- 1College of Economics and Management, Shenyang Agricultural University, Shenyang, China
- 2College of Innovation and Entrepreneurship, Quzhou University, Quzhou, China
Introduction: Household energy transition is the key to changing and upgrading China’s energy consumption pattern. Directly using traditional biomass fuels is not only one of the reasons why the opportunity between urban and rural areas is inequality but also a critical symbol of the inequality of energy consumption within rural areas.
Method: This study investigates the association between two information acquisition mechanisms, namely, Internet use and social interaction, and rural household energy transition, using CGSS 2015. After converting the consumption of each fuel to standard coal, according to the energy ladder theory, this study classifies the main types of household energy into three categories: primitive fuels, transition fuels, and advanced fuels. Then this study uses the ordered probit model to empirically analyze 1023 rural household samples in China.
Results: The results show that, compared to rural households that never use the Internet, an increase in the frequency of Internet use significantly enhances the probability of rural households using advanced fuels, while decreasing the probability of using primitive and transition fuels simultaneously. However, the effect direction of social interaction works is the opposite of Internet use completely. The intrinsic mechanism result shows that although social interaction reduces the strength of the role of Internet use in rural household energy transition, it has not yet completely offset the positive effect of Internet use on the rural household energy transition.
Discussion: The results of this study provide references for removing the blocking barriers to contact and use of the Internet by rural residents, improving the perceived quality of obtained information through social interaction, and solidly promoting rural energy transition and sustainable development of resources and the environment.
1 Introduction
In the face of global climate change, China is actively implementing the Paris Agreement, responding to the United Nations Sustainable Development Goals (SDGs). And the SDG71, ensuring access to affordable, reliable, sustainable, and modern energy for all, is precisely an indispensable part of promoting a comprehensive green transformation of China’s economic and social development2. However, the global developing world population, nearly 2.7 billion people, still uses kerosene and solid biomass fuels to meet their cooking and lighting needs3. Especially in rural areas of Asian developing countries and sub-Saharan Africa, traditional biomass fuels are dominant in household energy consumption (Karekezi and Kithyoma, 2002; Pokharel., 2007; Miah et al., 2010). Although the traditional biomass resource is renewable, crudely using way through direct combustion is not only detrimental to improving the living standard of rural households but also hinders developing countries from achieving high-quality economic development by improving the efficiency of the traditional biomass resource and exploring other environment-friendly purposes of these resources (Han et al., 2018). As one of the three largest energy consumers and carbon dioxide emitters throughout the world (Magazzino et al., 2021), China has achieved rapid economic growth by introducing foreign direct investment and vigorously developing steel and other heavy industries in the first 30 years of reform and opening up policy (Marco and Cosimo, 2020). However, while enjoying rapid economic growth, China is also suffered from serious air pollution and ecological environmental degradation (Udemba et al., 2020). In order to balance two goals, economic growth and environmental protection, the Chinese government announced that economic development has entered a "new normal" phase on 10 December 2013. This assertion implies that China’s economic development mode has shifted from focusing solely on the economic growth rate to achieving high-quality growth that is more sustainable economically and environmentally, and ultimately brings better social benefits to the Chinese people (Green and Stern, 2016). In this context, the determinants of reducing China’s energy consumption have quietly changed from improving energy efficiency to upgrading industrial structure, and the growth mode of China’s energy consumption is changing from investment-driven to consumption-driven (Mi et al., 2018).
The 2017 Global Energy & CO2 Status Report published by International Energy Agency (IEA) shows that the household sector is the largest energy consumer only second to the industry department4. Directly consuming traditional biomass fuels by rural households not only affects indoor and outdoor air quality but also increases the pathogenic risk (Liu and Bae, 2018; Tao et al., 2018). Although China eliminated absolute poverty in 2020, there are still more than 500 million rural people in China5, and opportunity inequality in energy consumption still exists between urban and rural residents (Shi, 2019). Especially for rural households, directly using traditional biomass fuels has become an important indicator of energy consumption inequality within rural areas (Wu et al., 2017). Cooking and space heating are the two most important terminal activities of household energy consumption (Zheng et al., 2014). The latest data on domestic cooking fuels in China shows that 67.2% of Chinese households use gas as cooking energy, 18% of Chinese households use electricity as cooking energy, and only 9.8% still use traditional biomass fuels such as firewood and straw to cook food, mainly distributed in rural areas of China6. Whereas for space heating, Chinese rural households have to use devices consuming electricity, coal, and firewood to satisfy heating needs because they have no infrastructure for central heating (Shi et al., 2021). Because the income level of rural households is relatively lower, they had not enjoyed any form of fuel subsidy when they use heating equipment (Zheng et al., 2014; Tao et al., 2018), which leads them to lack incentives to abandon non-clean fuels and start using clean fuels simultaneously. Therefore, the rural household energy transition is crucial for the transformation of energy consumption patterns in China (Xie et al., 2022).
The energy ladder theory indicates that household income is the most important driving factor for rural household energy transition (Leach, 1992). As a matter of fact, with income increasing, the use of modern clean fuels by Chinese rural households will not be completely synchronized with the upgrading direction of the energy ladder (Yadav et al., 2021), but a state of simultaneously using high-quality energy and low-quality energy (Zheng et al., 2014; Wu et al., 2017; Shi et al., 2021). Such low-quality energy represented by traditional biomass fuels still occupies the primary position in household energy consumption, with a proportion between 57% and 62% (Wang et al., 2017; Wu et al., 2019; Zou and Luo, 2019), showing typical energy stacking phenomenon (Masera and Navia-Antezana, 1997; Han et al., 2018). The reasons can be classified into two aspects. From the micro perspective, household economic factors mainly influence Chinese rural households to stop using traditional biomass fuels, and non-economic factors encourage them to start using clean fuels. (Carter et al., 2020). From the perspective of policy orientation, the lack of emphasis on information and education in established policies has led to the actual effects of policies deviating from the expected ones (Wu and Han, 2022).
Since the release of the Broadband China Strategy in August 2013, China has taken the Internet as a powerful tool to promote economic structural reform and innovation and improve people’s lives. It has not only broken the barriers to entry between traditional industries but also greatly changed people’s thinking methods (Wang et al., 2016). By December 2021, the number of rural Internet users in China has reached 284 million, and the Internet penetration rate in rural areas has reached 57.6 percent7. Although the Internet access gap between urban and rural areas has narrowed somewhat, there is still a large gap. Traditional rural areas in China are typical acquaintance society because it lacks necessary transportation networks (Qiu et al., 2022). Despite the rapid development of the Internet in Chinese rural areas in recent years, social interaction contacting through relationship networks is still an important way for rural households to obtain information (Qiu et al., 2021). Existing studies suggest that two information acquisition mechanisms, social interaction and Internet use, both play positive roles in rural residents’ production and life (Jalan and Somanathan, 2008; Maertens and Barrett, 2013; Courtois and Subervie, 2015; Ma and Wang, 2020; Yuan et al., 2021; Zhao et al., 2021). However, some papers point out that social interaction may also have a negative effect on rural households (Chen et al., 2017; Guo and Marchand, 2019; Li and Li, 2020). Moreover, the result of the intrinsic mechanism between two information acquisition mechanisms is disputed in the existing literature. Some pieces of literature argue that the two information acquisition mechanisms substitute for each other(Conley and Udry, 2010; Liang and Guo, 2015; Li Q et al., 2022). However, the other pieces argue that the two information acquisition mechanisms play a complementary role to each other (Jiang et al., 2021; Xiao et al., 2022).
Accordingly, the research question is posed. Does using the Internet drive rural households to achieve the goal of energy transition in a relatively information-closed Chinese rural society? Does the social interaction mechanism still play a positive role? How do the intrinsic mechanism between two information acquisition mechanisms in the process of achieving energy transition for rural households?
To answer the above questions, this study uses data from CGSS 2015, specifies the stage in the energy transition of rural households according to the energy ladder theory, using the ordered probit model to empirically analyze the effect of two information acquisition mechanisms, Internet use, and social interaction, on the energy transition of rural households and the intrinsic mechanism between two information acquisition mechanisms. The innovations are mainly in the following three aspects. First, after calculating the quantity of standard coal produced on each fuel, this study accurately measures the current position of households in the energy transition process based on the percentage of total household energy emissions from each type of energy. This approach minimizes reporting bias from respondents and erroneous estimates due to mixed using different types of fuels (Hanna and Oliva, 2015; Zhu et al., 2018; Poddar et al., 2021; Ma et al., 2022). Second, most existing research analyzed the information acquisition mechanism of social interaction based on the premise that what people learn through their acquaintance networks is always true. However, there is little literature on the possible negative effects of social interaction. And this paper based on the analysis of information horizon theory points out the precondition that social interaction exerts the role of obtaining information. Finally, as for the mechanism of action, most of the existing literature analyzed the impact of promoting energy transition in rural households from the perspective of economic factors. In contrast, this study explores the role of Internet use in promoting rural household energy transition in the context of traditional acquaintance society, and the moderating role of social interaction in the impact of Internet use on rural household energy transition from the perspective of information access as an entry point, taking into account the reality of Internet plus in China. It is useful to find the endogenous dynamics that drive the energy transition in rural households. The main findings are as follows. First, compared to never using the Internet, rural households rarely using the Internet, frequently using the Internet, and very frequently using the Internet can significantly reduce the probability that the household’s main energy type is primitive fuels, with effect sizes of 12.97%, 10.60%, and 13.50%, respectively. Meanwhile, compared to never using the Internet, rural households rarely using the Internet, frequently using the Internet, and very frequently using the Internet can significantly increase the probability of their household’s main energy type being advanced fuels, with effect sizes of 14.49%, 11.71%, and 15.13%, respectively. Second, for each unit increase in social interaction on average, the probability that the household’s main energy type is primitive fuels and transition fuels in rural households significantly increase with effect sizes of 5.28% and 0.32% respectively. However, for each unit increase in social interaction on average, the probability that the household’s main energy type is advanced fuels in rural households significantly decreases by 5.60%. Regardless of whether putting two information acquisition mechanisms into the regression models simultaneously, these findings are still robust. Third, although social interaction weakens the degree that Internet use promotes the energy transition of rural households, social interaction does not cancel out the positive effect of Internet use on rural households' energy transition.
The rest of this study is structured as follows. Section 2 is the literature review. Section 3 presents theoretical analyses and research hypotheses. Section 4 introduces data sources, the selection of main variables and their measurement, econometric models, and descriptive statistics of key variables. Section 5 demonstrates the empirical results. The final section proposes conclusions and policy implications.
2 Literature review
2.1 Relevant studies on Chinese rural household energy transition
Existing studies on rural household energy transition in China mainly focus on two aspects. One is the amount of household energy consumption, the other is the transformation of household energy structure. Among them, studies on household domestic energy consumption basically follow the lifestyle analysis method and are divided into direct consumption and indirect consumption (Zhang et al., 2011; Hu et al., 2019). Direct household energy consumption refers to the direct purchase and consumption of energy commodities by households, which mainly includes cooking, lighting, transportation, heating, and other activities from the perspective of using. Indirect household energy consumption refers to the energy consumption indirectly generated by households to meet the needs of daily activities, which mainly includes eight items: food, clothing, household equipment and services, education, culture and entertainment services, healthcare, transportation and communication, housing and miscellaneous goods and services. In this study, household energy consumption involves only two activities, cooking and space heating, so the household energy consumption is direct only. And the household energy transition refers to the shift in households' energy choices from primitive fuels represented by animal manure, straw, and firewood to clean energy represented by LPG and electricity (Leach, 1992). This is because, for households with different transition goals, household energy transition may lead to a decline in energy consumption but may lead to an energy rebound effect (Han and Wu, 2018). Therefore, this section refines the research content to specific energy types and retrospects the relevant driving factors of energy transition and their corresponding mechanisms of action.
