- 1College of Metrology and Measurement Engineering, China Jiliang University, Hangzhou, China
- 2Zhejiang Cangnan Instrument Group Co Ltd, Wenzhou, China
Introduction
During the strategic deployment of bridging the gap between eastern and western regions in computing resources, the data center is evolving from high-speed to high-quality development. An extremely significant part is to reduce energy consumption during operation and improve energy efficiency. As a gist for evaluating energy efficiency and a means of implementing energy-saving supervision, energy measurement is a powerful support and guarantee for achieving carbon peak and carbon neutrality goals (Zhao et al., 2021) and promoting the development of green energy (Wan, 2022). Fluid energy accounts for a large proportion in the energy structure, such as natural gas, steam, and crude oil. Among them, the proportion of natural gas in the primary energy structure continues to rise (Chang, 2019) in recent years. According to the “Statistical Review of World Energy”, in 2021, the natural gas demand exceeds four trillion m3 in the whole world, with a growth rate per annum of 4.8% and an average annual growth rate of 2.2% in the past decade (BP Group, 2022). Especially, Chinese natural gas supply and demand gap reached 167.3 billion m3, and its foreign dependence reached 44.9%. It is a challenge to ensure the accuracy of natural gas measurement and the smooth progress of the natural gas trade. The gas turbine flowmeter has obtained a broad development space and economic benefits in the international natural gas trading market (Xiang and Ceng, 2020) because of its high precision, good repeatability, high range ratio, and convenient maintenance. But researchers still found that there are some questions in the development of the gas turbine flowmeter. First, the flow parameters of natural gas are dynamic and diverse (Zhang et al., 2021), which increases the difficulty of tracing the flow measurement value. Second, the complex structure of gas turbine flowmeter’s performance is difficult to optimize. Furthermore, how to improve the measurement accuracy of small flow has always been a research difficulty. Finally, people often misunderstand the internal flow field because the numerical simulation flow–structure interaction of the gas turbine flowmeter is not accurate enough. This study mainly clarifies the aforementioned problems and puts forward some perspectives on the research of gas turbine flowmeters.
Flowrate measurement of gas turbine flowmeter
The structure of a gas turbine flowmeter mainly consists of a front rectifier, impeller, rear deflector, flow totalizer, and so on, which is illustrated in Figure 1A. During the measurement process, the natural gas pushes the impeller blades to rotate, and the rotation of the impeller will periodically change the magnetoresistance value of the magneto electric converter and generate an electric pulse signal. The signal will converse an analog current to obtain the instantaneous flow value and then the pulse signal is sent to the flow integrator to obtain the cumulative flow value. In the aforementioned processes, the gas turbine flowmeter must overcome many resistances, such as impeller resistance torque, shaft friction torque, and magnetic resistance torque (Thompson and Grey, 1970). Especially, the influence of these resistances is obvious when measuring a small flow (Nie et al., 2018) that will make errors, as shown in Figure 1B. Moreover, the measurement effect of the gas turbine flowmeter will also be affected by the fluid motion state and velocity distribution (Huang, et al., 2016). Therefore, people usually use the flow standard device to achieve the magnitude calibration to verify the measurement of the gas turbine flowmeter under various working conditions.
