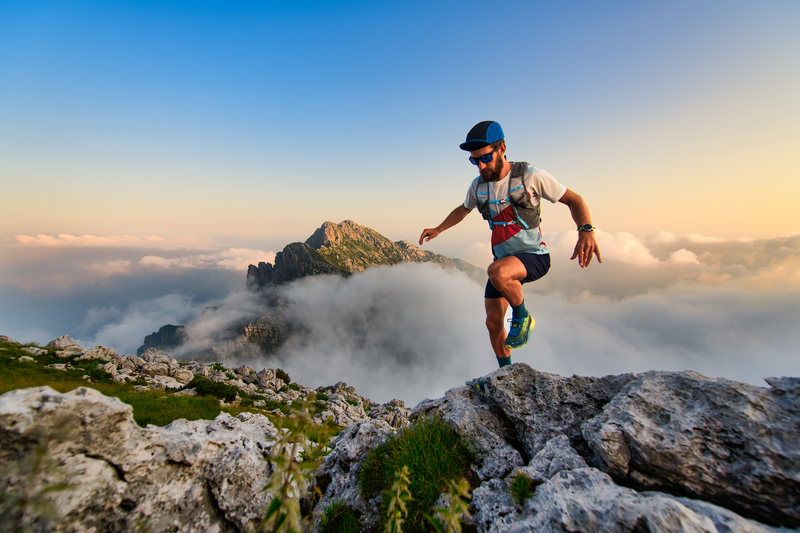
94% of researchers rate our articles as excellent or good
Learn more about the work of our research integrity team to safeguard the quality of each article we publish.
Find out more
ORIGINAL RESEARCH article
Front. Energy Res. , 06 June 2023
Sec. Smart Grids
Volume 11 - 2023 | https://doi.org/10.3389/fenrg.2023.1203633
This article is part of the Research Topic Recent Advances in Flexibility Analysis and Regulation Technology of Clean Energy System View all 7 articles
In the process of continuous promotion in the green electricity market, various risks have emerged due to the inherent uncertainty and complex trading environment of the market. Evaluating and controlling the risks through a multi-dimensional evaluation is crucial for the smooth and healthy development of the green electricity market. This work proposes a risk assessment method for the green electricity market based on the multi-dimensional cloud model. First, the comprehensive indicator evaluation system is established for both pre-transaction and post-transaction dimensions by analyzing the operating mechanism of the green electricity market. Second, each dimensional cloud is formed by the evaluation clouds of all indicator levels. The comprehensive risk level cloud is generated based on the indicator values and the evaluation clouds of each indicator. Finally, the sources of market risk are identified, and the corresponding risk management methods are proposed on the basis of the risk evaluation level and membership degree of each indicator. The case study results demonstrate that the proposed method can accurately evaluate and reduce the potential risks existing in the green electricity market through the two-stage detection mechanism.
The increasing proportion of renewable energy in the current power system is a key factor in achieving the goal of low-carbon transformation in the electric power industry (Wang et al, 2014). One of the challenges in promoting the development of renewable energy generation is in how to create a market-oriented approach to encourage investment and innovation (Chen et al, 2020; Hu et al, 2022). Hence, various countries have established corresponding market mechanisms based on the environmental value of renewable energy to address this issue (Zhao et al, 2022; Wȩ;dzik et al, 2017; Linnerud et al, 2017). The establishment of a green power trading market in China has been initiated to sell green electricity in an integrated way in which green electricity trading certificates are bundled with electricity (Wang et al, 2021). However, with the development of the green electricity market in China, there are some potential risks that have to be addressed, such as the performance risk caused by the uncertainty of green electricity (Gatzert et al, 2016) and inherent market risks. Therefore, it is essential to assess and control the potential risks of the green electricity market.
Assessing market risks of human and random factors involves evaluating both inherent uncertainties and market forces which are crucial issues in evaluating market risks (Bukarica et al, 2016; Bao et al, 2021). Currently, market risk assessment is carried out through the construction of the relevant indicator system and evaluation model. It has studied the method for evaluating price risk in the electric power market (Deng et al, 2020), identified and controlled market power in the spot market (Dong et al, 2021), and evaluated the power market from a holistic perspective (Tayşi̇ et al, 2015; Lian et al, 2018). However, the current indicator system does not consider the adaptability of the green electricity market, and there is limited research on indicators that address the uncertainty and unique characteristics of the green electricity market.
