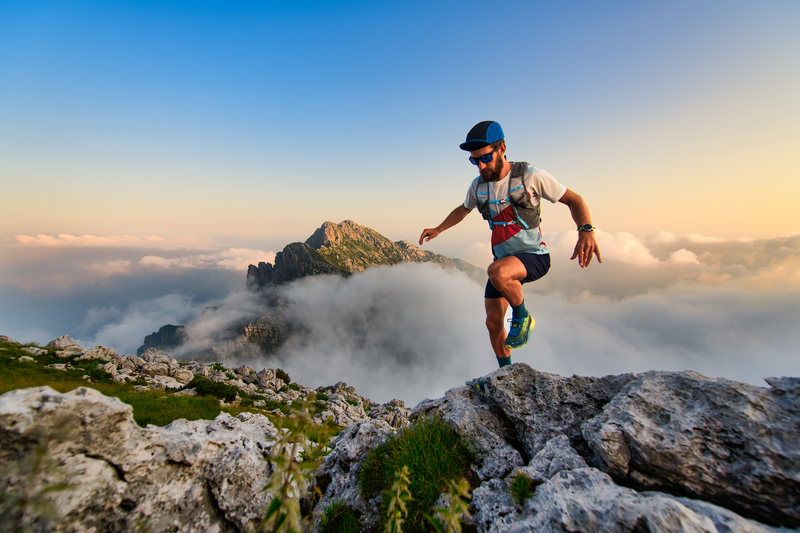
94% of researchers rate our articles as excellent or good
Learn more about the work of our research integrity team to safeguard the quality of each article we publish.
Find out more
REVIEW article
Front. Energy Res. , 15 February 2023
Sec. Wind Energy
Volume 11 - 2023 | https://doi.org/10.3389/fenrg.2023.1124203
This article is part of the Research Topic Climate Change Challenge: A Wind Energy Perspective View all 6 articles
A correction has been applied to this article in:
Corrigendum: Wind energy-harvesting technologies and recent research progresses in wind farm control models
Corrigendum: Wind energy-harvesting technologies and recent research progresses in wind farm control models
In order to sustain the overall competitiveness of the wind power industry, unrelenting focus is required on working toward the advancement of enabling technologies and research studies that are associated with wind farm systems. First, wind farm technologies that include various turbine generator systems coupled with different power transmission configurations have enormous impact in determining the quality of wind power production. In addition, modern wind farms are expected to implement robust power control algorithms to meet more advanced requirements of electricity generation. Accordingly, this study explores the statuses of wind energy harvesting technologies and wind farm control strategies by discussing their recent and future impact on transforming the wind power industry. Doubly fed induction generator (DFIG)-based wind energy harvesting technology is well-matured and has exhibited an excellent track-record in past and recent experiences, but its capability of being further scalable for large-scale power production is limited as it is largely incompatible with high-voltage power transmission networks. On the other hand, permanent magnet synchronous generator (PMSG)-based technology is making significant advancements to attain the maximum possible efficiency level in greatly facilitating larger scale power generation, although the construction of bulky and costly power transmission systems is required. In this regard, future technological advances in the wind farm industry are expected to reasonably optimize the design and cost of high-voltage power transmission systems. Similarly, an increasing number of research studies are introducing a number of power optimization-based control models to create an ideal integration of the aforementioned wind farm technologies so as to ultimately enhance the reliability of electricity production by maintaining the systems’ safety. Yet, additional work is still expected to be undertaken in the future for a more extended evaluation of the performances of many different control models under a similar environment.
As a part of ensuring successful improvements in global cumulative installations of wind power as shown in Figure 1, various systems of wind power technologies were proposed, developed, and used by researchers, manufacturers, and wind farm industries as the solutions for enhancing the extraction and transportation of onshore and offshore wind energy. In this regard, generator technologies and wind farm transmission systems have a considerable cumulative effect on onshore and offshore power production. More interestingly, wind generators and power transmission systems provide researchers and engineers with the options required for the significant achievement of wind power generation objectives, including a reduction in energy costs and maximization in wind power production. Consequently, the scale and cost of wind power production largely rely on the efficiencies, reliabilities, and configurations of the generators and power transmission systems (Cheng and Zhu, 2014; Biswas et al., 2021). In the case of generator systems, two main technologies are well-proven to be the leading candidates for onshore and offshore wind power applications: a partial-scale converter-based doubly fed induction generator (DFIG) system is widely popular for its better compatibility with onshore power generation (Mwaniki et al., 2017a), whereas a full-scale converter-based permanent magnet synchronous generator (PMSG)-based system is regarded as an attractive solution for offshore and multi-mega-scale wind power generation (Mohan and Vittal, 2018). Moreover, compared to the partial-scale converter DFIG-based wind farm, the reactive power capability of a full-scale converter PMSG-based wind farm can be significantly extended, and the grid-side converter in each configuration unit can provide the required reactive power locally. Regardless of its higher cost, full-scale converter-based wind energy conversion technology nowadays receives increasing recognition because of its superior performing efficiency and reliability, particularly in offshore wind farm applications (Chaithanya et al., 2019; Yaramasu and Wu, 2016).
FIGURE 1. Global onshore and offshore wind power installation trends (in GW) from 2012 to 2021 (I. Renewable Energy Agency, 2022).
Two options of electric power transmission systems can be implemented in interconnecting power generation systems (electric generators and power electronics converters) with wind farm substations, which are high-voltage alternating current (HVAC) and high-voltage direct current (HVDC). The HVAC transmission option is efficient and economical for the wind power industries for which the power substations can be built closer to the power-generating points. More specifically, when the distances between power-generating points and substations can be limited to shorter ranges (usually less than 50 km), the HVAC system shows excellent power transmission capability and economic benefits compared with the HVDC system for both onshore and offshore power applications (Wei et al., 2017). Nevertheless, substations are usually commissioned at points very far away from power-generating sites, particularly in the case of multi-mega-scale offshore wind power generation. Under this situation (for longer transmission lines and multi-scale offshore power generation), the HVAC option is not compatible with the power transmission application. The reason is that HVAC transmission cables are inherently characterized by a large capacitance per length, and thus, in addition to electrical current delivery, power transmission via extended cables of HVAC could also cause generation of capacitive currents. These capacitive currents are regularly rippling, and this significantly affects the transmission capability of electrical currents, and hence, the quality of power production. Consequently, excessive reactive power is required for the HVAC extended power transmission cables. This reactive power requirement can be met by making use of reactive shunt compensation; however, this results in the addition of extra expenses related to capital and operating costs (Machado et al., 2015).
Unlike HVAC-based configuration, the HVDC system is the standard solution in terms of power transmission capability and cost-effectiveness for applications of offshore wind farms that are commissioned far away (usually greater than 140 km) from power load centers. Moreover, the operation of HVDC-based power transmission configurations can be effectively scalable and no reactive power compensators are required at longer ranges. Different configurations of AC and DC power converter-connected HVDC transmission systems can be used for offshore wind farm applications (Kalair et al., 2016; Ryndzionek and Sienkiewicz, 2020). Currently, modular multilevel voltage source converter (MM VSC)-connected HVDC in AC parallel configuration, with 864 MW capacity, a voltage of
Furthermore, DC-based converter-HVDC parallel-connected transmission networks including three-level neutral point clamped (3L NPC) HVDC; DC converter-HVDC series connected transmission networks, such as solid-state transformer (SST)-HVDC; and pulse width modulator current source converter (PWM CSC)-HVDC were presented as potential alternatives for high-power transmission applications in recent literature reports. The DC-HVDC/3L NPC-HVDC design in parallel connection was proposed to simplify the complexity of the configuration of offshore power transmission substations by offering the benefit of cost. Series DC-connected designs (SST-HVDC and PWM CSC-HVDC) are also expected to further reduce the costs of bulky offshore wind power transmission substations (Yaramasu and Wu, 2016; Wei et al., 2017; Ryndzionek and Sienkiewicz, 2020; Peng et al., 2021).
On the other hand, research advances have achieved promising milestones in introducing potential strategies that could be practically implemented to enhance the operation of wind farms for maximizing and securing wind power production. These strategies mainly involve the application of optimization-based algorithms for wind farm control. In this context, wind farm control provides a cooperative strategy for the design and operation of the wind power plants, and it is a crucial development to alleviate the losses resulting from the turbine-to-turbine interaction within the plant. Many recent research works have revealed the improvement in wind farm performances, particularly in terms of power production, by using different optimization-based algorithms and models. In this work, the research results from implementing optimization-based wind farm control strategies that use feedforward, model-based closed-loop, and model-free closed-loop algorithms have been presented. For example, under the feedforward control approach, gradient-based optimization algorithms that include sequential quadratic programming, steepest descent, and conjugate gradient and heuristic algorithms that include genetic algorithm, particle swarm optimization (PSO) (Dursun et al., 2021), and artificial bee colony were implemented by using different optimization and evaluation models to increase wind farm power production, and varying results were reported.
With the model-based closed-loop approach, a model predictive optimization strategy was implemented by different researchers including Fontanella et al. (2021), and the output power of wind farms was claimed to increase with varying levels; the implementation of data-driven optimization methods, such as Bayesian optimization, knowledge-assisted deep deterministic policy gradient algorithm, and support vector machine (SVM) algorithm, also supported the achievability of increments in wind farm power productions. Furthermore, based on the model-free closed-loop approach, different optimization algorithms including simultaneous perturbation stochastic algorithm and nested extremum-seeking controller (NESC) were shown to have capabilities of maximizing wind farms’ output power. The model-based closed-loop approach is generally popular because it would help develop robust wind farm control designs with reduced complexity, cost, etc., in comparison to the other control strategies (Jain et al., 2021).
According to recent research trends, the increase in wind farms’ power production could be generally achieved by the implementation of different optimization-based algorithms along with various design optimization and evaluation models. The amount of increase in power production differs depending on various factors, which include the type of optimization algorithm that could be implemented, wind farm input control parameter that could be optimized, and models that could be used for optimization and evaluation. On the other hand, evaluating the performances of multiple wind farm optimization strategies under a single model environment is challenging; this could affect the acceptance of research reports and their practical implementation on real-world wind farms. In response to this challenge, the benchmark named FarmConners was recently introduced, and a project was also launched, aiming to determine the practical effect of wind farm control on loads of power systems. In its most recent report, the National Renewable Energy Laboratory (NREL) (Engaging Autopilot, 2021) revealed that it tested the practical implementation of the study model analyzed by Martínez-Tossas (2021), through the Composites Manufacturing Education and Technology (CoMET) facility, and validated that the ultimate objective of this study (to reduce the turbines’ cost) can be applied to real-world wind operation. Yet, more advanced works are still underway on the practical achievements of further wind farm control objectives that include design optimization, power reliability enhancement, and cost reduction.
