); Li Ling studied an energy storage train with supercapacitors as the sole power source and verified the feasibility of traction system operation through simulation (Ling et al., 2018).
The aforementioned research studies are mainly based on simulation technology and scenario analysis methods to study the impact of new energy storage batteries and new ESTS techniques on rail transit operation and to select energy storage equipment or optimize system design based on the obtained characteristics of energy storage batteries and system operation.
The aforementioned research provides a good foundation for this study, but the plan of the ESTS should not only consider the characteristics of the equipment but also consider the cost of the system, as well as reliability, availability, maintainability, and safety, abbreviated as RAMS, to ensure the sustainability of rail transit projects.
Therefore, the development of ESTS plans needs to be considered from seven aspects: energy storage battery characteristics, system operation characteristics, system cost, reliability, availability, maintainability, and safety. Currently, there is a lack of relevant decision index systems to guide the plan and decision of ESTS plans.
1.2 Review of relevant literature on decision models for the ESTS in rail transit
To achieve scientific decision of ESTS plans, in addition to a scientific decision index system, it is more important to develop a scientific decision model, and the most important aspect of the scientific nature of the decision model is its suitability for the specific decision environment. The characteristics of ESTS decisions are the uncertainty of decision information and risk aversion decision.
The uncertainty of decision information mainly comes from the qualitative evaluation of the system. Due to the need for qualitative evaluation to be scored by experts, who are limited by their knowledge level and background limitations, hesitation is inevitable when facing newly developed systems, such as the ESTS. Therefore, the qualitative evaluation values provided by experts are inevitably uncertain.
Fuzzy mathematics is often used to deal with the uncertainty of decision information, such as intuitionistic fuzzy numbers (IFNs) (Kumar and Chen, 2022), interval-valued intuitionistic fuzzy numbers (IVIFNs) (Percin, 2022), interval-valued Pythagorean intuitionistic fuzzy numbers (IVPIFNs) (Peng and Yang, 2016), or directly using linguistic terms, such as the probability linguistic term set (Malik et al., 2018)[6].
Due to the large amount of engineering data involved in the ESTS plan, the evaluation value of the plan is mainly based on quantitative data, so it is best to use fuzzy mathematics. According to the data expression ways of the IFN, IVIFN, and IVPIFN, as shown in Table A1, the sum of the satisfaction degree and non-satisfaction degree of the IVPIFN can be greater than 1, and this feature enables it to better handle uncertainty. Therefore, the IVPIFN will be used in this article.
In addition, the decision of the ESTS plan belongs to the risk aversion decision because rail transit involves people’s life and property safety, so the ESTS does not have to have the best performance, but must not have accidents.
The commonly used decision models in the field of rail transit are the AHP (Dong et al., 2022), ANP (Peng et al., 2022), and TOPSIS methods (Yin et al., 2022), which pursue the maximization of utility value (refer to Table 2 for details). Therefore, there is an implicit assumption that criteria can compensate each other, and the mutual compensation between criteria will lead to risk preference decision results. For example, the evaluation values of ESTS plans X and Y on the energy storage battery characteristic criterion (marked A) and reliability criterion (marked B) are (
In the field of decision science, the VIKOR method is different from other methods. It is a risk aversion decision-making method, which is the judgment standard for the optimal plan to determine whether the degree of regret is the minimum or not. Therefore, it is more suitable for the ESTS plan decision (Kim and Ahn, 2020).
1.3 Contributions and originality
This article will construct a decision index system for the rail transit ESTS from seven aspects, energy storage battery characteristics, system operation characteristics, system cost, reliability, availability, maintainability, and safety, to make scientific decisions. On this basis, the IVPIFN is used to deal with the uncertainty of the decision information of the rail transit ESTS, and the VIKOR model is used to deal with the risk aversion problem. Based on the decision index system and decision model, a decision framework for the ESTS of rail transit is jointly constructed to achieve scientific decisions. The specific innovation points are as follows:
• The decision index system for the ESTS in rail transit is established, providing direction for scientific decision.
• The IVPIFN is used to handle uncertainty in decision information and improve the robustness of decision results.
• A decision model of the rail transit ESTS based on the VIKOR model is constructed to realize risk aversion decisions and conform to the decision habits of decision makers.
