- National Engineering Research Center of Power Generation Control and Safety, School of Energy and Environment, Southeast University, Nanjing, China
Hybrid energy system based on renewable energy is an important way to solve current energy and environmental problems. However, its achievable benefits need to be realized through an integrated optimization that considers optimization objectives, optimization models, and optimization algorithms. In this paper, the integrated structure and configuration optimization techniques of the hybrid energy system based on renewable energy (RE-HES) is summarized. The performance indicators, system characteristics, model construction, and solutions of RE-HES optimized configuration are comprehensively reviewed. Current review highlights the diversification of performance evaluation index, consideration of uncertainty and dynamic properties, superstructure approaches and intelligent algorithms.
1 Introduction
The hybrid energy system based on renewable energy (RE-HES) has advantages of high efficiency, economy and low carbon emission, and is considered to be one of the effective ways to solve problems of energy shortage, environmental pollution and greenhouse gas emissions (Abba and Chee, 2019; Yi et al., 2021). RE-HES has high degree of flexibility and diversity, it provides a good platform for the comprehensive utilization of various energy sources (Mala and Rajeshwer, 2020), including the complementarity of continuous energy and intermittent energy (Merei et al., 2013), the complementarity of high-carbon energy and low-carbon energy (Yongpin et al., 2016; Junjie et al., 2022), the complementarity of electricity and gas (Merei et al., 2013), the complementarity of electricity and heat (Zeng et al., 2016), and so on.
RE-HES has many application scenarios, such as residential communities, office buildings, commercial areas, campuses, hospitals, and airports. Another potential application scenario is the data center (Pei et al., 2020). The data center consumes a large amount of energy (Caishan et al., 2021). RE-HES can provide efficient green energy for data centers to meet its power and cooling needs. At the same time, the large amount of waste heat generated by the data center can also be combined with RE-HES to further improve the overall efficiency and economy of the system (Howard and Shengwei, 2019).
The performance of RE-HES in various application scenarios must first be guaranteed through configuration optimization. During the system configuration process, various energy sources are added as needed, the type and capacity of each device are selected, and its operation strategy is determined to make the final configured production system more economical, energy-efficient, and environmentally friendly (Seyed et al., 2022). RE-HES has numerous devices, diverse structures, and flexible operation strategies, which bring great challenges to the configuration optimization of the hybrid energy system. To this end, many literatures focus on the configuration optimization problem of RE-HES (Barun and Mahmudul, 2021; Lin et al., 2017).
Performance evaluation, model building, and model solving are the three main elements of a configuration optimization problem. In terms of performance evaluation, most researches are based on economic, energy saving, and environmental protection indexes (Liu et al., 2022), but in order to analyze system performance more comprehensively, many new evaluation indexes have also been developed in recent years (Ni et al., 2020) (Zhong et al., 2021). The construction of the model is closely related to the research object and research needs. In addition to the need to reasonably construct the objective function (mainly based on the research results of evaluation indexes), it is more important to reasonably describe the constraints (Wei et al., 2017; Cao et al., 2018). The constraints here include not only the internal constraints of the system and the physical interaction constraints between the system and the outside world, but also various non-physical constraints, such as economic constraints, human preference settings, etc. (Ahmad, 2021). The solution of the model also deserves attention. The configuration optimization model of the hybrid energy system is usually complex, which brings many challenges to the research of the solution algorithm. Many solving algorithms have been proposed, including classical algorithms (Yunfei et al., 2020), intelligent algorithms (Paliwal et al., 2014) and hybrid algorithms (Askarzadeh, 2013).
This paper aims to address the issues related to configuration optimization of RE-HES with a detailed review of performance evaluation, model building and model solving and other aspects of configuration optimization. The paper is structured as follows: Section 2 presents the integrated structure of RE-HES; Section 3 summarizes performance evaluation indexes of RE-HES; Subsequently, uncertainty and dynamics characteristic are concern in Section 4; Section 5 presents configuration optimization techniques used for RE-HES. Finally, conclusions are made.
2 Integrated structure of RE-HES
According to the current literature, the most basic integration methods of hybrid energy systems can be divided into two categories. One is series integration, the other is parallel integration. In the series integration type, the two energy sources do not work independently, but are strongly coupled in the same device and work together. As shown in Figure 1, based on gas steam combined cycle, solar collector is used to heat the outlet water of high-pressure economizer. In this system, the solar collector plays a role like the steam drum of the heat recovery steam generator in the gas steam combined cycle. The two energies converge at the inlet of the superheater of the heat recovery steam generator, and then jointly drive the steam turbine to do work (Antonanzas et al., 2014). In the parallel integration type, the various energy sources are less coupled during the energy conversion process, but only converge before being supplied to the user, as shown in Figure 2. The integration structure of the former is more complicated with stronger coupling, so the current research mainly focuses on the integration mechanism and performance analysis. Although the integration structure of the latter is relatively simple, it has many types of units and complex combinations, which makes the integration optimization more difficult and becomes the focus of current research.
2.1 Series integration
In hybrid energy systems, the integration of solar energy and natural gas is the most common. In addition to the integrated form shown in Figure 1, Solar energy is also used for the synthesis and decarbonization of gaseous fuels (Wei et al., 2011). In this system, natural gas reacts with water vapor under the high temperature heating of solar energy to generate H2 and CO2, and the H2-rich fuel can be obtained by removing CO2. According to the literature, not only 92% CO2 can be removed, but also the power generation efficiency of the gas turbine reaches 39.2%, which is 7.9% higher than the reference system. Similarly, (Augusto et al., 2013) also uses solar energy for the synthesis of gaseous fuel, and then the synthesis gas is mixed with natural gas and then enters the gas turbine to be burned and do work. This study shows that when all syngas is used as fuel, about 20% of natural gas can be saved, which is quite close to the study by Wei et al. (2011) Solar and gas turbines are more closely integrated in Felsmann et al. (2015). Part of the air at the outlet of the compressor is heated by solar energy, mixed with the high-temperature flue gas at the outlet of the combustion chamber, and then enters the turbine to do work. In another proposed scheme, the solar collector directly replaced the function of the combustion chamber. All air at the compressor outlet is heated by solar energy to high temperature air and then goes directly to the turbine to do work. This scheme actually goes beyond the combination of solar energy and natural gas and can be classified as a pure Solar System. Another way of integrating solar energy into gas turbines is given in Soltani et al. (2014). Part of the air at the outlet of the regenerator is heated by the solar collector and then enters the combustion chamber, and the solar collector plays the role of secondary heating of the air here. In addition to pure power generation systems, solar energy is also often introduced into cogeneration or combined cooling, heating and power systems. For example, Yagoub et al. (2006) studies the system performance of solar energy integrated with a gas boiler and turbine type cogeneration unit. In this study, solar energy was used to heat the exhaust flue gas of the boiler, and then combined with the high temperature flue gas from the combustion chamber to enter the boiler to heat the working fluid.
It can be seen from the above research that the series integration of solar and natural gas distributed energy systems is very diverse. Solar energy can penetrate from the fuel end of the natural gas distributed energy system to the waste heat recovery end. In contrast, the series integration of wind energy, geothermal energy, etc. with the natural gas distributed energy system is much less, and its integration is mainly at the waste heat recovery end. Parallel integration is a relatively common way to realize the complementary utilization of these energies.
2.2 Parallel integration
Compared with the series integration method, the parallel integration method is much more flexible. Through parallel integration, Multiple energy sources can work together on the same platform to meet the user’s cooling, heating and power load needs. Kalantar and Mousavi G (2010) integrates 195 kW wind turbine, 85 kW solar power generation, 230 kW micro gas turbine and 2.14 kAh lead-acid battery and realizes the capacity optimization and operation control of the system. A similar study was conducted in Mousavi G (2012). In this study, a 315 kW wind turbine, a 175 kW tidal turbine, a 290 kW microturbine, and a 3.27 kAh lead-acid battery are operated together, and the capacity optimization and operation control methods of the system are studied. The introduction of renewable energy can significantly reduce greenhouse gas emissions from natural gas energy systems. This is fully reflected in the research in Braslavsky et al. (2015). In this study, DER-CAM tool was used to establish an economic optimization model for the integrated system, which includes natural gas combined cooling, heating and power plant, photovoltaic power generation, solar collectors, etc., and the minimum cost and minimum CO2 emissions are used as the objective function. The results show that the combined natural gas supply system alone can reduce CO2 emissions by 8.5% and 29.6% per year, respectively. After integrating solar energy, although the overall energy cost increases, the CO2 emission reduction rate can reach 72%.
In addition to the above classification, it can also be classified according to the form of energy contained in the hybrid energy system. Such as solar-coal hybrid energy system (Yongpin et al., 2016; Yinghong et al., 2008), PV-wind-Battery hybrid energy system (Garima et al., 2017), PV-Wind-Diesel-Battery hybrid system (Mohammad et al., 2017), wind-diesel system with compressed air storage (Ibrahim et al., 2011). In the comprehensive utilization of multiple energy sources, it can be further subdivided according to whether each energy source is equal or has priority. Such as the solar-assisted coal-fired power generation system based on coal-fired power generation (Yongpin et al., 2016), solar thermal integration in combined cycle gas turbines (Antonanzas et al., 2014), and hybrid energy system with natural gas as main fuel (Hossein et al., 2017).
