- Department of Mechanical and Industrial Engineering Technology, University of Johannesburg, Johannesburg, South Africa
The search for renewable, affordable, sustainable, and ecologically benign fuels to substitute fossil-based diesel fuels has led to increased traction in the search for biodiesel production and utilization in recent times. Biodiesel, a form of liquid biofuel, has been found to alleviate environmental degradation, enhance engine performance, and reduce emissions of toxic gases in transportation and other internal combustion engines. However, biodiesel production processes have been dogged with various challenges and complexities which have limited its expected progression. The introduction of data-based technologies is one of the remedies aimed at deescalating the challenges associated with biodiesel synthesis. In this study, the application of machine learning (ML) –based technologies including artificial neural network (ANN), response surface methodology (RSM), adaptive network-based fuzzy inference system (ANFIS), etc. As tools for the prediction, modeling, and optimization of the biodiesel production process was interrogated based on the outcomes of previous studies in the research domain. Specifically, we review the influence of input variables like alcohol: oil molar ratio, catalyst concentration, reaction temperature, residence time, and agitation speed on the biodiesel yield (output variable). The outcome of this investigation shows that the usage of ANN, RSM, ANFIS, and other machine learning technologies raised biodiesel yield to between 84% and 98% while the statistical verification shows that the Pearson correlation coefficient and coefficient of determination are close to 1. Going forward, more targeted and collaborative research is needed to escalate the use of innovative technologies for the entire biodiesel value chain to enhance production efficiency, ensure economic feasibility, and promote sustainability.
1 Introduction
Demand for affordable and efficient energy to meet rising consumption has continued to constitute a potent hindrance to achieving universal social, economic, and industrial development over the past decades. There are legitimate concerns that the current energy supply scenario is grossly inadequate and unsustainably deficient to meet the energy need of the industrialized global community. Fossil-based (FB) fuels, majorly coal, oil, and gas, have been the mainstay of the global energy supply for over 150 years. The total world energy consumption rose from 109, 858 TW-hours (TWh) in 2000 to 127, 0232 TWh, 151, 100 TWh, and 163, 709 TWh in 2005, 2015, and 2021, respectively (Ritchie et al., 2022). Over the same period, the global population rose from 6.1 billion in 2000 to 6.5 billion, 7.4 billion, and 7.9 billion in 2005, 2015, and 2021, respectively (UN, 2022). Increased population growth is among the reasons for the sustained rise in global energy consumption (Figure 1). As of 2019, about 84.3% of the global energy came from FB sources with oil, coal, and gas contributing 33.1%, 27%, and 24.3%, respectively, while 11.3% was supplied by renewable energy sources (Ritchie et al., 2022), as shown in Figure 2.
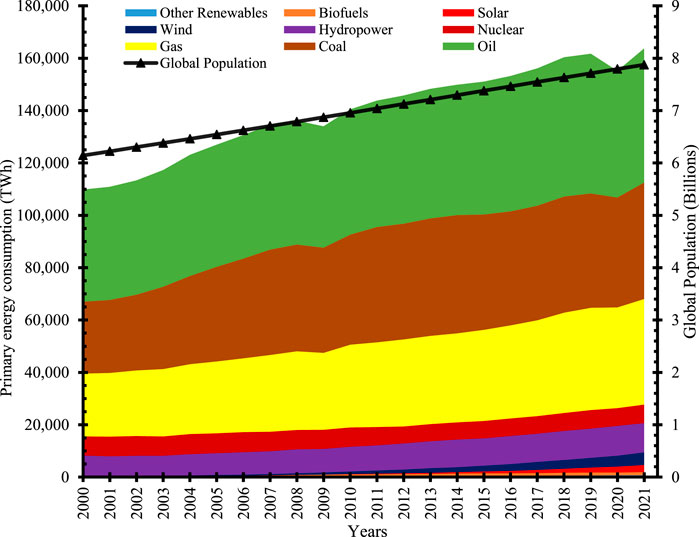
FIGURE 1. Global primary energy consumption (TWh) and population (Billions) (2000–2021). Adapted by the authors from (Ritchie et al., 2022; UN, 2022).
The process of exploration, exploitation, refining, and utilization of oil to generate liquid fuel for internal combustion engines has triggered environmental and health challenges. Diesel fuel is arguably the most commonly used fuel derived from petroleum. Diesel engines, also known as compression ignition (CI) are one of the most common reciprocating engines for diverse applications and offer better operational performance and strength when compared with spark ignition engines. Diesel engines are used for on-road, off-road applications, and non-automotive applications. Notable on-road application of CI engines includes light vehicles, commercial bus, trucks, etc., while power generation, marine applications, etc. Are examples of off-road applications. Generally, CI engines are used in transportation, industrial, construction, and agriculture equipment (Mejía et al., 2020). The fact that CI engines offer higher thermodynamic efficiency, better load-carrying capacity, and improved fuel economy when compared with spark ignition (SI) engines makes it a preferred choice for light and heavy-duty vehicles for transportation, agricultural machinery, and industrial applications. Consequently, the demand for diesel fuels was about 27.1 million barrels per day (mmb/d) rose to 30 mm b/d and has been predicted to become 31.6 mm b/d, 34.1 mm b/d, and 35.1 mm b/d by 2025, 2035, and 2040, respectively (Khan et al., 2019). In the same vein, there has been an increased in the use of diesel-fuel-powered engines particularly for power generation, construction, and agricultural sectors, and has increased the market share of diesel engines. Research and Markets (2019), a global research outfit predicted the global market value of diesel engines to reach USD 266.3 Billion in 2027 as against USD 291 Billion recorded in 2018.
Despite the enormous contributions of CI engines to social, economic, and industrial developments, increased avenues of utilization, and projections in the market value of CI engines, the use of CI engines results in enormous emissions that deteriorate air quality, impact human health, and exacerbate global climate. Heavy-duty vehicles and power-generating sets fuelled with diesel fuels emit carbon oxides, nitrogen oxides, particulate matter, sulfur oxides, and toxic gases which exacerbate environmental degradation (Cunanan et al., 2021). These have led to more studies aimed at improving the impact of petroleum diesel fuels and CI engines on the global ecosystem. One of the strategies to ameliorate the situation is the development and application of affordable, environmentally benign, and sustainable alternative fuels for CI engines.
The use of edible and inedible vegetable oils, alcohols, animal fats, and methane as an alternative to petroleum diesel has been fraught with many drawbacks. For example, the widespread utilization of neat vegetable oils as fuels for CI engines is hindered by high viscosity, low calorific value, low volatility, deterioration in storage, high price, and conflict with the food chain (Awogbemi et al., 2021a). Animal fats also suffer from inferior calorific value, poor combustion, and poor vaporization, and it requires preheating (Nicolici et al., 2018). Other drawbacks such as gum formation, lubricating oil congealing, incomplete combustion, injector clogging, atomization problems, high emission of toxic gases, and increased engine wear search for better alternative fuels inevitable (Tabatabaei et al., 2019; Awogbemi and Kallon, 2022a). The adaptation of biodiesel is seen as a panacea to the inadequacies experienced with the use of FB diesel fuels and vegetable oils as CI engines fuel.
Investigations into the generation, purification, and utilization of biodiesel have achieved traction over the past years. These have led to researchers and scholars adopting diverse strategies to enhance and simplify the production process. One such approach strategy is the application of innovative and advanced information technologies such as machine learning, modeling, optimization tools, and statistical approaches. This is more so because of the need to adequately understand the extremely complex chemical process and other competing factors involved in biodiesel production. The complexities involved in managing the competing process parameters cannot be achieved by try-and-error experimental procedures. The adaptation of appropriate technologies will save time, minimize cost, reduce material wastage, prevent repetitive experimentations, and tackle diverse eventualities.
The utilization of biodiesel and biodiesel blends as a low-cost and sustainable replacement of FB fuels in compression ignition (CI) engines has been investigated severally in recent years. The outcome of these studies revealed the multiple benefits derivable from running CI engines on blended and unblended biodiesel. Despite these outcomes, several other techniques have been adopted to improve the performance of biodiesel fuel in CI engines. Khan et al. (2020); Soudagar et al. (2019) investigated the effect of graphene oxide nanoparticle as additives to improve the performance and emission characteristics of CI engines. The outcome of their studies revealed that the brake thermal efficiency increased while the exhaust gas temperature, smoke, hydrocarbon, and carbon monoxide reduced by almost 35%. Other methodologies to improve the performance and emission characteristics of CI engines fuelled with biodiesel include blending with octanol and the use of combustion and emission strategies such as reactivity controlled compression ignition (Onuh et al., 2021; Wategave et al., 2021; Soudagar et al., 2022). ML technologies can also be applied to predict, model, and optimize engine performance and emission characteristics of CI engine fuelled by biodiesel.
In a different research, Verma and Sharma (2016), Sitepu et al. (2020), and Topare et al. (2022) examined the critical parameters that significantly influence biodiesel production by transesterification. They reported that the free fatty acid content of the feedstock, feedstock type, process temperature, type of alcohol, reaction time, catalyst type and concentration, the molar ratio of alcohol and oil, and agitation speed affect feedstock conversion efficiency and product yield. They, however, recommended the application of appropriate tools and technologies to optimize the effect of the identified parameters. Garg and Jain (2020), Selvaraj et al. (2019), and Ayoola et al. (2019) utilized machine learning (ML) technologies including response surface methodology (RSM) and artificial neural networks (ANN) to model, predict, and optimize process parameters for the transesterification of algal oil, waste cooking oil, and waste groundnut oil, respectively, to biodiesel. They concluded the robustness, superiority, accuracy, and capability of the RSM and ANN to predict and optimize production process parameters for improved biodiesel yield. The application of generic algorithm (GA) for the prediction and optimization of process parameters for biodiesel production was investigated by Betiku et al. (2015) and Srivastava et al. (2018) when they converted Shea butter oil and microalgae oil into quality biodiesel. They recommended more studies on the use of GA for obtaining optimal process parameters for biodiesel production.
To be able to appropriately fill the research gap and advance the trajectory of research in this field, the relevant question awaiting solutions is whether sufficient research has been accomplished to properly interrogate and situate the application of ML technologies in the biodiesel production research space. The aim of the study, therefore, is to examine the avenues for the application of ML technologies for the prediction and optimization of process parameters for sustainable biodiesel production. This is to attain optimal process parameters with the capability to stimulate cost-effective, fast, environmentally benign, and sustainable pathways for biodiesel synthesis. The outcome of the current intervention will deepen scholarship by delivering updated information that will stimulate the application of ML and other similar models in biodiesel research. This study will also expose more avenues for the utilization of ML in biodiesel research to achieve accelerated biodiesel production. This will further contribute towards an improved application of biodiesel, environmental sustainability, and green economy. The study is limited to the review of various ML technologies in predicting and optimizing process parameters for sustainable biodiesel generation. The application of mathematical and statistical backgrounds as well as the fundamental information and actual details relating to the modelling, prediction, and optimization of ML models are beyond the scope of this work but have been adequately presented elsewhere (Basile et al., 2017; Kubat, 2017; Mohri et al., 2018). Going forward, there is a need to escalate the application of statistical approaches, mathematical and numerical solutions, modelling, simulation, and optimization models, and other relevant innovative technologies for the enhancement of biodiesel production in all its ramifications. Multidisciplinary and collaborative efforts are needed for the intensification and advancement of cost-effective and sustainable biodiesel production pathways. The novelty of this study is derived from the investigation of the effect of the process parameters on biodiesel yield and to bring to the fore how ML technologies, mathematical techniques, and statistical tools can be applied to enhance biodiesel yield and adequately measure the influence of such methodologies on biodiesel yield.
2 Biodiesel production
Biodiesel is one of the prominent members of the renewable fuels family and a sustainable replacement for petroleum diesel fuel in CI engines. Due to its renewability, safe handling, easy production techniques, and conformity with the existing transport infrastructure of petroleum diesel biodiesel have gained wide acceptability among renewable fuel consumers. These factors have greatly influenced its production and consumption. To meet up with the global biodiesel demand, there has been an escalation in the quantum of biodiesel production. The worldwide biodiesel that was 38 billion litres in 2016 rose to 45.6 billion litres, 48.3 billion litres, and 53 billion litres in 2018, 2019, and 2021 respectively. The annual production has been estimated to reach 60.7 billion litres at the end of 2022 (Figure 3) (Statista, 2022). The negative production growth rate witnessed in 2020 was a result of the impacts of the global restriction occasioned by the outbreak of the COVID-19 pandemic (Awogbemi and Kallon 2022a). As shown in Figure 4, the global market size of biodiesel that was put at USD 32.09 Billion in 2021 and has been predicted to climb to about USD 127.13 Billion USD 161.64 Billion, and USD 189.7 Billion by 2025, 2028, and 2030, respectively (Precedence Research, 2022a). The expected growth in market size is propelled by increasing demand for renewable and clean fuel, especially biodiesel for power generation, and powering automotive, agriculture, and marine engines in global scale. The increased investment in biodiesel production and utilization is seen as feasible strategies to reverse the trend of the worrisome emission of greenhouse gases (Awogbemi and Kallon, 2022b).
2.1 Feedstock for biodiesel production
The choice of feedstock for biodiesel production is a major deciding factors that affect the production cost, infrastructure, conversion technique, conversion efficiency, the pump price, and sustainability of biodiesel production. For example, the feedstock accounted for about 75%–88% of production cost and is a key consideration in fixing the pump price of biodiesel (Ahmed et al., 2021). The selection of feedstock also greatly influences the type of catalyst, process parameters, intensification techniques, and purification methods (Athar and Zaidi, 2020; Sitepu et al., 2020; Mohiddin et al., 2021; Awogbemi and Kallon, 2022c). The use of non-edible feedstock, particularly waste cooking oil significantly reduces the cost of biodiesel production, ensures an appropriate strategy for disposing of waste vegetable oil, and averts pollution of aquatic and terrestrial ecosystems. On the other hand, employing edible vegetable oils as feedstock for biodiesel production impacts food security, sparks the food vs. fuel debate, and significantly leads to increased food prices. Algal biomass possesses high volatile fatty acid content, requires no land, water, and fertilizer for cultivation, easily convertible to biodiesel (Yaashikaa et al., 2022). Though the conversion of algae, microalgae, and seaweeds to biodiesel requires advanced technologies, and cannot be achieved at the household level, large-scale production and commercialization are still challenging (Liu et al., 2022; Taft and Canchaya, 2022). The feedstocks for biodiesel production can be categorized based on generations and edibility. Table 1 shows the examples, benefits, and drawbacks of different generations of feedstock for biodiesel generation.