2 Research on the decision index system of an ESTS plan of rail transit
In the introduction, the ESTS needs to be considered from seven aspects: energy storage battery characteristics, system operation characteristics, system cost, reliability, availability, maintainability, and safety. However, availability is reflected through relevant data on reliability and maintainability during post-project evaluation because availability cannot be reflected during the decision stage. Therefore, this factor is not considered when constructing a decision index system. In this article, the decision index system for ESTS plans is mainly examined from energy storage battery characteristics, system operation characteristics, system cost, reliability, maintainability, and safety attributes. The specific decision criteria, criteria characteristics, and sources under each attribute are shown in Table 1. The data of alternatives on each criterion in the decision index system can be obtained through expert scoring, experimentation, or examining projects of the same type.
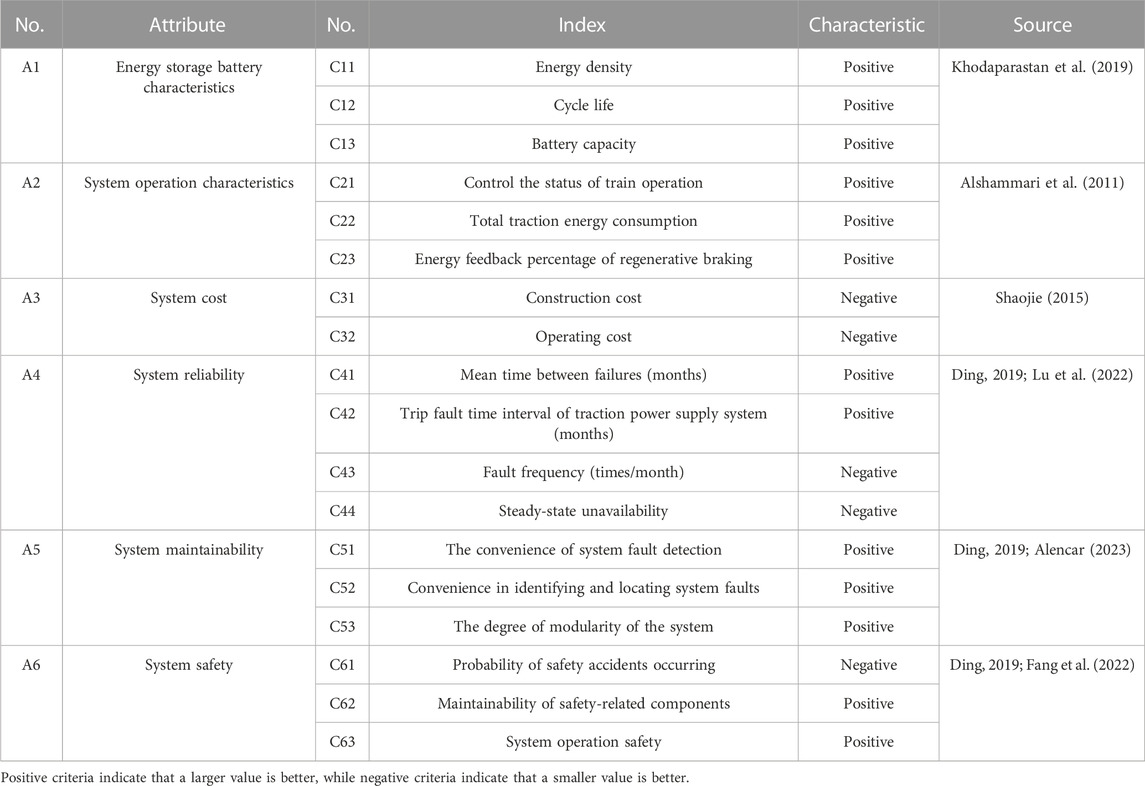
TABLE 1. Decision criterion system for the ESTS plan from the perspective of low-carbon development.
The energy storage traction system can be transformed from the determined traction system to the energy storage traction system. Since the traction system has been determined at this time, the problem of studying the energy storage battery is to consider the characteristics of the energy storage battery as an attribute when making the decision and meet the low-carbon, economical, efficiency, and sustainability requirements. To make planning decisions for energy storage traction systems from a low-carbon perspective, it is necessary to evaluate the system economy. To meet the dual carbon goals, high-performance batteries must be selected to meet the economic requirements of low-carbon development in energy storage traction systems. Otherwise, the battery life is short and the economy is poor. Therefore, the cycle life of energy storage batteries should be considered as an indicator. When the battery energy density and capacity are high, the power supply and storage efficiency of the system are higher, so energy density and battery capacity should be used as indicators. At the same time, when it belongs to a new project, the energy storage system and traction system are still uncertain, and optimization research based on simulation is needed for the system. Therefore, the operational characteristics of the system should be taken as the attribute. Because the main energy consumption of the project during system operation comes from the traction system, the total energy consumption of the system traction should be considered. In addition, the stable state of the system should be considered during operation, while the energy storage traction system should be applied in rail transit, so the control state of train operation should be considered.