Many studies have conducted comprehensive evaluations of the indicator system. The retail credit risk was evaluated through the fuzzy AHP method (De et al, 2019; Yu et al, 2021), but it cannot adapt to the changes of the market environment. The operation and development of renewable energy have been evaluated through the system dynamics method (Liu et al, 2017) and entropy weight method (He et al, 2018). Due to the problem of uncertainty and fuzziness in risk assessment in the green energy market (Song et al, 2019), the cloud model is based on the analysis of qualitative concepts using probability theory and fuzzy set theory (Li et al, 2009). In order to realize the rational transformation of qualitative and quantitative problems directly, the model consists of three eigenvalues that describe the uncertainty and fuzziness of evaluation. The cloud model is widely used in all kinds of evaluation, such as the electricity market (Yan et al, 2017; Peng et al, 2018; Wang et al, 2020). In the green electricity market, since it involves multiple processes such as quotation, transaction, and settlement, the risk should be evaluated from multiple dimensions (Yu et al, 2009). Therefore, the multi-dimensional cloud model is proposed to evaluate the green electricity market risk on the basis of the cloud model.
In summary, there are many evaluation indicator systems and models for the electric power market but fewer risk evaluation methods for the green electricity market from multiple dimensions. Hence, this work proposes a risk evaluation system for the green electricity market based on the multi-dimensional cloud model and establishes the corresponding risk prevention and control mechanism from the two dimensions of pre-transaction and post-transaction. The contributions of this work are as follows:
(1) In response to the uncertainty of risk sources in the current green electricity market, this work conducts a mechanism analysis from the operation processes of the green electricity market and establishes a pre- and post-market indicator evaluation system as the basis for the quantitative risk assessment of the green electricity market.
(2) Due to difficulty in qualitative assessment based on numerical values of multi-dimensional indicators, this work proposes the multi-dimensional cloud model to establish a comprehensive evaluation cloud of risk levels and assesses the risks of green electricity objectively.
(3) Based on the risks in the current green electricity market and the results of risk analysis, this work establishes corresponding risk control mechanisms from the pre- and post-market dimensions.
The remaining contents of the article are as follows: Section 2 introduces the method and model of risk evaluation in the green electricity market. Section 3 proposes a two-stage market risk management process. Section 4 analyzes the result through simulation. Section 5 summarizes the whole article and gives the conclusion.
The goal of operating a green electricity market is to maintain safe and effective competition in the market and to sustainably reduce the uncertainties associated with various factors. The risk may come from the uncertainty of renewable energy, abuse of market power, and other such reasons in the green electricity market, which may cause price manipulation, imbalance between supply and demand, and profit damage. Therefore, the work establishes a comprehensive risk evaluation model. According to the market-related data, the market risk assessment level and indicator radar chart are obtained. On the basis of risk assessment, risk management is carried out from the two dimensions of pre- and post-transaction. The overall structure of this work is illustrated in Figure 1.
This work combines the risk assessment of the power market and identification of market power abuse risk. Considering the uncertainty of renewable energy, the unique pricing mechanism, and other characteristics of the green electricity market, the existing indicator system should be changed or built. During the process of risk assessment of the green electricity market, considering the categories of risks and their specific dimensions, risks can be divided into two dimensions of pre-transaction and post-transaction evaluations. The pre-transaction dimension mainly assesses the risks for the next transaction based on the declaration data, such as the impact of market forces on prices. The post-transaction dimension mainly evaluates the problems existing in the transaction results, final actual performance, and safety of the entire transaction process as the supervision of market management and platform security. The schematic diagram of the indicator system design is shown in Figure 2.
Pre-transaction indicators are mainly used for risk review of the entity behavior during the transaction declaration stage, such as determining whether there is a situation of market disruption. Due to the uncertainty of green electricity, the reasonable declaration of electricity cannot be simply judged by capacity, so the historical performance rate is introduced to judge the final performance data of the declared quantity. At the same time, based on the declaration data, the market structure is preliminarily evaluated to determine the monopoly situation in the market.