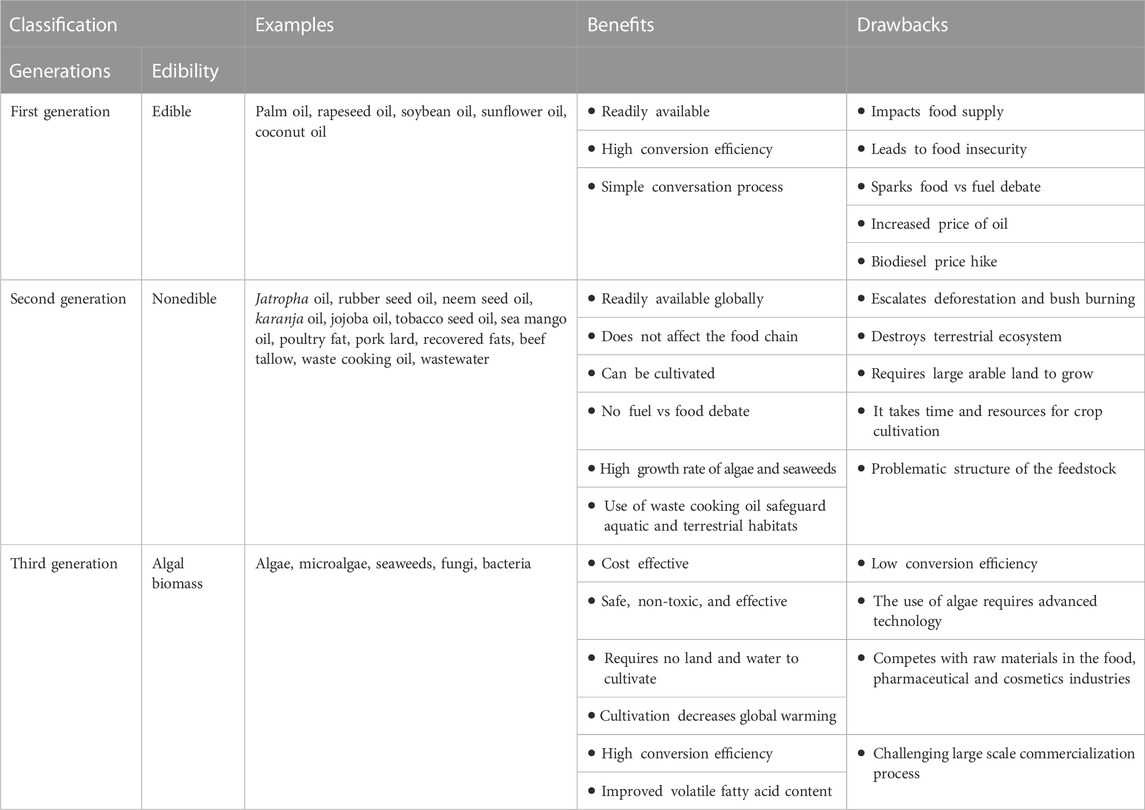
TABLE 1. Classification of feedstock for biodiesel production (Kesharvani and Dwivedi, 2021; Shaah et al., 2021; Zulqarnain et al., 2021).
2.2 Techniques for biodiesel production
Diverse pathways have been utilized to convert feedstock to biodiesel notable for direct use, micro-emulsification, pyrolysis, transesterification, and supercritical. These techniques are broadly categorized as physical techniques and chemical techniques (Awogbemi and Kallon, 2022a).
The direct use or dilution of vegetable oils as a replacement for FB diesel fuel in CI engines is the simplest avenue of alternative fuel utilization and was first used by Rudolph Diesel, a renowned German inventor of diesel engines, around the 1890s (Elghariani and Eshoul, 2021). Though this method is characterized by low production and capital costs, some of the properties of the vegetable oils make their use as direct fuel challenging, unsuitable, and impracticable. Some vegetable oils are highly acidic and have high viscosity, high density, low volatility, and reactivity of the unsaturated hydrocarbon chains. Therefore, when used in unmodified CI engines, vegetable oils result in incomplete combustion, engine deterioration, high carbon deposit, and congealing of the engine lubricating oil. Other shortcomings of the direct application of vegetable oils as a substitute fuel for unmodified CI engines include poor atomization, increased emission of pollutants, an accelerated rate of engine wear, high cost of engine maintenance, and poor engine performance (Dabi and Saha, 2019).
The micro-emulsification method is designed to remedy the drawback of the high viscosity of vegetable oils as CI engine fuel. During this process, appropriate solvents, surfactants or cetane improvers are mixed with vegetable oils and animal fats to achieve a clear, single-phase, and thermodynamically stable fluid as fuel. These emulsified oils form isotropic fluids which are stable, well dispersed, and with the required microstructures and droplet diameter. Notable solvents used for micro-emulsion include 1-butanol, 2-octanol, butanol, propanol, ethanol, hexanol, and methanol. Higher alcohols, sorbitan monooleate, octanol, and rhamnolipid are been used as surfactants while alkyl nitrates, nitroalkanes, and nitrocarbonates are commonly applied cetane improvers during micro-emulsification of vegetable oils (Mishra and Goswami, 2018). Emulsified vegetable oils and animal fats exhibit enhanced cold flow properties, improved stability, acceptable viscosity, and shorter ignition delay in CI engines (Abbaszadeh et al., 2016). However, there are reported cases of incomplete combustion, accumulation of carbon residue in the combustion chamber, thickening of the lubricating oil, and occasional blockage of the injector needle when products of micro-emulsification of oils were used in CI engines (Sanli et al., 2022).
Pyrolysis involves the amalgamation of thermal and chemical processes when feedstocks are converted into fuels and other useful products with the application of heat and in the presence or absence of some chemicals (catalysts) (Awogbemi et al., 2022; Nayab et al., 2022). Pyrolysis is one of the commonly used reforming techniques for the degradation of vegetable oils, animal fats, and other feedstocks by cracking their chemical bonds to generate renewable fuels with properties and structures comparable to petroleum diesel fuels. Biodiesel synthesized from the pyrolysis of vegetable oils demonstrates satisfactory physicochemical properties and improved engine performance. However, high production cost, complex and expensive infrastructural requirements, the low oxygen content of the fuels, and the production of chemically gasoline-like short-chain molecules are some of the factors limiting the application of the process (Su et al., 2022).
Transesterification is arguably the most widely used thermochemical technique for the conversion of triglycerides to biodiesel. During the process, 1 mol of triglycerides in oils and fats stoichiometrically reacts with 3 mol of alcohol to form 1 mol of alky ester and 1 mol of glycerol, as shown in Figure 5. The entire transesterification reaction proceeds in a three-step successive reversible reaction, as depicted in Figure 6. The produced crude biodiesel is purified to meet the required ASTM D6751 and EN 14214 standards while the glycerol is separated to prevent the formation of acetaldehyde or formaldehyde and other hazardous gases during combustion (Zhang et al., 2022). The glycerol can be utilized as fuel additives and raw materials for the production of bioethanol, cosmetics, livestock feeds, cosmetics, and other chemical products (Chilakamarry et al., 2022). The reversible reaction takes place with or without catalysts but under appropriate reaction parameters of temperature, pressure, reaction time, alcohol/oil ratio, and mixing speed of the reactants. Though methanol and ethanol are the most popular alcohols used in transesterification reactions, the lower cost and other physical features of methanol make it a preferred choice. For example, when compared with ethanol, methanol disperses more in homogeneous catalysts and reacts more efficiently with triglycerides (Awogbemi et al., 2021a). However, since the boiling point of methanol is 60°C, the maximum reaction temperature for a transesterification reaction involving methanol is usually 60°C to avert evaporation of methanol. The reaction time is indirectly related to the reaction temperature while the reaction takes place at atmospheric pressure, in most cases. The transesterification reaction is easily achievable under moderate process conditions and can be done domestically without the use of sophisticated infrastructure and technical manpower. However, the application of the transesterification process has been limited by poor mass transfer during the process, unpredictable product quality, generation of a high volume of wastewater, and involvement of multiple separation processes (Awogbemi et al., 2021a; Nayab et al., 2022).
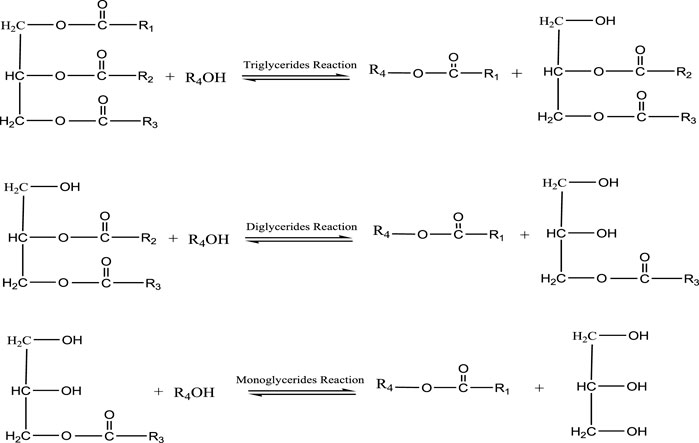
FIGURE 6. Three-step transesterification reaction equations (Awogbemi et al., 2021b).
The supercritical technique of biodiesel is a non-catalytic process of the biodiesel production process to replace the catalytic method of biodiesel production. During this process, compressed fluids that exhibit the properties of both gas and liquid are maintained and used above their critical temperature and pressure. Superfluids such as methanol, ethanol, and acetone maintained at 239.2 °C and 8.09 MPa, 240.9 °C and 6.14 MPa, and 235.1 °C and 4.70 MPa, respectively are used for biodiesel production (Awogbemi and Kallon, 2022a). At supercritical conditions, methanol witnesses an increase in density, solubility, mass transfer characteristics, and reduced polarity which results in better dissolution of the triglyceride in methanol and subsequent formation of biodiesel and glycerol (Farobie and Matsumura, 2017). The method requires no catalyst, consumes less energy, exhibits a high reaction rate, and requires easier product separation and purification processes. Supercritical techniques allow esterification and transesterification reactions to proceed simultaneously, can be applied to a wide range of feedstocks, and result in high conversion efficiency. Some of the drawbacks of the supercritical biodiesel production process include high process temperature and pressure, use of a high volume of alcohol, expensive production infrastructure, and possible denaturing of the products (Qadeer et al., 2021; Baydir and Aras, 2022).
2.3 Catalysts for biodiesel production
To further advance conversion efficiency and enhance the rate of biodiesel production, catalysts are often used. Though biodiesel can be synthesized without the use of catalyst and the cost of catalysts increase the total cost of biodiesel production, catalytic biodiesel production is advantageous and is mostly adopted. The use of catalysts reduces reaction time, improves conversion efficiency, and helps in lowering the reaction activation energy. Catalysts for biodiesel production are divided into three broad categories namely, heterogeneous catalysts, homogeneous catalysts, and enzymatic catalysts. The choice of an appropriate catalyst is dependent on the type of feedstock, free fatty acid (FFA) content, acid value, water content of feedstocks, etc. (Velusamy et al., 2021; Mukhtar et al., 2022).
Homogeneous catalysts are usually in liquid form and they maintain the same phase to the reacting materials during transesterification. This category of catalyst is used in converting triglycerides with high FFA content, acid value and is sometimes miscible with both glycerol and crude biodiesel. This partial miscibility with glycerol and biodiesel creates challenges in separating and recovery of the catalysts from the products (Tan S. X. et al., 2019). Homogeneous catalysts used for the transesterification process can either be acid or base catalysts. Notable examples of acidic homogeneous catalysts for transesterification reactions include H2SO4, H3PO4, HCl, C2HF3O2, and sulfonated acids. Examples of base homogeneous catalysts for transesterification reaction include NaOH, KOH, NaOH, NaOCH3, etc. (Changmai et al., 2020). Because homogeneous catalysts are usually in the same liquid phase as glycerol and biodiesel, there is the problem of separation and catalyst recovery. Several washing stages are involved to ensure complete catalysts removal, requires large volume of water for washing, and generate a large volume of wastewater in the process of purification. Similarly, homogeneous catalysts are relatively expensive and reuse is almost impossible (Rizwanul Fattah et al., 2020; Mukhtar et al., 2022).
Conversely, heterogeneous catalysts usually occur in a non-liquid state and are in a different phase from the reacting materials. They possess active sites with the reacting materials that ensure fast reaction and are subdivided into two types, namely acid heterogeneous catalysts and base heterogeneous catalysts (CaO, SrO, BaO, etc.). Generally, metal oxides, mixed metal oxides, sulfated metal oxides, zeolites, and some cation exchange resins have been successfully used as heterogeneous catalysts for biodiesel production (Gupta et al., 2020). Heterogeneous catalysts exhibit excellent catalytic activity under reasonable reaction conditions, high reusability, high recovery, easy to separate, low cost of purification, and generation of a low volume of wastewater. However, heterogeneous catalysts are only suitable with feedstocks with low FFA content and a high rate of soap formation (Changmai et al., 2020; Mandari and Devarai, 2021). Heterogeneous base catalysts can be derived from wastes such as chicken eggshell, quail eggshell, crab shell, crop residues, and food wastes to lower the production cost, ensure resource recovery and contribute to environmental sanitation and sustainability (Awogbemi and Kallon, 2022a; Awogbemi and Kallon, 2022b).
Greater research interest has been focused on the application of enzyme catalysts or biocatalysts for biodiesel production over the past few decades. Enzymatic catalytic biodiesel production involves using living and biological organisms to stimulate chemical reactions without the organisms being chemically affected in the process. The commonest and most widely used enzymes for biodiesel production is lipase. In most cases, lipases are invented from microorganisms such as Burkholderia cepacia, Thermomyces lanuginosus, Candida antarctica, Aspergillus niger, Anoxybacillus gonensins etc. During fermentation under specially monitored conditions (Toldrá-Reig et al., 2020; Altinok et al., 2023). Lipases are subdivided into intracellular lipases and extracellular lipases. Intracellular lipases involve utilizing complete cell of microorganisms or yeasts and are domicile within the cell-producing walls. Intracellular lipases are easy and economical to extract, and require minimal purification but suffer from low conversion efficiency and relatively low biodiesel yield. On the other hand, extracellular lipases are derived from microorganism broth, and subsequently separated and purified before application. Unlike intracellular lipase, the downstream processing cost of extracellular lipase is high and suffers from complex separation procedures (Toldrá-Reig et al., 2020; Chuengcharoenphanich et al., 2023).
Table 2 compares the examples, advantages, and drawbacks of homogeneous, heterogeneous, and enzyme catalysts in biodiesel production while Table 3 shows some examples of the application of various categories of catalysts for biodiesel generation.
