- 1Key Laboratory of Aerospace Information Security and Trusted Computing, Ministry of Education, School of Cyber Science and Engineering, Wuhan University, Wuhan, China
- 2College of Electrical Engineering, Zhejiang University, Hangzhou, China
With the rapid development of integrated energy system, the large-scale and high-permeability access of distributed generations (DGs) is making the distribution networks develop into active distribution networks (ADNs). The increased complexity of ADNs also increases the vulnerabilities for cyberattacks. It is a new challenge how to evaluate the situation of an ADN so as to support the decision-making of grid control policies in the condition of cyberattacks probably occur. Hence, in this paper, we proposed a method of situation assessment for ADNs considering cyberattacks. This method is aggregated by two parts. 1) An index system is presented, which includes the indexes of DGs stability, the indexes of security risk considering cyberattacks along with the traditional safety indexes. 2) The entropy weight method is used to assign weights to each index, and taking the normal operation status of ADNs as the reference scenario, an operating situation assessment method for ADNs is proposed based on grey correlation analysis method. Finally, in order to verify the effectiveness of the proposed index system and assessment method, 12 attack scenarios are established from three categories: attacks on DGs, attacks on controllable loads and attacks on both of them, and the situation of the ADN, a case based on IEEE 33-node standard distribution system, is evaluated under each scenario.
1 Introduction
The rapid development of integrated energy systems alleviate the energy crisis, but also brings security threats to the power system. However, situation assessment of power grid can help to grasp the operation status of power grid in time, provides basis for the projection and early warning of power grid situation, and assists the operation control decision of power grid so as to ensure the safe and stable operation of power grid (Lin et al., 2018; Russell, et al., 2018; Wang et al., 2019; Wang et al., 2020; Lai et al., 2022).
As a substantial component of ADNs, the large integration of DGs, controllable loads (CLs), and distributed energy storages (DESs) have caused severe challenges for the safe and stable operation of the ADNs. 1) In terms of DGs, power flows are now bidirectional rather than unidirectional, and it also alters the architecture of the conventional distribution network (Sultan et al., 2013). Furthermore, different manufacturers of DG use different communication protocols (Wang X. et al., 2017). Attackers could substantially threaten the safe and stable operation of ADNs and even cause power outages if they successfully utilize communication protocol vulnerabilities and other crucial information (Ismail et al., 2020). 2) In terms of CLs, with the uninterrupted improvement of inhabitants’ living standards, household terminal load is changed into household CLs via the Internet of Things. However, some household equipment cyber security protection measures are inadequate. When attackers utilize vulnerabilities to launch cyber attacks on large-scale household CLs, it may causes ADNs voltage overruns, frequency oscillations, circuit breaker disconnection, and power outage in severe case (Gallo et al., 2020). 3) In terms of DESs, the essential protocol standards for DESs access into ADNs are still in the initial stage, and communication management has not attracted much attention. Taking the electric vehicle charging and discharging station as an example, due to the user side of information security protection is relatively vulnerable, the attackers are more likely to use parking intelligent terminal embedded system vulnerabilities embedded malicious code and send malicious control command via the Internet, which can destroy the mode of electric vehicle charging and discharging, cause the power quality problems and ADNs power balance of demand and supply in severe cases (McLaughlin et al., 2016). It can be seen that the attackers launch cyber attacks through using the cyber security vulnerability, the adverse impact on ADNs can not be underestimated. Hence, in order to ensure the safe and stable operation of the ADNs, it is urgent to establish the operation situation assessment method of ADNs considering cyberattacks, so as to adjust the operation status of power grid, formulate control strategies and emergency plans.
At present, situation assessment as the core content of power grid situation awareness, the study of situation assessment can be mainly divided into three categories. 1) From the perspective of power grid dispatching control center, the situation awareness technology to the power grid operation control, and an intelligent dispatching system based on situation awareness are applied by Lai et al. (2020), Shahsavari et al. (2019) and Li et al. (2015). 2) The operation situation assessment and projection methods of power grid based on massive data collected by wide-area measurement system are studied by Li et al. (2020), Liu et al. (2018), Li et al. (2021), Jena et al. (2017) and Ren et al. (2019). 3) The main components and functional hierarchy of power grid situation awareness system are analyzed by Li et al. (2019), Wang and Govindarasu. (2020) and Zhao et al. (2019), and propose the smart grid situation awareness model and conceptual design. The above studies mainly focus on the power grid operation safety status assessment and the theoretical framework of power grid situation awareness, but the situation awareness methods are rarely discussed in detail and need to be further studied.
