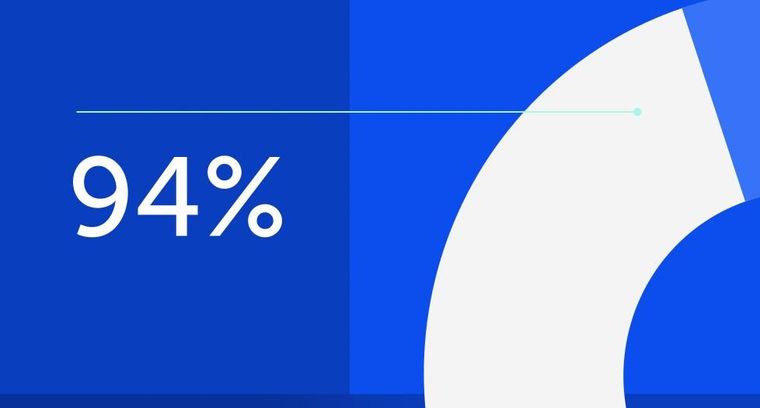
94% of researchers rate our articles as excellent or good
Learn more about the work of our research integrity team to safeguard the quality of each article we publish.
Find out more
MINI REVIEW article
Front. Energy Res., 27 January 2023
Sec. Smart Grids
Volume 11 - 2023 | https://doi.org/10.3389/fenrg.2023.1071291
This article is part of the Research TopicExplainability in Knowledge-based Systems and Machine Learning Models for Smart GridsView all 6 articles
Traditional electrical power grids have long suffered from operational unreliability, instability, inflexibility, and inefficiency. Smart grids (or smart energy systems) continue to transform the energy sector with emerging technologies, renewable energy sources, and other trends. Artificial intelligence (AI) is being applied to smart energy systems to process massive and complex data in this sector and make smart and timely decisions. However, the lack of explainability and governability of AI is a major concern for stakeholders hindering a fast uptake of AI in the energy sector. This paper provides a review of AI explainability and governance in smart energy systems. We collect 3,568 relevant papers from the Scopus database, automatically discover 15 parameters or themes for AI governance in energy and elaborate the research landscape by reviewing over 150 papers and providing temporal progressions of the research. The methodology for discovering parameters or themes is based on “deep journalism,” our data-driven deep learning-based big data analytics approach to automatically discover and analyse cross-sectional multi-perspective information to enable better decision-making and develop better instruments for governance. The findings show that research on AI explainability in energy systems is segmented and narrowly focussed on a few AI traits and energy system problems. This paper deepens our knowledge of AI governance in energy and is expected to help governments, industry, academics, energy prosumers, and other stakeholders to understand the landscape of AI in the energy sector, leading to better design, operations, utilisation, and risk management of energy systems.
Energy has fundamentally shaped the geopolitics of our world and transformed our lives in the last century (Vakulchuk et al., 2020; Blondeel et al., 2021). A look at the global past and current conflicts reveal that energy has been central to many of them involving oil, natural gas, battery minerals, among others. Energy availability enabled modern technological advancements including the ubiquity of computing and power (e.g., batteries), and transformed us into smart societies.
Traditional electrical power grids have long suffered from operational unreliability, instability, inflexibility, and inefficiency. Since power systems traditionally comprised large regional and national grids, monitoring the electrical systems of those grids and long distribution lines has been challenging causing many major electrical system failures, human lives, and hefty economic losses.
Smart grids continue to transform the energy sector with emerging technologies, renewable energy sources, decentralisation, decarbonisation, and others. We hereon will use the term “smart energy systems” for “smart grid” as a broader term that incorporates smart grids, electrical power systems, and related business and other developments. These advancements offer many exciting opportunities such as the availability of solar, wind, hydro, and other forms of energy to organisations and homes. Development of microgrids (Hussain et al., 2019), mini-grids (Gill-Wiehl et al., 2022), community grids (Ceglia et al., 2020; Kong and Song, 2020), and supergrids (Zarazua de Rubens and Noel, 2019) have paved the way, alongside many other possibilities, for energy independence and energy trading between individuals, corporations, and nations. These smart energy systems are complex and produce massive data.
Artificial Intelligence (AI) presents an unimaginable potential for innovation, process optimisation, productivity, and other benefits in many sectors such as smart societies (Janbi et al., 2022), healthcare (Alahmari et al., 2022), education (Mehmood et al., 2017), and transportation (Alomari et al., 2021). The energy sector is not an exception (Alkhayat et al., 2022). AI is being applied to smart energy systems to process massive and complex data in the energy sector and make smart and timely decisions. AI algorithms are black-box (Castelvecchi, 2016) needing interpretability and explainability (Doran et al., 2017; Goebel et al., 2018; Hagras, 2018; Hoffman et al., 2018; Lundberg et al., 2020) so that the decision made by AI could be explained to various stakeholders such as for regulatory and legal reasons. AI algorithms are usually imperfect or inaccurate. These AI algorithms are developed by human designers and developers trained using imperfect data and therefore they are likely to inherit bias and prejudice from them. The unregulated developments of AI have focussed on maximising efficiencies, and economic and other objectives rather than human values and priorities.
We adopt in this paper the definition of explainable AI (XAI) by NIST (National Institute of Standards and Technology) (Phillips et al., 2021) that proposes XAI systems to observe four principles, namely i) Explanation [“a system delivers or contains accompanying evidence or reason (s) for outputs and/or processes”]; ii) Meaningful [“a system provides explanations that are understandable to the intended consumer (s)”]; iii) Explanation Accuracy (“an explanation correctly reflects the reason for generating the output and/or accurately reflects the system’s process”); and iv) Knowledge Limits (“a system only operates under conditions for which it was designed and when it reaches sufficient confidence in its output”). Explainability and interpretability are among many desirable characteristics to support trustworthiness in AI systems including, among others, “accuracy, privacy, reliability, robustness, safety, security (resilience), mitigation of harmful bias, transparency, fairness, and accountability” (Phillips et al., 2021). Responsibility could be another characteristic for AI trustworthiness (Yigitcanlar et al., 2021).
The lack of explainability and governability of AI had affected stakeholders’ confidence in AI systems and consequently, the uptake of AI in the energy sector has been slow. Moreover, the complexity of the design and operations space of energy systems that involves many parameters and stakeholders is on the rise and the consequent severity of risks is catastrophic due to the social, national, environmental, and geopolitical criticality of these matters.
This paper provides a review of AI explainability and governance in smart energy systems. We collected 3,568 relevant papers from the Scopus database using a specific query (see Section 2), automatically discovered 15 parameters for AI governance in smart energy systems, and group them into four macro-parameters, namely AI Behaviour and Governance, Technology, Design and Development, and Operations. We elaborate on the research landscape by reviewing over 150 papers and providing temporal progressions of the research. The methodology for discovering parameters or themes is based on “deep journalism,” our data-driven deep learning (DL)-based big data analytics approach to automatically discover and analyse cross-sectional multi-perspective information to enable better decision-making and develop better instruments for governance. We introduced the deep journalism approach (Ahmad et al., 2022) and applied it to different sectors (Alqahtani et al., 2022; Alswedani et al., 2022; Mehmood, 2022).
The findings of this paper show that research on AI explainability in energy systems is segmented and narrowly focussed on a few AI traits (fairness, interpretability, explainability, trustworthiness) and energy system problems (stability and reliability analysis, energy forecasting, power system flexibility). The paper deepens our knowledge of AI governance in energy and is expected to help governments, industry, academics, energy prosumers, and other stakeholders to understand the landscape of AI in the energy sector, leading to better design, operations, utilisation, and risk management of energy systems.
To the best of our knowledge, this is the first comprehensive review of AI governance in the energy sector. It is a novel work due to its scope, methodology, and findings. There are several literature reviews on smart grids but they are not aimed at AI explainability or governance. We have found only two works that can be considered related to our work. A review of AI interpretability in smart grids is presented by (Xu et al., 2022) using papers collected from Google Scholar over a 5-year period. A review of AI explainability research in energy and power systems is provided by (Machlev et al., 2022) using literature from 2019 to 2022. Firstly, none of these works have used BERT (bidirectional encoder representations from transformers) to automatically discover parameters. Secondly, they do not have similar scope to ours (search query and data collection), i.e., they do not consider AI explainability and governance in its broader sense incorporating AI behaviour (governance, explainability, interpretability, responsibility, ethics, trustworthiness, and fairness) as extensively as we do (see Section 2). Thirdly, our deep journalism methodology allows us to use AI to collect a comprehensive selection of papers (a dataset) and provide a summary of a 55-year period of research on AI in energy systems.
The rest of the paper is organised as follows. Section 2 briefly describes the methodology of this work. Section 3 discusses the parameters and reviews the literature. Section 4 provides a discussion and concludes the paper.
We briefly describe the methodology and software tool design for automatic parameter discovery here. The word limit limits us, hence the brevity, for details, see (Ahmad et al., 2022; Alqahtani et al., 2022).