3 Introduction to machine learning in biodiesel research
Machine learning (ML), also known as predictive analytics, is a domain of computer science dedicated to imitating and predicting the working of humans towards improving its accuracy. It is often referred to as a branch of artificial intelligence (AI) that empowers machines to learn from previous data and past experiences to discover recurring patterns and make informed predictions with the least possible interventions and involvements from humans (El Naqa and Murphy, 2015). It involves the utilization of algorithms and statistical models in the training of the computer system to evaluate supplied data and draw inferences for key decision-making with a view to progressively improving accuracy. The development and training of ML algorithms can be achieved through supervised learning, unsupervised learning, semi-supervised learning, or reinforcement learning. No doubt, ML is among the fastest growing applications of data science with increasing practical applications in the healthcare industry, financial sector, retail sector, social media, travel industry, and research (Anand et al., 2022). As a result of the continued relevance and applications of ML technologies in our contemporary world, the market share has continued to increase steadily. The global ML service that was worth USD 2.4 billion in 2019 became USD 15.47 in 2021, and has been predicted to rise to USD 58.26, USD 157.49, and USD 305.62 in 2025, 2028, and 2030, respectively (Figure 7) (Precedence Research, 2022a). The relevance of ML became more pronounced in the aftermath of the recent COVID-19 global pandemic which restricted movements and person-to-person contact. The driving factor behind the increased investment and market value is the utilization of ML and other data science technologies in decision making in retail, automotive, transportation, e-commerce, manufacturing, banking, diagnostic, therapeutic, cyber security, and education sectors.
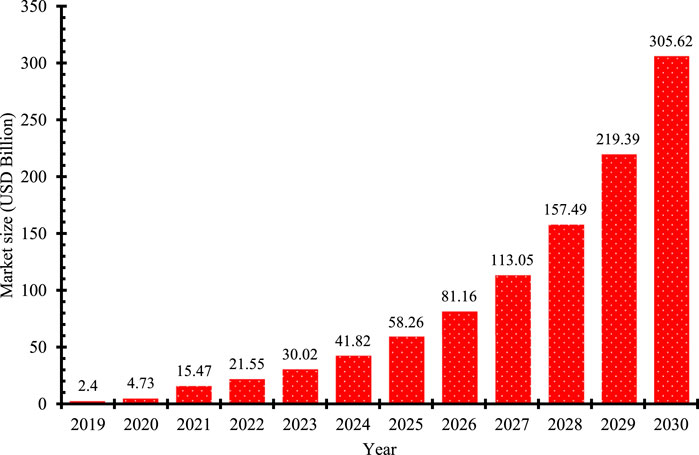
FIGURE 7. Machine learning service market share (Billion USD). Adapted from (Precedence Research, 2022b).
Major benefits of the adoption of ML technologies include its reliability, creativity, accuracy, and multidimensional applications. The design and development of models and algorithms have resulted into reduction in workload and time thereby encouraging faster and more reliable decision making. The use of ML algorithms has led to accuracy and efficiency in service delivery without human intervention. Many multifaceted tasks in most uncertain and inhuman environmental conditions are easily handled by ML technologies. ML algorithms have the capacity to handle large quantities of data, identify trends, and utilize the information to make informed decisions with optimal accuracy (Wuest et al., 2016; Wazid et al., 2022). However, for improved accuracy and reliability, ML requires enormous unbiased data sets to train on and sourcing for these data can be a herculean task. Another drawback for the adoption of ML technologies is the requirement of time and computer infrastructure to allow the algorithms to learn, understand, and develop enough capacity to correctly interpret the generated outcomes and render reliable decisions that suit the purpose. Though ML makes decisions after rigorous training, there is still high susceptibility of errors, especially when there are no sufficient wide-ranging data sets to train from. Such inaccuracies can lead to other chains of errors which may take long time to detect and rectify (Aliramezani et al., 2022).
Due to increased application of ML algorithms, diverse approaches including Artificial Neural Networks (ANN), Genetic Algorithms (GA), Linear regression, Random Forests regression (RF), and Support Vector Machines (SVM), etc. Have been exploited to monitor, predict, optimize, control, and take decisions in critical sectors of biodiesel research. ANN, a domain of AI, is a computational network constructed based on neurobiology networks and works like the human nervous system (Kukreja et al., 2016; Techopedia, 2022). Just like the human brain, ANN consists of neurons which are interconnected by some unidirectional communication channels called synapses (Figures 8A,B). The input data are produced by neuron as input signals and transferred to the network where they are amplified by their associated synaptic weights and released as output data. Generally, the ANN has three layers, as shown in Figure 9. The input layer receives data in form of signals from an external body and gives out its output to an intermediary nodes called the hidden layer. The hidden neurons are joined to the output neuron which gives out the result of the trained data in the output layer (Aghbashlo et al., 2021). Simply put, the input layer receives input signal which is trained and processed in the hidden layer and the outcome of the training is released to the output layer as the outcome of the entire network. Though there are many types of ANN models such as perception, feed forward neural network, multilayer perception, convolutional neural network, etc., but they all abide by the same working principle. ANN is arguably the most widely used ML algorithm due to some of its basic advantages which include faulty detection, simple adaptability, accurate approximation of continuous and non-linear function, capacity to perform multiple tasks simultaneously, huge data storage ability, and superb fault-tolerance properties. However, ANN is hardware dependent, network structure cannot be predetermined but achieved only through experience, trial, and error, and the duration of the network is unknown. Can approximate any continuous function to any desired accuracy (Walczak, 2019).
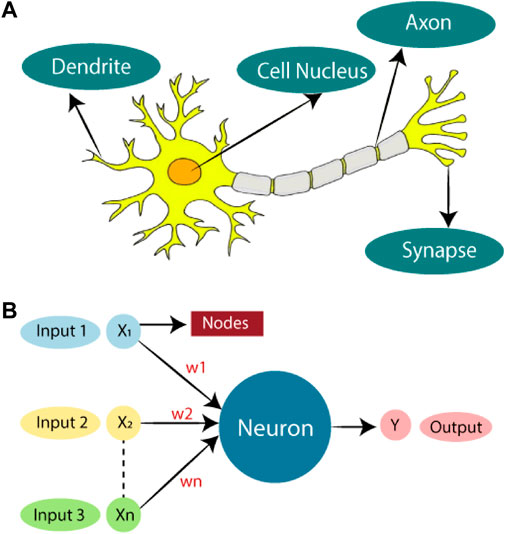
FIGURE 8. (A) Biological neural network (B) artificial neural network. Adapted from (Javapoint, 2021).
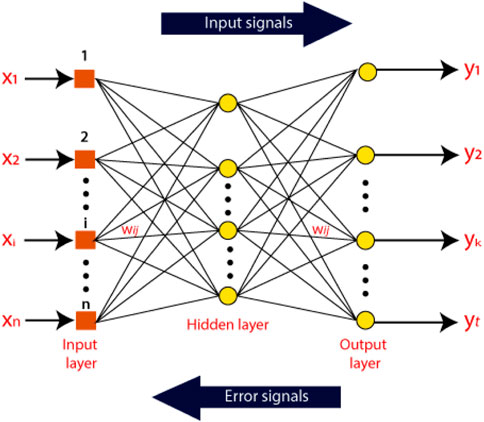
FIGURE 9. ANN structure. Adapted from (Javapoint, 2021).
In recent years, researchers have carried out meaningful investigations of the applications of ML technologies and other similar models for biodiesel research. Table 4 compiles some of the research outcomes on the applications of ML technologies in biodiesel research. Similarly, published review efforts by Xing et al. (2021), Aghbashlo et al. (2021), Gupta et al. (2021), and Suvarna et al. (2022) have shed some lights on the subject matter. However, only a handful of the aforementioned interventions has significantly touched on the application of ML for the modelling, prediction, and optimization of process parameters for biodiesel synthesis. Up till now, efforts to fill this obvious research gap has not yielded required results due partly to inadequate manpower, infrastructural deficit, and other challenges in the data science research space. This further justifies the current intervention.
The process for the application of ANN models usually involves data collection, data processing, building network architecture, training, model testing, and validation of results. Aghbashlo et al. (2021) presented the major steps to be followed in developing a typical ANN model for biodiesel research (Figure 10). The collection and preparation of data is arguably the most important factor in determining the attainment of on effective modelling process. In order to ensure the efficiency and integrity of the model, enough data needs to be collected and the data must be from trusted sources (Bali and Singla, 2022). The data collected must be analysed to enhance the training process and boost the integrity of the ANN model. The precision and dependability of the outcome needs to be authenticated to ensure it meets the expectations. Various statistical parameters including standard deviation, standard deviation of error, mean square error, root mean square error, square of Pearson correlation coefficient, etc. Have been established to measure the correctness and reliability of the model result. Table 5 compiles some of the statistical parameters commonly used to verify the accuracy and reliability of ANN and other ML models.
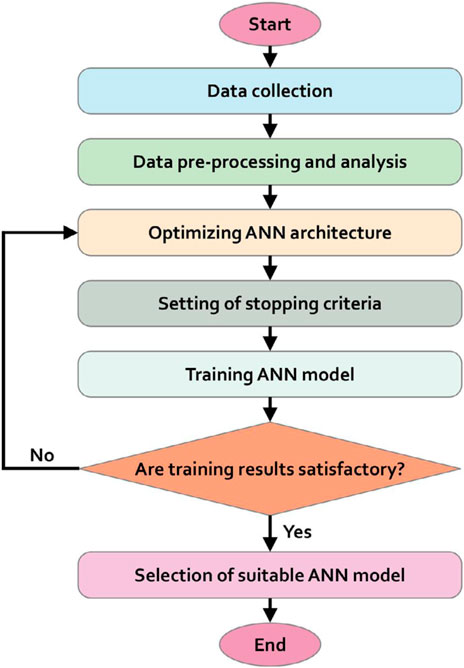
FIGURE 10. A typical flowchart for ANN model (Aghbashlo et al., 2021).
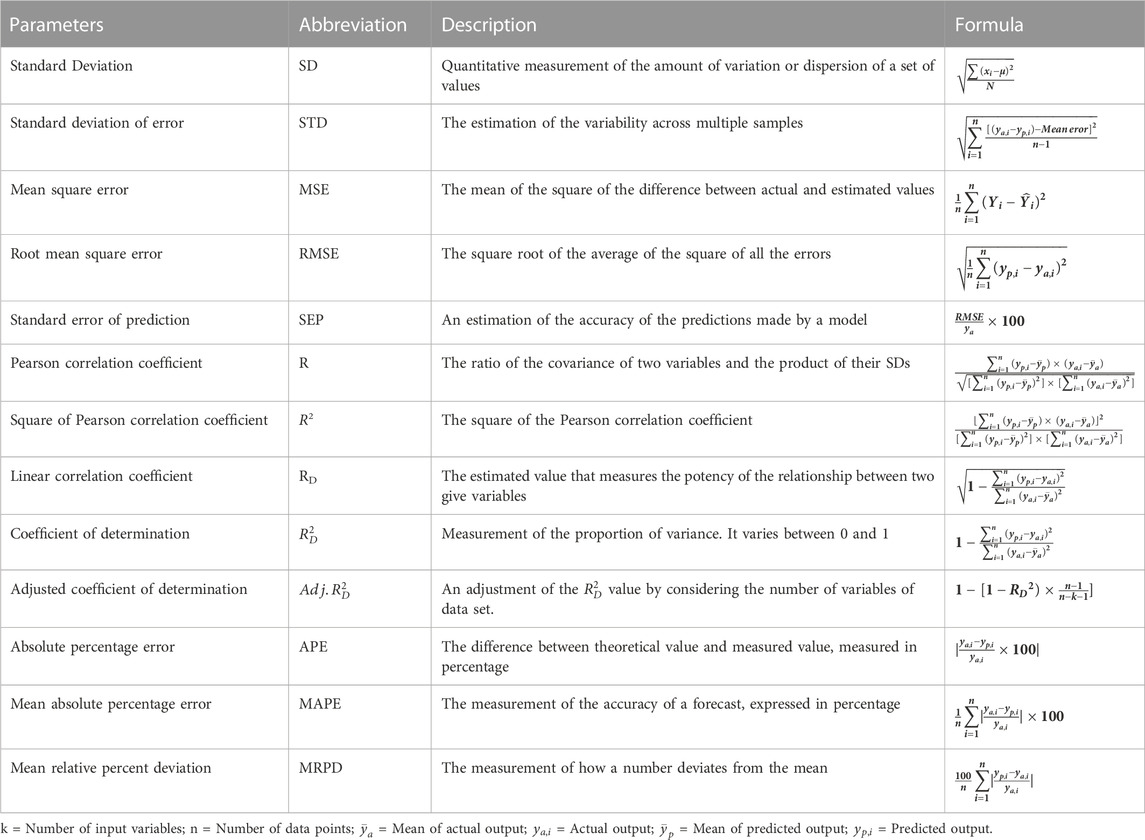
TABLE 5. Statistical parameters commonly used in ANN models (Khair et al., 2017; Ofoefule et al., 2019; Galvan et al., 2020).
4 Process parameters affecting biodiesel production
After making the choice of raw materials (feedstock, catalyst, and alcohol), production technique, and reactor the choice of process parameters is an important decision that must be made. The choice of production process parameters has a direct effect on the conversion efficiency, yield, and overall success of the biodiesel production. Factors such as alcohol to oil ratio, catalyst concentration/dosage, reaction temperature, and reaction time play significant roles in the success or otherwise of biodiesel synthesis. Making the right decision to ensure optimal conversion efficiency and product yield among these competing factors is not a straightforward task. The use of trial by error experimental methods to determine the optimal production process parameters is cumbersome, complicated, expensive, time-consuming, and often results in acute material wastage. The application of data-based ML technology has been adopted to make informed decisions that will guarantee value for money on biodiesel production. The input variables to the ANN model used are alcohol to oil ratio, catalyst concentration/dosage, reaction temperature, reaction time, stirring/agitation speed while the output variable is conversion efficiency or biodiesel yield.
