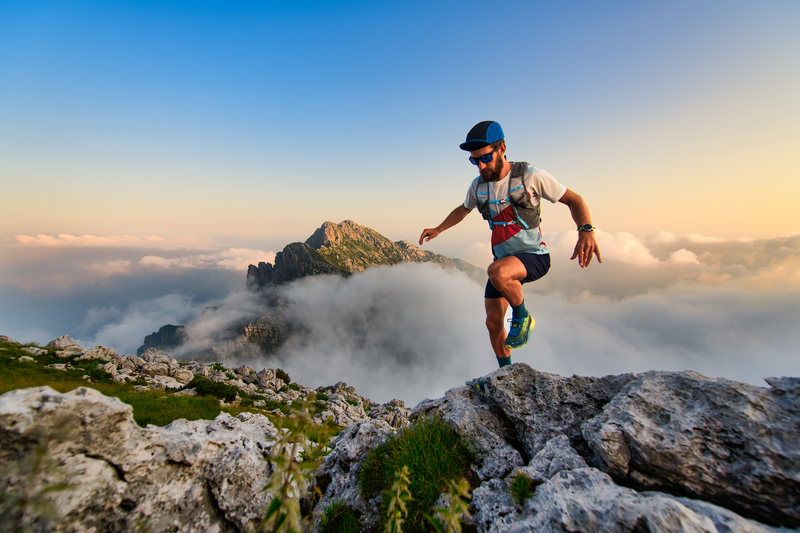
95% of researchers rate our articles as excellent or good
Learn more about the work of our research integrity team to safeguard the quality of each article we publish.
Find out more
ORIGINAL RESEARCH article
Front. Energy Res. , 02 February 2023
Sec. Sustainable Energy Systems
Volume 11 - 2023 | https://doi.org/10.3389/fenrg.2023.1112635
The technological innovation of clean energy enterprises is conducive to the transformation of energy structure, and the green credit policy is just a potential opportunity for clean energy enterprises to seek financing convenience. This paper constructs quasi-natural experiments during two different lengths of period (2008–2015 and 2008–2020) based on the 2012 Green Credit Guidelines, selecting a group of A-share listed clean energy enterprises and non-clean energy enterprises as samples. The aim is to examine the impact of green credit policies on the technological innovation of clean energy enterprises. This paper further conducts mechanism tests as well as heterogeneity analysis on the basis of the results. The results show that green credit policies can promote technological innovation for clean energy enterprises, and this effect is reinforced with the accumulation of years following policy implementation. The mechanism test indicates that green credit policies affect the level of technological innovation of clean energy enterprises through credit cost and financing constraints. The heterogeneity analysis demonstrates that the impact of green credit policies is more significant in the non-state-owned enterprises and economically developed regions. The results provide insights for the promotion of green credit policy and the ultimate transformation of China’s energy structure, thus providing reference for developing countries and emerging economies. The formulation of green credit policy should take into account the long-term effect, reduce the burden of enterprises from the perspective of credit cost and financing constraints, and also expand the influence of the policy in state-owned enterprises and underdeveloped areas.
With the growing establishment of the concept of green development and the ever-increasing promotion of ecological civilization, China’s energy system is facing greater and more stringent requirements than ever before. It has become imperative to proactively yet strategically promote the transformation of the traditional energy system and the development of clean energy. In September 2020, at the 75th session of the United Nations General Assembly, China announced to the world its long-term goal of achieving “carbon peaking” by 2030 and “carbon neutrality” by 2060. With the background of the “double carbon policy,” the promotion of clean energy over traditional forms will help optimize the nation’s energy structure and build a sustainable, low-carbon energy system. Due to the continuous impact of COVID-19 on the economy and the sudden conflict between Russia and Ukraine in 2022, global energy governance is fraught with dangers. The development of clean energy is more important for improving the efficiency of energy, alleviating energy shortages and ensuring energy security and longevity.
It is crucial to promote technological progress in the field of clean energy in order to realize the transformation of the energy system. As a key subject of technological innovation, clean energy enterprises are also confronted with more challenges at this stage. In its capacity as a financial tool, green credit can guide the flow of funds to environmentally friendly enterprises rather than harmful ones to a certain extent, thus encouraging enterprises to carry out renewable R&D and production activities. Specifically, providing financial support for clean energy enterprises through green credit is conducive to improving the core competitiveness of the industry, and increasing investment in the clean energy sector is in line with the current demand of energy transition. Green credit policies prompt financial institutions to internalize environmental factors when reviewing loans. The clean energy industry supports the promotion of green and low-carbon economic development, achieving greater energy conservation and emission reduction, and generating more environmental benefits. All of the above benefits are in line with the guiding objectives of the policies. Green credit policies thus increase the availability of credit funds for clean energy enterprises. In order to meet the standards of green credit, these policies also encourage enterprises to continuously improve their technological innovation.
A large number of studies have been conducted on green credit in recent years with mixed findings. From the perspective of green credit and enterprise financing, research on green credit is currently dominated by empirical analysis. In terms of green credit and enterprise financing, some scholars affirm the policy effects of these concepts: Liu et al. (2019) argue that green credit policy, property rights and debt financing for the first time and found that the green credit policy system plays a guiding role in the allocation of credit resources. Zhang et al. (2022) believed that the green credit policy not only affects the financing cost of corporate debt by changing the financing scale and commercial credit, but also affects the financing cost of corporate equity by changing the financial situation and the degree of information disclosure. Zhou et al. (2021) used a double difference method to study green credit policy, and found that green credit enables green enterprises to obtain a greater range of credit resources and lower credit costs, and that green credit policies ultimately achieve a Pareto improvement in financial resource allocation. However, some scholars question this: Xue and Zhu (2021) argue that the overall effect of green credit policies in China are not yet satisfactory; although they can improve the financing of green enterprises to some extent, enterprises who do not meet the criteria can simply seek alternative financing options, and green credit policy does not have a wide enough control of the market to effectively control the flow of funds.
