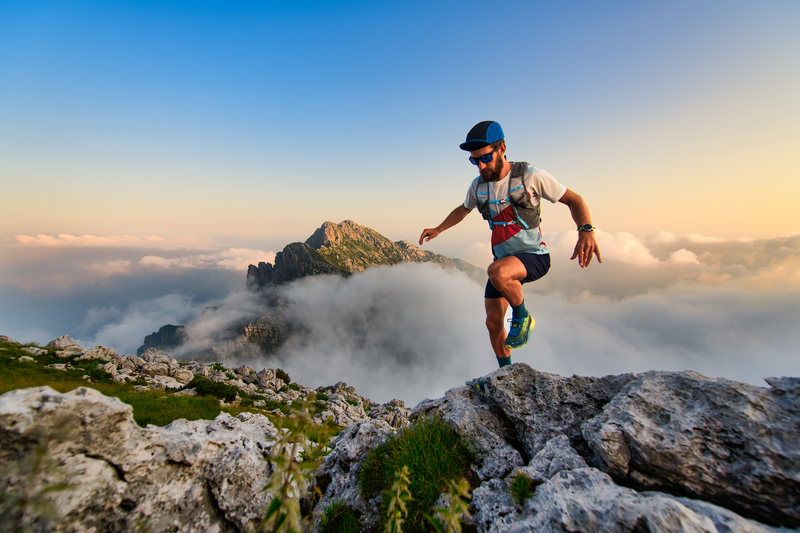
95% of researchers rate our articles as excellent or good
Learn more about the work of our research integrity team to safeguard the quality of each article we publish.
Find out more
ORIGINAL RESEARCH article
Front. Energy Res. , 27 November 2023
Sec. Sustainable Energy Systems
Volume 11 - 2023 | https://doi.org/10.3389/fenrg.2023.1211179
This article is part of the Research Topic New Trends in Energy-Economy-Environment System View all 22 articles
Global value chains (GVCs) involve production and distribution cooperation as well as pollution redistribution. With the increasing frequency of global trade activities and the growing problem of environmental pollution, the impact of GVC participation on environmental pollution has attracted considerable academic attention. In this study, a dynamic panel Gaussian mixture model was constructed using data from several databases, including Chinese industrial enterprise pollution emission and customs data from 2005 to 2013. We found that the degree of GVC participation of enterprises and pollution emission show a non-linear inverted U-shaped relationship. This indicates that the pollution emissions of enterprises increased and then decreased as the degree of GVC participation increased. We constructed separate models for the effects of output upstreamness and input downstreamness on pollution emissions and found that upstreamness is negatively related to pollution emissions, with the upstream economic components of GVC (such as technology research and development, international marketing, and key component manufacturing) producing emission reduction effects. Downstreamness, on the other hand, is positively correlated with emissions, which means that the downstream economic components of the GVC (e.g., processing, assembly, and production of non-critical components) will increase emissions. Based on the second finding, we proposed that the inverted U-shaped relationship is the result of the combined influence of upstream and downstream participation and confirmed this hypothesis by constructing a multiple mediating effects model. This study will help enterprises consciously implement environmental protection measures and broaden the scope of research on the environmental impact of GVCs, providing a broader segmented research perspective on the degree of participation in GVCs and the environmental performance of exporting enterprises, and guiding governments to formulate more effective industrial policies for foreign trade and promote better environmental performance of enterprises.
From the creation of the United Nations Framework Convention on Climate Change to the implementation of the Kyoto Protocol, and finally to the entry into force of the Paris Agreement, the world’s determination to combat climate change is evident. With the development of communication and transportation technologies, the division of labor patterns in global value chains (GVCs) has brought opportunities and challenges for international emission reduction. Developed countries participate in the division of labor in GVCs through high-end segments, such as R&D and design, while allocating manufacturing segments, such as processing and assembly, to developing countries. Simultaneously, developing countries can learn from the advanced technology and management experience of developed countries to improve their energy efficiency and reduce pollution emissions. The relationship between GVC integration and a country’s pollution emissions has been debated, and the Chinese experience provides a wealth of evidence for answering this question. First, China is the largest developing country in terms of global trade, and has become increasingly embedded in GVCs. China’s GVC participation increased from 29.55% in 1990 to 44.49% in 2018 (Shao, 2021a). Second, the role of Chinese companies in GVCs is gradually changing from being downstream production manufacturers to being upstream suppliers, with an increasing international division of labor (Sudani, 2020), which has an important impact on the environmental effects of China’s participation in GVCs. Finally, as environmental issues, such as PM2.5, become more acute, Chinese residents are becoming more concerned about environmental pollution. The Chinese government attaches great importance to pollution prevention and control and has pledged to achieve carbon peaking by 2030 and carbon neutrality by 2060. Research into the impact of Chinese companies’ involvement in GVCs on pollution emissions is important for environmental governance and the double carbon goal and is globally representative.
