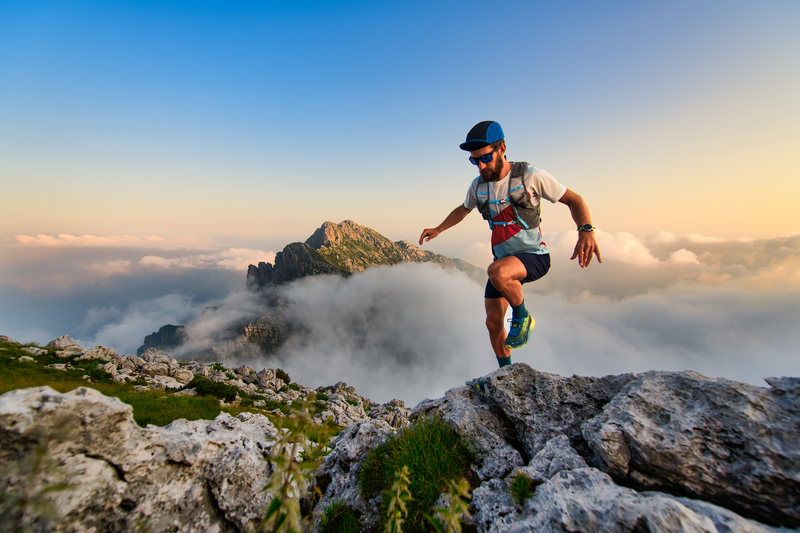
95% of researchers rate our articles as excellent or good
Learn more about the work of our research integrity team to safeguard the quality of each article we publish.
Find out more
ORIGINAL RESEARCH article
Front. Energy Res. , 21 July 2023
Sec. Sustainable Energy Systems
Volume 11 - 2023 | https://doi.org/10.3389/fenrg.2023.1197030
The massive consumption of energy promotes rapid economic growth, but it also unavoidably results in a large amount of greenhouse gas emissions, which seriously hinders society’s green and low-carbon development. This paper aims to explore the real impact of renewable energy and digitalization on greenhouse gas emissions from an energy-related perspective using advanced panel econometrics methods based on G7 panel data for 1990–2020. Economic growth and energy efficiency are also considered as control variables. Due to the nonlinear properties of panel data, the moment quantile regression approach is utilized in this research. The findings show that slope heterogeneity is widespread, section-dependent, and has a long-term equilibrium relationship. In addition, digitalization, renewable energy, and energy efficiency can reduce energy-related greenhouse gas emissions and ease environmental pressures. Economic expansion, on the other hand, remains an important positive driver for energy-related greenhouse gas emissions. The results of this study are robust and the causal relationships between variables are tested. Based on the conclusion presented above, this study advises the G7 economies to expand investments in renewable energy and digitalization to promote energy system transformation and pave the road for global decarbonization objectives to be met.
Energy is a critical physical underpinning for economic progress. Energy consumption has increased due to economic expansion, however the increased use of nonrenewable energy sources endangers ecosystems (Adebayo and Rjoub, 2022). The amount of greenhouse gas emissions in the atmosphere has increased dramatically in recent years, causing a slew of natural disasters such as global warming, droughts, and iceberg melting that represent a severe threat to human civilization (Li et al., 2023a). According to the research “CO2 Emissions in 2022”, despite the fact that the growth of global GHG emissions in 2022 was less than expected, non-renewable energy sources continued to account for a large share of GHG emissions, and many fossil fuel businesses were even reaping record profits (IEA, 2023). Furthermore, the growth in CO2 intensity of energy usage, a substantial contributorto greenhouse gas emissions, is increasing at a quicker rate than the previous 10-year average, which is clearly out of step with the global emission reduction objective (Kirikkaleli et al., 2023). As previously stated, greater attention should be paid to the issue of energy-related greenhouse gas emissions, and more in-depth research on this topic is urgently needed to overcome this conflict and achieve low-carbon development.
The G7 countries accounted for 23.2% of global greenhouse gas emissions in 2020, with fossil fuels accounting for 75% of those emissions, according to the research (Dale, 2021). When the G7 nations signed the Paris Climate Agreement in 2015, they promised to establishing a green, low-carbon society, reducing environmental strains, and achieving sustainable development. G7 members have created policies to modify energy consumption, boost renewable energy consumption while decreasing use of fossil fuels, and promote digitalization to support the transition of energy systems to energy efficiency and low-carbon. These tactics are meant to help the agreement’s aims be met more effectively (Voumik et al., 2023). The G7 countries continue to enjoy a significant advantage in terms of renewable energy consumption and digitalization, but this does not change the reality that the G7 countries remain large producers of greenhouse gas emissions. As a result, the G7 nations’ transition to renewable energy and progress of the energy system through digitalization is slow, and there is still a long way to go before meeting the greenhouse gas emission reduction target (Lei et al., 2022). Therefore, investigating energy-related greenhouse gas emissions in the G7 is essential to meeting the global decarbonization goals.
Several factors, such as energy efficiency and economic growth, have been proven to have an impact on energy-related greenhouse gas emissions (Mirza et al., 2022; Wang et al., 2023a; Gyamerah and Gil-Alana, 2023). However, research on the influence of renewable energy and digitalization on energy-related greenhouse gas emissions is lacking. Renewable energy is regarded as a critical measure for reducing greenhouse gas emissions, protecting ecosystems, and ensuring electricity supply (Xu and Ullah, 2023). Few studies have linked renewable energy to energy-related greenhouse gas emissions in the past, and greater emphasis has been made to the influence of renewable energy on greenhouse gases in recent years. Simultaneously, digitalization, as a virtual approach to complete energy transformation, can increase company resource use, cut energy consumption, and even offer low-carbon financing to enterprises (Wu et al., 2023). Digitalization is also an important aspect in promoting green enterprise transformation and improving green performance (Zhao et al., 2023). Existing studies focus on improving digitalization at the corporate level while neglecting its impact on a country’s or economy’s total energy system and green development. Although some studies have demonstrated that digitalization can reduce greenhouse gas emissions due to dematerialization effects, further empirical research is needed to evaluate whether it has a major impact on energy-related greenhouse gas emissions. As a result, the following objectives are sought by this essay. The influence of renewable energy on energy-related greenhouse gas emissions is first investigated. Second, evaluate the effect of digitalization on energy-related greenhouse gas emissions. Finally, investigate the effects of energy efficiency and economic growth on greenhouse gas emissions related to energy. To accomplish the research objectives, the study utilizes ENGHG as energy-related greenhouse gas emissions, REC as renewable energy consumption, DIGT as Digitalization, ENERF as energy efficiency, GDP as economic growth.