From the perspective of green credit and energy industry, On the one hand, some scholars call for green credit to play a role in the development of clean energy enterprises. Bei and Wang (2023) believes that due to the reduction of energy demand caused by the global economic recession, renewable energy projects have lost the interest of public and private capital participation, and more need green credit to help them practice sustainable goals. Zhang et al. (2021) found that green credit effectively promoted financing in the renewable energy sector and played a mediating role for short-term debt, but was insignificant against long-term debt. The research of Ren et al. (2020) found that the impact of green financial policy on China’s non-fossil energy consumption continues to be insufficient and lacks continuity. He et al. (2019a) used empirical analysis to study the mediating effects of green financial development on renewable energy investment efficiency through bank loans, short-term loans, and long-term loans, and the results indicated that green financial development inhibited the improvement of renewable energy investment efficiency.
Meanwhile, some scholars have studied the effect of green credit on corporate innovation: Sun and Shi (2019) argued that, compared with conventional bank credit, environmentally harmful enterprises have responded to being subjected to stricter environmental constraints by beginning to use more environmentally friendly technologies and products, while green enterprises that meet banks’ environmental judging criteria can promote technological innovation by obtaining green credit. Wang H et al. (2022) believed that the inhibition effect of green credit only exists in companies with performance deficits. When enterprises have performance surpluses, green credit policy will promote green technology innovation. Xiao et al. (2022) found that green credit policies are conducive to greener production methods and more sustainable economic development through the PSM-DID approach. Regarding the heterogeneity of the impact, Chen S et al. (2022) argue that green credit policies promote low-carbon technology innovation, an effect which is more pronounced for state-owned enterprises (SOEs) and ESG-certified enterprises. Zhou et al. (2022) found that there is significant regional heterogeneity in green credit for green economic growth, and this impact is significantly stronger in the eastern region of China compared to the central and western regions.
Several scholars have also pointed out certain issues with green credit currently: Guo and Zeng (2021) identified that too wide a scope of credit would lead to an unnecessarily high supply of funds for green enterprises, and too narrow a scope would result in an excessively high loan threshold, outcomes of which would both hinder industrial growth performance. Lu et al. (2021) found that although green credit policies can influence internal decision making of enterprise and inter-enterprise resource reallocation through the establishment of a dual constraint mechanism of environmental access thresholds and credit quota control, in practice, the policy effects of compliance cost and credit constraint inhibit corporate innovation practices.
In general, scholars have different opinions on green credit. The current research on green credit policy f mainly affirms the punishment and inhibition effect of green credit on polluting enterprises It is believed that green credit restricts the financing of polluting enterprises and forces them to make green transformation. Among the remaining few studies that focus on green credit and green enterprises, few directly involve the clean energy industry. Although previous studies have shown the impact of green credit on enterprise financing and technological innovation, there is very little research on the specific impact mechanism and the actual effect of policies in the clean energy industry.
The contributions of this paper are threefold: firstly, this study specifically selects the clean energy industry which was relatively less studied, and evaluates the impact of green credit policies on technological innovation in clean energy enterprises. Through this, this paper expands research on green credit and clean energy industry, providing insights for the energy transition in developing countries and emerging economies. Secondly, this paper constructs quasi-natural experiments based on the Green Credit Guidelines from 2008–2015 and 2008–2020 respectively, in order to determine any changes in the effects of green credit policies over given periods of time after their implementation. Thirdly, this paper reveals the impact mechanisms of green credit for clean energy enterprises’ technological innovation from the channels of credit cost and financing constraints, and identifies differences in the effects of policy implementation from the perspectives of enterprise-level and regional heterogeneity.
Despite the fast pace of development of the national clean energy industry over recent years, further enhancement is still required to investments in essential research in order to continuously accelerate the breakthrough of core technology under the impetus of low-carbon economic transformation. When clean energy enterprises conduct relevant green technology research and development, they face higher costs and risks, and undergo a relatively long return cycle, which makes it difficult to directly generate economic benefits (He et al., 2019b). China is also a financial market dominated by the banking sector, and the main way for its enterprises to obtain financing is through bank loans. Banks, as creditors, will try to avoid risk and carefully monitor the flow of external funds to innovative areas for commercial efficiency reasons (Morck and Nakamura, 1999). Since the implementation of green credit policies, banks are able to consider resource and environmental factors when assessing their lending capabilities, so that clean energy enterprises receive greater support in terms of policy and finances. Similarly, green credit also guides the investment direction of enterprises, making enterprises more inclined to finance environmental protection projects. When faced with financing constraints, enterprises can increase environmental investment to improve environmental quality, and alleviate credit constraints by meeting the environmental requirements of credit. Obviously, if the environmental performance is not improved or the funds are not used according to the intended purpose, the credit financing of enterprises will be restricted for a long time (Fang et al., 2022). Therefore, policies can provide economic incentives for enterprises to increase environmental investment through financing constraint mechanism. To sum up, the green credit policy will screen the lending objects according to whether they are green enterprises and whether they carry out green activities.