4.1 Alcohol to oil ratio
The alcohol to oil ratio is an important factor influencing the conversion efficiency or biodiesel yield. During the transesterification process, the oil and catalyst are dissolved and dispersed in the alcohol. Alcohol provides the medium for the triglycerides to react with catalyst for the formation of biodiesel and glycerol. As shown in Figure 4, 1 mol of glycerides is needed to react with 3 mol of alcohol to generate 3 mol of biodiesel and 1 mol of glycerol. Therefore, to increase the biodiesel yield, a higher alcohol to oil ratio is essential (Okolie et al., 2022). However, very high alcohol to oil ratio can result in having excess methanol in the system thereby leading to higher production cost, difficult separation and purification process, low conversion efficiency, and ultimately poor biodiesel yield during transesterification reaction. Alcohol, especially methanol possesses a polar hydroxyl group which stimulate the emulsification of glycerol and biodiesel during transesterification reaction. The emulsification of the products facilitates the backward reaction thereby reducing conversion efficiency and biodiesel yield (Gupta and Pal Singh, 2022). Generally, methanol to oil ratio of 6:1 and ethanol to oil ratio of 9:1 are recommended as the optimal molar ratio for optimal biodiesel production (Musa, 2016; Abusweireh et al., 2022). However, depending on the choice of feedstock, catalyst type, and other process parameters, ANN and other statistical tools are employed to simulate the process towards arriving at the optimal alcohol to oil molar ratio to ensure optimal conversion efficiency and biodiesel yield.
4.2 Catalyst type and concentration/dosage
Though biodiesel can be generated in the absence of catalysts. The application of catalyst in biodiesel production by transesterification helps to reduce the activation energy, minimize energy consumption, lower reaction time, improve conversion efficiency and ultimately significantly increase biodiesel yield (Abdelmigeed et al., 2021; Awogbemi and Kallon 2022b). Various classes of catalysts i.e. alkali, acid, enzyme, biobased, heterogeneous, homogeneous, etc. Have been utilized for the transesterification of glycerides to biodiesel. The choice and concentration of catalysts greatly affect the quality and purity of product, production cost, ease of separation and purification, conversion rate, conversion efficiency, and biodiesel yield. For example, biodiesel yields of between 92% and 98% are achievable even with low grade and high FA content feedstock. Whereas, lower biodiesel yields are recorded with the same feedstock when base catalysts were used, under the same production conditions. Similarly, the application of heterogeneous catalysts results in higher conversion efficiency and biodiesel yield when compared with most homogeneous catalysts (Sitepu et al., 2020).
In most cases, higher concentration/dosage of catalysts results in higher biodiesel yield. However, the disproportionate application of catalysts can be counterproductive as it results in emulsification the and creation of highly viscous products mixture. This exacerbates product separation and purification, promotes saponification reaction, and substantially reduces biodiesel yield (Nayab et al., 2022). Transesterification reactions with inadequate quantity and concentration of catalyst proceed slowly, witness unreacted feedstock, generates low quality biodiesel and low biodiesel yield. On the other hand, excess dosage of catalysts in transesterification reaction leads to agglomeration, reduces mass and surface interaction among the reactants, and significantly impacts biodiesel yield (Mofijur et al., 2021; Xie and Li, 2023). While most researchers recommended between 2–10 wt% catalyst dosage will achieve between 75% and 100% biodiesel yield, the deployment of appropriate statistical tools to measure the reliability and validity of the ML models towards ensuring cost effective and optimal biodiesel yield (Teo et al., 2022; Xie and Li, 2023).
4.3 Reaction temperature
The temperature of a transesterification process plays a key role in the conversion of feedstock to biodiesel, biodiesel yield, and reaction kinetics. An increase in the reaction temperature reduces the viscosity and volatility of the reactants, encourages the miscibility of the reacting materials, and enhances the molecular interaction among the oil, alcohol and the catalyst. High temperature of the reacting medium also contributes to the thinning of the oil and enhances the dissociation of the biodiesel and glycerol (Bashir et al., 2022). However, excessive reaction temperature can also be counterproductive to the transesterification reaction. Apart from high energy cost, elevating the reaction temperature above the permissible threshold may result in saponification, complete hydrolysis of the esters into cognate acid and alcohol, and escalation of the backward reaction. The choice of alcohol should also be considered when determining the reaction temperature. Of the two alcohol commonly used in transesterification reactions, ethanol seems to tolerate higher temperatures than methanol. While a temperature of about 78°C is permissible with ethanol, 65°C is the recommended optimum reaction temperature for transesterification reaction involving methanol. Operating at temperature below 65°C will leads to slow reaction rate, poor conversion efficiency and low biodiesel yield while escalating the reaction temperature above 70°C will exacerbate methanol evaporation, lower the methanol to oil ratio, and hinder biodiesel synthesis reaction (Mathew et al., 2021; Stevanato et al., 2023).
The decision on reaction temperature must be taking along with other process parameters as the factors are interrelated and interdependent. In a separate research, Ahmad et al. (2019), Gimbun et al. (2013), and Al-Saadi et al. (2020) reported biodiesel yield of 98.6%, 96.9%, and 95.1% when they synthesized biodiesel by transesterification at reaction temperature of 59°C, 65°C, and 70°C, respectively. They concluded that other factors such as type of feedstock, catalyst, grade of feedstock, methanol to oil ratio, catalyst concentration, etc. Influenced the reaction temperature. One of the sustainable panacea to this conundrum is the deployment of ML models, simulation techniques and statistical tools to bring up an optimal reaction temperature for improved biodiesel yield.
4.4 Reaction time
Reaction time measures the entire duration of the transesterification reaction. It is a principal consideration in any chemical reaction as it is contingent on the type of catalyst, grade of feedstock, size of the reactor, quantity of the reactants, and reaction temperature (Bashir et al., 2022). Choosing adequate reaction time ensures sufficient product formation and the entire reaction is completed before within the reaction duration. The duration of the reaction should be such that there is adequate time for the diverse reacting materials to mix, interact and react with each other for product formation. Biodiesel yield has been observed to increase with reaction time as more time is allowed for the reactions to take place. In a separate study, Elkelawy et al. (2020) and Narowska et al. (2019) reported a corresponding increment in biodiesel yield with increased reaction time. They attributed this trend to the availability of enough time for the reactions to be completed. With prolonged reaction time, however, they reported a decline in biodiesel yield due to the attainment of equilibrium and activation of the formation of soap.
4.5 Mixing/agitation speed
The intensity of mixing of the reactants significantly affects the formation of the product in any chemical reaction. During transesterification, the reacting materials are mixed and agitated to ensure that are well dispersed, homogeneously distributed, and uniform reaction across the reactor. In cases where the triglycerides are completely soluble in alcohol, the reaction might occur only at the interface and not in all sections of the reactor. This often leads to slow reaction, unreacted materials at some part of the reactor, and poor yield. Mixing of the reacting materials ensures improved solubility of the oil in methanol, better reaction at all sections of the reactor, and enhanced product formation. Tabatabaei et al. (2019) experimented the effect of mixing speed of 200 rpm, 400 rpm, and 600 rpm on biodiesel yield while Likozar and Levec (2014) studied 100—600 rpm mixing speed. They reported that increased mixing speed leads to better product yield and that a speed of about 400 rpm is the optimal speed for maximum biodiesel yield. Higher mixing speed favours soap formation and higher energy consumption.
5 Application of machine learning technologies in optimization of process parameters
The application of ML technologies such as ANN, RSM, Adaptive Neuro-Fuzzy Inference System (ANFIS), etc. For predicting and optimizing biodiesel production process parameters has gained traction in recent years. This is due to their capability to predict and optimize the process parameters accurately. In a separate study, Tan Y. H. et al. (2019), Fangfang et al. (2021), and Farobie et al. (2015) applied ANN to model and predict biodiesel yield using Jatropha oil, waste cooking oil, and canola oil, respectively, as feedstock. They reported the predicted results agreed with the experimental results and the application of the ANN model eradicated material wastage and saves time. The experience of other scholars in the application of ANN to modelling, prediction, and optimization of the various variables that affect the generation of biodiesel shows that the technique is accurate, can model linear and non-linear situations, and has the capacity to store information on the entire network. However, ANN has been criticized for poor interpretability, not easily explainable, and demand lots of data for training, validation, and testing. Also, ANN is hardware dependent and requires high degree of computational skill and power. These drawback has necessitated the use of other tools.
Among several other modeling and optimization tools, RSM has gained prominence and wide acceptability. The RSM is a mathematical and statistical technique for the optimization of a process whose response or output is affected by various factors or variables. The dependent variables are the responses or output while the independent variables are the input or the predictor variable (Anggoro et al., 2022). The utilization of RSM technique for modeling, prediction, and optimization of biodiesel yield was investigated by Wahidin et al. (2018), Yesilyurt et al. (2019), and Anwar et al. (2018). They exploited RSM to model and optimize the process parameters in the transesterification of Nannochloropsis sp. Biomass, mustard seed oil, and stone fruit seed oil, respectively, to quality biodiesel. They reported R2 = 0.96912 and
The trajectory of research has move to comparison between the ANN, RSM, and other similar techniques. This is to help researchers decide and select better and cost effective techniques for prediction, modelling, and optimization with a view to further advancing biodiesel production research (Samuel et al., 2020). investigated and compared the application of RSM and Grey wolf optimizer (GWO) to model and optimize biodiesel yield during the transesterification process of waste sunflower oil. The authors reported that two the two techniques were reliable in predicting waste sunflower biodiesel yield, GWO was found more accurate and user friendly. Similarly, Fayyazi et al. (2015); Kolakoti et al. (2020) compared RSM and genetic algorithm (GA) and reported that the GA was faster, more efficient, and displayed 4.96% more improvement over the RSM technique. The desirability of Adaptive neuro-fuzzy inference system (ANFIS) integrated with GA and RSM were investigated by (Chizoo et al., 2022). The authors reported that ANFIS-GA demonstrated better predictive capability, faster, and cheaper process than RSM. In the same vein, Jisieike et al. (2023) and Ishola et al. (2019) in their separate studies, confirmed that though ANN and RSM possess the capability to model and predict biodiesel yield, ANFIS is more robust, accurate and faster than ANN and RSM.
The application of both ANN and RSM for predicting and optimizing biodiesel production process has gained traction among researchers. This might be due to their accuracy, robustness, and easy usability. Several authors have combined the two techniques to model and predict biodiesel yield. In their respective works, Maran and Priya (2015), Sarve et al. (2015), Prakash Maran and Priya (2015), and Rajković et al. (2013) compared the capability and the predictive efficiency of ANN and RSM using muskmelon oil, sesame oil, neem oil, and sunflower oil, respectively. They unanimously concluded that ANN model is more reliable, precise, and outperformed RSM models and therefore widely used in modelling biodiesel production. Other techniques such as extreme learning machine (ELM) and a support vector machine (SVM), extreme learning machine (ELM), artificial bee colony (ABC), etc. Have been applied to predict and model biodiesel production. For example, ELM was applied to model and optimize biodiesel generation from Ceiba pentandra oil by microwave irradiation assisted transesterification process. The ELM technique was found effective and resulted in high biodiesel yield (Silitonga et al., 2020). Faizollahzadeh Ardabili et al. (2018) also reported successful utilization of SVM-RSM and ELM-RSM approaches for the optimization of the biodiesel production process. The hybrid methodologies performed creditably and showed high estimation capability in optimizing quality biodiesel production process. The application of ABC algorithm for the optimization of biodiesel production from Mountain Almond oil was demonstrated by Rostami et al. (2016). The ABC algorithm technique demonstrated high accuracy in estimating the optimal process parameters for biodiesel production. The summary of the application of ML technology in the optimization of process parameters for biodiesel production is shown in Table 6.
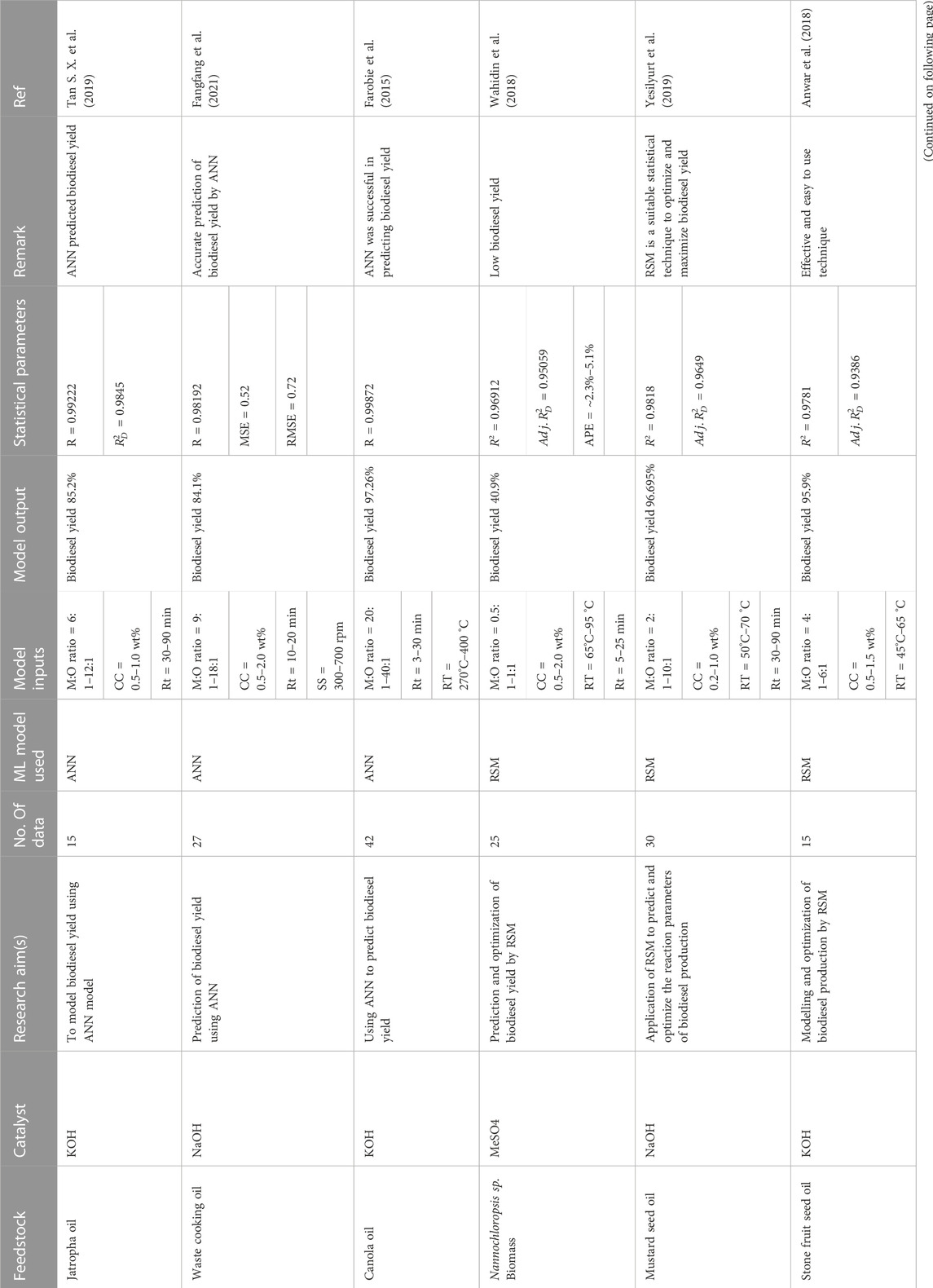
TABLE 6. Application of ML models for modelling, predicting, and optimizing biodiesel process parameters.
