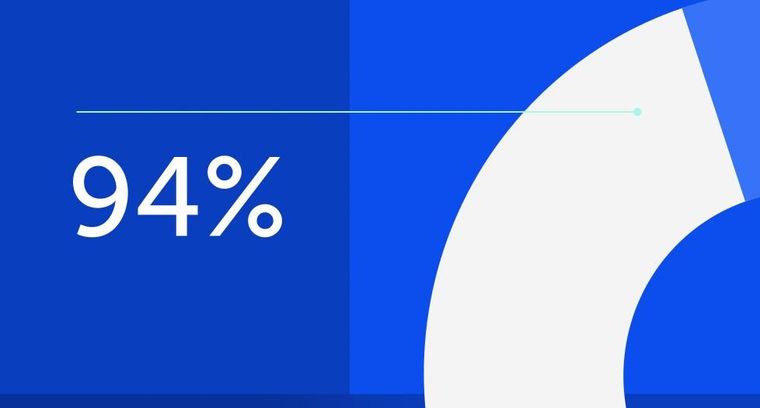
94% of researchers rate our articles as excellent or good
Learn more about the work of our research integrity team to safeguard the quality of each article we publish.
Find out more
EDITORIAL article
Front. Energy Res., 10 May 2023
Sec. Smart Grids
Volume 11 - 2023 | https://doi.org/10.3389/fenrg.2023.1195418
This article is part of the Research TopicRecent Advances in Renewable Energy Automation and Energy ForecastingView all 14 articles
Editorial on the Research Topic
Recent advances in renewable energy automation and energy forecasting
Renewable energy sources like solar, wind, and hydroelectric power are gaining popularity as we work towards a more sustainable future. However, their intermittent and often unpredictable nature, creates challenges for the energy industry in terms of being able to ensure continuous electric power generation over regular periods of time. Thus, accurate forecasting of renewable energy output is crucial for their reliable integration into the power grid. In this regard, automation and machine learning have made significant improvements in energy forecasting by enabling more precise predictions of energy output. Advanced algorithms and high-performance computing systems allow for better grid management and increased power generation systems’ efficiency. Automation is also being used for the operation and maintenance of renewable energy systems. Real-time monitoring and control systems enable a rapid response to changes in weather conditions, optimizing energy production. This editorial summarizes recent advancements in renewable energy automation and energy forecasting, which are critical areas for achieving a sustainable energy future. The Research Topic covers areas like machine learning-based energy forecasting, control and optimization of renewable energy systems, and the integration of renewable energy into microgrids as shown in Figure 1. Continued research and development in renewable energy automation and energy forecasting are essential for the transition towards a sustainable energy future.
The global energy crisis on one hand and the advancement of climate change threat on the other have prompted countries worldwide to seek alternative energy solutions, such as solar, wind, hydrogen, etc., accelerating the pace towards decarbonization of their energy matrix. Thus, renewable power generation, and the advancement of sustainable energy are becoming an important concern for many countries. With these important priorities, so grows the need for advanced technologies that can support such transformation in the countries’ power grid to transition towards renewable and sustainable energy future. These technologies must be able to monitor systems continuously and safeguard their continuous operation over regular periods of time so as to ensure the technical and economic feasibility of such large-scale projects. However, the electrical power being produced at the generation plants is transmitted to the points of utilization, which is achieved by means of the power grids (an interconnected network for electricity transmission and distribution from producers to the consumers). Hence, traditional power grids support a one-way flow of power from centralized sources, such as gas, nuclear and hydroelectric to points of consumption. Therefore, based on the devices used and their functionality, the electric power grids are classified into two categories:
• Conventional grid
• Smart grid
A smart grid is much different from the conventional power grid in the sense that it provides more reliable and consistent electric power supply, thanks to advanced power electronics, control and communications systems. The smart grid is the electrical system which is capable for monitoring the activities of the grid connected system and provides the real-time information of all the events occurring in the power system. Part of the technology that goes into a smart grid are advanced sensors and real-time advanced data processing systems, with machine learning capabilities incorporated in such systems, which can ensure the continuous monitoring of all of the systems that comprise the smart grid, thus safeguarding its continuous operation. The emergence of improved sensors, actuators, and automation technologies has consequently improved the control, monitoring and communication techniques within the energy sector, helping to advance the smart grid system. Thus, with the support of aforementioned modern technologies, the information flows in two-ways between the consumer and supplier. This data communication helps the supplier in overcoming challenges like integration of renewable technologies, management of energy demand, load automation and control. Renewable energy (RE) is intermittent in nature and therefore difficult to predict. The accurate RE forecasting is very essential to improve the power system operations. The forecasting models are based on complex functions’ combination that include seasonality, fluctuation, and dynamic nonlinearity. The advanced intelligent computing algorithms for forecasting should consider the proper parameter determinations for achieving optimization process. For this we need, new generation research areas like Machine learning (ML), and Artificial Intelligence (AI) to enable the efficient integration of distributed and renewable generation at large scale and at all voltage levels. The modern research in the above areas will improve the efficiency, reliability and sustainability of the Smart grid.