3 Configuration optimization architecture of HE-RES
The optimal configuration of a system means that the system has the best combination of equipment and capacity, which can optimize the overall performance of the system by optimizing operation under the expected load demand. Therefore, the configuration optimization of the hybrid energy system needs to solve two problems at the same time: one is the configuration problem, i.e., to determine the type and capacity of each device in the system; the other is the operation problem, i.e., to determine the optimal operation strategy of the system under the expected load demand. These two issues are not independent but affect each other (Ryohei et al., 2015). According to the way to deal with these two problems, the existing literature can be divided into two architecture: centralized architecture and layered architecture.
3.1 Centralized architecture
The centralized architecture refers to the unified construction and solution of the model to obtain the equipment type and size and the optimal operation strategy, as shown in Figure 3
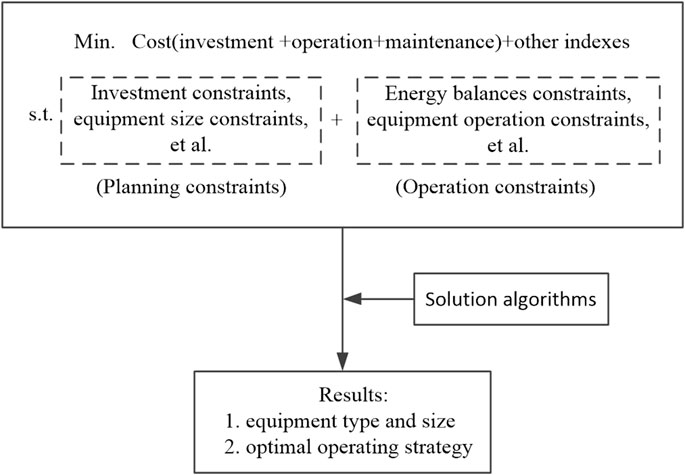
FIGURE 3. Load response curves under two different equipment capacity configurations at system level (Yuxuan et al., 2022)
Based on the centralized architecture, many studies have been carried out on hybrid energy systems. Yi et al. (2022) proposed a mixed integer nonlinear programming (MINLP) model and solved it using GAMS/DICOPT to obtain the optimal configuration of a solar-assisted natural gas distributed energy system with energy storage. Jianli et al. (2021) established a mixed integer programming model for a gas-wind-light-hydrogen integrated energy system with the goal of minimizing the total annual cost and carbon emissions, etc. The advantage of the centralized architecture is that he construction of the model can be closer to the original appearance of the problem, and there is no need to worry about the loss of information that may be caused by the separation of the planning optimization model and the operation optimization model in the layered architecture. Therefore, with the support of efficient solving algorithms, the Centralized architecture is more likely to obtain theoretical optimal values than the layered architecture. However, as the complexity of the problem increases, the shortcomings of the overall architecture will become more and more obvious. The model obtained based on this method may be difficult to solve, and the accuracy of the model description and the solution efficiency are not easy to balance, which eventually leads to the problem of the quality of the optimization results.
3.2 Layered architecture
The layered architecture refers to dividing the configuration optimization problem into multiple problems to model and solve separately, as shown in Figure 4
In the layered architecture, the configuration problem is divided into the planning problem layer and the operation problem layer. These two layers can be modeled and solved separately, but the coupling problem between the two layers needs to be properly handled. The layered architecture has greater flexibility than the centralized architecture, and the choice of modeling and solution methods can be more free and targeted. Therefore, many researches have been carried out based on this architecture in recent years. Aiming at an isolated grid energy system composed of hydropower stations, hydrogen production equipment, hydrogen storage devices and fuel cells, a configuration optimization model based on a two-layer structure is designed in Huang et al. (2021), in which the upper layer aims to minimize the investment cost, and the lower layer aim for minimal operating costs. The Asynchronous Advantage Actor-Critic (A3C) reinforcement learning algorithm and Gurobi are used to solve the model. To solve the problem of optimal configuration of park integrated energy system under the influence of uncertainty, Min et al. (2022) proposes a two-stage robust optimization model based on a dynamic programming strategy. Wang et al. (2019a) presents a two-stage optimal configuration method. The first stage determines the capacity size, equipment selection and the number of units; the second stage considers the operation constraints, verifies and compares the reliability and economy of the configuration scheme, so as to obtain the optimal capacity configuration scheme.
For multi-objective optimization, more layers can be used. Since multi-objective optimization problems are often handled with a two-layer structure (Xu et al., 2020a), in the first layer, the multi-objective optimization results are obtained; in the second layer, the weight coefficients of each objective are determined. Therefore, if the multi-objective optimization results are computed in the first layer using the layered architecture, the entire optimization problem actually adopts a three-layer structure. Problems at different levels can be solved by different algorithms, which provides more possibilities for the combination of more algorithms to solve the problem. It is worth noting that the more layers are divided, the easier the optimization problem may be to solve, but the more difficult it is to obtain the theoretical optimal value.
4 Model description and construction of RE-HES
4.1 Model construction method
The construction of an optimization model is one of the keys whether a centralized architecture or a layered architecture is adopted. In the process of model establishment, in addition to accurately describing various influencing factors of the system, the logic of model expression and the convenience of solution should also be considered. There are many structural forms of the hybrid energy system, and the operation strategy is also very flexible, especially in the case of the coupling of multiple energy flows, the system configuration optimization results have many possibilities. This brings great difficulties to the efficient construction of the model.
An efficient solution is to use a superstructure-based method (Tetsuya and Ryohei, 2014), where all possible or mainly possible structures are put into an overall structure, resulting in more structural forms than are required for the actual system. Then describe the characteristics of each device, the logic between each device, the relationship between each energy flow, etc., so as to construct models such as mixed integer nonlinear programming (Juan et al., 2016; Zheng et al., 2022) and mixed integer linear programming (Yun et al., 2015a). The advantage of this method is that it can make the logical description of the optimization model clearer and reduce the impact of meaningless structures. Based on this method, Yun et al. (2015b) establishes a mixed-integer linear programming model of the coupled system of distributed energy and energy distribution network and realizes the optimal annual total cost of the system. It should be noted that when the system is more complex, the scale of the superstructure should be properly defined (Philip et al., 2012). The size of the scale affects the optimization results and efficiency of the solution. When the scale is small, it is easier to solve, but the optimal value may have been excluded from the structure. When the scale is large, although the chance of obtaining the optimal solution is greater, it may also introduce many meaningless structures, making the model difficult to solve.
4.2 Performance evaluation indexes of RE-HES
The performance evaluation index of the hybrid energy system is the basis for constructing the configuration optimization objective function, which has a significant impact on the configuration optimization results. Among the existing index, the three most commonly used indexes are economic, energy and environment related indexes and their combination. In recent years, with the increase in the proportion of renewable energy in the system, it is important to improve the reliability and flexibility of the system, and the related performance evaluation index has therefore received more attention.
4.2.1 Economic, energy, and environment related indexes
4.2.1.1 Economic
In most configuration optimization studies, the influence of economic index is considered when constructing the objective function. For example, Askarzadeh (2013) established a configuration optimization model for photovoltaic, wind, and battery hybrid energy systems with the annual total cost as the optimization goal. Ryohei et al. (2015) optimizes the number of photovoltaic panels and wind turbines in the wind-solar hybrid system with the lowest cost as the optimization goal. Yi et al. (2022) takes the minimum annual cost as the optimization goal optimizes and optimizes the configuration of a solar-assisted natural gas distributed energy system (DES) with energy storage. In these literatures, it can be found that the economic evaluation of the system is basically carried out with the lowest total annual cost. This index mainly includes two parts, one is fixed cost, such as equipment investment cost. The other is variable cost, such as fuel cost. Since the fuel cost of photovoltaic, wind power and other renewable energy utilization devices is basically negligible (except for biomass), and the investment cost of the equipment has been decreasing in these years, the configuration optimization results of RE-HES based on economic index tend to increase the proportion of the renewable energy consumption.
4.2.1.2 Energy
Energy-related indexes can be divided into two categories, one based on the first law of thermodynamics and the other based on the second law of thermodynamics.
1) Based on the first law of thermodynamics
The performance evaluation index based on the first law of thermodynamics mainly considers how much energy is lost in the process of energy utilization. In order to solve the problem that the traditional system cycle thermal efficiency and other evaluation indexes are difficult to scientifically evaluate the performance of the hybrid energy system, Yawen et al. (2014) puts forward the relative efficiency evaluation index of solar complementary power generation, which provides a new idea for the integrated design and optimization of solar complementary power generation. Yixun et al. (2017) considers the multi-energy flow characteristics of the integrated energy system and the influence of renewable energy access, and proposes a comprehensive energy utilization index suitable for multi-energy collaborative remote energy efficiency evaluation, which reflects the utilization level of renewable energy and non-renewable energy in the park. Like energy efficiency, primary energy consumption is often used as a performance evaluation index for configuration optimization (Xiaomin et al., 2017). No matter which index form is adopted, the index based on the first law of thermodynamics fails to take into account the difference in energy quality, which may lead to insufficient improvement of the performance of the system.