6 Implications, challenges, and future research direction
Demand for affordable, easily accessible, and environmentally benign energy sources will continue to increase in the foreseeable future. There will be more pressures on the fuel refiners, energy producers, researchers, and all stakeholders to escalate energy production to meet up with the global energy demand. Therefore, researches geared towards making more renewable energy available will continue to be at the front burner. Biodiesel, a prominent member of renewable fuel, will continue to occupy a prime place in the renewable energy space. The current research is therefore relevant to the contemporary times as it provides an updated information in the research domain with a view to provoke the interest of researchers in the field. Bearing in mind the continuous application of innovative technologies is diverse fields, including R and D, the application of ML and similar technologies will simplify and create technology-based techniques for the expansion of the biodiesel research. The deployment of modelling, simulation, numerical, mathematical, statistical, and optimization techniques in biodiesel production will contribute towards democratizing biodiesel production and energy sufficiency.
Researchers and biodiesel refiners must evolve fast, cost effective, and efficient methodologies that will not waste materials (Goswami et al., 2022). Efficient conversion of various natural oil, waste oil, animal fats and other feedstocks into biodiesel will continue to attract the attention of researchers and biodiesel refiners alike. The application of ML and other novel technologies for the modelling, prediction, estimation, and optimization of biodiesel; production will continue to be in demand. Ml technologies will continue to be preferred to mathematical and numerical models in modelling biodiesel yield. The implication of continuous use of ML technologies in advancing biodiesel research will be manifested in the discovery and utilization of other novel and easy to use technologies to advance biodiesel research. The implication of the current research efforts in the application of ML technologies for biodiesel research will be escalation of more research particularly in cost effective production strategies and eco-friendly utilization pathways (Albuquerque et al., 2022).
Real life production of biodiesel is very challenging. There are several non-linear factors and unpredictable occurrences that can affect the process. The use of simulation, optimization and modelling tools to such as ANN, ML, numerical, mathematical, multiphysics, etc. Are also not straightforward and requires painstaking efforts and concentration to be able to survive with the high degree of uncertainty and complexity involved. Selection of raw materials, choice of production process and methods, choice of reaction conditions, optimization of input variables, improving the procedures, intensification and upscaling of the production and purification infrastructure are crucial to taking biodiesel production to the next phase. The economic, infrastructural, manpower, environmental factors, policy formulation and implementations, biodiesel mandates and standards and other factors connected to biodiesel value chain needs holistic interrogation and improvement. The infrastructural requirements of modelling, prediction, and optimization of biodiesel generation process must be met and developed to meet the demands of the new age.
There is no doubt that the introduction of ML technologies and other prediction and optimization tools have been beneficial to biodiesel research. However, it is not yet a flawless and total solution that can solve all the complexities involved in the research domain. There are obvious inherent shortcomings that must be tackled headlong. For instance, there are challenges in the interpretation of ANN, RSM, and other statistical results. Some of the results are not completed, cannot stand alone, and difficult to interpret. Some of these need to be fine-tuned, analysed, and calibrated according to the training data. ML technologies are designed to monitor biodiesel production, purification, characterization, and utilization processes in a real life. More studies are required to develop and upgrade ML technologies and similar tools to be able to monitor and control biodiesel quality and maintain standards. The impact of biodiesel production and utilization on the environment, ecosystem, humanity, bioeconomy and biodiversity needs further scrutiny.
7 Conclusion
Population explosion, need for renewable energy, and environmental sustainability have continued to ensure increased demand for renewable fuel. Biodiesel, a form of liquid biofuel is a renewable, cost effective, biodegradable and environmentally benign fuel capable of replacing the fossil-based diesel fuel. Production of biodiesel involved several non-linear factors that must be considered to ensure the effective conversion of the diverse feedstocks to quality biodiesel. Factors such as choice of feedstock, state of feedstcoks, choice of catalysts, method of conversion, reaction time, process temperature and pressure, stirring or agitation speed, alcohol: oil molar ratio, catalysts concentration, catalysts particle size, choice of reactor, etc. Affects not only the conversion efficiency, but also biodiesel yield, biodiesel properties, and application.
In this study, we have examined the use of ML technologies in modeling, predicting, estimating, and optimizing biodiesel production. We surveyed the trend in global biodiesel production and market size and other issues relating to biodiesel production including feedstock, techniques, and catalysts for biodiesel production. A brief introduction to ML and other technologies for predicting, estimating, and optimizing biodiesel production. Specifically, we considered the five major factors affecting biodiesel production such as methanol: oil ratio, reaction temperature, reaction time, catalyst concentration, and agitation speed. The use of technologies such as ANN, RSM, ANFIS, GWO, GA, SVM, and other ML technologies commonly used in biodiesel research space was studied. Published works on the applications of ML technologies for the prediction, estimation, and optimization of biodiesel yield were interrogated and summarized. The implications, challenges, and future research trajectory of ML application in optimizing biodiesel yield were offered. The outcome of this current study will help to stimulate further investigations on the application of novel and innovative technologies that can enhance biodiesel production. After a careful study, it is, therefore, safe to conclude as follows.
• The most influential factors commonly optimized to increase biodiesel yield are alcohol: oil molar ratio, reaction time, process temperature, catalysts concentration and dosage, and stirring or agitation speed.
• The application of ML technologies, statistical techniques, modelling, and optimization tools in biodiesel research has opened a new vista in biodiesel production research.
• Biodiesel is one of the most popular and easy-to-produce biofuels. Applying ML and other innovative technologies to its production will stimulate more interest in biodiesel synthesis and utilization.
• As a way of recommendation, the deployment of ML technologies for monitoring, controlling, predicting, stimulating, and modelling of biodiesel production processes should first be implemented in a laboratory scale before escalating the methodology to large industrial scales. This way, challenges and problems are easier to mitigate and at lower cost.
There is a necessity for the development and implementation of simple, easy-to-use, and robust advanced technologies for the monitoring and controlling of the entire biodiesel production ecosystem. More training and capacity building are recommended to ensure better understanding and wider application of technologies in the entire biodiesel value chain, including raw materials selection, oil extraction, feedstock pre-treatment, reactor selection and configuration, biodiesel purification and quality assurance, biodiesel utilization, and emission reduction in biodiesel fuelled engines. Targets policies and programmes aimed are encouraging production and utilization of biodiesel for diverse applications towards decarbonisation of the environment should be enacted and consistently implemented. Government should democratise and encourage the use of biodiesel and other biofuels, particularly at the household levels to relieve the pressure on the fossil-based diesel fuels and its attendant implications.
Author contributions
OA, conceptualization, methodology, literature survey, original draft writing, reviewing, editing, project administration, corresponding author. DVVK, reviewing, editing, supervision, project administration, funding acquisition. Both authors approve the version of the article submitted.
Acknowledgments
The authors appreciate the valuable encouragement received from the Faculty of Engineering and Built Environment and the College of Postgraduate Studies, University of Johannesburg, South Africa.
Conflict of interest
The authors declare that the research was conducted in the absence of any commercial or financial relationships that could be construed as a potential conflict of interest.
Publisher’s note
All claims expressed in this article are solely those of the authors and do not necessarily represent those of their affiliated organizations, or those of the publisher, the editors and the reviewers. Any product that may be evaluated in this article, or claim that may be made by its manufacturer, is not guaranteed or endorsed by the publisher.
References
Abbaszadeh, S., Wan Alwi, S. R., Webb, C., Ghasemi, N., and Muhamad, I. I. (2016). Treatment of lead-contaminated water using activated carbon adsorbent from locally available papaya peel biowaste. J. Clean. Prod. 118, 210–222. doi:10.1016/j.jclepro.2016.01.054
Abdelbasset, W. K., Alrawaili, S. M., Elsayed, S. H., Diana, T., Ghazali, S., Felemban, B. F., et al. (2022). Optimization of heterogeneous Catalyst-assisted fatty acid methyl esters biodiesel production from Soybean oil with different Machine learning methods. Arab. J. Chem. 15 (7), 103915. doi:10.1016/j.arabjc.2022.103915
Abdelmigeed, M. O., Al-Sakkari, E. G., Hefney, M. S., Ismail, F. M., Ahmed, T. S., and Ismail, I. M. (2021). Biodiesel production catalyzed by NaOH/Magnetized ZIF-8: Yield improvement using methanolysis and catalyst reusability enhancement. Renew. Energy 174, 253–261. doi:10.1016/j.renene.2021.04.057
Abid, M., Touzani, A., and Benhima, R. (2019). Synthesis of biodiesel from chicken’s skin waste by homogeneous transesterification. Int. J. Sustain. Eng. 12 (4), 272–280. doi:10.1080/19397038.2018.1521883
Abusweireh, R. S., Rajamohan, N., and Vasseghian, Y. (2022). Enhanced production of biodiesel using nanomaterials: A detailed review on the mechanism and influencing factors. Fuel 319, 123862. doi:10.1016/j.fuel.2022.123862
Aghbashlo, M., Peng, W., Tabatabaei, M., Kalogirou, S. A., Soltanian, S., Hosseinzadeh-Bandbafha, H., et al. (2021). Machine learning technology in biodiesel research: A review. Prog. Energy Combust. Sci. 85, 100904. doi:10.1016/j.pecs.2021.100904
Ahmad, T., Danish, M., Kale, P., Geremew, B., Adeloju, S. B., Nizami, M., et al. (2019). Optimization of process variables for biodiesel production by transesterification of flaxseed oil and produced biodiesel characterizations. Renew. Energy 139, 1272–1280. doi:10.1016/j.renene.2019.03.036
Ahmed, M., Abdullah, A., Patle, D. S., Shahadat, M., Ahmad, Z., Athar, M., et al. (2021). Feedstocks, catalysts, process variables and techniques for biodiesel production by one-pot extraction-transesterification: A review. Environ. Chem. Lett. 20, 335–378. doi:10.1007/s10311-021-01358-w
Al-Humairi, S. T., Lee, J. G., Salihu, M., and Harvey, A. P. (2022). Biodiesel production through acid catalyst in situ reactive extraction of chlorella vulgaris foamate. Energies 15 (12), 4482. doi:10.3390/en15124482
Al-Saadi, A., Mathan, B., and He, Y. (2020). Esterification and transesterification over SrO–ZnO/Al2O3 as a novel bifunctional catalyst for biodiesel production. Renew. Energy 158, 388–399. doi:10.1016/j.renene.2020.05.171
Albuquerque, A. A., Ng, F. T., Danielski, T., and Stragevitch, L. (2022). Reactive separation processes applied to biodiesel production from residual oils and fats: Design, optimization and techno-economic assessment of routes using solid catalysts. Energy 240, 122784. doi:10.1016/j.energy.2021.122784
Aliramezani, M., Koch, C. R., and Shahbakhti, M. (2022). Modeling, diagnostics, optimization, and control of internal combustion engines via modern machine learning techniques: A review and future directions. Prog. Energy Combust. Sci. 88, 100967. doi:10.1016/j.pecs.2021.100967
Altinok, F., Albayrak, S., Arslan, N. P., Taskin, M., Aygun, E., Sisecioglu, M., et al. (2023). Application of Anoxybacillus gonensins UF7 lipase as a catalyst for biodiesel production from waste frying oils. Fuel 334, 126672. doi:10.1016/j.fuel.2022.126672
Anand, A., Trivedi, N. K., Abdul Wassay, M., AlSaud, Y., and Maheshwari, S. (2022). “Application and uses of big data analytics in different domain,” in Machine intelligence and data science applications. Editors V. Skala, T. P. Singh, T. Choudhury, R. Tomar, and M. Abul Bashar (Singapore: Springer), 481–500.
Anggoro, P. W., Purharyono, Y., Anthony, A. A., Tauviqirrahman, M., Bayuseno, A. P., and Jamari, A. (2022). Optimisation of cutting parameters of new material orthotic insole using a Taguchi and response surface methodology approach. Alex. Eng. J. 61 (5), 3613–3632. doi:10.1016/j.aej.2021.08.083
Anwar, M., Rasul, M. G., Ashwath, N., and Rahman, M. M. (2018). Optimisation of second-generation biodiesel production from Australian native stone fruit oil using response surface method. Energies 11 (10), 2566. doi:10.3390/en11102566
Atadashi, I. M., Aroua, M. K., Abdul Aziz, A. R., and Sulaiman, N. M. N. (2013). The effects of catalysts in biodiesel production: A review. J. Ind. Eng. Chem. 19 (1), 14–26. doi:10.1016/j.jiec.2012.07.009
Athar, M., and Zaidi, S. (2020). A review of the feedstocks, catalysts, and intensification techniques for sustainable biodiesel production. J. Environ. Chem. Eng. 8 (6), 104523. doi:10.1016/j.jece.2020.104523
Avramović, J. M., Veličković, A. V., Stamenković, O. S., Rajković, K. M., Milić, P. S., and Veljković, V. B. (2015). Optimization of sunflower oil ethanolysis catalyzed by calcium oxide: RSM versus ANN-GA. Energy Convers. Manag. 105, 1149–1156. doi:10.1016/j.enconman.2015.08.072
Awogbemi, O., and Kallon, D. V. V. (2022a). Application of tubular reactor technologies for the acceleration of biodiesel production. Bioengineering 9 (8), 347. doi:10.3390/bioengineering9080347
Awogbemi, O., and Kallon, D. V. V. (2022b). Pretreatment techniques for agricultural waste. Case Stud. Chem. Environ. Eng. 6, 100229. doi:10.1016/j.cscee.2022.100229
Awogbemi, O., and Kallon, D. V. V. (2022c). Valorization of agricultural wastes for biofuel applications. Heliyon 8 (10), e11117. doi:10.1016/j.heliyon.2022.e11117
Awogbemi, O., Kallon, D. V. V., and Aigbodion, V. S. (2021a). Trends in the development and utilization of agricultural wastes as heterogeneous catalyst for biodiesel production. J. Energy Inst. 98, 244–258. doi:10.1016/j.joei.2021.06.017
Awogbemi, O., Kallon, D. V. V., Onuh, E. I., and Aigbodion, V. S. (2021b). An overview of the classification, production and utilization of biofuels for internal combustion engine applications. Energies 14 (18), 5687. doi:10.3390/en14185687
Awogbemi, O., Kallon, D. V. V., and Pelemo, J. (2022). “Performance and emission characteristics of hydrogenation derived renewable diesel as diesel engine fuel,” in Diesel engines and biodiesel engines TechnologiesDiesel engines and biodiesel engines technologies. Editor F. L. Inambao (London, UK: IntechOpen), 173–194. doi:10.5772/intechopen.104820
Ayoola, A. A., Hymore, F. K., Omonhinmin, C. A., Olawole, O. C., Fayomi, O. S. I., Babatunde, D., et al. (2019). Analysis of waste groundnut oil biodiesel production using response surface methodology and artificial neural network. Chem. Data Collect. 22, 100238. doi:10.1016/j.cdc.2019.100238
Babadi, A. A., Rahmati, S., Fakhlaei, R., Barati, B., Wang, S., Doherty, W., et al. (2022). Emerging technologies for biodiesel production: Processes, challenges, and opportunities. Biomass Bioenergy 163, 106521. doi:10.1016/j.biombioe.2022.106521
Bali, N., and Singla, A. (2022). Emerging trends in machine learning to predict crop yield and study its influential factors: A survey. Arch. Comput. Methods Eng. 29 (1), 95–112. doi:10.1007/s11831-021-09569-8
Bashir, M. A., Wu, S., Zhu, J., Krosuri, A., Khan, M. U., and Ndeddy Aka, R. J. (2022). Recent development of advanced processing technologies for biodiesel production: A critical review. Fuel Process. Technol. 227, 107120. doi:10.1016/j.fuproc.2021.107120
Basile, A. B., Alavi, M., and Curcio, M. (2017). Artificial neural networks in chemical engineering. Hauppauge, NY: Nova Science Publishers, 50–65. Incorporated.