We collected the data for this work from the Scopus database using the following keywords in the query: artificial intelligence, machine learning, deep learning, grid, electricity, energy, power system, governance, explainability, interpretability, responsibility, ethics, trustworthiness, and fairness. This generated 3,568 paper abstracts published between 1967 and 2022 from various disciplines. No limits on disciplines or years were applied in collecting data. Duplicates, stop words, and irrelevant and noisy data were removed using pandas and NumPy. BERT, UMAP (uniform manifold approximation and projection), HDBSCAN (hierarchical density-based spatial clustering of applications with Noise), and class-based TF–IDF (term frequency-inverse document frequency) score were used to capture contextual relations, reduce the number of clusters, and cluster data (Grootendorst, 2021; Ahmad et al., 2022; Alqahtani et al., 2022). Finally, we used domain knowledge and a range of analysis and visualisation techniques (hierarchical clustering, topic word score, similarity matrix, term score decline) to discover parameters for AI governance in energy.
Table 1 lists the names of the 15 discovered parameters in Column 1 sorted by the four macro-parameters, AI Behaviour and Governance, Technology, Design and Development, and Operations. These macro-parameters will be discussed in Sections 3.2–3.5. The table provides one example research work for each parameter (Column 2) along with its research dimension (Column 3), the AI behaviour addressed by each work (Column 4) and the summary of the work (Column 5). The papers selected in the table were chosen to showcase a variety of perspectives and AI behaviours. The intention was not to rank them as the “best” works and they should not be viewed as such. Section 3.6 provides the taxonomy and temporal progression of the parameters.
This parameter is about the governance and management of AI in the energy sector by identifying the requirements to build ethical, responsible, trustworthy applications and to discuss its policies, regulations, and data privacy concerns. It captures various dimensions of AI behaviour and governance including AI responsibility and accountability in smart grids (Volkova et al., 2022), AI governance and regulations in power-related general-purpose technologies (Nitzberg and Zysman, 2022), reviewing the European law for the governance of AI in the electricity sector in order to allow transparent and responsible grid management (Niet et al., 2021), promoting fairness and consumer protection via the use of automated decision-making to get access to fundamental services such as electricity and telecommunications (Przhedetsky, 2021), ethics of AI and power systems.
This parameter is about the use of the Internet of Things (IoT) and edge computing for energy systems’ monitoring and efficient governance. It captures various dimensions of “IoT and Edge,” including detecting power consumption attacks for promoting vehicular edge devices reliability and AI chips’ trustworthiness (Zhu et al., 2022), enabling sustainable energy and ethical stable power applications by self-powered, learning sensor systems (Alagumalai et al., 2022), and improving the data exchange for mobile sensors to increase the energy efficiency and trustworthiness of the IoT network (Haseeb et al., 2022). Additional dimensions include efficiency in energy use via trustworthy intelligent IoT environments (Soret et al., 2022), cloud computing to monitor Wireless Sensor Network (WSNs), cloud and edge computing in energy systems applications, IoT devices in the power network, edge-cloud computing in energy monitoring, and edge computing for IoT energy systems.
This parameter involves the design of fair and trustworthy solutions to support the management and resource allocation in smart energy systems using Unmanned Aerial Vehicles (UAVs). It captures different dimensions of unmanned aerial vehicles, including distributed control of UAVs for enhancing the degree of coverage with limited and fair consumption of energy (Nemer et al., 2022), a fair design for multi-UAV pathway (Zhang et al., 2022), UAVs trajectory design and time allocation for fair communication in wireless NOMA-IoT networks (Zhang et al., 2022), and fair wireless communication in UAV base stations (Qin et al., 2021). Other dimensions include a fairness methodology to federated learning in vehicular edge computing (Xiao et al., 2022), resource allocation in 5G Integrated Backhaul and Access (IAB) networks to increase the trustworthiness of access links (Huang et al., 2022), and allocating resources across multiple UAVs in the IoT networks using a DL approach (Seid et al., 2021).
This parameter is about improving the performance of AI applications for smart grids by integrating them with IoT and Blockchain technologies to obtain reliable and fair solutions. It captures various dimensions of “Blockchain,” including the management of electricity demand in smart grids using blockchain as a trustworthy platform (Jose et al., 2022), accountability and fairness in the energy environment using blockchain (Baashar et al., 2021), and using trustworthy blockchain-based federated learning to fight malicious devices with significant efficacy and low energy demand (Yang et al., 2022). Further dimensions include reliable, fair, and secure solutions for energy applications using blockchain (Al-Abri et al., 2022), providing data privacy and fairness via the use of blockchain and AI-powered IoT for energy management and power trading (Lin et al., 2022), a blockchain-based framework for privacy and security in energy networks, and attack detection.
This parameter is about adopting trustworthy systems to enhance the energy efficiency, lifetime, and performance of sensor networks (SNs) and WSNs for smart grids. It captures various dimensions of “Senser Networks,” including task off-loading at smart grids’ edge for trustworthiness (Gunaratne et al., 2022), enhancing the effectiveness of IoT-WSN by using the Secure Energy-Aware Meta-Heuristic Routing (SEAMHR) protocol (Gurram et al., 2022), and reliable and trustworthy DL method to detect black-hole attacks in wireless sensors and maximize energy efficiency (Kolangiappan and Kumar, 2022). Further dimensions include temperature-aware trustworthy routing in SNs to optimize energy efficiency (Khan et al., 2022) and DL techniques to improve energy usage fairness across the cluster members in Cognitive Radio Sensor Networks (CRSN) (Stephan et al., 2021).
This parameter involves adopting machine learning (ML) interpretable methodologies for analyzing characteristics of chemical materials, energy systems, and batteries. It captures various dimensions of “Materials for Energy Storage and Systems,” including using interpretable machine learning (IML) for estimating decomposition enthalpy that measures the durability of Chevrel phases for batteries (Singstock et al., 2021), forecasting material characteristics using IML models to provide transparency (Allen and Tkatchenko, 2022), and trustworthy approach using DL for data evaluation of battery energy storage systems (Lee et al., 2020). Additional dimensions comprise modeling and explainability of the formation energy of inorganic chemicals using DL (Huang and Ling, 2020) and developing a predictive model using IML to predict the Fermi energy level needed to build electrically conductive materials, heterostructures, and devices (Motevalli et al., 2022).
This parameter is about developing explainable and reliable ML models and Deep Neural Networks (DNNs) in energy systems physics. It captures various dimensions of the “Physics of Energy System,” involving developing reusable and fair intelligent systems in high-energy particle physics (Chen et al., 2021), IML methodology to improve propulsion and power systems (Longmire and Banuti, 2022), classifying and interpreting the wasted energy of high-energy jets (Du et al., 2021), ML approach in Kondo physics to optimize explainability (Miles et al., 2021), and the reliability of semiconductors (Amrouch et al., 2021).
This parameter is about investigating AI governance’s role in promoting sustainable energy and sustainable development without putting essential energy requirements at risk and considering strategies to fight climate change. It captures different dimensions of “Sustainable Energy and Climate,” including AI-powered solutions to achieve sustainable energy (Saheb et al., 2022), the aspects of water governance in urban areas (Goulas et al., 2022), the significance of AI in promoting sustainable development in the water, food, and energy industries (D’amore et al., 2022), sustainable education and society in the energy domain (Skowronek et al., 2022), and the governance of AI to confront climate change and achieve sustainable development (Raper et al., 2022).
This parameter is about detecting and governing the power demand level in energy markets. It captures various dimensions of “Energy Markets and Management,” including using the IML method for the management of decentralized optimal power flow (Serna Torre and Hidalgo-Gonzalez, 2022), designing an interpretable Deep reinforcement learning (DRL) approach for transmission network expansion in wind power (Wang Y et al., 2021), and power distribution systems’ reliability, interpretability, and security (Gao and Yu, 2021). Further dimensions include optimal multi-agent energy management for interconnected energy systems in the context of a co-trading market to promote fair commerce and to maintain the privacy of entities (Sun et al., 2022), management of energy pipeline infrastructure (Belinsky and Afanasev, 2021), and applying IML and collaborative game theory for market regression analysis and its use in energy forecasting (Pinson et al., 2021).
This parameter is about data analysis to predict energy consumption and the costs for its associated services. It captures different dimensions of “Energy Demand Forecasting,” including the application of XAI in the assessment of power grid control (Kruse et al., 2022), employing Recurrent Neural Network (RNN) explainable method to predict short-term electric demands (Gürses-Tran et al., 2022), and interpretability for forecasting of probabilistic load in power network (Arora et al., 2022). Moreover, using a two-stage interpretable model for short-term energy load prediction in power management (Xie et al., 2021), improves the effectiveness of energy resource management and increases the accuracy of forecasting power consumption over the short term with IML models (Sujan Reddy et al., 2022). Additional dimensions include a multi-step interpretable probabilistic model for predicting residential energy consumption (Xu et al., 2022), short-term energy forecasting, energy consumption forecasting, load forecasting, demand forecasting, and various machine learning models for energy forecasting.
This parameter is about solar energy forecasting to enhance the management of power generation and propose trustworthy and explainable approaches. It captures various dimensions of “Solar Energy Systems,” including the interpretability of solar energy forecasting (Liu et al., 2022), the prediction of photovoltaic (PV) power generation that includes interpretable temporal dynamics (López Santos et al., 2022), and interpretable and trustworthy approach for global solar radiation forecasting (del Campo-Ávila et al., 2021), predicted energy output and carbon dioxide (CO2) emissions (Bouziane and Khadir, 2022), a hybrid approach involving ML and IoT for solar radiation prediction (Ghosh et al., 2020), and irradiance in solar energy, power generation by solar energy.