Since its reform and especially its accession to the World Trade Organization in late 2001, China’s aggressive integration into the GVC production system has been accompanied by an increasing deterioration of the domestic ecological environment, while creating export and growth miracles. Studies have shown that 17%–36% of China’s emissions of the four major air pollutants are brought about by China’s embedding in GVC downstream production and manufacturing with low-end elements. However, along with the deepening of GVC embedding, the production links undertaken by China in the GVC and the division of the labor position it occupies have changed significantly, which has important implications for the environmental effects of China’s participation in the GVC. Therefore, against the background of the evolving international labor divisions and the increasingly severe domestic environmental situation in China, this article examines how the global division of the labor system affects polluting behavior from the perspective of different ways of participation in the global division of labor. This has important theoretical and practical value for China to improve its domestic environment by adjusting its GVC embedding strategy in the new international division of labor.
After constructing a dynamic panel Gaussian mixture model (GMM), this study finds that the degree of enterprises’ GVC participation and pollution emissions show a non-linear inverted U-shaped relationship. This indicates that the pollution emissions of enterprises increased and then decreased with an increase in GVC participation. Second, we constructed separate models for the effects of output upstreamness and input downstreamness on pollution emissions and found that upstreamness is negatively related to pollution emissions, with the upstream economic components of GVC (such as technology research and development, international marketing, and key component manufacturing) producing emission reduction effects. The downstream degree is positively correlated with pollution emissions, which means that the downstream economic components of the GVC (assembly and noncritical component production) increase pollution emissions. Third, based on the second finding, we hypothesized that the cause of the inverted U-shaped relationship is the result of the joint influence of enterprises’ upstream and downstream participation, and constructed a multiple mediating effect model. This model showed that output upstreamness and input downstreamness have multiple parallel mediating effects on GVC participation. This finding suggests that the inverted U-shaped relationship generated by GVC participation is the sum of the environmental impacts of a firm’s output upstreamness and input downstreamness. These findings will help governments formulate more precise industrial and environmental policies that meet their own development needs and achieve a balance between trade development and environmental protection. In summary, this study provides a reference for developing economies worldwide to choose their mode and path of participation in GVC, which has important implications for the governance of global pollution.
The “pollution paradise hypothesis” was developed by academics as early as 1994. This hypothesis is primarily used to describe the fact that energy-intensive, heavily polluting industries involved in the GVC process move to developing economies embedded at the lower end of the spectrum. Participation in GVCs implies that international production fragmentation enables the transfer of environmental pollution between countries. By transferring highly polluting downstream production and manufacturing to developing countries through a “keep the core, outsource the rest” strategy, developed countries can improve their own environmental quality, but may exacerbate environmental pollution in developing countries. Upstream economies in GVCs mainly engage in technology development and international marketing or hold key high value-added component production, with capital and humans being the main factors of production consumed. Downstream economies, on the other hand, are involved in assembly production and manufacturing of low-value-added components that are easily substitutable, consuming more traditional energy sources, thereby increasing their pollution emissions (Shao and Sudanese, 2019). found that the increased level of trade openness of economies with lower GVC positions worsened their polluting terms of trade instead of expanding the scale of trade. Whereas GVC location and participation positively affect pollution emissions (Pan et al., 2020), Pan (2017) found that GVC participation caused higher levels of implied carbon emissions based on the MRIO model combined with Koopman value-added decomposition (Pan, 2017).
In contrast to the “pollution paradise hypothesis,” some scholars have proposed the “environmental gain hypothesis” (pollution halo hypothesis), which states that GVC is embedded in developing economies to absorb advanced foreign technology through a push-back mechanism, and that pollution emissions are curbed in the production chain. The GVC embeds a pushback mechanism that allows developing economies to absorb advanced foreign technologies and curb pollutant emissions at the production level. As trade barriers, such as pollution tariffs, restrict the entry of highly polluting and emitting products (Wang et al., 2021), developing economies need to move up the value chain to ensure that they can export while improving their trade structure and environmental performance. In addition, this move meets internationally prevailing environmental standards in the short term, allowing developing countries to set more stringent pollution emission targets, thus creating a virtuous cycle for controlling pollution emissions. Thus, in the process of moving up in the GVC position, the structure of production, level of technology, scale of the situation, laws, and regulations have an important influence on pollution emissions.
In addition to the positive “pollution paradise hypothesis” and the negative “pollution halo hypothesis,” many scholars have found a non-linear effect of the GVC status on pollution emissions (Shi and Li, 2020; Yan et al., 2020; Liu et al., 2018) introduced corporate value chain participation based on the Copland and Taylor model and found that GVC embedding, especially forward embedding, significantly reduced the overall trade-implied carbon emissions of secondary industries. The process of GVC embedding may result in fluctuating growth in industrial pollution emissions and changing conditions in pollution emissions and trade. Li et al. (2022) found a U-shaped relationship between pollution emissions and forward-linked GVC embedding in Chinese industry. (Ju and Yu, 2015) used non-linear regression to find an inverted U-shaped relationship between GVC location and carbon emissions and proposed a mechanism for GVC location upgrading to reduce pollution emissions. However, there is a threshold effect of GVC location enhancement on the export reduction of pollution emissions (Yan et al., 2020). The same threshold effect of GVC participation on pollution trading conditions exists, showing a U-shaped structure with technological change (Lv and Lv, 2019; Lv and Zhang, 2021).