First, China is a vast country with great differences in climatic conditions, degree of economic development, and topographic characteristics across regions. When using rural households across the country as the study population, one must take into account the traits of different regions in terms of resource endowment, population size, and the resulting possible inequality of opportunity (Shi et al., 2014; Wu et al., 2017; Ai et al., 2021; Wang et al., 2021). Sun et al. (2012) analyzed whether there were differences in the main types of energy used by farm households in different topographical conditions, using the mountainous areas of southwestern China as an example. The results show that households living in valley areas mainly use coal, while households living in high-altitude areas mainly use firewood. To explore the extent to which regional resource endowments affect rural households' energy use, Song et al. (2018) used data from nature reserves in eastern China as an example to explore the role of ecological conservation policies in promoting rural household energy transition. The results show that neither the Ecological Welfare Forestry Program nor the Conversion of cropland to forestry program can help households abandon the use of fuelwood or adopt more modern fuels and that rural residents living in nature reserves are still at the beginning stages of the household energy transition.
Secondly, most of the existing studies are based on the energy ladder theory to analyze the intrinsic mechanisms of driving the energy transition and upgrading of rural households mainly from the supply and demand sides and economic perspective. On the demand side, labor wage rates in the nonfarm sector can not only directly contribute to household energy transition by increasing farmers' opportunity costs of collecting traditional biomass fuels and promoting farmers' participation in nonfarm work and increasing nonfarm income (Qiu et al., 2018; Ma et al., 2019), but also stimulate farmers' positive emotions and optimistic expectations for the future, helping them jump out of the energy poverty trap (Chang et al., 2020). On the supply side, the availability of a stable supply of clean energy in the market, whether the region invests in end-use devices for clean energy, the price level of clean energy in the local energy market, and whether local energy policies take into account the ability of subsidizers to pay will directly affect the effectiveness of rural household energy transition (Shupler et al., 2021; Li M et al., 2022; Xie et al., 2022).
2.2 The impact of the internet on Chinese rural families
Currently, only two papers have discussed the impact of the Internet on household energy transition. He et al. (2022) analyzing Internet use on cooking energy transition in rural households in China found that Internet use not only helps to increase their probability of using clean cooking fuels but also generates positive externalities for their acquaintances in their social networks. Emodi et al. (2022) found that Internet access drives household energy transition only exists in urban households in Nigeria, while rural households are stagnant in their energy transition process because of unaffordable Internet access devices.
However, according to the existing studies, this study finds that the changes of the Internet on rural households can be summarized into two paths. For one, it helps rural households broaden income sources and improve individuals' subjective wellbeing and economic welfare. On the other, it helps broaden rural households' information acquisition sources, enhance the perceived risk of environmental quality, improve environmental literacy, and develop the concept of sustainable development of resources and the environment.
In terms of welfare improvement, Ma (2022) used endogenous transformation models to analyze the role played by the Internet in the income gap between urban and rural residents based on data from China Family Panel Studies (CFPS) for three periods from 2014 to 2018. The results show that the availability of Internet access is the main reason for widening the income gap between urban and rural residents. And from the perspective of Internet use, the income return from Internet use is significantly higher for rural households than for urban residents, and this income return from Internet use skills plays an important role in narrowing urban-rural income gaps. Yang et al. (2021) used an endogenous transformation model to analyze the impact of cell phone Internet access on multidimensional poverty based on field survey data in rural areas of three provinces in eastern, central, and western China in 2019 to construct a relative deprivation index based on objective wellbeing and subjective wellbeing. The results indicate that cell phone Internet access helps reduce the incidence of multidimensional poverty in rural households; Vasta et al. (2022) constructed a household consumption diversity index in both quantitative and proportional dimensions, based on data from China Family Panel Studies in 2018, and measured Internet use according to whether rural households use terminal devices to access the Internet. The results show that regardless of the device used to access the Internet, Internet use significantly improves consumption diversity and quality of life in rural households.
In forming the view of sustainable development of resources and environment, Zhang et al. (2019) analyzed the effect of whether Chinese residents use the Internet on the perception of environmental quality based on the 2013 China General Social Survey data to construct the degree of the perception of environmental quality deterioration in three aspects: environmental pollution, ecological degradation and sharp decline of natural resources. The results show that Internet use has a stronger perception of the degree of environmental quality deterioration among rural residents compared to urban residents. Deng et al. (2022) measured rural residents' perceptions of environmental pollution in four areas: air pollution, water pollution, noise pollution, and soil pollution, based on data from the 2016 China Labor-force Dynamics Survey, according to the availability of Internet access devices to measure Internet use. The counterfactual analysis framework was used to analyze the effect of Internet use on the level of perceived environmental pollution in rural households and the results show that rural residents' perceptions of environmental pollution are significantly enhanced by having an Internet access device. Based on the same data, Ma and Zhu (2020) starting with Internet access terminal devices, applied a recursive bivariate probit model to analyze the effect of Internet use on Chinese residents' willingness to separate garbage. The results show that Internet use can significantly increase the probability of Chinese rural residents' willingness to participate in garbage classification by about 4.6 percentage points. However, for Chinese urban residents, the positive effect exerted by the Internet only increases by 2.6 percentage points, and this result is not statistically significant. Ma and Wang (2020) measured the state of Internet use by whether or not access to the Internet, based on field survey data from rural areas of three provinces in eastern, central, and western China in 2019. An endogenous treatment Poisson regression model was used to explore the relationship between rural households' Internet use and the intensity of adopting sustainable agricultural production technologies. The results show that Internet use has a significant positive effect on the intensity of adopting sustainable agricultural production technologies, but such environmental-friendly agricultural production technologies have a negative effect on both current household agricultural income and current household income.
3 Theoretical analysis and research hypotheses
3.1 Internet use and rural household energy transition
According to the information horizon theory, the information horizon is a particular structure that is formed by arranging all the contacted information sources to meet the specific goal of information seeking (Savolainen and Kari, 2004). The concept of "horizon" is based on the definition of the term "horizon" in astronomy, which can be understood as the perceived information environment of an agent, or the expected range of interests or activities. This perceived information environment is the background where the agent can select and rank all information sources according to the set goals.
In the information-seeking8 process, the agent’s attention is limited. When selecting an appropriate information source for a particular goal, the agent not only considers the external factors which are not related to the information source characteristic but also needs to consider the internal factors that are highly relevant to the information source. The external factors include the complexity of the task or problem and the motivation to achieve the goal. The internal factors are the expected time and effort searching for and using sources, and the expected quality of extracted information from sources (Choo et al., 2000). From the perspective of internal factors, the time and effort spent on contacting and using sources by agents belong to the dimension of perceived source accessibility. Accordingly, the accuracy, reliability, and usefulness of information extracted from sources belong to the dimension of perceived source quality.
The accessibility and quality of perceived sources are the criteria that determine how individuals filter and locate all available information sources within their information horizon. According to the description of the information horizon map by Savolainen and Kari (2004), the structure of the zones in which individuals arrange information sources based on their preferences is very similar to concentric circles. For this analysis, the concentric circles consist of three different radii circles. First, the zone formed by the small circle is zone 1, which places the most preferred information source by the agent. Second, the ring formed by the circle with a medium radius and the small circle is zone 2, and the placed sources in this area rank only second to the ones in zone 1. Finally, the ring formed by the circle with a large radius and medium circle is zone 3, which places the least preferred information source because the boundary of zone 3 is the "horizon" of the information horizon.
Based on the above analysis, this study classifies information sources into the interpersonal source, which is made up of the social network, and non-interpersonal sources based on the category of information carriers (Wang, 2021). From this foundation, the rationality of using the Internet as a proxy variable for non-interpersonal information sources is explained from two aspects. One is information source accessibility, and the other is information source quality.
First, from the perspective of perceived source accessibility, compared to traditional mass media, the scope of information seeking through the Internet is closer to perfect information (Qiu et al., 2016). Searchers can use their digital human capital (Bach et al., 2013) to continuously adjust and refine the search scope based on the massive information results fed by the Internet to obtain effective information that best matches their needs and accumulate their private information. Secondly, from the perspective of the perceived source quality, compared with the Internet, traditional mass media usually decide the content of the information released by themselves, which leads to the fact that searchers can only receive passively, not actively, when obtaining information through traditional mass media. In addition, mass media lacks professionalism and accuracy in its expression of information content because of the extensive audience groups, which makes the information content noisy in transmission. Therefore, the Internet can reflect the information acquisition mechanism of searchers using non-personal information sources.
Existing studies show that Internet use changes the main energy type of rural households from primitive fuels to advanced fuels not only by helping households cultivate awareness of energy conservation and learning energy knowledge (Zyadin et al., 2014; Li et al., 2021) but also by increasing household wage income (Wu and Zheng, 2022; Zhu et al., 2022). Accordingly, the first research hypothesis is proposed.
H1: Internet use will promote rural household energy transition.
3.2 Social interaction and rural household energy transition
The theory of Social Interaction suggests that interactions between actors can be generated through constraints, expectations, and preferences, which affect actors' decision-making behaviors and their outcomes (Manski, 2000). Social interaction mainly includes three types: endogenous interaction, contextual interaction, and correlated effects. In economics, endogenous interaction is not only the core of analyzing the impact of social interaction but also an important way for individuals to acquire information (Li, 2006). Based on the definition of endogenous interaction, Bikhchandani et al. (1998) defined the process of acquiring information by observing the past behavior of reference groups as social learning and pointed out that the individual’s knowledge sources include private information and social learning. To analyze the possible negative effects of endogenous interactions on decision-making behavior and its outcomes, this section next defines "farmers" as the actors who make decisions in the current period and "neighbors" as the actors who make decisions in the previous period.
Because of bounded rationality, farmers obtain information mainly by observing their neighbors' decision-making behaviors in the previous period. When the information obtained through social learning does not exceed private information, farmers will continue to obtain information through social learning until observing a large amount of evidence9 to support one of the decision outcomes10, then social learning will be stopped. At that moment, it is no longer the private information that plays a dominant role in decision-making, but the information acquired through social learning. If the farmer makes the same decision behavior as the observed neighbors, then the farmer triggers the information cascade for this decision outcome. However, the decisions made by the farmer through social learning may be wrong. If the farmer communicates directly with neighbors to obtain information, even if the neighbors can transmit and express the information truthfully and clearly, the bounded ability in perception or recall may make the transmitted information contain much noise, leading to distorted information.