The energy grid is one of the most complex infrastructures and requires quick decision-making in real-time, which big data and AI algorithms enable for utilities. Beyond grid analytics and management, AI’s applications in the renewable sector include power consumption forecasting and predictive maintenance of renewable energy sources. It further enables the internet of energy applications that predict grid capacity levels and carry out time-based autonomous trading and pricing. Future smart power systems need the intelligent field devices to help in the implementation of effective control mechanisms and protection schemes. The researchers should focus on development of human and machine interaction (HMI) system based on advanced AI and ML techniques in plant control and monitoring systems. An increase in the application of advanced automation by RE based research may lead to an eventual total shift from conventional energy sources to RE.
This Research Topic is entitled “Recent advances in renewable energy automation and energy forecasting.” It aims to bring out new methods and models that can help improve the operations on RE, improves grid security and can sustainable energy future. This Research Topic calls for novel and innovative research submissions that focus on application-oriented studies improving RE automation and Energy forecasting models.
Specific Research Topic of interest include (but are not limited to):
• Intelligent systems, solving methods, optimization, and advanced heuristics for improving operation of renewable integrated energy systems;
• Advanced control and operation methods to improve power system flexibility;
• Artificial intelligence and its application in RE automation;
• Modelling of Intelligent Field Devices;
• Smart energy, IoT and modern power systems modelling of RE operations;
• Energy-forecasting technologies;
• Deep learning and machine learning applications in smart grids;
• Modelling of data analytics for smart grid operations;
• Business models for different electricity market players;
The Smart Grid concept has been defined as the integration of the electrical generation, transmission and distribution networks and the data communications networks. As such, Smart Grid systems use digital and other advanced technologies to monitor and manage the transport of electricity from all generation sources to meet the varying electricity demands of end users. Reviewing the literature, we find, for example, that Praveena et al. proposed a new optimization method that combines Crow Search Algorithm and Harris Hawks Optimization to improve power quality and reduce harmonic distortion in microgrid systems with photovoltaic systems and multi-level inverters. By optimizing the performance of a recurrent neural network controller, the proposed approach outperforms other methods in enhancing power quality and reducing THD. The authors suggest its potential for application in other microgrid systems with different configurations to enhance renewable energy system performance.
Likewise, Kondaiah et al. projected a modified deep residual network (MDRN) for short-term load forecasting (STLF) that enhances accuracy and efficiency. Combining the residual network and convolutional neural network, the MDRN comprises four main components and outperforms other deep learning models, including (Long-Short Term Memory) LSTM model and (Contextual LSTM) CLSTM, an extension of the recurrent neural network LSTM, in forecasting accuracy and computational efficiency. The proposed MDRN can potentially enhance energy management systems’ accuracy and efficiency and may be useful in other time-series forecasting applications.