2) Based on the second law of thermodynamics
Compared with the single-energy energy system, the energy conversion structure of RE-HES is more complicated and the energy form of RE-HES is more diversified, which means, not only the amount of energy but also the energy grade needs to be considered. Therefore, many scholars have conducted research based on the second law of thermodynamics. Baghernejad and Yaghoubi (2010) studies the integrated system of solar energy and gas-steam combined cycle with exergy analysis method and obtains the main exergy loss components of the research object. Another paper further established the exergy economic optimization model of this type of system (Baghernejad and Yaghoubi, 2011), and used genetic algorithm to optimize it, which reduced the power generation cost of steam turbine and gas turbine by 7.1% and 1.17%, respectively. Calderón et al. (2011), Sayem and Ibrahim (2014) both apply the exergy analysis method to the wind-solar hybrid power generation system. In addition to the necessary exergy analysis, the latter literature also conducts research from the perspective of energy and exergy economics to more fully demonstrate the performance of such systems. Integrating renewable energy with conventional coal-fired systems is a type of system that has received a lot of attention in recent years (Yinghong et al., 2008; Xuemin et al., 2015; Rongrong et al., 2016). For a more comprehensive analysis of the performance of the hybrid energy systems, (Suresh et al., 2010) even discusses the technical and economic feasibility of such systems from the perspective of 4E (Energy, Exergy, Environment, and Economy).
4.2.1.3 Environment
Environmental indexes have received great attention in recent years. Many studies have proved that the hybrid energy system has advantages in increasing the penetration rate of renewable energy and reducing carbon emissions in the social production process. Xue et al. (2017) proposes a comprehensive energy utilization index by adding the energy non-renewable coefficient to the evaluation index of the multi-park hybrid energy system, which can reflect the impact of renewable energy in primary energy, and through case calculation and comparative analysis, It verifies the consistency between the comprehensive energy utilization index and the economic index, and can improve the penetration rate of renewable energy in the hybrid energy system. In order to improve the renewable energy penetration rate of the system, in addition to directly establishing the optimization index considering the non-renewable coefficient of energy, many scholars try to add carbon trading mechanism to the hybrid energy system. Wei et al. (2016) proposed to introduce a carbon trading mechanism into the hybrid energy system of electricity-gas interconnection, taking the minimum sum of carbon trading cost and power generation energy cost as the objective function of the system, and combining the carbon trading mechanism with the collection of carbon taxes and other factors. Mechanisms were compared, and it was verified that the carbon trading mechanism can reduce the cost of power generation more effectively and has a good development prospect. Qu et al. (2018) verifies the feasibility of the carbon trading mechanism in the multi-regional hybrid energy system and establishes the optimization goal of the multi-regional hybrid energy system considering the carbon transaction cost. The results show that under the background of low coal prices in my country, the multi-regional hybrid energy system can make each regional energy system actively use low-emission technologies such as cogeneration and other clean energy through the carbon trading mechanism game. Wang et al. (2019b) further considers the impact of the combination of LCA energy chain and carbon trading mechanism on the optimization of the hybrid energy system, and solves the difficulty in calculating the total carbon emissions caused by the mutual conversion of various energy sources in the hybrid energy system. This ensures the effective operation of the carbon trading mechanism. In addition, studies have also considered the impact of carbon taxes (Wang et al., 2021) and renewable energy subsidies (Liu et al., 2021) on hybrid energy systems. Shebaz et al. (2021) conducts a study on the optimal configuration of solar and wind hybrid renewable energy systems with and without energy storage for environmental and social criteria.
4.2.2 Reliability and flexibility
System reliability and flexibility become important due to the presence of large amounts of renewable energy.
4.2.2.1 Reliability
In the practical application of the hybrid energy system, not only its environmental protection performance needs to be better than that of the traditional energy system, but also its reliability must be guaranteed. This is one of the keys to the large-scale commercial application of hybrid energy systems. By establishing the reliability evaluation index of the hybrid energy system to evaluate the reliability of each equipment, it is helpful to find the deficiencies of the system and improve them effectively (Li et al., 2021). Some studies do not consider the influence of randomness and quantify the influence of the failure of each device on the reliability of the hybrid energy system by establishing the fault correlation matrix of each device (Zhao et al., 2022). However, affected by the characteristics of renewable energy, the operating state of each device has a certain randomness. Therefore, some scholars have carried out reliability assessment based on probabilistic operating state modeling for hybrid energy systems. Usually, the Monte Carlo method is applied to reliability evaluation, and a new reliability evaluation index is proposed (Cao et al., 2022; Fang et al., 2022). Optimizing based on reliability alone may result in system redundancy. Therefore, the reliability index needs to be used together with other indexes such as economics. Yin et al. (2021) proposed a two-layer optimization method based on the reliability objective and the economic objective. The minimum outage capacity is converted into the minimum outage penalty cost, which is the reliability cost. The reliability cost and the economic cost are integrated to a new optimization target to realize the comprehensive optimization of the system reliability and economy.
4.2.2.2 Flexibility
Due to the stochastic of renewable energy, the hybrid energy system needs to have higher flexibility than the traditional energy system to ensure the energy supply and demand balance of the system. The higher the flexibility of a hybrid energy system, the greater its ability to utilize renewable energy (Liu et al., 2019). Wang et al. (2022a) combines the power grid with the hydrogen grid by adding electricity-to-hydrogen equipment in the hybrid energy system and improve the flexibility of the system, helping the grid to absorb the uncertainty of renewable energy generation, smoothing the output power of renewable energy. Cui et al. (2022) cited the flexibility index of air source heat pump with waste heat recovery considering dynamic characteristics in integrated energy system. Hao et al. (2022) further studies the impact of energy storage devices on the flexibility of the hybrid energy system. In addition to the influence of equipment characteristics in the hybrid energy system on the flexibility index, the variability of the load side also has an impact on the flexibility index of the hybrid energy system. Chen et al. (2021), Zhang et al. (2021) respectively study the flexibility of hybrid energy systems from the perspective of load elasticity and load excitation response. The former’s flexibility takes into account the maximum variation range of the load and is a definite value. The latter’s flexibility is based on the value obtained from the game of incentives and market transaction mechanisms.
4.2.3 Multiple indexes
The configuration and development of hybrid energy systems are affected by various factors such as technology, cost, and policy. Therefore, the performance index of the hybrid energy system usually adopts the index based on thermodynamics, economy, environmental protection and so on (Wang et al., 2022b). In Xinjie et al. (2021) research, the synthesis of three indicators of energy efficiency, cost rate and emissions are considered for a novel hybrid solid oxide fuel cell (SOFC)-solar hybrid CCHP residential system. Arnette and Zobel (2012) considers both greenhouse gas emissions and annual operating costs. The minimum outage capacity in Yin et al. (2021) is converted into the minimum outage penalty cost, which is a combination of reliability indexes and economic indexes. The low-carbon indexes in Wei et al. (2016) are also represented by carbon transaction costs, which are ultimately reflected in economic goals. Zhong et al. (2021) builds a model that includes six evaluation indexes: energy saving rate, energy loss, new energy proportion, new energy consumption rate, and operation and maintenance cost. The system can be more comprehensively evaluated and optimized through multiple indexes, but how to reasonably determine the weight of each index is still one of the basic challenges of current multi-objective configuration optimization.
4.3 Considering uncertainty and dynamic characteristics in optimization
The system characterization has a significant impact on the configuration optimization model and its optimization results. Most of the existing configuration optimization studies ignore the uncertainty and dynamic characteristics of the system when describing the system. For hybrid systems that do not contain renewable energy, this treatment may not have a noticeable effect on the results. However, for systems with renewable energy sources, ignoring uncertainties and dynamics may lead to large deviations in system configuration results. This section is mainly divided into two aspects: considering uncertainty and considering dynamic characteristics.
4.3.1 Considering uncertainty
For the treatment of uncertainty, the commonly methods used are stochastic optimization methods and robust optimization methods.
4.3.1.1 Stochastic optimization methods
The main idea of the stochastic optimization method can be summarized as: based on a large amount of historical data, typical scenes are obtained by clustering, then the probability of occurrence of each scene is calculated, and finally the mathematical expectation model is solved. Stochastic optimization is mainly based on the probability prediction information of uncertain variables. The uncertain variables usually obey a certain probability density distribution, such as the wind speed obeys the Weibull distribution (Villanueva et al., 2011), and the forecast errors of wind power and photovoltaic output obey the normal distribution (Lange, 2005, Abedi et al., 2011). Using stochastic optimization method, (Yi et al., 2022) established an optimization model for the hybrid energy system and the uncertainty of cooling, heating and power load and the stochastic of renewable energy are described by Monte Carlo simulation method. Amirhossein et al. (2017) established a two-stage stochastic programming model for hybrid energy system configuration based on typical scenarios obtained by Monte Carlo sampling and K-Means clustering.