Finally, more extended discussions of this work are organized under the sections to follow. Accordingly, Section 2 presents the two most popular wind turbine generator technologies (DFIG and PMSG systems); Section 3 explores technological trends in high-power transmission systems; Section 4 provides summaries of previous studies on different strategies of optimization-based wind farm control models; Section 5 presents brief assessments on the recent and future prospects of wind power systems control; and Section 6 summarizes the impact of relevant technologies and research studies on wind power production.
The most common device components used for energy conversion from wind to electrical power in a modern wind energy conversion system comprise a rotor with turbine blades, optionally a gearbox (it can be removed in gearless technologies), an electric generator, a power electronic converter, and a transformer, as shown in Figure 2. Designs of wind energy conversion systems can be classified into various notions on the basis of the type of generator, speed regulation capability, and strategy by which the aerodynamic power is restricted. In these notions of wind energy conversion systems, the power electronics plays entirely special roles and contributes to power ratings of the system with varying capacities. Two main wind energy harvesting technologies are widely adopted in modern wind energy industries. In the past decade, the DFIG technology designed with partial-scale power electronic converters was a prime choice in wind energy industries; however, the PMSG topology developed with a full-scale power electronic converter is recently receiving prominence due to the fact that it involves full power-regulation capability.
The DFIG (its configuration is shown in Figure 3) is the most recognized technology yet, and it has been installed widely since 2000. This wind energy harvesting technology uses both multiple- and single-gearbox systems; but the system with multiple gearboxes had widely gained acceptance until recent years, while single-gearbox technology is currently reported to have outstanding features in several research studies. The stator windings in a DFIG are directly tied to the grid by means of a transformer, and the rotor windings are tied to the power grid via a power electronic converter with approximately 30% power rating of the generator (Cheng and Zhu, 2014; Desalegn et al., 11912). In this technology, the frequency and the current in the rotor of the generator can be smoothly regulated by the power electronic converter, and hence, the rotational speed of rotor blades can be adjusted in an acceptable range to increase energy harvesting and minimize the mechanical stress. The comparatively lower rating of the power converter makes this technology preferable in terms of cost. Yet, the major limitations of this technology are the application of slip rings with poor reliability and inadequate power regulation capability with regard to grid or generator power fluctuations. This technology has a globally dominant share in onshore wind power generation, and it is less suitable for offshore wind power application.
Due to the fact that the power rating capacity for the power electronics converter in DFIG-based wind energy conversion technology is comparatively small, the two-stage voltage source converter (VSC) topology is widely recognized in this technology. Conventionally, two-stage VSCs are developed with a back-to-back design along with a common direct current (DC) link. A special feature of this back-to-back design is that it can help to implement complete power regulation under system operation. Moreover, this design has comparatively low structure complexity with a low component number, and this contributes to excellent efficiency and reduced cost of the DFIG-based system.
PMSG-based wind energy conversion technology (Figure 4) is another interesting system, and it is highly recognized in the most recently installed wind farm industries. By developing a full-scale power electronics converter and transformer to couple the power grid and the stator windings of the machine, the energy harvested by this wind energy conversion system can be entirely handled (Le et al., 12023). In comparison to the DFIG-based wind energy conversion design, the most important features that can be recognized are the absence of slip rings, uncomplicated or even unneeded gearbox, enhanced power and speed regulation on the broader scale, and superior grid compensation efficiency. However, increased stress and high cost of power electronics devices and increased power loss in the converter phase are the major disadvantages (Yaramasu et al., 2017). This design is usually not preferable in recently installed onshore wind energy conversion systems. On the other hand, PMSG-based wind power technology has been reported in multiple recent studies as a highly attractive candidate for recent and future offshore wind power applications because its converter device can be scalable to large-scale MW power.
Since the power electronics converter in the PMSG-based wind energy harvesting technology needs to embrace the full power harvested at large-scale megawatts, the two-stage VSC topology may be prone to maximum loss at this power scale. In addition, the cabling in the instance of low-voltage scales below 1 kV with increased current is a design limitation. In order to get along with increasing power rating, various multi-cell converter configurations have been developed for different synchronous generator technology-based systems. The multi-cell converter topology generally has the benefits of standard and robust low-voltage converter technologies. For instance, multi-cell two-stage VSC in parallel connection is the state-of-the-art option for PMSG-based wind energy-harvesting technologies exceeding 3 MW (Yaramasu and Wu, 2016). The advanced configurations of power electronics converters for offshore wind farm applications are presented in Section 3.
The performances of DFIG- and PMSG-based wind electric power generation systems are qualitatively summarized and compared in Table 1. Based on their design types of mechanical and electrical system alignments, different configurations of DFIG- and PMSG-based wind power technologies have been introduced to wind farms for harnessing onshore and offshore wind power. In some cases, the nature of the alignments between mechanical and electrical devices can serve to generally characterize these technologies as geared and direct-drive systems. For example, DFIG-based wind turbines are generally geared technologies as they may depend on either three-stage gearbox or single-stage gearbox design systems, while PMSG-based wind turbines can depend on either single-stage gearbox design or they are direct-drive (gearless) technologies. However, the recent state-of-the-art wind power plants generally rely on gearbox-based technologies, such as three-stage gearbox-based DFIG, single-stage gearbox-based DFIG, and single-stage gearbox-based PMSG. Direct-drive PMSG technology has recently been undergoing research and technological advancements, and reports have claimed its promising development for the application of offshore wind multi-mega scale power generation.
Table 1 provides comparative summaries pertinent to the performances and operation characteristics of DFIG- and PMSG-based wind power systems based on multiple requirements. These summaries are designed such that the listed requirements for comparisons generally apply to all configurations of DFIG-based systems (three-stage and single-stage gearbox technologies) and PMSG-based systems (single-stage gearbox and direct-drive technologies). In consideration of the cost of the system as one of the requirements for the comparisons, an average of the costs of two configurations (three-stage and single-stage gearbox) of DFIG technologies is compared with an average of the costs of two configurations (single-stage and direct-drive) of PMSG technologies. As shown in Table 1, DFIG-based systems are desirable for their lower general cost than PMSG-based systems, while PMSG-based systems are generally attractive solutions due to their suitability for multi-scale offshore wind power application.
The operational characteristics of DFIG- and PMSG-based wind power systems can be quantitatively evaluated based on research findings. Most research works were focused on the studies of DFIG- and PMG-based systems’ reliabilities, active and reactive power performances, etc. Here, the capability of power generation reliability for both generator systems is analyzed and compared based on the reports that were presented in the research works. In addition, active and reactive power performances and energy harvesting ranges of these two generator-based systems are considered for comparative discussion.
The junction temperature of power devices that correspond to DFIG- and PMSG-based systems of a 2-MW rated power capacity is shown in Figures 5A, B comparatively. For these two generator-based wind energy-harvesting technologies, the power devices’ thermal cycling is demonstrated within 0.2 s. According to Figure 5A, the performance of a partial-scale power converter in the DFIG-based system could deteriorate due to its thermal cycle that is characterized by a larger amplitude than the performance of a full-scale power converter in the PMSG-based system, whose thermal cycles are characterized by a smaller amplitude as shown in Figure 5B. This indicates that the reliability performance of the power device in a DFIG-based system could be severely affected due to a larger amplitude, which is associated with the system’s thermal characteristics. Consequently, advanced modeling and testing approaches should be proposed in helping adjust the reliability by establishing the power devices’ thermal behavior more effectively based on the wind power converter’s mission profile (Blaabjerg and Ma, 2017). A robust strategy has been presented by Ma et al. (2015), which resembles lenses with varying focal lengths used in photography. The wind power converter’s loading analysis and modeling are partitioned subject to some given time constants and various modeling methods and tools.
FIGURE 5. Junction temperature of power devices with generator systems of a 2-MW wind power converter: (A) DFIG system’s rotor side converter and (B) PMSG system’s machine side converter (Blaabjerg and Ma, 2017).
Furthermore, Figures 6A, B show active power control performances for DFIG- and PMSG-based marine current wind energy-harvesting systems under the variable speed control strategy. As shown in Figure 6A, the major advantage of the DFIG-based marine current system is its capability to supply fixed voltage and frequency within the range of
FIGURE 6. Active power performances of (A) DFIG-based wind energy harvesting technology and (B) PMSG-based wind energy harvesting technology (Benelghali et al., 2010).
As noted, the comparison of DFIG- and PMSG-based wind energy-harvesting systems is qualitatively shown in Table 1 by considering their annual power production capability as one of the criteria. Herein, Figures 7A, B show the annual energy-harvesting performances of DFIG- and PMSG-based marine current turbine technologies for the given ranges of tidal velocities, based on the research findings by Benelghali et al. (2010). Accordingly, Figure 7A represents the annual energy harvested by a DFIG-based marine current wind power system; the annual harvested energy corresponding to different tidal velocities, with DFIG technology, is calculated to be around 1,530 MWh/year according to the study. On the other hand, by the application of a PMSG-based marine current wind power system (Figure 7B), the cumulative generated power under the similar ranges of tidal velocities is reported to be about 1,916 MWh/year by the same study. As noticed, there is nearly a 25% difference in the power produced over a year between these two power systems, and this difference can be further extended when using a larger turbine system. The DFIG-based power system is characterized by its restricted speeds, and this is the reason for the reduction in its annual power production compared to the full-scale PMSG-based system. Meanwhile, according to a study by Fischereit et al. (2015), the relationship between tidal currents and wind speed was quantified such that the wind speed over a marine was observed to increase or decrease by around 0.2 m/s depending on the direction of the tidal flow with respect to wind direction.
FIGURE 7. Comparison of energy ranges harvested by (A) DFIG-based system and (B) PMSG-based system (Benelghali et al., 2010).