However, there are some unresolved issues in the current studies: on the one hand, the findings of the pollution paradise hypothesis, the pollution halo hypothesis, the U-shaped relationship and the inverted U-shaped relationship are contradictory, and the reason for this disagreement is the lack of convincing micro data studies. In addition, pollution emissions are highly correlated with energy consumption, and it is very difficult to obtain micro energy data of Chinese enterprises, which makes the control variables in the model inadequate. On the other hand, most studies only stay at the level of conclusion, without exploring the formation mechanism of the correlation between GVC and pollution emissions, failing to explain the structure and interrelationship within the system, and can only roughly understand the relationship between the degree of GVC participation and the environmental performance of enterprises, which is insufficient to guide policy formulation. The environmental regulatory policy framework designed on this basis undoubtedly has room for optimization in terms of relevance and effectiveness. Therefore, this study uses more convincing micro data and refines key control variables, such as energy consumption, using a variety of methods to construct a more robust dynamic panel GMM to further investigate the causes of the non-linear relationship and refine and extend the existing mechanism analysis of the issue.
First, the data for measuring enterprise GVC participation, output upstreamness, input downstreamness, and pollution emission indices include the China Industrial Enterprise Pollution Emission Database, China Industrial Enterprise Census Database, and China Customs Database from 2005 to 2013. The corresponding industry data were obtained from the World Input-Output Database (WIOD), the China Regional Input-Output Tables 2012, and the China Input-Output Tables 2012. In this study, we referred to mainstream processing models and matched the Chinese industrial enterprise database, Chinese customs database, and Chinese industrial enterprise pollution emission database to obtain basic operation, import and export, and pollution emission data of each enterprise, which were used to calculate the upstream and downstream link participation and pollution emission index of each enterprise (Sudani and Yang, 2021). The second panel, which measures energy intensity data for prefecture-level cities, used data from the China DMSP/OLS (Defense Meteorological Satellite Program/Operational Linescan System) stable night lighting data from 2005 to 2013, the National Statistical Yearbook, China Energy Statistical Yearbook, China City Statistical Yearbook, statistical bulletins, and geographic information data for selected cities (WU et al., 2014).
Pollution emission data for enterprises were obtained from the pollution emission database of Chinese industrial enterprises from 2005 to 2013 from the National Bureau of Statistics. In this study, we follow the processing methods of Shao (Shao, 2021b; Sudani and Yang, 2021) and selected industrial wastewater, chemical oxygen demand emissions, ammonia nitrogen, sulfur dioxide, nitrogen oxides, soot, and industrial dust emissions with relatively high data quality to construct a comprehensive index system. First, pollution emission indicators were standardized to eliminate the differences in the magnitudes of different pollutants. The specific formula is as follows:
where
Further, the entropy value was calculated using the following equation:
Finally, the corresponding index weights were obtained from the hierarchical analysis method and summed with weights to obtain the Synthetic pollution index (SPI). The larger the value of this index, the higher is the pollution intensity of the enterprise. In practice, the logarithm of the value of this index after adding one is used as the explanatory variable in this study and is expressed as
In the benchmark regression of the non-linear relationship, the explanatory variable is the participation in GVCs (GVC) of firms. This study constructs the most comprehensive method for calculating GVC participation by referring to the method proposed by Ge and Chen (2022) and assuming a 5% overseas import component in intermediate goods purchased domestically by firms.
Where IIP, EXP, and YD denote the import, export, and domestic sales scales of the enterprise, respectively, and subscripts p and g refer to processing and general trade, respectively. The enterprises’ import and export data can be obtained from sources such as industrial enterprises and customs data, as described in the previous section. For enterprises with sales below the declared export delivery value in the raw data, it was assumed that there was no domestic purchase of components. Where the foreign value added is greater than the total exports, the domestic value added is set to zero and the foreign value added rate is set to one; that is, GVC participation is set to one, where
In the multiple mediating effects model, the mediating variables are output upstreamness (OU) and input downstreamness (ID). Based on the formulae proposed by Sudanese et al. (2020); Yuan et al. (2021); Yuan et al. (2021), this study optimizes the original treatment of national industry data as an approximation of the indirect value added export data of enterprises to the industry-average data of the provinces in which the enterprises are located to reflect inter-provincial differences. Consequently, the OU and ID calculation formulae are as follows:
where i, j, and t represent the company code, industry code, and year, respectively; p, g, and m represent processing, general, and mixed trade, respectively. In this study, the intermediate products imported by the processing trade are regarded as all used for processing trade exports. Among intermediate products imported through general trade, the export ratio was the same as that of domestic and general trade exports. Therefore,
This study first describes industrial OU and ID heat distributions. As shown in Figure 2, 30 of the 36 industries achieved an increase in OU, while industries such as coal mining and washing, non-ferrous metal smelting and rolling processing, and electricity and heat production and supply declined upstream. Most of the declining industries are high-energy-consuming and high-polluting industries, and the technology level is generally low; thus, the OU space is more limited. At the same time, 28 industries reduced their ID, while industries such as petroleum processing, coking and nuclear fuel processing, waste resources, and waste material recycling processing failed to significantly reduce their ID, indicating that the above industries have low-end lock-ins, key technologies, and raw materials are restricted, and there is high pollution, high energy consumption, and low value-added features.