The Herfindahl–Hirschman Index (HHI) coefficient is used to measure the concentration of the market, which is measured by the square of the proportion of each entity in the market to the size of the market. It is considered to be judged from both the sale and purchase sides. The maximum value of the two sides is used as the HHI indicator of the whole market. It can be calculated as follows:
where
The supply–demand matching ratio (SDR) is used to describe the ratio between the production and demand of green electricity. The balance between the supply and demand is used to assess the stability of a transaction. The supply–demand matching ratio is calculated as follows:
where
The green electricity price manipulation rate (PMR) is used to describe the ratio of the entity quotation that differs from the benchmark green electricity price by more than the benchmark deviation rate. It is calculated from both the sale and purchase sides, and the maximum value is taken. The indicator is evaluated to prevent the green electricity generation side from inflating prices on the one hand and green electricity consumption side from undercutting prices and hindering the development of renewable energy on the other. It is calculated as follows:
where
The performance settlement rate (PSR) is used to describe the expected performance of the market, which is a judgment on the uncertainty of green electricity production and consumption. It is calculated by the weighted accumulation of each entity declaration and historical performance rate for all entities. It is calculated as follows:
where
The abnormal quotation rate (AQR) is used to describe the abnormal non-profit quotation of the entities in order to determine whether the quotation information has been tampered with or if there are any other information security problems. It is calculated by using the rate of entities whose quotations exceed 20% of the benchmark power price and is as follows:
where
The post-transaction indicators are mainly the assessment of the exceptional clearing, actual performance, market regulation, and information security in the whole process of a transaction.
The Lerner indicator has often been used to judge market power, measured as the percentage markup of price above the marginal cost. It is calculated by the deviation rate of the clearing price from the marginal cost and uses it to set the quotation threshold for the next trading. Due to the low marginal cost of green electricity, the original calculation has low practicality. The current pricing basis for green electricity is divided into electric power price and environmental premium. Therefore, the benchmark price of renewable energy is used instead of the marginal cost. Meanwhile, deviation may have a positive or negative value, which is the result of the game between green electricity companies and power consumption users. Therefore, the absolute value has to be taken when calculating the deviation between the clearing price and benchmark power price, and the modified Lerner indicator is as follows:
where
The market liquidity indicator (MLI) is used to describe the current participation of market participants. If the market is not active enough, it indicates that the market is at risk of shrinking in trading size. It is calculated by the ratio of the current market volume to the historical average.
where
The actual performance rate (APR) is used to describe the ratio of total production to total consumption in the settlement process.
The information security level (ISL) is used to describe whether the entity information and transaction information are stolen or tampered with in the transaction process beyond reasonable disclosure. The ISL is assessed as a subjective indicator and is scored through problems arising in the transaction process.
The regulatory level (RL) is used to judge whether the potential problems in the market should be timely regulated. It is also a subjective indicator to evaluate the supervision level of managers and is scored through the problems in the transaction.
As seen in the previous section 2.1.1 and section 2.1.2, indicators are set from two dimensions: pre-transaction and post-transaction. According to the level of division of each indicator, the evaluation grade of each indicator is fuzzy divided. For the evaluation of the risk level of each dimension, four levels—1, 2, 3, and 4—are set. The specific division method is shown in Table 1. For the objective evaluation indicators, some indicators are based on the existing evaluation division methods, such as the HHI and Lerner index. The risk division of other indicators is based on the current existing index system and the characteristics and empirical values of the green electricity market operation. For the subjective evaluation indicators, according to the risk level, the corresponding scoring is carried out to divide the risk level.