This parameter is about detecting, monitoring, and classifying faults and security threats in smart energy systems using transparent and knowledge-based methods. It captures various dimensions of “Anomaly Detection and Security,” including applying XAI methods to identify conductive galloping in power grids (Sun et al., 2021), achieve transparency of fault diagnosis in electrical grids (Ardito et al., 2022), and monitoring data poisoning attacks in smart grids using white-box and black-box analysis (Bhattacharjee et al., 2022). Other dimensions include identifying erroneous measurements in the smart grid measuring system using trustworthy data sources (Badr et al., 2022), improving distributed denial-of-service (DDoS) security of software defined networks (SDN)-based smart grids to increase security and trustworthiness (Nagaraj et al., 2021), fault detection (Landwehr et al., 2022), anomaly classification for power consumption data in smart grid (Bhattacharjee et al., 2021), anomaly attacks detection in power networks, and ML and DL models for fault detection in power systems.
This parameter is related to adopting reliable AI models for effective management and accurate building energy consumption forecast. It captures different dimensions of “Energy-Efficient Buildings,” including the generalizability of IML for estimating building energy consumption and making buildings more energy efficient (Manfren et al., 2022), classification of building energy performance certificates using XAI (Tsoka et al., 2022), and XAI approach for forecasting long-term building energy consumption (Wenninger et al., 2022). Further dimensions include providing smart recommendations based on XAI for evaluating building energy efficiency systems (Himeur et al., 2022), improving the effective use of energy by adopting an IML model to forecast room occupancy (Abdel-Razek et al., 2022), predicting energy consumption in buildings, and machine learning-based models for energy efficiency in buildings.
This parameter is about improving energy system operations via reliable assessment models and enhancing transparency in decision-making to assure supply security and system integrity. It captures dimensions of “Grid Reliability and Stability Management,” including investigating the hazards of operating a state power grid to support responsible decision-making and management (Zhang et al., 2023) and applying ML to improve the performance of nuclear power plants. Other dimensions include discussing the ability to apply interpretable solutions (Volodin and Tolokonskij, 2022) and designing a data-integration model to forecast the frequency response of a power system to help enhance the interpretability of the outcomes (Wang X et al., 2021). Moreover, reviewing the future of AI applications in the power system to support interpretability and stability (Zhao et al., 2021), improve the reliability of smart grid’s short-term voltage stability evaluation to avoid power interruptions (Luo et al., 2021), power system stability, and accuracy of ML methods for the assessment of power system, and evaluating the performance of ML methods for fault selection in power lines depending on the accuracy and explainability (Gutierrez-Rojas et al., 2022).
This parameter involves using AI and IoT technologies to enhance the governance of smart cities systems and applications for energy. It captures various dimensions of “Smart City Energy Systems,” including the usage of edge AI and blockchain to manage vehicle surveillance and traffic congestion through trustworthy automobile communications and smart energy trading (Bracco et al., 2022), AI technologies in smart city governance to boost the innovative value and measurable efficiency of smart grids, electric cars, and smart buildings (Zamponi and Barbierato, 2022), and examine the most recent strategies for integrating AI and Analytics (AIA) into smart grid developments in order to enhance energy governance (Khosrojerdi et al., 2022). Additional dimensions include AI-based fairness methods for transportation localization utilizing sustainable standards (Kleisarchaki et al., 2022), smart city governance and planning using AI-based applications, such as smart transportation, smart education, and smart grid (Ashwini et al., 2022), applying DL to smart city environments and power forecasting (Naoui et al., 2021), IoT applications in smart cities such as smart transportation, smart energy, and smart governance (Ilyas, 2021), and deployment of smart energy meters for smart homes using AI-interpretable cloud analytics (Chen et al., 2019).
Figure 1 depicts the taxonomy of AI governance of energy systems (the top) and the temporal progression of all the 15 parameters grouped into four macro-parameters. The overall intensity of research in each macro-parameter can be seen by integrating the research of its parameters.
FIGURE 1. (A) taxonomy of parameters; temporal progression for: (B) ai behaviour and governance (C) technology (D) design and development (E) operations.
Finally, we provide here an overview of research on AI and energy systems carried out between 1967 and 2022 without regard to the discovered parameters. Our methodology has afforded us a means for developing a comprehensive dataset and comprehension of research on AI in energy systems.
1967–1989: There are a total of 31 works in this period. These include AI, ethics, and human responsibility (Boden, 1987) and expert systems for reliable electric power (Siddiqi and Lubkeman, 1988). Some of the collected works are not related to energy or AI and fell under the search due to key terms such as power, responsibility, and reliability, for instance, responsible energy-rich biological compounds (Hager, 1967), ethical responsibility of automation (George, 1976), responsible decision-making in mental health organizations (Kochen, 1975), responsible AI in military applications (Beusmans and Wieckert, 1989), conserving power in robots (Selfridge and Franklin, 1990), and responsible utilization of AI (Stamper, 1988).
1990–1999: There are a total of 47 works in this period. These include the impact of AI on power plant reliability (Christie, 1990), power management and distribution for automation (Ashworth, 1990), nuclear power plant (Trovato et al., 1990), explainability of electric circuits (Kashihara et al., 1992), AI in power systems management (Janik and Gholdston, 1992; Hasan et al., 1994; Irisarri, 1996), short-term electric load forecasting (Stroemich and Thomas, 1997), reliability of nuclear weapons stockpile protection measures (Molley, 1996), AI in fault diagnosis systems for power plants (Kiupel et al., 1995), electricity transportation management (Jennings, 1995), decision tree interpretability to manage electric power utilities (Wehenkel et al., 1994), explainability for the evaluation of power system security (Boyen and Wehenkel, 1999), and trustworthy intelligent agents to power markets (Krishna and Ramesh, 1998).
2000–2009: There are a total of 168 works in this period. These include electrical stimulation systems (Fisekovic and Popovic, 2001), ethical implications and responsibility of AI (Perri, 2001), improving quality of electricity (The engagements of the management of the French transmission system [RTE, R seau de Transport Fran ais] in the matter of quality in providing electricity, no date), fair allocation of neural networks (Fidalgo et al., 2007), automatic diagnosis system for power transformers using artificial neural networks (Moreira et al., 2007), engineering ethics (Berne, 2001), and renewable energy applications (Belu, 2009).
2010–2019: There are a total of 1,142 works in this period. These include energy consumption in wireless sensor networks (Ebadi et al., 2010), reliability and efficiency of smart grids (Rosic et al., 2013), simulation of electric power markets (Vale et al., 2011), social responsibility for low energy consumption and public building energy management (Egging, 2013), smart grid IT governance (Parra et al., 2014), energy markets agent-based modelling (Lupo and Kiprakis, 2015), cybersecurity for smart grids (Yardley et al., 2015), solar power prediction (Cabrera et al., 2016), pricing systems in smart grids (Bandyopadhyay et al., 2016), smart grid congestion management (MacDougall et al., 2017), electricity theft in smart grids (Yeckle and Tang, 2018), and load scheduling in smart grids (Senevirathne et al., 2019).
2020–2022: There are a total of 2,180 works during this less than a 3-year period. We can say that the last 3 years have seen a surge in AI explainability research in energy systems. The works in this period are based on the latest trends in ML and DL, explainability and interpretability, and smart approaches toward multi-source energy systems.
This paper provided a review of AI explainability and governance in smart energy systems. We automatically discovered 15 parameters and elaborated the research landscape by reviewing over 150 papers. The parameters were grouped into four macro-parameters, namely AI Behaviour and Governance, Technology, Design and Development, and Operations.
Our work supports and extends the existing literature, particularly (Machlev et al., 2022; Xu et al., 2022), that identified stability, reliability, energy forecasting, and power system flexibility as major activities in the field. This work has provided an extensive view of AI governance in energy systems and thereby has broadened and deepened the understanding of the field.
The work has identified a range of specific and broad challenges including resource allocation in wireless sensor networks with multiple UAVs (Seid et al., 2021; Zhang et al., 2022), governance of AI in power-related general-purpose technologies (Niet et al., 2021; Przhedetsky, 2021; Nitzberg and Zysman, 2022), fault detection, fault diagnosis, and anomaly detection in smart energy systems (Sun et al., 2021; Badr et al., 2022), edge computing for detecting power demand attacks (Alagumalai et al., 2022; Haseeb et al., 2022; Zhu et al., 2022), blockchain-based reliability and security (Al-Abri et al., 2022; Jose et al., 2022), governance of energy markets and energy pipeline systems (Belinsky and Afanasev, 2021; Serna Torre and Hidalgo-Gonzalez, 2022; Sun et al., 2022), forecasting short-term energy demand (Xie et al., 2021; Gürses-Tran et al., 2022), energy trading using federated learning in smart cities (Bracco et al., 2022), energy-saving edge AI applications (Khosrojerdi et al., 2022), performance optimization and stability of smart grid operations and nuclear power systems (Luo et al., 2021; Volodin and Tolokonskij, 2022), and others. All these areas are candidates for future research.