The production phase of an industrial enterprise occurs when pollutant emissions are concentrated, primarily through the use of fossil energy sources. Improving energy efficiency and using more efficient carbon-based energy sources such as natural gas can significantly reduce pollutant emissions at the end of production. Therefore, this article uses the Chinese DMSP/OLS steady night lighting data from 2005 to 2013 to derive an energy intensity indicator for each administrative unit at each prefecture level as the average local energy consumption level, which is then matched with data from enterprises with micro-energy consumption data to obtain the average energy consumption share of all local enterprises. Figure 3 shows the trend in energy consumption intensity for over 280 prefecture-level administrative areas between 2005 and 2013. Based on the amount of coal, oil, and natural gas used by industrial enterprises, converted into standard coal, and processed with the average energy consumption share of prefecture-level municipalities and the single-value moving time-series smoothing method for enterprises with missing or non-existent data, more complete energy intensity data for industrial enterprises from 2005 to 2013 were obtained. The share of clean energy (SCE) was obtained by dividing the energy data by the share of natural gas (in standard coal). The variable takes values in the range of 0–1, with data closer to 1 indicating that the enterprise has a higher share of clean energy and should, in theory, produce lower pollutant emissions.
In addition to SCE, the control variables include total enterprise assets (CAPI), total profits (PROFIT), total enterprise exports and imports (total export–import volume, TEIV), and the number of workers (LABOR). Additionally, the dummy variable for ownership structure (OWERSHIP) was set according to the economic attributes of the enterprises, where public enterprises (including state-owned and collective enterprises) were set as 1, private enterprises as 2 and foreign enterprises as 3. Descriptive statistics are shown in Table 1.
The dynamic panel generalized method of moments regression (DPGMR) is an econometric method for estimating models of panel data that address the problem of endogeneity in panel data. In the dynamic panel GMM regressions, lagged variables were used as instrumental variables to control for endogeneity. This approach is based on assumptions about the plausibility and validity of the instrumental variables. The advantage of DPGMR is that it can control for both individual fixed effects and endogeneity, and can better deal with the dynamic characteristics present in the panel data. First, a multicollinearity test was conducted and the results showed that the correlation coefficients among the variables were all less than 0.7, the variance inflation factors were all less than 2, and the mean value was 1.28, indicating that there was no serious multicollinearity problem for all variables within the constructed model. To investigate the impact of GVC participation on the environmental performance of enterprises, this study follows the model framework constructed by benchmark regression and introduces the primary and secondary terms of GVC participation in the model. In systematic GMM, the endogenous explanatory variable lagged in the original model is used as its own instrumental variable, which significantly improves the estimation efficiency and robustness of the results obtained. The baseline model is established using the following set of equations:
where i and t denote the corresponding market micro-entity and time, respectively; j denotes the prefecture-level administrative area where the sample is located; k denotes the industry to which it belongs;
The results of fixed-effects and systematic GMM regression conducted on the original model are shown in Table 2. From this result, it can be concluded that after systematic GMM estimation, the characteristics and significance of the coefficients of the GVC and GVC quadratic terms are consistent with the fixed-effects model. In addition, the systematic GMM regression was subjected to AR(1), AR(2), and Sargan tests. First, the p-value of the AR(1) test was 0.004 and that of AR(2) was 0.197. This result indicates that there is autocorrelation in the first-order residuals, while there is no autocorrelation in the second-order residuals, indicating that the results of the systematic generalized moment estimation are reliable. Second, to ensure the validity of the instrumental variables, the Sargan test was conducted, which showed a test p-value of 0.21. This result indicates acceptance of the original hypothesis: the instrumental variables are reasonable and valid, and there is no over-identification problem. Drawing an inverted U-shaped conclusion from the coefficient characteristics alone is not sufficient. This study further uses the testing scheme proposed by Lind and Mehlum (2010) to perform a U-test on the baseline regression findings. Based on the test results, the GVC extreme value point of 0.644095 is within the range of values that can be taken and can reject the original hypothesis at the 1% statistical level. Meanwhile, the slope takes the value [0.2675233, −0.1478243], and there are negative values in this interval; thus, an inverted U-shaped relationship can be identified.