The inspiration for this research arises from the G7 countries’ increasing emissions of energy-related greenhouse gases and the absence of relevant studies to examine the influencing elements of energy-related greenhouse gas emissions. The G7 should be a global leader in decreasing energy emissions, but their growing reliance on chemical fuels has resulted in large emissions of hazardous gases, which have had a severe impact on the global environment. This work has significant implications for worldwide environmental protection. It is critical to emphasize that the G7 study adds to the body of knowledge about the factors that influence energy-related greenhouse gas emissions. Investing in renewable energy and supporting digitalization can help to cut greenhouse gas emissions and enhance environmental quality. The empirical evaluation results are also confirmed. Thus, this study yields novel findings for environmental protection and sustainable development, particularly in G7 economies.
The main contribution of this article is the following three points. The study firstly investigates the influence of renewable energy use and digitalization on energy-related greenhouse gas emissions in G7 economies from 1990 to 2020. Previously, Ahmadi and Frikha (2022) investigated the role of environmental innovation and renewable energy consumption in international trade and discovered novel conclusions. However, digitalization is a novel issue that has not been explored in terms of its impact on energy-related gas emissions. A few studies (Alina-Petronela et al., 2023; Li et al., 2023b; Deshuai et al., 2022) integrate digitalization with renewable energy consumption in non-G7 economies. Therefore, this paper presents new empirical evidence for G7 countries’ greenhouse gas emission reductions and energy transformation. Second, the literature on energy-related greenhouse gas emissions and renewable energy consumption is limited. This study examines the actual influence of renewable energy consumption on gas emissions from an energy standpoint, adding to the existing mainstream literature. Third, a thorough empirical examination of the influence of digitalization on energy-related greenhouse gas emissions is carried out. This paper also provides the first simultaneous causal analysis of renewable energy consumption, digitalization, and energy efficiency, as well as energy-related emissions in G7 economies. As a result, this work adds to the current empirical literature in a novel and useful way.
The remainder of the manuscript is organized as follows. The second section examines relevant literature for research analysis. Section 3 contains information on the research’s data, model, and methods. Section 4 discusses the findings and comments, while Section 5 discusses the conclusions and policy implications.
Understanding the nexus between the variables under research is documented in this manuscript section.
REC and DIGT are important factors that influence ENGHG emissions. Several authors have investigated this link and discovered diverse results. There are numerous studies in the existing literature on the relationship between renewable energy consumption and ENGHG emissions that demonstrate that REC has a significant influence on decreasing carbon emissions. Qing et al. (2023) studied the relationship between renewable energy and energy-related emissions using moment quantile regression and discovered that they are interrelated and that renewable energy has a favorable influence. Leng and Zhang (2023) thought that renewable energy may help to reduce carbon emissions while also assisting in the restructuring of the global energy system. Zhang and Zhang (2022) examined renewable energy and ENGHG emissions and discovered that using renewable energy significantly lowered ENGHG emissions in the region. Anser et al. (2021) investigated the impact of renewable energy consumption on BRICS countries and discovered that renewable energy has the potential to considerably cut ENGHG emissions. However, empirical studies from Lei et al. (2022) reveal that the positive shock of renewable energy consumption has a large negative influence on ENGHG emissions, whereas the negative shock of renewable energy consumption leads to an increase in ENGHG emissions in the long run. As a result, it is important to note that the effect of renewable energy consumption on ENGHG emissions may be unclear. The majority of mainstream research concludes that renewable energy consumption has a beneficial environmental impact (Ren et al., 2023a; Yuan et al., 2022; Abbas et al., 2022; Sharma et al., 2021; Mohsin et al., 2021; Hu et al., 2021; Hussain et al., 2021). Furthermore, the following body of work explores the connection between REC and ENGHG emissions (Borzuei et al., 2022; Chien et al., 2022). For causality analysis, Mohsin et al. (2021) showed causal associations in their research.
The influence of digitalization on ENGHG emissions is determined by the national level of digitalization. Huang and Zhang (2023) recently explored the relationship between digitalization, global value chain placement, and carbon emissions. The empirical findings indicated that technological advancements in digitalization can boost low-carbon growth. Digitalization has greatly lowered regional ENGHG emissions, and this effect will be long-lasting (Ma and Wu, 2023). The empirical findings of (Zhang et al., 2023b) demonstrated that digitalization may stimulate energy storage technology innovation and coordinate energy systems, hence lowering carbon emissions. Kuzior et al. (2022) examined the effect of digitalization on ENGHG emissions using EU member states as an example. The empirical findings of Dong et al. (2022) demonstrated that digitalization reduces the intensity of emissions; Ma et al. (2022) predicted that the Chinese economy’s digitalization may achieve the carbon-neutrality objective, and empirical results showed that digitalization can limit energy emissions to minimize carbon dioxide production. Chen (2022) evaluated the long-term and significant relationship between digitalization and ENGHG emissions. By lowering energy consumption and increasing the structure and efficiency of energy systems, digitalization can assist accomplish the Sustainable Development Goals (Ali et al., 2023; Ren et al., 2023b; Xu et al., 2022a; Mondejar et al., 2021).
Energy efficiency is critical in reducing CO2 emissions and managing the environment. Li et al. (2022a) researched the impact of energy efficiency and green innovation on ENGHG emissions in China between 1991 and 2019. The empirical analysis found that increasing energy efficiency and green innovation reduces emissions, whereas decreasing energy efficiency and green innovation increases China’s CO2 emissions in the long run. Qing et al. (2023) examined the significance of energy efficiency in reducing ENGHG emissions in BRICS countries. Calvillo (2023) evaluated the influence of five different energy system models on energy efficiency and gas emissions, and the empirical results demonstrate that energy system selection is significant in enhancing energy efficiency and, as a result, lowering gas emissions. Mirza et al. (2022) checked the impact of energy efficiency on energy emissions in developing nations. According to the research, energy efficiency is a substantial factor to lowering energy emissions. Tu et al. (2022) evaluated energy efficiency and CO2 emissions connected to energy in RCEP economies. The discovery reveals that energy efficiency can be used as a corrective action to dramatically cut emissions and increase environmental sustainability. Furthermore, the following publications support the favorable impact of environmental innovation on ENGHG emissions (Wang et al., 2023b; Ali et al., 2022; Sattar, 2022; Bao et al., 2022; Mahapatra and Irfan, 2021).
There are plenty of studies in the literature that suggest that economic growth exerts a significant impact on ENGHG emissions (Ren et al., 2022; Wen et al., 2022; Kartal et al., 2023; Kirikkaleli et al., 2023). Su et al. (2023) observed that the effect of economic expansion on ENGHG emissions revealed EKC features. Liu and Ma (2023) demonstrated the link between green economic growth and ENGHG emissions in Belt and Road member nations. Chen et al. (2023) investigated the influence of economic growth on emissions reduction in China’s power system, and the empirical findings imply that long-term economic measures to minimize greenhouse gas emissions should be implemented. Chen (2022) evaluated the relationship between CO2 emissions and French economic development from 1975 to 2019. Economic expansion increases CO2 emissions, according to empirical evidence. Obobisa et al. (2022) analyzed the long-term impact of institutional quality and economic growth on CO2 emissions in 25 African nations between 2000 and 2018. According to the findings, economic growth and institutional quality have a favorable effect on CO2 emissions. Other research has demonstrated a positive relationship between ENGHG emissions and GDP (Xu et al., 2022b; Sun et al., 2022). The implication is that GDP will increase, reducing environmental sustainability. The empirical conclusion of Mujtaba et al. (2022) implies that economic expansion suppresses environmental quality in OECD nations; the NARDL model estimates that each 1% rise in economic growth reduces ENGHG emissions by 0.4%. Methodology.