To meet the requirements of low-carbon environmental protection and economy, the energy feedback percentage of regenerative braking should also be considered.
The energy storage traction system is an important heart that provides power for the normal operation of rail transit and is a core component of the entire high-speed railway system. The operation of high-speed railways is risky and accidents might occur due to the influence of the environment and operating conditions. Moreover, due to the nature of the high-speed railway system’s work and operation, which involves people’s livelihoods, the consequences and subsequent impacts of accidents are very serious and severe. According to statistics, the proportion of accidents causing rail operation interruption due to traction system failures is quite large in all types of rail transit accidents. Therefore, to avoid rail transit accidents, the reliability, maintainability, and safety of the system should be considered in the planning and decision making of the rail transit energy storage traction system.
Under the reliability attribute, its characteristic quantity is generally a quantitative indicator that reflects the overall reliability of the system. Therefore, the average number of faults in the system and the number of tripping faults in the traction power supply system should be counted. Based on this, the interval time and fault frequency should be calculated, and the reliability of the system in terms of sustainability should be represented by the interval time and fault frequency between system faults. Since the energy storage traction system provides energy for the rail transit system and the unavailability represents the ratio of the system failure time to the sum of the failure time and the normal power supply time, the unavailability of the system in the stable state is also an important indicator to measure the system reliability.
Under the maintainability attribute, it is very important to quickly check the cause of system faults when a system malfunctions. Therefore, the convenience of system fault detection, identification, and location of system faults should be considered. The modularization level of the system can make it easier to check the system’s partition. Therefore, to facilitate inspection and maintenance, it should also become a key indicator in decision making and planning.
Under the safety attribute, the probability of safety accidents occurring can directly reflect the safety level of the system, and the maintainability of safety-related components and the safety level of the system operation can indirectly reflect the safety of the system.
When evaluating a system solution, cost is an essential attribute. It can be divided into construction cost and operating cost, of which construction cost is inevitable but can be compressed through improved project plans and construction cost is a factor that must be considered before project implementation. The operating cost is directly related to the profitability and survival and development of the project, which can directly reflect the competitiveness and sustainable development ability of the project, and is conducive to resource allocation. Therefore, operating cost is also a necessary indicator to consider.
3 Research on the decision model of the ESTS plan from the perspective of low-carbon development
After determining the decision index system for the ESTS plan, it is necessary to determine the decision model based on the decision characteristics of the ESTS plan. In this paper, the IVPIFN will be used as the data expression of decision values to reduce the impact of uncertainty, and risk aversion decisions will be realized through the basic principles of the VIKOR model. In this section, the relevant theories of the IVPIFN are introduced first, and based on this, a decision model for ESTS plans will be constructed based on the basic principles of the VIKOR model and the decision characteristics of the ESTS plan.
3.1 Relevant theory of the IVPIFN
Definition 1. (Peng and Yang, 2016). Let X be a finite nonempty set, and the IVPIFN can be defined as follows:
where
Definition 2. (Peng and Yang, 2016). We assume
Definition 3. (Peng and Li, 2019). According to the Shannon entropy, the IVPIFN entropy
Definition 4. (Peng and Yang, 2016). Assuming
where
Definition 5. (Peng and Yang, 2016) For any IVPIFN
If
• When
• When
3.2 Decision model for the ESTS plan based on the IVPIFN
For the sake of expression, assuming that there are m alternative ESTS plans
3.2.1 Phase 1: establishing a decision matrix for ESTS plans based on the IVPIFN
Step 1:. Converting the quantitative decision values of the ESTS plan into the IVPIFN. The quantitative decision value of the ESTS plan can be converted into the IVPIFN decision value by the following equation:
where
Step 2:. Obtaining IVPIFN decision values for ESTS plans based on qualitative decision criteria. Experts evaluate ESTS plans based on qualitative decision criteria. First, the interval value of the satisfaction degree and the interval value of the non-satisfaction degree are determined between [0,1], respectively. The satisfaction and non-satisfaction degrees together form the IVPIFN decision value of ESTS plans on the qualitative decision criterion. When the qualitative decision criterion is negative, it should be converted into complementary values through Eq. 6.