The regression results show that the estimated coefficient of the primary term of the GVC is significantly positive, whereas the estimated coefficient of its squared term is significantly negative, indicating that the impact of the GVC on the pollution emissions of enterprises shows a clear inverted U-shaped trend. In other words, at the beginning of a firm’s participation in a GVC, as the level of participation deepens, the characteristics of the firm’s pollution emissions generally tend to expand. However, as the level of GVC participation continues to rise, when it breaks through the extreme value, the increase in the level of GVC participation will have a dampening effect on the enterprise’s pollution emissions, and further deepening of international production capacity cooperation will give positive local environmental improvement effects. This shows that at a time when globalization is advancing with difficulty, it is important to continue to maintain an open, cooperative, and inclusive stance, to accumulate momentum in industrial development, and to participate in the process of integrating and improving GVC, to ultimately achieve a win-win situation in terms of economic benefits and environmental emission reduction. This aligns with the Chinese government’s approach of dual domestic and international recycling. In addition, after increasing the energy consumption constraint on the proportion of clean energy, the coefficient of
A moderating effect is the change in the degree or direction of a variable’s influence on the relationship between two other variables. In statistics and social science research, the moderation effect is used to explain how a variable changes or modulates the relationship between independent and dependent variables. In this study, we argue that differential financing constraints are prevalent among firms’ participation in GVC, which affects a number of areas, such as technology purchase and production equipment replacement, causing fluctuations in the environmental performance impact exhibited by firms when participating in GVC. For this reason, this study introduces the financing constraint (
Table 3 presents the regression results of the moderating effects. The signs of the coefficients of the GVC and GVC quadratic terms are opposite to the signs of the coefficients of the interaction terms
To verify the robustness of the model estimation results, industrial wastewater (IWit), chemical oxygen demand (CODit), ammonia nitrogen (ANit), sulfur dioxide (SDit), and soot (FDit) emissions were estimated as separate explanatory variables. The estimation results are shown in Table 4. The separate regressions with pollutants were consistent with the findings of the baseline regression model. This result once again validates the statement that “corporate GVC participation has an inverted U-shaped effect on corporate pollution emissions,” which is also applicable to a specific pollutant of importance.
The highly uneven level of economic development in China necessitates a location-based heterogeneity analysis. We grouped enterprises by region into East, Central, West and North, South. In order to eliminate the problem of sample decline, we construct regional dummy variables
The model we designed to study regional heterogeneity is shown in Table 5. The coefficient of the primary term of GVC participation is significantly positive with the cross product of each regional dummy variable, while the cross product of the secondary term with the regional dummy variable is significantly negative. This indicates that there is a significant inverted U-shaped effect of the level of GVC participation on the emission intensity of enterprises, and this finding is still very robust after differentiating regions. Further observation of the distribution characteristics of the inflection points reveals that the extreme points of the three curves in the east, central and west are 0.642156863, 0.616915423 and 0.63592233, respectively, which indicates that the threshold for reversing the trend of GVC participation level on enterprises’ pollution emissions is the highest in the eastern region, which requires local enterprises to reach the GVC participation level criterion of 0.642156863. This may be due to the fact that the eastern region was the first part of the Chinese mainland to participate in GVCs, and thus has accumulated more low-end industrial sectors over time, and the aggregate effect is more clearly characterized. The higher GVC reversal threshold in the west compared to the central part is mostly attributed to the weakest economic base of the western region, which makes it differ from the central and eastern parts in terms of introducing mid- and high-end GVC participation firms. And the attractiveness of low-end participation degree enterprises is limited by geographical transportation and other factors, also weaker than the central region. Thus, there is a more obvious behavior of local administration to relax the constraints of environmental protection to attract investment in the west in the past. In addition, the coefficient values under the North-South distinction happen to be the same (but the remaining variables and standard deviations, etc. are significantly different), making the extreme value points of GVC under the South-North distinction the same, and there is no more significant difference between the South and North in terms of GVC influencing pollution emissions of exporting enterprises.
Different enterprise sizes will affect the level of technology and the potential for various environmental investments, and thus should also be grouped according to differences in enterprise size to verify the reliability of the conclusions of the trend relationship in this paper. To this end, this paper classifies enterprises into three categories according to their asset size: small, medium and large enterprises. The regression results are shown in Table 6, and the variable superscripts small, medium, and large denote the corresponding small, medium, and large firms, respectively. After grouping the samples, the primary and secondary terms of GVC participation for small, medium, and large enterprises still maintain the correlation relationship with the composite indicator of pollution emission intensity, and the significance and coefficient sign are as expected. Even when distinguished into small, medium and large enterprises, there is still a nonlinear inverted U-shaped relationship between emissions and GVC participation at different scales. In addition, examining the distribution of inflection points for enterprises of different sizes shows that the extreme value points for small, medium and large enterprises are 0.6300, 0.6322 and 0.6650, respectively, and the threshold for large enterprises to enter the downward range of pollution emissions is the highest, while the threshold levels for small and medium enterprises are approximately the same. Large enterprises with larger initial investment in fixed assets may have more obvious obstacles in introducing and absorbing new equipment. Blindly promoting SME consolidation may not produce more desirable environmental performance results.