Nevertheless, since the green credit policy is non-mandatory for financial institutions, the question remains as to whether banks will strictly follow the policy when selecting lending targets. This matter requires further discussion, since banks, as the main executors of green credit policies, have certain flexibility in the implementation of these policies, and are by no means immune to including profitability in their discretionary scope of assessment. However, from China’s macroeconomic perspective, negative production capacity is consistently being eliminated, while emerging clean energy enterprises are not only more likely to be developed, but also to have more reliable repayment capacity, because they are in line with the current trend of green economic transformation. To that end, the banks’ objective of profitability is ultimately consistent with the environmental goal of green credit policies. Banks will be more inclined to lend to clean energy enterprises, and at the same time the obstacles for clean energy enterprises to obtain credit funding will be diminished. As the green credit policy increases the long-term funding available to clean energy enterprises by exercising the reallocation of credit resources, with the support of a longer funding cycle, enterprises are able to invest surplus funds in R&D. This allows them to gradually fill the gap consumed by credit funds to ensure repayment, with both the short-term benefits obtained from business operations and long-term benefits obtained from technological upgrades. Meanwhile, the enterprises’ R&D activities are also supported by the green credit policy, which is conducive to their continued technological innovation. In summary, it is proposed that.
Hypothesis 1. The green credit policy has a significant contribution to the technological innovation of clean energy enterprises relative to other enterprises.The increase in the accessibility of credit facilities to public enterprises implies an increase in the availability of external financing. Greater access to external financing has positive implications for technological innovation development in listed enterprises (Ayyagari et al., 2011). Prior to the implementation of the green credit policy, clean energy enterprises faced a more stringent financing environment and criteria, making it difficult for them to obtain sufficient funds for additional R&D activities due to various factors including information asymmetry, R&D risks, and bank restrictions on the use of funds. These financing constraints significantly inhibited the R&D investments in enterprises who could not access bank credit, which in turn further reduced their R&D activities going forwards (Zhang et al., 2017). Furthermore, since R&D investment is the main technological resource for corporate innovation (Brown et al., 2012), if the financing constraints faced by enterprises are too high, this may limit innovation investment due to insufficient autonomous funding, resulting in the weakening of the level of corporate investment in innovation (Xiang et al., 2021). Technological innovation often requires stable financial support, and enterprises’ R&D activities are more likely to struggle under such conditions. However, the implementation of green credit policies has transformed this situation, and green enterprises, including those operating within the clean energy industry, have been provided with more credit resources, which has alleviated their financing constraints and effectively converted potential green investment demand into productivity (Wu and Yin, 2021). In addition, due to the implementation of the green credit policy, financial institutions can also provide certain preferential interest rates for green projects when considering loans, for the purpose of guiding the development of low-carbon green industries through credit means. Clean energy enterprises can also enjoy lower credit costs through the policy and exchange fewer expenses for a greater free cash flow, so they may also obtain more funds for R&D specifically. In summary, it is proposed that.
Hypothesis 2. Green credit policy reduces the credit cost and financing constraints of clean energy enterprises, and thus promotes technological innovation of clean energy enterprises.Taking into consideration the economic and institutional situation in China, it can be seen that SOEs and non-state-owned enterprises (non-SOEs) experience certain differences in their respective financing challenges, business performance, and social responsibility, and their responses to technological innovation and green credit policies correspond to these differences. Green credit policy intends to promote the technological innovation of green enterprises by providing more credit resources to achieve the capture effect for enterprises with more green innovation intention, and this effect will demonstrate heterogeneity based on the specific nature of the enterprise’s property rights (Wang and Yang, 2018). Ownership structure is an important mechanism for enterprises to gather and guide the resources needed for R&D. The innovation efficiency of non-state-owned holding enterprises is significantly higher than that of state-owned holding enterprises (Chen et al., 2014). Due to property rights, the government’s policy convenience will naturally tilt towards state-owned enterprises. The operating environment of state-owned enterprises is usually protected by the government, and the pressure of market competition is relatively slight. Technological innovation is an effective way to enhance the core competitiveness of enterprises and seize market share. From the above financing pressure and competitive pressure, the market forces state-owned enterprises to innovate with insufficient motivation (Xu and Zhou, 2020). For the established tenure of leaders and the long- and short-term performance of enterprises, innovation, which is an investment activity with a long return cycle, is less valued in SOEs. Non-SOEs, on the other hand, tend not to prioritize short-term goals, and as such typically allocate most of the resources which they obtain through social responsibility for exploratory innovations that reap long-term benefits (Gu et al., 2019). The contradiction between the state-owned enterprise managers’ right to profit from innovation and their right to control innovation has led to the low willingness of innovation investment (Zhu et al., 2021). Based on the above motivations for innovation, the effects of green credit policies for state-owned and non-state-owned clean energy enterprises are likely to be different: SOEs have a more relaxed financing environment, better banking relationships, and, especially in the energy sector, also tend to be stronger in scale than non-SOEs due to natural and government monopolies. Furthermore, they may not be sensitive to small changes in credit costs, nor motivated to intensify their technological innovation activities to obtain credit resources. Non-SOEs, on the other hand, need to bear their own profits, losses and risks, and are mainly profit-oriented, and gaining an advantage in the market competition through technological innovation is what it needs. In addition, the financing needs of non-SOEs are often greater, yet the financing conditions they face are more stringent, so the green credit policy is welcomed by non-state-owned clean energy enterprises as an opportunity to further the development of their industry, as well as to reduce their financing constraints and credit costs. At this time, with the dual purpose of enhancing technological innovation and securing credit resources, the R&D activities of non-state-owned clean energy enterprises may be intensified compared to that when it is only for the single purpose of technological innovation. As the non-SOEs receive more comprehensive financial support and incentives, it contributes to greater levels of technological innovation. In summary, it is proposed that.