Step 3:. Building a decision matrix for the ESTS plan. We sort the IVPIFN decision values on qualitative and quantitative decision criteria in the order of decision criteria and alternative ESTS plans. A decision matrix for ESTS plans is constructed by the following equation:
3.2.2 Phase 2: determining the weight of decision criteria for ESTS plans
Step 1:. Determining the weight of subjective decision criteria. Experts determine the importance of decision criteria between [1,10], with larger values indicating greater importance. The importance of the i-th decision criterion is marked as
where
Step 2:. Determining the weight of objective decision criteria. The objective weight of the decision criteria for the ESTS plan is calculated by using the entropy weight method, where the IVPIFN entropy on each criterion can be calculated using Eq. 7, while the objective weight calculation equation is
Step 3:. Determining the comprehensive weight of decision criteria. The comprehensive weight can be obtained through the following equation:
where
3.2.3 Phase 3: obtaining the optimal plan through the IVPIFN–VIKOR model
Step 1:. Determining the positive and negative ideal solutions according to Definition 4,
Step 2:. The group utility measure
where
Step 3:. Assuming that the optimal ESTS plan is determined based on the size of the compromise measure Q, and the minimum compromise measure value is optimal,
C1: The alternative ESTS plan
C2: The alternative ESTS plan
If one of the conditions is not met, a set of compromise solutions can be submitted, but the following conditions must be met:
• If only condition C1 is satisfied, then there is a compromise solution set {
• If only condition C2 is satisfied, then the inequality can be satisfied as
4 Case study
4.1 Data sources
The data, in this case, come from the feasibility study report, preliminary design plan, and meeting minutes of the previous plan argumentation of Kunming Metro Line 5. The IVPIFN decision value, importance score of decision attributes, and importance score of decision criteria for the alternative ESTS plans are derived from the statistical analysis of expert scoring in plan argumentation.
4.2 Case analysis
Kunming Metro Line 5 starts from Expo Park Station in the north and ends at Baofeng Village Station in the south. It runs through Panlong District, Wuhua District, Xishan District, Resort, and Guandu District, connecting tourist attractions, such as Expo Park, Yuantong Park, Cuihu Lake, and the International Convention and Exhibition Center. The total length of the line is about 26.45 km, and it is laid underground with a total of 22 stations. The construction of this rail transit project needs to reflect the concept of “ecological livability in Kunming—harmonious coexistence between humans and nature.” Therefore, from this perspective, the decision of ESTS plans is made, and the most important thing is to reflect the low-carbon nature of the traction system. Therefore, in the preliminary design stage, three ESTS design plans are proposed, labeled S1, S2, and S3 in this case.
Experts have demonstrated these three alternative ESTS plans and simulated the operation of the ESTS on rail transit. Based on the organization of the aforestated data, the decision data of these three alternatives are shown in Table 2. At the same time, experts rate the importance degree of decision attributes and criteria, and the attribute weights, criterion weights, and criterion weights considering attribute could be calculated using Eqs 12–14, the weights can been seen in Table 3. From the weight of decision attributes, it can be seen that experts have less consideration for cost, and the importance of system reliability, maintainability, and safety is slightly higher than that of energy storage battery performance and system operating characteristics. This means that under the influence of policies, it is necessary to strike a balance between system reliability, maintainability, and safety and energy storage battery performance and system operating characteristics, and cost has instead become a non-important criterion.
On this basis, the group utility measure, individual regret measure, and compromise measure of these three ESTS plans are calculated based on the IVPIFN–VIKOR model, as shown in Table 4. According to the VIKOR optimal solution judgment rules, from the compromise measure Q, S2 is optimal, but the difference between the Q value of S3 and the Q value of S2 in the second place is not greater than 0.5. Therefore, according to the judgment condition: if only condition C2 is met, then the inequality can be satisfied:
5 Discussion
The sensitivity analysis is conducted to test the robustness of the decision results. The specific steps are to adjust the parameter
To prove the progressiveness of the model, this paper uses real numbers instead of IVPIFNs as comparison scenario 1 and the TOPSIS method instead of the VIKOR model as comparison scenario 2 for the comparison experiments. The comparison experimental results are shown in Table 6. According to comparison scenario 1, after using real numbers, the compromise measure of S2 decreases due to the influence of uncertainty, but S1 and S3 do not change. This effect, which causes the difference between values to change, will lead to changes in the decision results in the VIKOR model. That is, when the two values are exactly at the boundary of C1 conditions, the change in the difference between values will lead to changes in the decision results. According to comparison scenario 2, after the TOPSIS model is adopted, S2 is the optimal solution, but this solution does not take into account the biggest weakness of alternatives, which cannot meet the needs of risk aversion decision makers. Therefore, the model proposed in this article can better solve the problem of ESTS plan decisions.