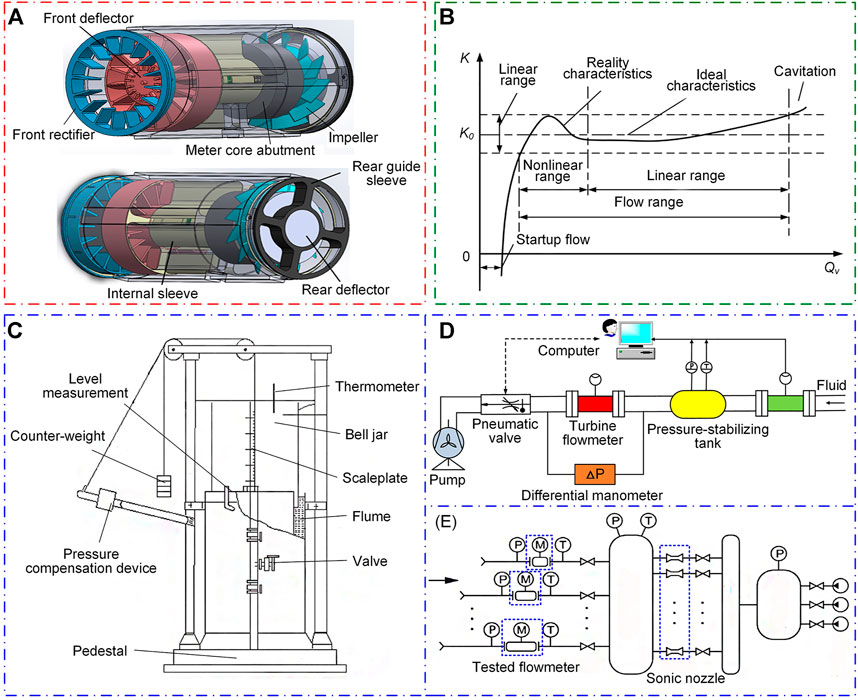
FIGURE 1. Flow rate measurement of gas turbine flowmeter: (A) Physical model of a gas turbine flowmeter, (B) K-Q curve of the gas turbine flowmeter, (C) bell jar–type gas flow standard device, (D) standard meter method flow standard device, and (E) sonic nozzle method gas flow standard devices.
The commonly used flow standard devices (Figure 1C–E) include the sonic nozzle method gas flow standard device (Jiang, et al., 2021), bell jar–type gas flow standard device (Yang and Peng, 2021), and standard meter method flow standard device (Yu and Jian, 2022). The working principle of the first two flow standard devices is different from the gas turbine flowmeter, which cannot reflect the dynamic parameters of natural gas truly. As for the standard meter method flow standard device, the accuracy demand of the standard flowmeter is high in the verification of a high-precision gas turbine flowmeter, which increases the difficulty of the device assembly. For tracing the dynamic value of state and physical parameters of natural gas under actual operating conditions, the actual flow calibration (Gao, et al., 2014) is much account of. To be specific, the high-pressure piston prover (HPPP) method can trace the measurement value to the national length and time benchmark (Yan, et al., 2019); the mass–time (MT) method (Li, et al., 2014) can trace the measurement value to the national quality and time benchmark; and the p.V.T.t method (Wang, et al., 2021) can calculate the gas mass flow according to the pressure, volume, temperature, and time and complete the traceability of quantity value. Nevertheless, this research was carried out late in China due to technology, process, and other factors. It is important to transfer the quantity value to the standard device by using the gas turbine flowmeter in the future.
Relationship between performance and structure of the gas turbine flowmeter
In the current study, the performance of the gas turbine flowmeter is mainly assessed by linearity error, repeatability error, and pressure loss (Li et al., 2008). The linearity error and repeatability error can be calculated by the instrument coefficient. Both the instrument coefficient and pressure loss are significantly affected by the physical structure of the gas turbine flowmeter. Therefore, many researchers focus on optimizing the structure of a gas turbine flowmeter to reduce the pressure loss and make the instrument coefficient more stable.
The structure optimization of the gas turbine flowmeter mainly focuses on the front rectifier, impeller, and rear deflector. Research shows that in the part of the front rectifier, increasing its length can effectively improve flow field uniformity, reduce the linear error, repeatability error, and pressure loss, and shorten the front rectifier blade can improve the performance furthermore (Sun, et al., 2016). Moreover, the linearity error of the instrument coefficient will be reduced by changing the inflow angle, axial width, and coincidence degree of the blade (Sun, et al., 2022). As for the rear deflector, prolonging the length and position of the rear deflector vane can smooth the flow channel shape, to achieve the effect that the fluid decelerated and pressurized first and then diverted and significantly improved the stability of the instrument coefficient (Shao, et al., 2022).
To sum up, scholars have studied various structures of gas turbine flowmeters, but they only conducted qualitative research on structural design. As for how to obtain the optimal solution on the structural data, there is, no one can give a quantitative answer. With the development and popularization of machine learning algorithms, artificial intelligence can help people find the optimal structural design scheme, which will be the research focus in the design of gas turbine flowmeters in the future.