The impact of renewable energy consumption (REC), digitalization (DIGT), energy efficiency (ENERF), and economic growth (GDP) on energy-related greenhouse gas emissions (ENGHG) is discussed in this section. Policymakers around the world have adopted a range of strategies to reduce greenhouse gas emissions, including expanding renewable energy consumption (Xu and Ullah, 2023). Renewable energy development is a critical method for achieving carbon neutrality and mitigating climate change (Tang et al., 2023). Countries must raise the amount of renewable energy, adapt the energy structure, and discover more suitable energy sources to reduce greenhouse gas emissions and enhance environmental quality (Zhang et al., 2023a). In other words, renewable energy is critical for environmental protection, combating climate change, and attaining long-term economic and social growth. Based on the preceding explanation, this analysis assumes that the negative impact of REC on ENGHG is:
Five variables are chosen based on the theoretical framework and research aims. Energy-related greenhouse gas (ENGHG) emissions were the dependent variable. Renewable energy consumption (REC) and digitalization (DIGT) are, on the other hand, critical factors. In addition, two control variables were added: energy efficiency (ENERF) and economic development (GDP). Since the combined impact of renewable energy use and digitalization on energy-related greenhouse emissions is still unknown, this study tends to explore the true association between them in the Group of Seven (G7) economies, including United States, United Kingdom, France, Germany, Japan, Italy and Canada. This study covers the period of the last three decades, ranging from 1990 to 2020. Following the literature (Zheng et al., 2023) and (Lei et al., 2022), this study constructes the following general model:
For the empirical estimations, the model can be given below:
where
Initially, this research summarizes the data using descriptive statistics such as mean, median and range. Additionally, the study also evaluated the standard deviation of the data to measure the overall volatility of each data set. Further, this research investigates the regularity of each variable. Specifically, skewness and kurtosis are estimated to understand if the data have a regular distribution. In this sense, the current study calculates skewness and Kurtosis against critical values of 1 and 3, respectively. This research additionally uses the Jarque and Bera (1987) normalcy test, which assumes skewness and excess Kurtosis to be equivalent to zero. The statistics of may be calculated using the following equation:
Since this study focuses on panel data, panel data techniques are appropriate to use. This research examines panel data properties including Slope heterogeneity and Cross-section Dependence. These two panel data issues are considered crucial and if not solved, the results will be biased and inaccurate (Wei et al., 2022). Considering the G7 countries are all sophisticated economies, it is critical to determine whether they have any similarities. Using the slope coefficient homogeneity test devised by Pesaran and Yamagata (2008) is better, since it produces both the standard slope coefficient homogeneity and the adjusted slope coefficient homogeneity, as follows:
where
Under the influence of economic globalization, activities such as foreign trade, capital flows, and technology transfer potentially increase a country’s dependence on other economies, as well as the dependence of other economies or regions on it. Yet, ignoring
Due to the possible commonality of the panel data, this study uses a unit root estimator to address SCH and PCD issues. In particular, this study employs the Pesaran’s (2007) cross-sectional IPS (CIPS) test. Pesaran (2006) skillfully constructed a factor model to analysis the cross-sectional dependence of unexplained cross-sectional averages; Pesaran (2007) managed to modify the Augmented Dickey-Fuller regression by combining the average and first differed cross-section lags. This methodology produces cross-sectional dependence even though the panels are unbalanced (T > N or N > T). The basic CIPS equation is as follows:
The CIPS test assumes the existence of a unit root in the time series.
This study uses the error correction framework of Westerlund (2007) to assess the long-run equilibrium relationship between the variables under consideration. This test is designed to provide accurate estimates despite the cross-sectional dependence and slope fluctuations. Since it considers both group mean statistics, i.e.,
As the estimation results verify the cointegration between the variables, the study considers the non-normality, which leads to a new estimation method, i.e., Method of Moments Quantile Regression (MMQR) (Koenker and Bassett, 1978). Quantile regression works well when the dataset’s distribution is asymmetrical or follows the properties of non-normal distribution (Shahzad et al., 2023). Machado and Santos Silva (2019) designed the MMQR technique for assessing the dispersion of quantile estimates (Sarkodie and Strezov, 2019), which is a solution to the problem of non-normality. Equation 8 provides the conditional quantile location-scale variant
Here, the probability representation
where
where
where
As robustness estimation, this work used Bootstrap Quantile Regression (BSQR) technique after obtaining empirical data for each variable by MMQR. The BSQR method is a gap technique for analyzing confidence intervals and statistical significance, which uses algorithmic capabilities to estimate the sample distribution of the evaluation model. The BSQR method has the merit of obtaining quantifiable information, which avoids asymptotically normal sample distribution restrictions. The BSQR approach could offer more efficient estimation and empirical results (Markus and Groenen, 1998).
Due to the lack of causality between the dependent and explanatory variables in the above method, even the presence of an unbalanced panel (T is not equal to N) will not provide an optimal and accurate estimate. This study uses the panel Granger causality test developed by Dumitrescu and Hurlin (2012), which is more powerful and deals well with the panel data including cross-section dependency and slope variability (Banday and Aneja, 2020).
To begin with, this study performed a descriptive diagnosis of the statistics including mean, median, maximum and minimum values. The means and medians of all variables in this study are positive, indicating that these variables have increased over time. The standard deviation of variables illustrates the volatility of the data and the extent to which they deviate from the mean position. Kurtosis and skewness can reflect the symmetry and peakedness of the data distribution. According to Table 1, it can be seen that DIGT, REC and ENERF show a skewed negative distribution, and ENGHG emissions and GDP show a skewed normal distribution. In this study, the non-normality of the data distribution was verified using the Jarque Bera method, and the probability statistics showed significant results, leading to the rejection of the original hypothesis and the conclusion that all variables are asymmetrically distributed.
Since this study deals with panel data from seven developed countries and spans the period 1990–2020, it is necessary to perform slope homogeneity and cross-sectional dependence tests before panel data analysis. The estimation results of these checks are presented in Tables 2, 3. According to the empirical result in Table 2, the empirical result in Table 2 indicates that SCH and ASCH statistics are significant at the 1% level of significance, thus rejecting the original hypothesis of homogeneity. According to the empirical result of PCD in Table 3, all variables are statistically significant at the 1% level of significance, thereby rejecting the null hypothesis and concluding that all variables in G7 countries are interrelated and cross-dependent.