We have listed above a range of challenges related to XAI in energy systems. It is apparent that both XAI and energy systems are rich and complex fields. A proper discussion of specific limitations and challenges on the subject requires several pages. Due to the lack of space, we briefly mention below a few examples of specific challenges in XAI for energy systems. Fault detection, diagnosis, and prediction are among the most important challenges in energy systems. These are problematic due to the complexity of energy systems covering large and uninhabited geographic regions involving difficult terrains. Specific fault detection-related challenges include the management and storage issues arising due to the use of multiple data sources (solar or wind power forecasting and related faults using numerical and image data), as opposed to a single data source, for fault detection (Landwehr et al., 2022), the influence of measurement noise on fault prediction performance (Sun et al., 2021), privacy issues in fault-diagnosis and examination of security and stability-sensitive scenarios (Ardito et al., 2022), and low accuracies of AI algorithms in fault detection, diagnosis, and prediction (Wu et al., 2022). Another increasingly important area is the security of ML and DL software (Altoub et al., 2022). The challenges in this area include, among others, data poisoning attacks and the performance of related solutions (Bhattacharjee et al., 2022), and anomaly detection methods for smart grid meter security against poisoning attacks (Bhattacharjee et al., 2021).
The parameters discovery shows that most of the research is focussed on Operations followed by research activities in Design and Technology. The least research is in AI Behaviour and Governance where much effort is needed in the future. The methods and tools to support trustworthiness (explainability and other AI traits) in AI for energy systems include, among others, visual explanation techniques using Gradient-weighted Class Activation Mapping (Grad-CAM) (Ardito et al., 2022), sequence-to-sequence RNN methods for visual explanation of short-term load forecasting (Gürses-Tran et al., 2022), the Scale-Invariant Feature Transform (SIFT) method (Singstock et al., 2021), post hoc interpretability (Allen and Tkatchenko, 2022), SHapley Additive exPlanation (SHAP) (Pinson et al., 2021; Abdel-Razek et al., 2022; Kruse et al., 2022), interpretable Tiny Neural Networks (TNN) (Longmire and Banuti, 2022), model-agnostic methods (Gürses-Tran et al., 2022), the use of Temporal Fusion Transformer (TFT) method to enhance interpretability (López Santos et al., 2022), the decision tree and Classification and Regression Tree (CART) algorithms for ML explainability (Sun et al., 2021), visual data exploration for the interpretability of fault diagnosis (Landwehr et al., 2022), a partially interpretable method using Long short-term memory (LSTM) and MLP (multilayer perceptron) for short-term load forecasting (Xie et al., 2021), and Local Interpretable Model-Agnostic Explanation (lime) (Tsoka et al., 2022). We expect that many more methods will be developed for XAI in the future.
Note that the review and analysis presented in this paper are based on the works indexed in the Scopus database. Incorporating other databases in our deep journalism tool is expected to create additional parameters and structure of research on AI in energy systems. Future work will investigate the use of our deep journalism tool with additional research databases.
RA and RM conceived, developed, analysed, and validated the study. RA developed the software. RA and RM prepared the initial draft, reviewed and edited by RM and IK. RM and IK provided supervision, funds, resources, and contributed to the article editing.
The authors acknowledge with thanks the technical and financial support from the Deanship of Scientific Research (DSR) at the King Abdulaziz University (KAU), Jeddah, Saudi Arabia, under Grant No. RG-11-611-38.
The work carried out in this paper is supported by the HPC Center at the King Abdulaziz University.
The authors declare that the research was conducted in the absence of any commercial or financial relationships that could be construed as a potential conflict of interest.
All claims expressed in this article are solely those of the authors and do not necessarily represent those of their affiliated organizations, or those of the publisher, the editors and the reviewers. Any product that may be evaluated in this article, or claim that may be made by its manufacturer, is not guaranteed or endorsed by the publisher.
Abdel-Razek, S. A., Marie, H. S., Alshehri, A., and Elzeki, O. M. (2022). Energy efficiency through the implementation of an AI model to predict room occupancy based on thermal comfort parameters. Sustainability 14 (13), 7734. doi:10.3390/SU14137734
Ahmad, I., Alqurashi, F., Abozinadah, E., and Mehmood, R. (2022). Deep journalism and DeepJournal V1.0: A data-driven deep learning approach to discover parameters for transportation. Sustain. Switz. 14 (9), 5711. doi:10.3390/SU14095711
Al-Abri, T., Onen, A., Al-Abri, R., Hossen, A., Al-Hinai, A., Jung, J., et al. (2022). Review on energy application using blockchain Technology with an introductions in the pricing infrastructure. IEEE Access 10, 80119–80137. doi:10.1109/ACCESS.2022.3194161
Alagumalai, A., Shou, W., Mahian, O., Aghbashlo, M., Tabatabaei, M., Wongwises, S., et al. (2022). Self-powered sensing systems with learning capability. Joule 6 (7), 1475–1500. doi:10.1016/J.JOULE.2022.06.001
Alahmari, N., Alswedani, S., Alzahrani, A., Katib, I., Albeshri, A., and Mehmood, R. (2022). Musawah: A data-driven AI approach and tool to Co-create healthcare services with a case study on cancer disease in Saudi Arabia. Sustainability 14 (6), 3313. doi:10.3390/SU14063313
Alkhayat, G., Hasan, S. H., and Mehmood, R. (2022). Senergy: A novel deep learning-based auto-selective approach and tool for solar energy forecasting. Energies 15 (18), 6659. doi:10.3390/EN15186659
Allen, A. E. A., and Tkatchenko, A. (2022). Machine learning of material properties: Predictive and interpretable multilinear models. Sci. Adv. 8 (18), 7185. doi:10.1126/SCIADV.ABM7185/SUPPL_FILE/SCIADV.ABM7185_SM
Alomari, E., Katib, I., Albeshri, A., Yigitcanlar, T., and Mehmood, R. (2021). Iktishaf+: A big data tool with automatic labeling for road traffic social sensing and event detection using distributed machine learning. Sensors 21 (9), 2993. doi:10.3390/s21092993
Alqahtani, E., Janbi, N., Sharaf, S., and Mehmood, R. (2022). Smart homes and families to enable sustainable societies: A data-driven approach for multi-perspective parameter discovery using BERT modelling. Sustainability 14, 13534. doi:10.20944/PREPRINTS202208.0233.V1
Alswedani, S., Katib, I., Abozinadah, E., and Mehmood, R. (2022). Discovering urban governance parameters for online learning in Saudi Arabia during COVID-19 using topic modeling of twitter data. Front. Sustain. Cities 4, 1–24. doi:10.3389/FRSC.2022.751681
Altoub, M., AlQurashi, F., Yigitcanlar, T., Corchado, J. M., and Mehmood, R. (2022). An ontological knowledge base of poisoning attacks on deep neural networks. Appl. Sci. 12 (21), 11053. doi:10.3390/APP122111053
Amrouch, H., Chowdhury, A. B., Jin, W., Karri, R., Khorrami, F., Krishnamurthy, P., et al. (2021). “Special session: Machine learning for semiconductor test and reliability,” in Proceedings of the IEEE VLSI Test Symposium, San Diego, CA, USA, 25-28 April 2021. doi:10.1109/VTS50974.2021.9441052
Ardito, C., Deldjoo, Y., Noia, T. D., Sciascio, E. D., and Nazary, F. (2022). Visual inspection of fault type and zone prediction in electrical grids using interpretable spectrogram-based CNN modeling. Expert Syst. Appl. 210, 118368. doi:10.1016/J.ESWA.2022.118368
Arora, P., Khosravi, A., Panigrahi, B. K., and Suganthan, P. N. (2022). Remodelling state-space prediction with deep neural networks for probabilistic load forecasting. IEEE Trans. Emerg. Top. Comput. Intell. 6 (3), 628–637. doi:10.1109/TETCI.2021.3064028