4.3.1.2 Robust optimization methods
Unlike stochastic optimization methods, which have higher requirements on historical data, robust optimization methods have much lower requirements on it. The method only needs to determine a reasonable fluctuation range of random variables to ensure the feasibility of configuring results within this range (Cao et al., 2018, Zugno and Conejo, 2015). Robust optimization methods can be summarized as traditional robust optimization methods and distributed robust optimization methods.
1) Traditional robust optimization method
The traditional robust optimization method is mainly to find the worst scenario in the uncertainty interval and calculate the optimal solution in this scenario. According to this idea, (Chen et al., 2019) established a robust optimization model considering the uncertainty factors of load demand and used the Benders algorithm to solve it. The optimization results obtained in the worst case are very conservative. To reduce the conservativeness of the method, a two-stage robust model is proposed. Based on two-stage robust method, Zeng and Zhao (2013) proposes a column-and-constraint generation (C&CG) algorithm based on the Benders decomposition method. By continuously introducing optimal cut sets and feasible constraints in the loop, it can quickly solve two-stage robust optimization problems. To further improve the robustness, Yan et al. (2021) uses box uncertainty sets, ellipsoid uncertainty sets, and convex uncertainty sets to describe the distribution of uncertain factors and establishes a two-stage robust optimal configuration model.
2) Distribution robust optimization method
To achieve the balance of performance such as economy and conservatism, distributed robust optimization methods have been proposed and developed in recent years. The distribution-robust optimization (DRO) method is mainly to find the decision results under the worst probability distribution of uncertain parameters (Wang et al., 2018). It is mainly divided into two methods: probability density and distance information. DRO methods based on probability density mainly include three forms: multi-discrete scene (He et al., 2019), Wasserstein distance (Zhu et al., 2019) and KL divergence (Chen et al., 2018). DRO methods based on range information mainly include two forms: deterministic range (Chen et al., 2016) and indeterminate range (Alvarado et al., 2019; Lu et al., 2018a). The mathematical description of the DRO method based on distance information is more accurate, but the conversion and solution process are complicated. The DRO method based on probability density has the advantages of simple mathematical logic and easy solution, so it is currently used more in hybrid energy systems.
4.3.2 Considering dynamic characteristics
To cope with the uncertain influence of load demand and renewable energy output, the dynamic tracking performance of the system needs to be improved (Chen and Pan, 2021). Existing studies are mainly based on the steady-state characteristics of the system, and the improvement of system dynamic tracking performance by advanced control methods is limited (Blaud et al., 2020). Different device configurations result in different dynamic tracking performance, as shown in Figure 5. Therefore, ignoring the dynamic tracking performance in the capacity configuration may result in the inability of the hybrid energy system to track the load demand in time.
For scheduling optimization problems, some studies have considered the influence of dynamic characteristics, mainly the influence of thermal network and thermal inertia of buildings (Wei et al., 2017; Yang et al., 2020). However, the impact of considering dynamic properties in configuration optimization has only recently received attention. Existing studies have shown that the system exhibits more advantageous performance after adopting an optimized configuration that considers the dynamic characteristics (Yuxuan et al., 2022; Bingle and Xiao, 2022). With the increasing proportion of renewable energy in the system and the in-depth understanding of the dynamic characteristics of the hybrid energy system, considering the dynamic characteristics in the configuration and scheduling optimization are expected to bring more benefits to the system.
5 Model solution algorithms for RE-HES’s configuration
The model solution algorithm plays an important role in the configuration optimization of the hybrid energy system. At present, a variety of solution algorithms have been developed, which can be mainly divided into two basic categories: classical optimization algorithms and intelligent optimization algorithms. There are also some studies using commercial solver to solve optimization models. Such Gurobi (Jiang et al., 2022) and CPLEX (Zhou et al., 2022), which are widely used due to their superior performance. Some other solvers such as MOSEK (Zhang et al., 2018), XPRESS (Andrychowicz and Olek, 2016), LINGO (Xu et al., 2020b), INTLINPROG (Ibrahimi et al., 2019) are also commonly found in the literature. However, the core algorithms of these solvers are also based on classical algorithms or intelligent algorithms or hybrid algorithms of classic and intelligent. This paper mainly focuses on these two basic algorithms.
5.1 Classical optimization algorithms
The configuration optimization problem of RE-HES is essentially a nonlinear programming problem. When considering the discrete characteristics of the problem, such as discontinuity of equipment size, start-stop logic of equipment, etc., the problem becomes a nonlinear mixed integer programming problem. In practical applications, for the convenience of solving, nonlinear programming (or mixed integer nonlinear programming) problems are often transformed into linear programming (Peng et al., 2016) [or mixed integer linear programming (Jiang et al., 2022; Mazaheri et al., 2021)] problems through simplification or linearization. When using traditional solving methods, different solving algorithms are used for different models. For example, sequential quadratic programming methods can be used to solve nonlinear programming problems (Ahmad, 2021; Lu et al., 2018b); branch and bound methods and cutting plane methods can be used to solve mixed integer programming problems (Yi et al., 2022), etc.
Different from the generality pursued by some intelligent methods, traditional methods are generally more targeted to the problem, and the algorithm can often be improved according to the characteristics of the problem (Ryohei et al., 2015). For example, Lahdelma and Hakonen (2003) establishes a linear programming model for the cogeneration problem and proposes an improved simplex method according to the characteristics of the model, which achieves good results. Then, a TCS algorithm suitable for solving the linear programming model of the trigeneration system is proposed Rong and Lahdelma (2005). The enumeration method may also be an effective method when the model complexity is low or the value range of the decision variable is small (Shaneb et al., 2012; Sanaye et al., 2008).
5.2 Intelligent optimization techniques
The rapid development of intelligent optimization techniques in recent years has been clearly reflected in the comprehensive optimization of hybrid energy systems. Various novel and hybrid algorithms are selected and proposed to solve complex integrated optimization problems of hybrid energy systems. Paliwal et al. (2014) applies particle swarm optimization (PSO) to the study of capacity allocation optimization of wind-solar-diesel-storage hybrid energy systems. Mei et al. (2022) uses a multi-objective particle swarm algorithm to optimize the regional hybrid energy system. Arabli et al. (2013) studies the integrated optimization problem of the heating ventilation system (HVAC) powered by wind-solar storage and uses the genetic algorithm (GA)-based two-point estimation method to optimize the model. Chen et al. (2020) established an exergy analysis model for the hybrid energy system using an adaptive genetic algorithm and verified the validity of the model for the Bali hybrid energy system model. There are also some other new algorithms that can also be used to solve the configuration optimization problem of hybrid energy systems, such as ant colony algorithm (Abdolvahhab and Ehsan, 2015), big bang–big crunch algorithm (Saeedeh and Shirzad, 2016) and bee swarm algorithm (Akbar and Alireza, 2014). Due to the limitations of a single algorithm, some studies use a combination of multiple algorithms to improve the optimization performance. Shilaja (2021) combines the chaotic particle swarm optimizer and the gravitational search algorithm to deal with the power flow calculation problem in the hybrid energy system. Askarzadeh (2013) combines chaos search (CS), harmony search (HS) and simulated annealing (SA), and proposes a new discrete chaotic harmony-based simulated annealing method (DCHSSA) to solve the configuration optimization model of hybrid energy systems.
At present, the effective combination of intelligent algorithms and configuration optimization models is still insufficient. This is a general problem that deserves attention. Compared with the traditional method, the intelligent method does have better generality, but the excessive pursuit of generality will inevitably lead to the difficulty of improving the algorithm in combination with the specific characteristics of the model. To solve this problem, in addition to improving the intelligent algorithm itself, it is also an effective method to combine the intelligent algorithm with the traditional method. How to find a balance between the generality and individuality of the algorithm and realize the fast and high-quality solution of the configuration optimization model is a topic worthy of continuous discussion.
6 Conclusion
The integration of RE-HES can be summarized into two structures, series and parallel, among which the parallel structure is the focus of attention at present. A lot of research has been carried out on performance evaluation, optimization model construction and optimization algorithm application under parallel structure. Based on the configuration optimization problem processing framework, existing optimization techniques can be divided into holistic frameworks and hierarchical frameworks. The performance evaluation index is the basis for the construction of the optimization objective function. In addition to common indexes such as economy, energy consumption, and environmental protection, related indexes such as reliability, flexibility and dynamic characteristics also received attention. The construction of the optimization model is closely related to the system and operational requirements. Due to the introduction of renewable energy, uncertainty and dynamic characteristics cannot be ignored in configuration optimization. Optimizing the dynamic characteristics of the system in the capacity allocation stage may effectively improve the system’s ability to cope with renewable energy and load uncertainty. But this also brings new challenges to the solution of the optimization model. According to the way to deal with these two problems, the existing literature can be divided into two architecture: centralized architecture and layered architecture. Based on these two architectures, a lot of work has been carried on model building and model solving, and technologies such as superstructure methods and intelligent algorithms have been effectively applied in RE-HES.