Different ownership structures also have some impact on the model estimation findings. Therefore, this paper further classifies the sample according to public (state-owned + collective), private and foreign enterprises, and the obtained estimation results are presented in Table 7. After distinguishing different economic sectors and ownership structures, the primary and secondary terms of corporate GVC participation still maintain the expected correlations, and the signs of the estimated coefficients are all consistent with the regression results of the nonlinear relationship, which indicates that the trend findings of this paper are still robust after distinguishing different corporate ownership structures. Further examining the performance of the extremes under different economic structures, it can be seen that the curves for the public, private, and foreign economies achieve inflection points of 0.64893617, 0.636138614, and 0.649038462, respectively, in terms of GVC participation. The relatively rigid and less market-oriented public economy and the foreign economy have a more backward GVC inflection point. The public economy has a larger legacy of backward production capacity, while the foreign economy sees China as a low-cost production base in the early stages of investment, and the industrial investment made has a greater intention of low-end lock-in. The private economy, on the other hand, pays more attention to the level of GVC participation based on its disadvantaged position in market competition, and faces greater pressure from environmental regulations and a lower threshold for the inflection point of pollution decline.
By constructing a non-linear regression model, it is clear that the relationship between China’s participation in GVC and firms’ pollution emissions has an inverted U-shape. We speculate that the possible reason for the inverted U-shape is the different patterns of enterprise embedding in the GVC–the different emissions impacts produced by upstream and downstream embedding. In the early stage, the effect of low-end locking, path dependence and factor input expansion is more obvious, and enterprises are in the downstream position among GVCs; in the later stage, with a certain extent of technology accumulation and industrial upgrading, the original GVC division of labor position has changed, and enterprises are more involved in the upstream high value-added aspects of GVCs, and the key to achieving improved environmental performance and lower emissions is whether they can achieve progress in the GVC division of labor. Therefore, we constructed a fixed effects model that combines OU and ID to influence firms’ pollution emissions. To strengthen the energy constraint, this chapter constructs the variable
The model is set up as with the following set of equations:
In Table 8, the results obtained in column (1) show that in this scenario, the participation of the upstream link of the enterprise remains significantly negatively correlated with its integrated pollution emission level, and each unit increase in the participation level of the upstream link of the enterprise decreases its pollution emission intensity by 0.653 basis points. In contrast, the share of newly added nonclean energy sources was positively correlated with pollution emissions, which is consistent with theoretical expectations. In addition, we constructed the cross-product term
From the regression results, it can be seen that OU has a significant negative correlation effect on the pollution emission intensity of enterprises, and ID has a significant positive correlation effect on the pollution emission intensity of enterprises, regardless of whether factors such as the local energy consumption intensity of enterprises and other control variables are introduced. For developing economies, the higher the economic share of upstream industries, the higher the level of OU, and the overall environmental performance will significantly improve. Enterprises with higher participation in the upstream part of the global value chain have a more concentrated accumulation of knowledge, technology, capital, and market information, which are all advanced factors of production in the market operation, thus creating strong conditions for them to undertake the high value-added parts of the entire industry chain (such as R&D, after-sales service, brand marketing, and core parts supply), enabling them to take the lead in the distribution of benefits in the global industry chain. The company has also begun to focus on social perception and corporate responsibility to create a good market reputation and social recognition, which in turn will jointly contribute to a gradual reduction in the company’s pollution emission level. The higher the level of ID, the greater the proportion of the downstream economy of the enterprise and the increase in pollutant emissions after the enterprise participates in the GVC.
Thus, OU has a negative effect on corporate pollution, whereas ID has a positive effect on corporate pollution. If both are the mediating variables of the GVC, an inverted U-shaped relationship is formed by combining these two effects. As there are two mediating variables, there may be multiple mediating effects. Figure 5 shows a schematic of a parallel multiple mediating model with i mediating variables (where i is greater than 1), where X is the independent variable, Y is the dependent variable, and M is the corresponding multiple mediating variable. The figure shows that there is no correlation between the multiple parallel mediating variables.
According to the relevant settings of the parallel multiple mediated effects model, the designed model is shown below, and the corresponding regression results are presented in Table 9.
First, we examine the significance levels of the primary and squared terms of the independent variable
FIGURE 6. Impact share of the output upstreamness and input downstreamness multiple mediation effects.
The empirical results indicate that the upstream and downstream participation paths produce opposite directions of corporate environmental performance, and that the upstream and downstream participation levels change dynamically during the GVC participation process. Eventually, the trade-off between the two opposite roles creates an inverted U-shaped trend, as shown in Figure 7. Therefore, the partially mediated transmissions in the two directions jointly formed an inverted U-shaped curve. When
The findings of previous researches have mostly focused on the environmental impact of GVC participation in general, lacking a more convincing data base. This is mainly due to the difficulty of obtaining data on micro enterprises. In addition, the calculation of GVC-related indicators is complicated, and the required data are scattered in databases such as industrial enterprise surveys and customs data, which makes it more difficult to study the issue from a micro perspective. In addition, at the mechanism level, the discussion of existing studies is very weak, and the conclusions obtained from studies conducted only from the perspective of GVC participation undoubtedly lack precise policy implementation. Unlike the existing “pollution halo” and “pollution paradise” hypotheses, we started with the trend between pollution emissions and GVC participation, and suggested a non-linear inverted U-shaped relationship between the two. In order to test this hypothesis, we increased the size of the micro-sample (most of the previous studies used less accurate macro data) and used a more accurate model to construct a dynamic panel GMM with a non-linear relationship, and obtained the “inverted U-shaped” conclusion. Based on this, we further analyzed the models and found that the OU of a firm represented by upstream link embedding and the ID of a firm represented by downstream link embedding have opposite environmental effects. Enterprise OU is negatively correlated with pollution, whereas enterprise ID is positively correlated with pollution, and the two form a parallel multiple mediation effect with GVC participation; the inverted U-shaped relationship of GVC is the result of the joint influence of OU and ID environmental effects.