In the study of power grid situation awareness, there are relatively few studies about situation awareness of ADNs. A framework of ADNs situation awareness, constructs an optimal dispatching framework based on analysis of the linkage relationship between situation awareness and optimal dispatching, and elaborates the key technology for optimal dispatching of ADNs (Wang H. et al., 2017). From the initiative perspective of ADNs, a framework of situation awareness and points out the key problem should be solved in realizing situation awareness is given by Lin et al. (2016). Huang et al. (2017) mines a large amount of historical data values of ADNs, adds the ADNs virtual measurement information data, so as to improve the accuracy of state estimation and provides technical support for the online status perception of ADNs. Tao et al. (2020) proposes a situation awareness system of ADNs based on distributed monitoring and multi-source information fusion, and elaborates the situation awareness technology of ADNs based on multi-source information fusion. Above all, most of the above research works focus on the theoretical level of the ADNs situation awareness system framework, the key technologies such as multi-source information fusion ADNs situation assessment and projection methods are not in-depth enough studied, and there are rarely relevant study of considering cyberattacks and operation law of ADNs.
In order to evaluate the operation status of ADNs effectively, this paper studies the situation assessment method of ADNs considering cyberattack. The main contributions are as follows.
(1) Considering the uncertain outputs of DGs and the vulnerabilities for cyberattacks to DGs and controllable loads, the indexes of DGs stability and the indexes of security risk considering cyberattacks along with the traditional safety indexes are employed as the indexes of situation assessment of ADNs.
(2) On the basis of the proposed index system, the operation situation assessment method of ADNs is established based on the entropy weight method and grey relation method. This method can quantitative assessment the operation safety status of ADNs to provide the basis for operation situation projection and operation control decision of ADNs.
(3) Twelve attack scenarios of the ADN, a case based on IEEE 33-node standard distribution system, are established from three categories: attacks on DGs, attacks on controllable loads and attacks on both of them to verify the effectiveness of the proposed index system and assessment method.
The rest of paper is organized as follows. Section 2 proposes situation assessment index system. Sections 3 investigates situation assessment method of ADNs based on entropy weight method and grey correlation analysis method. Section 4 verifies the proposed method in IEEE 33-node active distribution system. And conclusions are presented in Section 5.
2 Situation assessment index system
The safe and stable operation of ADNs depends on its safety characteristics during operation, the stability of DGs in ADNs and the risk when it suffering cyberattacks (Canizes et al., 2017). On the one hand, the normal operation of ADNs require sufficient capacity margin to maintain the normal level of the voltage and frequency. The voltage value should not deviate too much from the rated voltage, and the number of voltage qualified nodes should not be less than the normal operation status standards. The branch line should not run under heavy load for a long period. The output of the DGs should not fluctuate too much in operation status. On the other hand, the cyberattack events against power system in recent years show that the potential cyberattacks risks also have a crucial impact on the safe and stable operation of ADNs. Therefore, we present the situation assessment index system of ADNs as shown in Figure 1.
2.1 Safety indexes of ADNs
The safe operation characteristics of ADNs are related to the power supply capacity margin, the voltage violation severity, the voltage qualification rate and the load rate (Fauzan et al., 2019). Those indexes can reflect the security margin of power supply capacity, the harmful degree of system voltage fluctuation and the security risk of ADNs.
2.1.1 Power supply capacity margin
The power supply capacity margin represents the percentage of the loads that can be increased based on the current loads. It can be defined as follows:
In Eq. 1,
2.1.2 Voltage violation severity
The voltage violation severity represents the degree of voltage deviation from the rated voltage. While the power grid failure has happened, the voltage value also be impacted, and the operation voltage value deviates from the normal voltage may aggravate the vulnerability of the ADNs. In severe cases, it directly impacts the safe and stable operation status of the ADNs. Hence, voltage violation severity can be defined as follows:
In Eq. 2,
2.1.3 Voltage qualified rate
Voltage qualified nodes need to satisfied the following requirements: 1) Power supply voltage exist on the nodes. 2) The nodes voltage value do not exceed the threshold. The voltage qualification rate refers to the percentage of voltage qualified nodes account for the total number nodes of the ADNs. Voltage qualified rate also reflects the comprehensive voltage quality during the operation status of ADNs. To some extent, it represents characterizes the security of ADNs operation status. Hence, voltage qualified rate can be defined as follows:
In Eq. 3,
2.1.4 Load rate
If the load rate of the main transformer approaches the threshold or run with heavy loads, once the distribution network suffered cyberattacks or failures happened, it may cause the load changed or large-scale power flow of a certain node transfer into another node. Therefore, it may lead to the overload of the main transformer and large-scale cascading failures happened in the future in severe case. From the perspective of safe operation of ADNs, no matter whether the failure of ADNs happened or not, we expect that the main transformer running in a safe range, and security risk decreases as the load rate decreases. Hence, the load rate can be defined as follows:
In Eq. 4,
2.2 Security indexes of DGs
The distribution network contains a large number of renewable energy DGs. Such as photovoltaic power stations, wind farms and so on. The output of those kinds of DGs are greatly impacted by climate, and climate can lead to uncertain output of DGs (Arya, 2016). What’s more, DGs are more likely to fluctuate under all kinds of disturbance, those disturbances can cause the change of the direction and value of the power flow in the distribution network, and even result in the fluctuation of the system voltage and bring the challenge to itself safe operations status. At the same time, due to many uncertain factors of DGs, the high permeability of DGs may increase the risk of stable operation of the ADNs and result in different degrees of impact on the ADNs security. Therefore, the output volatility and the penetration rate of DGs are play an important role in index evaluating the security risks of ADNs.