Author contributions
JZ: Conceptualization, Methodology, Literature arrangement and review, Writing original draft. HW: literature arrangement and review.
Funding
This work was supported by National Natural Science Foundation of China under Grant 51936003 and National Key R&D Program of China under Grant 2018YFB1502900 and the Fundamental Research Funds for the Central Universities 2242021k10010.
Conflict of interest
The authors declare that the research was conducted in the absence of any commercial or financial relationships that could be construed as a potential conflict of interest.
Publisher’s note
All claims expressed in this article are solely those of the authors and do not necessarily represent those of their affiliated organizations, or those of the publisher, the editors and the reviewers. Any product that may be evaluated in this article, or claim that may be made by its manufacturer, is not guaranteed or endorsed by the publisher.
References
Abba, L. B., and Chee, W. T. (2019). A review on stand-alone photovoltaic-wind energy system with fuel cell: System optimization and energy management strategy. J. Clean. Prod. 221, 73–88. doi:10.1016/j.jclepro.2019.02.228
Abdolvahhab, F., and Ehsan, K. (2015). Size optimization for hybrid photovoltaic–wind energy system using ant colony optimization for continuous domains based integer programming. Appl. Soft Comput. 31, 196–209. doi:10.1016/j.asoc.2015.02.047
Abedi, S., Riahy, G. H., Hosseinian, S. H., and Alimardani, A. (2011). Risk-Constrained unit commitment of power system incorporating PV and wind farms. Isrn Renew. Energy 2011 (1)–8. doi:10.5402/2011/309496
Ahmad, R. J. (2021). Economic dispatch in grid-connected and heat network-connected CHP microgrids with storage systems and responsive loads considering reliability and uncertainties. Sustain. Cities Soc. 73, 103101. doi:10.1016/j.scs.2021.103101
Akbar, M., and Alireza, A. (2014). Artificial bee swarm optimization for optimum sizing of a stand-alone PV/WT/FC hybrid system considering LPSP concept[J]. Sol. Energy 107, 227–235. doi:10.1016/j.solener.2014.05.016
Alvarado, D., Moreira, A., Moreno, R., and Strbac, G. (2019). Transmission network investment with distributed energy resources and distributionally robust security. IEEE Trans. Power Syst. 34 (6), 5157–5168. doi:10.1109/tpwrs.2018.2867226
Amirhossein, D., Behnam, M., Mehdi, A., and Sajjad, T. (2017). Optimal stochastic design of wind integrated energy hub[J]. IEEE Trans. Industrial Inf. 13 (5), 2379–2388. doi:10.1109/TII.2017.2664101
Andrychowicz, M., and Olek, B. (2016). "Optimal structure of the RES in distribution systems," in International Conference on the European Energy Market, EEM 13th International Conference on the European Energy Market, Portugal, June 6-9, 2016 (IEEE). doi:10.1109/EEM.2016.752133
Antonanzas, J., Jimenez, E., Blanco, J., and Antonanzas-Torres, F. (2014). Potential solar thermal integration in Spanish combined cycle gas turbines. Renew. Sustain. Energy Rev. 37, 36–46. doi:10.1016/j.rser.2014.05.006
Arabli, S., Ghofrani, M., Etezadi-Amoli, M., Fadali, S., and Baghzouz, Y. (2013). Genetic-algorithm-based optimization approach for energy management. IEEE Trans. Power Deliv. 28, 162–170. doi:10.1109/TPWRD.2012.2219598
Arnette, A., and Zobel, C. W. (2012). An optimization model for regional renewable energy development. Renew. Sustain. Energy Rev. 16 (7), 4606–4615. doi:10.1016/j.rser.2012.04.014
Askarzadeh, A. (2013). A discrete chaotic harmony search-based simulated annealing algorithm for optimum design of PV/wind hybrid system. Sol. Energy 97, 93–101. doi:10.1016/j.solener.2013.08.014
Augusto, B., Marco, P., and Cesare, S. (2013). Solar steam reforming of natural gas integrated with a gas turbine power plant. Sol. energy 96, 46–55. doi:10.1016/j.solener.2013.06.030
Baghernejad, A., and Yaghoubi, M. (2011). Exergoeconomic analysis and optimization of an integrated solar combined cycle system (ISCCS) using genetic algorithm. Energy Convers. Manag. 52 (5), 2193–2203. doi:10.1016/j.enconman.2010.12.019
Baghernejad, A., and Yaghoubi, M. (2010). Exergy analysis of an integrated solar combined cycle system. Renew. Energy 35 (10), 2157–2164. doi:10.1016/j.renene.2010.02.021
Barun, K. D., and Mahmudul, H. (2021). Optimal sizing of a stand-alone hybrid system for electric and thermal loads using excess energy and waste heat. Energy 214, 119036. doi:10.1016/j.energy.2020.119036
Bingle, Z., and Xiao, W. (2022). Integrated capacity configuration and control optimization of off-grid multiple energy system for transient performance improvement. Appl. Energy 311, 118638. doi:10.1016/j.apenergy.2022.118638
Blaud, P. C., Haurant, P., Claveau, F., Lacarrière, B., Chevrel, P., and Mouraud, A. (2020). Modelling and control of multi-energy systems through multi-prosumer node and economic model predictive control. Int. J. Electr. Power & Energy Syst. 118, 105778. doi:10.1016/j.ijepes.2019.105778
Braslavsky, J. H., Wall, J. R., and Reedman, L. J. (2015). Optimal distributed energy resources and the cost of reduced greenhouse gas emissions in a large retail shopping centre. Appl. Energy 155, 120–130. doi:10.1016/j.apenergy.2015.05.085
Caishan, G., Fengji, L., Zexiang, C., Zhao, Y. D., and Rui, Z. (2021). Integrated planning of internet data centers and battery energy storage systems in smart grids. Appl. Energy 281, 116093. doi:10.1016/j.apenergy.2020.116093
Calderón, M., Calderón, A. J., Ramiro, A., Gonzalez, J. F., and Gonzalez, I. (2011). Evaluation of a hybrid photovoltaic-wind system with hydrogen storage performance using exergy analysis. Int. J. Hydrogen Energy 36 (10), 5751–5762. doi:10.1016/j.ijhydene.2011.02.055
Cao, M., Shao, C., Hu, B., Xie, K., Li, W., Peng, L., et al. (2022). Reliability assessment of integrated energy systems considering emergency dispatch based on dynamic optimal energy flow. IEEE Trans. Sustain. Energy 13 (1), 290–301. doi:10.1109/TSTE.2021.3109468
Cao, Y., Wei, W., Wang, J., Mei, S., Shafie-khah, M., and Catalao, J. P. S. (2018). Capacity planning of energy hub in multi-carrier energy networks: A data-driven robust stochastic programming approach. IEEE Trans. Sustain. Energy 11 (1), 3–14. doi:10.1109/TSTE.2018.2878230
Chen, C., Shen, X., Guo, Q., and Sun, H. (2019). Robust planning-operation co-optimization of energy hub considering precise model of batteries’ economic efficiency. Energy Procedia 158, 6496–6501. doi:10.1016/j.egypro.2019.01.111
Chen, H., Chen, S., Li, M., and Chen, J. (2020). Optimal operation of integrated energy system based on exergy analysis and adaptive genetic algorithm. IEEE Access 8, 158752–158764. doi:10.1109/ACCESS.2020.3018587
Chen, K., Chen, W., Lin, C., Zhang, S., Yu, D., Yang, Y., et al. (2021). “Integrated energy system day-ahead dispatch considering supply-side and demand-side flexibility,” in 5th IEEE Conference on Energy Internet and Energy System Integration: Energy Internet for Carbon Neutrality, EI2 2021 5th IEEE Conference on Energy Internet and Energy System Integration, Taiyuan, China, October 22, 2021 (IEEE). EI2 2021. doi:10.1109/EI252483.2021.9713552
Chen, K., and Pan, M. (2021). Operation optimization of combined cooling, heating, and power superstructure system for satisfying demand fluctuation. Energy 237, 121599. doi:10.1016/j.energy.2021.121599
Chen, Y., Guo, Q., Sun, H., Li, Z., Wu, W., and Li, Z. (2018). A distributionally robust optimization model for unit commitment based on kullback–leibler divergence. IEEE Trans. Power Syst. 33 (5), 5147–5160. doi:10.1109/tpwrs.2018.2797069
Chen, Y., Wei, W., Liu, F., and Mei, S. (2016). Distributionally robust hydro-thermal-wind economic dispatch. Appl. Energy 173 (1), 511–519. doi:10.1016/j.apenergy.2016.04.060
Cui, Y., Guo, F., Fu, X., Zhao, Y., and Han, C. (2022). Source-load coordinated optimal dispatch of integrated energy system based on conversion of energy supply and use to promote wind power accommodation. Dianwang Jishu/Power Syst. Technol. 46 (4), 1437–1446. doi:10.13335/j.1000-3673.pst.2021.0625