Ashwini, B. P., Savithramma, R. M., and Sumathi, R. (2022). “Artificial intelligence in smart city applications: An overview,” in Proceedings - 2022 6th International Conference on Intelligent Computing and Control Systems, ICICCS, Madurai, India, 25-27 May 2022, 986–993. doi:10.1109/ICICCS53718.2022.9788152
Ashworth, B. R. (1990). Managing autonomy levels in the SSM/PMAD testbed. Proc. Intersoc. Energy Convers. Eng. Conf. 1, 263–268. doi:10.1109/IECEC.1990.716891
Baashar, Y., Alkawsi, G., Alkahtani, A. A., Hashim, W., Razali, R. A., and Tiong, S. K. (2021). Toward blockchain Technology in the energy environment. Sustainability 13 (16), 9008. doi:10.3390/SU13169008
Badr, M. M., Ibrahem, M. I., Mahmoud, M., Fouda, M. M., Alsolami, F., and Alasmary, W. (2022). Detection of false-reading attacks in smart grid net-metering system. IEEE Internet Things J. 9 (2), 1386–1401. doi:10.1109/JIOT.2021.3087580
Bandyopadhyay, S., Narayanam, R., Kumar, P., Ramchurn, S., Arya, V., and Petra, I. (2016). An axiomatic framework for ex-ante dynamic pricing mechanisms in smart grid. 30th AAAI Conf. Artif. Intell. AAAI 30, 3800–3806. doi:10.1609/aaai.v30i1.9900
Belinsky, A., and Afanasev, V. (2021). Optimal control of energy pipeline systems based on deep reinforcement learning. Lect. Notes Netw. Syst. 155, 1348–1355. doi:10.1007/978-3-030-59126-7_148
Belu, R. (2009). A project-based power electronics course with an increased content of renewable energy applications. ASEE Annu. Conf. Expo. Conf. Proc. doi:10.18260/1-2--4994
Berne, R. W. (2001). “Reaching and teaching through “The Matrix”; robosapiens, transhumanism, and the formidable in engineering ethics,” in ASEE Annual Conference Proceedings, Albuquerque, New Mexico, June 24, 2001, 8343–8350. doi:10.18260/1-2--9710
Beusmans, J., and Wieckert, K. (1989). Computing, research, and war: If knowledge is power, where is responsibility? Commun. ACM 32 (8), 939–951. doi:10.1145/65971.65973
Bhattacharjee, S., Islam, M. J., and Abedzadeh, S. (2022). “Robust anomaly based attack detection in smart grids under data poisoning attacks,” in CPSS 2022 - Proceedings of the 8th ACM Cyber-Physical System Security Workshop, New York, NY, United States, 30 May 2022, 3–14. doi:10.1145/3494107.3522778
Bhattacharjee, S., Madhavarapu, P., and Das, S. K. (2021). “A diversity index based scoring framework for identifying smart meters launching stealthy data falsification attacks,” in ASIA CCS 2021 - Proceedings of the 2021 ACM Asia Conference on Computer and Communications Security, New York, NY, United States, 4 June 2021, 26–39. doi:10.1145/3433210.3437527
Blondeel, M., Bradshaw, M. J., Bridge, G., and Kuzemko, C. (2021). The geopolitics of energy system transformation: A review. Geogr. Compass 15 (7), e12580. doi:10.1111/GEC3.12580
Boden, M. (1987). Artificial intelligence: Cannibal or missionary? AI Soc. 1 (1), 17–23. doi:10.1007/BF01905886
Bouziane, S. E., and Khadir, M. T. (2022). Towards an energy management system based on a multi-agent architecture and LSTM networks. J. Exp. Theor. Artif. Intell. doi:10.1080/0952813X.2022.2093407
Boyen, X., and Wehenkel, L. (1999). Automatic induction of fuzzy decision trees and its application to power system security assessment. Fuzzy Sets Syst. 102 (1), 3–19. doi:10.1016/S0165-0114(98)00198-5
Bracco , , Stefano , , Rosales-Asensio, E., Gonzalez-Martinez, A., Rosen, R. M., and Badidi, E. (2022). Edge AI and blockchain for smart sustainable cities: Promise and potential. Sustainability 20221414 (13), 7609. doi:10.3390/SU14137609
Cabrera, W., Benhaddou, D., and Ordonez, C. (2016). “Solar power prediction for smart community microgrid,” in 2016 IEEE International Conference on Smart Computing, St. Louis, MO, USA, 18-20 May 2016. doi:10.1109/SMARTCOMP.2016.7501718
Castelvecchi, D. (2016). Can we open the black box of AI? Nat. Int. Wkly. J. Sci. 538 (7623), 20–23. doi:10.1038/538020a
Ceglia, F., Esposito, P., Marrasso, E., and Sasso, M. (2020). From smart energy community to smart energy municipalities: Literature review, agendas and pathways. J. Clean. Prod. 254, 120118. doi:10.1016/J.JCLEPRO.2020.120118
Chen, Y., Huerta, E. A., Duarte, J., Harris, P., Katz, D. S., Neubauer, M. S., et al. (2021). A FAIR and AI-ready Higgs boson decay dataset. Sci. Data 9 (1), 31. doi:10.1038/s41597-021-01109-0
Chen, Y. Y., Lin, Y. H., Kung, C. C., Chung, M. H., and Yen, I. H. (2019). Design and implementation of cloud analytics-assisted smart power meters considering advanced artificial intelligence as edge analytics in demand-side management for smart homes. Sensors (Basel, Switz. 19 (9), 2047. doi:10.3390/S19092047
Christie, R. D. (1990). Impact of artificial intelligence on plant and system operations. Instrum. Control, Automation Power Industry Proc. 33, 193–197.
D’amore, G., Di Vaio, A., Balsalobre-Lorente, D., and Boccia, F. (2022). Artificial intelligence in the water–energy–food model: A holistic approach towards sustainable development goals. Sustainability 14, 867. doi:10.3390/SU14020867
del Campo-Ávila, J., Takilalte, A., Bifet, A., and Mora-López, L. (2021). Binding data mining and expert knowledge for one-day-ahead prediction of hourly global solar radiation. Expert Syst. Appl. 167, 114147. doi:10.1016/J.ESWA.2020.114147
Doran, D., Schulz, S., and Besold, T. R. (2017). What does explainable AI really mean? A new conceptualization of perspectives. CEUR Workshop Proc. 2017, 2071. doi:10.48550/arxiv.1710.00794
Du, Y.-L., Pablos, D., and Tywoniuk, K. (2021). Classification of quark and gluon jets in hot QCD medium with deep learning. Proc. Sci. 380. doi:10.22323/1.380.0224
Ebadi , , Saeed , , Ghasembaglou, M., Navin, A. H., and Mirnia, M. K. (2010). “Energy balancing in wireless sensor networks with selecting two cluster-heads in hierarchical clustering,” in Proceedings - 2010 International Conference on Computational Intelligence and Communication Networks, CICN, Bhopal, India, 26-28 November 2010, 230–233. doi:10.1109/CICN.2010.55
Egging, R. (2013). Drivers, trends, and uncertainty in long-term price projections for energy management in public buildings. Energy Policy 62, 617–624. doi:10.1016/J.ENPOL.2013.07.022
Fidalgo, J. N., Torres, J. A. F. M., and Matos, M. (2007). “Fair allocation of distribution losses based on neural networks,” in 2007 International Conference on Intelligent Systems Applications to Power Systems, ISAP, Kaohsiung, Taiwan, 05-08 November 2007. doi:10.1109/ISAP.2007.4441685
Fisekovic, N., and Popovic, D. B. (2001). New controller for functional electrical stimulation systems. Med. Eng. Phys. 23 (6), 391–399. doi:10.1016/S1350-4533(01)00069-8
Gao, Y., and Yu, N. (2021). “Deep reinforcement learning in power distribution systems: Overview, challenges, and opportunities,” in 2021 IEEE Power and Energy Society Innovative Smart Grid Technologies Conference, ISGT, Washington, DC, USA, 16-18 February 2021. doi:10.1109/ISGT49243.2021.9372283
Ghosh , , Sagnik , , Sarkar, A., Mitra, A., and Das, A. (2020). “Smart cropping based on predicted solar radiation using IoT and machine learning,” in Proceedings of IEEE International Conference on Advent Trends in Multidisciplinary Research and Innovation, ICATMRI, Buldhana, India, 30-30 December 2020. doi:10.1109/ICATMRI51801.2020.9398323
Gill-Wiehl, A., Miles, S., Wu, J., and Kammen, D. (2022). Beyond customer acquisition: A comprehensive review of community participation in mini grid projects. Renew. Sustain. Energy Rev. 153, 111778. doi:10.1016/J.RSER.2021.111778
Goebel , , Randy , , Chander, A., Holzinger, K., Lecue, F., Akata, Z., et al. (2018). Explainable AI: The new 42? Lect. Notes Comput. Sci. Incl. Subser. Lect. Notes Artif. Intell. Lect. Notes Bioinforma. 11015, 295–303. doi:10.1007/978-3-319-99740-7_21/FIGURES/6
Goulas, A., Goodwin, D., Shannon, C., Jeffrey, P., and Smith, H. M. (2022). Public perceptions of household IoT smart water “event” meters in the UK—implications for urban water governance. Front. Sustain. Cities 4, 10. doi:10.3389/frsc.2022.758078
Grootendorst, M. (2021). Interactive topic modeling with BERTopic. Tilburg, North Brabant, Netherlands: Towards Data Science.