A major Research Topic within energy management systems, especially in the context of renewables being incorporated to the energy matrix and the enhancement of Smart Grid technologies, are the strong non-linear and non-stationary characteristics of power loads, wherein there is the need to develop short-term power load forecasting methods based on bald eagle search (BES) optimization variational mode decomposition (VMD), convolutional bi-directional long short-term memory (CNN-Bi-LSTM) network and considering error correction are developed to improve the accuracy of such load forecasting. In this context Wang and Li recommended a BES-VMD and CNN-Bi-LSTM method with an error correction mechanism for short-term power load forecasting. The method outperforms traditional methods, including ARIMA and CNN-Bi-LSTM without error correction, in accuracy and efficiency. The authors suggest its potential for other time-series forecasting problems.
With regard to the Smart Grid and demand response management ―a major Research Topic within the subject’s key areas of research―most consumers of energy are familiar with the grid and its hourly periods of high and low electricity rate of consumption. Thus, demand response programs seek to give consumers the opportunity to voluntarily reduce or shift their electricity consumption during peak hours by incentivizing them with lower rates or other forms of compensation. This way, demand response programs are being used by utilities and operators to balance supply and demand of electricity in an evolving market. In this context, Puppala et al. proposed a demand response program for a smart grid that incentivizes customers to reduce peak electricity demand through rebates. The framework outperforms other programs in reducing peak demand and improving customer satisfaction. It can scale up to support energy sustainability and grid stability. Along the same line, Vishnuvardhan et al. proposed a review of technical advancements and stability analysis in wind-penetrated power generation systems. The authors emphasize the significance of integrating renewable energy into the power grid and the challenges associated with high wind penetration. The review discusses recent advancements in power electronic devices, grid-forming inverters, and control strategies, as well as stability challenges like frequency and voltage stability. The review highlights the current state-of-the-art in wind-penetrated power generation systems and identifies the need for further research. The findings can inform policy and strategy for grid stability and wind energy integration.
The growing need to diversify the energy matrix and the electric power distribution sector has drawn electric utilities to pursue renewable energy projects that can advance the green energy agenda towards 2030 and beyond. In this context, Din et al. examine the public perception and willingness to pay (WTP) for renewable energy in Pakistan using the theory of planned behaviour (TPB). The study employs a survey questionnaire to investigate public attitudes, subjective norms, perceived behavioural control, and WTP for renewable energy. The results reveal that public attitudes and subjective norms have a significant influence on WTP for renewable energy, while perceived behavioural control has a weaker effect. The authors suggest that these findings can inform policy and strategy development to encourage renewable energy adoption in Pakistan and other developing countries.
An important Research Topic that is also at centre stage in the Smart Grid discussion is the potential impact of electric vehicles (EVs) on global energy systems. There is no doubt that the growing trend in the electrification of private and public transport vehicles accelerating at a somewhat rapid pace towards 2035, energy producers and distributors need to take into account and plan ahead for the potential impact of EVs on electricity demand. Thus, Dharavat et al. emphasized a review on the impact of plug-in electric vehicles (PEVs) on grid integration with distributed energy resources (DERs). They discuss the challenges and opportunities of PEV integration, such as the need for grid infrastructure upgrades and the potential for PEVs to provide grid services. The authors also explore strategies and technologies, including vehicle-to-grid systems and smart charging, to manage the impact of PEVs on the grid. The review provides an overview of the current state-of-the-art in PEV grid integration and highlights the need for further research.
High impedance faults (HIF) are abnormal conditions in power distribution networks, that occur when an energized primary conductor establishes an improper contact with a highly resistive surface. Therefore, the need to prevent such occurrences are also a major Research Topic of research. To accomplish this, HIF detection methods are employed considering the presence of non-linear loads in the distribution network. Along this line, Gogula and Edward recommended a new method for detecting high-impedance faults in a distribution network, where they propose to use a combination of discrete wavelet transform (DWT) and radial basis function (RBF) neural network. The authors highlight the difficulties in detecting such faults using traditional methods due to their low fault currents, hence they may go generally undetected for extensive periods of time, decreasing the distribution network’s reliability and effectiveness. Moreover, energized conductor on the ground present great risk of electric shock to anyone nearby. The proposed method of the authors is evaluated using simulations and outperforms traditional methods in accuracy. This study’s findings can inform the development of more effective fault detection methods for distribution networks, improving grid reliability and reducing downtime.
