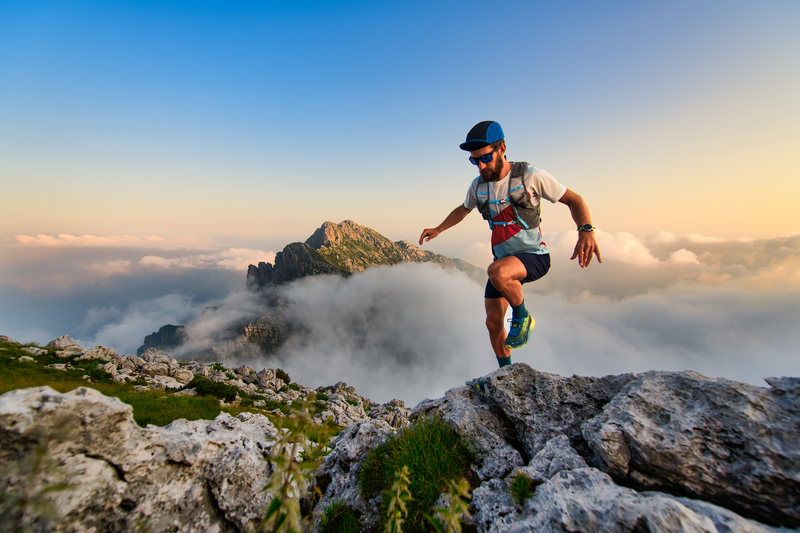
95% of researchers rate our articles as excellent or good
Learn more about the work of our research integrity team to safeguard the quality of each article we publish.
Find out more
OPINION article
Front. Energy Res. , 11 July 2023
Sec. Process and Energy Systems Engineering
Volume 11 - 2023 | https://doi.org/10.3389/fenrg.2023.1221841
This article is part of the Research Topic Advanced Technologies for Planning and Operation of Prosumer Energy Systems, volume III View all 32 articles
Due to global low-carbon and environmental concerns, modern power grids are gradually dominated by various renewable energy sources (RESs) (Yu et al., 2022). Nevertheless, large-scale grid connection of RESs significantly impacts operational stability and reliability of power grids due to intrinsic intermittence and volatility from RESs (Impram et al., 2020), resulting in remarkable renewable energy curtailments in recent years. In particular, the wind and solar power curtailment rates in the “Three-North” (i.e., northeast, north, and northwest) regions in China reached 17.1% and 10% in 2016, respectively (Zhang X. et al., 2021). Generally, the hosting capacity of renewable energy is inevitably affected by multiple factors related to power grid infrastructure planning, such as the location of RESs (Yang and Xia, 2022), inter-regional transmission capacity (Li et al., 2019), peak-valley load difference (Li et al., 2022), and extreme climates (Cao et al., 2022). Reasonable power grid infrastructure investments could increase the system flexibility and hosting capacity of renewable energy to mitigate the adverse impacts caused by high shares of grid-connected RESs. Consequently, this study aims to provide insightful perspectives and discussions on the power grid infrastructure investments for promoting the accommodation capacity of renewable energy.
The opinions of this study are twofold as follows: 1) a brief survey on prioritizing power grid infrastructure investments for upgrading the hosting capacity of RESs in China is presented, and two evaluation indicators, namely, system flexibility and RES accommodation factor, are then formulated to express the coordination degree of renewable energy installations and investment in upgrading grid infrastructure; 2) a capsule network-driven forecasting method is proposed to deduce the dynamic variation of the RES hosting capacity under a given grid infrastructure investment plan, thereby facilitating the coordinated allocation of renewable energy and grid infrastructure investments to promote the RES accommodation.
Over the past decades, China, a global leader in boosting renewable energy investments,has raised the total installed capacity of RESs by 80 times (Yang and Xia, 2022). By the end of 2022, the total installed capacity of grid-connected photovoltaic and wind generation in China reached 365 and 393 GW, respectively, ranking first among worldwide countries (The State Council of the People’s Republic of China, 2020). However, the problems with wind and solar power curtailments are severe in Northwest China. For instance, the wind (solar) power curtailment climbed to 43% (30.45%) and 38% (32.23%) in Gansu and Xinjiang provinces, China, in 2016, respectively (Zhang X. et al., 2021). The primary reasons for the massive renewable energy curtailment in China are threefold: 1) there are significant differences in resource endowments and load demand between eastern and western regions in China, and resource-rich provinces generally have an oversupply of electricity; 2) The insufficient peak shaving capacity limits the integration of large-scale RESs into the existing power grids (Wang et al., 2020); 3) the construction of the inter-regional transmission infrastructure fails to match the rapid growth of renewable energy installations (Yang et al., 2021).