Under this section, the general comparison of power transmission systems of wind farms that are based on HVAC and HVDC technologies is shown in Table 2 based on capital expenditure. Parallel AC-connected HVAC, parallel AC-connected HVDC, parallel DC-connected HVDC, and series DC-connected HVDC transmission configurations are also comparatively shown in Table 3 based on main energy criteria, which include the operational condition of the system, cost of the system, and system’s capability for power scalability. Moreover, different HVDC-based state-of-the-art and more advanced technologies for the transmissions of offshore wind farms are shown in Table 4 on the basis of important requirements: the technology’s energy conversion quality, range of applications for recent offshore wind power generation, and the possibility of the development for future offshore wind power application.
TABLE 2. Comparison of HVAC and HVDC in terms of their capital costs (Li et al., 2021).
TABLE 4. Comparison of different converter–HVDC networks for offshore power transmission applications.
The general structure of offshore wind farm transmission with the application of HVDC and HVAC systems that include the components, such as power converter, transformer, offshore substation, and onshore substation, is shown in Figure 8. Here, the main focus is to evaluate the economic efficiencies of HVAC- and HVDC-based transmission systems by comparing the capital expenditures required for their respective components in commissioning offshore wind farms. Accordingly, the outlines of capital costs for the electrical components of HVAC and HVDC systems are shown in Table 2 based on the most recent study by Li et al. (2021). According to this study, the required capital expenditures are mainly allotted to cover the costs of transformers, onshore GIS switchgear, offshore substations, cable trench, shunt reactors, and submarine cable in the case of the HVAC system. In the case of the HVDC system, the capital expenditures are divided among the costs of cable trench, HVDC cable, onshore inverter, offshore rectifier, transformers, and additional offshore facilities. Based on the outlines of these costs for the components of HVAC and HVDC systems, it can be deduced that at a bigger power capacity and extended transmission line, HVDC presents more attractive cost benefits.
The HVAC system produces a huge amount of capacitive current that corresponds to the length of the transmission line. Here, the real power transmission capability may be determined with specified and reactive currents of a specific HVAC cable. With 50 Hz HVAC, the highest transmission length is around 140 km without compensation for reactive power. The HVDC system is characterized by considerable capability of power transmission compared to the HVAC system, particularly over an extended distance.
Moreover, the HVDC system offers additional advantages without being limited to cost and transmission capability by outperforming the HVAC system over an extended distance; for the vast offshore wind farm power transmission, the HVDC system also demonstrates lower transmission losses and its cables are commercially available (for larger voltages, HVAC cables are not available), and therefore, the HVDC system helps control excessive load as its power can be inherently adjusted. In general, HVDC is a more desirable solution than HVAC in the transmission of multi-mega-scale offshore wind power that is commissioned far away from the shore.
Different configurations of HVAC and HVDC systems are given in Table 3 based on various factors, which include operational condition, overall cost, and capability of the system’s configuration. The configurations include parallel AC-connected HVAC system, parallel AC-connected HVDC system, parallel DC-connected HVDC system, and series DC-connected HVDC system. Parallel AC-connected HVAC system-based configuration is characterized by its low complexity under the condition that the transmission distance between offshore and onshore sites is not long and is economically efficient under the same condition. However, this configuration shows serious disadvantages when the distance between offshore power and onshore substations is extended.
Parallel AC-connected HVDC configuration, which is based on the modular multilevel converter (MMC) topology, is the state-of-the-art solution to offshore wind farms with larger power capacity and longer transmission lines. However, the main disadvantage of this configuration is the high overall cost of power conversion components and the requirement of a bulk offshore substation. On the other hand, this configuration is characterized by its high capability of power scalability, and MMC topology with a power capacity of 864 MW, voltage of
Furthermore, parallel and series DC-connected HVDC configurations are designed to have reduced size and weight, and are recently under development for commercial use in offshore wind farms. The disadvantages that are being posed with the applications of other AC-connected HVDC configurations could be eliminated by DC-connected HVDC configurations. The discussions for different power converter–HVDC networks for offshore wind power transmission are given in Table 4 based on the criteria mainly related to energy conversion quality and range of applications in recent wind farms and possibility for future developments. The power converter–HVDC networks that are considered for discussions here include line-commutated current source converter (LC CSC)-based parallel medium voltage-alternating current (MVAC)-connected HVDC, two-level voltage source converter (2L-VSC)-based parallel MVAC-connected HVDC, multi-modular voltage source converter (MM VSC)-based parallel MVAC-connected HVDC, cascaded rectifier (diode)-based parallel MVAC-connected HVDC, three-level neutral point clamped (3L-NPC)-based parallel MVDC-connected HVDC, solid-state transformer (SST)-based series medium voltage direct current (MVDC)-connected HVDC, and pulse width modulator current source converter (PWM CSC)-based series MVDC-connected HVDC. Each of these HVDC-based power transmission networks is discussed in the following paragraph.
The application of LC CSC-based parallel MVAC connection with HVDC has shown well-proven performance in onshore wind power generation for about the last five decades. Nevertheless, this LC CSC-based network is inapplicable for offshore wind power, because it is incompatible with huge-scale power generation. On the other hand, the application of 2L back-to-back (BTB) VSC topology that is based on parallel MVAC connection with an HVDC system has been dominating over the onshore wind farm, and is less suitable for offshore wind farm due to its compatibility issue with multi-scale wind power generation. Modular multilevel (MM) VSC, based on parallel MVAC connection with an HVDC system, has been applied to offshore wind power transmission with various power capacity, voltage, and cable length levels. Currently, MM VSC is under further development for further improvement so that it would help ensure power maximization from offshore wind farms. At a wind power scale of 200 MW, cascaded rectifier (diode)-based parallel MVAC connection with HVDC was implemented, and it improved power production by 20% and reduced weight by 60% against traditional 2L-VSC-based parallel connection with HVDC according to study assessment by Blaabjerg and Ma (2017).
Furthermore, various offshore wind power transmission networks that are based on different converter technologies have been proposed in studies to enhance wind power conversion. For example, a three-level (3L) NPC-HVDC parallel MVDC-connected power transmission network has been developed in literature reports in response to the limitations posed while using a power transmission network that is based on the parallel MVAC-connected 2L VSC-HVDC. Series MVDC-connected transmission networks can also be implemented with a (3L) NPC converter (Peng et al., 2021). A series MVDC-connected (3L) NPC-HVDC system can be configured to have a relatively uncomplicated structure, higher power density, and lower cost than a parallel DC-connected system. In addition, SST- and PWM CSC-based series MVDC-connected HVDC configurations are undergoing promising development with attractive features, such as lower costs and weight than parallel MVAC and MVDC-connected networks such as 2L VSC-HVDC, MM VSC-HVDC, and (3L) NPC-HVDC (Wei et al., 2017). Further discussions on this section are given in Tables 3, 4.
Wind farms with larger capacities are required to perform under grid integration in order to ensure more reliable and efficient ways of generating wind power, which include reduced energy losses, maximized power production, lowered energy costs, enhanced power quality, and minimized loads on power systems as the ultimate objectives. Multiple recent studies have indicated that implementing control to individual wind turbines is not an effective strategy to achieve these objectives due to the fact that this strategy is unable to emphasize the complicated aerodynamic interactions across different turbines. Consequently, the strategy for wind farm control design has been aimed at enhancing controllers, which regulate and oversee the performance of a group of wind turbines from a supervisory level as shown in Figure 9 with a hierarchical method. The wind farm controller operates as the supervisory control system and comprises control levels that supervise production of power, system operation and maintenance, and power system services. The supervisory control system uses power grid requirement, energy costs, and turbine condition inputs to deliver the reference inputs to all turbines so that the desired operation can be ultimately met by the wind farm. The hierarchical control structure (Figure 9) enables robust and efficient control of turbines and wind farms by handling the turbines’ power output and affecting the power transfer in the electrical devices so as to achieve the aforementioned objectives.
The purpose of the discussion under this particular section is to assess the recent research results that have been reported in claiming to achieve the improvements in wind turbines/wind farm performances as per the aforementioned objectives by using different control approaches. In the research practices, two types of universal control approaches are usually proposed for the implementation of the required control objectives. A number of control strategies that rely on non-optimization- and optimization-based approaches are introduced by different researchers in aiming to achieve various wind farm control objectives, such as power maximization, power system load reduction, and grid services provision. In the most recent works, the optimization-based approach, which encompasses standard feedforward, model-based closed-loop, and model-free closed-loop control strategies has been consistently underlined to show outstanding performances compared with the non-optimization-based approach that uses conventional feedback control and feedforward control strategies. Therefore, this study summarizes research findings that have been recently reported in the efforts to realize some wind farm control objectives (primarily power gain/maximization) based on standard feedforward, model-based closed-loop, and model-free closed-loop control algorithms with the implementation of different wind farm optimization strategies and optimization and evaluation models while considering various input parameters, such as yaw angle, blade pitch angle, tip–speed ratio, and axial induction factor. Summaries of the studies are given in Sections 4.1–4.3 and Tables 5–7.
TABLE 5. Performances of feedforward control strategies with different optimization and evaluation models.
The research studies on wind farm optimization using the concepts of standard feedforward control are summarized in Table 5. This approach uses different control algorithms, optimization strategies, input parameters, and wind farm design optimization and evaluation models in enhancing wind farm performances so as to achieve the desired wind farm control objectives. Accordingly, the gradient-based control algorithms with different optimization strategies, such as sequential quadratic programming, steepest descent, and conjugate gradient, were proposed (Table 5) to maximize wind farm power production by adjusting wind turbines’ yaw angles and induction factors. For instance, Annoni et al. (2018) used sequential quadratic programing by implementing the Gaussian wake concept as both the optimization and evaluation model in increasing power production by adjusting the wind turbine’s yaw angle. Under this framework, power production was claimed to increase by 17% compared with the greedy control algorithm. On the other hand, Thomas et al. (2017), with a similar strategy (sequential quadratic programming), used the Jensen–Park model to optimize the yaw angle and layout of the wind farm, and power production was reported to increase up to 24% compared with the greedy control algorithm. Moreover, steepest descent- and conjugate gradient-based optimizations were proposed by Park and Law (2017) and Thøgersen et al. (2017) to adjust the axial induction factor and yaw angle (in the case of the steepest descent strategy) and the yaw angle (in the case of the conjugate gradient strategy) of wind farms, and the results indicated that the power production could be maximized by 7% and 7.5%, respectively.