6 Conclusion
The main energy consumption of rail transit projects comes from the electrical system, and the main power consumption system is the traction system. Therefore, in the context of the dual carbon targets, if the low-carbon development of rail transit is to be achieved, the low-carbon development of the traction system is crucial. To achieve low-carbon traction systems, the ESTS is an important development direction. For new projects, the following problems must be faced when scientifically selecting an ESTS: ① lack of a scientific decision index system for ESTS plans; ② the adverse impact of uncertainty in decision information on the scientific nature of decision; and ③ the decision of the ESTS belongs to risk aversion decision.
Therefore, based on the ESTS characteristics, this article constructs a decision index system for ESTS plans. The criterion system includes six decision attributes and 18 decision criteria, among which the decision attributes are energy storage battery characteristics, system operation characteristics, system cost, reliability, maintainability, and safety. According to the characteristics of the plan decision of the ESTS, this paper uses the IVPIFN as the expression form of the decision data to reduce the adverse impact of uncertainty on the scientificity of the decision and realizes risk aversion decisions through the VIKOR model.
In this case, experts have given less consideration to cost, and the importance of system reliability, maintainability, and safety is slightly higher than that of energy storage battery performance and system operating characteristics. This means that under the influence of policies, it is necessary to strike a balance between system reliability, maintainability, safety, and energy storage battery performance and system operating characteristics, and cost becomes a non-important criterion.
The issues that need further research in this article are as follows: the correlation between decision criteria was not considered in this study, and the correlation between criteria also has a significant impact on the scientific nature of the decision. Therefore, how to scientifically measure the correlation between the ESTS decision criteria is a problem that needs to be solved.
Data availability statement
The raw data supporting the conclusion of this article will be made available by the authors, without undue reservation.
Author contributions
WX: conceptualization. BL: methodology. WW: writing—original draft. YZ: writing—original draft. All authors contributed to the article and approved the submitted version.
Conflict of interest
MT was employed by the company Kunming Rail Transit Group Co., Ltd.
The remaining authors declare that the research was conducted in the absence of any commercial or financial relationships that could be construed as a potential conflict of interest.
Publisher’s note
All claims expressed in this article are solely those of the authors and do not necessarily represent those of their affiliated organizations, or those of the publisher, the editors, and the reviewers. Any product that may be evaluated in this article, or claim that may be made by its manufacturer, is not guaranteed or endorsed by the publisher.
References
Alencar, A. R. “Reliability and maintainability strategies for repairable systems in rail transportation fleets,” in Proceedings of the 69th Annual Reliability and Maintainability Symposium (RAMS), Orlando, FL, USA, January 2023.
Alshammari, B. M., El-Kady, M. A., and Al-Turki, Y. A. (2011). Power system performance quality indices. Eur. Trans. Electr. Power 21, 1704–1710. doi:10.1002/etep.515
Dan, Z., Jianguo, J., Ying, C., Liang, Z., Sifeng, Z., Jianping, Z., et al. (2020). Research on control strategy of high speed flywheel energy storage system in metro traction power supply system. Electr. Mach. Control 24, 1–8. doi:10.15938/j.emc.2020.12.001
Ding, F. (2019). Research on reliability and risk assessment of high-speed railway traction power supply system based on operation characteristics. Chengdu, China: Southwest Jiaotong University.