2.2.1 Output volatility of DGs
Output volatility of DGs can be defined as follows:
In Eq. 5,
2.2.2 Penetration rate of DGs
Penetration rate of DGs can be defined as follows:
In Eq. 6,
2.3 Risk indexes
According to the three elements of network security proposed by the National Institute of Standards and Technology (Zhao et al., 2019), cyberattacks can be classified into three categories according to their consequences as follows: 1) Destroying the confidentiality; 2) Destroying the integrity; 3) Destroying the availability. Among them, the first category of attacks aims to steal data and does not directly impact the power grid. The second category of attacks aims to control the power generations or loads maliciously by tampering or falsifying measurement data or control commands, which can directly impact the operation status of the power grid. The third category of attacks makes the cyber system partially or completely lose control of the power grid by blocking communication or increasing time delay, which mainly impacts the observability and controllability of the power grid. It can be seen that only the second category of attacks can be awareness through the operation status data of the power grid. Therefore, this paper established ADNs security risk index for second category of attacks.
2.3.1 Mutation severity of power supply capacity
Cyberattacks can cause the main transformers and DGs out of running, aggravate the power supply burden of the remaining transformers, and result the output shortage or voltage collapse of ADNs. Cyberattacks can also lead to increase output of DGs, aggravate the instability of ADNs, and excessive reactive power output can cause the voltage to exceed the safe operation range. The sudden changes of power supply capacity can impact the reliability and quality of ADNs, which may bring hidden impact to the safe operation status of the ADNs (Liang et al., 2021).
Therefore, the mutation severity index of power supply capacity can be defined as follows:
In Eq. 7,
2.3.2 Mutation severity of loads
Cyberattacks can lead to the large-scale controllable loads casting/dropping synchronously or frequent and synchronous casting and dropping, threaten the safe and stable operation of the ADNs(Kurt et al., 2018; Wei et al., 2020; Liang et al., 2021). Hence, we use the mutation severity of loads represent the impact of the cyberattacks.
The index of mutation severity of loads can be defined as follows:
In Eq. 8, Li(t+1) represents the loads of node i in time (t+1). Li(t) represents the loads of node i in time t. Ltotal(t+1) is the total loads of ADNs in time t.
3 Situation assessment method
In this section, the basic thought of the situation assessment method of ADNs is as follows: first, after establishing the ADNs situation assessment indexes according to Section 2, the weights are assigned to the indexes according to the impact degree of each index on the assessment results; then, the normal operation status of ADN is taken as the reference scenario, and the correlation degrees between the attack scenarios to be assessed and the reference scenario are calculated based on the grey correlation analysis method; finally, the security risk levels of those scenarios can be determined according to the pre-defined criteria.
3.1 Calculating the weights of situation assessment indexes based on entropy weight method
In the situation assessment of ADNs, each index has different functions and impacts on the assessment results, so it is necessary to assign corresponding weights to different indexes. The index weight reflects the importance in the index systems, and a reasonable weight distribution is the basis for accurately assessing the operating situation of the ADNs. The entropy weight method needs to calculate only once, which can help to obtain the suitable index weight to each evaluation object, so that the calculation of the weights are no longer complexity, and that is a most widely used objective weight method. To sum up, this paper adopts this method to obtain the weight of each index.
Suppose that there are m indexes, and n samples,
The original data assessment matrix can be represented as follows:
The process of calculating the weights of situation assessment indexes based on entropy weight method is as follows.
(1) Standardizing the index values. The index system proposed in this paper includes positive indexes and negative indexes. Among them, the positive index has property that the larger the index value is, the better the index will be. However, the negative index has property that the smaller the index value is, the better the index will be. In the ADNs safety index system, we should consider the impact of cyberattacks. Therefore, the voltage qualification rate and the power supply capacity margin should be included in the positive index system. Similarly, the voltage violation severity, the load factor, the output volatility of DGs, the permeability of DGs, the power supply capacity mutation severity and the load mutation severity should be included in the negative index system. The range transformation method is used to standardize the original calculated values of each index in the safety index system of ADNs considering cyberattacks. If the kth index is positive index, it can be calculated as follows:
If the kth index is negative index, it can be calculated as follows:
(2) Calculating the entropy of each index. The entropy of each index can be calculated as follows:
It shows, when
(3) Calculating the weight of each index. The weight of each index can be calculated as follows:
3.2 Situation assessment method based on grey correlation
Grey correlation analysis method is an important part of grey system theory. The essence of grey correlation analysis method is to judge the correlation degree between the reference sequence curve and the research sequence curve according to their similarity degree. Compared with the method of mathematical statistics in system analysis, this method does not require a large number of sample data and it also does not satisfies the rule of typical probability distribution either. Meanwhile, this method has uncomplicated calculation processing, and the calculation results are consistent with the results of qualitative analysis, so as to this method is widely used.