In terms of policy recommendations, the government should first fully respect international rules and raise implementation standards at the level of environmental regulation and administration. For the heterogeneous characteristics of environmental performance exhibited by enterprises of different scales and industries, the government should avoid the same level of policy intervention; second, the government should increase the financial governance budget, innovate diversified market-incentivized environmental regulation policies, increase efficient publicity and delivery, and improve public participation in the implementation of environmental regulations; third, the government and the business community should try to break the barriers between research institutions and market players, and actively promote the extension of the latest research results to the foreign trade sector. Fourth, the government and the business sector should be adept at developing new technological innovations and R&D in the export sector, focusing on developing new technology, participating in the process of formulating market frontiers and emerging patent rules, and improving the level of enterprise OU; Fifth, enterprises should compensate for their shortcomings in the existing mature technology areas; sixth, the government should fully mobilize the financial system to help capacity and deepen financial support in the field of science and technology. Through various ways to alleviate the external financing difficulties of export enterprises and enhance their domestic industrial value added. Seventh, the government and enterprises should make joint efforts to optimize and adjust the export structure, focusing on stabilizing the supply chain, optimizing the export market structure, and continuously expanding new business models in the field of foreign trade; eighth, the government should pay attention to the use of various multilateral platforms and trade agreement tools, comply with various international trade standards and rules. Finally, the government and the business community should optimize and upgrade the factor input areas, change the “demographic dividend” into a “talent dividend” and continuously improve the domestic business environment.
This research can also be better optimized in terms of data, which is the prospective direction for future research: on the one hand, the underlying data are relatively old and the research findings have a certain lag. Although the data used in this paper are the mainstream micro data in today’s academia, and representative research results such as Economic Research and China’s Industrial Economy were conducted on this basis after 2020. However, in the post-epidemic era, there has been a more obvious adjustment in the representation of globalization, leading to a more drastic trend of change in the division of labor system of global value chains, which will bring more research possibilities from both theoretical model construction and empirical verification. However, due to the difficulty of data acquisition and other objective factors, there is still much room for improvement in the research content of this article. In particular, at a time when the external environment is changing drastically and the internal economic structure is constantly transforming, the differences in enterprise heterogeneity factors will be more obvious, which will lead to new changes and trends in the participation of micro market players in the GVC division of labor system. The screening and examination of these new factors need to be supported by more timely and reliable micro data, which is the direction to be improved in the next stage of this issue. Whether it is the key explanatory variables or general control variables, this paper has deepened on the basis of the previous arithmetic logic. However, due to the influence of different data bases and domestic and international statistical standards, some industry indicators are replaced by approximate values, and the corresponding data of regional energy sources stop at the level of prefecture-level administrative districts. Industry data are more desirable, but extremely difficult to obtain. In the subsequent research, this paper will further optimize the calculation field of indicators, and use more complex approximations based on the existing data resources to overcome the existing data shortcomings and better explain the realistic economic operation state.
The original contributions presented in the study are included in the article/Supplementary Material, further inquiries can be directed to the corresponding author.
Conceptualization, XS and BW; Methodology, XS and BW; Software, XS; validation, XS and BW; formal analysis, XS; investigation, XS; resources, XS; data organization, XS and HH; writing-original draft preparation, XS and BW; writing review and editing, XS, BW, and XS; visualization and XS; visualization, XS; supervision, BW and XS; project management, BW; funding acquisition, XS. All authors contributed to the article and approved the submitted version.
The authors declare that the research was conducted in the absence of any commercial or financial relationships that could be construed as a potential conflict of interest.
All claims expressed in this article are solely those of the authors and do not necessarily represent those of their affiliated organizations, or those of the publisher, the editors and the reviewers. Any product that may be evaluated in this article, or claim that may be made by its manufacturer, is not guaranteed or endorsed by the publisher.
Ge, Y., and Chen, Y. (2022). Participation in global production networks and value chain upgrading in East Asia: an empirical analysis based on the embeddedness model. J. Nanjing Univ. Finance Econ. 6, 86–97.