Gunaratne, N. G. T., Abdollahian, M., Huda, S., Ali, M., and Fortino, G. (2022). An edge tier task offloading to identify sources of variance shifts in smart grid using a hybrid of wrapper and filter approaches. IEEE Trans. Green Commun. Netw. 6 (1), 329–340. doi:10.1109/TGCN.2021.3137330
Gurram, G. V., Shariff, N. C., and Biradar, R. L. (2022). A secure energy aware meta-heuristic routing protocol (SEAMHR) for sustainable IoT-wireless sensor network (WSN). Theor. Comput. Sci. 930, 63–76. doi:10.1016/J.TCS.2022.07.011
Gürses-Tran, G., Körner, T. A., and Monti, A. (2022). Introducing explainability in sequence-to-sequence learning for short-term load forecasting. Electr. Power Syst. Res. 212, 108366. doi:10.1016/J.EPSR.2022.108366
Gutierrez-Rojas, D., Christou, I. T., Dantas, D., Narayanan, A., Nardelli, P. H. J., and Yang, Y. (2022). Performance evaluation of machine learning for fault selection in power transmission lines. Knowl. Inf. Syst. 64 (3), 859–883. doi:10.1007/s10115-022-01657-w
Hager, A. (1967). Studies on the light-induced reversible xanthophyll-conversions in Chlorella and spinach leaves. Planta 74, 148–172. doi:10.1007/BF00388326
Hagras, H. (2018). Toward human-understandable, explainable AI. Computer 51 (9), 28–36. doi:10.1109/MC.2018.3620965
Hasan, K., Ramsay, B., and Moyes, I. (1994). Object oriented expert systems for real-time power system alarm processing: Part I. Selection of a toolkit. Electr. Power Syst. Res. 30 (1), 69–75. doi:10.1016/0378-7796(94)90061-2
Haseeb, K., Rehman, A., Saba, T., Bahaj, S. A., and Lloret, J. (2022). Device-to-Device (D2D) multi-criteria learning algorithm using secured sensors. Sensors 22 (6), 2115. doi:10.3390/S22062115
Himeur, Y., Alsalemi, A., Bensaali, F., Amira, A., Varlamis, I., Bravos, G., et al. (2022). Techno-economic assessment of building energy efficiency systems using behavioral change: A case study of an edge-based micro-moments solution. J. Clean. Prod. 331, 129786. doi:10.1016/J.JCLEPRO.2021.129786
Hoffman, R. R., Mueller, S. T., Klein, G., and Litman, J. (2018) ‘Metrics for explainable AI: Challenges and prospects’. doi:10.48550/arxiv.1812.04608
Huang, C., Wang, X., and Wang, X. (2022). Effective-capacity-based resource allocation for end-to-end multi-connectivity in 5G IAB networks. IEEE Journals Mag. 21, 6302–6316. doi:10.1109/TWC.2022.3148203
Huang, L., and Ling, C. (2020). Practicing deep learning in materials science: An evaluation for predicting the formation energies. J. Appl. Phys. 128 (12), 124901. doi:10.1063/5.0012411
Hussain, A., Bui, V. H., and Kim, H. M. (2019). Microgrids as a resilience resource and strategies used by microgrids for enhancing resilience. Appl. Energy 240, 56–72. doi:10.1016/J.APENERGY.2019.02.055
Ilyas, M. (2021). “IoT applications in smart cities,” in 2021 IEEE International Conference on Electronic Communications, Internet of Things and Big Data, ICEIB, Yilan County, Taiwan, 10-12 December 2021, 44–47. doi:10.1109/ICEIB53692.2021.9686400
Irisarri, G., Kirschen, D., Miller, B., and Mokhtari, S. (1996). Integration of artificial intelligence applications in the ems: Issues and solutions. IEEE Trans. Power Syst. 11 (1), 475–482. doi:10.1109/59.486136
Janbi, N., Mehmood, R., Katib, I., Albeshri, A., Corchado, J. M., and Yigitcanlar, T. (2022). Imtidad: A reference architecture and a case study on developing distributed AI services for skin disease diagnosis over cloud, fog and edge. Sensors 22 (5), 1854. doi:10.3390/S22051854
Janik, D. F., and Gholdston, E. W. (1992). A prototype ground support system security monitor for space based power system health monitoring. SAE Tech. Pap. 1992, 929332. doi:10.4271/929332
Jennings, N. R. (1995). Controlling cooperative problem solving in industrial multi-agent systems using joint intentions. Artif. Intell. 75 (2), 195–240. doi:10.1016/0004-3702(94)00020-2
Jose, D. T., Holme, J., Chakravorty, A., and Rong, C. (2022). Integrating big data and blockchain to manage energy smart grids—TOTEM framework. Blockchain Res. Appl. 3 (3), 100081. doi:10.1016/J.BCRA.2022.100081
Kashihara, A., Hirashima, T., Toyoda, J., and Nakamura, Y. (1992). Advanced explanation capabilities for intelligent tutoring systems: The explanation structure model (EXSEL). Syst. Comput. Jpn. 23 (12), 93–107. doi:10.1002/SCJ.4690231209
Khan, T., Singh, K., Manjul, M., Ahmad, M. N., Zain, A. M., and Ahmadian, A. (2022). A temperature-aware trusted routing scheme for sensor networks: Security approach. Comput. Electr. Eng. 98, 107735. doi:10.1016/J.COMPELECENG.2022.107735
Khosrojerdi , , Farhad , , Akhigbe, O., Gagnon, S., Ramirez, A., and Richards, G. (2022). Integrating artificial intelligence and analytics in smart grids: A systematic literature review. Int. J. Energy Sect. Manag. 16 (2), 318–338. doi:10.1108/IJESM-06-2020-0011/FULL/XML
Kiupel, N., Koppen-Seliger, B., Kellinghaus, H., and Frank, P. (1995). “Fuzzy residual evaluation concept (FREC),” in Proceedings of the IEEE International Conference on Systems, Man and Cybernetics, Vancouver, BC, Canada, 22-25 October 1995, 13–18. doi:10.1109/ICSMC.1995.537725
Kleisarchaki , , Sofia , , Gurgen, L., Kassa, Y. M., Krystek, M., and Vidal, D. G. (2022). “Optimization of soft mobility localization with sustainable policies and open data,” in 2022 18th International Conference on Intelligent Environments, IE 2022 - Proceedings, Biarritz, France, 20-23 June 2022. doi:10.1109/IE54923.2022.9826779
Kochen, M. (1975). Hypothesis processing as a new tool to aid managers of mental health agencies in serving long-term regional interests. Int. J. Bio-Medical Comput. 6 (4), 299–312. doi:10.1016/0020-7101(75)90013-6
Kolangiappan, J., and Kumar, A. S. (2022). A novel framework for the prevention of black-hole in wireless sensors using hybrid convolution network. Sci. Tech. J. Inf. Technol. Mech. Opt. 22 (2), 317–323. doi:10.17586/2226-1494-2022-22-2-317-323
Kong, P. Y., and Song, Y. (2020). Joint consideration of communication network and power grid topology for communications in community smart grid. IEEE Trans. Industrial Inf. 16 (5), 2895–2905. doi:10.1109/TII.2019.2912670
Krishna, V., and Ramesh, V. C. (1998). Intelligent agents for negotiations in market games, part 1: Model. IEEE Trans. Power Syst. 13 (3), 1103–1108. doi:10.1109/59.709106
Kruse, J., Schäfer, B., and Witthaut, D. (2022). Secondary control activation analysed and predicted with explainable AI. Electr. Power Syst. Res. 212, 108489. doi:10.1016/J.EPSR.2022.108489
Landwehr, J. P., Kühl, N., Walk, J., and Gnädig, M. (2022). Design knowledge for deep-learning-enabled image-based decision support systems: Evidence from power line maintenance decision-making. Bus. Inf. Syst. Eng. 64, 707–728. doi:10.1007/S12599-022-00745-Z/FIGURES/9
Lee, H., Bere, G., Kim, K., Ochoa, J. J., Park, J., and Kim, T. (2020). “Deep learning-based false sensor data detection for battery energy storage systems,” in 2020 IEEE CyberPELS, CyberPELS, Miami, FL, USA, 13-13 October 2020. doi:10.1109/CYBERPELS49534.2020.9311542
Lin, Y. J., Chen, Y. C., Zheng, J. Y., Chu, D., Shao, D. W., and Yang, H. T. (2022). Blockchain power trading and energy management platform. IEEE Access 10, 75932–75948. doi:10.1109/ACCESS.2022.3189472
Liu, W., Liu, Y., Zhang, T., Han, Y., Zhou, X., Xie, Y., et al. (2022). Use of physics to improve solar forecast: Part II, machine learning and model interpretability. Sol. Energy 244, 362–378. doi:10.1016/J.SOLENER.2022.08.040
Longmire, N., and Banuti, D. T. (2022). Onset of heat transfer deterioration caused by pseudo-boiling in CO2 laminar boundary layers. Int. J. Heat Mass Transf. 193, 122957. doi:10.1016/J.IJHEATMASSTRANSFER.2022.122957
López Santos, M., Garcia-Santiago, X., Echevarria Camarero, F., Blazquez Gil, G., and Carrasco Ortega, P. (2022). Application of temporal fusion transformer for day-ahead PV power forecasting. Energies 15 (14), 5232. doi:10.3390/EN15145232
Lundberg, S. M., Erion, G., Chen, H., DeGrave, A., Prutkin, J. M., Nair, B., et al. (2020). From local explanations to global understanding with explainable AI for trees. Nat. Mach. Intell. 2 (1), 56–67. doi:10.1038/s42256-019-0138-9
Luo, Y., Lu, C., Zhu, L., and Song, J. (2021). Graph convolutional network-based interpretable machine learning scheme in smart grids. IEEE Trans. Automation Sci. Eng. 20, 47–58. doi:10.1109/TASE.2021.3090671
Lupo, S., and Kiprakis, A. (2015). Agent-based models for electricity markets accounting for smart grid participation. LNICST 154, 48–57. doi:10.1007/978-3-319-25479-1_4
MacDougall, P., Ran, B., Huitema, G. B., and Deconinck, G. (2017). “Multi-goal optimization of competing aggregators using a web-of-cells approach,” in 2017 IEEE PES Innovative Smart Grid Technologies Conference Europe, ISGT-Europe, Turin, Italy, 26-29 September 2017, 1–6. doi:10.1109/ISGTEUROPE.2017.8260335
Machlev, R., Heistrene, L., Perl, M., Levy, K., Belikov, J., Mannor, S., et al. (2022). Explainable Artificial Intelligence (XAI) techniques for energy and power systems: Review, challenges and opportunities. Energy AI 9, 100169. doi:10.1016/J.EGYAI.2022.100169
Manfren, M., James, P. A., and Tronchin, L. (2022). Data-driven building energy modelling – an analysis of the potential for generalisation through interpretable machine learning. Renew. Sustain. Energy Rev. 167, 112686. doi:10.1016/J.RSER.2022.112686
Mehmood, R., Alam, F., Albogami, N. N., Katib, I., Albeshri, A., and Altowaijri, S. M. (2017). UTiLearn: A personalised ubiquitous teaching and learning system for smart societies. IEEE Access 5, 2615–2635. doi:10.1109/ACCESS.2017.2668840
Mehmood, R. (2022). Deep journalism” driven by AI can aid better government. Available at: https://www.themandarin.com.au/201467-deep-journalism-driven-by-ai-can-aid-better-government/(Accessed October 15, 2022).