In rural areas, especially in poverty-stricken areas, rural households are extremely dependent on natural resources and will choose traditional biomass fuels such as firewood, straw, and animal waste to sustain their livelihoods based on local resource endowments. Although traditional biomass fuels are classified as primitive fuels because of the negative externalities of inadequate combustion on farmers' health and environmental quality (Qiu et al., 2019), farmers prefer to spend much time collecting traditional biomass fuels, because it costs essentially nothing to obtain them in their daily lives, rather than completely abandon their use of traditional biomass fuels in their daily lives. In addition, if farmers discover through social learning that their neighbors are using advanced fuels while not completely abandoning primitive fuels or even not using advanced fuels at all, the information obtained through social learning will instead increase the probability that farmers trigger the information cascade of using primitive fuels, hindering household energy transition. In summary, the information acquisition mechanism of social learning may increase the probability to make wrong decisions, hindering energy transition. Accordingly, the second research hypothesis is proposed.
H2: Social interaction will hamper rural household energy transition.
3.3 Internet use, social interaction, and rural household energy transition
Although social interaction hinders the upgrading of the rural household energy transition, the positive effect of Internet information acquisition mechanisms still holds. Even if Banerjee and Drew, (2004) had already shown from the sampling technique that if farmers learn socially under a random sample condition, their information acquisition process through social learning is similar to acquiring information from the perfect information, thus enhancing the probability of making optimal decisions. However, this opinion ignored the reality that preferences differ between individuals, and the preferences between individuals are no longer transitive. The general equilibrium result of breaking the transitivity axiom of preferences is that individuals with the same preferences cluster into different groups, forming the "sorting effect"(Lu and Zhang, 2007). This study assumes that farmers access information only through the following two means: the Internet and social interaction11. If the Internet is not used, then the farmer will acquire information through social learning, either by observing the energy consumption behavior of neighbors or by communicating with others in the same preference group. And the farmer risks triggering the misinformation cascade, which is a disadvantage to the household energy transition. Conversely, if that farmer uses the Internet to obtain information, the amount of time spent on social interaction shrinks considerably. Accordingly, the time spent by that farmer on the Internet channel increases significantly, and eventually, its private information level rises rapidly because of Internet usage. At this time, Internet use dominates the energy decision-making of this farmer and the probability of triggering a misinformation cascade is greatly reduced. The third research hypothesis is proposed accordingly.
H3: Social interaction will weaken the positive effect of Internet use on household energy transition.Based on the above analysis process, the theoretical framework of this study is shown in Figure 1.
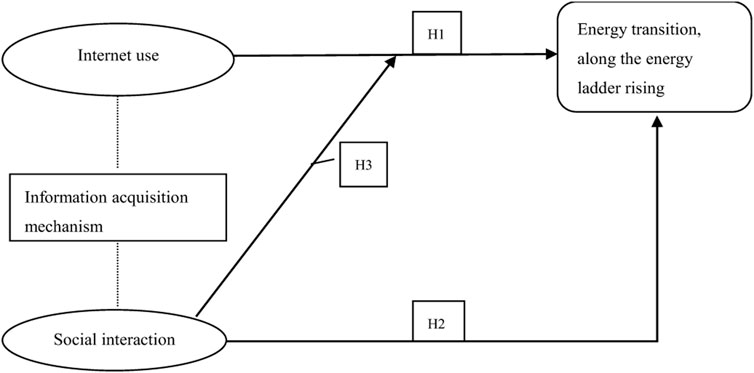
FIGURE 1. A theoretical framework for Internet use, social interaction, and rural household energy transition.
4 Data
4.1 Data sources
The Chinese General Social Survey (CGSS) is the first nationwide, comprehensive, and continuous large-scale social survey project in China. The CGSS is conducted by the National Survey Research Center (NSRC) at the Renmin University of China. A multi-stage stratified probability proportionate to size sampling method was used for this project. Firstly, this project determines the primary sampling unit of the province (or autonomous region or municipality directly under the central government), and district (or county or county-level city). Next, this project samples the streets or townships. After selecting the neighborhood committee (or village committee), the respondents and their households are identified. The purpose is to comprehensively collect basic information on Chinese people’s behavior, attitudes, life, and work, and to summarize the long-term trends of social changes in China. The project began in 2003 and has completed two stages of surveys, yielding a total of 10 high-quality annual data sets. The questionnaire for this project consists of three parts. (1) Core module. The respondents of this part are the whole sample. The survey content includes 11 sections: family relationships, socio-demographic attributes, health, migration, lifestyle, social attitudes, political participation behavior and attitudes, individual cognitive ability, labor market, social security, and family. (2) Thematic module. The respondents of this section are also the entire sample. The survey content is determined by the project team and is repeated every 5 years. (3) Additional Module. The respondents of this part are randomly selected from the whole sample. And the size of respondents in this module accounts for one-third or fourth of the whole sample. The survey content of this part is decided by the project team, and there is no guarantee of repetition period or content.
This study selected CGSS 2015 as the data source for the empirical analysis. The main reasons are as follows. The questionnaire of CGSS 2015 not only covers questions highly relevant to the use of individual information sources such as frequency of Internet use and social interaction but also records detailed information on households' housing status, electricity consumption, cooking and heating methods, fuel types, energy consumption, and so on. The data used can satisfy our requirements for calculating households' direct consumption of different types of fuels. Although in the 2015 CGSS data, the questions on the household energy consumption component are additional modules and the size of the respondents is only 1/3 of the total sample. The number of valid total samples in CGSS 2015 is 10,968. However, the respondents were still from 478 villages and neighborhood committees in 28 provinces, cities, and autonomous regions12. The distribution of respondents is still consistent with the full sample, having national representation. Because the study population is rural households, this study defines rural households as those whose hukou type is agricultural. Therefore, this study excludes respondent households with other hukou types. On this basis, samples with missing key variables and those whose response contents did not conform to the normal range were excluded, and 1023 valid samples were finally retained.
4.2 Main variables and descriptive statistics
4.2.1 Dependent variable: household main energy type
This study constructs an energy ladder following Van der Kroon et al. (2013), targeting rural households’ energy consumption in the context of cooking and space heating activities13. First, the fuel types are divided into three levels from the lowest to the highest. The first level is primitive fuels, mainly including firewood, straw, and animal waste three kinds of traditional biomass fuels. The second level is transition fuels, bulk coal, including briquettes and coal balls. The third level is advanced fuels, including liquefied petroleum gas (LPG), natural gas, pipeline water gas, and electricity. It is worth noting that although bulk coal is also by nature non-clean energy, it is traded in a completely different way from primitive fuels. Because bulk coal is a commodity energy source, rural households can only obtain it through market transactions. In contrast, farmers can use primitive fuels directly without paying money. Therefore, bulk coal ranks higher than traditional biomass fuels on the energy ladder. Secondly, according to part E89 of the questionnaire, this study transformed the household energy consumption of firewood, straw, animal manure, and bulk coal into kilograms and the energy consumption of liquefied gas, natural gas, and piped water gas into cubic meters. In terms of electricity consumption, this study is first based on the question that who provides electricity for your household and calculating the household’s electricity consumption in kWh for the past year monthly based on the answer that average monthly electricity consumption. Then, according to the standard coal coefficient in Table 1, this paper converted each fuel consumption into standard coal.
Finally, the actual consumption of primitive fuels, transition fuels, and advanced fuels are divided by the total household energy consumption respectively. This study obtains the proportion of primitive fuels consumption, the proportion of transition fuels consumption, and the proportion of advanced fuels consumption three indicators. Household main energy type is determined based on the maximum of three indicators, the proportion of primitive fuels consumption, the proportion of transition fuels consumption, and the proportion of advanced fuels consumption. The specific distribution of the household main energy types for the whole sample is shown in Table 2.
4.2.2 Independent variables measurement
This study measures Internet use based on the question of how often you have used the Internet in the past year. The values and specific meanings of the variables are as follows: 1 = never using, 2 = seldom using, 3 = sometimes using, 4 = often using, and 5 = very frequently using. A total of 649 households never went online, accounting for 63.44% of the total sample. Among the rural households who use the Internet, 81 households seldom use the Internet, 74 households sometimes use the Internet, 112 households often use the Internet, and 107 households use the Internet very frequently. Although the number of rural households using the Internet was not large in the whole sample, the proportions of rural households with different frequencies of use were in the range of 7%–11% of the whole sample steadily.
(2) Social Interaction
Chinese rural society is a typical acquaintance society. To ensure rural households obtain information within the same geo-regional context, this study, based on Zimmer and Henry, (2017) analytical process for how people use and evaluate interpersonal information sources, measures rural households' information acquisition mechanisms through social interaction in two dimensions: information accessibility and information quality. And then, summing the scores of two dimensions of rural households' acquiring information. The measurement questions, variable assignments, and calculation procedures for each dimension of social interaction are shown in Table 3. The minimum value of the social interaction score of the whole sample was 2 and the maximum value was 10, with a mean of 7.8384 and a variance of 1.3524.
4.2.3 Descriptive statistical analysis of main variables
As shown in Figure 2, rural household energy transition and upgrading largely maintain a homogeneous relationship with the frequency of Internet use. Internet use helps rural households make the energy transition mainly by encouraging them to use fewer primitive fuels while increasing their use of advanced fuels.
Figure 3 shows a bivariate descriptive statistics analysis between social interaction and rural households’ main energy type for each frequency of Internet use. The result suggests that, regardless of using the Internet or not, the reliance on the social interaction of rural households all decrease as the energy transition process upgrades. From a subsample perspective, for rural households whose main energy type is primitive fuels, social interaction is an important way to obtain information, regardless of using the Internet or not. In contrast, for rural households whose main energy type is advanced fuels, Internet use significantly reduces their propensity to obtain information through social interaction.
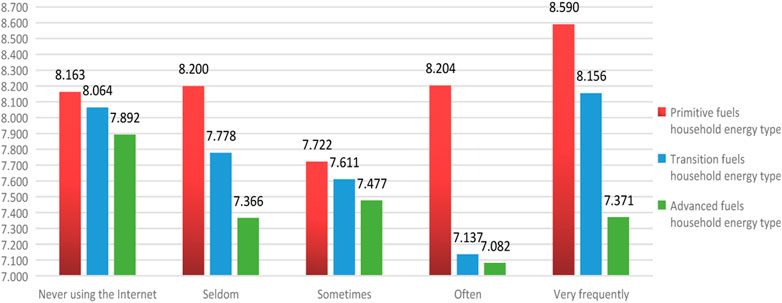
FIGURE 3. Distribution of the social interaction under different Internet usage frequencies and household main energy types.
4.2.4 Controlled variables
Controlled variables included individual characteristics of the respondent, household characteristics, and geographic characteristics. Individual characteristics include eight variables: gender, age, education, ethnicity, marital status, self-rated health status, political status, and social security (Chen et al., 2016; Ma et al., 2022; Shi et al., 2022). Household characteristics include five variables: family size, family economic status, housing area, current socioeconomic status, and the total number of using fuels in the household (Kim and Park, 2015; Zhu et al., 2018; Karatasou and Santamouris, 2019). Geographic characteristics refer to the area where the respondent is living. In addition to this, this study also controls for county or district fixed effects16.