Hypothesis 3. Green credit policies have a more significant effect on technological innovation in non-state-owned clean energy enterprises relative to SOEs.The regional level of economic development also impacts the effectiveness of green credit policy implementation. In regions with higher levels of economic development, environmental problems are considered to be an important factor hindering further economic development. Therefore, governments and enterprises are likely to pay closer attention to this (Yu, 2021) and accordingly invest more credit resources in green credit. The economic foundation of regions with high economic development level is solid and the market space is larger. The introduction of green credit and free capital base can better support green projects, thus promoting the further improvement of TFP level (Hu et al., 2022). Moreover, developed regions enjoy a more developed financial market, and a higher supply and demand for green credit than in less developed regions. Therefore, in developed regions, not only will banks examine green projects more seriously and offer loans more frequently, but also enterprises face greater financing needs and are more willing to seek funding through financial institutions, so consequently clean energy enterprises in developed regions will receive a greater proportion of available green credit funds. In addition, a higher level of scientific and technological innovation and a concentrated high-tech industry have formed advantages, which can better drive the upgrading of green industries in developed regions. However, the lack of these factors in underdeveloped areas has led to the development of industrial economy mainly based on traditional heavy industry and manufacturing industry, with high pollution and high emissions (Ma et al., 2022). Therefore, both in terms of financing environment and technological innovation capability, clean energy enterprises in developed regions have greater opportunities than those in less-developed regions. In summary, it is proposed that.
Hypothesis 4. Green credit policies have a more significant impact on the technological innovation of clean energy enterprises in developed regions relative to less-developed regions.
In this paper, 91 clean energy listed A-share companies from 2008 to 2020 were selected as the experimental group and 489 non-clean energy companies were selected as the control group. In defining whether the enterprises are clean energy listed companies, according to the study of Xu et al. (2019), clean energy is first defined as hydroelectric, nuclear, wind, biomass, solar, geothermal and ocean energy. By reviewing the enterprises’ annual reports in previous years, the listed companies whose disclosed main business and main products are involved in the field of clean energy are listed as clean energy enterprises. In selecting the control group, non-clean energy industries that are not easily affected by the green credit policy, such as wholesale and retail trade, accommodation and catering, software and information technology services, education, culture, sports and entertainment, and residential services and other services, were selected in conjunction with the industry classification of the Securities and Futures Commission promulgated in 2012.
In terms of selecting the research time period, this paper takes the 2012 Green Credit Guidelines as the research event. Considering that the effects of this important green credit policy may be long-term, and the Green Credit Guidelines may not show the same effects in shorter and longer time periods after its promulgation, this paper will examine the effects of this policy in the period 2008 to 2015 and 2008 to 2020, respectively.
In addition to the original sample, we also exclude listed companies with ST and PT, gearing ratio greater than 1, missing indicators, and delisted companies during the period, and we apply a tailing process to the upper and lower 1% quantile of the continuous variables. The financial data and other data of listed companies required in this paper are obtained from the WIND database, and 3418 and 6170 observations are obtained from 2008 to 2015 and 2008 to 2020, respectively.
Dependent variable: technological innovation (TI). In this paper, the measurement of enterprise innovation emphasizes the technological innovation aspect, so it is expressed in terms of the total factor productivity (TFP) of the enterprise, following the research of Huang and Gao (2020). The improvement of TFP mainly depends on technological innovation. Through new technology, the basic production factors are optimized and combined, so as to improve the production quality and make the economy operate more effectively. For the measurement of this indicator, the OP method of Lu and Lian (2012) is used to calculate it. According to the idea of the OP method, the consistent unbiased estimated coefficients of labour term and capital direction are obtained by establishing the relationship between the capital stock and investment amount of the enterprise, and then the production function is brought back to estimate TFP. The advantage of this method is that the current investment of enterprises is used as the proxy variable of unobservable productivity shocks, which helps to solve the problem of simultaneous bias.
Independent variables: cross-product term (treat*post) for clean energy enterprises and green credit policies. For clean energy enterprises, treat takes the value of 1, otherwise 0. For years after the implementation of the Green Credit Guidelines (2012 and later), post takes the value of 1, otherwise 0.
Control variables: Referring to Wang M et al. (2021) and Ding and Hu (2020), enterprise size (size), gearing (lev), profitability (ROA), growth (growth), board independence (board), concentration of equity (hold), cash flow (cash), capital intensity (fix) and GDP (GDP) are selected, as detailed in Table 1.
To examine the impact of green credit policies on technological innovation in the clean energy industry, the following model is constructed in this paper using the difference-in-difference (DID) method with the inclusion of two-way fixed effects.
Where the subscript i denotes the firm, the subscript t denotes the year, the parameters
The results of descriptive statistics are shown in Table 2 and Table 3. All variables pass the variance inflation factor and unit root test. It can be seen that the mean value of corporate technological innovation from 2008 to 2015 is 5.582 with a standard deviation of 0.399. The mean value of corporate technological innovation from 2008 to 2020 is 5.670 with a standard deviation of 0.421. This shows that the mean level of corporate innovation has increased over time and its dispersion has expanded.
The essence of the difference-in-difference method is to examine the effect of green credit policy on enterprise innovation using an experimental group and a control group with a common trend, i.e., without the implementation of green credit policy, there would not be a significant difference in enterprise innovation between the two groups. To avoid the self-selection problem of the sample, this paper proposes to use the propensity score matching (PSM) method with a radius of 0.01 to find enterprises in the control group that are as consistent as possible with the experimental group sample to investigate the effect of the policy dash.