As shown in Table 5, further standard feedforward optimization strategies that are based on heuristic algorithms (genetic algorithm, particle swarm optimization, and artificial bee colony) and game theory optimization were proposed by different researchers for optimizing various input parameters of wind farms. For instance, a genetic algorithm was proposed by Serrano González et al. (2015) to optimize wind turbines’ tip–speed ratio and blade pitch angle based on the Jensen–Park evaluation model, and it resulted in 1.5% increase in power production. The genetic algorithm was also used by Wang et al. (2018), aimed at maximizing power production by adjusting the axial induction factor and layout of the wind power system based on the Jensen–Park optimization and evaluation model, and it ended up increasing wind power production by 1%–2%. Another powerful heuristic algorithm-based standard feedforward strategy includes particle swarm optimization, which has been widely proposed in recent research works to achieve various wind farm control objectives. In Table 5, the results of several studies that implemented the particle swarm algorithm in optimizing wind farm performance are given. Accordingly, in Behnood et al. (2014), the tip–speed ratio and blade pitch angle were adjusted for a wind farm of 16 turbines based on the Jensen–Park model, and approximation of the power coefficient (as a function of the turbines’ tip–speed ratio and blade pitch angle) and 10.6% increase in power production were reported. The study results that were presented by Bo et al. (2016), Hou et al. (2016), and Gionfra et al. (2019) also indicated the robustness of particle swarm optimization in helping to maximize wind farm output power. In addition, the heuristic algorithm-based wind farm optimization strategy, artificial bee colony, was proposed to adjust the tip–speed ratio and yaw angle of wind turbines based on the Jensen–Park model and FLORIS for design optimization and evaluation in Abbes and Allagui (2016) and Quick et al. (2017) and output power increment of 4%–6% and 3% were, respectively, reported to be achieved.
The algorithmic game theory-based wind farm optimization strategy can be implemented to minimize load fluctuations of power systems in addition to maximizing output power by facilitating the adjustments of the yaw angle and axial induction factor. By using this strategy along with different wind farm performance optimization and evaluation models, some research works were introduced by researchers. Accordingly, in Gebraad et al. (2016), the yaw angle was optimized by using the FLORIS model, whereas output power performance was evaluated by using both SOWFA and FLORIS models, and the wind farm power production was reported to be improved by 1% with FLORIS and SOWFA optimization and evaluation models, and by 13% when the FLORIS platform was used as both the optimization and evaluation model. In addition, in van Dijk et al. (2021), the FLORIS model was used as both a single platform and in combination with the CC-Blade model for optimizing wind turbines’ yaw angle and evaluating wind farm output performance, where power production was reported to be maximized by 3.7% wind and a single model (FLORIS) and load fluctuation of power systems decreased by 18.7% with combined modes (FLORIS + CC-Blade). More work was also introduced in Herp et al. (2015), where the axial induction factor was optimized with the Jensen–Park model under different settings and resulted in 1.4%–5.4% increase in wind farm power production.
Additional works that are based on standard feedforward control strategies involving different algorithms and optimization and evaluation models for the design and performance of wind farms are given in Table 5 as extended summary from Table 5. A dynamic programming strategy was proposed by Rotea (2014) to optimize axial induction of a wind farm by applying the actuator disk model as the design optimization and evaluation standard. The result of this study shows that the power production was maximized by around 5% and wind farm load fluctuation was reduced up to 38% compared to the greedy algorithm strategy. In addition, a dynamic programming optimization strategy was implemented by Santhanagopalan et al. (2018) and Dar et al. (2016) by adjusting the tip–speed ratio through the RANS model and the yaw angle and axial induction factor through the enhanced Jensen–Park model to achieve output power increment by 0.8% and 4.5%–26.5%, respectively.
Additional standard feedforward optimization strategies that are based on different wind farm control objectives and with various wind farm design optimization and evaluation models were also presented in many research works, and the summaries of some of their parts are given in Table 5. Accordingly, the Nelden–Mead simplex algorithm was implemented with the simplified Ainslie model for optimization and evaluation power reference of wind farms in Kim et al. (2017), and the results showed an increase in the output power by 2.4% and reduction in the load fluctuation at upstream wind by 16.7%. According to a study presented in Bossanyi (2018), the generator torque, blade pitch angle, and yaw angle were adjusted and evaluated in aiming at minimizing the overall power losses and power system stress, and power production was saved by 2%. In addition, the research works based on field test optimization were discussed by Fleming et al. (2019) and Fleming et al. (2020) claiming a power gain of 2% and minimization of wake losses by 6.6%, respectively, by using FLORIS as the optimization model in adjusting the yaw angle. In addition, some studies that are specific to floating offshore wind farms were conducted based on blade pitch angle adjustments, and the results of these studies are given in the last row of Table 5. For instance, in the study by Kheirabadi and Nagamune (2019), the FLORIS benchmark was used as the optimization and evaluation model for maximizing power production by adjusting the floating offshore wind farm blade pitch angle under different settings, and 16%–54% increase in output power was reported. In addition, the adjustment of floating offshore wind farms under different design optimization and evaluation benchmarks: deep neural learning optimization model and LSTM evaluation model by Sierra-Garcia and Santos (2021) and the Jensen–Park optimization model and FarmFlow evaluation model by Rodrigues et al. (2015) were implemented; power production growth of 7%–21% and 4.4% were, respectively, reported.
Under this control approach, the research works that are based on model predictive optimization and data-driven optimization strategies are summarized in Table 6. Accordingly, a model predictive optimization algorithm was implemented by Heer et al. (2014), using the Jensen–Park platform as the optimization model and the SimWindFarm platform as evaluation in aiming to increase wind farm power production with adjustments of the blade pitch angle and tip–speed ratio. Under these two adjustments, the output power showed an increment of 0.4%–1.4%. On the other hand, the axial induction factor was adjusted in the study by Vali et al. (2016), making use of another wind farm performance optimization and evaluation platform (WFSim) based on the model predictive optimization strategy to achieve a power production growth of 3.8%. Furthermore, with the implementation of the model predictive optimization strategy through the application of the WFSim model for optimizing the axial induction factor and evaluating wind farm output power performance, additional works were presented by Vali et al. (2017) and Vali et al. (2019), reporting an improvement in power production by 2%–8% and 4%, respectively. On the other hand, larger increments of power production were reported to be achieved while using the SP-wind benchmark as the optimization and evaluation model for adjusting thrust coefficients (Goit and Meyers, 2015; Munters and Meyers, 2016; Munters and Meyers, 2017), and thrust coefficients and yaw angle rates (Munters and Meyers, 2018a; Munters and Meyers, 2018b) of wind farms under different control settings.
TABLE 6. Performances of model-based closed-loop control strategies with different optimization and evaluation models.
Different wind farm performance optimization and evaluation models (FLORIS and SOWFA, deep neural learning model using LES and CNN + LSTM, and DB-PC and extended Kalman filter model) were used to adjust the yaw angle (Doekemeijer et al., 2019a), tip–speed ratio and blade pitch angle (Yin and Zhao, 2021), and rotor rotational speed and axial induction factor (Abdelrahem et al., 1596); output power growths of 7%–11%, up to 38%, and 20%, respectively, were claimed to be achieved. On the other hand, various data-driven model-based optimization strategies (Bayesian ascent, Bayesian optimization, etc.) were implemented by researchers such as Park and Law (2016), Zhao et al. (2020), and Yin et al. (2020) by making use of different wind farm optimization models (Gaussian regression model, FLORIS, deep reinforcement learning, and support vector machine, respectively), and evaluation models (wind tunnel, SOWFA, WFSim, and FLORIS, respectively) in adjusting input parameters (yaw angle and blade pitch angle, yaw angle, axial induction factor, and yaw angle, respectively) to determine the levels of increments in the resulted power productions and reliability improvement. Accordingly, output power growths were reported to be achieved by 30.4%–33.2%, 4.4%, and 10%, and reliability was enhanced by 1.7%, respectively.
As shown in Table 7, model-free closed-loop control algorithm-based wind farm optimization strategies, which include multiple resolution-based simultaneous perturbation stochastic algorithm, game theory, gradient descent, and nested extremum controller, were implemented in optimizing wind turbines’ input parameters, such as axial induction factors and generator torque gains in order to evaluate the possible outcomes in generated power. Axial induction factors were optimized under different settings [simultaneous perturbation stochastic algorithm (Ahmad et al., 2014), game theory (Marden et al., 2013), and gradient descent (Gebraad et al., 2013)], and the outcomes were evaluated with the same model (Jensen–Park), and different levels of increments (32%, up to 25%, and 1%) in the output power were reported to be achieved. On the other hand, generator torque gains and yaw angle were adjusted by implementing the same optimization strategy (nested extremum-seeking controller) and using different evaluation models [SimWindFarm (Yang et al., 2015), UTD-WF (Ciri et al., 2016); Ciri et al., 2017), and wind tunnel (Campagnolo et al., 2016)]; varying levels of increase (1.3%, 10%/7.8%, and 15%) in the power production were claimed to be achieved.
Standardizing the effect of wind farm optimization strategies on loads of wind power plants would be highly helpful in applying the results of the research studies for their practical implementation in real-world wind farm industries. This is because the optimization of wind farm performance through research studies is modeled and evaluated by using different strategies (as the summaries of multiple studies in Table 5–7 indicate), and this still raises challenges in evaluating the performances of different optimization models under a unified environment. As a result, the technological approach for collaborated wind farm control is presently under advancement in multiple research institutions and industries. In particular, the FarmConners environment was introduced recently for the purpose of producing datasets as the basis for the evaluation of different control models in aiming to alleviate the barriers for wind farm control acceptance (Göcmen et al., 2020). In addition, the project was started by FarmConners to eliminate the challenges associated with the commercial application of wind farm control by collaborating a genuine assessment of the state-of-the-art of wind farm control in the FarmConners environment. Thus, a reliable evaluation of the efficiency of wind farm control models should be conducted so as to accurately determine the performance of wind farm controllers. Correspondingly, in order to maximize the acceptance of the wind farm control models and, ultimately, the FarmConners environment, FarmConners presents an extensive validation framework for wind farm control-oriented flow and load models, at which high-fidelity simulation generates (imitative dataset), wind tunnel experiments, and the field data from a fully operating wind farm in natural environmental conditions are drawn together (as shown in Figure 10).