However, there are some limitations in the grey correlation analysis method, such as its need to select reference sequence, that is, to determine the optimal value of each index, which is too subjective. At the same time, it is difficult to determine the optimal value of part of indexes. In the processing of situation assessment of ADNs, the data in the normal operation status can be taken as a reference sequence, and this reference sequence without any strong subjectivity. So it is best choices to apply in situation assessment of ADNs.
The main steps and methods are as follows.
(1) Selecting the reference sequence. It is necessary to draft the reference sequence before doing grey correlation analysis, and reference sequence should be an ideal reference standard. We use the data sample when the ADNs is not suffering from attacks as the reference sequence. Suppose that there are m indexes and n samples, according to Eq. 9, the reference sequence can be represented as follows:
(2) Calculating the difference sequences and determine the maximum and minimum values of the difference sequence. Calculate the absolute difference between each element of the original data sequences and the reference sequences, which can be used to form the difference sequence, it can be calculated as follows:
(3) Calculating the correlation coefficient according to the maximum value
In Eq. 17,
(4) Calculating the correlation degree. The correlation degree can be calculated as follows:
In the above Equation, the value of the weight
3.3 Grading the risk level of ADNs situations
According to the situation assessment method of the ADNs, the situation security risk degree of the ADNs can be determined by the correlation degree. It means if the security risk degree is high, the correlation degree will be low. Therefore, according to the numerical range of the correlation degree, the security risk level can be graded into 6 levels, namely 0, 1, 2, 3, 4 and 5. The numerical range of the correlation degree of each security risk level is shown in Table 1.
4 Case study
In this section, we select the IEEE 33-node standard distribution system as a basis case, and connect DGs to it to form an ADN case for study. As shown in Figure 2, we take photovoltaic power (PV) as an example of renewable energy DGs, and connect PVs to the node 18 and node 22. In addition, micro gas turbine units are connected to the node 33.
Firstly, 12 attack scenarios are presented based on the possible cyberattacks on the ADN. Then, the security risk level of the ADN under each attack scenario is evaluated according the proposed method. Where, the operation parameters of the ADN for the calculation are obtained through simulations. Finally, we theoretically analyze the security risk of the ADN under the comparing attack scenarios to illustrate the rationality of the assessment results.
All the experiments are programmed on toolbox Matpower, and all the simulations run on a Dell PC with a 3.3 GHz CPU and 16 GB ram.
4.1 Attack scenarios
Based on the possible cyberattacks on the ADN, we envisage 12 attack scenarios from three categories: attacks on DGs, attacks on controllable loads and attacks on both of them, which can be shown in Table 2. In addition, we take the ADN operation status in normal as the reference scenario and set it as scenario 0.
4.2 Situation assessment under attack scenarios
In this part, we firstly obtain the operation parameters of the ADN under each scenario through simulations, and then calculate the indexes according to Eqs 1–8.
Taking scenario 1 as an example. The operation parameters obtained by simulation are shown in Table 3. It should be noted that the operating parameters need to be obtained from the measurement system in practice.
Then, according to Eqs 1–8, the situation assessment indexes are calculated as shown in Table 4.
Similarly, we can calculate the situation assessment indexes of other scenarios, and the final calculation results are shown in Table 5.
The power supply capacity margin and voltage qualified rate can be standardized by Eq. 11, and voltage violation severity, load rate, output volatility of DGs, penetration rate of DGs, mutation severity of power supply capacity and mutation severity of load can be standardized by Eq. 12. The situation assessment indexes after standardization are shown in Table 6.