In recent years, China has struggled to prioritize infrastructure investments in developing inter-regional transmission channels, enhancing thermal plant flexibility, and deploying energy storage systems. The infrastructure investments in the inter-regional ultra-high-voltage transmission networks have reached $35.4 billion from 2020 to 2022 (Ke et al., 2022). The State Grid Corporation of China (SGCC) reported that the ultra-high-voltage projects had accumulatively transmitted 28.346 million TWh of electrical power in 2022, an increase of 36.51% compared to 2020. Meanwhile, the deployment of energy storage systems, an essential measure to enhance power grid flexibility, is rapidly expanding. At the end of 2022, the newly installed capacity of energy storage projects in China has grown to 16.5 GW and increased five times over 2020 with a total investment of $393 billion (The State Council of the People’s Republic of China, 2020). Consequently, on average, the curtailment rates of wind/solar power in China decreased from 2.0%/3.5% in 2020 to 1.7%/3.2% in 2022 (Ke et al., 2022). With the accelerated implementation of renewable energy integrations, various RES plants, including offshore wind power, geothermal energy, and wave and tidal energy, will expand impressively in the coastal and rural regions to decarbonize energy systems in China (The National Development and Reform Commission, 2022).
In recent years, the extension and upgrade of existing power grids cannot match the rapid growth of grid-connected RES installations, leading to substantial curtailment of wind and solar energy (Yu et al., 2022). Hence, sufficient investments for hosting renewable energy and upgrading grid infrastructures should be equitably allocated to effectively accommodate a high share of variable RESs. In addition, the interaction of source, grid, load, and storage enables system flexibility enhancement. Based on this, coordination evaluation indicators should be presented to characterize the mutual adaptability and compatibility between renewable energy installations and power grid planning (Zhang et al., 2016).
System flexibility is a crucial coordination indicator for assessing the ability to cope with uncertainties from solar and wind generation (Impram et al., 2020). In addition to the reserve capacity of power generators and transmission lines, energy storage systems and demand response are also becoming valuable sources of system flexibility. The flexibility of the power system
where G, E, T, and D denote the set of power system flexibility sources of conventional generation units, energy storage system, transmission lines, and demand response sources, respectively;
On the other hand, the renewable energy accommodation capacity factor
where
Due to the complex non-linear temporal characteristics within power grid infrastructure investments and the accommodation capacity of renewable energy, a deep learning-driven capsule network (CapsNet) method is proposed to deduce the variation of two coordination evaluation indicators with power grid infrastructure planning. In the capsule network algorithm, a convolution structure is used to capture hierarchically temporal features of system flexibility and renewable energy accommodation from the historical infrastructure investment data (Zheng et al., 2021). Through conducting the deep learning network training, the coordination evaluation indicators on a monthly basis exhibit the dynamic variations of the renewable energy accommodation capability with various power grid infrastructure investments.
The proposed CapsNet algorithm is composed of convolution and capsule networks. The convolution and linear layers are employed to extract and integrate the global associated flexibility enhancement features of various power infrastructure investment projects (Zheng et al., 2021). Capsule networks are used to further capture the local temporal features and projects labels of various power grid infrastructure projects at adjacent time periods, including the voltage level, reserve capacity, project duration, and completion time. Furthermore, the extracted characteristics are integrated through a regression layer to predict power system flexibility under renewable energy infrastructure investments. Taking the system flexibility indicator as an example, the CapsNet-based power grid infrastructure planning deduction processes are shown as the following steps:
• Forming CapsNet structure hierarchically to develop a deep learning-driven renewable energy accommodation evaluation model with the multi-dimensional vector input dataset on the extracted features of infrastructure investment projects.
• Training the CapsNet evaluation model using historical infrastructure investment data to explore the associated temporal characteristics between multi-dimensional vectors of investment project labels and system flexibility, and a dynamic routing mechanism is used to determine optimal algorithm parameters of the capsule layer.
• Verifying the accuracy and validity of the proposed model to deduce and predict the power system flexibility capacity, including power generation reserves, energy storage systems, and available transfer capacity of transmission lines, with practical infrastructure investment data.