4.3 Econometric models and estimation methods
The explanatory variable in this study is the household’s main energy type, which includes three categories: primitive fuels, transition fuels, and advanced fuels. According to the energy ladder theory, different households have the corresponding rank order in terms of main energy type. If the household’s main energy type is primitive fuels, it means that the household is still using traditional biomass fuels such as firewood, straw, or livestock manure directly to maintain its daily life, and the household is at the primary stage of the energy transition. If the main energy type of the household is transition fuels, it means that the household mainly uses bulk coal for cooking and space heating, which means that the household is in the transition stage from the primitive fuels to the advanced fuels in the energy ladder. If the household’s main energy type is advanced fuels, the household is using modern clean energy such as LPG, natural gas, piped water gas, and electricity for cooking and heating activities. And the household is at the top of the energy ladder. The ordered probit model is suitable for the type where the values of the explanatory variables are ordered and discrete. Therefore, this study refers to the analytical process of Wu and Zheng (2022) regarding residential energy use choices to construct an ordered probit benchmark regression model, as shown in Eq. 1.
Where Energy* denotes the unobservable latent variable, X1i denotes the frequency of Internet use of household i, X2i denotes the social interaction of household i, Zji denotes the j th control variable of individual i, including individual characteristics, household characteristics, and geographic characteristics.
α0 denotes the constant term, εi denotes the random error term, α1, α2, βj denote the parameters to be estimated. The relationship between Energyi, the main energy type of household i, and Energy* is as follows.
(Household’s main energy type is primitive fuels.), if
(Household’s main energy type is transition fuels.), if
(Household’s main energy type is advanced fuels.), if
r1 and r2 are the tangent points, both are parameters to be estimated.
Based on Eq. 1, the interactive term X1X2 of X1 and X2 is introduced to obtain Eq. 2. Eq. 2 is used to test the intrinsic mechanism between two information acquisition mechanisms in promoting rural household energy transition.
5 Results
First, this section uses Model 1 and Model 2 in the baseline regression to verify the effect of Internet use and social interaction respectively on the household main energy type. Second, both Internet use and social interaction are included in the regression to form Model 3. Model 3 is used to perform robustness checks on the baseline regressions. Finally, based on model 3, the interaction term of Internet use and social interaction is introduced into the regression equation to form model 4. Model 4 is used to verify the intrinsic mechanism between the two information acquisition mechanisms in driving the rural household energy transition.
5.1 Baseline regression
5.1.1 The effect of internet use on rural household energy transition
The results of model 1 in Table 4 consider only the effect of Internet use on the rural households’ main energy type. In terms of coefficients, the regression coefficients of Internet use at different frequencies are positive, and except for "sometimes use", other regression coefficients have good statistical significance. Calculating the marginal effects shows that compared to never using the Internet, seldom using, often using, and very frequently using the Internet can significantly reduce the probability that the main energy type of rural households is primitive fuels, with effect sizes of 12.97%, 10.60%, and 13.50%, respectively. Simultaneously, compared to never using the Internet, seldom using, often using, and very frequently using the Internet are all able to significantly increase the probability that the main energy type of rural households is advanced fuels, with effect sizes of 14.49%, 11.71%, and 15.13%, respectively. Although seldom and very frequently using the Internet compared to never using the Internet can significantly reduce the probability that the main energy type of rural households is transition fuels. However, the effect was very weak, and therefore no economic significance is interpreted for these results. In summary, Internet use promotes rural households' energy transition upgrade by substituting advanced fuels for primitive ones. This result is fully consistent with the findings of He et al. (2022) and Emodi et al. (2022) that the Internet can drive households toward an energy transition and upgrading process. As a new information acquisition mechanism, not only do people using the Internet quickly and accurately find solutions to their problems, but the level of private information is also increasing (Ahn and Chae, 2019; Hills and Shah, 2020; Zhang and Qiu, 2022).
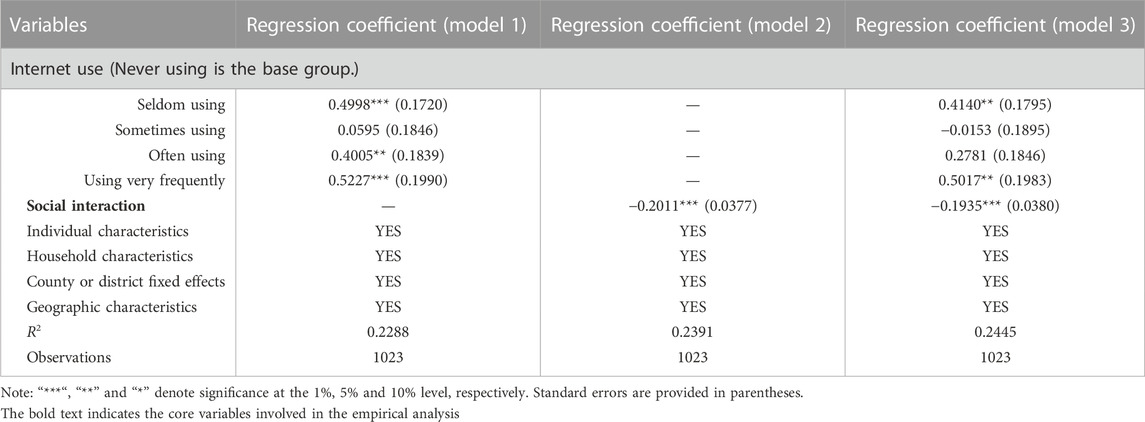
TABLE 4. Baseline regression effect results of information acquisition mechanisms on rural household energy transition.
Although this paper proves the positive role of using the Internet in promoting rural households' energy transition and upgrading once again, there remain shortcomings as follows. First, because of data limitations, this paper selected internet usage frequency only as the proxy variable for the internet information acquisition mechanism. Although the following part will test and remove potential endogeneity issues, this measure is still not perfect. The reason is that although the search engine is the primary function of the Internet, the Internet also has the functions of instant communication, business transactions, entertainment, and public services17, and the search engine is just the most fundamental one. Therefore, future researchers should consider both the internet usage frequency and usage context when measuring internet use. Second, the findings of this paper are generated by the analysis of the cross-sectional data, and whether the positive role of Internet use in promoting the energy transition and upgrading of rural households is persistent in different time points needs to be further verified. At present, with the construction of digital infrastructure, the widespread use of Internet technology contributes to enterprises expanding innovation boundaries, enhancing innovation quality and efficiency, as well as forming new commercial patterns (Shen et al., 2023). At this point, the degree of social division and specialization in the labor market will deepen (Tian and Zhang, 2022). Once rural residents are involved in the digital economy, their non-farm employment opportunities will be expanded as never before, and the probability of engaging in non-farm work will increase, which will ultimately enable them to achieve their household energy transition goals (Zhou et al., 2021; Wenfeng et al., 2022; He et al., 2022). Meanwhile, the data used in this paper did not contain certain advanced fuels such as solar energy, biogas, etc., in the energy consumption statistics of rural households, which led to the accuracy of the energy ladder built in this paper needing to be perfected. Subsequent research exploring the long-term impact of Internet use on the rural household energy transition requires not only understanding the dynamics of changes in the use of energy types by rural households to facilitate timely updates in their questions for questionnaire design but also making sufficient preparations for the organization and administration of the survey to conduct future studies and obtain high-quality panel data..
To ensure the effect of Internet use on the energy consumption of rural households is clean, the possible sources of endogeneity problems due to Internet use are discussed in detail below. It has been documented that there is likely to be a systematic difference in whether or not to use the Internet, and such selection bias may be induced by both observed and unobserved variables (Zheng et al., 2023). For example, the price of advanced fuels is typically much higher than the other two types ones, and Internet use by rural households may be positively correlated with their household wealth status (Zhou et al., 2021; Wenfeng et al., 2022). The validity of the empirical results might be doubted if endogenous regressors in the ordered probit model were not completely addressed. Therefore, this section follows the approach of Kawakatsu and Largey (2009), constructing an endogenous ordered probit (EOP) model to remove systematic biases from observed and unobserved variables in the ordered probit model.
The basic principles of the EOP model are as follows. First, Internet use is the dependent variable of the equation of stage 1, and the Internet information source preference, the instrumental variable, is introduced into the equation of stage 1, too. Second, the main household energy type is the dependent variable in the equation of stage 2, and Internet use and controlled variables are introduced in the equation of stage 2, too. Third, using the limited information maximum likelihood (LIML) algorithm jointly estimates the above two equations. In this process, the correction coefficient of the error term between the two regression equations, ρεμ, is generated. If ρεμ is statistically significant, it indicates that there is indeed a selective bias problem in the empirical results derived from directly using the ordered probit model regression. Conversely, if ρεμ is statistically insignificant, it indicates that there is no potential endogeneity problem, and the empirical results derived from directly using the ordered probit model can be trusted at this time.
Table 5 reports the empirical results using the EOP model regression. First, the bottom coefficient, ρεμ, represents whether there exists a systematic selection bias between Internet use and household main energy type. The coefficient is −0.0444 and is not statistically significant. It suggests that there is not any observed and unobserved variable simultaneously affecting both of the above behaviors when estimating the impact of Internet use on the main energy type of rural households. Therefore, this paper is justified to argue that the empirical results derived from using the EOP model are equally valid as those from directly using the ordered probit model. Next, this paper explains the empirical results in Stage 1 and Stage 2 in the EOP model.
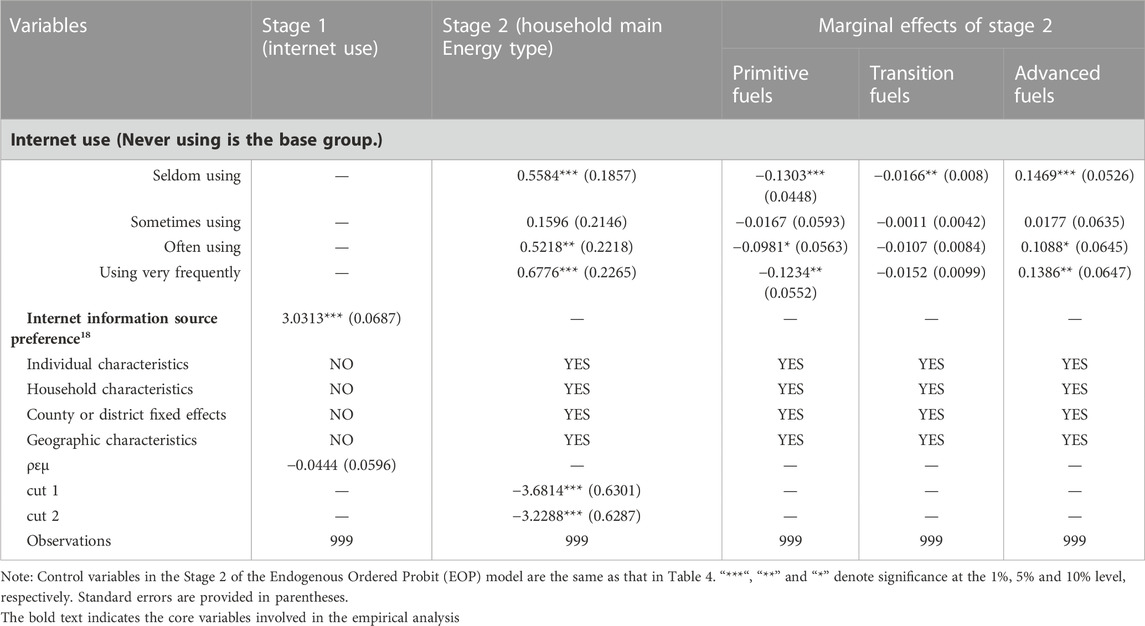
TABLE 5. The Endogenous Ordered Probit (EOP) model regression results for the Internet use on household main energy type.