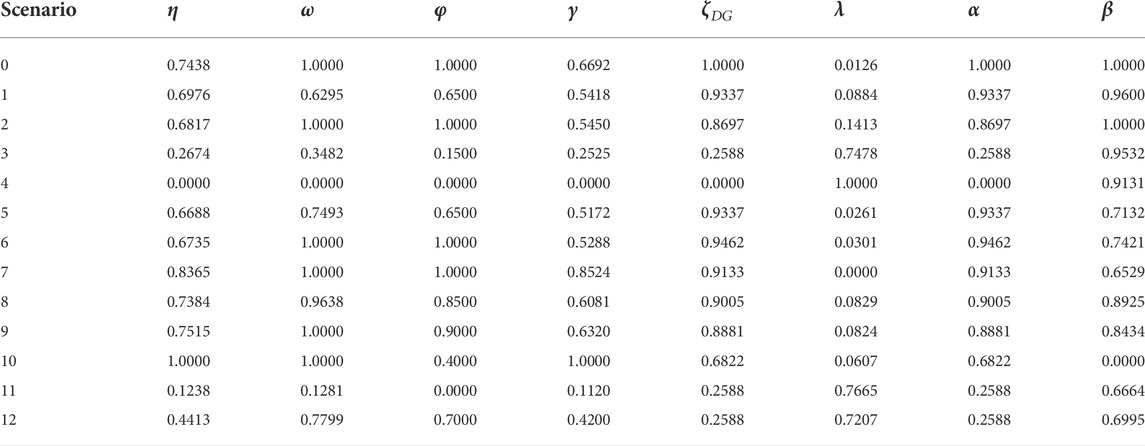
TABLE 6. Standardized situation assessment indexes of the ADN under different cyberattack scenarios.
The weight of different situation assessment index in situation assessment can be calculated by Eqs 13–14, and the calculation results are shown in Table 7.
Taking scenario 0 as the reference scenario, and calculate the correlation coefficient of each situation assessment index between the reference scenario and the different attack scenarios according to the Eqs 15-17. The calculation results are shown in Table 8.
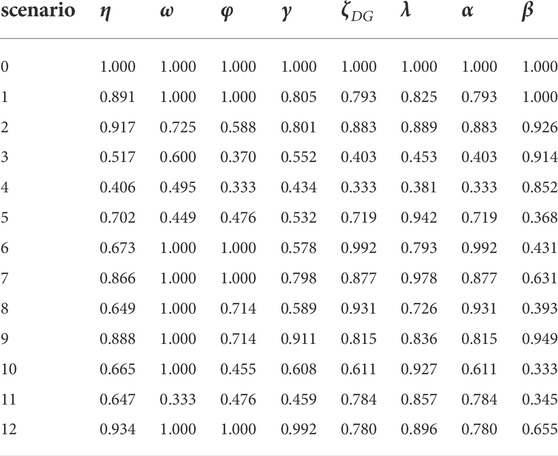
TABLE 8. Correlation coefficient of each situation assessment index under different cyberattack scenarios.
The correlation degree of each scenario with references scenario 0 is calculated by Eq. 18, and the calculation results are shown in Table 9.
Based on the security risk level graded in Table 1, the correlation degree calculation under each attack scenario is determined in the interval, and the final security risk level of the ADNs model under different attack scenarios is obtained, which can be shown in Table 10.
4.3 Analysis
In this part, we illustrate the rationality of the assessment results shown in Table 9 and Table 10 through theoretically analyzing the security risk of the ADN under the comparing attack scenarios.
(1) Comparing Scenario 1 with Scenario 2. In those two scenarios, the types and the total output power of the removed DGs in scenario 1 is consistency with scenario 2. But in scenario 1, the distance of the removed DGs from the main power supply of the distribution network is farther than in Scenario 2, and the result of calculating security risk higher than scenario 2. The reason is that the removed DGs far away from the main power source, and it have the heavier task of the local ADN power balancing, so as to the security risk level of scenario 1 is higher than scenario 2 while the ADN suffered cyberattacks.
(2) Comparing scenario 1 with scenario 3. The total output power of the removed DGs in scenario 1 is consistency with scenario 3, and the distance of the removed DGs in scenario 1 is farther from the main power supply than scenario 3, which lead to the security risk level of scenario 3 is higher than scenario 1, and the security risk of scenario 3 is level 4. The micro gas turbine serving as PV node in scenario 3 outputs more reactive power than the PV power supply serving as PQ node in scenario 1. Therefore, it has heavier task of balancing the reactive power and maintains voltage level in ADN, the security risk level of scenario 3 is higher after suffering attacked. So as to the security risk of scenario 3 is higher than scenario 1 after the ADN suffered cyberattacks.
(3) Comparing scenario 1, scenario 2, scenario 3 and scenario 4. Removing all of the DGs lead to the security risk of the ADN is level 5 in scenario 4. The reason is that the more DGs are removed, the more power will be loss, and the total of demand of power on the power grid become very high. At the meantime, the capacity of the power grid to maintain the voltage balance have decreased and results the voltage fluctuations.
(4) Comparing scenario 5 with scenario 6. During the normal operation of the ADN, the voltage of nodes 12, 13, 14 and 15 are lower than reference value, but the voltage of nodes 18, 19, 20 and 21 are close to the reference voltage value. While increasing the same loads, the security risk of increasing loads of the nodes with low voltage value is higher than increasing loads of the nodes with close to the reference voltage value. The low voltage nodes are more likely to exceed the voltage limit and become the voltage unqualified nodes. While nodes with voltage value are close to the baseline voltage value, it have greater margin and not likely to exceed the voltage limit, so as to the security risk of scenario 5 is higher than scenario 6.