In this study, the CIPS unit root test of Pesaran (2007) was used. Table 4 provides the stationary results of unit root analysis. The test result reveals that REC, DIGT, and ENERF are significant, but ENGHG emissions and GDP are not significant, which indicates the existence of unit roots for these two variables. In addition, by testing the first-order difference data for these two non-stationary variables, this verifies their stationary and permits this research to investigate the long-term relationship.
The results of the unit root test indicate that all variables are stationary, so the existence of a long-run cointegration relationship between them needs to be examined. The Westerlund ECM Cointegration Test is employed in this study. The empirical result from Table 5 reveals that there is no cointegration in the original hypothesis. The significant p-values demonstrate a long-term correlation between the variables, indicating that ENERF, REC, GDP, and DIGT are cointegrated with ENGHG emissions.
The above empirical findings indicate that long term relationships exist between the variables explored, therefore, this study attempts to explore their effects on ENGHG emissions. The non-normality of the information leads to the choice of a new approach, i.e., MMQR for empirical results. The primary results are provided in Table 6. First, energy efficiency is significantly and negatively correlated with ENGHG emissions in all quartiles, which suggests that in the G7, improving energy efficiency can reduce ENGHG emissions. The variable finding is consistent with (Li et al., 2022a; Lei et al., 2022; Mirza et al., 2022). Next, the coefficients of all quartiles of economic growth are negative, indicating a significant negative correlation between GDP and ENGHG emissions. The negative impact of economic growth is consistent with (Iqbal et al., 2022; Obobisa et al., 2022; Sufyanullah et al., 2022; Xue et al., 2022). This means that economic growth will largely aggravate environmental pollution. Further, renewable energy consumption is significant in the first and second quartiles and the coefficients are all negative, which indicates that increased REC could lower ENGHG emissions. The estimated results are in line with the existing studies of (Apergis et al., 2023; Mukhtarov et al., 2023). Finally, all coefficients of the digitalization are also negative, which demonstrates a negative correlation between DIGT and ENGHG emissions. The result is consistent with (Ke et al., 2022; Ma and Wu, 2023). This suggests that the advancement of digitalization allows for a reduction in ENGHG emissions while also improving environmental quality. Figure 1 depicts the trend graphs between all variables in the moment quantile regression and energy-related greenhouse gas emissions.
This study used Bootstrap Quantile regression to assess the model’s robustness, and the results indicate that the model utilized in this study is stable and dependable. Significant robustness analysis results are presented in Table 7, especially at the (Q0.75) and (Q0.90) quartiles. The trend of all variable coefficients in Bootstrap Quantile regression is plotted in Figure 2.
Since moment quantile regression is unable to reveal the causal relationship between variables, this study employs the Dumitrescu and Hurlin’s (2012) panel Grander causality test, and estimated results are shown in Table 8. The variable pairs ENERF≠ENGHG, ENGHG≠ENERF; REC≠ENGHG, ENGHG≠REC; GDP≠ENGHG, ENGHG≠GDP are significant. However, no significant causal relationship was found between DIGT and ENGHG emissions. For the assessment of causal relationships between variables in line with the literature (Anser et al., 2021; Eskander and Istiak, 2021; Lei et al., 2022; Tufail et al., 2022; Mukhtarov et al., 2023; Zheng et al., 2023).
The empirical econometric results above illustrate a long-term relationship between four variables in G7 advanced countries: energy efficiency (ENERF), renewable energy consumption (REC), digitalization (DIGT), and economic development (GDP). Empirical results reveal that REC has a negative influence on ENGHG of G7 economies, which is consistent with (Xiong et al., 2022; Zhang and Zhang, 2022). These two studies, conducted in BRICS and Belt and Road nations, looked at the detrimental impact of renewable energy on energy-related greenhouse gas emissions. These studies give actual evidence of renewable energy’s negative influence on greenhouse gas emissions in various economies. The essence of renewable energy’s negative impact on energy-related greenhouse gas emissions is a shift in energy mix. Renewable energy, as an alternative to traditional fossil fuels, can have a low carbon footprint or minimize greenhouse gas emissions and other chemical pollutants in the manufacturing process. According to the findings of Lei et al. (2022)’s survey on renewable energy in China, renewable energy has some benefits over traditional fossil fuels in terms of energy supply diversification and environmental sustainability. However, renewable energy confronts several challenges, including expensive construction and development costs, challenging storage, and a long payback period. Sanchez et al. (2022) illustrated this in their investigation of alternative energy choices. Therefore, finding acceptable alternative energy sources, boosting renewable energy conversion efficiency, and lowering investment prices are critical ways to minimize greenhouse gas emissions from energy sources.
DIGT, on the other hand, has a negative influence on ENGHG, as evidenced by Alina-Petronela et al. (2023)’s European nation survey. The results of our research suggest that digitisation reduces energy-related greenhouse gas emissions. Previously, the OECD (2010) estimated that digitalization would expand the manufacturing scale of information and communication technology, resulting in increased energy consumption and greenhouse gas emissions. However, current empirical studies on digitalization indicate that this viewpoint is certainly no longer prevalent. Digitalization is considered as a blessing for lowering greenhouse gas emissions (Chen, 2022). Digitalization promotes national energy system transformation, increases clean energy efficiency, decreases energy consumption in the economic system, and reduces energy-related greenhouse gas emissions. ENERF had a detrimental influence on ENGHG as well. Mirza et al. (2022) and JinRu and Qamruzzaman (2022) also corroborated this conclusion. These studies indicate that increasing energy efficiency may save the country money while also lowering energy-related greenhouse gas emissions. Empirical results reveal that GDP boosts ENGHG emission, which is consistent with (Gyamerah and Gil-Alana, 2023; Yahyaoui, 2023). Economic development is still heavily reliant on nonrenewable resources, resulting in significant greenhouse gas emissions.
In conclusion, investigating the link between energy efficiency, renewable energy consumption, digitalization, and economic growth, as well as energy-related greenhouse gas emissions in the G7, is critical for balancing economic and environmental progress. Many industries rely on energy inputs to grow, which increases greenhouse gas emissions and environmental pressures. Consumption of renewable energy and digitalization necessitate large investment expenditures that middle-income or rising nations may be unable to finance. Improvements in energy efficiency may result from technology breakthroughs, investment in R&D expenses, and other causes, but no immediate advantages should be expected. Large-scale energy efficiency gains take time, and ineffective energy efficiency switching can stymie national economic development. Storage and other issues hinder green progress when it comes to adopting and integrating renewable energy sources. In this context, the empirical outcomes of this study may give a path for academics, politicians, and regulators to take appropriate action in order to achieve low-carbon development.