Simulation of a gas turbine flowmeter
For saving experimental resources and working time, people often predict the start-up flow and pressure loss by using the numerical simulation method (Liu and Yan, 2016). The commonly used numerical simulation module is the moving reference frame module (MRF), which is often used for CFD simulation of translation and rotation equipment. However, with the progress of the times, the accuracy of MRF cannot meet the requirements of our people, and the numerical simulation of fluid-structure in a passive mesh is becoming more popular (Pei, 2015). In fact, the passive mesh is difficult to converge and takes a long time in practical applications, people often optimized the grid generation technology, such as moving mesh generation technology, overlapping mesh generation technology, and adaptive mesh generation technology (Meng, et al., 2022) (Hong, et al., 2020) for fear of waste of times. The complex grid generation method needs to consume vast computing resources. Therefore, scholars have designed and implemented an Open Source Field Operation and Manipulation (OpenFOAM) grid generation server for a cloud computing environment to improve the efficiency of grid computing (Gao et al., 2022).
After mesh generation, the fluid motion is theoretically described by the Naiver–Stokes equation, but the analytical solution of the equation can be obtained only in a few cases for the non-linear conditions caused by the flow term (Tong, et al., 2016). Recently, a new research paradigm of fluid mechanics emerged with the development of artificial intelligence, which can select the grid input features or design the grid architecture based on the laws of fluid physics, called the physics-inspired deep learning method (Jin, et al., 2021). Scholars have found that this method can solve difficult problems in complex flows. For examples, the hydraulic simulation model of verification station constructed based on a BP neural network can better simulate the real flow state of the station while ensuring the calculation speed (Wen et al., 2021). The subgrid-scale (SGS) stress in large Eddy simulation (LES) is improved by artificial neural network-based non-linear algebraic models and obtains a high accuracy (Xie, Yuan, et al., 2020). Nevertheless, this method has not been widely used in the research of gas turbine flowmeters. In future research, applying the physics-inspired deep learning method to the gas turbine flowmeter and combining a gas turbine flowmeter with multidisciplinary will become a great challenge for contemporary scholars.
Challenges
From before, the development of the Chinese gas turbine flowmeter has faced the following main challenges:
1) Improving the ability of China’s natural gas measurement traceability and the sound measurement standard system by developing the gas turbine flowmeter as the working level standard device for the transfer of the value of the function.
2) There is still a lot of space for the development of gas turbine flowmeter structure optimization, such as using deep learning methods to better determine the optimal structure optimization scheme.
3) Keeping the instrument coefficient stable in the small flow range is still a big problem, maybe can find a solution from each moment of resistance.
4) It is difficult to improve the accuracy of the numerical simulation results of gas turbine flowmeter and develop new algorithms for computational fluid dynamics. For example, how to use neural networks to incorporate the non-linear terms in the fluid motion process into the numerical simulation still needs people to research.
Conclusion
As the mainstream device of natural gas measurement, the gas turbine flowmeter plays a significant role in the field of energy measurement. Recently, the research on gas turbine flowmeters mainly through the methods of experiment and simulation to improve the metering performance of gas turbine flowmeters. As for the experimentation, people continuously improve the original standard device of measurement to reach the quantity traceability of natural gas. In the aspect of simulation, the traditional stand-alone numerical simulation is changed into the ‘cloud + end’ platform mode to improve the simulation efficiency, and the algorithm formula is optimized through the deep learning method to improve the simulation accuracy. The structure of the gas turbine flowmeter is analyzed and optimized to improve its performance by combining the experiment and simulation. A more perfect measurement standard system needs to be established in the future to guarantee a reasonable, fair, and just natural gas measurement. In addition, the machine learning algorithm is used to improve the structure of the gas turbine flowmeter, optimize the formula of the numerical simulation algorithm, and combine the traditional methods with the era of big data to improve the performance of the gas turbine flowmeter furthermore.
Author contributions
GW: investigation, data curation, and writing—original draft preparation. WY: conceptualization, methodology, and writing—reviewing and editing. CW: reviewing, editing, and supervision. YX: supervision, methodology, and validation. JL: supervision, methodology, and validation. SZ: supervision, methodology, and validation.