Ju, J., and Yu, X. (2015). Productivity, profitability, production and export structures along the value chain in China. J. Comp. Econ. 43 (1), 33–54. doi:10.1016/j.jce.2014.11.008
Li, Q., Wu, S., and Li, S. (2022). Weighing China's embodied CO2 emissions and value added under global value chains: trends, characteristics, and paths. J. Environ. Manag. 316, 115302. doi:10.1016/j.jenvman.2022.115302
Liu, H., Li, J., Long, H., Li, Z., and Le, C. (2018). Promoting energy and environmental efficiency within a positive feedback loop: insights from global value chain. Energy Policy 121, 175–184. doi:10.1016/j.enpol.2018.06.024
Lv, C., Suo, Qi, and Yang, H. (2021). Is the economic gap between "north-south" and "east-west" regions large? --A comparative study of regional economic disparities and their influencing factors in China. Res. Quantitative Tech. Econ. 38 (9), 80–97. doi:10.13653/j.cnki.jqte.2021.09.005
Lv, Y., and Lv, Y. (2019). Analysis of environmental effects of China's participation in global value chains. China Population-Resources Environ. 29 (7), 91–100.
Lv, Y., and Zhang, H. (2021). Will breaking market segmentation promote emission reductions by Chinese firms? Financ. Stud. 47 (9), 4–18. doi:10.16538/j.cnki.jfe.20210707.201
Pan, A. (2017). The impact of global value chain division of labor on China's implied carbon emissions from foreign trade. Int. Econ. Trade Explor. 33 (3), 14–26. doi:10.13687/j.cnki.gjjmts.2017.03.002
Pan, A., Hao, R., and Xue, H. (2020). Impact of global value chain division of labor on trade and environment imbalance. J. Zhongnan Univ. Econ. Law 6, 143–153. doi:10.19639/j.cnki.issn1003-5230.2020.0058
Shao, C.-Y. (2021a). How import competition affects firms' environmental performance - a quasi-natural experiment from China's WTO accession. Econ. Q. 21 (5), 1615–1638. doi:10.13821/j.cnki.ceq.2021.05.06
Shao, C.-Y. (2021b). How import competition affects firms' environmental performance - a quasi-natural experiment from China's WTO accession. Econ. Q. 21 (5), 1615–1638. doi:10.13821/j.cnki.ceq.2021.05.06
Shao, C. Y., and Sudanese, L. (2019). Industrial agglomeration and firms' export domestic value-added:The localization path of GVC upgrading. Manag. World 35 (8), 9–29. doi:10.19744/j.cnki.11-1235/f.2019.0102
Shi, D., and Li, S. (2020). Emissions trading system and energy utilization efficiency: measurement and empirical evidence for prefecture-level and above cities. China Ind. Econ. 9, 5–23. doi:10.19581/j.cnki.ciejournal.2020.09.001
Sudanese, H., Sheng, B., Shao, C. Y., and Chen, S. (2020). The interactive effects of global value chains, localized industrial agglomeration and firm productivity. Econ. Res. 55 (3), 100–115.
Sudani, H. (2020). How does global value chain embeddedness affect the environmental performance of Chinese firms? Nankai Econ. Res. 5, 66–86. doi:10.14116/j.nkes.2020.05.004
Sudani, H., and Yang, Q. (2021). Intermediate goods trade liberalization, environmental regulation and corporate emission reduction. J. Zhongnan Univ. Econ. Law 5, 89–100+160. doi:10.19639/j.cnki.issn1003-5230.2021.0057
Wang, S., He, Y., and Song, M. (2021). Global value chains, technological progress, and environmental pollution: inequality towards developing countries. J. Environ. Manag. 277, 110999. doi:10.1016/j.jenvman.2020.110999
Wu, J., Yan, N., and Wu Jiansheng, N. Y. (2014). Dynamics of energy consumption in Chinese prefecture-level cities from 1995-2009 based on DMSP/OLS nighttime lighting data. Geogr. Res. 33 (4), 625–634. doi:10.11821/dlyj201404003
Yan, Y., Wang, R., Zheng, X., and Zhao, Z. (2020). Carbon endowment and trade-embodied carbon emissions in global value chains: evidence from China. Appl. Appl. Energy 277, 115592. doi:10.1016/j.apenergy.2020.115592
Keywords: GVC participation path, output upstreamness, input downstreamness, non-linear relationships, multiple mediating effects
Citation: Wang B, Shao X and Xu H (2023) Non-linear effects of corporate global value chain participation on pollution emissions: evidence from China. Front. Energy Res. 11:1211179. doi: 10.3389/fenrg.2023.1211179
Received: 24 April 2023; Accepted: 31 October 2023;
Published: 27 November 2023.
Edited by:
Michael Carbajales-Dale, Clemson University, United StatesCopyright © 2023 Wang, Shao and Xu. This is an open-access article distributed under the terms of the Creative Commons Attribution License (CC BY). The use, distribution or reproduction in other forums is permitted, provided the original author(s) and the copyright owner(s) are credited and that the original publication in this journal is cited, in accordance with accepted academic practice. No use, distribution or reproduction is permitted which does not comply with these terms.
*Correspondence: Honghai Xu, eHVob25naGFpQHNjcnNzLm5ldA==
Disclaimer: All claims expressed in this article are solely those of the authors and do not necessarily represent those of their affiliated organizations, or those of the publisher, the editors and the reviewers. Any product that may be evaluated in this article or claim that may be made by its manufacturer is not guaranteed or endorsed by the publisher.
Research integrity at Frontiers
Learn more about the work of our research integrity team to safeguard the quality of each article we publish.