The research investigated the influence of renewable energy consumption, digitalization, energy efficiency, and economic growth on energy-related greenhouse gas emissions in the G7 economies objectively. The simultaneous evaluation of these factors in G7 economies was unique. Despite substantial research, their relevance to energy-related greenhouse gas emissions has received little attention. As a result, this research investigated the genuine impact of renewable energy consumption and digitalization on energy-related greenhouse gas emissions. Advanced econometric tools were employed in this work to analyze in depth the factors influencing energy-related greenhouse gas emissions. On the one hand, empirical studies suggested that promoting renewable energy may reduce reliance on fossil fuels, achieve carbon neutrality and ameliorate climate change, and promote green and low-carbon growth. By facilitating energy system transition and improving energy sector structure, digitalization can help to reduce energy-related greenhouse gas emissions. On the other hand, economic development and energy efficiency are major elements in reducing emissions and improving the environment. The estimated results are consistent with the existing mainstream literature. Overall, our research uncovered novel connections between renewable energy use, digitalization, energy efficiency, and energy-related greenhouse gas emissions. This discovery has the potential to significantly improve environmental quality and achieve sustainable development.
Based on the findings of the empirical research, this paper suggests some policy recommendations that may assist governments and policymakers in developing and implementing effective policies to reduce energy emissions. Renewable energy consumption should be encouraged in developed countries, and government incentives and aid should be offered to sectors transitioning to clean energy. Simultaneously, investment and government spending in energy technology must be expanded, prompting policymakers to place a greater emphasis on energy-related technical innovation. A higher degree of digitalization will reduce environmental impact; consequently, the development of smart technology in the digital sphere should be supported. Furthermore, for global environmental sustainability, economic development in industrialized economies must minimize reliance on nonrenewable energy sources.
This study primarily looks at the influence of these elements in G7 nations; nevertheless, the impact may be significant in other economies throughout the world, particularly in terms of digitalization. Future studies might look into the impact of digitalization in different economies. In addition, the larger data set can improve the comprehensiveness of the study model, which future researchers can accomplish.
The original contributions presented in the study are included in the article/Supplementary Material, further inquiries can be directed to the corresponding author.
YuC: contributed to developing the idea, software, analysis, overall writeup and estimations of the results. YiC: Proofread, review. LZ: estimations, preparing draft. ZL: review. All authors contributed to the article and approved the submitted version.
The authors would like to acknowledge the support from the program “Research and application of key technologies of new generation enterprise digital platform” (No. 0050205502322).
The authors declare that the research was conducted in the absence of any commercial or financial relationships that could be construed as a potential conflict of interest.
All claims expressed in this article are solely those of the authors and do not necessarily represent those of their affiliated organizations, or those of the publisher, the editors and the reviewers. Any product that may be evaluated in this article, or claim that may be made by its manufacturer, is not guaranteed or endorsed by the publisher.
The Supplementary Material for this article can be found online at: https://www.frontiersin.org/articles/10.3389/fenrg.2023.1197030/full#supplementary-material
1Data for ENGHG and DIGT [Individuals using the Internet (% of population)] are obtained from the OECD (2022) website, available at: https://stats.oecd.org/
2Data for GDP (constant US dollars 2015), REC (% of total final energy consumption), and ENERF [GDP per unit of energy use (constant 2017 PPP $ per kg of oil equivalent)] from the World Development Indicators of the World Bank (2022), available at: https://databank.worldbank.org/source/world-development-indicators#
Abbas, S., Gui, P., Chen, A., and Ali, N. (2022). The effect of renewable energy development, market regulation, and environmental innovation on CO2 emissions in BRICS countries. Environ. Sci. Pollut. Res. 29 (39), 59483–59501. doi:10.1007/s11356-022-20013-7
Adebayo, T. S., and Rjoub, H. (2022). A new perspective into the impact of renewable and nonrenewable energy consumption on environmental degradation in Argentina: A time–frequency analysis. Environ. Sci. Pollut. Res. 29 (11), 16028–16044. doi:10.1007/s11356-021-16897-6
Ahmadi, Z., and Frikha, W. (2022). Consumption-based carbon dioxide emissions and their impact on energy productivity in the G7 countries. J. Knowl. Econ. 2022. doi:10.1007/s13132-022-00966-3
Ali, N., Phoungthong, K., Khan, A., Abbas, S., Dilanchiev, A., Tariq, S., et al. (2023). Does FDI foster technological innovations? Empirical evidence from BRICS economies. PLOS ONE 18 (3), e0282498. doi:10.1371/journal.pone.0282498
Ali, N., Phoungthong, K., Techato, K., Ali, W., Abbas, S., Dhanraj, J. A., et al. (2022). FDI, green innovation and environmental quality nexus: New insights from BRICS economies. Sustainability 14 (4), 2181. doi:10.3390/su14042181
Alina-Petronela, H., Mirela, S., Ionela, B. G., and Cristina, B. R. (2023). Climate neutrality through economic growth, digitalisation, eco-innovation and renewable energy in European countries. Kybernetes. doi:10.1108/K-09-2022-1254
Anser, M. K., Syed, Q. R., and Apergis, N. (2021). Does geopolitical risk escalate CO2 emissions? Evidence from the BRICS countries. Environ. Sci. Pollut. Res. 28 (35), 48011–48021. doi:10.1007/s11356-021-14032-z
Apergis, N., Kuziboev, B., Abdullaev, I., and Rajabov, A. (2023). Investigating the association among CO2 emissions, renewable and non-renewable energy consumption in Uzbekistan: An ARDL approach. Environ. Sci. Pollut. Res. 30, 39666–39679. doi:10.1007/s11356-022-25023-z
Banday, U. J., and Aneja, R. (2020). Renewable and non-renewable energy consumption, economic growth and carbon emission in BRICS Evidence from bootstrap panel causality. Int. J. Energy Sect. Manag. 14 (1), 248–260. doi:10.1108/ijesm-02-2019-0007
Bao, Z., Ferraz, D., and do Nascimento Rebelatto, D. A. (2022). Energy efficiency and China's sustainable carbon neutrality target: Evidence from novel research methods quantile on quantile regression approach. Econ. Research-Ekonomska Istraz. 35 (1), 6985–7007. doi:10.1080/1331677x.2022.2054456