Conflict of interest
Authors YX, JL and SZ were employed by Zhejiang Cangnan Instrument Group Co., Ltd.
The remaining authors declare that the research was conducted in the absence of any commercial or financial relationships that could be construed as a potential conflict of interest.
Publisher’s note
All claims expressed in this article are solely those of the authors and do not necessarily represent those of their affiliated organizations, or those of the publisher, the editors, and the reviewers. Any product that may be evaluated in this article, or claim that may be made by its manufacturer, is not guaranteed or endorsed by the publisher.
References
BP Group. (2022). Statistical Review of world energy. Available at: https://www.bp.com/en/global/corporate/energy-economics/statistical-review-of-world-energy/oil-gas-and-coal-trade.html.
Chang, J. C. (2019). Status and development trend on domestic and abroad natural gas flow metering technology. Res. Discuss. 2, 36–38.
Gao, R. C., Huaiet, X. Y., Zhang, Z. D., and Li, B. W. (2022). OpenFOAM mesh generation service foe cloud computing environment. Comput. Technol. Its Appl. 48 (4), 71–75. doi:10.16157/j.issn.0258-7998.211506
Gao, Z. G., Zhu, R. M., Wu, J., and Wang, M. Y. (2014). Design and research on actual flow calibration primary standard device of natural gas. Automation Petro-Chemical Industry 50, 13–16.
Hong, Z. C., Zong, Z., Wang, Y. G., Zhao, M. J., Liu, L., and Liang, G. J. (2020). Research on uncertainty of overset mesh technology based on orthogonal experiment method. J. Ship Mech. 24 (7), 865–873. doi:10.3969/j.issn.1007-7294.2020.07.003
Huang, C. H., Su, M. G., Kao, H. J., Jhong, J. H., Weng, S. L., and Lee, T. Y. (2016). UbiSite: Incorporating two-layered machine learning method with substrate motifs to predict ubiquitin-conjugation site on lysines. BMC Syst. Biol. 40, 6. doi:10.1186/s12918-015-0246-z
Jiang, H. C., Guo, G., and Tou, W. M. (2021). Online calibration of sonic nozzle gas flow standard device. Process Autom. Instrum. 42, 4. doi:10.16086/j.cnki.issn1000-0380.2020040034
Jin, X. W., Laima, S., and Li, H. (2021). Physics-enhanced deep learning methods foe modelling and simulating flow fields. Chin. J. Theor. Appl. Mech. 53 (10), 2616–2629. doi:10.6052/0459-1879-21-373
Li, X., Wang, Y. T., Li, C. J., Miao, Y. S., Yin, X. J., Sun, X. D., et al. (2008). Verification regulation of turbine flowmeter. Beijing: Inspection and Quarantine of the People’s Republic of China.
Li, D. J., Zhang, M. Z., Li, S. X., and Ji, W. F. (2014). MT method for gas flow primary standard device. Meas. Equip. Appl. 24, 4. doi:10.13228/j.boyuan.issn1002-1183.2014.04.013
Liu, M. J., and Yan, B. (2016). Simulation and performance analysis on inner flow field of rotating part in the turbine flowmeters. Mech. Eng. 2, 42–45.
Meng, X. Q., Liu, Z. W., Liu, T. R., and Chen, J. J. (2022). Auto-generation algorithm of surface meshes on dirty geometries. Acta Aerodyn. Sin. 40, 1–8. doi:10.7638/kqdlxxb-2021.0400
Nie, Q., et al. (2018). Application of boundary flow in gas full - wheel flowmeter selection. Ind. Meas. 28, 1. doi:10.13228/j.boyuan.issn1002-1183.2016.0305
Pei, J. H. (2014). Study on the optimal design of CNIM-TM series gas turbine flowmeter. Hangzhou: China Jiliang University.