Regardless of this technological progress by FarmConners, and even though recent studies demonstrated through simulations that the power production by wind farms can be maximized with collaborative control strategies, the practical confirmation on a real-world wind farm is still lacking. For instance, it raises the question of whether axial induction control can effectively result in increasing the wind farm’s annual power generation. Even for the more promising approach in simulation, wake-steering wind farm control, its direct practical applicability for a real-world wind farm has not been implemented yet. The considerable measurement noise and unpredictability is a challenge in wake-steering control, making it hard to evaluate the outcomes of measurement campaigns. Because of the unpredictability in models such as wind direction and aerodynamics, collaborative control strategies can become counterproductive over a number of periods, and this may not lead to enhancing the annual power output. However, even if these control concepts are unable to maximize the production of power, they may still be effective in reducing wind farms loads.
In general, wake steering is assumed to be a favorable control strategy for wind farm optimization. Yet, it is not fully convincing whether wake steering can practically be effective in helping to minimize the cost of energy. Turbines are not oriented to being yawed into the wind all the time. These will result in the addition of dynamic fatigue on various components of the turbines, which could result in increased costs for maintenance. Reports by several simulation studies demonstrated that the fatigue load on the blade and drive-train can be significantly improved. However, more advanced studies supported with extended experiments are required to accurately determine the effects of the wake-steering control strategy on the lifespan of the components of the turbines. If this control strategy proves to be effective, it could probably even help redesign various components of wind power systems (Andersson et al., 2021). On the other hand, the most recently published report by the National Renewable Energy Laboratory (NREL) has revealed that it has practically validated that the wake-steering wind farm control, which was based on the study model in Martínez-Tossas (2021), can be implemented to minimize the costs of wind turbines.
Many related studies were carried out to explore these problems and they came up with various indications. The study by Howland et al. (2019) proposed a wake-steering approach based on yaw misalignment that deflects wakes away from downstream turbines in order to maximize wind farm power production. The evaluation of this approach was conducted with site-specific analytic gradient ascent by using historical operational data; power increases of 7%–13% for wind speeds near the site average and 28%–47% for low wind speeds were observed. This study also reported that the wake steering minimized the power production variability of the wind farm by up to 72%. This work finally indicated that even though the wake-steering results demonstrated the potential to enhance the efficiency and predictability of power production, the resulting gains in annual power production were insignificant at the wind farm. Another study that focused on wind turbines under yawed conditions is a work by Rahimi et al. (2018), which claimed to present a significantly improved engineering model for the prediction of the loads in yawed flow based on the skewed wake effect. This work put particular emphasis on the contribution of the root vorticity to the azimuthal variation of induced velocity for the prediction of fatigue loads and determining the yawing moment; the new model was derived from computational fluid dynamics of three multi-megawatt wind turbines (each rated 5 MW) and two 10-MW turbines. Simulations were conducted by means of an actuator line model, whereas the proposed model was evaluated based on results from a free vortex wake code and actuator line model simulations for all wind turbines and different yaw angles. The results of this study finally indicated that the proposed model significantly improved the estimation of the azimuthal variation of the axial induction factor, and also considerably improved the prediction of the resulting variation in blade loads.
Furthermore, a dynamic wind farm wake modeling approach that was based on a bilateral convolutional neural network and high-fidelity LES data was proposed by Li et al. (2022); another approach that relied on a point vortex transportation model for yawed wind turbine wakes was introduced by Zong and Porté-Agel (2020). The former (Li et al., 2022) used a novel deep learning method, named the bilateral convolutional neural network (BiCNN), for accurate modeling of dynamic wind farm wakes based on flow field data generated by high-fidelity simulations; its discussion indicated that the developed machine learning-based wake model would capture the spatial variations of the dynamic wakes closely as high-fidelity wake models and would run as fast as low-fidelity static wake models. In addition, this model was shown to outperform high-fidelity numerical models that would be used for the same scenario. On the other hand, the latter (Zong and Porté-Agel, 2020) performed stereo particle imaging velocimetry measurements at multiple stream-wise locations behind a yawed wind turbine in order to study the formation mechanisms of the counter-rotating vortex pair; the results of this study showed that the counter-rotating vortex pair formed behind a yawed wind turbine would originate from the complex interactions between the hub vortex and stream-wise components of the blade tip vortices, which was observed to be essentially different from a yawed drag disk case where the hub vortex would be absent. Furthermore, the model by this study was considered to be the first model that would be capable of accurately simulating the wake deformation behind a yawed wind turbine.
As it has been generally indicated, the popular collaborative wind farm control approaches are based on optimization, which involves model-based closed-loop control, etc. In order to implement a model-based optimizer in a closed-loop, a state estimator needs to be used. However, the utilization of a state estimator will reduce the capability of the optimization approach in some way compared to the non-optimization-based noise-free full state feedback strategy. Recently, a very limited number of studies have been conducted by proposing the enhanced state estimators in optimizing input parameters of wind farms for enabling the provision of grid services.
This work presents a comprehensive review on the wind energy engineering approaches by incorporating research and technological issues in order to reflect the recent and future advances, challenges, and opportunities in wind power industry development. Wind power research and technological advancements have greatly contributed to the progress achieved by the wind power industry so far, and the future fate of this industry will also largely rely on the potential of wind power-related studies and technologies. Evidently, the energy-related policies and projections would be directed based on the status of ongoing research studies and technological innovations in the given energy field, which can also be a usual trend in the case of wind power installation. Based on this fundamental premise, advanced research studies and technological progresses that could potentially impact the recent and future onshore and offshore wind power developments have been discussed in this study in line with the directions (zero-emission goals) set by the United Nations Convention on Climate Change (UNCCC).
Numerous wind energy system-related recent research studies are largely inclined toward the technological developments of offshore wind power due to the reason that offshore power conversion technologies can be further scalable for high-power generation applications. Many HVDC-power transmission system-based converters are currently undergoing promising developments with various designs to achieve the weight reduction, cost optimization, and further enhancement of power conversion efficiency of offshore systems in the future. In general, based on the recent advances in the power transmission technologies, the future of the wind power industry seems be more dependent on the offshore wind farms than those onshore.
Moreover, the current advancements in automation-based research projects indicated a very promising future about the objective implementation of some optimization theories on real-world farms. Challenges with wind farm control modeling and evaluation tools were generally projected to be addressed toward the realization of fundamental wind farm optimization objectives. One of the objectives (wind turbines’ cost minimization) has already been reported to be practically validated based on the results of the recently proposed research model. These recent developments and more enhanced works that may be subsequently conducted by researchers and engineers on the wind farm optimal control are of great importance, which could partly contribute to enabling the wind power industry to see grand transition in the not-too-distant future.
All authors listed made a substantial, direct, and intellectual contribution to the manuscript and approved it for publication.
This work was supported by Bahir Dar University Institute of Technology, Bahir Dar Energy Center, and Wolaita Sodo University.
The authors declare that the research was conducted in the absence of any commercial or financial relationships that could be construed as a potential conflict of interest.
All claims expressed in this article are solely those of the authors and do not necessarily represent those of their affiliated organizations, or those of the publisher, the editors, and the reviewers. Any product that may be evaluated in this article, or claim that may be made by its manufacturer, is not guaranteed or endorsed by the publisher.
CC, component certification; CoMET, composites manufacturing education and technology; DFIG, doubly fed induction generator; FLORIS, flow redirection and induction in steady state; HVAC, high-voltage alternating current; HVDC, high-voltage direct current; MM VSC, modular multilevel - voltage source converter; NREL, national renewable energy laboratory; PMSG, permanent magnet synchronous generator; PSO, particle swarm optimization; 3L NPC, three-level neural point clamped; SST, solid-state transformer; PWM CSC, pulse width modulator-current source converter; SVM, support vector machine; NESC, nested extremum-seeking controller; SOWFA, simulator for wind farm applications; RANS, reynolds-averaged navier–stokes; LSTM, long short-term memory; WFSim, wind farm simulator.