(5) Comparing scenario 5 with scenario 7. Simultaneous increasing loads lead to the higher security risk than simultaneous removing loads. The increasing of loads aggravate the distribution network burden of the power supply and reducing the node voltage. When the loads are removed, the load rate of the main transformers are reduced after the fluctuation become stabilization, the power supply pressure also alleviated, the nearby node voltage value is closer to the reference value. It is more beneficial to the stable operation of the distribution network, and not easy to cause security risks, so as to the security risk of scenario 5 is higher than scenario 7.
(6) Comparing scenario 8, scenario 9 and scenario 10. When the cut off line contains little load, the security risk decreases slightly with increasing of the cut off line loads. The security risk level increased as the load contained by the cut line increasing substantially. When the cut off line contains a small amount of loads, and the length of lines are slightly longer (the amount of load contained is slightly increasing), which are benefit for alleviating the power demand pressure of the power supply. Making the power supply for other node loads are more stable, and the voltage is closer to the reference voltage value. Therefore, it can be explained that why the security risk of scenario 9 is slightly lower than that of scenario 8 (see Table 9). With the total of the loads contained in the cut off line are increased substantially, the adverse effects of the long line being cut off and the fluctuations and user losses will exceed the beneficial effects of the load reduction. The security risk level increase accordingly, thus the security risk of scenario 10 is higher than scenario 8 and scenario 9.
(7) Comparing scenario 11 with scenario 12. Removing DGs and aggravating loads value may lead to security risk of the ADN become highest. Removing DGs and shedding loads synchronously may lead to security risk of the ADN become relatively low. After removing the DGs and increasing loads, it may aggravate the burden of power supply. However, shedding a certain amount of loads can alleviate the power supply capacity decline that caused by removing DGs, and make the ADN relatively difficult to have security risks, so the security risk of scenario 12 is lower than scenario 11.
Through the above comparative analysis, it can be seen that the situation assessment results of all attack scenarios are consistent with the theoretical analysis conclusions, which verified the effectiveness and practicability of the situation assessment index system and assessment methods of ADNs that we proposed in this paper.
5 Conclusion
With the rapid development of integrated energy system, the large-scale and high-permeability access of DGs is making the distribution networks develop into ADNs. The increased complexity of ADNs also increases the vulnerabilities for cyberattacks, and the factors of cyberattacks should be considered in situation assessment system. At present, research on the situation awareness of ADNs is relatively preliminary, there are few relevant study considering cyberattacks and the operation rules of ADNs. Therefore, in this paper, the index system and assessment method of situation assessment for ADNs considering cyberattacks are proposed and verified through the IEEE 33-node ADN system. The characteristics of this work are as follows:
(1) The index system includes three parts: safty indexes of ADNs, security indexes of DGs and the security risk indexes of ADNs suffering from cyberattacks.
(2) The assessment method includes three steps. Firstly, the entropy weight method is used to assign weights to each assessment index according to its impact on the assessment results, which avoids the subjectivity of the traditional expert weight method. Then, the normal operation status of ADNs is taken as the reference scenario, and the grey correlation analysis method is used to calculate the correlation degree of the scenario to be evaluated to the reference scenario. Finally, the security risk level of the scenario to be evaluated is assessed based on the pre-established grading standard for ADNs situations.
(3) For case study, 12 attack scenarios are established considering cyberattacks that the DGs and controllable loads in ADNs might suffering, the situation of each attack scenario are assessed using our proposed method, and the rationality of the assessment results is illustrated by the theoretical analysis. By the case study, the effectiveness of the proposed index system and assessment method are verified.
This paper can provide a practical method for the on-line operation situation assessment of ADNs. The assessment results can help the operation and maintenance staff to grasp the real-time operation status of ADNs, and provide a basis for the situation projection and early warning of ADNs. It can also support for off-line research on the projection and early warning method, operation control strategy and network planning of ADNs. The results of the case study can directly provide reference for the study of situation awareness and planning of ADNs.
The future work will conduct the study on situation projection method for ADNs considering cyberattacks to complete the ADNs situation awareness system.
Data availability statement
The original contributions presented in the study are included in the article/supplementary material, further inquiries can be directed to the corresponding author.
Author contributions
JL and JL has done the main theory research work. JL and JL conceived the project and wrote the manuscript. JL, JL, JZ and DQ designed and participated in the experiment. All authors discussed the results, read, and commented on the manuscript.
Funding
This work was supported by the National Natural Science Foundation of China (no. 51977155).
Conflict of interest
The authors declare that the research was conducted in the absence of any commercial or financial relationships that could be construed as a potential conflict of interest.
Publisher’s note
All claims expressed in this article are solely those of the authors and do not necessarily represent those of their affiliated organizations, or those of the publisher, the editors and the reviewers. Any product that may be evaluated in this article, or claim that may be made by its manufacturer, is not guaranteed or endorsed by the publisher.