Shao, J. C., Yan, W. W., Lin, J. D., and Zhang, S. Y. (2022). Structure optimization design of rear deflector for gas turbine flowmeter. Chin. J. Sci. Instrum. 43 (1), 46–53. doi:10.19650/j.cnki.cjsi.2108364
Sun, H. J., Feng, Y., and Wang, B. (2016). Numerical simulation and optimal design of front diversion body in gas turbine flowmeter. J. Electron. Meas. Instrum. 30 (4), 550–557. doi:10.13382/j.jemi.2016.04.007
Sun, P. F., Xu, H. P, Li, T., Li, D. H., Li, B. T., and Hong, J. (2022). Structural optimization design of turbine flowmeter for tests of high-thrust liquid rocket engine. J. Xi’an Jiaot. Univ. 56 (5), 74–84. doi:10.7652/xjtuxb202205008
Thompson, R. E., and Grey, J. (1970). Turbine flowmeter performance model. J. Fluids Eng. 4, 712–722. doi:10.1115/1.3425117
Tong, N. G., Yu, Y. L., and Wang, Z. H. (2016). Some remarks on theoretical modelling approach in applied fluid mechanics and analysis of flow physics. Phys. Gases 1 (5), 1–8. doi:10.19527/j.cnki.2096-1642.2016.05.001
Wan, Y. Y. (2022). Green economy development, clean energy consumption and carbon dioxide emissions. Ecol. Econ. 38 (5), 40–46.
Wang, P., Zhang, Y. S., and Zhang, Y. T. (2021). Gas flow calibration facility by p. V. T. t technique. Meas. Tech. 41 (2), 159–166. doi:10.11823/j.issn.1674-5795.2021.02.24
Wen, K., et al. (2021). Neural network based intelligent control system of natural gas flowmeter verification process. Nat. Gas. Ind. 41 (7), 124–133. doi:10.1109/ITC.2010.47
Xiang, W. J., and Ceng, L. (2020). Application of turbine flowmeter in natural gas metering. Metrology Meas. Tech. 47 (2), 54–56. doi:10.15988/j.cnki.1004-6941.2020.2.017
Xie, C., Yuan, Z., and Wang, J. (2020). Artificial neural network-based nonlinear algebraic models for large eddy simulation of turbulence. Phys. Fluids (1994). 32, 115101. doi:10.1063/5.0025138
Yan, W. C., Xu, M., and Lu, Y. C. (2019). Uncertainty evaluation of sonic nozzle verification device by HPPP. Ind. Meas. 30, 2. doi:10.13228/j.boyuan.issn1002-1183.20619.0206
Yang, Y. T., and Peng, L. (2021). Research on the metrological traceability for bell prover. Metrology Sci. Technol. 65, 7. doi:10.12338/j.issn.2096-9015.2020.0291
Yu, Z. Y., and Jian, X. Z. (2022). Software design for calibration of gas flow standard facility with master meter method. Electron. Sci. Technol. 35, 10. doi:10.16180/j.cnki.issn1007-7820.2022.10.005
Zhang, H. Z., Cheng, Z. T., Jia, R., Zhou, C. W., and Li, M. Y. (2021). Economic optimization of electric-gas integrated energy system considering dynamic characteristics of natural gas. Power Syst. Technol. 45, 4. doi:10.13335/j.1000-3673.pst.2020.1997
Keywords: energy measurement, data center, flow rate measurement, gas turbine flowmeter, artificial intelligence
Citation: Wan G, Yan W, Wu C, Xiao Y, Lin J and Zhang S (2023) Research progress and challenges of the gas turbine flowmeter in energy measurement. Front. Energy Res. 10:977140. doi: 10.3389/fenrg.2022.977140
Received: 24 June 2022; Accepted: 08 July 2022;
Published: 10 January 2023.
Edited by:
Chengbin Zhang, Southeast University, ChinaReviewed by:
Huilin Lai, Fujian Normal University, ChinaCopyright © 2023 Wan, Yan, Wu, Xiao, Lin and Zhang. This is an open-access article distributed under the terms of the Creative Commons Attribution License (CC BY). The use, distribution or reproduction in other forums is permitted, provided the original author(s) and the copyright owner(s) are credited and that the original publication in this journal is cited, in accordance with accepted academic practice. No use, distribution or reproduction is permitted which does not comply with these terms.
*Correspondence: Weiwei Yan, eWFud3dAY2psdS5lZHUuY24=