The standardized deviations of all variables are reduced to the 10% level after matching, and indicates that the data are better matched. In addition, the parallel trend test in Figures 1, 2 shows that the trend of the sample is roughly the same before the policy impact year and shows different changes after the policy impact year, which is consistent with the premise of the DID model.
The regression results obtained after substituting the matched results into the model (1) are recorded in Table 4. Among them, columns (1) and (2) are the regression results for 2008 to 2015, and columns (3) and (4) are the regression results for 2008 to 2020. Column (1) and (3) are not added the control variables and fixed effects, and (2) column (4) comes with control variables and fixed effects. As can be seen, the regression results for 2008 to 2015 are significantly positive at the 1% level regardless of whether control variables and fixed effects are added, while the regression results for 2008 to 2020 are significantly positive at the 1% level. The regression coefficients and significance of the difference-in-difference item are increased by the extension of the policy implementation years, indicating that the green credit policy promotes the technological innovation of clean energy enterprises, confirming Hypothesis 1. The possible explanation is that the green credit policy tends to favour financial institutions to invest in green projects of enterprises, and the loan projects of clean energy enterprises meet this requirement, so their technological innovation activities are more likely to be supported by green credit funds. Since the implementation of policies and the financing and innovation activities of enterprises need time to produce results, the effect of policies in the later stage is better.
TABLE 4. Regression results of the impact of green credit policy on enterprise technological innovation.
In order to avoid the endogeneity problem that may still exist, the System GMM method is used again to test conclusions. When the TI variable lagging one phase is added to the explanatory variable of model (1) and still controls the time dummy variable, all data is brought into the two-step System GMM model. The results are as shown in Table 5. The coefficients of the DID items are still positive at a significant level. The results of AR test show that the regression disturbance term does not have a sequence correlation of more than second order. The Hansen test results are greater than 0.1, indicating that the original hypothesis of exogenous instrumental variables cannot be rejected. Therefore, the setting of System GMM model is reasonable as a whole, and confirms the conclusion that green credit policies promote technological innovation of clean energy enterprises.
Counterfactual construction: The effect of policy implementation is first tested by the counterfactual method by advancing the year of policy implementation to 2010 and matching and regressing again, and the regression results for 2008 to 2015 and 2008 to 2020 are recorded in columns (1) and (2) of Table 6, respectively. The coefficients of the difference-in-difference items for both time periods are not significant, indicating that the level of technological innovation did not produce significant changes before the implementation of the green credit policy and that the changes in technological innovation were not caused by exogenous variables.
Excluding part of the sample: the sample of enterprises registered in Guangdong Province and Beijing accounted for about 30% of the total sample, and there might be sample selection bias, so the enterprises in these two places were excluded and regressed again, and the regression results for 2008 to 2015 and 2008 to 2020 were recorded in columns (3) and (4) of Table 6, respectively. The sign and significance of the coefficients of the difference-in-difference items were consistent with the original results to support the hypothesis of this paper.
Replacing the matching method: To avoid the effect of green credit policy implementation being affected by the sample division, the sample is re-screened using the nearest neighbour 1:1 matching method and regressed again based on the matched results. Regression results for 2008 to 2015 and 2008 to 2020 are recorded in columns (5) and (6) of Table 6, respectively, which are still consistent with the previous paper.
To study the channels of green credit policy’s influence on clean energy enterprises’ technological innovation, this paper refers to the study of Wen et al. (2004) and constructs a mediating effect model from the channels of credit cost and financing constraint. The indicator of credit cost is chosen as the interest expense of the enterprise in the current year. The indicator of financing constraint refers to Hadlock and Pierce (2010) and Miao et al. (2019), and the SA index is selected to measure the degree of financing constraint. The SA index is a negative indicator, and the larger its absolute value, the greater the financing constraint of the enterprise. The intermediary effect model is constructed as follows:
Where M is the mediating variable, it is denoted by the cost of credit (cc) and financing constraint (fc) respectively.
According to the test procedure of mediating effects, firstly model (1) is regressed and if it is significant then it indicates the existence of aggregate effects and the test can be continued. Next, model (2) is regressed to determine the effect of explanatory variables on mediating variables, and finally, model (3) is regressed to test the direct effect of green credit policy on technological innovation and the indirect effect transmitted through mediating variables. If
Given the significance of the impact of green credit policy on technological innovation, the sample for the period 2008 to 2020 were regressed in this paper, with the results shown in Table 7. The regression coefficients of the control variables are not shown due to space constraints, and the regression results of the total effect in columns (1) and (4) are consistent with the baseline regression in the previous section. The coefficients of the difference-in-difference item and the mediating variables in columns (3) and (6) are both significant at the 1% level, but the regression coefficients of green credit policy on the mediating variables in columns (2) and (5) are not significant. Therefore, further tests are needed. In this paper, the coefficients of the mediating effects are tested and calculated by using the Sobel test at the 1% level of significance. The results show that the mediating effect of credit cost is negative, and the mediating effect of financing constraints is positive. It indicates that the green credit policy leads to the reduction of interest expenses and credit cost of clean energy enterprises, while the SA index of clean energy enterprises increases and credit constraints are reduced, thus promoting technological innovation. Hypothesis 2 is therefore verified.
In addition, in order to eliminate the dimension influence, the method of deviation standardization is used to process all variables, and also brings them into the above models for inspection. The results can still support the conclusions, and the process is not shown to save space.