References
Arya, R. (2016). Determination of customer perceived reliability indices for active distribution systems including omission of tolerable interruption durations employing bootstrapping. IET Generation Transmission &. Distribution 10 (15), 3795–3802. doi:10.1049/iet-gtd.2016.0198
Canizes, J., Soares, J., Vale, Z., and Lobo, C (2017). Optimal Approach for Reliability Assessment in Radial Distribution Networks. IEEE Systems Journal 11 (3), 1846–1856. doi:10.1109/JSYST.2015.2427454
Fauzan, H., Victor, W., and Jaesung, J. (2019). State-of-the-art review on power grid resilience to extreme weather events: Definitions, frameworks, quantitative assessment methodologies, and enhancement strategies. Applied Energy 239, 1049–1065. doi:10.1016/j.apenergy.2019.02.017
Gallo, J., Turan, S., Boem, F., Parisini, T., and Ferrari, T. (2020). A Distributed Cyber-attack Detection Scheme with Application to DC Microgrids. IEEE Trans. Automat. Contr. 65 (9), 3800–3815. doi:10.1109/tac.2020.2982577
Huang, M., Wei, Z., Sun, G., Zang, H., and Huang, Q. (2017). A Novel Situation Awareness Approach Based on Historical Data-Mining Model in Distribution Network. Power Syst. Tech. 41 (4), 1139–1145. doi:10.13335/j.1000-3673.pst.2016.2813
Ismail, M., Shaaban, F., Naidu, M., and Serpedin, E. (2020). Deep Learning Detection of Electricity Theft Cyber-attacks in Renewable Distributed Generation. IEEE Trans. Smart Grid 11 (4), 3428–3437. doi:10.1109/TSG.2020.2973681
Jena, M., Panigrahi, B., and Samantaray, S. (2017). A New Approach to Power System Disturbance Assessment Using Wide-Area Postdisturbance Records. IEEE Trans. Ind. Inf. 14 (3), 1253–1261. doi:10.1109/TII.2017.2772081
Kurt, M., Yilmaz, Y., and Wang, X. (2018). Distributed Quickest Detection of Cyberattacks in Smart Grid. IEEE Trans. Inform. Forensic. Secur. 13 (8), 2015–2030. doi:10.1109/TIFS.2018.2800908
Lai, J., Lu, X., Dong, Z., and Cheng, S. (2022). Resilient Distributed Multiagent Control for AC Microgrid Networks Subject to Disturbances. IEEE Trans. Syst. Man Cybern. Syst. 52 (1), 43–53. doi:10.1109/TSMC.2021.3056559
Lai, J., Lu, X., Yu, X., and Monti, A. (2020). Stochastic Distributed Secondary Control for AC Microgrids via Event-Triggered Communication. IEEE Trans. Smart Grid 11 (4), 2746–2759. doi:10.1109/TSG.2020.2966691
Li, H., Liu, Z., and Song, Z. (2015). Real-time Static Security Situational Awareness of Power Systems Based on Relevance Vector Machine. Proceedings of the CSEE 35 (2), 294–301. doi:10.13334/j.0258-8013.pcsee.2015.02.005
Li, Q., Tang, H., Liu, Z., Li, J., Xu, X., Sun, W., et al. (2021). Optimal Resource Allocation of 5G Machine-Type Communications for Situation Awareness in Active Distribution Networks. IEEE Syst. J. 10 (6), 1–11. doi:10.1109/JSYST.2021.3110502
Li, Y., Gao, W., Gao, W., Zhang, H., and Zhou, J. (2020). A Distributed Double-Newton Descent Algorithm for Cooperative Energy Management of Multiple Energy Bodies in Energy Internet. IEEE Trans. Ind. Inf. 17 (9), 5993–6003. doi:10.1109/TII.2020.3029974
Li, Y., Zhang, H., and LiangHuang, X, B. (2019). Event-triggered Based Distributed Cooperative Energy Management for Multienergy Systems. IEEE Trans. Ind. Inf. 15 (14), 2008–2022. doi:10.1109/TII.2018.2862436
Liang, J., Wu, Y., Li, J., Chen, X., Tong, H., Ni, M., et al. (2021). Security Risk Analysis of Active Distribution Networks with Large-Scale Controllable Loads under Malicious Attacks. Complexity 2021, 1–12. doi:10.1155/2021/6659879
Lin, J., Wan, C., Song, Y., Huang, R., Chen, X., and Guo, W. (2016). Situation Awareness of Active Distribution Network: Roadmap, Technologies, and Bottlenecks. CSEE Jour. Power & Ener. Syst. 2 (3), 35–42. doi:10.17775/CSEEJPES.2016.00033
Lin, Z., Wen, F., Ding, Y., Xue, Y., Liu, S., Zhao, Y., et al. (2018). WAMS-based Coherency Detection for Situational Awareness in Power Systems with Renewables. IEEE Trans. Power Syst. 33 (5), 5410–5426. doi:10.1109/TPWRS.2018.2820066