Borzuei, D., Moosavian, S. F., and Ahmadi, A. (2022). Investigating the dependence of energy prices and economic growth rates with emphasis on the development of renewable energy for sustainable development in Iran. Sustain. Dev. 30 (5), 848–854. doi:10.1002/sd.2284
Calvillo, C. (2023). The impacts of energy efficiency modelling in policy making. ENERGIES 16 (4), 1811. doi:10.3390/en16041811
Campello, M., Galvao, A. F., and Juhl, T. (2019). Testing for slope heterogeneity bias in panel data models. J. Bus. Econ. Statistics 37 (4), 749–760. doi:10.1080/07350015.2017.1421545
Chen, L. (2022). How CO2 emissions respond to changes in government size and level of digitalization? Evidence from the BRICS countries. Environ. Sci. Pollut. Res. 29 (1), 457–467. doi:10.1007/s11356-021-15693-6
Chen, Y., Lin, J., Roland-Holst, D., Liu, X., and Wang, C. (2023). Declining renewable costs, emissions trading, and economic growth: China's power system at the crossroads. ENERGIES 16 (2), 656. doi:10.3390/en16020656
Cheng, F., He, Q. P., and Zhao, J. (2019). A novel process monitoring approach based on variational recurrent autoencoder. Comput. Chem. Eng. 129, 106515. doi:10.1016/j.compchemeng.2019.106515
Chien, F., Hsu, C.-C., Ozturk, I., Sharif, A., and Sadiq, M. (2022). The role of renewable energy and urbanization towards greenhouse gas emission in top Asian countries: Evidence from advance panel estimations. Renew. Energy 186, 207–216. doi:10.1016/j.renene.2021.12.118
Dong, A., Vyshnevskyi, O., and Trushkina, N. (2022). Assessment of the impact of digitalization on greenhouse gas emissions on the example of EU member states. Prod. Eng. Arch. 28 (4), 407–419. doi:10.30657/pea.2022.28.50
Dumitrescu, E.-I., and Hurlin, C. (2012). Testing for Granger non-causality in heterogeneous panels. Econ. Model. 29 (4), 1450–1460. doi:10.1016/j.econmod.2012.02.014
Eskander, S. M. S. U., and Istiak, K. (2021). Energy efficiency and CO2 emissions: Evidence from the UK universities. Appl. Econ. 55, 4727–4744. doi:10.1080/00036846.2022.2130872
Gyamerah, S. A., and Gil-Alana, L. A. (2023). A multivariate causality analysis of CO2 emission, electricity consumption, and economic growth: Evidence from Western and Central Africa. Heliyon 9 (1), e12858. doi:10.1016/j.heliyon.2023.e12858
Hu, X., Ali, N., Malik, M., Hussain, J., Jiang, F., and Nilofar, M. (2021). Impact of economic openness and innovations on the environment: A new look into ASEAN countries. Pol. J. Environ. Stud. 30 (4), 3601–3613. doi:10.15244/pjoes/130898
Huang, Y., and Zhang, Y. (2023). Digitalization, positioning in global value chain and carbon emissions embodied in exports: Evidence from global manufacturing production-based emissions. Ecol. Econ. 205, 107674. doi:10.1016/j.ecolecon.2022.107674
Hussain, J., Zhou, K., Muhammad, F., Khan, D., Khan, A., Ali, N., et al. (2021). Renewable energy investment and governance in countries along the belt & Road Initiative: Does trade openness matter? Renew. Energy 180, 1278–1289. doi:10.1016/j.renene.2021.09.020
Iqbal, A., Tang, X., and Rasool, S. F. (2022). Investigating the nexus between CO2 emissions, renewable energy consumption, FDI, exports and economic growth: Evidence from BRICS countries. Environ. Dev. Sustain. 25, 2234–2263. doi:10.1007/s10668-022-02128-6
Jarque, C. M., and Bera, A. K. (1987). A test for normality of observations and regression residuals. Int. Stat. Review/ Rev. Int. Stat. 55 (2), 163–172. doi:10.2307/1403192
JinRu, L., and Qamruzzaman, M. (2022). Nexus between environmental innovation, energy efficiency, and environmental sustainability in G7: What is the role of institutional quality? Front. Environ. Sci. 594. doi:10.3389/fenvs.2022.860244
Kartal, M. T., Depren, S. K., Ali, U., and Nurgazina, Z. (2023). Long-run impact of coal usage decline on CO2 emissions and economic growth: Evidence from disaggregated energy consumption perspective for China and India by dynamic ARDL simulations. Energy & Environ. 2023, 0958305X2311524. doi:10.1177/0958305x231152482
Ke, J., Jahanger, A., Yang, B., Usman, M., and Ren, F. (2022). Digitalization, financial development, trade, and carbon emissions; implication of pollution haven hypothesis during globalization mode. Front. Environ. Sci. 10. doi:10.3389/fenvs.2022.873880
Kirikkaleli, D., Awosusi, A. A., Adebayo, T. S., and Otrakci, C. (2023). Enhancing environmental quality in Portugal: Can CO2 intensity of GDP and renewable energy consumption be the solution? Environ. Sci. Pollut. Res. 30, 53796–53806. doi:10.1007/s11356-023-26191-2
Koenker, R., and Bassett, G. (1978). Regression quantiles. Econometrica 46 (1), 33–50. doi:10.2307/1913643
Lei, W., Xie, Y., Hafeez, M., and Ullah, S. (2022). Assessing the dynamic linkage between energy efficiency, renewable energy consumption, and CO2 emissions in China. Environ. Sci. Pollut. Res. 29 (13), 19540–19552. doi:10.1007/s11356-021-17145-7
Leng, Y.-J., and Zhang, H. (2023). Comprehensive evaluation of renewable energy development level based on game theory and TOPSIS. Comput. Industrial Eng. 175, 108873. doi:10.1016/j.cie.2022.108873
Li, H., Tang, H., Zhou, W., and Wan, X. (2022a). Impact of enterprise digitalization on green innovation performance under the perspective of production and operation. Front. Public Health 10, 971971. doi:10.3389/fpubh.2022.971971
Li, Y., Yan, C., and Ren, X. (2023b). Do uncertainties affect clean energy markets? Comparisons from a multi-frequency and multi-quantile framework. Energy Econ. 121, 106679. doi:10.1016/j.eneco.2023.106679
Li, Y., Zhang, C., Li, S., and Usman, A. (2022b). Energy efficiency and green innovation and its asymmetric impact on CO2 emission in China: A new perspective. Environ. Sci. Pollut. Res. 29 (31), 47810–47817. doi:10.1007/s11356-022-19161-7
Li, Z., Yang, F., and Huang, B. (2023a). The assessment of energy-related greenhouse gas emissions in China's chemical industry. Sustain. Prod. Consum. 37, 112–125. doi:10.1016/j.spc.2023.02.010
Liu, J., Sun, S., Han, Y., Meng, J., Chen, Y., Yu, H., et al. (2021). Lignin waste as co-substrate on decolorization of azo dyes by Ganoderma lucidum. J. Taiwan Inst. Chem. Eng. 122, 85–92. doi:10.1016/j.jtice.2021.04.039
Liu, M., and Ma, H. (2023). Untangling the effects of the Belt and Road Initiative on carbon dioxide emissions. J. Environ. Manag. 325, 116628. doi:10.1016/j.jenvman.2022.116628
Ma, Q., Tariq, M., Mahmood, H., and Khan, Z. (2022). The nexus between digital economy and carbon dioxide emissions in China: The moderating role of investments in research and development. Technol. Soc. 68, 101910. doi:10.1016/j.techsoc.2022.101910
Ma, Z., and Wu, F. (2023). Smart city, digitalization and CO2 emissions: Evidence from 353 cities in China. Sustainability 15 (1), 225. doi:10.3390/su15010225
Machado, J. A. F., and Santos Silva, J. M. C. (2019). Quantiles via moments. J. Econ. 213 (1), 145–173. doi:10.1016/j.jeconom.2019.04.009
Mahapatra, B., and Irfan, M. (2021). Asymmetric impacts of energy efficiency on carbon emissions: A comparative analysis between developed and developing economies. Energy 227, 120485. doi:10.1016/j.energy.2021.120485
Markus, M. T., and Groenen, P. J. F. (1998). An introduction to the bootstrap. Psychometrika 63 (1), 97–101.