With the proposed CapsNet-driven RES accommodation capacity evaluation model, the infrastructure investment project labels and system reserve capacity data
During the capsule computation processes to evaluate the accommodation capacity of renewable energy, coupling coefficients between two capsule layers should be trained and determined by a dynamic routing mechanism. It is an iterative routing-by-agreement process for information extraction (Ye et al., 2022). The global associated features extracted from input data on different power infrastructure investment projects by a convolutional network are enclosed in correspondingly lower-level capsules represented by a multi-dimensional vector
where
Dynamic routing usually updates
In this paper, historical data and two coordination evaluation indicators obtained from numerous power grid infrastructure investment projects in Hunan Province, China, are introduced to demonstrate the effectiveness of the proposed CapsNet algorithm. The monthly deduction results of the Hunan provincial power grid flexibility and renewable energy accommodation capacity are shown in Figure 1.
Figure 1 shows that with the growing power grid investments, the system flexibility and renewable energy accommodation capacity factor are both increased and ranged with different types of infrastructure investment projects. In general, these two coordinated evaluation indicators increase significantly along with the completion of power grid infrastructure projects with high voltage levels, large substation, and transmission capacities, especially for ultra-high-voltage projects. Overall, with the total amount of about 43.046 billion CNY for power grid infrastructure investments in 2021–2022, the total system flexibility capacity increases by 4.183 GW and the renewable energy accommodation capacity factor can increase by 49.8%.
According to the statistical analysis of Hunan practical power infrastructure investments and renewable energy accommodation data, the key findings of this paper can be summarized as follows: 1) power grid infrastructure investments should give priority to ultra-high-voltage transmission channels and energy storage systems with optimizing their capacities and layouts to improve the accommodation capability of renewable energy sources; 2) the flexibility of thermal power plants should be improved with a high ramp rate to enhance the system accommodation capability of RESs; 3) the coordination of renewable energy and power grid infrastructure projects shall be strengthened, and the ratio of the system reserve capacity to renewable energy installation should be increased to over 60% for rational power grid infrastructure investments.
JS: writing the original draft and editing. YL and KS: conceptualization. KS, LZ, and TJ: formal analysis. TJ, MT, and QD: visualization and contributed to the discussion of the topic. All authors contributed to the article and approved the submitted version.
This work is supported by the State Grid Science and Technology Project (no. 5100-202123009A).
Authors JS and YL were employed by the State Grid Hunan Electric Power Company Limited Economic and Technical Research Institute. Authors KS and TJ were employed by the State Grid Hunan Electric Power Company Limited.
The remaining authors declare that the research was conducted in the absence of any commercial or financial relationships that could be construed as a potential conflict of interest.
All claims expressed in this article are solely those of the authors and do not necessarily represent those of their affiliated organizations, or those of the publisher, the editors, and the reviewers. Any product that may be evaluated in this article, or claim that may be made by its manufacturer, is not guaranteed or endorsed by the publisher.
Cao, Y., Zhou, B., Chung, C. Y., Shuai, Z., Hua, Z., and Sun, Y. (2022). Dynamic modelling and mutual coordination of electricity and watershed networks for spatio-temporal operational flexibility enhancement under rainy climates. IEEE Trans. Smart Grid, 1. doi:10.1109/TSG.2022.3223877
Ke, H., He, H., Zhou, Y., and Li, Z. (2022). Analysis of renewable energy and development path of new power system under China’s “dual-carbon” situation. 2022 IEEE Sustain. Power Energy Conf. (iSPEC). doi:10.1109/iSPEC54162.2022.10033067
Impram, S., Nese, S., and Oral, B. (2020). Challenges of renewable energy penetration on power system flexibility: A survey. Energy Strategy Rev.31, 100539. doi:10.1016/j.esr.2020.100539
Li, G., Li, G., and Zhou, M. (2019). Model and application of renewable energy accommodation capacity calculation considering utilization level of inter-provincial tie-line. Prot. Control Mod. Power Syst.4, 1. doi:10.1186/s41601-019-0115-7
Li, X., Liu, C., Sheng, K., Wen, B., Xie, H., Zhao, W., et al. (2022). A multi-level investment demand assessment model for renewable-dominated power system planning. Front. Energy Res.9. doi:10.3389/fenrg.2021.807590
The National Development, and Reform Commission (2022). The national development and Reform commission and the national energy administration answer reporters' questions on the 14th five-year plan for renewable energy development (in Chinese) [online]. Available online at: https://www.ndrc.gov.cn/xxgk/jd/jd/202207/t20220704_1330048.html.