In the actual evaluation process, the general evaluation model usually deals with information between language and real or fuzzy numbers that is difficult to overcome distortion, loss, and other problems in information processing. The cloud model is an uncertainty model that realizes the transformation from qualitative to quantitative problems on the basis of the fuzzy theory and probability theory, which can effectively solve the uncertainty problem. Specifically, the cloud model can express qualitative concepts with three digital features, Ex, En, and He, which are shown in Figure 3. It can transform qualitative concepts into quantitative values through the corresponding cloud generator to realize the organic combination of ambiguity and randomness in the concept. Among them, the expected value Ex represents the center of the spatial distribution of cloud droplets in the discussion domain, which represents the concept of each risk level in the green electricity market. Entropy describes the uncertainty of the attribute concept, which is determined by the discreteness and fuzziness of the indicator data and classification boundaries. The hyper-entropy He is the entropy of entropy, which objectively describes the degree of correlation between fuzziness and randomness of each risk evaluation indicator and is calculated as follows:
In the application of multi-indicator evaluation, the multi-dimensional cloud model avoids the problem of determining the weight of each indicator in most models by comparing the similarity between each cloud that improves the efficiency and accuracy of evaluation. The multi-dimensional cloud model is an expansion based on the cloud model. Let U be an m-dimensional universe and T be the qualitative concept on U, the elements of U (x1, x, …, xm) the membership degree μ for T is a random number with a stable tendency. The membership degree is calculated as follows:
In this work, the membership of the risk level is calculated by the cloud generation algorithm. The cloud generation algorithm is called a cloud generator and includes a forward cloud generator and reverse cloud generator. The forward cloud generator is also called the basic cloud generator. For the m-dimensional normal cloud, the algorithm is as follows:
1) Generate k m-dimensional random numbers with
2) Generate k m-dimensional random numbers with
3) Calculate the degree of membership.
There are many pre-transaction and post-transaction indicators in the green electricity market that it is difficult to reasonably establish the weight of each part when conducting risk assessment. Therefore, it is more appropriate to use the multi-dimensional cloud model for evaluation. The risk assessment process of the green electricity market is based on the multi-dimensional cloud model, which is summarized in Section 2.2. First, the cloud expansion is carried out at the comments level of every indicator in each dimension, and the data on the market transaction operation are collected. Second, the forward cloud generator is used to generate enough cloud droplets to establish the multi-dimensional comprehensive cloud model describing the sample attributes. Third, the sample cloud droplets generated by the forward cloud generator are taken as the input. The evaluation results are finally obtained by using the multi-dimensional cloud model evaluation method. The specific process is as follows, and the flowchart is shown in Figure 4.
1) The indicator level evaluation cloud corresponding to level j is generated according to indicator i, and its three characteristic quantities are calculated as follows:
where
2) In each dimension, m-dimensional random numbers
3) The membership of the sample data is calculated with m-dimensional random numbers.
4) The risk level evaluation is calculated in dimension m.
5) The random number in (2) is taken 2,000 times, and the operations (2)–(4) are cycled. The average of the three eigenvalues is calculated as the final result. Among these, the final Ex is taken as the result value of its evaluation, and the ratio of the mean value
After the multi-dimensional cloud comprehensive level evaluation, the current market operation is subdivided according to the evaluation level results. On the result of cloud evaluation, the membership degree of each indicator level is divided according to the indicator value. The m-dimensional normal random number is generated according to the entropy and hyper-entropy in the cloud model. The cloud correlation degree between the value to be evaluated and each indicator level is calculated. According to the principle of maximum membership, the rank of each indicator is determined. Then, the corresponding prevention and control are carried out according to the specific indicators which is shown in the Figure 5.
There are two kinds of problems in the price declared by the entities: the abnormal price of the individual entity and string bidding of multiple entities. For the first kind of problem, if caused by factors such as information tampering, then the entity has to declare again. If caused by its own factors, the reference price will be modified. For the other kind of problem, if it is the risk of multiple entities making a serial bid together, the reference price has to be modified by the quotation. The reference quotation is mainly based on the construction input cost and operating cost of the enterprise, and the calculation method is as follows:
where
There are also two types of problems with the price declared by an entity. One is that the market share of the entity may be too high, which tends to make the market structure monopolistic, and the other is that the current performance rate of the entity is low, which may cause a default risk in the later stage of transaction settlement. The first problem requires the entity to make a separate quotation in order to reduce the impact of its own behavior on the market price. The second type of problem is modified according to the average level of its historical power generation, considering the performance rate.
The default of the entity may have a serious impact on the operation of the whole power grid. The prevention of the default risk of the entity is mainly from two aspects: calculating the current performance of the entity and specifying the declarable scale of its transaction in the next stage according to the performance rate of the entity. A total of four levels are set according to the performance rate, and each level corresponds to a different proportion of declaration and margin. The scale of declaration in the next stage is calculated by the current trading volume and proportion of declaration. The admission level design is shown in Table 2.