In stage 1, the empirical results indicate a significant positive correlation between Internet information source preference and Internet use. In stage 2, the marginal effects calculated by the EOP model are highly consistent with those in Section 4.1.1 in terms of direction, strength, and statistical significance compared to directly using the ordered probit model. Hence, there is no need to overly worry about the estimation bias caused by observed and unobserved variables, and this paper continues directly using the ordered probit model for empirical testing.
5.1.2 The effect of social interaction on the rural household energy transition
The results of Model 2 in Table 4 consider the effect of social interaction only on the rural household energy transition. In terms of coefficients, the regression coefficient for social interaction is negative and significant at the 1% statistical level. Calculating the marginal effects shows that for each unit increase in social interaction on average, the probability that the rural households’ main energy type is primitive fuels and transition ones increases by 5.28% and 0.32% respectively. Correspondingly, social interaction decreases the probability of rural households’ main energy type being advanced fuels by 5.60%. All of these results are statistically significant at the 1% level. The above results suggest that social interaction does hinder rural households’ energy transition. Although the empirical results suggest that social interaction hinders the energy transition process of rural households, the above results inspire us to think deeply about the prerequisites for accessing information through social interaction.
First, whether rural households obtain public information through social learning depends on whether the observed neighbors use private information in their behavioral decision-making process. According to the interpretive process of social learning by Bikhchandani et al. (2008), if a neighbor’s behavioral decision is based on his or her private information, because the information has externalities, then the private information used by the neighbor in the decision-making process partially spills over through his or her behavioral decision and becomes public information accessible to the farmer. At this point, the farmer’s access to information through social interaction mechanism is established. On the contrary, if the neighbor does not base his or her private information in the decision-making process and the farmer makes the same decision-making behavior as this neighbor. At this time, the information cascade of adopting or rejecting a certain behavior is triggered, and the information acquisition mechanism through social interaction does not exist.
Second, the learning order of neighbors, the private information level of the neighbor, and the private information level of the farmer and subsequent followers are all key factors that affect the time and direction of triggering the information cascade. In terms of the direction of triggering information cascades, why people form convergences in a particular behavioral outcome is largely based on the strong assumption that the first person will not make a wrong judgment based on private information (Anderson and Holt, 2008). Bikhchandani et al. (2008), after collating the Bayesian decision rules analyzed by Welch, Bikhchandani, and Banerjee et al., stated that if the first person, Asterix, makes a wrong decision behavior based on private information, the probability that the second person, Beatrix, also makes a wrong decision will be as high as 75%. If Beatrix makes the same decision outcome as Asterix, then regardless of the level of private information of the third person, Cade, he will not make a decision based on his private information, but will directly make a decision that is consistent with Asterix and Beatrix. At this point, Cade triggered the information cascade of the wrong decision. However, the information cascade is vulnerable, how do utilize cascade effects or break misinformation cascades to guide people to converge rationally? It is well established that subsequent followers can use the response time of the foregoers to infer their private information level and thus improve learning efficiency (Frydman and Krajbich, 2022). In addition, effective prior information interventions can change individuals' attitudes or preferences and increase the probability of triggering the correct information cascade (Lee et al., 2006; Vabø and Hansen, 2014).
Compared with the existing literature, this paper extends applying the scope of information horizon theory and reveals the principle of the follower-triggered wrong information cascades. Specifically, to realize the information acquisition mechanism of social interaction, it is necessary to pay attention to information quality perception while satisfying the accessibility of information sources. The sustainable development concept is the core guidance to help developing countries gradually shift from the traditional crude development mode that relies heavily on natural resources to a modern intensive economic development mode centered on science and technology. It implies that avoiding triggering a misinformation cascade requires reaching down to foregoers' cognitive level to find out the real reasons hindering household energy transition in rural areas so that the authorities can take measures to address specific situations. If foregoers did not realize that using advanced fuels is of great importance for current socio-economic development during the specific survey about household energy, the authorities would correct their stereotypes first so that they initiate awareness of how critical and crucial the use of advanced fuels is. Once the wrong behaviors of the foregoers are corrected in time, it will be much easier to urge other local farmers to upgrade their main household energy. Conversely, if foregoers were indeed clear about the contribution of using advanced fuels to their own health and environment quality during the specific survey, then the authorities would be well advised to find out what specifically hinders local residents' energy transition and upgrade from aspects apart from cognition, develop solutions, and make the policy implementation goals more precise.
5.1.3 Robustness test
To verify the robustness of the effect of two information acquisition mechanisms on the rural household energy transition, this study simultaneously introduces both Internet use and social interaction into the baseline regression model to form Model 3, and the results are shown in Table 4. In model 3, the regression coefficient and statistical significance of social interaction are consistent with model 2. Although regression coefficients of the Internet use frequency decreased, except for sometimes using the Internet, the sign and statistical significance of the regression coefficients are largely the same as those of Model 1. In summary, whether Internet use and social interaction are introduced into the baseline regression model separately or simultaneously, the results of the effect of two information acquisition mechanisms on rural household energy transition have robust explanatory power. The research hypotheses H1 and H2 were confirmed.
5.2 The intrinsic mechanism between two information acquisition mechanisms on rural household energy transition
The results of model 4 are shown in Table 6. The regression coefficient of the interaction term is negative and statistically significant at the 10% level, which indicates that the two information acquisition mechanisms are not independent of each other in promoting the rural household energy transition. Then this study calculates the marginal effects of the two information acquisition mechanisms and the interaction term.
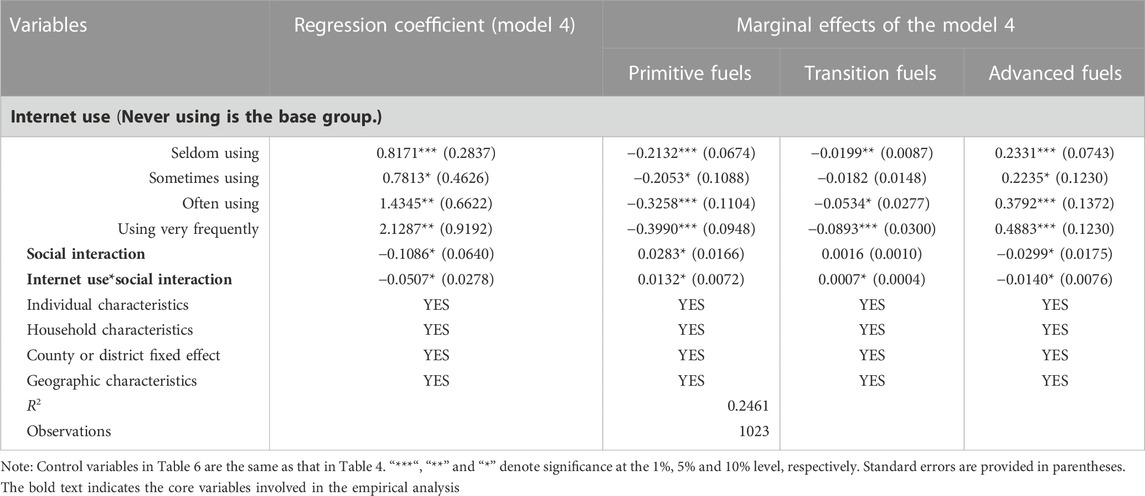
TABLE 6. Marginal effects of the Ordered Probit model with the intrinsic mechanism between two information acquisition mechanisms.
The results of the second column of marginal effects in Table 6 show that social interaction significantly weakens the effect of Internet use on rural households reducing consumption of primitive fuels. Its share in the intensity of the effect of rarely using, sometimes using, often using, and very often using the Internet is 6.19%19, 6.43%, 4.05%, and 3.31%, respectively.
The results of the third column of marginal effects in Table 6 show that social interaction significantly weakens the effect of Internet use on rural households reducing consumption of transition fuels. Its share in the intensity of the effect of rarely using, sometimes using, often using, and very often using the Internet is 3.52%, 3.85%, 1.31%, and 0.78%, respectively.
The results of the last column of marginal effects in Table 6 show that social interaction significantly weakens the effect of Internet use on rural households increasing consumption of advanced fuels. Its share in the intensity of the effect of rarely using, sometimes using, often using, and very often using the Internet is 6.01%, 6.26%, 3.69%, and 2.87%, respectively.
In summary, although social interaction can weaken the strength of the effect of Internet use in promoting the energy transition in rural households, it has not yet completely offset the positive effect of Internet use on the energy transition in rural households. Moreover, this weakening effect diminishes as the frequency of Internet use increases. Research hypothesis H3 is confirmed.
However, the results of the existing literature on the competitive relationship between the two information acquisition mechanisms are controversial. Some of the literature supports the conclusion that private information plays a decisive role. Huber, (2015) used virtual stock market trading as an example to explore the relationship between the weight that investors assign to private information and public information and their probability of triggering an information cascade. It is found that if an investor believes more in private information, he or she will assign a higher weight to private information, reinforces the belief in private information, and reduces the tendency to converge the same behavior and the probability of triggering an information cascade. Schöbel et al. (2016) analyzed the decision-making process of individuals using established information based on the information influence hypothesis. The result found that after excluding the influence of confounding factors, such as social norms, people will integrate private and public information according to their cognitive level. And people gave more weight to their private information20. Miklós et al. (2020) analyzed the causes of information cascades and argued that if people occasionally ignore information cascades in the decision-making process and base their decisions only on private information, the information cascades that lead to misbehavior will be skillfully avoided. However, it has also been argued that an individual’s private information does not always break the information cascade. Ziegelmeyer and Koessler, (2010) argued that although theoretically, subjects with high levels of private information do not trigger or fall into the information cascade effect, experimental results suggest that the probability of breaking the information cascade for subjects with high levels of private information is only one-third. This is because people’s beliefs about private information decrease as the number of people in the cascade effect increases.
In summary, it is necessary to make additional improvements to the intrinsic mechanism in individual behavior decision-making theoretical framework from the perspective of information acquisition. Future researchers need to examine the relationship between the two information acquisition mechanisms in individual behavior decision-making by combining cutting-edge methods and techniques from other subject fields.
5.3 Analysis of the results of control variables
This section provides a brief explanation of the control variables that passed significance tests in all four models. In terms of individual characteristics, compared to males, females are more conducive to promoting home energy transition and upgrading. Compared with the educated level below elementary school, education levels of junior high school, high school and equivalent, college diploma, and bachelor’s degree or above all significantly contribute to the rural households’ energy transition. Compared with Han Chinese, rural households of ethnic minorities are slightly less motivated to upgrade their energy types. Only respondents who reported their health status is relatively unhealthy used more primitive fuels and less advanced fuels to sustain their livelihoods. In terms of household characteristics, the improvement in socioeconomic status helps rural households to substitute advanced fuels for primitive fuels. There is a negative relationship between the total number of household fuels and energy transition. In terms of geographic characteristic, compared to China’s eastern regions, rural households living in central, western, and northeastern regions uses more primitive fuels and less advanced fuels for their daily needs.