The State Council of the People's Republic of China (2020). The National Energy Administration released the development of renewable energy in 2022 and introduced the progress of work related to the improvement of renewable energy green power certificate system and other information (in Chinese) [Online]. Available online at: http://www.gov.cn/xinwen/2023-02/14/content_5741481.htm.
Wang, Y., Lou, S., Wu, Y., and Wang, S. (2020). Flexible operation of retrofitted coal-fired power plants to reduce wind curtailment considering thermal energy storage. IEEE Trans. Power Syst.35, 1178–1187. doi:10.1109/TPWRS.2019.2940725
Wu, Y., Li, X., Zhang, L., Liu, C., Zhao, W., and Zhang, T. (2022). Machine learning-driven deduction prediction methodology for power grid infrastructure investment and planning. Front. Energy Res.10. doi:10.3389/fenrg.2022.893492
Yang, X., Chai, G., Liu, X., Xu, M., and Guo, Q. (2021). Storage-transmission joint planning method to deal with insufficient flexibility and transmission congestion. Front. Energy Res.8. doi:10.3389/fenrg.2020.612909
Yang, Y., and Xia, S. (2022). China must balance renewable energy sites. New York, N.Y.: Science. doi:10.1126/science.adf3720
Ye, X., Yan, J., Wang, Y., Wang, J., and Geng, Y. (2022). A novel U-Net and capsule network for few-shot high-voltage circuit breaker mechanical fault diagnosis. Measurement199, 111527. doi:10.1016/j.measurement.2022.111527
Yu, Y., Zhao, H., Zeng, Y., Chen, F., Yang, D., Wang, B., et al. (2022). Accommodation capacity evaluation of renewable energy in power systems considering peak and frequency regulation. Front. Energy Res.10. doi:10.3389/fenrg.2022.1067884
Zhang, N., Hu, Z., Shen, B., Dang, S., Zhang, J., and Zhou, Y. (2016). A source–grid–load coordinated power planning model considering the integration of wind power generation. Appl. Energy168, 13–24. doi:10.1016/j.apenergy.2016.01.086
Zhang, X., Wang, J., Cao, Z., Shen, S., Meng, S., Fan, J., et al. (2021a). What is driving the remarkable decline of wind and solar power curtailment in China? Evidence from China and four typical provinces. Renew. Energy174, 31–42. doi:10.1016/J.RENENE.2021.04.043
Zhang, Z., Wang, W., Zhang, X., Ma, X., Chu, Y., Wang, K., et al. (2021b). Renewable energy installation planning method based on power system carrying capacity index. Power Syst. Technol. doi:10.13335/j.1000-3673.pst.2019.1541
Keywords: accommodation capacity, deep learning, infrastructure investment, power grid planning, renewable energy
Citation: Sha J, Liu Y, Sheng K, Zhang L, Jiang T, Tan M and Dou Q (2023) Opinions on power grid infrastructure investments for renewable energy accommodation in China. Front. Energy Res. 11:1221841. doi: 10.3389/fenrg.2023.1221841
Received: 13 May 2023; Accepted: 23 June 2023;
Published: 11 July 2023.
Edited by:
Xueqian Fu, China Agricultural University, ChinaReviewed by:
Xiaokang Liu, Polytechnic University of Milan, ItalyCopyright © 2023 Sha, Liu, Sheng, Zhang, Jiang, Tan and Dou. This is an open-access article distributed under the terms of the Creative Commons Attribution License (CC BY). The use, distribution or reproduction in other forums is permitted, provided the original author(s) and the copyright owner(s) are credited and that the original publication in this journal is cited, in accordance with accepted academic practice. No use, distribution or reproduction is permitted which does not comply with these terms.
*Correspondence: Yuyou Liu, MTE5NTYxMDUyNEBxcS5jb20=; Man Tan, dGFubWFuMTAwOEBobnUuZWR1LmNu
Disclaimer: All claims expressed in this article are solely those of the authors and do not necessarily represent those of their affiliated organizations, or those of the publisher, the editors and the reviewers. Any product that may be evaluated in this article or claim that may be made by its manufacturer is not guaranteed or endorsed by the publisher.
Research integrity at Frontiers
Learn more about the work of our research integrity team to safeguard the quality of each article we publish.