In this work, the green electricity market risk assessment is carried out in two scenarios, namely, pre-transaction and post-transaction, by setting two market transaction data for comparison. In this case, five electricity buyers and seven power generation entities are set up to conduct transactions through a centralized bidding to unify the clearing price.
Two sets of data are set before the transaction, where one group of healthy market entities declare normally but with a market structure biased to monopoly and the other group has market power and multiple entities deliberately bid up the electricity price, and there is an abnormal quotation situation of one entity. The specific declaration data are given in the Table 3 and Table 4.
The evaluation cloud of each indicator level is calculated according to the value of the pre-transaction indicator and the grade range. At the same time, the top and bottom of some indicators are changed, considering the availability of some ranges. The characteristic values of the cloud model of each grade are shown in Table 5. According to the declaration data, the indicator evaluation values of control sets A and B are generated which is shown in Table 6.
According to the evaluation grade, five types of indicators are fused to generate the multi-dimensional indicator evaluation comprehensive cloud. Then, the normal multi-dimensional random number is generated, and the membership degree is calculated by combining the current evaluation indicator value with the generated random number, which forms a group of cloud droplets. In order to reduce the randomness of random number generation, the average membership is obtained by performing the generation for 10,000 times. The expected mean value of control set A is 2.9366, and the credible factor obtained by the ratio of expected variance to the mean value is 0.0638. The expected average value of control set B is 3.9324, and the expected credibility factor is 0.0453. Thus, the evaluation cloud of each of its control group is constructed, as shown in Figure 6.
Among them, dark blue, red, yellow, and purple are the risk-level standard cloud maps, while green and light blue are the evaluation cloud maps of control sets A and B, respectively. According to the current evaluation cloud correlation degree, it can be seen that control set A is of level Ⅲ slight risk and control set B is riskless. By further analyzing control set A by indicator, the grade evaluation of each indicator can be obtained according to the principle of maximum membership, as shown in Figure 7. It can be seen that there are two serious level I risks, one important level II risk, and two minor level III risks in the declaration data of control set A. In the market structure, there is a level I risk that is biased toward monopoly, and there is a level I risk where multiple entities bid up the electricity price. Therefore, in the process of pre-site risk prevention and control, it is necessary to modify the quotation of the entity by reference and make separate price declarations for the entity with large market declarations, such as the G1 entity. In addition, there is a level Ⅱ risk of abnormal quotation of the entity, so it is necessary to verify the quotation before the transaction for the entity and judge whether the information system of the market platform is affected by an attack that leads to abnormal data. At the same time, there is an asymmetry between supply and demand in the declaration-matching rate, and the overall performance rate is low, so it is necessary to control the access trading volume before trading. The membership degree is calculated by combining the current evaluation indicator value with the generated random number, thus forming a group of cloud droplets..
After pre-transaction risk assessment and identification, the transaction result is generated, and the uniform clearing price is specified according to the principle of maximum social welfare. In the process of evaluation, the evaluation cloud of each level is also generated for the post-transaction indicator, and the comprehensive cloud is generated by collection. According to the random number generated by the comprehensive evaluation cloud and the post-transaction data (Table 7), the membership degree calculation is carried out to generate cloud droplets. It is assumed that the abnormal price before halftime in control set A is due to data tampering, and its information security is scored as 2 points according to the degree of influence which is shown in the Table 7.
The post-transaction risk grade evaluation cloud map generated by combining the aforementioned factors is shown in Figure 8. The expected mean value of control set A is 3.0322, and the credibility factor obtained by the ratio of expected variance to the mean value is 0.0582. The expected average value of control set B is 3.9998, and the expected credibility factor is 0.0453.
Among these, dark blue, red, yellow, and purple are the risk level standard cloud maps, while green and light blue are the level evaluation cloud maps of control sets A and B, respectively. It can be seen that there is no risk in control set B, while there is a slight risk in control set A. Through the indicator evaluation of membership matrix in control set B, it can be seen that the existing risk mainly lies in the influence of high Lerner coefficient and information security risk.