Baydir, E., and Aras, O. (2022). Increasing biodiesel production yield in narrow channel tubular reactors. Chem. Eng. Process. Process Intensif. 170, 108719. doi:10.1016/j.cep.2021.108719
Bergamasco, J., de Araujo, M. V., de Vasconcellos, A., Luizon Filho, R. A., Hatanaka, R. R., Giotto, M. V., et al. (2013). Enzymatic transesterification of soybean oil with ethanol using lipases immobilized on highly crystalline PVA microspheres. Biomass Bioenergy 59, 218–233. doi:10.1016/j.biombioe.2013.09.006
Betiku, E., Okunsolawo, S. S., Ajala, S. O., and Odedele, O. S. (2015). Performance evaluation of artificial neural network coupled with generic algorithm and response surface methodology in modeling and optimization of biodiesel production process parameters from shea tree (Vitellaria paradoxa) nut butter. Renew. Energy 76, 408–417. doi:10.1016/j.renene.2014.11.049
Can, Ö., Baklacioglu, T., Özturk, E., and Turan, O. (2022). Artificial neural networks modeling of combustion parameters for a diesel engine fueled with biodiesel fuel. Energy 247, 123473. doi:10.1016/j.energy.2022.123473
Cao, H., Zhang, Z., Wu, X., and Miao, X. (2013). Direct biodiesel production from wet microalgae biomass of Chlorella pyrenoidosa through in situ transesterification. Biomed. Res. Int. 2013, 1–6. doi:10.1155/2013/930686
Changmai, B., Vanlalveni, C., Ingle, A. P., Bhagat, R., and Rokhum, S. L. (2020). Widely used catalysts in biodiesel production: A review. RSC Adv. 10 (68), 41625–41679. doi:10.1039/d0ra07931f
Chen, C., Liang, R., Xia, S., Hou, D., Abdoulaye, B., Tao, J., et al. (2023). Fast characterization of biodiesel via a combination of ATR-FTIR and machine learning models. Fuel 332, 126177. doi:10.1016/j.fuel.2022.126177
Cheng, M. Y., Prayogo, D., Ju, Y. H., Wu, Y. W., and Sutanto, S. (2016). Optimizing mixture properties of biodiesel production using genetic algorithm-based evolutionary support vector machine. Int. J. Green Energy 13 (15), 1599–1607. doi:10.1080/15435075.2016.1206549
Chilakamarry, C. R., Sakinah, A., Zularisam, A., Sirohi, R., Khilji, I. A., Reddy, V. J., et al. (2022). Bioconversion of glycerol into biofuels—Opportunities and challenges. BioEnergy Res. 15 (1), 46–61. doi:10.1007/s12155-021-10353-6
Chizoo, E., Augustine, S. C., Chukwu, E. G., and Gerald, U. (2022). Adaptive neuro-fuzzy inference system-genetic algorithm versus response surface methodology-desirability function algorithm modelling and optimization of biodiesel synthesis from waste chicken fat. J. Taiwan Inst. Chem. Eng. 136, 104389. doi:10.1016/j.jtice.2022.104389
Chuengcharoenphanich, N., Watsuntorn, W., Qi, W., Wang, Z., Hu, Y., and Chulalaksananukul, W. (2023). The potential of biodiesel production from grasses in Thailand through consolidated bioprocessing using a cellulolytic oleaginous yeast, Cyberlindnera rhodanensis CU-CV7. Energy 263, 125759. doi:10.1016/j.energy.2022.125759
Corral Bobadilla, M., Fernández Martínez, R., Lostado Lorza, R., Somovilla Gómez, F., and Vergara González, E. P. (2018). Optimizing biodiesel production from waste cooking oil using genetic algorithm-based support vector machines. Energies 11 (11), 2995. doi:10.3390/en11112995
Costa, M. J., Silva, M. R. L., Ferreira, E. E. A., Carvalho, A. K. F., Basso, R. C., Pereira, E. B., et al. (2020). Enzymatic biodiesel production by hydroesterification using waste cooking oil as feedstock. Chem. Eng. Process. Process Intensif. 157, 108131. doi:10.1016/j.cep.2020.108131
Cunanan, C., Tran, M. K., Lee, Y., Kwok, S., Leung, V., and Fowler, M. (2021). A review of heavy-duty vehicle powertrain technologies: Diesel engine vehicles, battery electric vehicles, and hydrogen fuel cell electric vehicles. Clean. Technol. 3 (2), 474–489. doi:10.3390/cleantechnol3020028
Dabi, M., and Saha, U. K. (2019). Application potential of vegetable oils as alternative to diesel fuels in compression ignition engines: A review. J. Energy Inst. 92 (6), 1710–1726. doi:10.1016/j.joei.2019.01.003
dos Santos, T. C., Santos, E. C. S., Dias, J. P., Barreto, J., Stavale, F. L., and Ronconi, C. M. (2019). Reduced graphene oxide as an excellent platform to produce a stable Brønsted acid catalyst for biodiesel production. Fuel 256, 115793. doi:10.1016/j.fuel.2019.115793
Du, L., Li, Z., Ding, S., Chen, C., Qu, S., Yi, W., et al. (2019). Synthesis and characterization of carbon-based MgO catalysts for biodiesel production from castor oil. Fuel 258, 116122. doi:10.1016/j.fuel.2019.116122
Efavi, J. K., Kanbogtah, D., Apalangya, V., Nyankson, E., Tiburu, E. K., Dodoo-Arhin, D., et al. (2018). The effect of NaOH catalyst concentration and extraction time on the yield and properties of Citrullus vulgaris seed oil as a potential biodiesel feed stock. S. Afr. J. Chem. Eng. 25, 98–102. doi:10.1016/j.sajce.2018.03.002
El Naqa, I., and Murphy, M. J. (2015). “What is machine learning?” in Machine learning in radiation oncology. Editors I. El Naqa, R. Li, and M. Murphy (Cham: Springer), 3–11. doi:10.1007/978-3-319-18305-3_1
Elghariani, S. A., and Eshoul, N. M. (2021). “A new vision for the future of sustainable biodiesel for the EU,” in 12th international renewable engineering conference (IREC), Hammamet, Tunis, 26-28 October 2021, 1–5. doi:10.1109/IREC51415.2021.9736674
Elkelawy, M., Bastawissi, A. E., Esmaeil, K. K., Radwan, A. M., Panchal, H., Sadasivuni, K. K., et al. (2020). Maximization of biodiesel production from sunflower and soybean oils and prediction of diesel engine performance and emission characteristics through response surface methodology. Fuel 266, 117072. doi:10.1016/j.fuel.2020.117072
Faizollahzadeh Ardabili, S., Najafi, B., Alizamir, M., Mosavi, A., Shamshirband, S., and Rabczuk, T. (2018). Using SVM-RSM and ELM-RSM approaches for optimizing the production process of methyl and ethyl esters. Energies 11 (11), 2889. doi:10.3390/en11112889
Fangfang, F., Alagumalai, A., and Mahian, O. (2021). Sustainable biodiesel production from waste cooking oil: ANN modeling and environmental factor assessment. Sustain. Energy Technol. Assess. 46, 101265. doi:10.1016/j.seta.2021.101265
Farobie, O., Hasanah, N., and Matsumura, Y. (2015). Artificial neural network modeling to predict biodiesel production in supercritical methanol and ethanol using spiral reactor. Procedia Environ. Sci. 28, 214–223. doi:10.1016/j.proenv.2015.07.028
Farobie, O., and Matsumura, Y. (2017). State of the art of biodiesel production under supercritical conditions. Prog. Energy Combust. Sci. 63, 173–203. doi:10.1016/j.pecs.2017.08.001
Fayyazi, E., Ghobadian, B., Najafi, G., Hosseinzadeh, B., Mamat, R., and Hosseinzadeh, J. (2015). An ultrasound-assisted system for the optimization of biodiesel production from chicken fat oil using a genetic algorithm and response surface methodology. Ultrason. Sonochem 26, 312–320. doi:10.1016/j.ultsonch.2015.03.007
Galvan, D., Cremasco, H., Gomes Mantovani, A. C., Bona, E., Killner, M., and Borsato, D. (2020). Kinetic study of the transesterification reaction by artificial neural networks and parametric particle swarm optimization. Fuel 267, 117221. doi:10.1016/j.fuel.2020.117221
Garg, A., and Jain, S. (2020). Process parameter optimization of biodiesel production from algal oil by response surface methodology and artificial neural networks. Fuel 277, 118254. doi:10.1016/j.fuel.2020.118254
Gautam, S., Kanakraj, S., and Henry, A. (2022). Computational approach using machine learning modelling for optimization of transesterification process for linseed biodiesel production. AIMS Bioeng. 9 (4), 319–336. doi:10.3934/bioeng.2022023
Gimbun, J., Ali, S., Kanwal, C. C. S. C., Shah, L. A., Ghazali, N. H. M., Cheng, C. K., et al. (2013). Biodiesel production from rubber seed oil using activated cement clinker as catalyst. Procedia Eng. 53, 13–19. doi:10.1016/j.proeng.2013.02.003
Goswami, L., Kayalvizhi, R., Dikshit, P. K., Sherpa, K. C., Roy, S., Kushwaha, A., et al. (2022). A critical review on prospects of bio-refinery products from second and third generation biomasses. Chem. Eng. J. 448, 137677. doi:10.1016/j.cej.2022.137677
Gouran, A., Aghel, B., and Nasirmanesh, F. (2021). Biodiesel production from waste cooking oil using wheat bran ash as a sustainable biomass. Fuel 295, 120542. doi:10.1016/j.fuel.2021.120542
Guo, J., Sun, S., and Liu, J. (2020). Conversion of waste frying palm oil into biodiesel using free lipase A from Candida Antarctica as a novel catalyst. Fuel 267, 117323. doi:10.1016/j.fuel.2020.117323
Gupta, J., Agarwal, M., and Dalai, A. K. (2020). An overview on the recent advancements of sustainable heterogeneous catalysts and prominent continuous reactor for biodiesel production. J. Ind. Eng. Chem. 88, 58–77. doi:10.1016/j.jiec.2020.05.012
Gupta, K. K., Kalita, K., Ghadai, R. K., Ramachandran, M., and Gao, X. Z. (2021). Machine learning-based predictive modelling of biodiesel production—a comparative perspective. Energies 14 (4), 1122. doi:10.3390/en14041122
Gupta, V., and Pal Singh, K. (2022). The impact of heterogeneous catalyst on biodiesel production; a review. Mat. Today Proc. 2022, 1–5. doi:10.1016/j.matpr.2022.10.175
Hashemzehi, M., Pirouzfar, V., Nayebzadeh, H., and Su, C. H. (2022). Modelling and optimization of main independent parameters for biodiesel production over a Cu0.4Zn0.6Al2O4 catalyst using an RSM method. J. Chem. Technol. Biotechnol. 97 (1), 111–119. doi:10.1002/jctb.6916
Helmi, M., Tahvildari, K., Hemmati, A., Aberoomand Azar, P., and Safekordi, A. (2021). Phosphomolybdic acid/graphene oxide as novel green catalyst using for biodiesel production from waste cooking oil via electrolysis method: Optimization using with response surface methodology (RSM). Fuel 287, 119528. doi:10.1016/j.fuel.2020.119528
Huang, Y., Li, F., Bao, G., Xiao, Q., and Wang, H. (2022). Modeling the effects of biodiesel chemical composition on iodine value using novel machine learning algorithm. Fuel 316, 123348. doi:10.1016/j.fuel.2022.123348
Ishola, N. B., Okeleye, A. A., Osunleke, A. S., and Betiku, E. (2019). Process modeling and optimization of sorrel biodiesel synthesis using barium hydroxide as a base heterogeneous catalyst: Appraisal of response surface methodology, neural network and neuro-fuzzy system. Neural. comput. Appl. 31 (9), 4929–4943. doi:10.1007/s00521-018-03989-7
Jambulingam, R., Srinivasan, G. R., Palani, S., Munir, M., Saeed, M., and Mohanam, A. (2020). Process optimization of biodiesel production from waste beef tallow using ethanol as co-solvent. SN Appl. Sci. 2 (8), 1454–1518. doi:10.1007/s42452-020-03243-7
Javapoint (2021). ANN structure. Available at: https://www.javatpoint.com/artificial-neural-network (Accessed November 4, 2022).