6 Conclusion and policy implications
Based on CGSS 2015, this study combines rural households' energy consumption in various types of fuels with energy ladder theory to determine the main energy types of households, using the ordered probit model to empirically analyze the impact of two information acquisition mechanisms, the Internet and social interaction, on energy transition and upgrading of rural households and the intrinsic mechanism between the Internet and social interaction. The specific findings are as follows. First, compared to never using the Internet, Internet use helps rural households participate in the energy transition. In other words, the positive impact of Internet use is mainly manifested in encouraging rural households to use advanced fuels such as electricity and LPG and reducing the direct burning of primitive fuels such as firewood and straw. However, social interaction hinders the energy transition process of rural households. The above findings are robust whether the two information acquisition mechanisms are included in the regression model separately or simultaneously. Second, as to the intrinsic mechanism, social interaction weakens the intensity of Internet use in driving the energy transition process in rural households. But this weakening effect will diminish as the frequency of Internet use increases and it does not reverse the facilitating effect of Internet use in driving the energy transition.
The findings of this study have the following policy implications. First, rural grassroots organizations and relevant government departments should identify the causes of infrequent Internet use based on the actual situation of local Internet infrastructure construction. Generally speaking, there are two main reasons for the low frequency of Internet use: one is the existence of barriers to contacting the Internet, and the other is the lack of Internet use skills. Currently, as Internet infrastructure coverage continues to increase nationwide, the barrier to Internet usage is shifting from the "access divide" to the "usage divide". Second, rural China is a typical acquaintance society, and people with prestige within a village (also called elites) in a certain geo-regional range will play an important role in local economic development, social harmony, and villagers' happiness. Therefore, the government and other relevant departments must target elite groups, especially those who had been disgusted by the Internet because of inaccurate news, with special training about identifying the information content from the Internet. Such intervention activity can improve the information perceived quality from the social interaction by rural residents, reducing the probability that rural residents fall into the misinformation cascade. More importantly, it also contributes to the multiplier effect, promoting rural households to participate in energy transition and fostering the concept of sustainable development of resources and the environment. Finally, in promoting rural households participating in the energy transition and upgrading, attention should be paid to the positive effects of using advanced fuels on individuals' health. This will enable rural households to more fully consider the positive role that energy transition can play in improving the living standard in the short and long term and multiple dimensions.
Data availability statement
The raw data supporting the conclusion of this article will be made available by the authors, without undue reservation.
Author contributions
JG and JZ conceptualized and designed the study, revising it critically for important intellectual content. JG and YL coordinated and supervised data collection, carried out the initial analyses. JG drafted the manuscript and discussed with YL. YL reviewed and revised the manuscript. JZ corrected the data analyses and critically reviewed the manuscript. All authors read and approved the final manuscript. All authors contributed to the article and approved the submitted version.
Funding
This work was supported by the National Natural Science Foundation of China (72203212).
Acknowledgments
This research used data from the Chinese General Social Survey (CGSS). The authors thank the National Survey Research Center (NSCR) at Renmin University of China.
Conflict of interest
The authors declare that the research was conducted in the absence of any commercial or financial relationships that could be construed as a potential conflict of interest.
Publisher’s note
All claims expressed in this article are solely those of the authors and do not necessarily represent those of their affiliated organizations, or those of the publisher, the editors and the reviewers. Any product that may be evaluated in this article, or claim that may be made by its manufacturer, is not guaranteed or endorsed by the publisher.
Footnotes
1Source: https://sdg.js.org/index.html
2Source: http://www.nea.gov.cn/2021-01/15/c_139669173.htm.
3Source: https://iea.blob.core.windows.net/assets/680c05c8-1d6e-42ae-b953-68e0420d46d5/WEO2016.pdf (on page 77).
4Source: https://iea.blob.core.windows.net/assets/94aa834c-2f1e-4e71-9e2f-ec61467bd475/Global_Energy_and_CO2_Status_Report_2017.pdf
5Data source: http://www.stats.gov.cn/tjsj/zxfb/202105/t20210510_1817176.html
6Data source: China Population Census Yearbook 2020. Network linking: http://www.stats.gov.cn/tjsj/pcsj/rkpc/7rp/zk/indexce.htm
7The latest report is “The 49th Statistical Report on China’s Internet Development”.Network linking: http://www.cnnic.net.cn/hlwfzyj/hlwxzbg/hlwtjbg/202202/P020220407403488048001.pdf
8According to the definition of information-seeking activity by Choo et al. (2000), information-seeking activity is a series of behaviors sequentially including information need, information seek, and information use.
9Rogers (1983), analyzing the minimum conditions for farmers to trigger information cascades using the example of diffusion of agricultural technology innovation, pointed out that the information cascade effect occurs whenever farmers observe 2 neighbors who made the same decision in the previous period. At that moment, the information acquired through social learning becomes decisive for technology adoption decisions.
10Although Bikhchandani et al. (1998) explained the rationale for endogenous interaction triggered information cascades in terms of decisions with binary discrete behavioral outcomes, the theory is equally applicable when behavioral outcomes are more than two. Only the decision maker needs to acquire more information and spend much longer time through social learning, delaying the time to trigger the information cascade.
11Considering that some readers have argued that it is also possible for individuals to interact socially through the Internet. However, this study has already emphasized the research purpose that rural households use the Internet or social interaction for acquiring information. So, the use of Internet social software (e.g., Wechat, QQ, MSN) by rural households does not belong to the scope of this study. This study will introduce the conceptual operationalization process regarding social interaction in subsequent sections.
12Excluding Hong Kong Special Administrative Region, Macau Special Administrative Region, Taiwan Province, Xinjiang Uygur Autonomous Region, Tibet Autonomous Region, and Hainan Province.
13In the CGSS 2015, when asking questions related to household energy access and consumption, although diesel is an option for space heating, In rural areas, gasoline and diesel are mainly used for traffic and agricultural machinery which are not intra-household production activities. In addition, existing literature, other microdata sets, for example, China Labor-force Dynamics Survey data (CLDS), and China Health and Retirement Longitudinal Study data (CHARLS), and studies on household domestic energy consumption do not include household consumption in transportation and agricultural machinery when analyzing topics related to intra-household energy consumption, energy poverty, and clean energy use (Chinese Residential Energy Consumption Report 2015; ZHENG et al., 2016; Yang and Wan, 2022).
14According to the second law of thermodynamics, electricity is a high-grade energy, and thermal energy is a low-grade energy. In the process of converting thermal energy into electricity, there must be energy loss. Therefore, the equal value method to convert the standard coal coefficient of electricity precisely reflects the nature that electricity is a type of secondary energy. The standard coal coefficient of electricity according to the equal value method is from the “General Rules for Calculation of Comprehensive Energy Consumption (GBT2589-2020)”, whose source is: 2021081217202347802674.pdf (panzhihua.gov.cn).
15Internet use includes cell phone access.
16Due to space limitations, the variable values of the control variables and their definition cannot be reported in the text. Interested readers can contact the authors directly for a copy.
17The latest report is “The 49th Statistical Report on China’s Internet Development”. Network linking: http://www.cnnic.net.cn/hlwfzyj/hlwxzbg/hlwtjbg/202202/P020220407403488048001.pdf
18According to the question "Which of the above media is your main source of information", there are 6 media, including newspapers, magazines, radio, television, Internet, and cell phone customized messages. If the respondent chooses the Internet, this study assumes that the respondent thinks it is the most important to get information through the Internet and assign it a value of 1, otherwise it will be assigned a value of 0. Because this question is a skip question (if the respondent selects "never" for all six information sources, then this question is skipped), the remaining sample size in the EOP model is 999. Although some households were lost due to constructing the instrument variable, the loss rate was only about 2.35%. And the distribution of the remaining sample on the Internet use frequency is almost the same as the whole sample. Therefore, this approach does not lead to a serious bias in the estimation results.
19Calculate process: 0.0132/-0.2132 = −0.0619, the same below.
20The idea that people assign higher weights to their private information can be understood in the following two aspects. One aspect is when the private information is consistent with the public one, people will make convergent behaviors based on the public one, and it is equivalent to people making decisions based on their private information. The other is when the private information contradicts the public one, people will prefer to consider their private information to make decisions rather than the public one.
References
Ahn, J., and Chae, D. (2019). The influences of socio-individual determinants and health information seeking on health-promoting behaviors among migrant women: A cross-sectional study. Jpn. J. Nurs. Sci. 16 (4), 481–490. doi:10.1111/jjns.12259
Ai, X., Du, Y.-F., Li, W.-M., Li, H., and Liao, H. (2021). The pattern of household energy transition. Energy 234, 121277. doi:10.1016/j.energy.2021.121277
Anderson, L. R., and Holt, C. A. (2008). Chapter 39 information cascade experiments. Handb. Exp. Econ. results 1, 335–343. doi:10.1016/S1574-0722(07)00039-X
Bach, A., Shaffer, G., and Todd, W. (2013). Digital human capital: Developing a framework for understanding the economic impact of digital exclusion in low-income communities. J. Inf. Policy 3 (1), 247–266. doi:10.5325/jinfopoli.3.1.247
Banerjee, A., and Drew, F. (2004). Word-of-mouth learning. Games Econ. Behav. 46 (1), 1–22. doi:10.1016/S0899-8256(03)00048-4
Bikhchandani, S., Hirshleifer, D. A., Welch, I., and Cascades, I. (2008). “The new palgrave dictionary of economics,”. Editors S. N. Durlauf, and L. E. Blume Second Edition (Palgrave Macmillan/U.K.).
Bikhchandani, S., Hirshleifer, D., and Welch, I. (1998). Learning from the behavior of others: Conformity, fads, and informational cascades. J. Econ. Perspect. 12, 151–170. doi:10.1257/jep.12.3.151
Carter, E., Yan, Li, Fu, Yu, Robinson, B., Kelly, F., Elliott, P., et al. (2020). Household transitions to clean energy in a multiprovincial cohort study in China. Nat. Sustain. 3, 42–50. doi:10.1038/s41893-019-0432-x
Chang, H. Y., He, K., and Zhang, J. B. (2020). Energy poverty in rural China: A psychological explanations base on households. China Popul. Resour. Environ. 2, 11–20. (in Chinese).