Fang, L., Xu, X.-Y., Xia, J., and Tarasiuk, T. (2022). Reliability assessment of the port power system based on integrated energy hybrid system. Bull. Pol. Acad. Sci. Tech. Sci. 70 (2). doi:10.24425/bpasts.2022.140372
Fatih, O. H., Ömer, N. G., and Mehmet, K. (2009). A novel hybrid (wind–photovoltaic) system sizing procedure[J]. Sol. Energy 83, 2019–2028. doi:10.1016/j.solener.2009.07.010
Felsmann, C., Heide, S., Gampe, U., and Freimark, M. (2015). Modeling and simulation of the dynamic operating behavior of a high solar share gas turbine system. J. Eng. Gas Turbines Power 137, 1–9. doi:10.1115/1.4028445
Garima, S., Prashant, B., Anand, S., and Kurup, D. (2017). Optimal sizing and location of PV, wind and battery storage for electrification to an island A case study of Kavaratti, Lakshadweep. J. Energy Storage 12, 78–86. doi:10.1016/j.est.2017.04.003
Hao, J., Gou, X., Wang, S., Chen, Q., and Gao, K. (2022). Dynamic modeling and flexibility analysis of an integrated electrical and thermal energy system with the heat PumpThermal storage. Front. Energy Res. 10. doi:10.3389/fenrg.2022.817503
He, S., Gao, H., Liu, J., Liu, Y., Wang, J., and Xiang, Y. (2019). Distributionally robust optimal DG allocation model considering flexible adjustment of demand response [J]. Proc. CSEE 39 (8), 2253–2264. doi:10.1109/PESGM40551.2019.8973569
Hossein, Y., Mohammad, H. G., and Alibakhsh, K. (2017). Multi-objective optimal component sizing of a hybrid ICE + PV-T driven CCHP microgrid. Appl. Therm. Eng. 122, 126–138. doi:10.1016/j.applthermaleng.2017.05.017
Howard, C., and Shengwei, W. (2019). Optimal design of data center cooling systems concerning multi-chiller system configuration and component selection for energy-efficient operation and maximized free-cooling. Renew. Energy 143, 1717–1731. doi:10.1016/j.renene.2019.05.127
Huang, Y., Shi, M., Wang, W., and Lin, H. (2021). A two-stage planning and optimization model for water - hydrogen integrated energy system with isolated grid. J. Clean. Prod. 313, 127889. doi:10.1016/j.jclepro.2021.127889
Ibrahim, H., Younes, R., Basbous, T., Ilinca, A., and Dimitrova, M. (2011). Optimization of diesel engine performances for a hybrid wind–diesel system with compressed air energy storage. Energy 36 (5), 3079–3091. doi:10.1016/j.energy.2011.02.053
Ibrahimi, A. M., Howlader, H. O. R., Danish, M. S. S., Shigenobu, R., Sediqi, M. M., and Senjyu, T. (2019). Optimal unit commitment with concentrated solar power and thermal energy storage in Afghanistan electrical system. Int. J. Emerg. Electr. Power Syst. 20 (3), 0264. doi:10.1515/ijeeps-2018-0264
Jiang, H., Zhou, H., Xie, X.-P., Yang, D.-S., and Zhao, Y. (2022). Optimal control of integrated energy system considering temperature comfort and carbon trade. Kongzhi Lilun Yu Yingyong/Control Theory Appl. 39 (3), 519–526. doi:10.7641/CTA.2021.10665
Jianli, Z., Yunna, W., Zhiming, Z., Chuanbo, X., Yiming, K., and Jianwei, G. (2021). Modeling and configuration optimization of the natural gas-wind-photovoltaic-hydrogen integrated energy system: A novel deviation satisfaction strategy. Energy Convers. Manag. 243, 114340. doi:10.1016/j.enconman.2021.114340
Juan, I. M., Ana, M. A., Nicolás, J. S., Miguel, C. M., and Sergio, F. M. (2016). A discrete and continuous mathematical model for the optimal synthesis and design of dual pressure heat recovery steam generators coupled to two steam turbines. Energy 103, 807–823. doi:10.1016/j.energy.2016.02.129
Junjie, X., Yonghui, S., Jianxi, W., Zhipeng, L., Zhou, X., and Zhai, S. (2022). Multi-stage equipment optimal configuration of park-level integrated energy system considering flexible loads. Int. J. Electr. Power & Energy Syst. 140, 108050. doi:10.1016/j.ijepes.2022.108050
Kalantar, M., and Mousavi G, S. M. (2010). Dynamic behavior of a stand-alone hybrid power generation system of wind turbine microturbine, solar array and battery storage. Appl. Energy 87 (10), 3051–3064. doi:10.1016/j.apenergy.2010.02.019
Lahdelma, R., and Hakonen, H. (2003). An efficient linear programming algorithm for combined heat and power production. Eur. J. Oper. Res., Eur. J. Operation Res. 148, 141–151. doi:10.1016/s0377-2217(02)00460-5
Lange, M. (2005). On the uncertainty of wind power predictions—analysis of the forecast accuracy and statistical distribution of errors. J. Sol. Energy Eng. 127 (2), 177–184. doi:10.1115/1.1862266
Li, Z., Wang, Z., Fu, Y., and Zhao, N. (2021). Energy supply reliability assessment of the integrated energy system considering complementary and optimal operation during failure. IET Gener. Transm. Distrib. 15 (13), gtd2.12143–1907. doi:10.1049/gtd2.12143
Lin, G., Zetao, J., and Zongshun, T. (2017). Optimal diameter sizing based on centralized quality-quantity regulation and economic analysis of IES piping network. Electr. Power Autom. Equip. 37 (6), 75–83. (In Chinese).
Liu, T., Lu, J., He, C., and Xie, Y. (2019). Day-ahead economic dispatch of multi-energy parks considering integrated thermo-electric demand response and high penetration of renewable energy. Electr. Power Autom. Equip. (08), 261–268. doi:10.16081/j.epae.201908039
Liu, X., Li, N., Liu, F., Mu, H., Li, L., and Liu, X. (2021). Optimal design on fossil-to-renewable energy transition of regional integrated energy systems under co2 emission abatement control: A case study in dalian, China. Energies 14 (10), 2879. doi:10.3390/en14102879
Liu, X., Liu, F., Li, N., Mu, H., and Li, L. (2022). Hierarchical multi-objective design of regional integrated energy system under heterogeneous low-carbon policies. Sustain. Prod. Consum. 32, 357–377. doi:10.1016/j.spc.2022.04.027
Liuchen, L., Guomin, C., Jiaxing, C., Xiaohuang, H., and Di, L. (2022). Two-stage superstructure model for optimization of distributed energy systems (DES) part I: Model development and verification. Energy 245, 123227. doi:10.1016/j.energy.2022.123227
Lu, S., Li, Y., and Xia, H. (2018). Study on the configuration and operation optimization of CCHP coupling multiple energy system. Energy Convers. Manag. 177, 773–791. doi:10.1016/j.enconman.2018.10.006
Lu, X., Chan, K. W., Xia, S., Zhou, B., and Luo, X. (2018). Security-Constrained multiperiod economic dispatch with renewable energy utilizing distributionally robust optimization. IEEE Trans. Sustain. Energy 10 (2), 768–779. doi:10.1109/tste.2018.2847419
Mala, R., and Rajeshwer, P. S. (2020). Dispatch strategies based performance analysis of a hybrid renewable energy system for a remote rural area in India. J. Clean. Prod. 259, 120697. doi:10.1016/j.jclepro.2020.120697
Mazaheri, H., Abbaspour, A., Fotuhi-Firuzabad, M., Moeini-Aghtaie, M., Farzin, H., Wang, F., et al. (2021). An online method for MILP co-planning model of large-scale transmission expansion planning and energy storage systems considering N-1 criterion. IET Gener. Transm. Distrib. 15 (4), 664–677. doi:10.1049/gtd2.12050
Mei, A., Liu, P., Lu, C., Wei, J., and Zhang, K. (2022). “Hierarchical optimal scheduling of regional integrated energy power system based on multi-objective particle swarm optimization algorithm,” in Proceedings - 2022 14th International Conference on Measuring Technology and Mechatronics Automation, ICMTMA 2022 14th International Conference on Measuring Technology and Mechatronics Automation, Changsha, China (Changsha, China: IEEE), 2022–January 15-16, 2022.2022, 110–115. doi:10.1109/ICMTMA54903.2022.00029
Merei, G., Berger, C., and Sauer, D. U. (2013). Optimization of an off-grid hybrid PV–Wind–Diesel system with different battery technologies using genetic algorithm. Sol. Energy 97, 460–473. doi:10.1016/j.solener.2013.08.016
Min, W., Jiazhu, X., Linjun, Z., Chang, L., Yuxing, L., Yuqin, Y., et al. (2022). Two-stage robust optimization model for park integrated energy system based on dynamic programming. Appl. Energy 308, 118249. doi:10.1016/j.apenergy.2021.118249