Liu, W., Zhang, D., Wang, X., Hou, J., and Liu, L. (2018). A Decision Making Strategy for Generating Unit Tripping under Emergency Circumstances Based on Deep Reinforcement Learning. Proceedings of the CSEE 38 (1), 109–119. doi:10.13334/j.0258-8013
McLaughlin, S., Konstantinou, C., Wang, X., Davi, L., Sadeghi, A., Maniatakos, M., et al. (2016). The Cybersecurity Landscape in Industrial Control Systems. Proc. IEEE 104 (5), 1039–1057. doi:10.1109/JPROC.2015.2512235
Ren, C., Xu, Y., Zhang, Y., and Zhang, Rui. (2019). A Hybrid Randomized Learning System for Temporal Adaptive Voltage Stability Assessment of Power Systems. IEEE Trans. Ind. Inf. 16 (6), 3672–3684. doi:10.1109/TII.2019.2940098
Russell, L., Goubran, R., Kwamena, F., and Knoefel, F. (2018). Agile IoT for Critical Infrastructure Resilience: Cross-Modal Sensing as Part of a Situational Awareness Approach. IEEE Internet Things J. 5 (6), 4454–4465. doi:10.1109/JIOT.2018.2818113
Shahsavari, A., Farajollahi, M., Stewart, E., Cortez, E., and Mohsenian, H. (2019). Situational Awareness in Distribution Grid Using Micro-PMU Data: A Machine Learning Approach. IEEE Trans. Smart Grid 10 (6), 6167–6177. doi:10.1109/TSG.2019.2898676
Sultan, K., Hatem, Z., and Vinod, K. (2013). Planning Active Distribution Networks Considering Multi-DG Configurations. IEEE Trans. Power Syst 29 (2), 785–793. doi:10.1109/PESGM.2014.6939178
Tao, X., Kong, K., Zhao, F., Cheng, S., and Wang, S. (2020). An Efficient Method for Network Security Situation Assessment. Int. J. Distrib. Sens. Netw. 16 (11), 155014772097151. doi:10.1177/1550147720971517
Wang, H., Shi, L., and Ni, Y. (2017b). Distribution system planning incorporating distributed generation and cyber system vulnerability. J. Eng. (Stevenage). 3, 2198–2202. doi:10.1049/joe.2017.0720
Wang, P., and Govindarasu, M. (2020). Multi-agent based attack-resilient system integrity protection for smart grid. IEEE Trans. Smart Grid 11 (4), 3447–3456. doi:10.1109/TSG.2020.2970755
Wang, Q., Bu, S., He, Z., and Dong, Z. (2020). Toward the prediction level of situation awareness for electric power systems using CNN-LSTM network. IEEE Trans. Ind. Inf. 17 (10), 6951–6961. doi:10.1109/TII.2020.3047607
Wang, S., Dehghanian, P., and Li, L. (2019). Power grid online surveillance through PMU-embedded convolutional neural networks. IEEE Trans. Ind. Appl. 56 (2), 1146–1155. doi:10.1109/TIA.2019.2958786
Wang, X., Chen, N., Li, Y., Wang, C., and Pu, T. (2017a). Multi-source optimal dispatch architecture for active distribution network based on situational linkage power. Power Syst. Tech. 41 (2), 349–354. doi:10.13335/j.1000-3673.pst.2016.2913
Wei, F., Wan, Z., and He, H. (2020). Cyberattack recovery strategy for smart grid based on deep reinforcement learning. IEEE Trans. Smart Grid 11 (3), 2476–2486. doi:10.1109/TSG.2019.2956161
Keywords: integrated energy systems, active distribution network, situation assessment, cyberattack, index system, assessment method
Citation: Li J, Liang J, Liu Q, Qi D, Zhang J and Chen Y (2022) Research on situation assessment of active distribution networks considering cyberattacks. Front. Energy Res. 10:971725. doi: 10.3389/fenrg.2022.971725
Received: 17 June 2022; Accepted: 12 July 2022;
Published: 08 August 2022.
Edited by:
Qihe Shan, Dalian Maritime University, ChinaReviewed by:
Guangzheng Yu, Shanghai University of Electric Power, ChinaPing Hu, EPFL, Switzerland
Peiyin Chen, Tianjin University, China
Copyright © 2022 Li, Liang, Liu, Qi, Zhang and Chen. This is an open-access article distributed under the terms of the Creative Commons Attribution License (CC BY). The use, distribution or reproduction in other forums is permitted, provided the original author(s) and the copyright owner(s) are credited and that the original publication in this journal is cited, in accordance with accepted academic practice. No use, distribution or reproduction is permitted which does not comply with these terms.
*Correspondence: Jun’e Li, jeli@whu.edu.cn