Miles, C., Carbone, M. R., Sturm, E. J., Lu, D., Weichselbaum, A., Barros, K., et al. (2021). Machine learning of Kondo physics using variational autoencoders and symbolic regression. Phys. Rev. B 104 (23), 235111. doi:10.1103/PhysRevB.104.235111
Molley, P. A. (1996). Computer vision challenges and technologies for agile manufacturing. Proc. SPIE 2727, 1036–1037. doi:10.1117/12.233237
Moreira, M. P., Santos, L. T. B., and Vellasco, M. M. B. R. (2007). “Power transformers diagnosis using neural networks,” in IEEE International Conference on Neural Networks - Conference Proceedings, Orlando, FL, USA, 12-17 August 2007, 1929–1934. doi:10.1109/IJCNN.2007.4371253
Motevalli, B., Fox, B. L., and Barnard, A. S. (2022). Charge-dependent Fermi level of graphene oxide nanoflakes from machine learning. Comput. Mater. Sci. 211, 111526. doi:10.1016/J.COMMATSCI.2022.111526
Nagaraj, K., Starke, A., and McNair, J. (2021). “Glass: A graph learning approach for software defined network based smart grid DDoS security,” in IEEE International Conference on Communications, Montreal, QC, Canada, 14-23 June 2021. doi:10.1109/ICC42927.2021.9500999
Naoui, M. A., Lejdel, B., Ayad, M., Amamra, A., and kazar, O. (2021). Using a distributed deep learning algorithm for analyzing big data in smart cities. Smart Sustain. Built Environ. 10 (1), 90–105. doi:10.1108/sasbe-04-2019-0040
Nemer, I. A., Sheltami, T. R., Belhaiza, S., and Mahmoud, A. S. (2022). Energy-efficient UAV movement control for fair communication coverage: A deep reinforcement learning approach. Sensors 22 (5), 1919. doi:10.3390/S22051919
Niet, I., van Est, R., and Veraart, F. (2021). Governing AI in electricity systems: Reflections on the EU artificial intelligence bill. Front. Artif. Intell. 4, 690237. doi:10.3389/frai.2021.690237
Nitzberg, M., and Zysman, J. (2022). Algorithms, data, and platforms: The diverse challenges of governing AI. J. Eur. Public Policy 2022, 26. doi:10.1080/13501763.2022.2096668
Parra, I., Arroyo, G., and Garcia, A. (2014). “Experiences and practices in the implementation of IT Governance in Mexican electric utility,” in CIGRE Session 45 - 45th International Conference on Large High Voltage Electric Systems, 2014-August.
Perri, S. (2001). Ethics, regulation and the new artificial intelligence, part I: Accountability and Power. Inf. Commun. Soc. 4 (2), 199–229. doi:10.1080/713768525
Phillips, P. J., Carina, A. H., Peter, C. F., Yates, A. N., Greene, K., Broniatowski, D. A., et al. (2021). Four principles of explainable artificial intelligence. Gaithersburg, MD: National Institute of Standards and Technology. NIST Interagency/Internal Report (NISTIR) - 8312. doi:10.6028/NIST.IR.8312
Pinson, P., Han, L., and Kazempour, J. (2021). Regression markets and application to energy forecasting. TOP 30, 533–573. doi:10.1007/s11750-022-00631-7
Przhedetsky, L. (2021). “Designing effective and accessible consumer protections against unfair treatment in markets where automated decision making is used to determine access to essential services: A case study in Australia’s housing market,” in AIES 2021 - Proceedings of the 2021 AAAI/ACM Conference on AI, Ethics, and Society, New York, NY, United States, 30 July 2021, 279–280. doi:10.1145/3461702.3462468
Qin, Z., Liu, Z., Han, G., Lin, C., Guo, L., and Xie, L. (2021). Distributed UAV-BSS trajectory optimization for user-level fair communication service with multi-agent deep reinforcement learning. IEEE Trans. Veh. Technol. 70 (12), 12290–12301. doi:10.1109/TVT.2021.3117792
Raper, R., Boeddinghaus, J., Coeckelbergh, M., Gross, W., Campigotto, P., and Lincoln, C. N. (2022). Sustainability budgets: A practical management and governance method for achieving goal 13 of the sustainable development goals for AI development. Sustainability 14 (7), 4019. doi:10.3390/SU14074019
Rosic, D., Novak, U., and Vukmirovic, S. (2013). “Role-based access control model supporting regional division in smart grid system,” in Proceedings - 5th International Conference on Computational Intelligence, Communication Systems, and Networks, CICSyN, Madrid, Spain, 05-07 June 2013, 197–201. doi:10.1109/CICSYN.2013.59
Saheb, T., Dehghani, M., and Saheb, T. (2022). Artificial intelligence for sustainable energy: A contextual topic modeling and content analysis. Sustain. Comput. Inf. Syst. 35, 100699. doi:10.1016/J.SUSCOM.2022.100699
Seid, A. M., Boateng, G. O., Anokye, S., Kwantwi, T., Sun, G., and Liu, G. (2021). Collaborative computation offloading and resource allocation in multi-UAV-assisted IoT networks: A deep reinforcement learning approach. IEEE Internet Things J. 8 (15), 12203–12218. doi:10.1109/JIOT.2021.3063188
Selfridge, O. G., and Franklin, J. A. (1990). “The perceiving robot: What does it see? What does it do?,” in Proceedings. 5th IEEE International Symposium on Intelligent Control 1990, Philadelphia, PA, USA, 05-07 September 1990, 146–151. doi:10.1109/ISIC.1990.128453
Senevirathne, P. R., Senarathne, L. R., Muthunaike, I. U., and Wij, J. V. (2019). “Optimal residential load scheduling in dynamic tariff environment,” in 2019 IEEE 14th International Conference on Industrial and Information Systems: Engineering for Innovations for Industry 4.0, ICIIS, Kandy, Sri Lanka, 18-20 December 2019, 547–552. doi:10.1109/ICIIS47346.2019.9063296
Serna Torre, P., and Hidalgo-Gonzalez, P. (2022). Decentralized Optimal Power Flow for time-varying network topologies using machine learning. Electr. Power Syst. Res. 212, 108575. doi:10.1016/J.EPSR.2022.108575
Siddiqi, U., and Lubkeman, D. (1988). “Expert system DISPATCHER’S aid for distribution feeder fault diagnosis,” in Proceedings of the Annual Southeastern Symposium on System Theory, Charlotte, NC, USA, 20-22 March 1988, 519–523. doi:10.1109/SSST.1988.17105
Singstock, N. R., Jessica, C. O.-R, Perryman, J. T., Sutton, C., Velazquez, J. M., Musgrave, C. B., et al. (2021). Machine learning guided synthesis of multinary Chevrel phase chalcogenides. J. Am. Chem. Soc. 143 (24), 9113–9122. doi:10.1021/JACS.1C02971/SUPPL_FILE/JA1C02971_SI_001
Skowronek, M., Gilberti, R. M., Petro, M., Sancomb, C., Maddern, S., and Jankovic, J. (2022). Inclusive STEAM education in diverse disciplines of sustainable energy and AI. Energy AI 7, 100124. doi:10.1016/J.EGYAI.2021.100124
Soret, B., Nguyen, L. D., Seeger, J., Broring, A., Issaid, C. B., Samarakoon, S., et al. (2022). Learning, computing, and trustworthiness in intelligent IoT environments: Performance-energy tradeoffs. IEEE Trans. Green Commun. Netw. 6 (1), 629–644. doi:10.1109/TGCN.2021.3138792
Stamper, R. (1988). Pathologies of AI: Responsible use of artificial intelligence in professional work. AI Soc. 2 (1), 3–16. doi:10.1007/BF01891439
Stephan, T., Al-Turjman, F., and Balusamy, B. (2021). Energy and spectrum aware unequal clustering with deep learning based primary user classification in cognitive radio sensor networks. Int. J. Mach. Learn. Cybern. 12 (11), 3261–3294. doi:10.1007/s13042-020-01154-y
Stroemich, C., and Thomas, M. (1997). Short-term load forecasting system using adaptive logic networks. Proc. Am. Power Conf. 59 (1), 161–165.