Mohammad, J. K., Amit, K. Y., and Lini, M. (2017). Techno economic feasibility analysis of different combinations of PV-Wind-Diesel-Battery hybrid system for telecommunication applications in different cities of Punjab, India. Renew. Sustain. Energy Rev. 76, 577–607. doi:10.1016/j.rser.2017.03.076
Mousavi G, S. M. (2012). An autonomous hybrid energy system of wind-tidal-microturbine-battery storage. Int. J. Electr. Power & Energy Syst. 43 (1), 1144–1154. doi:10.1016/j.ijepes.2012.05.060
Ni, W., Lu, L., Xiang, Y., Liu, J., Huang, Y., and Wang, P. (2020). Reliability evaluation of integrated energy system based on markov process Monte Carlo method. Dianwang Jishu/Power Syst. Technol. 44 (1), 150–158. (In Chinese). doi:10.13335/j.1000-3673.pst.2019.0047
Paliwal, P., Patidar, N. P., and Nema, R. K. (2014). Determination of reliability constrained optimal resource mix for an autonomous hybrid power system using Particle Swarm Optimization. Renew. Energy 63, 194–204. doi:10.1016/j.renene.2013.09.003
Pei, H., Benedetta, C., Xingxing, Z., Jingchun, S., Isabelle, L., Mats, R., et al. (2020). A review of data centers as prosumers in district energy systems: Renewable energy integration and waste heat reuse for district heating. Appl. Energy 258, 114109. doi:10.1016/j.apenergy.2019.114109
Peng, Z., Fu, C., Zhu, K., Zhang, Q., Ni, D., and Yang, S. (2016). An improved LP model for energy optimization of the integrated iron and steel plant with a cogeneration system in China. J. Syst. Sci. Syst. Eng. 25 (4), 515–534. doi:10.1007/s11518-015-5293-x
Philip, V., Matthias, L., Gregor, W., and André, B. (2012). Superstructure-free synthesis and optimization of distributed industrial energy supply systems. Energy 845, 424–435. doi:10.1016/j.energy.2012.01.041
Qu, K., Huang, L., Yu, T., and Zhang, X. (2018). Decentralized dispatch of multi-area integrated energy systems with carbon trading. Proc. CSEE 38 (03), 697–707. (In Chinese). doi:10.13334/j.0258-8013.pcsee.170602
Rong, A., and Lahdelma, R. (2005). An efficient linear programming model and optimization algorithm for trigeneration. Appl. Energy 82, 40–63. doi:10.1016/j.apenergy.2004.07.013
Rongrong, Z., Hongtao, L., Chao, L., Miaomiao, Z., and Yongpin, Y. (2016). Analysis of a solar-aided coal-fired power generation system based on thermo-economic structure theory. Energy 102, 375–387. doi:10.1016/j.energy.2016.02.086
Ryohei, Y., Yuji, S., Syusuke, T., Masashi, O., and Tetsuya, W. (2015). Optimization of energy supply systems by MILP branch and bound method in consideration of hierarchical relationship between design and operation. Energy Convers. Manag. 92, 92–104. doi:10.1016/j.enconman.2014.12.020
Saeedeh, A., and Shirzad, A. (2016). Application of the Hybrid Big Bang–Big Crunch algorithm for optimal sizing of a stand-alone hybrid PV wind battery system[J]. Sol. Energy 134, 366–374. doi:10.1016/j.solener.2016.05.019
Sanaye, S., Meybodi, M. A., and Shokrollahi, S. (2008). Selecting the prime movers and nominal powers in combined heat and power systems. Appl. Therm. Eng. 28 (10), 1177–1188. doi:10.1016/j.applthermaleng.2007.08.003
Sayem, Z., and Ibrahim, D. (2014). Energy, exergy and exergoeconomic analyses of a combined renewable energy system for residential applications. Energy Build. 71, 68–79. doi:10.1016/j.enbuild.2013.12.006
Seyed, M. H., Arezoo, H., Miadreza, S., Mohamed, L., and João, P. S. C. (2022). Optimal sizing of renewable energy systems in a Microgrid considering electricity market interaction and reliability analysis. Electr. Power Syst. Res. 203, 107678. doi:10.1016/j.epsr.2021.107678
Shaneb, O. A., Taylor, P. C., and Coates, G. (2012). Optimal online operation of residential μCHP systems using linear programming. Energy Build. Energy Build. 44, 17–25. doi:10.1016/j.enbuild.2011.10.003
Shebaz, A. M., Darshit, S. U., and Rajesh, N. P. (2021). Optimal configuration of solar and wind-based hybrid renewable energy system with and without energy storage including environmental and social criteria: A case study. J. Energy Storage 44, 103446. doi:10.1016/j.est.2021.103446
Shilaja, C. (2021). “Perspective of combining chaotic particle swarm optimizer and gravitational search algorithm based on optimal power flow in wind renewable energy,” in 3rd International Conference on Computing and Communication, IC3 2020, India, March 20-21, 2020 (Rangpo: Springer Science and Business Media Deutschland GmbH). 2020. doi:10.1007/978-981-15-7394-1_44
Soltani, R., Mohammadzadeh Keleshtery, P., Vahdati, M., Khoshgoftarmanesh, M. H., Rosen, M. A., and Amidpour, M. (2014). Multi-objective optimization of a solar-hybrid cogeneration cycle: Application to CGAM problem. Energy Convers. Manag. 81, 60–71. doi:10.1016/j.enconman.2014.02.013
Suresh, M. V. J. J., Reddy, K. S., and Kolar, A. K. (2010). 4-E (Energy, Exergy, Environment, and Economic) analysis of solar thermal aided coal-fired power plants. Energy Sustain. Dev. 14, 267–279. doi:10.1016/j.esd.2010.09.002
Tetsuya, W., and Ryohei, Y. (2014). Optimal structural design of residential cogeneration systems in consideration of their operating restrictions. Energy 64, 719–733. doi:10.1016/j.energy.2013.10.002
Villanueva, D., Pazos, J. L., and Feijoo, A. (2011). Probabilistic load flow including wind power generation. IEEE Trans. Power Syst. 26 (3), 1659–1667. doi:10.1109/tpwrs.2010.2096436
Wang, C., Gao, R., Wei, W., Shafie-khah, M., Bi, T., and Catalao, J. P. S. (2018). Risk-based distributionally robust optimal gas-power flow with Wasserstein distance. IEEE Trans. Power Syst. 34 (3), 2190–2204. doi:10.1109/tpwrs.2018.2889942
Wang, J., Zeng, P., Li, Y., and Liu, J. (2022). Optimal capacity planning of power to hydrogen in integrated ElectricityHydrogenGas energy systems considering flexibility and hydrogen injection. Front. Energy Res. 10. doi:10.3389/fenrg.2022.845637
Wang, M., Yu, H., Yang, Y., Lin, X., Guo, H., Li, C., et al. (2021). Unlocking emerging impacts of carbon tax on integrated energy systems through supply and demand co-optimization. Appl. Energy 302, 117579. doi:10.1016/j.apenergy.2021.117579
Wang, S., Zhao, C., Liu, H., Gao, R., Liu, S., and Su, X. (2022). Comprehensive evaluation method and index system for electric-hydrogen-storage integrated energy network. In (Vol. 119, pp. 128–137). Springer Sci. Bus. Media Deutschl. GmbH. doi:10.1007/978-3-030-97064-2_13
Wang, Y., Wang, Y., Huang, Y., Li, F., Zeng, M., Li, J., et al. (2019). Planning and operation method of the regional integrated energy system considering economy and environment. Energy 171 (15), 731–750. doi:10.1016/j.energy.2019.01.036
Wang, Z., Shi, Y., Tang, Y., Men, X., Cao, J., and Wang, H. (2019). Low carbon economy operation and energy efficiency analysis of integrated energy systems considering LCA energy chain and carbon trading mechanism. Proc. CSEE 39 (06), 1614–1626+1858. (In Chinese). doi:10.13334/j.0258-8013.pcsee.180754
Wei, G., Jun, W., Shuai, L., Zhao, L., and Chenyu, W. (2017). Optimal operation for integrated energy system considering thermal inertia of district heating network and buildings. Appl. Energy 199, 234–246. doi:10.1016/j.apenergy.2017.05.004
Wei, H., Hongguang, J., and Rumou, L. (2011). A novel multifunctional energy system for CO2 removal by solar reforming of natural gas. J. Sol. energy Eng. 133, 1–8. doi:10.1115/1.4004034
Wei, Z., Zhang, S., Sun, G., Xu, X., Chen, S., and Chen, S. (2016). Carbon trading based low-carbon economic operation for integrated electricity and natural gas energy system. Electr. Power Autom. Equip. 40 (15), 9–16. (In Chinese). doi:10.1002/ese3.967
Xiaomin, L., Lei, C., Fei, X., Zeheng, L., Xiaohai, W., Youhua, H., et al. (2017). Optimal energy-source capacity ratios of integrated electictiy-heat energy system of west Inner Mongolia power grid. Electr. Power Autom. Equip. 37 (6), 132–138. (In Chinese). doi:10.16081/j.issn.1006-6047.2017.06.018
Xinjie, Y., Yuanchang, L., and Richard, B. (2021). Optimised MOPSO with the grey relationship analysis for the multi-criteria objective energy dispatch of a novel SOFC-solar hybrid CCHP residential system in the UK. UK: Energy Conversion and Management, 114406.