Chen, H., Zhou, H., and Sun, D. (2017). Impact of information transmission on farmers’ application behavior and rice yield: An empirical analysis of rice farmers in Jiangxi Province” [J]. J. Agrotechnical Econ. (in Chinese) 12, 23–31. doi:10.13246/j.cnki.jae.2017.12.003
Chen, Q., Yang, H., Liu, T., and Zhang, L. (2016). Household biomass energy choice and its policy implications on improving rural livelihoods in Sichuan, China. Energy Policy 93, 291–302. doi:10.1016/j.enpol.2016.03.016
Choo, C., Detlor, B., and Turnbull, D. (2000). Web work: Information seeking and knowledge work on the world wide web. Berlin, Germany: Springer. doi:10.1007/978-94-015-9405-9
Conley, T. G., and Udry, C. R. (2010). Learning about a new technology: Pineapple in Ghana. American economic review 100, 35–69. doi:10.1257/aer.100.1.35
Courtois, P., and Subervie, J. (2015). Farmer bargaining power and market information services. American Journal of agricultural economics 97, 953–977. doi:10.1093/ajae/aau051
Deng, X., Song, Y., He, Q., Xu, D., and Qi, Y. (2022). Does internet use improve farmers’ perception of environmental pollution? Evidence from rural China. Environmental Science and Pollution Research 29, 44832–44844. doi:10.1007/s11356-022-19076-3
Emodi, N., Haruna, E., Husen, N., Aldana, S., Dioha, M., Abraham, D., et al. (2022). Urban and rural household energy transition in Sub-Saharan Africa: Does spatial heterogeneity reveal the direction of the transition? Energy Policy 168, 113118. doi:10.1016/j.enpol.2022.113118
Frydman, C., and Krajbich, I. (2022). Using response times to infer others’ private information: An application to information cascades. Management Science 68 (2022), 2970–2986. doi:10.1287/mnsc.2021.3994
Green, F., and Stern, N. (2016). China’s changing economy: Implications for its carbon dioxide emissions. Climate Policy 17, 423–442. doi:10.1080/14693062.2016.1156515
Guo, H., and Marchand, S. (2019). Social interactions and spillover effects in Chinese family farming. The Journal of Development Studies 55 (11), 2306–2328. doi:10.1080/00220388.2018.1443206
Han, H., Wu, S., and Zhang, Z. (2018). Factors underlying rural household energy transition: A case study of China. Energy Policy 114, 234–244. doi:10.1016/j.enpol.2017.11.052
Han, H., and Wu., S. (2018). Rural residential energy transition and energy consumption intensity in China. Energy Economics 74, 523–534. doi:10.1016/j.eneco.2018.04.033
Hanna, R., and Oliva, P. (2015). Moving up the energy ladder: The effect of an increase in economic well-being on the fuel consumption choices of the poor in India. American Economic Review 105 (5), 242–246. doi:10.1257/aer.p20151097
He, J., Qing, C., Guo, S., Wenfeng, Z., Deng, X., and Xu, D. (2022). Promoting rural households' energy use for cooking: Using Internet. Technological Forecasting and Social Change 184, 121971. doi:10.1016/j.techfore.2022.121971
Hills, O., and Shah, D. (2020). Online health information seeking, medical care beliefs and timeliness of medical check-ups among African Americans. Patient Education and Counseling 103 (12), 2468–2476. doi:10.1016/j.pec.2020.06.006
Hu, Z., Wang, Y., and He, J. J. (2019). Characteristics of urban household energy consumption and carbon emissions in Western China: Evidence from China Family Panel Studies (CFPS). Journal of Arid Land Resources and Environment 4, 1–8. (in Chinese). doi:10.13448/j.cnki.jalre.2019.098
Huber, R. E., Klucharev, V., and Rieskamp, J. (2015). Neural correlates of informational cascades: Brain mechanisms of social influence on belief updating. Social Cognitive and Affective Neuroscience 10 (4), 589–597. doi:10.1093/scan/nsu090
Jalan, J., and Somanathan, E. (2008). The importance of being informed: Experimental evidence on demand for environmental quality. Journal of Development Economics 87 (1), 14–28. doi:10.1016/j.jdeveco.2007.10.002
Jiang, W., Yan, T., and Chen, B. (2021). Impact of media channels and social interactions on the adoption of straw return by Chinese farmers. Science of the Total Environment 756 (2021), 144078. doi:10.1016/j.scitotenv.2020.144078
Karatasou, S., and Santamouris, M. (2019). Socio-economic status and residential energy consumption: A latent variable approach. Energy and Buildings 198, 100–105. doi:10.1016/j.enbuild.2019.06.013
Karekezi, S., and Kithyoma, W. (2002). Renewable energy strategies for rural Africa: Is a PV-led renewable energy strategy the right approach for providing modern energy to the rural poor to sub-Saharan Africa? Energy Policy 30, 1071–1086. doi:10.1016/S0301-4215(02)00059-9
Kawakatsu, H., and Largey, A. (2009). EM algorithms for ordered probit models with endogenous regressors. Econometrics Journal 12, 164–186. doi:10.1111/j.1368-423X.2008.00272.x
Kim, J. H., and Park, E. C. (2015). Impact of socioeconomic status and subjective social class on overall and health-related quality of life. BMC Public Health 15, 783. doi:10.1186/s12889-015-2014-9
Leach, G. (1992). The energy transition. Energy policy 20 (2), 116–123. doi:10.1016/0301-4215(92)90105-B
Lee, L., Frederick, S., and Ariely, D. (2006). Try it, you'll like it: The influence of expectation, consumption, and revelation on preferences for beer. Psychological science 17 (12), 1054–1058. doi:10.1111/j.1467-9280.2006.01829.x
Li, M., Tianyu, J., Liu, S., and Zhou, S. (2022). The cost of clean energy transition in rural China: Evidence based on marginal treatment effects. Energy Economics 97, 105167. doi:10.1016/j.eneco.2021.105167
Li, P., and Li, H. (2020). Neighborhood effect on farmers’ pesticide application rate selection: Based on the analysis of external technology acquisition and empirical capital” [J]. Resources and Environment in the Yangtze Basin (in Chinese) 29 (11), 2508–2518.
Li, Q., Brounen, D., Li, J., and Wei, X. (2022). Social interactions and Chinese households’ participation in the risky financial market. Finance Research Letters 49 (2022), 103142. doi:10.1016/j.frl.2022.103142
Li, T. (2006). Social interaction and investment choice” [J]. Economic Research Journal (in Chinese) 08, 45–57.
Li, X., Zhang, D., Zhang, T., Ji, Q., and Lucey, B. (2021). Awareness, energy consumption and pro-environmental choices of Chinese households. Journal of Cleaner Production 279, 123734. doi:10.1016/j.jclepro.2020.123734
Liang, P., and Guo, S. (2015). Social interaction, internet access and stock market participation—an empirical study in China. Journal of Comparative Economics 43 (4), 883–901. doi:10.1016/j.jce.2015.02.003
Liu, X., and Bae, J. (2018). Urbanization and industrialization impact of CO2 emissions in China. Journal of Cleaner Production 172, 178–186. doi:10.1016/j.jclepro.2017.10.156
Lu, M., and Zhang, S. (2007). Non-market interaction and sorting: A literature review”[J. ].China Economic Quarterly 2007 (03), 991–1020. (in Chinese).
Ma, W., and Wang, X. (2020). Internet use, sustainable agricultural practices and rural incomes: Evidence from China. Australian Journal of Agricultural and Resource Economics 64 (4), 1087–1112. doi:10.1111/1467-8489.12390
Ma, W., Zheng, H., and Gong, B. (2022). Rural income growth, ethnic differences, and household cooking fuel choice: Evidence from China. Energy Economics 107, 105851. doi:10.1016/j.eneco.2022.105851
Ma, W., Zhou, X., and Renwick, A. (2019). Impact of off-farm income on household energy expenditures in China: Implications for rural energy transition. Energy Policy 127, 248–258. doi:10.1016/j.enpol.2018.12.016
Ma, W., and Zhu, Z. (2020). Internet use and willingness to participate in garbage classification: An investigation of Chinese residents. Applied Economics Letters 28, 788–793. doi:10.1080/13504851.2020.1781766
Ma, X. (2022). Internet use and income gaps between rural and urban residents in China. Journal of the Asia Pacific Economy 2022, 1–21. doi:10.1080/13547860.2022.2054133
Maertens, A., and Barrett, C. B. (2013). Measuring social networks' effects on agricultural technology adoption. American Journal of Agricultural Economics 95 (2), 353–359. doi:10.1093/ajae/aas049
Magazzino, C., Mele, M., and Schneider, N. (2021). A machine learning approach on the relationship among solar and wind energy production, coal consumption, GDP, and CO2 emissions. Renewable Energy 167, 99–115. doi:10.1016/j.renene.2020.11.050
Manski, C. F. (2000). Economic analysis of social interactions. Journal of economic perspectives 14 (3), 115–136. doi:10.1257/jep.14.3.115
Marco, M., and Cosimo, M. (2020). A machine learning analysis of the relationship among iron and steel industries, air pollution, and economic growth in China. Journal of Cleaner Production 277, 123293. doi:10.1016/j.jclepro.2020.123293
Masera, O., and Navia-Antezana, J. (1997). Fuel switching or multiple cooking fuels? Understanding inter-fuel substitution patterns in rural Mexican households. Biomass and Bioenergy 12, 347–361. doi:10.1016/S0961-9534(96)00075-X
Mi, Z., Zheng, J., Meng, J., Shan, Y., Zheng, H., Ou, J., et al. (2018). China's energy consumption in the new normal. Earth's Future. 6, 1007–1016. doi:10.1029/2018EF000840
Miah, M. D., Kabir, R., Koike, M., Akther, S., and Shin, M. (2010). Rural household energy consumption pattern in the disregarded villages of Bangladesh. Energy Policy 38, 997–1003. doi:10.1016/j.enpol.2009.10.051
Miklós, Y. P., Allan Sly, Z. R., and Stuhl, I. (2020). How fragile are information cascades? The Annals of Applied Probability 30 (6), 2796–2814. doi:10.1214/20-AAP1573
Poddar, P., Pal, D., and Chatterjee, S. (2021). Moving up the energy ladder: Does socio-religious status matter? Popul Environ 42, 325–359. doi:10.1007/s11111-020-00365-3
Pokharel, S. (2007). An econometric analysis of energy consumption in Nepal. Energy Policy 35, 350–361. doi:10.1016/j.enpol.2005.11.004
Qiu, H., Yan, J., Lei, Z., and Sun, D. (2018). Rising wages and energy consumption transition in rural China. Energy Policy 119, 545–553. doi:10.1016/j.enpol.2018.04.053
Qiu, Y., Yang, F. A., and Lai, W. (2019). The impact of indoor air pollution on health outcomes and cognitive abilities: Empirical evidence from China. Popul Environ 40, 388–410. doi:10.1007/s11111-019-00317-6
Qiu, Z., Huang, S., and Acquaintance Society, (2021). External market and imitation plus innovation in rural E-commerce entrepreneurship [J]. Sociological Studies (in Chinese) 36 (04), 133–229.
Qiu, Z., Li, Y., and Xu, W. (2022). Digitalization and the changing structure of rural government[J]. Journal of Xi'an Jiaotong University (Social Sciences) (in Chinese) 42 (02), 74–84. doi:10.15896/j.xjtuskxb.202202008
Qiu, Z., Zhang, S., and Liu, S. (2016). From digital divide to dividend difference—from the perspective of internet capital[J]. Social Sciences in China (in Chinese) 2016 (10), 93–204.
Rogers, E. M. (1983). Diffusion of innovations. University of Illinois at urbana-champaign's Academy for entrepreneurial leadership historical research reference in entrepreneurship. Available at: https://ssrn.com/abstract=1496176.