This work proposes a method to comprehensively evaluate the risk in the green electricity market based on the multi-dimensional cloud model and establish relevant risk management from pre-transaction and post-transaction. First, this article establishes the green electricity market risk evaluation indicator system from the two dimensions of pre-transaction and post-transaction. Second, the multi-dimensional normal cloud model is used to classify the risk level in each dimension combined with multiple types of indicators. Finally, according to the evaluation results, risk management was carried out pre-site and post-site, so as to form a two-stage control mode. Next, the optimization of multi-dimensional normal cloud models and various indicator-level fusions have to be studied. The calculation methods of various indicators are further refined and improved and quantify the risk reduction effect of various risk control mechanisms.
The original contributions presented in the study are included in the article/Supplementary Material; further inquiries can be directed to the corresponding author.
WP and SQ contributed to the conception and design of the study. ZL and RL organized the simulation, analyzed the results, and wrote parts of the manuscript. ZL gave suggestions in technical support. TR and RL contributed to and wrote parts of the manuscript. TR contributed to the improvement of the study design. All authors contributed to the article and approved the submitted version.
This work was supported by the Science and Technology Project of the State Grid Zhejiang Electric Power Company Limited (No. B311DJ220002). The funder was not involved in the study design, collection, analysis, interpretation of data, the writing of this article, or the decision to submit it for publication.
WP, SQ, and TR were employed by the Zhejiang Power Exchange Center Co., Ltd.
The remaining authors declare that the research was conducted in the absence of any commercial or financial relationships that could be construed as a potential conflict of interest.
All claims expressed in this article are solely those of the authors and do not necessarily represent those of their affiliated organizations, or those of the publisher, editors, and reviewers. Any product that may be evaluated in this article, or claim that may be made by its manufacturer, is not guaranteed or endorsed by the publisher.
Bao, M., Ding, Y., Zhou, X., Guo, C., and Shao, C. (2021). Risk assessment and management of electricity markets: A review with suggestions. CSEE J. Power Energy Syst. 7, 1322–1333. doi:10.17775/CSEEJPES.2020.04250
Bukarica, V., and Tomsic, Z. (2016). Design and evaluation of policy instruments for energy efficiency market. IEEE Trans. Sustain. energy 8, 354–362. doi:10.1109/tste.2016.2599584
Chen, S., Liu, P., and Li, Z. (2020). Low carbon transition pathway of power sector with high penetration of renewable energy. Renew. Sustain. Energy Rev. 130, 109985. doi:10.1016/j.rser.2020.109985
De, G., Tan, Z., Li, M., Huang, L., Wang, Q., and Li, H. (2019). A credit risk evaluation based on intuitionistic fuzzy set theory for the sustainable development of electricity retailing companies in China. Energy Sci. Eng. 7, 2825–2841. doi:10.1002/ese3.464
Deng, T., Yan, W., Nojavan, S., and Jermsittiparsert, K. (2020). Risk evaluation and retail electricity pricing using downside risk constraints method. Energy 192, 116672. doi:10.1016/j.energy.2019.116672
Dong, L., Wang, S., Hua, H., and Guo, H. (2021). Identification of market power abuse in spot market of Chinese electric market. Proc. CSEE 41 (24), 8397–8408. doi:10.1088/1757-899X/740/1/012077
Gatzert, N., and Kosub, T. (2016). Risks and risk management of renewable energy projects: The case of onshore and offshore wind parks. Renew. Sustain. Energy Rev. 60, 982–998. doi:10.1016/j.rser.2016.01.103
He, Y., Jiao, Z., and Yang, J. (2018). Comprehensive evaluation of global clean energy development index based on the improved entropy method. Ecol. Indic. 88, 305–321. doi:10.1016/j.ecolind.2017.12.013
Hu, B., and Zhou, P. (2022). Can the renewable power consumption guarantee mechanism help activate China's power trading market? Energy 253, 124182. doi:10.1016/j.energy.2022.124182
Lian, J., Ren, H., Sun, Y., and Hammerstrom, D. J. (2018). Performance evaluation for transactive energy systems using double-auction market. IEEE Trans. Power Syst. 34, 4128–4137. doi:10.1109/tpwrs.2018.2875919
Linnerud, K., and Simonsen, M. (2017). Swedish-Norwegian tradable green certificates: Scheme design flaws and perceived investment barriers. Energy Policy 106, 560–578. doi:10.1016/j.enpol.2017.04.012
Liu, X., and Zeng, M. (2017). Renewable energy investment risk evaluation model based on system dynamics. Renew. Sustain. Energy Rev. 73, 782–788. doi:10.1016/j.rser.2017.02.019
Peng, H., and Wang, J. (2018). A multicriteria group decision-making method based on the normal cloud model with zadeh's Z -numbers. IEEE Trans. Fuzzy Syst. 26, 3246–3260. doi:10.1109/tfuzz.2018.2816909
Song, D., Yang, M., Bai, H., Li, H., Wang, J., Wang, J., et al. (2019). A comprehensive evaluation model of power market operation efficiency based on extension cloud theory. Energy Procedia 156, 302–309. doi:10.1016/j.egypro.2018.11.146
Tayşi̇, Z. C., Bi̇ri̇ci̇k, G., and Bozkurt, Ö. Ö. (2015). “Evaluation of price forecast systems for Turkish Electric Market,” in 2015 23nd Signal Processing and Communications Applications Conference (SIU), Malatya, Turkey, 16-19 May 2015, 620–623.