Xu, C., Ke, Y., Li, Y., Chu, H., and Wu, Y. (2020). Data-driven configuration optimization of an offgrid wind/PV/hydrogen system based on modified NSGA-II and CRITIC-TOPSIS. Energy Convers. Manag. 215, 112892. doi:10.1016/j.enconman.2020.112892
Xu, Z., Sun, Y., Xie, D., Wang, J., and Zhong, Y. (2020). Optimal configuration of energy storage for integrated region energy system considering power/thermal flexible load. Dianli Xit. Zidonghua/Automation Electr. Power Syst. 44 (2), 53–59. doi:10.7500/AEPS20190620005
Xue, Y., Guo, Q., Sun, H., Shen, X., and Tang, L. (2017). Comprehensive energy utilization rate for park-level integrated energy system. Electr. Power Autom. Equip. 37 (06), 117–123. (In Chinese). doi:10.16081/j.issn.1006-6047.2017.06.016
Xuemin, Y., Jia, W., and Chunxi, L. (2015). Energy conservation and emission reduction potentials of renewable energy integrated with coal-fired power generation system. ACTA Energyiae Solaris Sinca 36 (7), 717–1723. (In Chinese).
Yagoub, W., Doherty, P., and Riffat, S. B. (2006). Solar energy-gas driven micro-CHP system for an office building. Appl. Therm. Eng. 26 (14-15), 1604–1610. doi:10.1016/j.applthermaleng.2005.11.021
Yan, R., Wang, J., Lu, S., Ma, Z., Zhou, Y., Zhang, L., et al. (2021). Multi-objective two-stage adaptive robust planning method for an integrated energy system considering load uncertainty. Energy Build. 235 (2), 110741. doi:10.1016/j.enbuild.2021.110741
Yang, L., Chunling, W., Guoqing, L., Jinlong, W., Dongbo, Z., and Chen, C. (2020). Improving operational flexibility of integrated energy system with uncertain renewable generations considering thermal inertia of buildings. Energy Convers. Manag. 207, 112526. doi:10.1016/j.enconman.2020.112526
Yawen, Z., Hui, H., and HongGuang, J. (2014). Relative Efficiency improvement of solar-coal hybrid power. plant J. Eng. Thermophys. 35 (10), 1895–1900. (In Chinese).
Yi, G., Jitian, H., Qingzhao, M., and Jiahui, F. (2022). Optimal configuration and operation analysis of solar-assisted natural gas distributed energy system with energy storage. Energy 246, 123429. doi:10.1016/j.energy.2022.123429
Yi, Z., Chuntian, C., Cao, R., Gang, L., Jianjian, S., and Xinyu, W. (2021). Multivariate probabilistic forecasting and its performance’s impacts on long-term dispatch of hydro-wind hybrid systems. Appl. Energy 283, 116243. doi:10.1016/j.apenergy.2020.116243
Yin, B., Li, Y., Miao, S., Lin, Y., and Zhao, H. (2021). An economy and reliability co-optimization planning method of adiabatic compressed air energy storage for urban integrated energy system. J. Energy Storage 40, 102691. doi:10.1016/j.est.2021.102691
Yinghong, C., Yongping Y, , Zhiping, Y., Hongjuan, H., and Xiyan, G. (2008). Coupling mechanism of solar supported coal-fired electric generation system. Proc. CSEE 28 (29), 99–104. (In Chinese). doi:10.13334/j.0258-8013.pcsee.2008.29.019
Yixun, X., Qinglai, G., Hongbin, S., Xinwei, S., and Lei, T. (2017). Comprehensive energy utilization rate for park-level integrated energy system. Electr. Power Autom. Equip. 37 (6), 117–122. (In Chinese. doi:10.16081/j.issn.1006-6047.2017.06.016
Yongpin, Y., Yong, Z., and Rongrong, Z. (2016). Study on solar contribution of solar tower aided coal-fired power generation system. J. North China Electr. Power Univ. 43 (3), 56–63. (In Chinese). doi:10.3969/j.ISSN.1007-2691.2016.03.09
Yun, Y., Shijie, Z., and Yunhan, X. (2015). An MILP (mixed integer linear programming) model for optimal design of district-scale distributed energy resource systems. Energy 90, 1901–1915. doi:10.1016/j.energy.2015.07.013
Yun, Y., Shijie, Z., and Yunhan, X. (2015). Optimal design of distributed energy resource systems coupled with energy distribution networks. Energy 85, 433–448. doi:10.1016/j.energy.2015.03.101
Yunfei, M., Wanqing, C., Xiaodan, Y., Hongjie, J., Kai, H., Congshan, W., et al. (2020). A double-layer planning method for integrated community energy systems with varying energy conversion efficiencies. Appl. Energy 279, 115700. doi:10.1016/j.apenergy.2020.115700
Yuxuan, L., Junli, Z., Xiao, W., Jiong, S., and Kwang, Y. L. (2022). Optimal design of combined cooling, heating and power multi-energy system based on load tracking performance evaluation of adjustable equipment. Appl. Therm. Eng. 211, 118423. doi:10.1016/j.applthermaleng.2022.118423
Zeng, B., and Zhao, L. (2013). Solving two-stage robust optimization problems using a column-and-constraint generation method. OPERATIONS Res. Lett. 41 (5), 457–461. doi:10.1016/j.orl.2013.05.003
Zeng, R., Li, H., Jiang, R., Liu, L., and Zhang, G. (2016). A novel multi-objective optimization method for CCHP–GSHP coupling systems. Energy Build. 112, 149–158. doi:10.1016/j.enbuild.2015.11.072
Zhang, B., Sun, Y., Zhong, Y., and Shen, M. (2018). “Optimal energy flow of electricity-gas integrated energy system using second-order cone Program,” in Proceedings of the 30th Chinese Control and Decision Conference, CCDC 2018 30th Chinese Control and Decision Conference, June 9, 2018Shenyang, China (CCDC).
Zhang, P., Lu, X., and Li, K. (2021). Achievable energy flexibility forecasting of buildings equipped with integrated energy management system. IEEE Access 9, 122589–122599. doi:10.1109/ACCESS.2021.3110657
Zhao, J., Xiong, J., Yu, H., Bu, Y., Zhao, K., Yan, J., et al. (2022). Reliability evaluation of community integrated energy systems based on fault incidence matrix. Sustain. Cities Soc. 80, 103769. doi:10.1016/j.scs.2022.103769
Zheng, L., Yingzong, L., Xianglong, L., Jianyong, C., Zhi, Y., Chao, W., et al. (2022). Superstructure-based mixed-integer nonlinear programming framework for hybrid heat sources driven organic Rankine cycle optimization. Appl. Energy 307, 118277. doi:10.1016/j.apenergy.2021.118277
Zhong, Y., Hu, B., Lin, L., Zhang, W., Li, Y., and Qi, Y. (2021). “Comprehensive evaluation strategy of optimal operation mode of integrated energy system based on analytic hierarchy process,” in 5th IEEE Conference on Energy Internet and Energy System Integration: Energy Internet for Carbon Neutrality, EI2 2021 5th IEEE Conference on Energy Internet and Energy System Integration, Taiyuan, China, October 22, 2021 (IEEE). EI2 2021. doi:10.1109/EI252483.2021.9713176
Zhou, B., Xia, H., and Zang, T. (2022). Station and network coordinated planning of park integrated energy system considering energy cascade utilization. Dianli Zidonghua Shebei/Electric Power Autom. Equip. 42 (1), 20–27. doi:10.16081/j.epae.202109038
Zhu, R., Wei, H., and Bai, X. (2019). Wasserstein metric based distributionally robust approximate framework for unit commitment. IEEE Trans. Power Syst. 34 (4), 2991–3001. doi:10.1109/tpwrs.2019.2893296
Keywords: hybrid energy system (HES), configuration optimization, renewable energy, dynamic, uncertainty, integrated structure
Citation: Zhang J and Wei H (2022) A review on configuration optimization of hybrid energy system based on renewable energy. Front. Energy Res. 10:977925. doi: 10.3389/fenrg.2022.977925
Received: 25 June 2022; Accepted: 27 July 2022;
Published: 24 August 2022.
Edited by:
Xiangdong Liu, Yangzhou University, ChinaReviewed by:
Yali Xue, Tsinghua University, ChinaYu Huang, North China Electric Power University, China
Copyright © 2022 Zhang and Wei. This is an open-access article distributed under the terms of the Creative Commons Attribution License (CC BY). The use, distribution or reproduction in other forums is permitted, provided the original author(s) and the copyright owner(s) are credited and that the original publication in this journal is cited, in accordance with accepted academic practice. No use, distribution or reproduction is permitted which does not comply with these terms.
*Correspondence: Junli Zhang, zjli@seu.edu.cn