Mirza, F. M., Sinha, A., Khan, J. R., Kalugina, O. A., and Zafar, M. W. (2022). Impact of energy efficiency on CO2 Emissions: Empirical evidence from developing countries. Gondwana Res. 106, 64–77. doi:10.1016/j.gr.2021.11.017
Mohsin, M., Kamran, H. W., Nawaz, M. A., Hussain, M. S., and Dahri, A. S. (2021). Assessing the impact of transition from nonrenewable to renewable energy consumption on economic growth-environmental nexus from developing Asian economies. J. Environ. Manag. 284, 111999. doi:10.1016/j.jenvman.2021.111999
Mondejar, M. E., Avtar, R., Diaz, H. L. B., Dubey, R. K., Esteban, J., Gomez-Morales, A., et al. (2021). Digitalization to achieve sustainable development goals: Steps towards a smart green planet. Sci. Total Environ. 794, 148539. doi:10.1016/j.scitotenv.2021.148539
Mujtaba, A., Jena, P. K., Bekun, F. V., and Sahu, P. K. (2022). Symmetric and asymmetric impact of economic growth, capital formation, renewable and non-renewable energy consumption on environment in OECD countries. Renew. Sustain. Energy Rev. 160, 112300. doi:10.1016/j.rser.2022.112300
Mukhtarov, S., Aliyev, F., Aliyev, J., and Ajayi, R. (2023). Renewable energy consumption and carbon emissions: Evidence from an oil-rich economy. Sustainability 15 (1), 134. doi:10.3390/su15010134
Obobisa, E. S., Chen, H., and Mensah, I. A. (2022). The impact of green technological innovation and institutional quality on CO2 emissions in African countries. Technol. Forecast. Soc. Change 180. doi:10.1016/j.techfore.2022.121670
OECD (2010). Greener and smarter: ICTs, the environment and climate change. Paris, France: Organization for Economic Co-operation and Development.
Ozcan, B., and Apergis, N. (2018). The impact of Internet use on air pollution: Evidence from emerging countries. Environ. Sci. Pollut. Res. 25 (5), 4174–4189. doi:10.1007/s11356-017-0825-1
Pesaran, M. H. (2007). A simple panel unit root test in the presence of cross-section dependence. J. Appl. Econ. 22 (2), 265–312. doi:10.1002/jae.951
Pesaran, M. H. (2006). Estimation and inference in large heterogeneous panels with a multifactor error structure. Econometrica 74 (4), 967–1012. doi:10.1111/j.1468-0262.2006.00692.x
Pesaran, M. H. (2004). General diagnostic tests for cross-sectional dependence in panels. Empir. Econ. 60 (1), 13–50. doi:10.1007/s00181-020-01875-7
Pesaran, M. H., and Yamagata, T. (2008). Testing slope homogeneity in large panels. J. Econ. 142 (1), 50–93. doi:10.1016/j.jeconom.2007.05.010
Qing, L., Dagestani, A. A., Shinwari, R., and Chun, D. (2023). Novel research methods to evaluate renewable energy and energy-related greenhouse gases: Evidence from BRICS economies. Econ. Research-Ekonomska Istraz. 36 (1), 960–976. doi:10.1080/1331677x.2022.2080746
Ren, X., Li, J., He, F., and Lucey, B. (2023b). Impact of climate policy uncertainty on traditional energy and green markets: Evidence from time-varying granger tests. Renew. Sustain. Energy Rev. 173, 113058. doi:10.1016/j.rser.2022.113058
Ren, X., Qin, J., Jin, C., and Yan, C. (2022). Global oil price uncertainty and excessive corporate debt in China. Energy Econ. 115, 106378. doi:10.1016/j.eneco.2022.106378
Ren, X., Zeng, G., and Zhao, Y. (2023a). Digital finance and corporate ESG performance: Empirical evidence from listed companies in China. Pacific-Basin Finance J. 79, 102019. doi:10.1016/j.pacfin.2023.102019
Sanchez, A., Zhang, Q., Martin, M., and Vega, P. (2022). Towards a new renewable power system using energy storage: An economic and social analysis. Energy Convers. Manag. 252, 115056. doi:10.1016/j.enconman.2021.115056
Sarkodie, S. A., and Strezov, V. (2019). A review on Environmental Kuznets Curve hypothesis using bibliometric and meta-analysis. Sci. Total Environ. 649, 128–145. doi:10.1016/j.scitotenv.2018.08.276
Sattar, U. (2022). A conceptual framework of climate action needs of the least developed party countries of the Paris agreement. Int. J. Environ. Res. Public Health 19 (16), 9941. doi:10.3390/ijerph19169941
Shahzad, U., Elheddad, M., Swart, J., Ghosh, S., and Dogan, B. (2023). The role of biomass energy consumption and economic complexity on environmental sustainability in G7 economies. Bus. Strategy Environ. 32 (1), 781–801. doi:10.1002/bse.3175
Sharma, G. D., Shah, M. I., Shahzad, U., Jain, M., and Chopra, R. (2021). Exploring the nexus between agriculture and greenhouse gas emissions in BIMSTEC region: The role of renewable energy and human capital as moderators. J. Environ. Manag. 297, 113316. doi:10.1016/j.jenvman.2021.113316
Su, L., Ji, T., Ahmad, F., Chandio, A. A., Ahmad, M., Jabeen, G., et al. (2023). Technology innovations impact on carbon emission in Chinese cities: Exploring the mediating role of economic growth and industrial structure transformation. Environ. Sci. Pollut. Res. Int. 30, 46321–46335. doi:10.1007/s11356-023-25493-9
Sufyanullah, K., Ahmad, K. A., and Ali, M. A. S. (2022). Does emission of carbon dioxide is impacted by urbanization? An empirical study of urbanization, energy consumption, economic growth and carbon emissions- using ARDL bound testing approach. Energy Policy 164, 112908. doi:10.1016/j.enpol.2022.112908
Sun, Y., Anwar, A., Razzaq, A., Liang, X., and Siddique, M. (2022). Asymmetric role of renewable energy, green innovation, and globalization in deriving environmental sustainability: Evidence from top-10 polluted countries. Renew. Energy 185, 280–290. doi:10.1016/j.renene.2021.12.038
Tang, Y., Liu, Y., Huo, W., Chen, M., Ye, S., and Cheng, L. (2023). Optimal allocation scheme of renewable energy consumption responsibility weight under renewable portfolio standards: An integrated evolutionary game and stochastic optimization approach. Energies 16 (7), 3085. doi:10.3390/en16073085
Tu, Z., Feng, C., and Zhao, X. (2022). Revisiting energy efficiency and energy related CO2 emissions: Evidence from RCEP economies. Econ. Research-Ekonomska Istraz. 35 (1), 5858–5878. doi:10.1080/1331677x.2022.2038651