Abbes, M., and Allagui, M. (2016). Centralized control strategy for energy maximization of large array wind turbines. Sustain Cities Soc. C25, 82–89. doi:10.1016/J.SCS.2015.11.007
Abdelrahem, M., Hackl, C., and Kennel, R. (1596). Robust predictive control scheme for permanent-magnet synchronous generators based modern wind turbines. Electron. (Basel) 10 (13), 1596. doi:10.3390/electronics10131596
Abo-Khalil, A. G., Eltamaly, A. M., Praveen, R. P., Alghamdi, A. S., and Tlili, I. (2020). A sensorless wind speed and rotor position control of pmsg in wind power generation systems. Sustain. Switz. 12 (20), 19. doi:10.3390/su12208481
Ahmad, M. A., Azuma, S., and Sugie, T. (2014). A model-free approach for maximizing power production of wind farm using multi-resolution simultaneous perturbation stochastic approximation. Energies 77 (9), 56245624–56465646. doi:10.3390/EN7095624
Almeida, A. O., Lopes, I. F., Almeida, P. M., Tomim, M. A., Passos Filho, J. A., and Barbosa, P. G. (2021). Series-DC connection of Offshore wind generating units - modeling, control and galvanic isolation. Electr. Power Syst. Res. 195, 107149. doi:10.1016/J.EPSR.2021.107149
Andersson, L. E., Anaya-Lara, O., Tande, J. O., Merz, K. O., and Imsland, L. (2021). “Wind farm control - Part I: A review on control system concepts and structures,” in IET renewable power generation (John Wiley and Sons), 15, 2085–2108. doi:10.1049/rpg2.12160
Annoni, J., Bay, C., Taylor, T., Pao, L., Fleming, P., and Johnson, K. (2018). Efficient optimization of large wind farms for real-time control. Proc. Am. Control Conf., 6200–6205. doi:10.23919/ACC.2018.8430751
Bay, C. J., King, J., Fleming, P., Mudafort, R., Martínez-Tossas, L. A., and Bay, C. (2021). Unlocking the full potential of wake steering: Implementation and assessment of a controls-oriented. Model.”. doi:10.5194/wes-2019-19
Behabtu, H. A., Coosemans, T., Berecibar, M., Fante, K. A., Kebede, A. A., Mierlo, J. V., et al. (2021). Performance evaluation of grid-connected wind turbine generators. Energies (Basel) 14 (20), 6807. doi:10.3390/en14206807
Behnood, A., Gharavi, H., Vahidi, B., and Riahy, G. H. (2014). Optimal output power of not properly designed wind farms, considering wake effects. Int. J. Electr. Power Energy Syst. 63, 44–50. doi:10.1016/J.IJEPES.2014.05.052
Benelghali, S., Benbouzid, M. E. H., and Charpentier, J. F. (2010). Comparison of PMSG and DFIG for marine current turbine applications. 19th Int. Conf. Electr. Mach. ICEM. doi:10.1109/ICELMACH.2010.5608118
Bhatt, P., Long, C., Wu, J., and Mehta, B. (2017). Dynamic participation of DFIG for frequency regulation in electrical power systems. Energy Procedia 142, 2183–2188. doi:10.1016/j.egypro.2017.12.586
Biswas, A. K., Ahmed, S. I., Akula, S. K., and Salehfar, H. (20212021). High voltage AC (HVAC) and high voltage DC (HVDC) transmission topologies of offshore wind power and reliability analysis. IEEE Green Technol. Conf., 271–278. doi:10.1109/GREENTECH48523.2021.00051
Blaabjerg, F., and Ma, K. (2013). Future on power electronics for wind turbine systems. IEEE J. Emerg. Sel. Top. Power Electron 1 (3), 139–152. doi:10.1109/JESTPE.2013.2275978
Blaabjerg, F., and Ma, K. (2017). Wind energy systems. Proc. IEEE 105 (11), 2116–2131, Nov. doi:10.1109/JPROC.2017.2695485
Bo, G., Yongqian, L., Jie, Y., Li, L., and Shun, K. (2016). A wind farm optimal control algorithm based on wake fast-calculation model. J. Sol. Energy Eng. 138 (2). doi:10.1115/1.4032297
Bossanyi, E. (2018). Combining induction control and wake steering for wind farm energy and fatigue loads optimisation. J. Phys. Conf. Ser. 1037 (3), 032011. doi:10.1088/1742-6596/1037/3/032011
Campagnolo, F., Petrovic, V., Schreiber, J., Nanos, E. M., Croce, A., and Bottasso, C. L. (2016). Wind tunnel testing of a closed-loop wake deflection controller for wind farm power maximization. JPhCS 753 (3), 032006. doi:10.1088/1742-6596/753/3/032006
Chaithanya, S., Reddy, V. N. B., and Kiranmayi, R. (2019). Performance evaluation of PMSG-based LFAC system for offshore wind power. Int. J. Ambient Energy 42 (14), 1643–1648. doi:10.1080/01430750.2019.161166110.1080/01430750.2019.1611661
Cheng, M., and Zhu, Y. (2014). The state of the art of wind energy conversion systems and technologies: A review. Energy Convers. Manag. 88, 332–347. doi:10.1016/J.ENCONMAN.2014.08.037
Chong, C. H., Rigit, A. R. H., and Ali, I. (2019). Wind turbine modelling and simulation using Matlab/SIMULINK. IOP Conf. Ser. Mater Sci. Eng. 1101 (1), 012034. doi:10.1088/1757-899x/1101/1/012034
Ciri, U., Rotea, M. A., and Leonardi, S. (2017). Nested extremum seeking control for wind farm power optimization. Proc. Am. Control Conf., 25–30. doi:10.23919/ACC.2017.7962925
Ciri, U., Rotea, M., Santoni, C., and Leonardi, S. (20162016). Large eddy simulation for an array of turbines with extremum seeking control. Proc. Am. Control Conf., 531–536. doi:10.1109/ACC.2016.7524968
Dang, L., Samb, S. O., and Bernard, N. (2020). “Design optimization of a direct-drive PMSG considering the torque-speed profile Application for Offshore wind energy,” in Proceedings - 2020 international conference on electrical machines (New York, NY: ICEM), 1875–1881, Aug. doi:10.1109/ICEM49940.2020.9271034
Dar, Z., Kar, K., Sahni, O., and Chow, J. H. (2016). Optimization of windfarm power by controlling the yaw angle using dynamic programming.
Desalegn, B., Gebeyehu, D., and Tamirat, B. (2022). Wind energy conversion technologies and engineering approaches to enhancing wind power generation: A review. Amsterdam: Heliyon. doi:10.1016/j.heliyon.2022.e11263
Desalegn, B., Gebeyehu, D., and Tamrat, B. (1191). Evaluating the performances of PI controller (2DOF) under linear and nonlinear operations of DFIG-based wecs: A simulation study. Heliyon 8 (12), e11912. doi:10.1016/J.HELIYON.2022.E11912
Doekemeijer, B. M., Fleming, P. A., and van Wingerden, J. W. (2019). A tutorial on the synthesis and validation of a closed-loop wind farm controller using a steady-state surrogate model. Proc. Am. Control Conf. 2019, 2825–2836. doi:10.23919/ACC.2019.8815126
Doekemeijer, B. M., van der Hoek, D. C., and van Wingerden, J. W. (2019). “Model-based closed-loop wind farm control for power maximization using bayesian optimization: A large eddy simulation study,” in Proceedings of the 3rd IEEE conference on control technology and applications (CCTA), New York, NY: 284–289. doi:10.1109/CCTA.2019.8920587
Dursun, E. H., Koyuncu, H., and Kulaksiz, A. A. (2021). A novel unified maximum power extraction framework for PMSG based WECS using chaotic particle swarm optimization derivatives. Eng. Sci. Technol. Int. J. 24 (1), 158–170. doi:10.1016/J.JESTCH.2020.05.005
Engaging Autopilot (2021). NREL explores automation to build better blades, lower costs, and transform wind technology manufacturing | news | NREL. https://www.nrel.gov/news/program/2021/automation-wind-manufacturing.html. (accessed Nov. 20, 2021).
Fischereit, J., et al. (2015). Influence of tides on the sea breeze in the German Bight: How much model complexity is needed? EGUGA 17 (2), 11807. [Online]. Available: https://ui.adsabs.harvard.edu/abs/2015EGUGA.1711807F/abstract (Accessed Jan 08, 2023).
Fleming, P., King, J., Dykes, K., Simley, E., Roadman, J., Scholbrock, A., et al. (2019). Initial results from a field campaign of wake steering applied at a commercial wind farm – Part 1. Wind Energy Sci. 4 (2), 273–285. doi:10.5194/WES-4-273-2019
Fleming, P., King, J., Simley, E., Roadman, J., Scholbrock, A., Murphy, P., et al. (2020). Continued results from a field campaign of wake steering applied at a commercial wind farm - Part 2. Wind Energy Sci. 5 (3), 945–958. doi:10.5194/WES-5-945-2020
Fontanella, A., Al, M., van Wingerden, J. W., and Belloli, M. (2021). Model-based design of a wave-feedforward control strategy in floating wind turbines. Wind Energy Sci. 6 (3), 885–901. doi:10.5194/WES-6-885-2021
Gebraad, P. M. O., Teeuwisse, F. W., van Wingerden, J. W., Fleming, P. A., Ruben, S. D., Marden, J. R., et al. (2016). Wind plant power optimization through yaw control using a parametric model for wake effects - a CFD simulation study. Wind Energy 19 (1), 95–114. doi:10.1002/WE.1822
Gebraad, P. M. O., van Dam, F. C., and van Wingerden, J. W. (2013). A model-free distributed approach for wind plant control. Proc. Am. Control Conf., 628–633. doi:10.1109/ACC.2013.6579907
Gionfra, N., Sandou, G., Siguerdidjane, H., Faille, D., and Loevenbruck, P. (2019). Wind farm distributed PSO-based control for constrained power generation maximization. Renew. Energy 133, 103–117. doi:10.1016/J.RENENE.2018.09.084
Göcmen, T., Kolle, K., Andersen, S. J., Eguinoa, I., Duc, T., Campagnolo, F., et al. (2020). Launch of the FarmConners wind farm control benchmark for code comparison. J. Phys. Conf. Ser. 1618, 022040. doi:10.1088/1742-6596/1618/2/022040
Goit, J. P., and Meyers, J. (2015). Optimal control of energy extraction in wind-farm boundary layers. J. Fluid Mech. 768, 5–50. doi:10.1017/JFM.2015.70
Goudarzi, N., and Zhu, W. D. (2013). A review on the development of wind turbine generators across the world. Int. J. Dyn. Control 1 (2), 192–202. doi:10.1007/s40435-013-0016-y
Haibo, Z., Florez, D., Saudemont, C., and Gruson, F. (2016). Control strategies of a dc based offshore wind farm with series connected collection grid. In 2016 IEEE International Energy Conference. New York, NY: ENERGYCON 2016. doi:10.1109/ENERGYCON.2016.7514062
Harzendorf, F., Schelenz, R., and Jacobs, G. (2021). Reducing cost uncertainty in the drivetrain design decision with a focus on the operational phase. Wind Energy Sci. 6 (2), 571–584. doi:10.5194/wes-6-571-2021
Heer, F., Esfahani, P. M., Kamgarpour, M., and Lygeros, J. (2014). “Model based power optimisation of wind farms,” in 2014 European control conference (New York, NY: ECC 2014), 1145–1150. doi:10.1109/ECC.2014.6862365
Herp, J., Poulsen, U. v., and Greiner, M. (2015). Wind farm power optimization including flow variability. Renew. Energy 81, 173–181. doi:10.1016/J.RENENE.2015.03.034
Hoffmann, M., Rathke, C., Menze, A., Hemdan, N. G. A., and Kurrat, M. (2019). Parallel operation of HVDC DRU and VSC converters for offshore wind farm connection: Technical and economic feasibility. IET Conf. Publ. 2019, CP751. doi:10.1049/CP.2019.0057
Hou, P., Hu, W., Zhang, B., Soltani, M., Chen, C., and Chen, Z. (2016). Optimised power dispatch strategy for offshore wind farms. IET Renew. Power Gener. 10 (3), 399–409. doi:10.1049/IET-RPG.2015.0176
Hou, P., Zhu, J., Ma, K., Yang, G., Hu, W., and Chen, Z. (2019). A review of offshore wind farm layout optimization and electrical system design methods. J. Mod. Power Syst. Clean Energy 7 (5), 975–986. doi:10.1007/s40565-019-0550-5
Howland, M. F., Lele, S. K., and Dabiri, J. O. (2019). Wind farm power optimization through wake steering. Proc. Natl. Acad. Sci. U. S. A. 116 (29), 14495–14500. doi:10.1073/pnas.1903680116
Heng, Z., Jing, C., Huan, Z., Xinmeng, L., Jiachen, L., Hanyu, C., et al. (2021). A multi-functional de-icing equipment using hybrid type parallel current source convertersE3S Web of Conferences, 256. doi:10.1051/e3sconf/202125602016
I. Renewable Energy Agency (2022). Renewable capacity statistics 2022 statistiques de capacité renouvelable 2022 estadísticas de capacidad renovable 2022. [Online]. Available: www.irena.org.