Sujan Reddy, A., Akashdeep, S., Harshvardhan, R., and Sowmya Kamath, S. (2022). Stacking Deep learning and Machine learning models for short-term energy consumption forecasting. Adv. Eng. Inf. 52, 101542. doi:10.1016/J.AEI.2022.101542
Sun, J., Wu, C., Cheng, Y., Liu, B., and Li, D. (2021). “Explainable AI enabled conductor galloping predictor design,” in 2021 IEEE Power and Energy Society Innovative Smart Grid Technologies Conference, ISGT, Washington, DC, USA, 16-18 February 2021. doi:10.1109/ISGT49243.2021.9372239
Sun, Q., Wang, X., Liu, Z., Mirsaeidi, S., He, J., and Pei, W. (2022). Multi-agent energy management optimization for integrated energy systems under the energy and carbon co-trading market. Appl. Energy 324, 119646. doi:10.1016/J.APENERGY.2022.119646
Trovato, S. A., Imai, M., and Touchton, R. A. (1990). Implementation of an on-line expert system in a nuclear power plant. Reactor emergency action level monitor. Am. Soc. Mech. Eng. Dyn. Syst. Control Div. Publ. DSC 23, 1–7.
Tsoka, T., Ye, X., Chen, Y., Gong, D., and Xia, X. (2022). Explainable artificial intelligence for building energy performance certificate labelling classification. J. Clean. Prod. 355, 131626. doi:10.1016/J.JCLEPRO.2022.131626
Vakulchuk, R., Overland, I., and Scholten, D. (2020). Renewable energy and geopolitics: A review. Renew. Sustain. Energy Rev. 122, 109547. doi:10.1016/J.RSER.2019.109547
Vale, Z., Pinto, T., Praca, I., and Morais, H. (2011). Mascem: Electricity markets simulation with strategic agents. IEEE Intell. Syst. 26 (2), 9–17. doi:10.1109/MIS.2011.3
Volkova , , Anna , , Patil, A. D., Javadi, S. A., and Meer, H. D. (2022). “Accountability challenges of AI in smart grid services,” in e-Energy 2022 - Proceedings of the 2022 13th ACM International Conference on Future Energy Systems, 597–601. doi:10.1145/3538637.3539636
Volodin, V. S., and Tolokonskij, A. O. (2022). Application of machine learning for solving problems of nuclear power plant operation. Stud. Comput. Intell. 1032, 585–589. doi:10.1007/978-3-030-96993-6_65/COVER
Wang, X., Ba, Y., Liu, R., Wang, H., Ju, R., Shi, W., et al. (2021). Model-data integration driven based power system frequency response model. 2021 IEEE Int. Conf. Artif. Intell. Comput. Appl. ICAICA 2021, 107–112. doi:10.1109/ICAICA52286.2021.9498140
Wang, Y., Zhou, X., Shi, Y., Zheng, Z., Zeng, Q., Chen, L., et al. (2021). Transmission network expansion planning considering wind power and load uncertainties based on multi-agent ddqn. Energies 14 (19), 6073. doi:10.3390/EN14196073
Wehenkel, L., Pavella, M., Euxibie, E., and Heilbronn, B. (1994). Decision tree based transient stability method a case study. IEEE Trans. Power Syst. 9 (1), 459–469. doi:10.1109/59.317577
Wenninger, S., Kaymakci, C., and Wiethe, C. (2022). Explainable long-term building energy consumption prediction using QLattice. Appl. Energy 308, 118300. doi:10.1016/J.APENERGY.2021.118300
Wu, J., Li, Q., Chen, Q., Peng, G., Wang, J., Fu, Q., et al. (2022). Evaluation, analysis and diagnosis for hvdc transmission system faults via knowledge graph under new energy systems construction: A critical review. Energies 15 (21), 8031. doi:10.3390/EN15218031
Xiao, H., Zhao, J., Pei, Q., Feng, J., Liu, L., and Shi, W. (2022). Vehicle selection and resource optimization for federated learning in vehicular edge computing. IEEE Trans. Intelligent Transp. Syst. 23 (8), 11073–11087. doi:10.1109/TITS.2021.3099597
Xie, Y., Ueda, Y., and Sugiyama, M. (2021). A two-stage short-term load forecasting method using long short-term memory and multilayer perceptron. Energies 14 (18), 5873. doi:10.3390/EN14185873
Xu, C., Li, C., and Zhou, X. (2022). Interpretable LSTM based on mixture attention mechanism for multi-step residential load forecasting. Electronics 11 (14), 2189. doi:10.3390/ELECTRONICS11142189
Xu, C., Liao, Z., Li, C., Zhou, X., and Xie, R. (2022). Review on interpretable machine learning in smart grid. Energies 15 (12), 4427. doi:10.3390/EN15124427
Yang, Z., Shi, Y., Zhou, Y., Wang, Z., and Yang, K. (2022). Trustworthy federated learning via blockchain. New Jersey, United States: IEEE Internet of Things Journal, 1. doi:10.48550/arxiv.2209.04418
Yardley, T., Uludag, S., Nahrstedt, K., and Sauer, P. (2015). “Developing a Smart Grid cybersecurity education platform and a preliminary assessment of its first application,” in Proceedings - Frontiers in Education Conference, FIE, Madrid, Spain, 22-25 October 2014. doi:10.1109/FIE.2014.7044273
Yeckle, J., and Tang, B. (2018). “Detection of electricity theft in customer consumption using outlier detection algorithms,” in Proceedings - 2018 1st International Conference on Data Intelligence and Security, ICDIS, South Padre Island, TX, USA, 08-10 April 2018, 135–140. doi:10.1109/ICDIS.2018.00029
Yigitcanlar, T., Corchado, J. M., Mehmood, R., Li, R. Y. M., Mossberger, K., and Desouza, K. (2021). Responsible urban innovation with local government artificial intelligence (AI): A conceptual framework and research agenda. J. Open Innovation Technol. Mark. Complex. 7 (1), 71. doi:10.3390/joitmc7010071
Zamponi, M. E., and Barbierato, E. (2022). The dual role of artificial intelligence in developing smart cities. Smart Cities 5 (2), 728–755. doi:10.3390/SMARTCITIES5020038
Zarazua de Rubens, G., and Noel, L. (2019). The non-technical barriers to large scale electricity networks: Analysing the case for the US and EU supergrids. Energy Policy 135, 111018. doi:10.1016/J.ENPOL.2019.111018
Zhang, C. Y., Liang, S., He, C., and Wang, K. (2022). Multi-UAV trajectory design and power control based on deep reinforcement learning. J. Commun. Inf. Netw. 7 (2), 192–201. doi:10.23919/JCIN.2022.9815202
Zhang, S., Zhao, Z., Xia, X., Cui, W., Zhang, Z., and Shan, R. (2023). Risk model and decision support system of state grid operation management based on big data. Lect. Notes Data Eng. Commun. Technol. 122, 419–427. doi:10.1007/978-981-19-3632-6_51/COVER
Zhang, Z., Xu, C., and Wu, R. (2022). Learning-based trajectory design and time allocation in UAV-supported wireless powered NOMA-IoT networks. 2022 IEEE Int. Conf. Commun. Work. ICC Work. 2022, 1041–1046. doi:10.1109/ICCWORKSHOPS53468.2022.9814676
Zhao, J. (2021). “A survey: New generation artificial intelligence and its application in power system dispatching and operation,” in 5th IEEE Conference on Energy Internet and Energy System Integration: Energy Internet for Carbon Neutrality, EI2, Taiyuan, China, 22-24 October 2021, 3178–3183. doi:10.1109/EI252483.2021.9713148
Keywords: AI explainability, AI governance, smart energy systems, smart grid, AI trustworthiness, natural language processing (NLP), topic modelling, BERTopic
Citation: Alsaigh R, Mehmood R and Katib I (2023) AI explainability and governance in smart energy systems: A review. Front. Energy Res. 11:1071291. doi: 10.3389/fenrg.2023.1071291
Received: 16 October 2022; Accepted: 16 January 2023;
Published: 27 January 2023.
Edited by:
Tiago Pinto, University of Trás-os-Montes and Alto Douro, PortugalReviewed by:
Brígida Teixeira, Polytechnic Institute of porto, PortugalCopyright © 2023 Alsaigh, Mehmood and Katib. This is an open-access article distributed under the terms of the Creative Commons Attribution License (CC BY). The use, distribution or reproduction in other forums is permitted, provided the original author(s) and the copyright owner(s) are credited and that the original publication in this journal is cited, in accordance with accepted academic practice. No use, distribution or reproduction is permitted which does not comply with these terms.
*Correspondence: Rashid Mehmood, Uk1laG1vb2RAa2F1LmVkdS5zYQ==
Disclaimer: All claims expressed in this article are solely those of the authors and do not necessarily represent those of their affiliated organizations, or those of the publisher, the editors and the reviewers. Any product that may be evaluated in this article or claim that may be made by its manufacturer is not guaranteed or endorsed by the publisher.
Research integrity at Frontiers
Learn more about the work of our research integrity team to safeguard the quality of each article we publish.