Tufail, M., Song, L., Umut, A., Ismailova, N., and Kuldasheva, Z. (2022). Does financial inclusion promote a green economic system? Evaluating the role of energy efficiency. Econ. Research-Ekonomska Istraz. 35 (1), 6780–6800. doi:10.1080/1331677x.2022.2053363
Voumik, L. C., Islam, M. A., Ray, S., Yusop, N. Y. M., and Ridzuan, A. R. (2023). CO2 emissions from renewable and non-renewable electricity generation sources in the G7 countries: Static and dynamic panel assessment. Energies 16 (3), 1044. doi:10.3390/en16031044
Wang, Z.-R., Fu, H.-Q., and Ren, X.-H. (2023b). The impact of political connections on firm pollution: New evidence based on heterogeneous environmental regulation. Petroleum Sci. 20 (1), 636–647. doi:10.1016/j.petsci.2022.10.019
Wang, Z., Fu, H., and Ren, X. (2023a). Political connections and corporate carbon emission: New evidence from Chinese industrial firms. Technol. Forecast. Soc. Change 188, 122326. doi:10.1016/j.techfore.2023.122326
Wei, J., Rahim, S., and Wang, S. (2022). Role of environmental degradation, institutional quality, and government health expenditures for human health: Evidence from emerging seven countries. Front. Public Health 10, 870767. doi:10.3389/fpubh.2022.870767
Wei, X., Ren, H., Ullah, S., and Bozkurt, C. (2023). Does environmental entrepreneurship play a role in sustainable green development? Evidence from emerging asian economies. Econ. Res. -Ekon Istraz. 36 (1), 73–85. doi:10.1080/1331677x.2022.2067887
Wen, J., Ali, W., Hussain, J., Khan, N. A., Hussain, H., Ali, N., et al. (2022). Dynamics between green innovation and environmental quality: New insights into South asian economies. Econ. Polit. 39 (2), 543–565. doi:10.1007/s40888-021-00248-2
Westerlund, J. (2007). Testing for error correction in panel data. Oxf. Bull. Econ. Statistics 69 (6), 709–748. doi:10.1111/j.1468-0084.2007.00477.x
Wu, Z., Wang, X., Liao, J. Y., Hou, H., and Zhao, X. (2023). Evaluation of digital transformation to support carbon neutralization and green sustainable development based on the vision of "channel computing resources from the east to the west. Sustainability 15 (7), 6299. doi:10.3390/su15076299
Xiong, C., Wang, G., Li, H., Su, W., and Duan, X. (2022). Examining key impact factors of energy-related carbon emissions in 66 Belt and Road Initiative countries. Environ. Sci. Pollut. Res. 30, 13837–13845. doi:10.1007/s11356-022-23125-2
Xu, L., and Ullah, S. (2023). Evaluating the impacts of digitalization, financial efficiency, and education on renewable energy consumption: New evidence from China. Environ. Sci. Pollut. Res. 30 (18), 53538–53547. doi:10.1007/s11356-023-25888-8
Xu, Q., Zhong, M., and Li, X. (2022a). How does digitalization affect energy? International evidence. Energy Econ. 107, 105879. doi:10.1016/j.eneco.2022.105879
Xu, Y., Umar, M., Kirikkaleli, D., Adebayo, T. S., and Altuntas, M. (2022b). Carbon neutrality target in Turkey: Measuring the impact of technological innovation and structural change. Gondwana Res. 109, 429–441. doi:10.1016/j.gr.2022.04.015
Xue, C., Shahbaz, M., Ahmed, Z., Ahmad, M., and Sinha, A. (2022). Clean energy consumption, economic growth, and environmental sustainability: What is the role of economic policy uncertainty? Renew. Energy 184, 899–907. doi:10.1016/j.renene.2021.12.006
Yahyaoui, I. (2023). How corruption mitigates the effect of FDI on economic growth?. J. Knowl. Econ. doi:10.1007/s13132-023-01098-y
Yuan, X., Su, C.-W., Umar, M., Shao, X., and Lobont, O.-R. (2022). The race to zero emissions: Can renewable energy be the path to carbon neutrality? J. Environ. Manag. 308, 114648. doi:10.1016/j.jenvman.2022.114648
Zhang, C., and Zhang, Z. (2022). Novel research methods to examine renewable energy and energy related greenhouse gases: Evidence from novel panel methods. Econ. Research-Ekonomska Istraz. 36, 1187–1204. doi:10.1080/1331677x.2022.2082998
Zhang, H., Gao, S., and Zhou, P. (2023a). Role of digitalization in energy storage technological innovation: Evidence from China. Renew. Sustain. Energy Rev. 171, 113014. doi:10.1016/j.rser.2022.113014
Zhang, T., Yin, J., Li, Z., Jin, Y., Ali, A., and Jiang, B. (2023b). A dynamic relationship between renewable energy consumption, non-renewable energy consumption, economic growth and CO2 emissions: Evidence from Asian emerging economies. Front. Environ. Sci. 10. doi:10.3389/fenvs.2022.1092196
Keywords: greenhouse gas emissions, renewable energy, digitalization, energy efficiency, economic growth
Citation: Chen Y, Chen Y, Zhang L and Li Z (2023) Revealing the role of renewable energy consumption and digitalization in energy-related greenhouse gas emissions—Evidence from the G7. Front. Energy Res. 11:1197030. doi: 10.3389/fenrg.2023.1197030
Received: 30 March 2023; Accepted: 06 July 2023;
Published: 21 July 2023.
Edited by:
Xiaohang Ren, Central South University, ChinaReviewed by:
Mucahit Aydin, Sakarya University, TürkiyeCopyright © 2023 Chen, Chen, Zhang and Li. This is an open-access article distributed under the terms of the Creative Commons Attribution License (CC BY). The use, distribution or reproduction in other forums is permitted, provided the original author(s) and the copyright owner(s) are credited and that the original publication in this journal is cited, in accordance with accepted academic practice. No use, distribution or reproduction is permitted which does not comply with these terms.
*Correspondence: Ying Chen, MjAyMTIyNTAyNTExOUBzdHUuc2N1LmVkdS5jbg==
Disclaimer: All claims expressed in this article are solely those of the authors and do not necessarily represent those of their affiliated organizations, or those of the publisher, the editors and the reviewers. Any product that may be evaluated in this article or claim that may be made by its manufacturer is not guaranteed or endorsed by the publisher.
Research integrity at Frontiers
Learn more about the work of our research integrity team to safeguard the quality of each article we publish.