Considering the different financing environments and strategic goals, the enterprises are divided into state-owned and non-state-owned based on the nature of property rights. Sub-sample regressions are conducted in model (1), and the regression results are shown in Table 8. (1) and (2) are the regression results for SOEs from 2008 to 2015 and 2008 to 2020; (3) and (4) are the regression results for non-SOEs for the years 2008–2015 and 2008 to 2020. For SOEs, the effect of the green credit policy on technological innovation was not significant from 2008 to 2015 but showed a significant effect from 2008 to 2020, indicating that the green credit policy did not significantly promote technological innovation of state-owned clean energy enterprises in the first years of implementation, and the enhancement effect of the green credit policy on technological innovation was only shown in the longer years of policy implementation. This may be because the financing constraints of state-owned enterprises are relatively light, and they are not sensitive to the green credit policy at first. The unique strategy adopted by state-owned enterprises to improve environmental performance is highly responsive to government objective (Wang Q et al., 2022), rather than making profits in the market. For the non-state-owned enterprises, the effect of the green credit policy on technological innovation is significant in both the years, from 2008 to 2015 and 2008 to 2020. From the short-term to long-term implementation of the green credit policy, the level of technological innovation of non-state clean energy enterprises has been improved. This is because non-state-owned enterprises are facing a worse financing environment for a long time. At the same time, market competition requires enterprises to continue technological innovation, and there is a gap in R&D funds. Private enterprises are more vulnerable to the impact of policies, and have relatively high requirements for green technological innovation, which is easy to produce technical and economic effect (Wang Y et al., 2021). When the green credit policy is implemented, non-state-owned enterprises can immediately utilize these policies to ease the pressure in the past. On the whole, the green credit policy has no obvious impact on state-owned enterprises before 2015, and has obvious impact on both state-owned and non-state enterprises before 2020. Therefore, the implementation effect of green credit policy in non-state enterprises is better. Hypothesis 3 is verified.
Considering that the imbalance of regional economic development affects the financing and innovation behaviors of enterprises, this paper conducts a heterogeneous study based on regional economic development levels. Referring to the study by Chen Z et al. (2022), a total of eight provincial regions, namely Zhejiang, Jiangsu, Shanghai, Fujian, Shandong, Guangdong, Beijing, and Tianjin, are classified as developed regions and other provincial regions as less developed. Samples are classified according to the registered locations of enterprises and substituted into the model (1) for regression respectively. The obtained results are shown in Table 8. (5) and (6) are the regression results for the developed regions from 2008 to 2015 and 2008 to 2020, and (7) and (8) are the regression results for the less developed regions for 2008 to 2015 and 2008 to 2020. In developed regions, the significance and influence coefficient of green credit policy on technological innovation have been improved. This is because the effect of green credit policy needs a certain period of time. Developed regions have good innovation endowment and financial resources, which is very suitable for enterprises to benefit from green credit. In less developed regions, the impact between the 2 years is not very significant., indicating that the effect of green credit policy on technological innovation of clean energy enterprises in less developed regions is weak. The possible reason is that the transmission of green credit policy in less developed region is too slow, the local financial development level and enterprise strength are also poor, and it is not easy to make progress in technological innovation. The performance of green credit policy in developed regions is obviously better than that in less developed regions, so Hypothesis 4 is verified.
Green credit policy, as an important environmental regulation tool, can promote green economic transformation through the rational allocation of credit resources and guide clean energy enterprises’ technological innovation. This paper examines the impact and mechanism of green credit policy on the technological innovation of clean energy enterprises. It uses the difference-in-difference method with the introduction of the 2012 Green Credit Guidelines as a quasi-natural experiment and Chinese A-share listed clean energy enterprises as the research objects. The study shows that: 1) the green credit policy represented by the Green Credit Guidelines has a significant positive effect on the technological innovation of clean energy enterprises, and the longer the policy is implemented, the more significant the effect is. 2) The green credit policy represented by the Green Credit Guidelines significantly reduces the financing cost and the degree of financing constraint of clean energy enterprises as a mechanism to influence the technological innovation of clean energy enterprises. 3) Heterogeneity analysis shows that the effect of green credit policy on the technology innovation of clean energy enterprises is more significant in non-state enterprises and economically developed regions.
Based on the above conclusions, this paper has the following implications for the green credit policy and the development of clean energy industry in China and other developing countries and emerging economies. Firstly, it is vital to enhance the level of green credit development and formulate long-term effective development plans. At present, the implementation period of green credit policy in China is still relatively short, and the positive effect on technological innovation and industrial guidance needs to be further promoted. It is necessary to insist on the implementation of a green credit system and to guide China’s energy transformation and sustainable economic development with a higher level of green credit. Secondly, in the process of policy implementation, it should be considered that the impact of green credit on enterprise innovation is achieved through credit costs and financing constraints. To this end, the government and financial institutions need to strengthen policy support, provide more preferential interest rates for enterprises in need, and try to improve the cooperative relationship between banks and enterprises, open up the channels of policy influence, and stimulate innovation vitality. Thirdly, the green credit policy should be paid more attention in state-owned enterprises and underdeveloped areas. State-owned clean energy enterprises should seize the policy opportunities, make proper use of green credit funds, strengthen technological innovation, and make contributions to the high-quality development of the clean energy industry. It is also important to increase policy support in underdeveloped areas and expand the coverage of green credit. Stimulate the innovation impetus of clean energy enterprises in underdeveloped regions, and further allocate green credit resources in a reasonable way.
The raw data supporting the conclusions of this article will be made available by the authors, without undue reservation.
Conceptualization, ZT and XG; methodology, ZT and XG; software, ZT and XG; validation, ZT and XG; formal analysis, ZT; investigation, ZT; resources, ZT; data curation, ZT; writing—original draft preparation, ZT; writing—review and editing, XG; supervision, XG; project administration, XG; funding acquisition, XG All authors have read and agreed to the published version of the manuscript.