Savolainen, R., and Kari, J. (2004). Placing the Internet in information source horizons. A study of information seeking by Internet users in the context of self-development. Library & Information Science Research 26, 415–433. doi:10.1016/j.lisr.2004.04.004
Schöbel, M., Rieskamp, J., and Huber, R. (2016). Social influences in sequential decision making. PloS one 11, e0146536. doi:10.1371/journal.pone.0146536
Shen, K., Lin, J., and Fu, Y. (2023). Network infrastructure construction, information accessibility and the innovation boundaries of enterprises[J]. China Industrial Economics 418 (01), 57–75. doi:10.19581/j.cnki.ciejournal.2023.01.014
Shi, Q. H., Peng, X. H., and Zhang, R. (2014). Field survey of rural energy consumption in China: A case study of 2253 farmers in shanxi, guizhou and zhejiang provinces. Management World 5, 80–92. (in Chinese). doi:10.19744/j.cnki.11-1235/f.2014.05.007
Shi, X. (2019). Inequality of opportunity in energy consumption in China. Energy Policy 124, 371–382. doi:10.1016/j.enpol.2018.09.029
Shi, X., Cheong, T. S., Yu, J., and Liu, X. (2021). Quality of life and relative household energy consumption in China. China & World Economy 29 (5), 127–147. doi:10.1111/cwe.12390
Shi, Y., Qin, G., and Zhao, M. (2022). Impact of neighborhood effect on herders’ stocking rate decision. China population, resource and environment 32 (1), 155–167. (in Chinese).
Shupler, M., Mangeni, J., Tawiah, T., Sang, E., Baame, M., Anderson de Cuevas, R., et al. (2021). Modelling of supply and demand-side determinants of liquefied petroleum gas consumption in peri-urban Cameroon, Ghana and Kenya. Nature Energy 6, 1198–1210. doi:10.1038/s41560-021-00933-3
Song, C., Bilsborrow, R., Jagger, P., Zhang, Qi, Chen, X., and Huang, Q. (2018). Rural household energy use and its determinants in China: How important are influences of payment for ecosystem services vs. Other factors? Ecological Economics 145, 148–159. doi:10.1016/j.ecolecon.2017.08.028
Sun, W., Han, X., Kerong, S., and Fan, J. (2012). Geographical differences and influencing factors of rural energy consumption in southwest mountain areas in China: A case study of zhaotong city. Journal of Mountain Science 9, 842–852. doi:10.1007/s11629-012-2355-0
Tao, S., Ru, M. Y., Du, W., Zhu, X., Zhong, Q. R., Li, B. G., et al. (2018). Quantifying the rural residential energy transition in China from 1992 to 2012 through a representative national survey. Nat Energy 3, 567–573. doi:10.1038/s41560-018-0158-4
Tian, Ge, and Zhang, X. (2022). Digital economy, non-agricultural employment, and division of labor[J]. Journal of Management World 38 (05), 72–84. doi:10.19744/j.cnki.11-1235/f.2022.0069
Udemba, E., Magazzino, C., and Bekun, V. (2020). Modeling the nexus between pollutant emission, energy consumption, foreign direct investment, and economic growth: New insights from China. Environmental Science and Pollution Research 27, 17831–17842. doi:10.1007/s11356-020-08180-x
Vabø, M., and Hansen, H. (2014). The relationship between food preferences and food choice: A theoretical discussion. International Journal of Business and Social Science 5 (7), 145–157. Corpus ID: 32700664.
Van der Kroon, B., Brouwer, R., and Beukering, P. Jh V. (2013). The energy ladder: Theoretical myth or empirical truth? Results from a meta-analysis. Renewable and sustainable energy reviews 20, 504–513. doi:10.1016/j.rser.2012.11.045
Vatsa, P., Li, J., Luu, P., and Botero, R. J. (2022). Internet use and consumption diversity: Evidence from rural China. Review of Development Economics 2022, 12935. doi:10.1111/rode.12935
Wang, F. (2021). Social capital or non-human sources? A cross-context study on information source selection of migrant farmer workers. Journal of Information Science 2021, 016555152110551. doi:10.1177/01655515211055129
Wang, S., Sun, S., Zhao, E., and Wang, S. (2021). Urban and rural differences with regional assessment of household energy consumption in China. Energy 232, 121091. doi:10.1016/j.energy.2021.121091
Wang, X., Kunquan, L., Hua, L., Di, B., and Jingru, L. (2017). Research on China’s rural household energy consumption–Household investigation of typical counties in 8 economic zones. Renewable and Sustainable Energy Reviews 68, 28–32. doi:10.1016/j.rser.2016.10.004
Wang, Z., Chen, C., Guo, B., Yu, Z., and Zhou, X. (2016). Internet plus in China. IT Professional 18, 47. doi:10.1109/MITP.2016.47
Wenfeng, Z., Qing, C., Guo, S., He, J., Deng, X., Song, J., et al. (2022). Labor off-farm employment and farmers’ cooking clean energy use: Evidence from rural China. Agriculture 12, 972. doi:10.3390/agriculture12070972
Wu, S., and Zheng, X. (2022). Revisit of household energy ladder –empirical evidence from a household survey in rural China” [J]. China Economic Quarterly (in Chinese) 22 (01), 45–66. doi:10.13821/j.cnki.ceq.2022.01.03
Wu, S., Zheng, X., You, C., and Wei, C. (2019). Household energy consumption in rural China: Historical development, present pattern and policy implication. Journal of Cleaner Production 211, 981–991. doi:10.1016/j.jclepro.2018.11.265
Wu, S., and Han, H. (2022). Energy transition, intensity growth, and policy evolution: Evidence from rural China. Energy Economics 105 (2022), 105746. doi:10.1016/j.eneco.2021.105746
Wu, S., Zheng, X., and Wei, C. (2017). Measurement of inequality using household energy consumption data in rural China. Nat Energy 2, 795–803. doi:10.1038/s41560-017-0003-1
Xiao, Yu, Qi, Z., and Xu, S. (2022). Social interaction and information acquisition ability on farmers’ adoption of rice-shrimp Co-cultivation technology” [J]. Journal of Ecology and Rural Environment (in Chinese) 38 (03), 308–318. doi:10.19741/j.issn.1673-4831.2021.0142
Xie, L., Hu, X., Zhang, X., and Zhang, X.-B. (2022). Who suffers from energy poverty in household energy transition? Evidence from clean heating program in rural China. Energy Economics 106, 105795. doi:10.1016/j.eneco.2021.105795
Yadav, P., Davies, P. J., and Asumadu-Sarkodie, S. (2021). Fuel choice and tradition: Why fuel stacking and the energy ladder are out of step? Solar Energy 214 (2021), 491–501. doi:10.1016/j.solener.2020.11.077
Yang, L., Lu, H., Wang, S., and Li, M. (2021). Mobile internet use and multidimensional poverty: Evidence from A household survey in rural China. Social Indicators Research 158, 1065–1086. doi:10.1007/s11205-021-02736-1
Yang, L., and Wan, J. (2022). ‘CHEAP high-quality consumption’: Household endowment and proximity effect on farmers’ clean energy consumption” [J]. Journal of Huazhong Agricultural University (Social Sciences Edition) 02, 131–145. (in Chinese). doi:10.13300/j.cnki.hnwkxb.2022.02.013
Yuan, F., Tang, K., and Shi, Q. (2021). Does internet use reduce chemical fertilizer use? Evidence from rural households in China. Environ Sci Pollut Res 28, 6005–6017. doi:10.1007/s11356-020-10944-4
Zhang, J., Cheng, M., Mei, R., and Wang, F. (2019). Internet use and individuals' environmental quality evaluation: Evidence from China. Science of The Total Environment 710, 136290. doi:10.1016/j.scitotenv.2019.136290
Zhang, S., and Qiu, Z. (2022). What makes rural E-commerce successful? An analytical framework for the realization of technology dividends” [J]. Sociological Studies 37 (02), 114–229. (in Chinese).
Zhang, X., Niu, S. W., and Zhao, C. S. (2011). Extraction and identification of isothiocyanates from broccolini seeds. China Soft Science 9, 65–66. (in Chinese).
Zhao, Q., Pan, Y., and Xia, X. (2021). Internet can do help in the reduction of pesticide use by farmers: Evidence from rural China. Environ Sci Pollut Res 28, 2063–2073. doi:10.1007/s11356-020-10576-8
Zheng, H., Ma, W., Li, J., and Botero, J. (2023). Relationship between internet use and negative affect. Applied Research in Quality of Life 2023, 10158. doi:10.1007/s11482-023-10158-z
Zheng, X., Wei, C., Song, F., Xie, L., Qin, P., Ma, B., et al. (2016). Chinese residential energy consumption report 2015”[M]. Beijing: Science Press. (in Chinese). ISBN: 978-7-03-047473-5.
Zheng, X., Wei, C., Qin, P., Guo, J., Yu, Y., Song, F., et al. (2014). Characteristics of residential energy consumption in China: Findings from a household survey. Energy Policy 75, 126–135. doi:10.1016/j.enpol.2014.07.016
Zhu, Q., Zhu, C., and Bai, J. (2022). Can informatization boost farmers’ income and narrow the income disparity in rural China? [J]. China Economic Quarterly 22 (01), 237–256. (in Chinese). doi:10.13821/j.cnki.ceq.2022.01.12
Zhu, Xi, Yun, X., Meng, W., Xu, H., Du, W., Shen, G., et al. (2018). Stacked use and transition trends of rural household energy in mainland China. Environmental science & technology 53 (1), 521–529. doi:10.1021/acs.est.8b04280
Ziegelmeyer, A., Koessler, F., Bracht, J., and Winter, E. (2010). Fragility of information cascades: An experimental study using elicited beliefs. Exp Econ 13, 121–145. doi:10.1007/s10683-009-9232-x
Zimmer, J. C., and Henry, R. M. (2017). The role of social capital in selecting interpersonal information sources. Journal of the Association for Information Science and Technology 68 (1), 5–21. doi:10.1002/asi.23577
Zou, B., and Luo, B. (2019). Rural household energy consumption characteristics and determinants in China. Energy 182, 814–823. doi:10.1016/j.energy.2019.06.048
Keywords: information acquisition mechanism, rural household energy transition, energy consumption, the ordered probit model, social interaction, internet use
Citation: Gao J, Zhou J and Liu Y (2023) The impact of internet use on rural household energy transition: moderating effect based on social interaction. Front. Energy Res. 11:1078692. doi: 10.3389/fenrg.2023.1078692
Received: 24 October 2022; Accepted: 04 May 2023;
Published: 22 May 2023.
Edited by:
Shigeyuki Hamori, Kobe University, JapanReviewed by:
Cosimo Magazzino, Roma Tre University, ItalyPeng Jiquan, Jiangxi University of Finance and Economics, China
Simona Andreea Apostu, Bucharest Academy of Economic Studies, Romania
Copyright © 2023 Gao, Zhou and Liu. This is an open-access article distributed under the terms of the Creative Commons Attribution License (CC BY). The use, distribution or reproduction in other forums is permitted, provided the original author(s) and the copyright owner(s) are credited and that the original publication in this journal is cited, in accordance with accepted academic practice. No use, distribution or reproduction is permitted which does not comply with these terms.
*Correspondence: Yunru Liu, 992050045@qq.com