Bishwal et al. have proposed a differential evolution (DE) algorithm to optimize an IPIDF controller for frequency management in a networked power system with superconducting magnetic energy storage (SMES) and high-voltage direct current (HVDC) links. The DE algorithm finds the optimal values of the IPIDF controller’s parameters for stable frequency response in the system. The study shows that the optimized IPIDF controller provides better frequency response than traditional PI controllers, particularly in scenarios with power system disturbances. The study suggests that the DE-optimized IPIDF controller is a promising approach for managing frequency in networked power systems with SMES and HVDC links.
Research on methods and techniques to optimize the energy efficiency in industrial processes, are also an important area of development, seeking to optimize the use of energy and enhance process optimization in various industrial sectors. In this vein, Parekh et al. review different techno-managerial methods for optimizing energy use in chemical process industries, such as energy audits, pinch analysis, process integration, advanced process control, and machine learning. The authors discuss the benefits and limitations of each approach, and emphasize that combining them can lead to substantial energy and cost savings, stressing the importance of considering both technical and managerial factors when implementing energy optimization strategies.
Power systems expansion parallels the increase in load demand both from residential and industrial users, especially now that large influx of renewable energy is being incorporated into the power systems almost everywhere, to satisfy the increasing load demand. Regarding this, Chetan H. R. et al. explain that with its potential to generate power and compensate for a large portion of the load demand, wind generators make a major renewable power contribution. Chetan H. R. et al. used an optimized PI controller with a static synchronous series compensator (SSSC) to improve the transient stability of a wind power conversion system. The optimized PI controller enhanced damping and stability, making it a promising approach for power system disturbances.
Finally, Ravinder and Kulkarni highlight the importance of safeguarding smart meters’ integrity when they state that the intrusion detection in network traffic for crucial smart metering applications based on radio sensor networks is becoming very important in the Smart Grid area. The network’s structure for smart meters under investigation should consider important security factors. Thus, they report on research using machine learning to detect intrusions in smart meter data. Their results show that three algorithms, including random forest, can effectively detect intrusions. The study concludes that machine learning can enhance smart grid security and privacy.
SS and FY: Contribute the aims and objectives of the Research Topic, writing—review and editing, VK and AK: present the contributing articles of the Research Topic, original draft preparation. All authors listed have made a substantial, direct, and intellectual contribution to the work and approved it for publication. All authors contributed to the article and approved the submitted version.
The authors declare that the research was conducted in the absence of any commercial or financial relationships that could be construed as a potential conflict of interest.
All claims expressed in this article are solely those of the authors and do not necessarily represent those of their affiliated organizations, or those of the publisher, the editors and the reviewers. Any product that may be evaluated in this article, or claim that may be made by its manufacturer, is not guaranteed or endorsed by the publisher.
Keywords: renewable energy, machine learning, energy forecasting, control and optimization, real-time monitoring
Citation: Sahoo SK, Yanine FF, Kulkarni V and Kalam A (2023) Editorial: Recent advances in renewable energy automation and energy forecasting. Front. Energy Res. 11:1195418. doi: 10.3389/fenrg.2023.1195418
Received: 28 March 2023; Accepted: 04 May 2023;
Published: 10 May 2023.
Edited and reviewed by:
ZhaoYang Dong, Nanyang Technological University, SingaporeCopyright © 2023 Sahoo, Yanine, Kulkarni and Kalam. This is an open-access article distributed under the terms of the Creative Commons Attribution License (CC BY). The use, distribution or reproduction in other forums is permitted, provided the original author(s) and the copyright owner(s) are credited and that the original publication in this journal is cited, in accordance with accepted academic practice. No use, distribution or reproduction is permitted which does not comply with these terms.
*Correspondence: Sarat Kumar Sahoo, c2tzYWhvby5lZUBwbWVjLmFjLmlu
Disclaimer: All claims expressed in this article are solely those of the authors and do not necessarily represent those of their affiliated organizations, or those of the publisher, the editors and the reviewers. Any product that may be evaluated in this article or claim that may be made by its manufacturer is not guaranteed or endorsed by the publisher.
Research integrity at Frontiers
Learn more about the work of our research integrity team to safeguard the quality of each article we publish.