This research was funded by Humanity and Social Science Research Project of the Ministry of Education of China 22YJA790015.
We are grateful for the financial support from the Research Project of the Humanity and Social Science Fund of the Ministry of Education of China (No. 22YJA790015) and the National Natural Science Foundation of China 71703007.
The authors declare that the research was conducted in the absence of any commercial or financial relationships that could be construed as a potential conflict of interest.
All claims expressed in this article are solely those of the authors and do not necessarily represent those of their affiliated organizations, or those of the publisher, the editors and the reviewers. Any product that may be evaluated in this article, or claim that may be made by its manufacturer, is not guaranteed or endorsed by the publisher.
Ayyagari, M., Demirgüç-Kunt, A., and Maksimovic, V. (2011). Firm innovation in emerging markets: The role of finance, governance, and competition. J. Financial Quantitative Analysis 46 (6), 1545–1580. doi:10.1017/s0022109011000378
Bei, J., Wang, C., and Huang, Z. (2023). Renewable energy resources and sustainable development goals: Evidence based on green finance, clean energy and environmentally friendly investment. Resour. Policy 74, 102402.
Brown, J. R., Martinsson, G., and Petersen, B. C. (2012). Do financing constraints matter for R&D? Eur. Econ. Rev. 56 (8), 1512–1529. doi:10.1016/j.euroecorev.2012.07.007
Chen, S., Lei, B., and Feng, H. (2022). Configuration path of industrial digitalization in developed and underdeveloped areas - Analysis Based on the theoretical framework of "technology organization environment. Sci. Res. 40 (03), 410–419. in Chinese.
Chen, V. Z., Jing, L., Shapiro, D. M., and Zhang, X. (2014). Ownership structure and innovation: An emerging market perspective. Asia Pac. J. Manag. 31 (11), 1–24. doi:10.1007/s10490-013-9357-5
Chen, Z., Zhang, Y., Wang, H., Ouyang, X., and Xie, Y. (2022). Can green credit policy promote low-carbon technology innovation? J. Clean. Prod. 359, 132061. doi:10.1016/j.jclepro.2022.132061
Ding, J., and Hu, R. (2020). Regional environmental regulation and the effectiveness of green credit policy - from the perspective of credit financing for heavily polluting enterprises. Soft Sci. 34 (12), 61–67. in Chinese.
Fang, J., Gozgor, G., and Nolt, J. H. (2022). Globalisation, economic uncertainty and labour market regulations: Implications for the COVID-19 crisis. World Econ. 45 (08), 2165–2187. Chinese. doi:10.1111/twec.13230
Gu, Q., Wang, W., and Zheng, Y. (2019). Will CSR affect innovation— from the perspective of R and D heterogeneity and property right nature. J. Guizhou Univ. Finance Econ. 06, 66–75. in Chinese.
Guo, W., and Zeng, X. (2021). Has green credit improved industrial green total factor productivity— empirical study based on spatial durbin model. Econ. Probl. 08, 44–55. in Chinese.
Hadlock, C. J., and Pierce, J. R. (2010). New evidence on measuring financial constraints: Moving beyond the KZ index. Rev. Financial Stud. 23 (5), 1909–1940. doi:10.1093/rfs/hhq009
He, L., Liang, X., Yang, X., and Zhong, Z. (2019b). Can green credit promote technological innovation of environmental protection enterprises. Res. Financial Econ. 34 (05), 109–121. in Chinese.
He, L., Liu, R., Zhong, Z., Xia, Y., and Xia, Y. (2019a). Can green financial development promote renewable energy investment efficiency? A consideration of bank credit. Renew. Energy 143, 974–984. doi:10.1016/j.renene.2019.05.059
Hu, Q., Li, F., and Xue, Y. (2022). Do green credit affect green total factor productivity? Empirical evidence from China. Front. Energy Res. 9. doi:10.3389/fenrg.2021.821242
Huang, T., and Gao, B. (2020). Financial development, financing constraints and enterprise innovation. Discuss. Mod. Econ. 03, 22–32. in Chinese.
Liu, X., Wang, E., and Cai, D. (2019). Green credit policy, property rights and debt financing: Quasi-natural experimental evidence from China. Finance Res. Lett. 29, 129–135. doi:10.1016/j.frl.2019.03.014
Lu, J., Yan, Y., and Wang, T. (2021). Research on the micro effect of green credit policy - from the perspective of technological innovation and resource reallocation. China's Ind. Econ. 01, 174–192. in Chinese.
Lu, X., and Lian, Y. (2012). Estimation of total factor productivity of Chinese industrial enterprises: 1999-2007. Econ. Q. 11 (02), 541–558. in Chinese.
Ma, Y., Tong, L., and Xiao, Q. (2022). The relationship between environmental regulation, green-technology innovation and green total-factor productivity—evidence from 279 cities in China. Int. J. Environ. Res. Public Health 19 (23), 16290. doi:10.3390/ijerph192316290
Miao, M., Su, Y., Zhu, X., Jiang, Y., and Zhang, H. (2019). The impact of environmental regulation on Enterprise Technological Innovation - a test of intermediary effect based on Financing Constraints. Soft Sci. 33 (12), 100–107. in Chinese.