Wang, K., Niu, D., Yu, M., Liang, Y., Yang, X., Wu, J., et al. (2021). Analysis and countermeasures of China’s green electric power development. Sustainability 13, 708. doi:10.3390/su13020708
Wang, P., Huang, S., and Cai, C. (2020). Dual linguistic term set and its application based on the normal cloud model. IEEE Trans. Fuzzy Syst. 29, 2180–2194. doi:10.1109/tfuzz.2020.2994994
Wang, T., Gong, Y., and Jiang, C. (2014). A review on promoting share of renewable energy by green-trading mechanisms in power system. Renew. Sustain. Energy Rev. 40, 923–929. doi:10.1016/j.rser.2014.08.011
Wȩdzik, A., Siewierski, T., and Szypowski, M. (2017). Green certificates market in Poland–The sources of crisis. Renew. Sustain. Energy Rev. 75, 490–503. doi:10.1016/j.rser.2016.11.014
Yan, H., Wu, D., Huang, Y., Wang, G., Shang, M., Xu, J., et al. (2017). Water eutrophication assessment based on rough set and multi-dimensional cloud model. Chemom. Intelligent Laboratory Syst. 164, 103–112. doi:10.1016/j.chemolab.2017.02.005
Yu, N., Liu, C., and Price, J. (2009). A new cognitive model: Cloud model. Int. J. intelligent Syst. 24, 357–375. doi:10.1002/int.20340
Yu, X., Zheng, D., and Zhou, L. (2021). Credit risk analysis of electricity retailers based on cloud model and intuitionistic fuzzy analytic hierarchy process. Int. J. Energy Res. 45, 4285–4302. doi:10.1002/er.6090
Keywords: green electricity market, multi-dimensional cloud, comprehensive indicator system, risk evaluation, risk management
Citation: Pan W, Qiao S, Ruan T, Luo R and Li Z (2023) Risk evaluation and management mechanism of green electricity market based on multi-dimensional cloud model. Front. Energy Res. 11:1203633. doi: 10.3389/fenrg.2023.1203633
Received: 11 April 2023; Accepted: 15 May 2023;
Published: 06 June 2023.
Edited by:
Nantian Huang, Northeast Electric Power University, ChinaReviewed by:
Chengcheng Shao, Xi’an Jiaotong University, ChinaCopyright © 2023 Pan, Qiao, Ruan, Luo and Li. This is an open-access article distributed under the terms of the Creative Commons Attribution License (CC BY). The use, distribution or reproduction in other forums is permitted, provided the original author(s) and the copyright owner(s) are credited and that the original publication in this journal is cited, in accordance with accepted academic practice. No use, distribution or reproduction is permitted which does not comply with these terms.
*Correspondence: Zhiyi Li, emhpeWlAemp1LmVkdS5jbg==
Disclaimer: All claims expressed in this article are solely those of the authors and do not necessarily represent those of their affiliated organizations, or those of the publisher, the editors and the reviewers. Any product that may be evaluated in this article or claim that may be made by its manufacturer is not guaranteed or endorsed by the publisher.
Research integrity at Frontiers
Learn more about the work of our research integrity team to safeguard the quality of each article we publish.