Dong, S. H., Yu, F., and Wang, K. (2022). Safety evaluation of rail transit vehicle system based on improved AHP-GA. Plos One 17, e0273418. doi:10.1371/journal.pone.0273418
Fang, Y., Zhang, W. L., Hu, H., Zhou, J. Y., Xiao, D. L., and Li, S. J. (2022). Adaptive aging safety of guidance marks in rail transit connection systems based on eye movement data. Int. J. Environ. Res. Public Health 19, 725. doi:10.3390/ijerph19020725
Khodaparastan, M., Mohamed, A. A., and Brandauer, W. (2019). Recuperation of regenerative braking energy in electric rail transit systems. Ieee Trans. Intelligent Transp. Syst. 20, 2831–2847. doi:10.1109/tits.2018.2886809
Kim, J. H., and Ahn, B. S. (2020). The hierarchical VIKOR method with incomplete information: supplier selection problem. Sustainability 12, 9602. doi:10.3390/su12229602
Kumar, K., and Chen, S. M. (2022). Multiple attribute group decision making based on advanced linguistic intuitionistic fuzzy weighted averaging aggregation operator of linguistic intuitionistic fuzzy numbers. Inf. Sci. 587, 813–824. doi:10.1016/j.ins.2021.11.014
Ling, L., Yonghui, W., and Jian, L. (2018). Study of energy storage traction system based on supercapacitors. J. Qingdao Univ. Sci. Technol. Nat. Sci. Ed. 39, 99–105. doi:10.16351/j.1672-6987.2018.01.016
Lu, Q. C., Zhang, L., Xu, P. C., Cui, X., and Li, J. (2022). Modeling network vulnerability of urban rail transit under cascading failures: A coupled map lattices approach. Reliab. Eng. Syst. Saf. 221, 108320. doi:10.1016/j.ress.2022.108320
Malik, M. G. A., Bashir, Z., Rashid, T., and Ali, J. (2018). Probabilistic hesitant intuitionistic linguistic term sets in multi-attribute group decision making. Symmetry-Basel 10, 392. doi:10.3390/sym10090392
Peng, X., and Li, W. (2019). Algorithms for interval-valued pythagorean fuzzy sets in emergency decision making based on multiparametric similarity measures and WDBA. Ieee Access 7, 7419–7441. doi:10.1109/access.2018.2890097
Peng, X., and Yang, Y. (2016). Fundamental properties of interval-valued Pythagorean fuzzy aggregation operators. Int. J. Intelligent Syst. 31, 444–487. doi:10.1002/int.21790
Peng, Z. X., He, L. N., Xie, Y. S., Song, W. Y., Liu, J., Ming, X. G., et al. (2022). A Pythagorean fuzzy ANP-QFD-Grey relational analysis approach to prioritize design requirements of sustainable supply chain. J. Intelligent Fuzzy Syst. 42, 3893–3907. doi:10.3233/jifs-212131
Pengqi, H., Yang, Y., and Hequn, L. (2022). Study of energy storage system model selection and simulation based on supercapacitor. Comput. Simul. 39, 148–152+159. doi:10.3969/j.issn.1006-9348.2022.08.028
Percin, S. (2022). Circular supplier selection using interval-valued intuitionistic fuzzy sets. Environ. Dev. Sustain. 24, 5551–5581. doi:10.1007/s10668-021-01671-y
Shaojie, F. (2015). A study of Key influence factor and optimization of Four-Electrical Railway Construction Project cost. Beijing, China: University of Chinese Academy of Sciences School of Engineering Management and Information Technology.
Wenzhe, D., Siyan, Y., Zongyou, L., and Yinyu, C. (2023). Research on optimal operation of traction power supply system with integrated hybrid energy storage and RPC. Energy Storage Sci. Technol. 12, 1185–1193. doi:10.19799/j.cnki.2095-4239.2022.0664
Yin, C. F., Ji, F., Wang, L. N., Fan, Z. C., and Geng, S. (2022). Site selection framework of rail transit photovoltaic power station under interval-valued Pythagorean fuzzy environment. Energy Rep. 8, 3156–3165. doi:10.1016/j.egyr.2022.02.073
Appendix
Keywords: rail transit, energy storage traction system, plan decision, interval Pythagorean, VIKOR
Citation: Lin L, Xu W, Liu B, Wang W, Zhao Y and Tao M (2023) Research on the decision framework of an energy storage traction system plan for rail transit from the low-carbon perspective—based on the interval-value Pythagorean intuitionistic fuzzy environment. Front. Energy Res. 11:1248605. doi: 10.3389/fenrg.2023.1248605
Received: 27 June 2023; Accepted: 21 August 2023;
Published: 28 September 2023.
Edited by:
Jianli Zhou, Xinjiang University, ChinaReviewed by:
Fangtong Liu, North China Electric Power University, ChinaYiming Ke, Jinan University, China
Zhiming Lu, Lanzhou University, China
Copyright © 2023 Lin, Xu, Liu, Wang, Zhao and Tao. This is an open-access article distributed under the terms of the Creative Commons Attribution License (CC BY). The use, distribution or reproduction in other forums is permitted, provided the original author(s) and the copyright owner(s) are credited and that the original publication in this journal is cited, in accordance with accepted academic practice. No use, distribution or reproduction is permitted which does not comply with these terms.
*Correspondence: Lijun Lin, linlijunxueshu@163.com