Javidialesaadi, A., and Raeissi, S. (2013). Biodiesel production from high free fatty acid-content oils: Experimental investigation of the pretreatment step. APCBEE Procedia 5, 474–478. doi:10.1016/j.apcbee.2013.05.080
Jayaraman, J., Dawn, S. S., Appavu, P., Mariadhas, A., Joy, N., Alshgari, R. A., et al. (2022). Production of biodiesel from waste cooking oil utilizing zinc oxide nanoparticles combined with tungsto phosphoric acid as a catalyst and its performance on a CI engine. Fuel 329, 125411. doi:10.1016/j.fuel.2022.125411
Jisieike, C. F., Ishola, N. B., Latinwo, L. M., and Betiku, E. (2023). Crude rubber seed oil esterification using a solid catalyst: Optimization by hybrid adaptive neuro-fuzzy inference system and response surface methodology. Energy 263, 125734. doi:10.1016/j.energy.2022.125734
Kalita, P., Basumatary, B., Saikia, P., Das, B., and Basumatary, S. (2022). Biodiesel as renewable biofuel produced via enzyme-based catalyzed transesterification. Energy Nexus 6, 100087. doi:10.1016/j.nexus.2022.100087
Kamran, E., Mashhadi, H., Mohammadi, A., and Ghobadian, B. (2020). Biodiesel production from Elaeagnus angustifolia.L seed as a novel waste feedstock using potassium hydroxide catalyst. Biocatal. Agric. Biotechnol. 25, 101578. doi:10.1016/j.bcab.2020.101578
Kesharvani, S., and Dwivedi, G. (2021). Algae as a feedstock for biodiesel production in Indian perspective. Mat. Today Proc. 47, 5873–5880. doi:10.1016/j.matpr.2021.04.295
Khair, U., Fahmi, H., Al Hakim, S., and Rahim, R. (2017). Forecasting error calculation with mean absolute deviation and mean absolute percentage error. J. Phys. Conf. Ser. 930, 012002. doi:10.1088/1742-6596/930/1/012002
Khan, H. M., Ali, C. H., Iqbal, T., Yasin, S., Sulaiman, M., Mahmood, H., et al. (2019). Current scenario and potential of biodiesel production from waste cooking oil in Pakistan: An overview. Chin. J. Chem. Eng. 27 (10), 2238–2250. doi:10.1016/j.cjche.2018.12.010
Khan, H., Soudagar, M. E. M., Kumar, R. H., Safaei, M. R., Farooq, M., Khidmatgar, A., et al. (2020). Effect of nano-graphene oxide and n-butanol fuel additives blended with diesel—nigella sativa biodiesel fuel emulsion on diesel engine characteristics. Symmetry 12 (6), 961. doi:10.3390/sym12060961
Kolakoti, A. (2020). Optimization of biodiesel production from waste cooking sunflower oil by Taguchi and ANN techniques. J. Therm. Eng. 6 (5), 712–723. doi:10.18186/thermal.796761
Kolakoti, A., Jha, P., Mosa, P. R., Mahapatro, M., and Kotaru, T. G. (2020). Optimization and modelling of mahua oil biodiesel using RSM and genetic algorithm techniques. Math. Model. Eng. 6 (2), 134–146. doi:10.21595/mme.2020.21357
Kubat, M. (2017). “Artificial neural networks,” in An introduction to machine learning. Editor M. Kubat (Cham: Springer), 91–111. doi:10.1007/978-3-319-63913-0_5
Kukreja, H., Bharath, N., Siddesh, C., and Kuldeep, S. (2016). An introduction to artificial neural network. Int. J. Adv. Res. Innov. Ideas Educ. 1, 27–30.
Lawer-Yolar, G., Dawson-Andoh, B., and Atta-Obeng, E. (2021). Synthesis of biodiesel from tall oil fatty acids by homogeneous and heterogeneous catalysis. Sustain. Chem. 2 (1), 206–221. doi:10.3390/suschem2010012
Likozar, B., and Levec, J. (2014). Effect of process conditions on equilibrium, reaction kinetics and mass transfer for triglyceride transesterification to biodiesel: Experimental and modeling based on fatty acid composition. Fuel Process. Technol. 22, 30–41. doi:10.1016/j.fuproc.2014.01.017
Liu, J. J., Dickson, R., Niaz, H., Van Hal, J. W., Dijkstra, J. W., and Fasahati, P. (2022). Production of fuels and chemicals from macroalgal biomass: Current status, potentials, challenges, and prospects. Renew. Sustain. Energy Rev. 169, 112954. doi:10.1016/j.rser.2022.112954
Mandari, V., and Devarai, S. K. (2021). Biodiesel production using homogeneous, heterogeneous, and enzyme catalysts via transesterification and esterification reactions: A critical review. BioEnergy Res. 15, 935–961. doi:10.1007/s12155-021-10333-w
Maran, J. P., and Priya, B. (2015). Comparison of response surface methodology and artificial neural network approach towards efficient ultrasound-assisted biodiesel production from muskmelon oil. Ultrason. Sonochem 23, 192–200. doi:10.1016/j.ultsonch.2014.10.019
Mathew, G. M., Raina, D., Narisetty, V., Kumar, V., Saran, S., Pugazhendi, A., et al. (2021). Recent advances in biodiesel production: Challenges and solutions. Sci. Total Environ. 794, 148751. doi:10.1016/j.scitotenv.2021.148751
Mejía, A., Leiva, M., Rincón-Montenegro, A., Gonzalez-Quiroga, A., and Duarte-Forero, J. (2020). Experimental assessment of emissions maps of a single-cylinder compression ignition engine powered by diesel and palm oil biodiesel-diesel fuel blends. Case Stud. Therm. Eng. 19, 100613. doi:10.1016/j.csite.2020.100613
Mekonnen, K. D., and Sendekie, Z. B. (2021). NaOH-catalyzed methanolysis optimization of biodiesel synthesis from desert date seed kernel oil. ACS omega 6 (37), 24082–24091. doi:10.1021/acsomega.1c03546
Mishra, V. K., and Goswami, R. (2018). A review of production, properties and advantages of biodiesel. Biofuels 9 (2), 273–289. doi:10.1080/17597269.2017.1336350
Moayedi, H., Aghel, B., Foong, L. K., and Bui, D. T. (2020). Feature validity during machine learning paradigms for predicting biodiesel purity. Fuel 262, 116498. doi:10.1016/j.fuel.2019.116498
Mofijur, M., Siddiki, S. Y. A., Shuvho, M. B. A., Djavanroodi, F., Fattah, I. M. R., Ong, H. C., et al. (2021). Effect of nanocatalysts on the transesterification reaction of first, second and third generation biodiesel sources- A mini-review. Chemosphere 270, 128642. doi:10.1016/j.chemosphere.2020.128642
Mohamed, R. M., Kadry, G. A., Abdel-Samad, H. A., and Awad, M. E. (2020). High operative heterogeneous catalyst in biodiesel production from waste cooking oil. Egypt. J. Pet. 29 (1), 59–65. doi:10.1016/j.ejpe.2019.11.002
Mohiddin, M. N. B., Tan, Y. H., Seow, Y. X., Kansedo, J., Mubarak, N. M., Abdullah, M. O., et al. (2021). Evaluation on feedstock, technologies, catalyst and reactor for sustainable biodiesel production: A review. J. Ind. Eng. Chem. 98, 60–81. doi:10.1016/j.jiec.2021.03.036
Mohri, M., Rostamizadeh, A., and Talwalkar, A. (2018). Foundations of machine learning. London, UK: MIT press.
Mukhtar, A., Saqib, S., Lin, H., Hassan Shah, M. U., Ullah, S., Younas, M., et al. (2022). Current status and challenges in the heterogeneous catalysis for biodiesel production. Renew. Sustain. Energy Rev. 157, 112012. doi:10.1016/j.rser.2021.112012
Musa, I. A. (2016). The effects of alcohol to oil molar ratios and the type of alcohol on biodiesel production using transesterification process. Egypt. J. Pet. 25 (1), 21–31. doi:10.1016/j.ejpe.2015.06.007
Narowska, B., Kułażyński, M., Łukaszewicz, M., and Burchacka, E. (2019). Use of activated carbons as catalyst supports for biodiesel production. Renew. Energy 135, 176–185. doi:10.1016/j.renene.2018.11.006
Nayab, R., Imran, M., Ramzan, M., Tariq, M., Taj, M. B., Akhtar, M. N., et al. (2022). Sustainable biodiesel production via catalytic and non-catalytic transesterification of feedstock materials – a review. Fuel 328, 125254. doi:10.1016/j.fuel.2022.125254
News (2019). Global diesel engine markets. PR News. Available at: https://www.prnewswire.com/news-releases/global-diesel-engine-markets-analysis-2018-2019–forecasts-to-2024 (Accessed October 24, 2022).
Nicolici, A., Pana, C., Negurescu, N., Cernat, A., and Nutu, C. (2018). The use of animal fats in the diesel fuelled engine. IOP Conf. Ser. Mat. Sci. Eng. 444, 072003. doi:10.1088/1757-899X/444/7/072003
Ofoefule, A. U., Esonye, C., Onukwuli, O. D., Nwaeze, E., and Ume, C. S. (2019). Modeling and optimization of African pear seed oil esterification and transesterification using artificial neural network and response surface methodology comparative analysis. Ind. Crops Prod. 140, 111707. doi:10.1016/j.indcrop.2019.111707
Ogaga Ighose, B., Adeleke, I. A., Damos, M., Adeola Junaid, H., Ernest Okpalaeke, K., and Betiku, E. (2017). Optimization of biodiesel production from Thevetia peruviana seed oil by adaptive neuro-fuzzy inference system coupled with genetic algorithm and response surface methodology. Energy Convers. Manag. 132, 231–240. doi:10.1016/j.enconman.2016.11.030
Okolie, J. A., Ivan Escobar, J., Umenweke, G., Khanday, W., and Okoye, P. U. (2022). Continuous biodiesel production: A review of advances in catalysis, microfluidic and cavitation reactors. Fuel 307, 121821. doi:10.1016/j.fuel.2021.121821
Onuh, E. I., Inambao, F., and Awogbemi, O. (2021). Performance and emission evaluation of biodiesel derived from waste restaurant oil and moringa oleifera: A comparative study. Int. J. Ambient. Energy 42 (8), 912–919. doi:10.1080/01430750.2019.1594377
Pan, H., Xia, Q., Wang, Y., Shen, Z., Huang, H., Ge, Z., et al. (2022). Recent advances in biodiesel production using functional carbon materials as acid/base catalysts. Fuel Process. Technol. 237, 107421. doi:10.1016/j.fuproc.2022.107421
Prakash Maran, J., and Priya, B. (2015). Modeling of ultrasound assisted intensification of biodiesel production from neem (Azadirachta indica) oil using response surface methodology and artificial neural network. Fuel 143, 262–267. doi:10.1016/j.fuel.2014.11.058
Prates, C. D., Ballotin, F. C., Limborço, H., Ardisson, J. D., Lago, R. M., and Teixeira, A. P. d. C. (2020). Heterogeneous acid catalyst based on sulfated iron ore tailings for oleic acid esterification. Appl. Catal. A Gen. 600, 117624. doi:10.1016/j.apcata.2020.117624
Precedence Research (2022a). Biodiesel market size. Available at: https://www.precedenceresearch.com (Accessed November 4, 2022).
Precedence Research (2022b). Machine learning as a service market. Available at: https://www.precedenceresearch.com/machine-learning-as-a-service-market# (Accessed November 4, 2022).
Qadeer, M. U., Ayoub, M., Komiyama, M., Khan Daulatzai, M. U., Mukhtar, A., Saqib, S., et al. (2021). Review of biodiesel synthesis technologies, current trends, yield influencing factors and economical analysis of supercritical process. J. Clean. Prod. 309, 127388. doi:10.1016/j.jclepro.2021.127388
Rajković, K. M., Avramović, J. M., Milić, P. S., Stamenković, O. S., and Veljković, V. B. (2013). Optimization of ultrasound-assisted base-catalyzed methanolysis of sunflower oil using response surface and artifical neural network methodologies. Chem. Eng. J. 15-216, 82–89. doi:10.1016/j.cej.2012.10.069
Ritchie, H., Roser, M., and Rosado, P. (2022). Energy. Available at: https://ourworldindata.org/energy-mix# (Accessed November 4, 2022).
Rizwanul Fattah, I., Ong, H., Mahlia, T., Mofijur, M., Silitonga, A., Rahman, S., et al. (2020). State of the art of catalysts for biodiesel production. Front. Energy Res. 8, 1–17. doi:10.3389/fenrg.2020.00101
Rostami, S., Hosseinzadeh, S. B., and Saeidi, K. (2016). Optimization of biodiesel production from prunus scoparia using artificial bee colony algorithm. J. Renew. Energy Env. 3 (1), 52–58.