Morck, R., and Nakamura, M. (1999). Banks and corporate control in Japan. J. Finance 54 (1), 319–339. doi:10.1111/0022-1082.00106
Ren, X., Shao, Q., and Zhong, R. (2020). Nexus between green finance, non-fossil energy use, and carbon intensity: Empirical evidence from China based on a vector error correction model. J. Clean. Prod. 277, 122844. doi:10.1016/j.jclepro.2020.122844
Sun, Y., and Shi, B. (2019). The impact of green credit policy on Enterprise Innovation - an Empirical Study Based on psm-did model. Ecol. Econ. 35 (07), 6. in Chinese.
Wang, H., Wang, S., and Zheng, Y. (2022). China green credit policy and corporate green technology innovation: From the perspective of performance gap. Environ. Sci. Pollut. Res. Int. 1, 1–13. doi:10.1007/s11356-022-23908-7
Wang, M., Zhang, H., Li, Y., and Tong, P. (2021). Research on the dual heterogeneity of the transmission path of green technology innovation performance - based on the survey data of 642 industrial enterprises. Sci. Sci. Technol. Manag. 42 (08), 141–166. in Chinese.
Wang, Q., Liu, M., and Zhang, B. (2022). Do state-owned enterprises really have better environmental performance in China? Environmental regulation and corporate environmental strategies. Resour. Conservation Recycl. 185, 106500. doi:10.1016/j.resconrec.2022.106500
Wang, X., and Yang, Y. (2018). Dynamic evolution of enterprise green technology innovation: Resource capture or value creation. Financial Sci. 12, 53–66. in Chinese.
Wang, Y., Lei, X., and Long, R. (2021). Does the green credit policy improve the investment efficiency of enterprises— from the perspective of financial resource allocation of heavily polluting enterprises. Popul. Resour. Environ. China 31 (01), 123–133. in Chinese.
Wen, Z., Zhang, L., Hou, J., and Liu, H. (2004). Intermediary effect test procedure and its application. J. Psychol. 05, 614–620. in Chinese.
Wu, H., and Yin, D. (2021). The "reward" and "punishment" of green credit policy on corporate debt financing - effect evaluation based on quasi natural experiment. Contemp. Finance Econ. 02, 49–62. in Chinese.
Xiang, G., Wu, C., and Hu, X. (2021). Financing decision, financing constraint and enterprise innovation investment - Empirical Evidence Based on GEM listed companies. Mod. Manag. Sci. 08, 57–67. in Chinese.
Xiao, Z., Lu, Y., Liu, Y. X., Yin, B., and Yin, Z. (2022). Does green credit policy move the industrial firms toward a greener future? Evidence from a quasi-natural experiment in China. Front. Environ. Sci. 9. doi:10.3389/fenvs.2021.810305
Xu, B., Chen, Y., and Shen, X. (2019). Clean energy development, carbon dioxide emission reduction and regional economic growth. Econ. Res. 54 (07), 188–202. in Chinese.
Xu, H., and Zhou, X. (2020). Research on the impact of institutional environment and integration of industry and finance on enterprise innovation performance. Sci. Res. 38 (01), 158–168. in Chinese.
Xue, J., and Zhu, D. (2021). Can the green credit policy improve the debt financing of listed companies? Econ. Surv. 38 (01), 152–160. in Chinese.
Yu, B. (2021). How the green credit policy affects the technological innovation of heavily polluting enterprises? Econ. Manag. 43 (11), 35–51. in Chinese.
Zhang, K., Wang, Y., and Huang, Z. (2021). Do the green credit Guidelines affect renewable energy investment? Empirical research from China. Sustainability 13 (16), 9331. doi:10.3390/su13169331
Zhang, M., Zhang, X., Song, Y., and Zhu, J. (2022). Resource allocation effect of green credit policy: Based on DID model. J. Clean. Prod. 375.
Zhang, X., Liu, B., Wang, T., and Chun, T. (2017). Credit rent-seeking, financing constraints and enterprise innovation. Econ. Res. 52 (05), 161–174. in Chinese.
Zhou, G., Liu, C., and Luo, S. (2021). Resource allocation effect of green credit policy: Based on DID model. Mathematics 9 (2), 159. doi:10.3390/math9020159
Zhou, G., Zhu, J., and Luo, S. (2022). The impact of fintech innovation on green growth in China: Mediating effect of green finance. Ecol. Econ. 193, 107308. doi:10.1016/j.ecolecon.2021.107308
Keywords: green credit, clean energy, industrial development, corporate innovation, quasi-natural experiment
Citation: Gu X and Tian Z (2023) Does the green credit policy promote the technological innovation of clean energy enterprises? Empirical evidence from China. Front. Energy Res. 11:1112635. doi: 10.3389/fenrg.2023.1112635
Received: 30 November 2022; Accepted: 20 January 2023;
Published: 02 February 2023.
Edited by:
Zhiming Yang, University of Science and Technology Beijing, ChinaReviewed by:
Zheming Yan, Xi’an Jiaotong University, ChinaCopyright © 2023 Gu and Tian. This is an open-access article distributed under the terms of the Creative Commons Attribution License (CC BY). The use, distribution or reproduction in other forums is permitted, provided the original author(s) and the copyright owner(s) are credited and that the original publication in this journal is cited, in accordance with accepted academic practice. No use, distribution or reproduction is permitted which does not comply with these terms.
*Correspondence: Zehua Tian, dGlhbnplaHVhMTAxMkBxcS5jb20=
Disclaimer: All claims expressed in this article are solely those of the authors and do not necessarily represent those of their affiliated organizations, or those of the publisher, the editors and the reviewers. Any product that may be evaluated in this article or claim that may be made by its manufacturer is not guaranteed or endorsed by the publisher.
Research integrity at Frontiers
Learn more about the work of our research integrity team to safeguard the quality of each article we publish.