Jain, A., Chan, L., Brown, D. S., and Dragan, A. D. (2021). Optimal cost design for model predictive control. Proc. Mach. Learn Res. 144 (1–13). Accessed: Nov. 25, 2021. [Online]. Available: https://arxiv.org/abs/2104.11353v2.
Kalair, A., Abas, N., and Khan, N. (2016)., Comparative study of HVAC and HVDC transmission systems Renew. Sustain. Energy Rev. 59. C, 1653–1675, Jun. doi:10.1016/J.RSER.2015.12.288
Kanev, S. (2020). Dynamic wake steering and its impact on wind farm power production and yaw actuator duty. Renew. Energy 146, 9–15. doi:10.1016/J.RENENE.2019.06.122
Kanev, S. K., Savenije, F. J., and Engels, W. P. (2018). Active wake control: An approach to optimize the lifetime operation of wind farms. Wind Energy 21 (7), 488–501. doi:10.1002/WE.2173
Khani, N. G. (2021). Improving fault ride through capability of induction generator-based wind farm using static compensator during asymmetrical faults. Int. Trans. Electr. Energy Syst. 31 (11), 2021. doi:10.1002/2050-7038.13103
Kheirabadi, A. C., and Nagamune, R. (20192019). Modeling and power optimization of floating offshore wind farms with yaw and induction-based turbine repositioning. Proc. Am. Control Conf., 5458–5463, Jul. doi:10.23919/ACC.2019.8814600
Kim, H., Kim, K., and Paek, I. (2017). Model based open-loop wind farm control using active power for power increase and load reduction. Appl. Sci. 7, 1068. doi:10.3390/APP7101068
Kimura, N., Morizane, T., Iyoda, I., Nakao, K., and Yokoyama, T. (2018). Application of solid-state transformer for HVDC transmission from offshore windfarm. In 7th International IEEE Conference on Renewable Energy Research and Applications. New York, NY: ICRERA 2018, 902–907. doi:10.1109/ICRERA.2018.8566702
Le, X. C., Duong, M. Q., and Le, K. H. (12023)., Review of the modern maximum power tracking algorithms for permanent magnet synchronous generator of wind power conversion systems 16. Basel, Switzerland: MDPI. doi:10.3390/en16010402Energies1
Li, R., Yu, L., Xu, L., and Adam, G. P. (2020). Coordinated control of parallel DR-HVDC and MMC-HVDC systems for offshore wind energy transmission. IEEE J. Emerg. Sel. Top. Power Electron 8 (3), 2572–2582, Sep. doi:10.1109/JESTPE.2019.2931197
Li, R., Zhang, J., and Zhao, X. (2022). Dynamic wind farm wake modeling based on a Bilateral Convolutional Neural Network and high-fidelity LES data. Energy 258, 1248452022. doi:10.1016/J.ENERGY.2022.124845
Li, Z., Song, Q., An, F., Zhao, B., Yu, Z., and Zeng, R. (2021). Review on DC transmission systems for integrating large-scale offshore wind farms. Energy Convers. Econ. 2 (1), 1–14. doi:10.1049/ENC2.12023
Luqman, M., Yao, G., Zhou, L., Zhang, T., and Lamichhane, A. (2019). A novel hybrid converter proposed for Multi-MW wind generator for offshore applications. Energies (Basel) 12, 16. doi:10.3390/en12214167
Ma, K., Liserre, M., Blaabjerg, F., and Kerekes, T. (2015). Thermal loading and lifetime estimation for power device considering mission profiles in wind power converter. IEEE Trans. Power Electron 30 (2), 590–602. doi:10.1109/TPEL.2014.2312335
Machado, J., Neves, M. V., and Santos, P. J. (2015). “Economic limitations of the HVAC transmission system when applied to offshore wind farms,” in Proceedings - 2015 9th international conference on compatibility and power electronics (New York, NY: CPE), 69–75. doi:10.1109/CPE.2015.7231051
Mahmoud, M. M., Aly, M. M., and Abdel-Rahim, A. M. M. (2020). Enhancing the dynamic performance of a wind-driven PMSG implementing different optimization techniques. SN Appl. Sci. 2 (4), 19. doi:10.1007/s42452-020-2439-3
Marden, J. R., Ruben, S. D., and Pao, L. Y. (2013). A model-free approach to wind farm control using game theoretic methods. IEEE Trans. Control Syst. Technol. 21 (4), 1207–1214. doi:10.1109/TCST.2013.2257780
Martínez-Tossas, L. A. (2021). Numerical investigation of wind turbine wakes under high thrust coefficient. Hoboken, New Jersey: Wind Energy. doi:10.1002/we.2688
Moghadam, F. K., and Nejad, A. R. (2020). Evaluation of PMSG-based drivetrain technologies for 10-MW floating offshore wind turbines: Pros and cons in a life cycle perspective. Wind Energy 23 (7), 1542–1563, Jul. doi:10.1002/WE.2499
Mohan, M., and Vittal, K. P. (2018). “Modeling and simulation of PMSG-based wind power generation system,” in 2018 3rd IEEE international conference on recent trends in electronics, information and communication technology (New York, NY: RTEICT 2018 - Proceedings), 57–62. doi:10.1109/RTEICT42901.2018.9012620
Mousa, H. H. H., Youssef, A. R., and Mohamed, E. E. M. (2020). Optimal power extraction control schemes for five-phase PMSG based wind generation systems. Eng. Sci. Technol. Int. J. 23 (1), 144–155. doi:10.1016/J.JESTCH.2019.04.004
Munters, W., and Meyers, J. (2017). An optimal control framework for dynamic induction control of wind farms and their interaction with the atmospheric boundary layer. Philosophical Trans. R. Soc. A Math. Phys. Eng. Sci. 375, 20160100. doi:10.1098/RSTA.2016.0100
Munters, W., and Meyers, J. (2018). Dynamic strategies for yaw and induction control of wind farms based on large-eddy simulation and optimization. Energies 11, 177. doi:10.3390/EN11010177
Munters, W., and Meyers, J. (2016). Effect of wind turbine response time on optimal dynamic induction control of wind farms. J. Phys. Conf. Ser. 753 (5), 052007. doi:10.1088/1742-6596/753/5/052007
Munters, W., and Meyers, J. (2018). Optimal dynamic induction and yaw control of wind farms: Effects of turbine spacing and layout. J. Phys. Conf. Ser. 1037 (3), 032015. doi:10.1088/1742-6596/1037/3/032015
Mwaniki, J., Lin, H., and Dai, Z. (20172017). A concise presentation of doubly fed induction generator wind energy conversion systems challenges and solutions. J. Eng. (United Kingdom) 2017, 1–13. doi:10.1155/2017/4015102
Mwaniki, J., Lin, H., and Dai, Z. (2017). A condensed introduction to the doubly fed induction generator wind energy conversion systems J. Eng. (United Kingdom: Hindawi Limited). doi:10.1155/2017/2918281
Nouri, B., Goksu, O., Gevorgian, V., and Ejnar Sørensen, P. (2020). Generic characterization of electrical test benches for AC- and HVDC-connected wind power plants. Wind Energy Sci. 5 (2), 561–575. doi:10.5194/wes-5-561-2020
Ogidi, O. O., Khan, A., and Dehnavifard, H. (2020). Deployment of onshore wind turbine generator topologies: Opportunities and challenges. Int. Trans. Electr. Energy Syst. 30 (5). doi:10.1002/2050-7038.12308
Okedu, K. E., al Tobi, M., and al Araimi, S. (2021). Comparative study of the effects of machine parameters on DFIG and PMSG variable speed wind turbines during grid fault. Front. Energy Res. 9, 174. doi:10.3389/fenrg.2021.681443
Pan, L., and Shao, C. (2020). Wind energy conversion systems analysis of PMSG on offshore wind turbine using improved SMC and Extended State Observer. Renew. Energy 161, 149–161. doi:10.1016/J.RENENE.2020.06.057
Park, J., and Law, K. H. (2016). A data-driven, cooperative wind farm control to maximize the total power production. Appl. Energy 165, 151–165. doi:10.1016/j.apenergy.2015.11.064
Park, J., and Law, K. H. (2017). Cooperative wind turbine control for maximizing wind farm power 1 using sequential. Convex Program. 2 3.