Sagiroglu, A., Isbilir, S. Ş., Ozcan, M. H., Paluzar, H., and Toprakkiran, N. M. (2011). Comparison of biodiesel productivities of different vegetable oils by acidic catalysis. Chem. Ind. Chem. Eng. 17 (1), 53–58. doi:10.2298/ciceq100114054s
Salimi, Z., and Hosseini, S. A. (2019). Study and optimization of conditions of biodiesel production from edible oils using ZnO/BiFeO3 nano magnetic catalyst. Fuel 239, 1204–1212. doi:10.1016/j.fuel.2018.11.125
Samuel, O. D., Okwu, M. O., Oyejide, O. J., Taghinezhad, E., Afzal, A., and Kaveh, M. (2020). Optimizing biodiesel production from abundant waste oils through empirical method and grey wolf optimizer. Fuel 281, 118701. doi:10.1016/j.fuel.2020.118701
Sanli, H., Alptekin, E., and Canakci, M. (2022). Using low viscosity micro-emulsification fuels composed of waste frying oil-diesel fuel-higher bio-alcohols in a turbocharged-CRDI diesel engine. Fuel 308, 121966. doi:10.1016/j.fuel.2021.121966
Sarve, A., Sonawane, S. S., and Varma, M. N. (2015). Ultrasound assisted biodiesel production from sesame (Sesamum indicum L.) oil using barium hydroxide as a heterogeneous catalyst: Comparative assessment of prediction abilities between response surface methodology (RSM) and artificial neural network (ANN). Ultrason. Sonochem 26, 218–228. doi:10.1016/j.ultsonch.2015.01.013
Selvaraj, R., Moorthy, I. G., Kumar, R. V., and Sivasubramanian, V. (2019). Microwave mediated production of FAME from waste cooking oil: Modelling and optimization of process parameters by RSM and ANN approach. Fuel 237, 40–49. doi:10.1016/j.fuel.2018.09.147
Shaah, M. A. H., Hossain, M. S., Allafi, F. A. S., Alsaedi, A., Ismail, N., Ab Kadir, M. O., et al. (2021). A review on non-edible oil as a potential feedstock for biodiesel: Physicochemical properties and production technologies. RSC Adv. 11 (40), 25018–25037. doi:10.1039/d1ra04311k
Sharma, P., Sahoo, B. B., Said, Z., Hadiyanto, H., Nguyen, X. P., Nižetić, S., et al. (2022). Application of machine learning and Box-Behnken design in optimizing engine characteristics operated with a dual-fuel mode of algal biodiesel and waste-derived biogas. Int. J. Hydrog. Energy 2022, 1–23. doi:10.1016/j.ijhydene.2022.04.152
Silitonga, A. S., Shamsuddin, A. H., Mahlia, T. M. I., Milano, J., Kusumo, F., Siswantoro, J., et al. (2020). Biodiesel synthesis from Ceiba pentandra oil by microwave irradiation-assisted transesterification: ELM modeling and optimization. Renew. Energy 146, 1278–1291. doi:10.1016/j.renene.2019.07.065
Singh Pali, H., Sharma, A., Kumar, N., and Singh, Y. (2021). Biodiesel yield and properties optimization from Kusum oil by RSM. Fuel 291, 120218. doi:10.1016/j.fuel.2021.120218
Singh, R., Bux, F., and Sharma, Y. C. (2020). Optimization of biodiesel synthesis from microalgal (Spirulina platensis) oil by using a novel heterogeneous catalyst, β-strontium silicate (β-Sr2SiO4). Fuel 280, 118312. doi:10.1016/j.fuel.2020.118312
Sitepu, E. K., Heimann, K., Raston, C. L., and Zhang, W. (2020). Critical evaluation of process parameters for direct biodiesel production from diverse feedstock. Renew. Sustain. Energy Rev. 123, 109762. doi:10.1016/j.rser.2020.109762
Soji-Adekunle, A. R., Asere, A. A., Ishola, N. B., Oloko-Oba, I. M., and Betiku, E. (2019). Modelling of synthesis of waste cooking oil methyl esters by artificial neural network and response surface methodology. Int. J. Ambient. Energy 40 (7), 716–725. doi:10.1080/01430750.2017.1423378
Soltani, S., Roodbar Shojaei, T., Khanian, N., Shean Yaw Choong, T., Asim, N., and Zhao, Y. (2022). Artificial neural network method modeling of microwave-assisted esterification of PFAD over mesoporous TiO2‒ZnO catalyst. Renew. Energy 187, 760–773. doi:10.1016/j.renene.2022.01.123
Soria-Figueroa, E., Mena-Cervantes, V. Y., García-Solares, M., Hernández-Altamirano, R., and Vazquez-Arenas, J. (2020). Statistical optimization of biodiesel production from waste cooking oil using CaO as catalyst in a Robinson-Mahoney type reactor. Fuel 282, 118853. doi:10.1016/j.fuel.2020.118853
Soudagar, M. E. M., Afzal, A., Safaei, M. R., Manokar, A. M., El-Seesy, A. I., Mujtaba, M., et al. (2022). Investigation on the effect of cottonseed oil blended with different percentages of octanol and suspended MWCNT nanoparticles on diesel engine characteristics. J. Therm. Anal. Calorim. 147, 525–542. doi:10.1007/s10973-020-10293-x
Soudagar, M. E. M., Nik-Ghazali, N. N., Kalam, M. A., Badruddin, I. A., Banapurmath, N. R., Yunus Khan, T. M., et al. (2019). The effects of graphene oxide nanoparticle additive stably dispersed in dairy scum oil biodiesel-diesel fuel blend on CI engine: Performance, emission and combustion characteristics. Fuel 257, 116015. doi:10.1016/j.fuel.2019.116015
Srivastava, G., Paul, A. K., and Goud, V. V. (2018). Optimization of non-catalytic transesterification of microalgae oil to biodiesel under supercritical methanol condition. Energy Convers. Manag. 156, 269–278. doi:10.1016/j.enconman.2017.10.093
Statista (2022). Global biodiesel production by country 2019. Available at: https://www.statista.com/statistics/271472/biodiesel-production-in-selected-countries (Accessed November 1, 2022).
Stevanato, N., de Mello, B. T. F., Saldaña, M. D. A., Cardozo-Filho, L., and da Silva, C. (2023). Production of ethyl esters from forage radish seed: An integrated sequential route using pressurized ethanol and ethyl acetate. Fuel 332, 126075. doi:10.1016/j.fuel.2022.126075
Su, G., Ong, H. C., Mofijur, M., Mahlia, T. M. I., and Ok, Y. S. (2022). Pyrolysis of waste oils for the production of biofuels: A critical review. J. Hazard. Mat. 424, 127396. doi:10.1016/j.jhazmat.2021.127396
Sudsakorn, K., Saiwuttikul, S., Palitsakun, S., Seubsai, A., and Limtrakul, J. (2017). Biodiesel production from Jatropha Curcas oil using strontium-doped CaO/MgO catalyst. J. Environ. Chem. Eng. 5 (3), 2845–2852. doi:10.1016/j.jece.2017.05.033
Suvarna, M., Jahirul, M. I., Aaron-Yeap, W. H., Augustine, C. V., Umesh, A., Rasul, M. G., et al. (2022). Predicting biodiesel properties and its optimal fatty acid profile via explainable machine learning. Renew. Energy 189, 245–258. doi:10.1016/j.renene.2022.02.124
Tabatabaei, M., Aghbashlo, M., Dehhaghi, M., Panahi, H. K. S., Mollahosseini, A., Hosseini, M., et al. (2019). Reactor technologies for biodiesel production and processing: A review. Prog. Energy Combust. Sci. 74, 239–303. doi:10.1016/j.pecs.2019.06.001
Taft, C. A., and Canchaya, J. G. S. (2022). “Overview: Catalysts, feedstocks in biodiesel production,” in Research topics in bioactivity, environment and energy. Editors C. A. Taft.,, and S. R. de Lazaro (Cham: Springer), 337–357. doi:10.1007/978-3-031-07622-0_13
Tan, S. X., Lim, S., Ong, H. C., Pang, Y. L., Fitranto, K., Goh, B. H. H., et al. (2019). Two-step catalytic reactive extraction and transesterification process via ultrasonic irradiation for biodiesel production from solid Jatropha oil seeds. Chem. Eng. Process. Process Intensif. 146, 107687. doi:10.1016/j.cep.2019.107687
Tan, Y. H., Abdullah, M. O., Kansedo, J., Mubarak, N. M., Chan, Y. S., and Nolasco-Hipolito, C. (2019). Biodiesel production from used cooking oil using green solid catalyst derived from calcined fusion waste chicken and fish bones. Renew. Energy 139, 696–706. doi:10.1016/j.renene.2019.02.110
Techopedia (2022). Artificial neural network. Available at: https://www.techopedia.com/definition/5967/artificial-neural-network-ann (Accessed November 1, 2022).
Teo, S. H., Islam, A., Mansir, N., Shamsuddin, M. R., Joseph, C. G., Goto, M., et al. (2022). Sustainable biofuel production approach: Critical methanol green transesterification by efficient and stable heterogeneous catalyst. Renew. Sustain. Energy Rev. 169, 112889. doi:10.1016/j.rser.2022.112889
Thangaraja, J., Zigan, L., and Rajkumar, S. (2023). A machine learning framework for evaluating the biodiesel properties for accurate modeling of spray and combustion processes. Fuel 334, 126573. doi:10.1016/j.fuel.2022.126573
Thoai, D. N., Tongurai, C., Prasertsit, K., and Kumar, A. (2017). A novel two-step transesterification process catalyzed by homogeneous base catalyst in the first step and heterogeneous acid catalyst in the second step. Fuel Process. Technol. 168, 97–104. doi:10.1016/j.fuproc.2017.08.014
Todorović, Z. B., Troter, D. Z., Đokić-Stojanović, D. R., Veličković, A. V., Avramović, J. M., Stamenković, O. S., et al. (2019). Optimization of CaO-catalyzed sunflower oil methanolysis with crude biodiesel as a cosolvent. Fuel 237, 903–910. doi:10.1016/j.fuel.2018.10.056
Toldrá-Reig, F., Mora, L., and Toldrá, F. (2020). Developments in the use of lipase transesterification for biodiesel production from animal fat waste. Appl. Sci. 10 (15), 5085. doi:10.3390/app10155085
Topare, N. S., Jogdand, R. I., Shinde, H. P., More, R. S., Khan, A., and Asiri, A. M. (2022). A short review on approach for biodiesel production: Feedstock’s, properties, process parameters and environmental sustainability. Mat. Today Proc. 57, 1605–1612. doi:10.1016/j.matpr.2021.12.216
Tran, T. T. V., Kaiprommarat, S., Kongparakul, S., Reubroycharoen, P., Guan, G., Nguyen, M. H., et al. (2016). Green biodiesel production from waste cooking oil using an environmentally benign acid catalyst. Waste Manage. 52, 367–374. doi:10.1016/j.wasman.2016.03.053
Treve, M., Patra, I., Prabu, P., Rama Sree, S., Keerthi Kumar, N., Methkal Abd Algani, Y., et al. (2022). Performance evaluation of artificial neural networks in sustainable modelling biodiesel synthesis. Sustain. Energy Technol. Assess. 52, 102098. doi:10.1016/j.seta.2022.102098
UN (2022). World population by year. Available at: https://www.worldometers.info/world-population/world-population-by-year/(Accessed October 21, 2022).
Velusamy, K., Devanand, J., Senthil Kumar, P., Soundarajan, K., Sivasubramanian, V., Sindhu, J., et al. (2021). A review on nano-catalysts and biochar-based catalysts for biofuel production. Fuel 306, 121632. doi:10.1016/j.fuel.2021.121632
Verma, P., and Sharma, M. P. (2016). Review of process parameters for biodiesel production from different feedstocks. Renew. Sustain. Energy Rev. 62, 1063–1071. doi:10.1016/j.rser.2016.04.054
Wahidin, S., Idris, A., Yusof, N. M., Kamis, N. H. H., and Shaleh, S. R. M. (2018). Optimization of the ionic liquid-microwave assisted one-step biodiesel production process from wet microalgal biomass. Energy Convers. Manag. 171, 1397–1404. doi:10.1016/j.enconman.2018.06.083
Walczak, S. (2019). Artificial neural networks. Advanced methodologies and technologies in artificial intelligence, computer simulation, and human-computer interaction. IGI Glob. 2019, 40–53. doi:10.4018/978-1-5225-7368-5.ch004
Wancura, J. H., Rosset, D. V., Brondani, M., Mazutti, M. A., Oliveira, J. V., Tres, M. V., et al. (2018). Soluble lipase-catalyzed synthesis of methyl esters using a blend of edible and nonedible raw materials. Bioprocess Biosyst. Eng. 41 (8), 1185–1193. doi:10.1007/s00449-018-1947-7
Wategave, S. P., Banapurmath, N. R., Sawant, M. S., Soudagar, M. E. M., Mujtaba, M. A., Afzal, A., et al. (2021). Clean combustion and emissions strategy using reactivity controlled compression ignition (RCCI) mode engine powered with CNG-Karanja biodiesel. J. Taiwan Inst. Chem. Eng. 124, 116–131. doi:10.1016/j.jtice.2021.04.055
Wazid, M., Das, A. K., Chamola, V., and Park, Y. (2022). Uniting cyber security and machine learning: Advantages, challenges and future research. ICT Express 8 (3), 313–321. doi:10.1016/j.icte.2022.04.007
Wong, K. I., Wong, P. K., Cheung, C. S., and Vong, C. M. (2013). Modeling and optimization of biodiesel engine performance using advanced machine learning methods. Energy 55, 519–528. doi:10.1016/j.energy.2013.03.057
Wuest, T., Weimer, D., Irgens, C., and Thoben, K. D. (2016). Machine learning in manufacturing: Advantages, challenges, and applications. Prod. Manuf. Res. 4 (1), 23–45. doi:10.1080/21693277.2016.1192517
Xie, W., and Li, J. (2023). Magnetic solid catalysts for sustainable and cleaner biodiesel production: A comprehensive review. Renew. Sustain. Energy Rev. 171, 113017. doi:10.1016/j.rser.2022.113017
Xie, W., and Wang, H. (2020). Immobilized polymeric sulfonated ionic liquid on core-shell structured Fe3O4/SiO2 composites: A magnetically recyclable catalyst for simultaneous transesterification and esterifications of low-cost oils to biodiesel. Renew. Energy 145, 1709–1719. doi:10.1016/j.renene.2019.07.092
Xing, Y., Zheng, Z., Sun, Y., and Agha Alikhani, M. (2021). A review on machine learning application in biodiesel production studies. Int. J. Chem. Eng. 2021, 1–12. doi:10.1155/2021/2154258
Yaashikaa, P. R., Keerthana Devi, M., Senthil Kumar, P., and Pandian, E. (2022). A review on biodiesel production by algal biomass: Outlook on lifecycle assessment and techno-economic analysis. Fuel 324, 124774. doi:10.1016/j.fuel.2022.124774
Yesilyurt, M. K., Arslan, M., and Eryilmaz, T. (2019). Application of response surface methodology for the optimization of biodiesel production from yellow mustard (Sinapis alba L.) seed oil. Int. J. Green Energy 16 (1), 60–71. doi:10.1080/15435075.2018.1532431
Zahed, M. A., Revayati, M., Shahcheraghi, N., Maghsoudi, F., and Tabari, Y. (2021). Modeling and optimization of biodiesel synthesis using TiO2–ZnO nanocatalyst and characteristics of biodiesel made from waste sunflower oil. Curr. Res. Green Sustain. Chem. 4, 100223. doi:10.1016/j.crgsc.2021.100223
Zhang, J., Wang, Y., Muldoon, V. L., and Deng, S. (2022). Crude glycerol and glycerol as fuels and fuel additives in combustion applications. Renew. Sustain. Energy Rev. 159, 112206. doi:10.1016/j.rser.2022.112206
Keywords: biodiesel, machine learning, artificial neural network, optimization, processing parameters, biodiesel yield, feedstock
Citation: Awogbemi O and Kallon DVV (2023) Application of machine learning technologies in biodiesel production process—A review. Front. Energy Res. 11:1122638. doi: 10.3389/fenrg.2023.1122638
Received: 13 December 2022; Accepted: 26 January 2023;
Published: 10 February 2023.
Edited by:
Muhammad Jamshaid, Bahauddin Zakariya University, PakistanReviewed by:
Manzoore Elahi M. Soudagar, Chandigarh University, IndiaJassinnee Mialno, Universiti Tenaga Nasional, Malaysia
Copyright © 2023 Awogbemi and Kallon. This is an open-access article distributed under the terms of the Creative Commons Attribution License (CC BY). The use, distribution or reproduction in other forums is permitted, provided the original author(s) and the copyright owner(s) are credited and that the original publication in this journal is cited, in accordance with accepted academic practice. No use, distribution or reproduction is permitted which does not comply with these terms.
*Correspondence: Omojola Awogbemi, jolawogbemi2015@gmail.com