Peng, X., Liu, Z., and Jiang, D. (2021). A review of multiphase energy conversion in wind power generation. In Renewable and Sustainable Energy Reviews, 147. Elsevier. doi:10.1016/j.rser.2021.111172
Quick, J., Annoni, J., King, R., Dykes, K., Fleming, P., and Ning, A. (2017). Optimization under uncertainty for wake steering strategies. J. Phys. Conf. Ser. 854 (1), 012036. doi:10.1088/1742-6596/854/1/012036
Rahimi, H., Martinez Garcia, A., Stoevesandt, B., Peinke, J., and Schepers, G. (2018). An engineering model for wind turbines under yawed conditions derived from high fidelity models. Wind Energy 21 (8), 618–633. doi:10.1002/we.2182
Rodrigues, S. F., Teixeira Pinto, R., Soleimanzadeh, M., Bosman, P. A. N., and Bauer, P. (2015). Wake losses optimization of offshore wind farms with moveable floating wind turbines. Energy Convers. Manag. 89, 933–941. doi:10.1016/j.enconman.2014.11.005
Rodriguez, P., and Rouzbehi, K. (2017). Multi-terminal DC grids: Challenges and prospects. J. Mod. Power Syst. Clean Energy 5 (4), 515–523. doi:10.1007/s40565-017-0305-0
Rotea, M. A. (2014). Dynamic programming framework for wind power maximization. IFAC Proc. Vol. (IFAC-PapersOnline) 19, 3639–3644. doi:10.3182/20140824-6-ZA-1003.02071
Ryndzionek, R., and Sienkiewicz, Ł. (2020). Evolution of the HVDC link connecting offshore wind farms to onshore power systems. Energies (Basel) 13 (8), 1914. doi:10.3390/en13081914
Samraj, D. B., and Perumal, M. P. (2019). Compatibility of electrical generators for harvesting extended power from wind energy conversion system. Meas. Control 52 (9–10), 1240–1251. doi:10.1177/002029401985818310.1177/0020294019858183
Santhanagopalan, V., Rotea, M. A., and Iungo, G. v. (2018). Performance optimization of a wind turbine column for different incoming wind turbulence. Renew. Energy 116, 232–243. doi:10.1016/j.renene.2017.05.046
Scott Semken, R., Polikarpova, M., Röyttä, P., Alexandrova, J., Pyrhönen, J., Nerg, J., et al. (2012). Direct-drive permanent magnet generators for high-power wind turbines: Benefits and limiting factors. IET Renew. Power Gener. 6 (1), 1–8. doi:10.1049/iet-rpg.2010.0191
Serrano González, J., Burgos Payán, M., Riquelme Santos, J., and González Rodríguez, Á. G. (2015). Maximizing the overall production of wind farms by setting the individual operating point of wind turbines. Renew. Energy 80, 219–229. doi:10.1016/J.RENENE.2015.02.009
Shi, G., Cai, X., Sun, C., Chang, Y., and Yang, R. (2016). All-DC offshore wind farm with parallel connection: An overview, IET Conf. Publ. 2016, CP696. doi:10.1049/CP.2016.0455
Shu, J., Zhang, B. H., and Bo, Z. Q. (2011). A wind farm coordinated controller for power optimization. IEEE Power Energy Soc. General Meet. doi:10.1109/PES.2011.6039552
Sierra-Garcia, J. E., and Santos, M. (2021). Deep learning and fuzzy logic to implement a hybrid wind turbine pitch control. Neural Comput. Appl. 2021, 1–15. doi:10.1007/S00521-021-06323-W
Simley, E., Fleming, P., and King, J. (2020). Design and analysis of a wake steering controller with wind direction variability. Wind Energy Sci. 5 (2), 451–468. doi:10.5194/WES-5-451-2020
Thøgersen, E., Tranberg, B., Herp, J., and Greiner, M. (2017). Statistical meandering wake model and its application to yaw-angle optimisation of wind farms. J. Phys. Conf. Ser. 854 (1), 012017. doi:10.1088/1742-6596/854/1/012017
Thomas, J. J., Gebraad, P. M., and Ning, A. (2017). Improving the FLORIS wind plant model for compatibility with gradient-based optimization. Wind Eng. 41 (5), 313–329. doi:10.1177/0309524X1772200010.1177/0309524X17722000
Tian, S., Campos-Gaona, D., Lacerda, V. A., Torres-Olguin, R. E., and Anaya-Lara, O. (2020). Novel control approach for a hybrid grid-forming HVDC offshore transmission system. Energies (Basel) 13 (7), 1681. doi:10.3390/EN13071681
Torres-Olguin, R. E., Garces, A., Molinas, M., and Undeland, T. (2013). Integration of offshore wind farm using a hybrid HVDC transmission composed by the PWM current-source converter and line-commutated converter. IEEE Trans. Energy Convers. 28 (1), 125–134. doi:10.1109/TEC.2012.2230535
Vali, M., Petrović, V., Boersma, S., van Wingerden, J. W., and Kühn, M. (2017). Adjoint-based model predictive control of wind farms: Beyond the quasi steady-state power maximization * *this work has been funded by the ministry for sciences and culture of the federal state of lower saxony, Germany as part of the PhD programme on system integration of renewable energies (SEE) and by the German ministry of economic affairs and energy (BMWi) in the scope of the WIMS-cluster project (FKZ 0324005). IFAC-PapersOnLine 50 (1), 4510–4515. doi:10.1016/J.IFACOL.2017.08.382
Vali, M., Petrović, V., Boersma, S., van Wingerden, J. W., Pao, L. Y., and Kühn, M. (2019). Adjoint-based model predictive control for optimal energy extraction in waked wind farms. Control Eng. Pract. 84, 48–62. doi:10.1016/J.CONENGPRAC.2018.11.005
Vali, M., van Wingerden, J. W., Boersma, S., Petrović, V., and Kühn, M. (2016). A predictive control framework for optimal energy extraction of wind farms. J. Phys. Conf. Ser. 753 (5), 052013. doi:10.1088/1742-6596/753/5/052013
van de Kaa, G., van Ek, M., Kamp, L. M., and Rezaei, J. (2020). Wind turbine technology battles: Gearbox versus direct drive - opening up the black box of technology characteristics. Technol. Forecast Soc. Change 153, 1199332020. doi:10.1016/J.TECHFORE.2020.119933
van Dijk, M. T., van Wingerden, J.-W., Ashuri, T., Li, Y., and Rotea, M. A. (2021). Yaw-misalignment and its impact on wind turbine loads and wind farm power output. J. Phys. Conf. Ser. 753, 062013. doi:10.1088/1742-6596/753/6/062013
van Dijk, M. T., van Wingerden, J. W., Ashuri, T., and Li, Y. (2017). Wind farm multi-objective wake redirection for optimizing power production and loads. Energy 121, 561–569. doi:10.1016/J.ENERGY.2017.01.051
Vijayaprabhu, A., Bhaskar, K. B., Jasmine susila, D., and Dinesh, M. (2004). Review and comparison of various types of generation using WECS topologies. IOP Conf. Ser. Mater Sci. Eng. 1177 (1), 012004. doi:10.1088/1757-899x/1177/1/012004
Wang, L., Cholette, M. E., Zhou, Y., Yuan, J., Tan, A. C. C., and Gu, Y. (2018). Effectiveness of optimized control strategy and different hub height turbines on a real wind farm optimization. Renew. Energy 126, 819–829. doi:10.1016/J.RENENE.2018.04.004
Wei, Q., Wu, B., Xu, D., and Zargari, N. R. (2017). “Overview of offshore wind farm configurations,” in IOP conference series: Earth and environmental science, 93. (1) doi:10.1088/1755-1315/93/1/012009
Yang, Z., Li, Y., and Seem, J. E. (2015). Optimizing energy capture of cascaded wind turbine array with nested-loop extremum seeking control. J. Dyn. Syst. Meas. Control 137 (12). doi:10.1115/1.4031593
Yaramasu, V., Dekka, A., Durán, M. J., Kouro, S., and Wu, B. (2017). PMSG-Based wind energy conversion systems: Survey on power converters and controls. IET Electr. Power Appl. 11 (6), 956–968. doi:10.1049/IET-EPA.2016.0799
Yaramasu, V., and Wu, B. (2016). “Basics of wind energy conversion systems (wecs),” in Model predictive control of wind energy conversion systems, 1–60. doi:10.1002/9781119082989.CH1
Yin, X., Zhang, W., Jiang, Z., and Pan, L. (2020). Data-driven multi-objective predictive control of offshore wind farm based on evolutionary optimization. Renew. Energy 160, 974–986. doi:10.1016/J.RENENE.2020.05.015
Yin, X., and Zhao, X. (2021). Deep neural learning based distributed predictive control for offshore wind farm using high-fidelity les Data. IEEE Trans. Industrial Electron. 68 (4), 3251–3261, Apr. doi:10.1109/TIE.2020.2979560
Zhang, B., Soltani, M., Hu, W., Hou, P., Huang, Q., and Chen, Z. (2018). Optimized power dispatch in wind farms for power maximizing considering fatigue loads. IEEE Trans. Sustain Energy 9 (2), 862–871. doi:10.1109/TSTE.2017.2763939
Zhang, H., Gruson, F., Flórez Rodriguez, D., Saudemont, C., Gruson, F., and Flórez Rodriguez, D. M. (2018). Overvoltage limitation method of an offshore wind farm with DC series-parallel collection grid. IEEE Trans. Sustain. Energy , IEEE 10 (1), 204–213. doi:10.1109/tste.2018.2829929
Zhang, J., Li, K. J., Liu, W., Sun, K., Liu, Z., and di Wang, Z. (2021). Grid side reactive power support strategy for MMC-HVDC connected to the wind farms based on unloading resistor. Electr. Power Syst. Res. 193, 1070102021. doi:10.1016/J.EPSR.2020.107010
Zhang, Z., Chen, A., Matveev, A., Nilssen, R., and Nysveen, A. (2013). High-power generators for offshore wind turbines. Energy Procedia 35, 52–61. doi:10.1016/j.egypro.2013.07.158
Zhao, H., Zhao, J., Qiu, J., Liang, G., and Dong, Z. Y. (2020). Cooperative wind farm control with deep reinforcement learning and knowledge-assisted learning. IEEE Trans. Ind. Inf. 16 (11), 6912–6921, Nov. doi:10.1109/TII.2020.2974037
Keywords: wind energy harvesting technologies, doubly fed induction generator system, permanent magnet synchronous generator system, high-voltage power transmission systems, optimization-based wind farm control models
Citation: Desalegn B, Gebeyehu D, Tamrat B and Tadiwose T (2023) Wind energy-harvesting technologies and recent research progresses in wind farm control models. Front. Energy Res. 11:1124203. doi: 10.3389/fenrg.2023.1124203
Received: 14 December 2022; Accepted: 26 January 2023;
Published: 15 February 2023.
Edited by:
Wen Zhong Shen, Yangzhou University, ChinaReviewed by:
Minh Quan Duong, University of Science and Technology-The University of Danang, VietnamCopyright © 2023 Desalegn, Gebeyehu, Tamrat and Tadiwose. This is an open-access article distributed under the terms of the Creative Commons Attribution License (CC BY). The use, distribution or reproduction in other forums is permitted, provided the original author(s) and the copyright owner(s) are credited and that the original publication in this journal is cited, in accordance with accepted academic practice. No use, distribution or reproduction is permitted which does not comply with these terms.
*Correspondence: Belachew Desalegn, YmVsYWNoZXdkZXNhbGVnbjc2QGdtYWlsLmNvbQ==
Disclaimer: All claims expressed in this article are solely those of the authors and do not necessarily represent those of their affiliated organizations, or those of the publisher, the editors and the reviewers. Any product that may be evaluated in this article or claim that may be made by its manufacturer is not guaranteed or endorsed by the publisher.
Research integrity at Frontiers
Learn more about the work of our research integrity team to safeguard the quality of each article we publish.